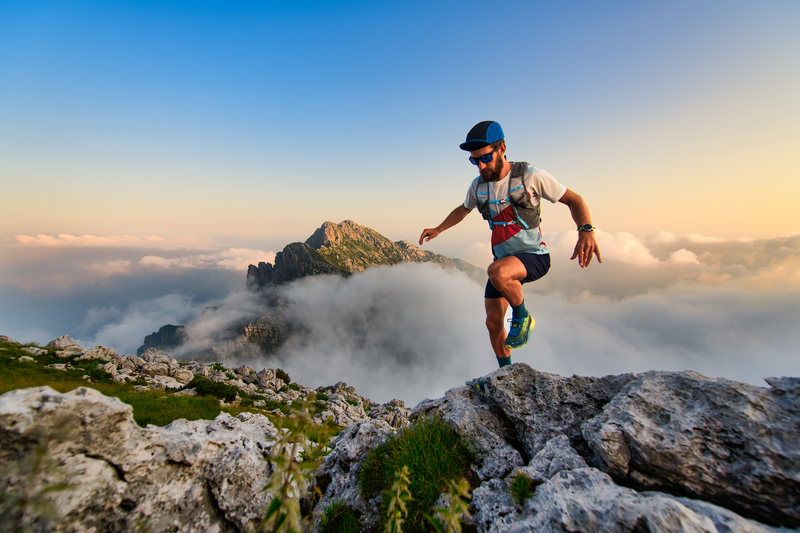
94% of researchers rate our articles as excellent or good
Learn more about the work of our research integrity team to safeguard the quality of each article we publish.
Find out more
REVIEW article
Front. Phys. , 21 June 2021
Sec. Biophysics
Volume 9 - 2021 | https://doi.org/10.3389/fphy.2021.666192
This article is part of the Research Topic Viscoelasticity: From Individual Cell Behavior to Collective Tissue Remodeling View all 11 articles
Changes in biomechanical properties of biological soft tissues are often associated with physiological dysfunctions. Since biological soft tissues are hydrated, viscoelasticity is likely suitable to represent its solid-like behavior using elasticity and fluid-like behavior using viscosity. Shear wave elastography is a non-invasive imaging technology invented for clinical applications that has shown promise to characterize various tissue viscoelasticity. It is based on measuring and analyzing velocities and attenuations of propagated shear waves. In this review, principles and technical developments of shear wave elastography for viscoelasticity characterization from organ to cellular levels are presented, and different imaging modalities used to track shear wave propagation are described. At a macroscopic scale, techniques for inducing shear waves using an external mechanical vibration, an acoustic radiation pressure or a Lorentz force are reviewed along with imaging approaches proposed to track shear wave propagation, namely ultrasound, magnetic resonance, optical, and photoacoustic means. Then, approaches for theoretical modeling and tracking of shear waves are detailed. Following it, some examples of applications to characterize the viscoelasticity of various organs are given. At a microscopic scale, a novel cellular shear wave elastography method using an external vibration and optical microscopy is illustrated. Finally, current limitations and future directions in shear wave elastography are presented.
Changes in mechanical properties of biological soft tissues are often associated with physiological dysfunctions. Viscoelasticity is an important mechanical biomarker to characterize structural changes and/or constituents of tissues. However, the assessment of elasticity through imaging has been more often exploited than viscosity, and an historical perspective of development has been to replace manual palpation by physicians and to answer an ultimate and natural question: ‘is the region hard or soft?’ The elasticity, represented by the Young’s modulus
Since biological soft tissues are hydrated, they are not only represented by their solid-like behavior using elasticity, but also by their fluid-like behavior using viscosity. Viscosity represents the hysteretic effect between stress and strain applied on a tissue. It is becoming an important biomarker of pathological changes in biological tissues. Mechanical test is the most basic method to measure the viscosity of ex vivo soft tissues. To this end, a constant strain is applied to a test specimen. The stress relaxation with time is used to characterize the viscosity of the tissue. Another way of mechanical test is dynamic mechanical analysis. Periodic strain or stress is imposed on a specimen. The dynamic responses in terms of different incentive frequencies are associated with tissue viscoelasticity. Relevant works on mechanical testing of biological soft tissues can be found in [2–9]. Alternatively, imaging-based approaches are becoming more popular for in vivo viscoelasticity assessment. These approaches exploit tissue deformations in acoustic, magnetic or optical fields to characterize viscoelasticity in a non-invasively manner. Soft tissues can maintain their function during the measurement avoiding destructive testing [10]. Based on a clinical perspective, the in situ and localized assessment of tissue viscoelasticity through imaging had major impacts on diagnosis (e.g., cancers, liver fibrosis, musculoskeletal disorders, cardiovascular diseases, etc…). Since biological tissues consist of cells, extracellular matrices, and structural proteins, a recent field of development has been to study sub-cellular biomechanical properties associated with pathological processes through imaging. This finding encouraged researchers to impel bio-elasticity research further into a microscopic scale.
This review aims to provide a state-of-the-art summary of developments made in the field of shear wave elastography, which concerns elasticity and viscosity imaging through mechanical shear wave analysis. This technology requires a shear wave source, the tracking of shear wave propagation through imaging, and the processing of the shear wave propagation characteristics through physical models or image processing algorithms. Shear waves can be generated by external or internal vibrating sources. An external mechanical actuator in physical contact with an organ or cell is a common way to induce shear wave propagation from the surface to the core, whereas acoustic radiation or Lorentz forces can be used as internal in situ localized shear wave sources. The detection of the shear wave propagation is usually performed using ultrasound (US), magnetic resonance (MR), optical or photoacoustic imaging methods. Elasticity and viscosity can be obtained from estimations of shear storage and loss moduli, which require the determination of the shear wave velocity and attenuation into the interrogated medium. In the following sections, the generation and tracking of shear waves are described. Determining elasticity and viscosity maps through the solution of an inverse problem based on elastic wave propagation equations and underlying assumptions are also addressed throughout the text. Note that this review is not intended to detail artifacts and confounders of shear wave imaging because these are organ, tissue structure, and tissue pathology specific. Nevertheless, such information is presented briefly in some sections.
The major approach since the early steps of elastography imaging has been to approximate the tissue as isotropic and purely elastic. This situation has been widely described so the main constitutive relations essential to shear wave elastography (SWE) understanding are recalled here. The relationship between the applied stress and the strain response of the solicited tissue is given by Hook’s law:
where T and S are the stress and strain tensors, respectively, and
where u is the 3-dimensional motion field in unit of (m) induced by stressing the tissue. The time domain wave equation describing the propagation of local displacements is obtained using Newton’s second law:
where ρ is the material’s density in [kg/m3] and f [Nm−3] is the source term. After Fourier transform into the frequency domain, Eqs 1–3 are expressed as:
where ω = 2πf with f the frequency, and the tilde (∼) and star (*) notations refer to complex numbers. Full development of Eq. 3 (available in Ref. [11]) leads to the governing equation of motion propagation in elastic solids known as the Navier’s equation:
where μ is the shear modulus in (Pa) reflecting the amount of energy the tissue can store as elastic deformation, and λ is the first Lamé coefficient in (Pa) reflecting the tissue’s compressibility. The Navier’s equation does not rely on rheological models and conveys an approximation of the material’s natural properties based on physical assumptions. The same development applied to Eq. 6 leads to:
where
and
The motion field u propagates as compression and shear waves of which velocities vc and vs are, respectively, given by (see Ref. [11] for details):
and
The large difference between the two velocities observed in biological tissues (typically vc is around 1,540 ms−1 and vs is found around 1–10 ms−1) suggests that λ is much greater than μ, thus allowing for the following approximation often used to report SWE measurements:
This observation is directly linked to the tissue incompressibility assumption, which causes λ to approach infinity [12]. Additionally, λ was shown to vary slightly as opposed to μ, which spans a few orders of magnitude in biological tissues [13]. Consequently, the shear modulus or the Young’s modulus is the mechanical parameter considered in elastography reconstruction processes.
Another approach much less often used to reconstruct material mechanical properties in SWE is to model the solid as isotropic and viscoelastic instead of purely elastic. Here, the response of the loaded tissue shows a delay with respect to actuation and elastic waves attenuate due to energy dissipation in the solid, which is specific to viscous materials. Attenuation may be accounted for in the time-domain Navier’s equation by introducing a damping term, and linking it to viscosity using rheological models such as the generalized Maxwell, standard linear solid, or Kelvin-Voigt, which are further discussed in the next section. The following Table 1 presents the stress-strain relationships used to derive wave equations from Newton’s second law for the three aforementioned rheological models [14].
TABLE 1. Stress-strain relationships for three common rheological models accounting for the viscoelastic behavior of soft tissues. Details about these models are reported in the next section. T, S, c and η are the stress, strain, elasticity and viscosity tensors respectively, E, E1, and E2 are Young’s moduli specific to the Maxwell and standard linear solid models.
A different option to integrate viscoelastic properties into the description of the material is to formulate the problem in the frequency domain, provided a harmonic actuation. In this case, the complex shear modulus, as described in Eq. 8, has a non-zero imaginary part accounting for shear wave dissipation due to the material’s shear viscosity. Relating G″ values to actual viscosity values depends on rheological modeling, as discussed in the next section. For instance, in the case of a solid described by the Kelvin-Voigt model, the complex shear modulus is given by
For G′ and G″ estimation, the displacement field
To date, isotropic elastic and viscoelastic characterization of soft matters have mostly been considered in shear wave elastography, owing to the availability of various inversion schemes. However, the anisotropic and poroelastic nature of certain biological tissues, such as the brain, has long been acknowledged. In poroelasticity, the medium is modeled as a porous solid matrix crossed by flowing fluid, and thus contains two separate phases, as opposed to more common models containing one phase. Consequently, the motion field measured in imaging protocols is not only due to the solid tissue deformation but also to the pressure gradient in fluid pores. Although poroelasticity was early studied using quasi-static deformations [15], implementation in shear wave elastography imaging remains in its infancy. Oscillatory deformations in poroelasticity have first been described by Biot [16, 17], and later by [18]. Assuming a viscous fluid flow, fluid saturation in pores, and a compressible linearly elastic solid, poroelasticity equations of propagation are given by:
where µ is the shear modulus, λ the first Lamé’s parameter, u the complex time harmonic displacement field, β the effective stress coefficient (dimensionless), p the complex time harmonic pressure field, ω the actuation frequency, ρ the bulk density, ρf the pore fluid density, ϕp the material porosity, κ the hydraulic conductivity, and ρa the apparent mass density.
Finally, anisotropy has been considered for biomechanical modeling in the context of shear wave elastography. In such cases, the material’s mechanical response (strain) is dependent on the direction in which it is solicited (stress). Full derivation of relevant mechanical parameters under different symmetry assumptions is beyond the scope of this review, and the interested reader is referred to the excellent pedagogical development in [19]. Briefly, Hooke’s law describes the relationship between applied stress and material strain:
where σ and ϵ are the stress and strain tensors, respectively. In isotropic materials, the tensor Cijkl is fully described by two parameters, E and ν. In anisotropic materials, more constants are needed to account for the direction dependance of ϵ. The most used anisotropic model is transverse isotropy, which is particularly used to characterize fibrous tissues (e.g., muscles). Transversely isotropic materials are organized in layers where in-plane mechanical properties are isotropic, and out-of-plane ones are anisotropic. In such cases, 5 parameters are necessary to describe Cijkl. Two of them, μ13 and μ12, characterize the shear motion along and perpendicular to the fiber axis, respectively. The other three, E1, E2, and E3 characterize the compression motion along, perpendicular in-plane, and perpendicular out-of-plane to the fiber axis, respectively. Injection of the Hooke’s law into Newton’s second equation allows to derive equations of shear wave propagation along directions of dependency the same way as to derive the Navier’s equation of elasticity. Other anisotropic models exist, such as the orthotropic one which shows a lower level of symmetry (three perpendicular planes) than the transverse isotropy model. The orthotropic model has been used to develop waveguide elastography, which describes the propagation of the different polarizations of shear waves along separate directions. The orthotropic tensor along with equations of polarized wave propagation are described in detail in [20].
Most soft bio-tissues contain more than 70-w% of water, thus they can be considered as fluid-like solids, which means these materials have characteristics of both solids and fluids [1]. Elasticity refers to the solid property that describes the ability of a material to return to its original shape after a stress is removed [21]. The fluid property is given by the viscosity (η) that describes the ability of a material to resist to its deformation due to a tensile stress or shear stress [21]. Three categories of properties are often used to characterize the viscoelasticity of a soft material: its compressibility, which is usually measured by the bulk modulus (K) and the Poisson’s ratio (ν); its tension, which mainly refers to the Young’s modulus (E); and shear properties, described by the second Lamé coefficient (µ) and the complex shear modulus (G*).
The complex shear modulus (see Eq. 8 and accompanied description) is self-sufficient to describe the viscoelasticity of biological tissues. In general, the storage modulus G′ reflects the shear elastic property while the loss modulus G″ reflects the viscous response of the material. Alternatively, rheological models were considered to relate experimental measurements to elastic and viscous properties of tissues. Mathematically, the Kelvin-Voigt and Maxwell models have been considered most frequently to describe viscoelastic tissues [22–24], i.e., quantifying the shear elasticity and viscosity. The two models are represented by a purely elastic spring connected to a purely viscous dashpot in parallel (Kelvin-Voigt) or in series (Maxwell), respectively. A material with only elasticity is called a purely elastic material (only a spring), while a material with only viscosity (only a dashpot) is called a Newtonian fluid [25]. A soft tissue or soft tissue-like material falls between these two extreme conditions and can be called as a viscoelastic material [26]. Other material models are used less frequently, while most of them are constructed with different combinations of single/multiple spring(s) and dashpot(s) in more complicated arrangements, such as the Zener model, generalized Maxwell model, and generalized Kelvin-Voigt model [27–29].
Note that in the field of SWE, the complex shear modulus G* may be sometimes confused with the storage modulus G′ and the second Lamé coefficient µ (also known as the shear modulus). Although the storage modulus reflects the tissue elasticity, they are not rigorously the same. For sake of clarity, in this review, the elasticity is denoted by |µ|, i.e. the real component of the second Lamé coefficient. Therefore, for incompressible soft tissues (i.e., for a Poisson ratio close to 0.5) with a negligible viscous component, it can be assumed that such a tissue is a purely elastic material so that G* solely represents the real shear elasticity and so G* = G′ = |µ| [30, 31]. Otherwise, the calculation of elasticity (as well as the viscosity) is rheological model dependent.
Mathematical relations between tissue excitation and response [32] may be divided into two groups according to the temporal difference of the excitation [30, 33]: namely quasi-static measurements and dynamic measurements [also termed shear wave (SW) measurements]. The quasi-static measurement methods mainly analyze the stress-strain behavior. On the other hand, SW measurement methods determine the complex shear modulus by tracing and analyzing a propagated SW in the specimen.
During the past years, studies in the field of SWE measurements were often targeting absolute values of elasticity |µ| and viscosity η. To do so, rheological models of the material are needed to derive those parameters. For instance, when the Kelvin-Voigt model or Maxwell model is considered, the complex shear modulus can be written as follows [28, 34, 35]:
where GKV represents G* of the Kelvin-Voigt model, and GM is that for the Maxwell model, both satisfy
Here, as synergized with Eq. 18, one can see that both |µ| and η are functions of vS, αS, and ωS. Since vS and αS can be experimentally measured at certain ωS, |µ| and η, in a specified rheological model, can be thereafter calculated.
In common practice, when the viscosity η is taken into account for tissue characterization, it can be determined either directly using both vS and αS with knowing the corresponding ωS, or alternatively by evaluating the dispersion of vS with respect to ωS without determining the value of αS, i.e., by knowing multiple pairs of vS and ωS [27, 36, 37]. However, one should also notice that the viscoelastic property of biological tissues are rather complex, depend on the tissue type, and on the presence of a pathological condition, so that there is not simply a best, or a most appropriate material model for all tissues. Furthermore, pathological changes with time of a tissue could also lead to a major change of its viscoelastic property, thus a certain material model may no longer be suitable for the tissue when it becomes abnormal and progresses toward a more severe pathological state. Meanwhile, the derivation of elasticity |µ|, and viscosity η, are rather different among different material models. That means, using different models with same measures (such as vS and αS) would lead to different results [28], and hence would be meaningless to clinical studies. Therefore, nowadays it is always suggested that no rheological model is assumed, and instead of that, directly access the shear storage G′ and loss G″ moduli would be not only more rigorous and appropriate, but also mathematically convenient to describe tissue viscoelastic properties [28, 37].
A shear wave, also called a transverse wave, is a moving mechanical wave that consists of particle oscillations occurring perpendicular to the direction of the energy transfer [38]. As briefly introduced, SWs in ultrasound imaging can be generated either from an external vibration source (such as a mechanical vibrator/shaker) [39–42], or internally by an acoustic radiation force (ARF) [13, 44–51], as illustrated in Figure 1. The control of the SW amplitude and frequency of the ARF is considered in [13]. In terms of waveforms, the SW can also be generated as continuous waves [39, 40, 42, 44–46] or impulse waves [41, 47–51], as can be seen in the examples of Figure 2. In ultrasound imaging, the probe fires longitudinal pressures and detects particle displacements along the axial direction, therefore only SWs that propagate along the lateral direction of the ultrasound beam, or SW components whose displacements occurred on the axial direction, can be detected.
FIGURE 1. Shear wave generation sources: (A) an external vibration source, and (B) am internal acoustic radiation force.
FIGURE 2. Examples of the generation of (A) continuous shear waves, adapted from [40] copyright 1990 IEEE, and (B) impulse shear waves, reproduced with permission from [47] copyright 2004 IEEE.
Many remarkable techniques were invented over the past 30 years based upon different combinations of external or internal SW sources, and continuous or impulse SWs. In 1988, Lerner et al. proposed a method to map the propagation of low frequency SWs with a Doppler ultrasound displacement detection technique to assess tissue stiffness [39]. Later in 1990, Yamakoshi et al. proposed a dynamic measurement method to determine the SW speed vS using an external mechanical vibration source [40], as seen in Figure 2A. The parameter vS was determined by analyzing the wavelength of a continuously propagated SW using the Doppler ultrasound technique. Catheline et al. developed in 1999 an impulse SW measurement method [41]. In this method, an ultrasonic probe was located at one side of the specimen to capture the propagation of the impulse SW generated by a mechanical vibrator located at the other side of the specimen. A plane wave ultrasound system was used, enabling a high frame rate in detection mode, then the parameter vS was determined through the time-of-flight (TOF) technique applied on successive images. See below for more information on the TOF method. Since such a method used an impulse SW, it is also called transient SW imaging, or transient elastography (TE). Nowadays, the largely used clinical device Fibroscan [52, 53] is based on TE. In 2004, a method using two external SW sources to generate continuous SWs toward each other with slightly different SW frequencies was proposed [42]. Due to the frequency difference, interfered SW patterns termed as “crawling waves” moved with a much slower speed than the expected vS, This allowed the observation of propagated crawling waves with a conventional low frame rate B-mode imaging system. Once the speed of the crawling wave is obtained, vS could be derived.
In 1998, Sarvazyan et al. developed a SW measurement method, termed shear wave elasticity imaging (SWEI), by using a SW remotely generated by an ARF of a focused ultrasound beam [13]. In this method, a transient SW pulse was firstly produced at the focus of the ultrasound beam and propagated along sideways. Then, imaging transducers were used to trace the moving of SW fronts and viscoelastic parameters were derived thereafter. The same year, Fatemi and Greenleaf developed a method to produce an oscillatory ARF by mixing two ultrasound beams with different frequencies [44]. A short period of harmonic (continuous) or tone-burst SWs was generated and propagated along sideways, which made the SW narrow-band (while the SW generated by Sarvazyan’s method was broadband) and then vS could be determined by finding the phase difference of the SW at two apart locations [45]. By repeating the measurement with continuous SWs at different frequencies, or retrieving different frequency components of a tone-burst SW, both G′ and η could be derived. This method is termed as SW dispersion ultrasound vibrometry (SDUV). Later in 2004, based on the combination of Catheline’s impulse SW method [41] and the ARF technique [13], Bercoff et al. developed an advanced SW measurement method known as supersonic shear imaging (SSI) [47]. With this method, an ultra-high-speed scanner is used, then multiple ARF impulses are triggered consecutively and very quickly at different depths. Each impulse produces a SW point-like source then all these SWs are interfering constructively and result in two SW planes propagating in opposite directions, as can be seen in Figure 2B. A two-dimensional vS image is obtained with this method. Moreover, since this technique is creating broadband SWs, tissue viscosity can also be estimated through the vS dispersion method (using the same principle as SDUV). In 2012, Song et al. developed a SW method, which also used multiple lateral ARFs as in [48]; the method was termed as comb-push ultrasound shear elastography (CUSE) [49]. It firstly generates multiple ARF excitations at different spatial locations to produce multiple impulse SWs by using the push mode of the ultrasound probe, and then quickly switches to the scanning mode of the probe to detect the SW propagation. Therefore, vS could be measured through the TOF technique by tracing the movement of the SW front from each SW source. The use of the comb-push excitation provided multiple SW sources in the specimen so that such method is effectively compensating for the worse signal-to-noise ratio (SNR) due to the SW attenuation at the location far away from a given SW source.
Knowing that vS = λS × fS, one may like to measure vS through the TOF technique, or instead to determine λS in the spatial domain. For most biological tissues, vS travels at a few m/s, which means it only takes a couple of ms for a SW to travel through the entire field of view (FOV) of a common ultrasound probe. Physically, a focused ultrasound system triggers transducer elements sequentially from one edge to another to complete a B-mode scan, as a result the frame rate is typically less than 100 frames/sec in such a system, which is not fast enough to measure the TOF without a particular modification of the experimental setup [42]. Therefore, measuring λS becomes the realistic option. In this scenario, the spatial resolution is determined and limited by λS. Although increasing fS is reducing λS, and so is improved the resolution, one should also notice that a higher frequency would cause a quick attenuation of the SW propagation. Thus, empirically fS is usually adjusted to a few hundreds of Hz, which leads the lateral resolution of elastography images to a sub-centimeter level with using standard focused ultrasound beamforming. On the other hand, a plane wave system can trigger all transducer elements of the probe at the same time to emit a plane compression wave, enabling it to have a very high frame rate in B-mode (up to 10,000 frames/sec) [47]. Thus, in this scenario, the TOF technique is applicable, and theoretically the distance that a SW travels within two consecutive frames could be as short as a few-tenth of mm. Since the distance is comparable to the physical interval of two adjacent transducer elements in a common ultrasound array probe, the lateral spatial resolution of SWE with using a plane wave system is approximately the same as that of B-mode imaging [54]. It is also worth noting that, when tissue boundaries/layers exist under the FOV, and a propagating SW passes through those interfaces, physical phenomena such as reflection, refraction, diffraction, and mode conversion could occur at the interfaces and cause artifacts. Although directional filters are usually applied to mitigate those effects [55, 56], practically it is still difficult to remove all the unwanted waves. Therefore, the vS measured within approximately one λS from the interfaces are usually considered unreliable, which to some extent would downgrade the spatial resolution at those areas [57].
As introduced earlier, ultrasound SWE contributed to the non-invasive assessment of mechanical properties of soft tissues [58–62]. One method largely used clinically is transient elastography (TE) [63–67], which utilizes a dynamic compression generated by the vibration of the transducer on the skin to produce shear waves. No structural imaging is provided with this method to guide the measure. Moreover, in some patients with morbid obesity and ascites, the attenuation of shear waves travelling from the surface of the body to the organ of interest (typically the liver) may avoid reliable measurements [68, 69]. The acoustic radiation force impulse (ARFI) [60, 70] and supersonic shear imaging (SSI) [47, 71, 72] methods use a radiation pressure to locally induce shear waves within the organ of interest. TE, ARFI and SSI are assessing tissue elasticity (no viscosity) based on the measurement of the shear wave speed [73]. The Young’s modulus is estimated and displayed as an image using
Most studies utilized a rheological model, which has been introduced earlier, e.g., the Kelvin-Voigt model, to find the viscosity after the reconstruction of elasticity [45, 76–78]. The complex shear modulus G* = G′+ jG″ was also estimated using different approaches, such as measuring the acoustic radiation force-induced creep [74], solving the Navier’s wave equation numerically [79], inverting analytically the solution of the shear wave scattering from a mechanical inclusion [80–82], and using a finite-element based method [83]. Note that a torsional SW source was used in the latter method, as originally proposed in [84].
Kazemirad et al. [50] developed a method for the quantitative measurement of viscoelastic parameters G′ and G″ at various frequencies, based on the assumption of a cylindrical shear wave front produced by a radiation pressure, allowing to avoid wave diffraction effects. Other studies have also used the same geometrical assumption for quantitative viscoelastic measurements [75, 85, 86]. Notice that the cylindrical wave front assumption would not necessarily hold when considering inhomogeneous media, such as a tissue embedding a tumor. A recent method for estimating tissue viscosity without geometrical assumption on the wave front was proposed by [51], which utilized the shear wave velocity vS and attenuation αS computed by the frequency shift method [87]. Recently, [88] performed a study to characterize viscoelastic properties of oil-in-gel viscoelastic phantoms and in vivo human livers. They found that the shear wave dispersion and attenuation were linked together and related to the tissue viscosity. As reviewed above, the shear wave speed and attenuation are widely used for reconstructing viscoelastic properties. Experimental methods to obtain those shear wave properties are separately described below.
One of the widely used methods implemented on clinical scanners is the group velocity [89–92] that assumes the tissue as elastic, homogeneous, isotropic, linear, and of infinite dimension with respect to the wavelength. The group velocity is estimated using time-of-flight (TOF)-based algorithms for particle displacement or particle velocity assessments in the time domain [72, 93]. TOF–based algorithms are usually based on cross-correlation (CC) [94] and time-to-peak (TTP) methods [90]. Basically, the CC provides a moving average estimate of the shear wave speed using all sample points, and performs multiple cross-correlations along the direction of the wave propagation, which may result in artifacts for periodic shear wave patterns, whereas TTP estimates the velocity based on the tracking of the movement of one point on the waveform [90]. Although group velocity estimation methods are considered robust [91], they are theoretically applicable to strictly elastic materials thus requiring resorting to other techniques for the evaluation of the viscous behavior [95].
The variation of the shear wave velocity with frequency refers to the wave dispersion happening in a viscoelastic medium [45]. Some methods have used this phenomenon to evaluate viscoelastic properties of tissues [45, 96]. Measuring shear wave velocities at specific frequencies is known as phase velocity estimation [45]. Beside the viscoelastic property of a tissue, its finite thickness can also affect the dispersion due to reflections during propagation, which may result in wave mode conversion [97–99]. The phase velocity and the group velocity are not equal in the presence of dispersion. It was shown that the phase velocity has a lower value by a factor of 8–9% compared with the group velocity in soft tissues [100].
One technique to measure the phase velocity is the phase gradient approach, which estimates the velocity using the phase difference evaluated at different spatial locations for specific frequencies [45, 85, 96]. An alternative method to estimate the shear wave phase velocity is performed by two-dimensional Fourier transform (2D-F) analysis, which converts spatiotemporal data to a wavenumber in the frequency domain, and uses the peak magnitude distribution to estimate the phase velocity [101, 102]. The dispersion either from the phase gradient or 2D-F can be fitted to rheological models to quantify viscoelastic parameters of the medium [45, 103]. The attenuating nature of a tissue is the cause of the dispersion of the phase velocity. The shear wave dispersion and attenuation can be estimated by the computation of a power law coefficient, with the assumption of a power law rheological model for the tissue [100, 104].
Local phase velocity imaging (LPVI) [105, 106] is another method that can produce a phase velocity map. The LPVI requires applying bandpass filters to obtain the maximal frequency range for the phase velocity. Although this method demonstrates good reconstructions of 2D shear wave phase velocity, results are sensitive to the frequency range selected, and they may change when using different transducers, focal configurations, and focal depths [78].
The dependency of the wave amplitude with distance is attributed to geometrical spreading of the wave energy and to viscoelastic attenuation. Wave diffraction by geometrical spreading can be reduced by using the method of [50] that is considering cylindrical shear waves produced by a supersonic radiation pressure source. Other methods including this assumption were based on a 2-D Fourier transform and the computation of the spectral width to assess the frequency dependent attenuation [75, 107, 108]. A robust method assuming a cylindrical wavefront and no rheological model is the attenuation-measuring ultrasound shear wave elastography (AMUSE) algorithm [107]. This method, however, does not provide any attenuation map since the computation requires all datasets within the selected region-of-interest. Since biological tissues such as the kidney, muscles, and tendons are anisotropic, and because the wave produced by a linear SW front may no longer be cylindrical in those media; then, abovementioned algorithms may lead to inaccurate results [96, 109–112].
Frequency-shift methods used for compression and seismic wave analyses [113–115] inspired the field of shear wave elastography to assess tissue viscosity. Frequency-shift methods are not based on wave amplitude, so the dependency of these methods to geometrical wave spreading is released [113]. Bernard et al. developed such a frequency-shift method for shear wave attenuation by model-fitting of the amplitude spectrum [87]. This method made a few assumptions, which may not hold in all viscoelastic media such as fatty liver. A two-point frequency-shift method was later proposed by Kijanka and Urban to soften assumptions made by Bernard et al.; in their report, they considered a varying shape parameter of the gamma distribution used to fit the shear wave amplitude spectrum [116]. This technique used only two spatial points instead of all points along the propagation path, as in [87], to estimate the attenuation coefficient [116]. Using only two spatial points reduces the computation time but may affect robustness in cases of noisy shear wave displacement maps. Viscosity maps based on the cylindrical wavefront assumption of [50] or frequency-shift method of [87] can be found in [51].
A few examples of ultrasound shear wave viscoelasticity imaging applications are presented next. The reader may refer to recent review papers on this subject for other examples [117–119]. The focus below is on the liver and breast as those organs were largely investigated in clinical studies using SWE.
Liver fibrosis occurs when an abnormal large amount of liver tissue becomes scarred. It can lead to cirrhosis, its long-term sequel, and further evolve as hepatocellular carcinoma [120]. Liver fibrosis can be differentiated into 5 categories, from F0 for a normal liver to F4 for cirrhosis; these categories have been obtained by biopsy intervention, which is the gold standard for liver classification. However, liver biopsy is invasive, could lead to bleeding or worse outcomes, and even death [121], and because a small amount of tissues is taken, it is not always representative of the full liver due to sampling errors [118, 122]. Fibrosis is one pathology known to increase liver stiffness [69, 123–126] along with inflammation, edema, congestion and extra hepatic cholestasis [64, 127, 128]. Shear wave elastography was mainly used to classify fibrosis based on liver elasticity using different cutoff values. This imaging method is accurate to assess liver fibrosis of stage 2 and higher [69, 123, 129–131], has a good repeatability [132], and may allow to diminish the number of biopsy [133]. Yet the impact of steatosis on liver stiffness is uncertain [88, 134–136]. To overcome this, some teams proposed investigating viscous properties. If no clear consensus is reached yet, a few studies showed promising results based on shear wave dispersion and attenuation to assess steatosis stages [88, 137] or necroinflammation [138, 139]. Shear wave elastography presents some limitations for liver imaging, such as difficult measurements in obese patients, and confounding impact of factors such as inflammation, which can increase liver stiffness or change the liver stiffness threshold for classification. In addition to fibrosis assessment and classification, SWE was also proven useful to follow patients with chronic liver disease [65]. An example of a liver SWE image is given in Figure 3.
FIGURE 3. Example of superimposing the shear wave elastogram on the corresponding B-scan in a 34-years-old man with histologic F1 fibrosis. The dynamic range of Young moduli was set between 0 and 40 kPa during the clinical exam, which was sufficient to cover the range of values expected for the four stages of liver fibrosis. Reproduced with permission from [69]. Copyright 2015, Elsevier.
Shear wave elastography is used to help identify breast cancers, since it has been shown that malignant tissues appear stiffer than its healthy counterpart [140–142]. X-ray mammography, MR imaging and ultrasonography are used to detect tissue lesions or to classify suspicious masses into different categories, typically classification 0 for incomplete data to 6 for histologically proven malignancy. Nonetheless, excluding the expensive MR imaging method, these approaches have poor specificity and mammography often find false negative results in dense breasts [143]. Category 4, which corresponds to suspicion for malignant tissues, has a degree of certainty varying from 2 to 95% to assess malignancy proven by biopsy, and has a cancer detection rate of 10–30% [144]. Shear wave elastography allowed improving breast lesion characterization [145–148], and reducing the number of unnecessary invasive biopsy due to the improvement in specificity [148–150]. Elasticity parameters such as the maximum or mean Young modulus E within the lesion, and in surrounding tissues, are used to separate benign from malignant masses. Recent works investigated viscosity behavior using the shear viscosity [151], linear dispersion slope [152], and storage and loss moduli [153] to differentiate malignant from benign tissues. Ultrasound data on the viscous behavior of breast lesions are scarce but may prove to be of clinical value in the future. Some studies investigated the use of SWE as a tool to monitor cancer treatment performance [154], with a decrease in malignant mass elasticity during treatment, or for early prediction of therapy successes [155, 156], with a better treatment response for softer tumors. Figure 4 gives examples of Young’s modulus elasticity maps of breast lesions.
FIGURE 4. Examples of shear wave elastography images of breast tissues. (Top two panels): A 50-year-old woman with an abnormality in her left breast on screening mammography is presented. Biopsy was made on the red region (high Young’s modulus), and concluded that the lesion was an invasive carcinoma (pT1a, pN0). The first panel gives the SWE map superimposed on the B-mode image, whereas the bottom panel is the B-mode image. (Bottom two panels): A 48-year-old woman who presented with an abnormality in her left breast on screening ultrasound is presented. Biopsy was made on the suspicious region, and concluded that the lesion was a fibroadenoma (benign tumor). The blue color on the shear wave elastography image indicates a low Young’s modulus. The third panel gives the SWE map superimposed on the B-mode image, whereas the bottom panel is the B-mode image. The range of Young’s moduli on the colorbar is from 0 to 180 kPa. Reproduced with permission from [157]. Copyright 2015, Elsevier.
Although SWE has targeted mainly the liver and breast, other organs and techniques have been developed. Prostate cancers [158], thyroid cancer nodules [159], and blood clot characterization [160] have been investigated, to name a few examples, with the Young’s modulus as the descriptive mechanical parameter. If the assumption of an isotropic medium is generally accepted for most organs, it is not the case for muscles and tendons. Anisotropic and transversely isotropic models using shear waves have been recently investigated [24, 161, 162], some other teams explored viscoelastic properties using different probe orientations [109, 163–165]. A non-exhaustive list of SWE clinical applications can be found in Table 2.
Magnetic resonance elastography (MRE) is another non-invasive imaging technology for assessment of mechanical properties of soft tissues. Since its first description by Muthupillai et al. in 1995 [166], MRE has been integrated into clinical routines for liver disease detection, and has shown great potential for other organs, notably the brain, of which only MRE can assess the in-vivo viscoelastic components without surgical intervention. Principles of MRE investigation are similar to those of any SWE method (Figure 5). A major feature of MRE resides in its ability to measure 3D displacement fields by simply changing the axes of encoding gradients, which is an advantage over other imaging devices operating elastography. The main drawback may be found in the longer scan times relative to ultrasound elastography for instance. MRE has a poor temporal resolution and relies on a stroboscopic-like recording arrangement to generate time resolved images, as opposed to ultrasound SWE where burst measurements are performed at a high acquisition rate. Typically, MRE data contain 4 to 8 images per harmonic actuation cycle. Spatially, MRE is sometimes referred to as a super resolution imaging modality as measured displacement amplitudes are much smaller than the image pixel size (tens of microns versus one to 3 mm). We review in this section the main three steps in MRE investigation, namely motion generation strategies, motion encoding techniques, and inversion methods. Finally, applications to the liver and brain are discussed. These organs were subjectively chosen as liver disease diagnosis is the only MRE protocol clinically established, and non-invasive in-vivo brain mechanics assessment is not enabled by any elastography techniques other than MRE.
FIGURE 5. Workflow in MRE investigation. Small time harmonic deformations are generated in the scanned tissue using an external actuator or natural body pulsation. These small deformations are recorded by the MR scanner using tailored acquisition sequences to produce time series propagation images. The complex MR signal is then processed to extract the motion field data, which is finally used as an entry to physical modeling allowing for calculation of mechanical parameters.
In MRE, most applications involve the generation of time-harmonic wave fields using external surface actuators. These actuators must meet the requirements imposed by magnetic resonance safety rules, in other words they must be made of non-magnetic materials and be adaptable to fit into the experimental or clinical magnet bore of the scanner. Design of actuators has been shown to be application dependent; we review in this section the main techniques to induce motion in soft tissues in the context of MRE. Loudspeakers have been widely used to transmit motion to tissues and may be divided into pneumatic and rigid categories. In the pneumatic one, air pulses are transmitted from the loudspeaker pulsing membrane to the investigated tissue by means of a wave guide (plastic tube). The tube is connected to an interface attached to the surface of the tissue. Plastic pads with a soft membrane and air cushions are the main examples of such interfaces. Main advantages are versatility allowing for applications to various organs [167–176], and the electricity-free transmission system (pneumatic) requiring no electrical current inside the magnetic resonance imaging (MRI) room. The main drawback may reside in the limitation to simple mono-frequency waveforms only. In the rigid category, loudspeakers transmit motion to tissues via a rigid rod attached to the membrane on one end, and to the patient on the other end. This configuration is also versatile [177–184] and handles arbitrary waveforms but requires the loudspeaker to be inside the MRI room. Lorentz-coil actuators have also been used to generate motion in various organs [185–192], and rely on the coupling of the MRI magnetic field B0 with an electrical current injected into the coil. These actuators must be designed according to the targeted organ as they are placed relative to B0. Piezoelectric drivers allow to deliver arbitrary waveform pulses to tissues while avoiding the constraint of positioning relative to B0. Significant displacement fields could be obtained using this technique in various conditions (human abdomen [193, 194], human brain [195, 196], human breast [197], and mouse brain [198, 199]).
A major bottleneck in generating sufficiently high motion deflection in tissues is the decrease of the motion amplitude with increasing excitation frequencies. Using a wide range of mechanical excitation finds its application in the analysis of frequency dependent mechanical behavior of soft tissues [8, 178, 191, 198, 200–203]. The more frequencies, the more valuable is the information. Centrifugal force based MRE drivers have been proposed to circumvent this limitation at high frequencies. The centrifugal force allows to maintain the displacement amplitude high regardless of the frequency [204]. The “air-ball” actuator and “gravitational actuator” are the first examples of the centrifugal force implementation. The “air-ball” actuator [205] consists in a ball circulating in a circular chamber under injection of compressed air. The revolution speed of the ball is imposed by the pressured air of which the pressure determines the actuator vibration frequency. The “gravitational transducer” is made of a mass attached to an axis and rotating around this axis. The rotation speed is driven by the rotating axis connected to a motor [206]. All the aforementioned techniques consist in shaking the surface of the probed tissue, which implies that elastic waves propagate to the region of interest with sufficient amplitude. This can be an issue if the imaged domain is deep under the surface thus increasing the risk of high attenuation. Producing a wave field in situ may be an alternative way to ensure that a sufficient amount of displacement remains in the region of interest. In that regard, focused ultrasounds have been used to generate shear waves along with an MR scanner for motion detection [207]. This technique requires a heavy experimental setup compatible with the MR environment and has not been, to date, more than a proof of concept. Instead of using external devices to produce motion at chosen locations, the concept of intrinsic actuation taking advantage of natural internal vibrations has gained interest. This method consists in encoding motion induced in organs by the natural pulsation of the heart and arteries, and presents the significant advantage of not requiring any additional equipment. Tailored MRE protocols must be adopted to adapt to the low frequency characteristics of natural pulsations (around 1 Hz). For now, intrinsic actuation has been applied to the brain [208–211].
Whereas most magnetic resonance imaging protocols attempt to reduce or compensate for motion, MR elastography seeks to take advantage of small vibrations in the scanned tissue. Numerous MRE specific pulse sequences [chronologically sorted application of radiofrequency (RF)-pulses and magnetic gradients to generate and manipulate the MR signal] have been designed to acquire driven or natural motion in biological tissues, while maintaining reasonable scan times and image quality. We review, in this section, the main concepts of MR elastography pulse sequences allowing for detecting acoustic wave propagation. More specific details and theory, along with fast acquisition strategies are available elsewhere [19, 212]. Motion encoding principles in MRI were first introduced by measuring sea-water velocity [213], and further applied in the context of angiography to measure blood flow [214]. The proof consisted in relying spin velocities to the phase shift spins experienced when space and time varying bipolar magnetic fields are applied. A similar concept, leading to MRE, was developed in which motion of spins around their position at rest is encoded in the phase of the complex MR signal using magnetic-field gradients, named motion encoding gradients (MEGs) [166]. The accumulated net phase of moving spins varies according to their trajectory while a time dependent MEG is applied. This net phase thus allows to track local motion of tissue eventually providing the displacement maps required for retrieving mechanical parameters. MRE sequences are generally based on existing MR encoding outfitted with MEGs. Not only are their setting key to be compatible with the characteristics of the induced motion but so are other inherent MR imaging parameters, leading to a broad variety of MRE sequences.
The timing of the chosen MR based-sequence rises a certain amount of constraints regarding scan time, motion sensitivity, and image quality. The MR sequence design and underlying physics are beyond the scope of the present review, thus only the main concepts relevant to overall MRE understanding is briefly discussed. For in-depth details, see [19, 215]. When a tissue is placed in the strong static magnetic field B0 of an MR scanner, a net magnetization aligned with the magnetic field is produced from the contribution of each uncoupled individual nuclear spin (those of hydrogen nuclei in clinical scanners) [215]. The key concept in MR signal generation consists in tipping the net magnetization of the desired portion of the scanned tissue out of its resting state using some excitation radiofrequency pulses tuned at the Larmor frequency of the spins in the scanner [216]. Magnetization enters a precession motion about the B0 axis under the effect of this RF-pulse. Once excited, the magnetization is no longer aligned with B0 and tends to realign and reach its resting state back again. This process called relaxation occurs at a certain time rate dictated by the interactions between spins themselves and with their environment. The time constant T1 characterizes the exponential regrowth of the magnetization parallel to B0 (longitudinal component of the magnetization) due to spin-lattice interactions. Time constants T2 and T2* characterize the exponential decay of the transverse magnetization component (perpendicular to the longitudinal one) due to spin-spin interactions and magnetic field inhomogeneity (combined with the spin-spin interaction), respectively.
Timewise, T2* < T2 < T1 and decay rates are given by R2* = 1/T2* and R2 = 1/T2, where R2* > R2. The time evolution of the MR signal immediately following the RF-pulse excitation is named free induction decay (FID) and is governed by T2* effects. Receive coils are used to monitor relaxation by measuring the voltage induced by the precessing magnetization according to Faraday’s law. Manipulation of the magnetization allows to generate MR signal peaks at adjustable delays after the application of the excitation RF-pulse, i.e., during the FID, or later when the signal appears to have vanished. MR sequences may be divided into two main categories of mechanisms leading to different timing for data acquisition. Spin echo (SE) sequences employ a second RF-pulse called refocusing RF-pulse, occurring after the FID, and allowing for compensation of T2* effects (magnetic field inhomogeneity). Some of the MR signal can thus be recovered after the FID. In this case, the limiting time constant becomes T2 > T2*. Passed the exponential decay due to T2 effects, the MR signal can no longer be recovered. The peak of the recovered MR signal, the echo, occurs at the “echo time” TE after the application of the RF-pulse excitation. Gradient recalled echo (GRE) sequences, however, typically operate within the FID (occurring immediately after the RF-pulse excitation) and do not allow for magnetic field inhomogeneity effects compensation. The operating window in such sequences is thus limited by the T2* weighted decay, and thus leads to much faster acquisition protocols. Magnetic field gradients are used, instead of a second RF-pulse, to manipulate the magnetization and generate a signal echo at TE. As aforementioned, MRE sequences usually consist in incorporating motion encoding gradients into an MR based-sequence. This modification is consequently subjected to timing limits of the base-sequence. The short timing of gradient echo type sequences presents a narrower time slot for the MEGs to operate than that of spin echo type sequences. The impact of such inherent characteristics is discussed below.
The first descriptions of the motion encoding mechanism in MRE were reported in Refs. [166, 217]. As aforementioned, a spin moving in the presence of a magnetic-field gradient G experiences a phase shift ϕ:
where γ is the gyromagnetic ratio of the material [rad s−1 T−1] and r is the time-dependent position vector of the spin. From this equation appears that the phase shift depends on both the spin trajectory r and the applied G. Consequently, a given arbitrary spin motion results in different accumulated phases depending on the magnetic gradient waveform. Hence, the remaining definition of G sets the type of motion the encoding process is sensitive to. Since the inherent function of magnetic field gradients is to add a controlled space-dependency to the static and homogeneous magnetic field B0, even static spins experience a space dependent phase accumulation while MEGs are switched on. In order to cancel this unwanted phase accumulation, G can be set to oscillate in time allowing the phase accumulated during the first half of the gradient oscillation period to be compensated during the second half. This technique is called zeroth moment nulling [19]. Non-oscillating MEGs are called unbalanced gradients and are thus rarely used in conventional MRE sequences. Additionally, the effect of constant velocity and constant acceleration background components in moving spins may also need to be cancelled. This can be achieved by applying first and second moment nulling, respectively [19]. Both consist in adjusting the MEGs oscillation profile so that the accumulated phase in Eq. 21 goes to zero for unwanted spin motion.
Many MRE applications have resorted to full wave encoding (MEGs tuned to the same frequency as that of the motion oscillation) with zeroth [188, 197, 198, 218–221] and first moment nulling [166, 172, 173, 175, 181, 203]. For all types of oscillating gradients, the area under the curve of MEGs over the operation time must equal zero for proper motion encoding. Early in MRE, several examples of motion encoding strategies were derived in the case of time-harmonic excitation, leading to time-harmonic spin trajectories and involving full-wave encoding [217]. Spin trajectories r can then be written as:
where r0 is the position vector of spins at rest, ξ0 is the spins displacement amplitude, k the wave vector, r the position vector, ω the oscillation frequency, and θ some initial phase offset. Solving Eq. 21 using Eq. 22 and the MEG temporal profile allows to quantify the encoding efficiency, which is defined as the amount of phase shift in the signal per displacement unit. Encoding efficiency formulas for common MEG waveforms are available in Refs. [19, 212].
Full wave encoding scheme ensures a good motion sensitivity but compels the minimum achievable value of the echo time dependent on the driver actuation period. GRE sequence short timing due to T2* effects is well suited to high actuation frequencies (short time slot for MEGs to operate); however, it limits the applicable number of MEG cycles [166, 188, 197, 220, 222, 223]. The optimal setting resides at the trade-off between the SNR increase permitted by short TEs and the higher motion sensitivity permitted by multiple MEG cycles. A similar conclusion can be drawn with regards to SE sequences, which present a more flexible timing enabled by their inherent longer echo and repetition times [215, 224]. Multiple MEG cycles can thus be incorporated into the sequence while maintaining the echo intensity sufficiently high. SE sequences are often implemented with fast readout strategies, for instance echo planar imaging (EPI) necessitating only one or few combinations of excitation- and refocusing-RF pulses to generate a whole image, which allows to circumvent the use of many long repetition times (TRs) [172, 175, 181, 203].
So far, single actuation frequency cases have been presented. Full-wave encoding can also be used to extract a frequency of interest from a multi-frequency oscillating wave field by selecting proper MEG profile (frequency and number of cycles) [177, 222, 225]. This configuration presents little interest in cases where the actuation frequency is chosen by the user. The only way of performing multi-frequency acquisitions using full-wave encoding is to repeat the encoding for each frequency separately [188, 191, 198, 201, 219, 223, 226], which has an impact on the acquisition time. However, multiple frequency components can be simultaneously encoded in a single acquisition using wide band MEGs, and manually selected using a temporal Fourier transform [177, 178, 180, 182, 210, 227–230]. The main advantage of simultaneous multi-frequency encoding is the time saving making them more suited to in vivo studies compared with repeated single frequency acquisitions over a given frequency range. The main drawback is the overall lower motion amplitude at each frequency of the multi-frequency actuation compared with the repeated acquisition scheme, due to total energy deposition divided into the total number of frequencies.
The wideband property of MEGs has been further extended to fractional encoding where the frequency of the mechanical oscillation is smaller than that of MEGs, and the mechanical time period is larger or equal to the repetition time [186]. With shorter MEG time periods, scan duration can be reduced and higher SNR can be obtained by shortening the echo time accordingly [190]. A major advantage of this approach is found in measurement of low frequency induced motion, such as heart pulsation driven actuation (around 1 Hz), where full-wave encoding would lead to unpractical echo times [208, 209, 211, 231]. Despite the lower motion sensitivity in fractional encoding, this method has proven successful using fast acquisition protocols (spoiled GRE and GRE/SE equipped with EPI readout strategy) [169, 170, 179, 190–192, 201, 209, 232–234]. Besides multi-frequency acquisitions, reduced TE and TR permitted by fractional encoding have also been exploited in balanced steady state free precession MRE [186, 235, 236], despite the original development circumventing the use of MEGs [237]. Although high phase-to-noise ratios were reported, this sequence type presents significant timing constraints (actuation frequency linked to TR), and non-linear phase accumulation between consecutive TRs leading to additional signal post-processing steps. It has consequently been used only sporadically [19, 190, 212].
The previous section reviewed some acquisition approaches to measure motion induced in the tissue of interest. The last essential step in elastography consists in relying these displacements to mechanical parameters using physical models. This section addresses the most reported inversion schemes employed in MRE. More specific information about processing times and modeling details can be found in Refs. [238, 239]. A major strength of magnetic resonance is the capacity of encoding motion in the three directions of space, allowing for full 3D inversion of the Navier equation. This strength comes at the cost of overall longer scan times for which alternatives have been discussed above. Despite the availability of fast 3D MRE sequences, all mechanical parameter reconstruction methods do not make use of complete displacement data sets and take advantage of physical assumptions allowing for processing of reduced dimension displacement data. We propose to classify inversion schemes into two categories. The direct approach consists in formulating the inverse problem with the mechanical parameters as unknowns. Experimentally obtained displacement data are inserted into the equations of elasticity, and quantities of interest are extracted through direct inversion. The iterative approach consists in iteratively solving the forward problem for displacements starting from an initial set of guessed mechanical parameters. These mechanical parameters are iteratively updated to minimize the difference between experimental displacement data and computed displacement solution. The final solution is the set of mechanical parameters that makes that difference converge to a global minimum.
The first reported inversion method in the context of MRE, assuming isotropy, local homogeneity, no attenuation, and incompressibility, consisted in estimating the local wavelength of the measured wave field. This technique is termed LFE (local frequency estimation). Briefly, pairs of filters centred on spatial frequencies usually separated by one octave are applied to the wave field. The ratio of displacements filtered by each filter of one pair equals the local wavelength [240]. To ensure that local spatial frequency is included in the bandwidth of the filter pair, the process is repeated over a certain range of frequencies. From the evaluated wavelength (inverse of spatial frequency), the magnitude of the shear modulus |μ| is retrieved using
Early in MRE were also reported direct methods assuming viscoelastic materials, as described in General Concepts in Shear Wave Elastography, and using the strong formulation of the Helmholtz equation [198, 220, 244]. The underlying assumptions of isotropy, local homogeneity, and incompressibility are used to neglect the stiffness gradient across the tissue, and to decouple motion components in the equation system. From there, a single motion component can be used to retrieve the complex-valued shear modulus (G* = G′ + jG″). Planar assumption allows to consider the 2D curvature of the wave field instead of its 3D, which further decreases the required amount of data to solve the inverse problem. This method has also been widely used [177, 178, 182, 219, 227–229, 243, 245] given its simplicity and low computational cost but strongly depends on data filtering and evaluation of second derivatives [244, 246]. Using this scheme, stiffness reconstruction was shown to be altered by the neglected first Lamé parameter (Eq. 8) [247]. Applying the Helmholtz decomposition to the wave field allows to separate divergence-free (shear) from irrotational (compressional) components. Taking the curl of the Helmholtz equation increases the differentiation order but physically isolates the shear component of interest. This approach has become prominent when the Helmholtz equation is employed to retrieve storage and loss moduli [172, 187, 189, 190, 198, 199, 232, 247, 248]. To improve resolution and stabilize the direct inversion of the mono-frequency Helmholtz equation, a multi-frequency approach named MDEV (multi-frequency dual elasto visco inversion) was introduced [200]. A multi-frequency wave field is built upon data sets of individual different frequencies, ignoring the dispersion of mechanical parameters with respect to frequency, reducing the risk of nodes due to standing waves and stabilizing the equation system by adding equations with same unknown. Various studies have resorted to inversion schemes based on this method [8, 193, 194]. As an alternative to the 2nd order derivative assessment required by MDEV, a phase-gradient based method termed k-MDEV was proposed. It consists in evaluating the complex wave number k of a plane wave (see General Concepts in Shear Wave Elastography), which can then be related to both phase velocity for elastic modulus estimation and attenuation for viscous behavior quantification [194].
A finite-element (FE) based inversion method was recently proposed, also assuming local homogeneity, for storage and loss moduli assessment. It takes advantage of the weak form of the equations of motion to reduce the differentiation order, and exploits divergence-free test functions to lower the impact of the compression field [249]. So far, most of the discussed approaches have in common the assumption of local homogeneity, neglecting the gradient of mechanical parameters across the tissue, and incompressibility, invoked to neglect terms involving the divergence of displacements (unless the curl operator is applied). The local homogeneity assumption was shown to alter the reconstructed mechanical parameters in regions where the latter are not constant [218], that is in most clinical cases and notably tumorous tissues. Direct methods, still employing the strong form of the Navier equation and neglecting the divergence of the wave field, were proposed to consider heterogeneity using single [250] and multifrequency (HMDI–heterogeneous multifrequency direct inversion) [251] approaches. To address the compression aspect in nearly incompressible materials, which was shown to lead to artefacts and inaccuracies [252, 253] when disregarded [253] or processed using displacement formulation only [254, 255], direct FE formulations of the inverse problem have been proposed using curl-based [256] and mixed displacement-pressure [253, 256, 257] schemes.
Overall, iterative methods make less restrictive assumptions on tissue mechanical properties than direct ones relying on the algebraic strong formulation of the elasticity equations, and have been reported to solve for more unknowns than FE-based direct methods by adjusting the number of parameters to update in the minimization process. From a computational standpoint, solving forward problems, that is, mapping data information (i.e., displacement field) from source information (i.e., elasticity distribution) is a smoothing process. On the contrary, inverse problem consisting in mapping source information (i.e., elasticity distribution) from data information (i.e., displacement field) is a noise-enhancing process [258]. Consequently, iterative approaches tend to be more robust against noise than direct ones. Following FE discretization approaches, similar to direct FE ones, iterative schemes have been developed [259–261]. Near incompressibility is often assumed and requires to modify the formulation of elasticity equations in order to solve for pressure in addition to displacements (aforementioned mixed “pressure-displacement” formulation), and material heterogeneity is mostly considered [257, 262]. In MRE, the subzone technique has gained significant interest amongst iterative processes [263]. It consists in dividing the imaged domain into overlapping subdomains termed subzones, and solving iteratively the forward problem for displacements in each subdomain parallelly [197]. Once the solution in each subzone has converged, subzones are randomly redistributed over the domain and the iterative solution calculation is performed again. Retrieved mechanical parameter distributions, corresponding to each subzone distribution, are finally averaged to form the final solution. This reconstruction method has been applied to phantoms, brain [176, 203, 264, 265] and breast [266, 267] data, and has proven its capacity to reconstruct multiple variables at various actuation frequencies using elastic and viscoelastic physical models (compressible elastic [268], compressible viscoelastic [269], and nearly incompressible viscoelastic [176, 184, 203, 231, 264–266, 268, 270–272]). Additionally, poroelastic models have been introduced for accurate consideration of the biphasic nature of entangled solid-liquid structures in biological tissues [168, 184, 211, 270, 271, 273]).
MRE can be applied to virtually any organ provided sufficient displacement data quality and suitable inversion scheme. We mainly restrict our discussion to liver applications due to the clinical availability of the technique, and to brain of which in-vivo mechanical properties have yet only been non-invasively accessible using this elastography method. MRE in clinics has so far been restricted to liver scanning for fibrosis, and diagnosis of chronic liver diseases using purely elastic models, i.e., assessing the shear modulus only and ignoring the tissue’s viscous behavior. Under these assumptions, meta-analyses over the past few years on liver MRE have highlighted the high performance of the method in distinguishing liver fibrosis stages in non-alcoholic fatty liver disease, and cirrhosis considering the stiffness increase under this condition [274, 275]. Viscoelastic parameters in human liver diseases have also been early investigated using multi-frequency MRE to assess the frequency dispersion of storage and loss moduli (G′ and G″) in healthy and fibrotic patients [180]. Results showed an increase in both G′ and G″ in fibrotic with respect to healthy livers. Evaluation of the elastic modulus and viscosity using a standard linear solid model in healthy and fibrotic human livers led to a similar conclusion, where both elasticity and viscosity increased in pathological liver tissues [178]. This trend was also reported in [276], and in a performance study of MRE in the detection of fibrotic livers [277]. Overall, stiffness only or both stiffness and viscosity increases have been observed against the fibrotic stage. Interestingly, a case of liver steatosis in rats where only viscosity varied while stiffness remained unchanged has been reported in [278]. Despite these findings, the storage modulus was found to correlate much better with stages of liver fibrosis than viscosity [279]. Additionally, measurement of wave damping for viscosity characterisation is influenced by reflections off boundaries and renders its measurement troublesome. To date, stiffness variations for estimation of liver fibrosis severity has been mostly investigated.
MRE has also been proven successful and robust in the brain [280]. Its high water content and the observed shear wave attenuation in MRE acquisitions suggest that restriction to purely elastic models may lack of accuracy. The healthy brain’s viscoelastic behavior has been highlighted by evaluating the dispersion of reconstructed mechanical parameters at varying frequencies in humans [8] and rats [198]. Both storage and loss moduli tended to increase with frequency. Additionally, cerebral viscoelasticity was shown to follow a frequency power law, where all reconstructed parameters vary independently [203]. These reconstructions suggested that the falx cerebri’s viscous behavior is singular in comparison with other brain regions. Along similar lines in healthy brain characterization, viscoelasticity changes due to physiological aging have been considered [227, 229, 281]. From these studies appear that the brain softens but sees its relative viscous-to-elastic behavior unchanged over time. Such investigations along with MRE of neurological diseases have underlined the high potential of this technique in detecting neurodegenerative pathologies [282]. Non-invasive differentiation of natural structures of the brain based on their mechanical response to stimulus imparts MRE a significant advantage. For instance, the cerebellum has been shown to be softer and tends to be less viscous than the cerebrum [189].
High resolution mapping of stiffness and dispersion effects have suggested that cortical white matter is stiffer and more viscous than grey matter [191]. MRE has also been used to quantify the viscoelastic changes of altered brains. Notably, Alzheimer’s disease was shown to reduce the brain’s stiffness (elasticity only) [181]. Glioblastoma has been shown to take lower stiffness and viscosity values using multi-frequency MRE in humans [201], and mono-frequency MRE in a rat model [283]. A similar softening trend was observed in multiple sclerosis [182, 228, 284], where viscoelasticity was assessed using a global parameter. On the other hand, normal pressure hydrocephalus appears to trigger the opposite effect [175, 211]. Recent research on brain viscoelasticity has opened new avenues in the understanding of connexions between cerebral functions and tissue mechanical behavior. For instance, joint investigation of hippocampus viscoelasticity (shear stiffness and damping ratio), and relational memory has allowed to correlate hippocampal viscoelastic variations to performance in completion of spatial reconstruction tasks [285]. Viscoelasticity was characterised using an adjusted damping ratio that indicates the dominant tissue behavior between elasticity and viscosity. Results showed that better relational memory performance correlated with a rather elastic mechanical behavior of the hippocampus. This constituted the first observation of the kind.
The same principle was applied to assess the correlation between cardiovascular health through aerobic fitness exercises, relational memory performance through spatial reconstruction tasks, and hippocampal viscoelasticity using MRE. The study showed that better memory performance was associated with higher values of the adjusted damping ratio, which was itself associated with better aerobic fitness performance [286]. Light fitness exercise has also been shown to have a potential impact on hippocampal viscoelasticity and associated cerebral functions in multi-sclerosis patients [287]. These investigations laid the first stone for the characterisation of relationships between physical and cerebral functional behaviors, and brain viscoelasticity [288–291].
Finally, most advanced improvements in viscoelasticity characterisation embed tissue anisotropy, which is particularly relevant in the brain given its fibrous structure. As a deviation from brain applications: the first use of anisotropy in MRE was proposed for breast tumor detection through the evaluation of an assumed symmetrical stiffness tensor [292]. Results suggested that carcinoma have an anisotropic structure revealing a preferred orientation, certainly due to vascularisation, and suggesting transverse isotropy. Breast cancer was then also characterized assuming a transversely isotropic model leading to a 5-parameter reconstruction [247]. Again, results showed a preferred orientation in the tumor structure. Transversely isotropic mechanical property recovery was experimentally validated in fibrous tissues using MRE and diffusion tensor imaging (DTI) [293]. In-vivo brain anisotropic stiffness assessment in humans assuming separately orthotropy and transverse isotropy suggested that white matter exhibits a transverse isotropy structure [20]. Shear wave speed analysis was performed from prior knowledge of fiber orientation using DTI. Two shear wave modes were then observed, a faster longitudinal mode relatively to a slower transverse mode. The transverse anisotropy of white matter was later nuanced in favor of a mild only anisotropy in a study on ex-vivo porcine brain, where a purely transverse shear wave mode was generated and used to estimate three isotropic parameters in the absence of longitudinal modes [294]. Human brain anisotropy was also highlighted using variations in reconstructed stiffness distribution depending on actuation direction [176]. This constitutes a direct measurement of the anisotropy impact in isotropic models. Such observation was quantified using a finite element formulation of a heterogeneous, nearly incompressible, and transverse isotropic model providing benchmark displacement fields for inversion testing [265].
In addition to the significant research effort in evaluating and understanding cerebral viscoelasticity, the high water content of the brain has motivated to consider it as poroelastic, i.e., made of two, solid and liquid, entangled phases. The impact of poroelasticity versus viscoelasticity on reconstruction has been shown to be relevant at low frequencies (a few Hertz) using the forward problem formulation in the harmonic regime, and the aforementioned subzone iterative scheme [184]. At higher frequencies, viscoelasticity seems to remain a more suitable model than poroelasticity. Overall, poroelasticity and low frequency intrinsic actuation thus constitute an interesting and original package in MRE investigation. This setup circumventing resorting to pulsing equipment has been used in a few studies, and holds promise for more accurate detection of brain pathologies [208, 211]. Another approach to highlight brain poroelasticity was to solve for both shear and bulk moduli using the algebraic inversion technique, which resulted in a bulk modulus much lower than expected, confirming the poroelastic nature of the brain (compressible solid matrix and incompressible fluid channels) [209]. More recently was proposed an improvement in MR poroelastography acquisition processes allowing to separate solid and fluid contributions to the shear motion field using an inversion recovery sequence adapted to MRE, along with a tailored MR signal modeling [295].
To conclude, Figure 6 illustrates typical wave maps and elastograms from MRE acquisitions in the liver, breast, heart, and brain. Table 3 presents an overview of main components constituting MRE investigations, from motion generation techniques to inversion categories described in previous paragraphs.
FIGURE 6. (A) MRE of the liver. Anatomic images, displacement and shear stiffness maps of healthy and stage 4 fibrotic livers. Reproduced with permission from [296]. Copyright 2007 AGA Institute. (B) MRE of the breast. Anatomic image and shear stiffness map of a biopsy-proven invasive ductal carcinoma. Reproduced with permission from [297]. Copyright 2020 Elsevier. (C) MRE of the heart. Shear wave amplitude maps of a healthy heart versus hearts with diastolic dysfunction. Reproduced with permission from [234]. Copyright 2014 RSNA. (D) Mean shear stiffness of the cerebrum in young and older adults. Reproduced with permission from [281]. Copyright 2018, Elsevier.
Elastography based on ultrasonography or MRI has found popular clinical applications facilitated by the implementation of those imaging technologies on clinical systems. These tools can provide images over centimeter to whole-body depth ranges. However, many applications require millimeter-scale spatial resolution images, which can only be made possible using optical means. For example, on the cellular scale, the measurement of mechanical properties requires higher resolutions to focus on the understanding of how cells respond to physical forces. Thus, the use of optical elastography provides an opportunity for microscale imaging and for numerous applications in fundamental research [298].
Within the last 2 decades, developments in this new area of imaging led to multiple scientific advancements at the interface between optics and mechanics, which included biomedical applications in ophthalmology, oncology, and cell mechanics. The following subsections discuss recent developments in cellular and optical elastography, and their applications across biomedical and life sciences.
Tissue elasticity at a microscopic scale is determined by the cell and the extracellular matrix elasticity. Main components of a cell are the membrane, cytoplasm, and cytoskeleton. The latter structure contributes to the cell mechanical stability and characteristics, and to its morphology. An imbalance in the mechanical homeostasis and defect in the cellular mechanotransduction can contribute to various human diseases. Therefore, cellular viscoelasticity can be viewed as a biomarker for determining the cellular state [299]. Determining mechanical properties of a cell during different stages of the disease progression could help to develop novel treatments by considering the role of mechanical factors into genetic and drug therapies [300]. As recently reviewed [301], there are several techniques for measuring cell mechanical properties, most of them requiring a mechanical stress (e.g., micropipette aspiration and atomic force microscopy). However, in this review, the focus is on rheological properties assessed using a mechanical stress based on acoustical shear wave propagation.
Grasland-Mongrain et al. [302] developed a novel method called optical microelastography, also labeled as “cell quake elastography”. This technique uses a high frequency shear wave excitation and an optical microscope to assess cell elasticity. High frequency shear waves inside the cell are produced by a vibrating micropipette at a wavelength comparable to the cell’s size. The wave propagation is captured optically by a high frame rate camera coupled to the microscope. The sampling rate of the camera is selected to avoid shear wave frequency aliasing with sufficient samples per wavelength to allow efficient speckle tracking. The spatial resolution of captured images should also be sufficient to track the shear wave speed from displacement maps (knowing the time elapsed between images). The proof-of-concept in [302] was made by using an ultrasound speckle tracking method adapted to optical images for obtaining displacement maps [303]. A passive elastography algorithm was used as a reconstruction method to obtain shear modulus images [304]. The passive elastography method was inspired by the seismology field [210], so the name “cell quake elastography” for this method. The main advantage of this technology compared with other cell elasticity methods is the time resolution of a few microseconds to produce elasticity maps with a good spatial resolution, which may allow studying dynamic cellular processes.
First experiments were performed on mouse oocytes, making a shear wave inside the cell by a 15 kHz vibrating micropipette, and capturing the traveling wave optically at a 200,000 acquisition frame rate [302]. The sensitivity and spatial resolution of the technique allowed to distinguish the shear modulus of different regions/zones of a cell (Figure 7). The technique was recently applied on mouse macrophage-like RAW 264.7 cell clusters, Abelson leukemia virus-transformed cell line derived from mice, using a 18 kHz stimulation and a 100,000 frames per second image capturing rate. Shear wave displacement maps at 10 µs intervals are given in Figure 8. Future developments should aim at assessing the viscous component of single cells, likely using finite-elements modeling (FEM) reconstruction methods.
FIGURE 7. (A) Experimental set up for optical microelastography, (B) intracellular displacement map through time, (C) elasticity map of the cell into the zona pellucida, cytoplasm, and nucleus. Adapted with permission from [302]. Copyright 2018, National Academy of Sciences.
FIGURE 8. Preliminary results on displacements of the shear wave propagation within adhesive cell clusters of macrophages RAW 264.7.
Optical coherence elastography (OCE) is a technique that can non-invasively assess tissue mechanical properties by measuring the localized deformation, strain or shear wave propagation properties inside a sample [305]. In OCE, a stimulation technique is utilized to load the tissue and its response is recorded with an OCT based detection method [306–308]. The high resolution structural images of OCE (1–10 μm in-vivo) provides it an advantage over the ultrasound or MRI modality [309], that stretches its potential for micron and submicron imaging of elastic properties of biological tissues. Microstructures of biological tissues can be quantified based on optical scattering properties of the tissue under investigation. OCE holds great potential for diagnosis of many clinical conditions and pathologies, particularly for detection and monitoring of cancers [310], cardiovascular diseases [311], and eye diseases [312].
While optical contrast signals are detected based on differences in two or multiple optical scattering events, the mechanical contrast requires only one scattering event to obtain an OCT signal. Thus, structural inclusions that cannot be detected by OCT can be revealed by OCE if a mechanical contrast exists for the inclusion. The first few studies in OCE development focused on static mechanical contact loading (i.e., no shear wave involved) [313, 314]. Later, the emergence of phase resolved OCT, which is detecting the interferometric phase information from complex OCT signals, enabled the assessment of tissue deformation with a high accuracy for tissue elasticity reconstruction [315–318]. A shear wave stimulus was involved in studies of [316] and [318].
The latest developments include OCE resolution to improve over the range from several microns to hundreds of microns [308, 319, 320]. The lowest range of OCE spatial resolution is similar to the cell quake elastography imaging method described earlier. In comparison, the spatial resolution of ultrasound or MRI elasticity imaging methods remain at a macroscopic level with a typical resolution of hundreds of micrometers to several millimeters, respectively [54, 191]. OCE is a great alternative to traditional elastography methods in terms of spatial resolution, acquisition speed, sub nanometer mechanical displacement sensitivity, but at the cost of a lower penetration depth into the probed tissue than ultrasound or MRI [308]. Additionally, shear wave OCE as a 3D imaging modality may enable its clinical applications in many areas, such as ophthalmology and cardiology using intravascular devices [321–323]. Shear wave based OCE has shown potential for measuring local elasticity changes of mouse brains [324, 325]. Details on these methods are given next.
An OCE system has two main components: a loading system that can deform the biological tissue, and an OCT imaging system for detection. Shear wave methods in OCE are relatively in the very early stages of development. Shear waves-based OCE utilize an excitation from a noncontact air-puff or air-coupled ultrasonic probe [326–328], or piezo-transducers (PZT) [320]. In addition, an OCT mechanism is then employed to detect the displacement field of generated shear waves. By monitoring the shear wave propagation in the sample, elasticity, shear wave speed, or the shear modulus can be quantified. Shear wave visualization was performed in tissue mimicking phantoms with phase sensitive optical coherence elastography [329]. Razani et al. [318] were one of the first to measure the shear wave speed and its associated properties with OCT phase maps. They utilized an external acoustic radiation force mechanism for excitation and a swept-source OCT system to acquire phase images. The central wavelength of the laser was 1,310 nm and the bandwidth was ∼110 nm. The system could register a lateral resolution of 13 μm in gelatin mixed with titanium dioxide phantoms. Images could be acquired at a depth of 3 mm. Song et al. [320] used a piezoelectric point loading to generate shear waves within samples. More recently, Zhu et al. [330, 331] developed a PZT-based system to induce longitudinal shear waves and they visualized the signals using OCT for the quantified mapping of shear moduli. A brief detail of their technique is presented next.
The OCE system included an OCT imaging unit and a PZT excitation unit, as shown in Figure 9A. Elastic waves were induced by a ring PZT actuator driven by a PZT amplifier. The vibrating mechanism of the PZT system could excite three types of waves in the sample under investigation: 1) Rayleigh waves, 2) compressional waves travelling from the top surface to the deep region, and 3) transverse and longitudinal shear waves traveling through the interior of the sample, as shown in Figure 9B. Rayleigh waves propagate at the surface of the sample. Compressional waves propagate parallel to the oscillation direction of the vibrator. Transverse shear waves propagate perpendicular to the displacement direction. Additionally, in the near field of the planar vibration source, which contained multiple sub-sources, a longitudinal shear wave much slower than the compressional wave also propagate along the displacement direction. This longitudinal shear wave is present due to the sum contributions of diffracted transverse shear waves [331]. These longitudinal shear waves could be visualized with the attached OCT imaging unit. The OCT system was based on a swept source at a central wavelength of 1,310 nm, and a wavelength tuning range of 141 nm. Axial and lateral resolutions of the employed OCT unit were 7.6 and 17.7 μm, respectively. The PZT unit utilized for excitation was driven by a function generator producing a sine wave cycle with a frequency of 1 kHz. The displacement observed in the near field was close to 10 μm.
FIGURE 9. (A) Schematic of the OCE system. The system employs a swept source OCT unit and a PZT excitation unit. The PZT unit induces displacements within the sample, and the OCT unit detects the signals and visualizes the longitudinal shear wave propagation. Reproduced with permission from [330]. Copyright 2017, AIP Publishing. (B) A representation of waves generated by the PZT unit: transverse shear waves, longitudinal shear waves, compressional waves, and Rayleigh waves. Reproduced with permission from [331]. Copyright 2017, AIP Publishing. (C) Schematic of the acoustic micro-tapping phase sensitive OCT imaging system developed by Ambrozinski et al. Reproduced with permission from [332]. Copyright 2016, Nature Research.
As introduced above, noncontact shear wave imaging optical coherence tomography (SWI-OCT) system has been developed using a focused air-puff device for localized tissue deformation [333]. The non-contact mechanical excitation in a sample could be performed with a PZT transducer that was specially designed to launch an US beam through air that was focused onto the air-medium interface. The reflection of the beam at this interface could produce significant acoustic radiation force toward the sample medium. This induced a transient displacement at the surface, including shear waves. The large difference in acoustic impedances of air and soft tissues could increase the efficiency of the acoustic energy conversion even to the extent of one hundred percent. Ambrozinski et al. [332] developed a non-invasive system that needed transient displacements to be only about 1 μm, and the acoustic pressure only a few kPa, which was within safety limits for clinical applications. This acoustic micro-tapping method had enabled 4D imaging of tissue stiffness by employing a focused air-coupled US to induce mechanical deformations at the boundary of a tissue [332]. A schematic representation of their system is shown in Figure 9C. In here, the cornea surface was aligned at the transducer focus and the US radiation push was sent through with a repetition period of 3 ms. The driving signal was having a bandwidth range of 0.95–1.05 MHz. The measured pressure amplitude at the transducer focus was about 7 kPa.
Wang et al. performed a quantitative biomechanical characterization of cardiac muscles and corneas using a noncontact SWI-OCT system [334, 335]. Shear waves had a frequency range of 0–2.5 kHz. This method employed a multi-wave imaging technique, where shear wave measurements in the tissue enabled mapping of the mechanical contrast in elastograms, and the OCT unit enabled improving the imaging resolution from a millimeter scale to a micron scale [334]. The system was capable of simultaneously providing structural images with depth wise maps of the tissue stiffness [335]. Recently, a confocal air-coupled US probe could also be co-focused with a phase-sensitive OCT system to generate elastic waves up to a 4 kHz frequency for quantitative elastography [336]. These noncontact excitation methods have found wide applications in ophthalmology and dermatology [337–339].
Spatial resolution in dynamic shear wave based OCE is governed by temporal and spatial characteristics of mechanical waves rather than optical waves. Hence, the mechanical resolution in dynamic OCE is different from the usual optical resolution of OCT systems [57]. Spatial resolution ideally should match the spatial resolution of the detection system, however, propagating mechanical waves undergo mode conversions at tissue interfaces causing artifacts in the elasticity image. The geometry of the tissue interface and its elasticity contrast can produce complex propagating fields near the tissue boundary affecting both the spatial resolution and contrast of the final reconstructed image [57].
Recent dynamic OCE systems provided elasticity information from local group velocity measurements [321, 330, 339], however, the complex geometry of bounded tissues like the cornea may not reflect a simple relationship between group velocity and elasticity [338]. Dynamic OCE has been successfully utilized in elasticity mapping of the cornea using noncontact excitation methods based on air-puffs and acoustic micro tapping [332, 333, 340–342]. Inversion of moduli from experimental data, especially in the case of bounded and anisotropic tissues such as cornea, is a challenging and complicated process in dynamic elastography. Recently, a nearly-incompressible transverse isotropic (NITI) model addressed this challenge and characterized corneal biomechanics while accounting for corneal microstructure and anisotropy, and presented a more accurate model for cornea shear moduli computation [337]. Viscosity assessment in shear wave OCE is in its early phase of development. Proposed methods used shear wave frequency dispersion [343–346], storage and loss moduli using a rheological model [347], and the elastic wave attenuation [345].
A trade-off in OCE is its reduced depth of field while evolving for higher resolution measurements due to the requirement of higher numerical aperture for such systems. On the other hand, the ability to measure and record depth scans with a single spectral acquisition can be used as an advantageous feature to enable phase-sensitive displacement measurements. Of course, the tissue penetration attained with OCE, although sufficient for numerous applications, is not comparable to ultrasound or MRI elastography methods. Song et al. implemented a beam-steering US as a wave source for shear wave optical coherence elastography of retinal and choroidal tissues within a porcine eyes ball ex vivo. Shear wave propagation imaged on a porcine retina by their system is shown in Figure 10 [323].
FIGURE 10. Images of shear wave propagation in a porcine retina generated with a single acoustic radiation force ultrasound push. The images are taken snapshots at (A) 0.2, (B) 0.25, (C) 0.3, and (D) 0.35 ms after the push. Lateral scale: 0.5 mm/div; axial (depth) scale: 0.25 mm/div. Reproduced with permission from [323]. Copyright 2015, OSA Publishing.
Photoacoustic elastography (PAE) research is rapidly growing due to its potential and promising features of clinical interest [348–350]. PAE can exhibit a mechanical contrast in biological tissues while also providing high spatial resolution images and an excellent penetration depth compared to commercially available optical imaging modalities [351]. It has the promise to provide great scalability, ranging from cellular levels to entire body with multiple resolution levels. Recent studies have demonstrated recovery of mechanical properties of biological tissues using PAE [352–355]. Several studies demonstrated computation of elastic properties of soft tissues [354, 356–359]. Nevertheless, clinical translation of PAE is still far way for research studies to accomplish, the development of the PAE technology has shown the potential to be used in life threatening diseases, such as breast and prostate cancers, and brain tumors [350, 360]. Photoacoustic elastography can be used for mapping elastic properties of diseased tissues with highly vascularized structures, such as carcinoma and glioblastoma [351]. Most PAE studies have focused on qualitative imaging and quantitative PAE is still a challenge. Moreover, PAE using propagating shear waves still need to be clearly addressed. A recent study by Wang et al. did develop a PA viscoelasticity technique for quantitative imaging of liver cirrhosis based on a PA shear wave model [359]. This viscoelasticity imaging model was inspired by the acoustic radiation force impulse (ARFI) technique (see Ultrasound Shear Wave Elastography). In this model, a laser beam was focused into a tissue that resulted in the tissue thermal expansion and a PA pressure field was generated. The pressure field induced a localized ultrasound impulse similar to ARFI, and subsequently a tissue displacement field could be observed. The study assumed that these forward propagating PA waves could be modeled using shear wave equations.
As a summary of methods addressed in this review, Table 4 compares photoacoustic elastography with other elastography modalities in term of performance.
Many notable ultrasound elastography methods have been translated into clinical applications, and adopted by clinicians for diagnosis of several organs, as introduced in Applications. A limitation lay in the depth of SW penetration due to attenuation, especially for the diagnosis of liver fibrosis and steatosis, which could result in unsuccessful measurements with large patients or patients with ascites [31]. Note that SW attenuation is a concern for any shear wave elastography method. Consequently, measurements on superficial regions showed a higher success rate, such as the diagnosis of breast lesions and tumors. Another limitation lies in the assumption often used in shear wave elastography; notably considering the tissue as isotropic and homogeneous. Certain tissues such as muscles or tendons do not respect the isotropy hypothesis and are rather considered as anisotropic or transverse isotropic media. To answer this problem, teams have developed stiffness tensors for assessing shear wave propagation and for evaluating mechanical properties in several directions [161, 361], even in three dimensions [162]. Bones, brains, or lungs are parts of the body that can be considered porous and for which the assumption of homogeneity is limited. Poroelasticity based on the estimation of the temporal response of tissues to compression [15, 362–364] is a technique derived from strain elastography. Although a little off topic because it does not use shear waves, its development in the characterization of tumors is promising [364, 365]. Other applications, such as the characterization of muscles, Achilles tendons, the cardiovascular system, and lymph nodes [110, 366–368], have shown good results that reflected the difference between normal and abnormal tissues.
At present, the measurement of the tissue elasticity has dominated the field, and technologies, such as the transient elastography, SSI, ARFI, and comb push ARFs are available on clinical scanners [67, 110, 144, 369–376]. In fact, most manufacturers have today a shear wave elastography package for clinical use, and the spatial resolution of those elastography systems are as good as ultrasound B-mode imaging. However for measurements on more complex tissues, such as anisotropic, layered, or near close to interface results should be taken with caution. The characterization of human tissue viscoelasticity without using a rheological model, and studies on viscosity, anisotropy, porosity, and nonlinearity, are important topics in development [23, 51, 377–383]. While those innovations have not yet received clinical approval, further validation with robustness and reproducibility results should allow manufacturers to consider these biomarkers. New applications of SW ultrasound elastography are also in developments. Studies pointed out that the measurement of the elastic property could be used to monitor thermal ablation [374, 384, 385].
Magnetic resonance elastography has been proven successful and robust in a broad range of applications, from clinical diagnosis of liver diseases [386] to brain [280], and breast [387] pathologies. However, generalization of MRE in clinics is impacted by the scan time required for the acquisition of complete data sets necessary for accurate reconstruction. Clinical sequences currently acquire one component of the motion field at one frequency, and rely on a 1D direct inversion assuming isotropy, incompressibility, local homogeneity, and pure elasticity. This package is fast and guaranties results. Mechanical solicitation of the tissue under multi-frequency loads may be a first step into the characterization of tissue viscoelastic responses by providing valuable information on the frequency dependent biomechanics in pathological cases [201]. Motion encoding may also reach a limit at high frequencies in the case of oscillating gradients due to peripheral nerve stimulation [388]. The actuation regime also determines the physics to consider for inferring mechanical parameters from experimental datasets [184]. An elegant avenue circumventing the use of external actuators is intrinsic actuation from low frequency heart beats along with poroelastic modeling of the tissue [211]. Along the same lines, natural vibrations in the brain have been exploited using the novel passive elastography technique based on time reversal concepts to quantify the vibration wavelengths, assumed to be related to brain stiffness [210]. A potential solution to shear wave attenuation in soft viscoelastic tissues may be to place motion sources closer to the region of interest. This may be achieved using ultrasound transducers generating an acoustic radiation force impulse (ARFI) along with MRE acquisition [207, 389]. Alternatively, a promising approach that may prove feasible with clinical MRI scanner is Lorentz force elastography for in situ actuation at different frequencies [390, 391]. An elasticity reconstruction obtained using a Lorentz force and a clinical MRI scanner is displayed in Figure 11, in the case of a gel phantom.
FIGURE 11. Preliminary results of Lorentz force MRE in gel phantoms. (A) Phase map due to the propagation of displacements induced by a Lorentz force. (B) Young’s modulus map of a heterogeneous phantom constructed from the Lorentz force induced motion.
On a similar note, localized motion generation using ARFI has been employed with MRE to measure elasticity changes during high intensity focused ultrasound ablations in ex-vivo porcine muscle samples [392]. Such monitoring requires sufficient displacement amplitude [393]. Assessment of stiffness changes due to ablation or percutaneous procedures has been performed both during [394, 395], and separately before and after ablation [396], all cases reporting a stiffness increase after the intervention.
On the acquisition side, significant amount of effort has been put into MR sequence developments to reduce scan time while preserving 3D motion encoding and signal amplitude. Although equipping an MR sequence with bipolar magnetic field gradients prevails, recent implementations took advantage of MR sequence inherent gradients to encode motion, thus keeping the timing shorter than conventional use of MEG [397]. As in any MRI scan, artefacts may occur due to patient motion. Sequence dependent artefacts include and are not restricted to signal loss in GRE sequences due to irregular geometries, and associated magnetic field inhomogeneity and distortion in echo-planar sequences. Specific to MRE, phase wrapping occurs when motion cannot be encoded in the [-π, π] range leading to phase jumps within this range. Three solutions appear and consist in either decreasing the gradient sensitivity, decreasing the motion generator strength, or using a phase unwrapping algorithm. Whilst a weakness of MRE may be viewed as a lack of universal protocol applicable to any organ, its strength resides in the capacity of providing usable data in multiple cases owing to various hardware and MR sequences, and in the availability of physical models to process produced experimental data for stiffness estimation. This technology is still mainly used in the context of clinical research, and additional validations might be required for robust viscosity, porosity, and anisotropy assessments.
Although the optical microelastography technique has an unprecedented high temporal resolution with the capability of producing elasticity images, its spatial resolution is not as good as other rheology methods, such as atomic force microscopy. The spatial resolution of this technique is currently limited to 10 μm, approximately, but it could be improved to resolutions close to optical microscopy (≈1 µm) by utilizing a higher stimulus frequency and by improvements in the reconstruction process. This technique is currently limited to elasticity measurements but viscosity might become available through FEM modeling, or by considering shear wave attenuation [50].
More improvements need to be done beyond solving limitations mentioned above. The mechanical behavior of the cell should be further investigated in a range of frequencies. One remaining difficulty is the absence of good standard rheology method to validate the microelastography technique at frequencies in the kHz range. This makes it difficult to compare results from different techniques on a similar cell type. Other reasons for the lack of concordance of results, obtained with different cell elasticity technologies, were recently addressed [398] and apply to the reported microelastography method. Recent developments might allow using mechanically stable microgel bead to compare different cell elasticity methods [399], which may reduce variability when performing such comparisons at different (non-overlapping) frequencies.
Also, to make these techniques more applicable and practical for biologists, and to promote using cell mechanics as a biomarker, improvements are required for the technique to be automatic, high throughput while being robust, accurate, and sensitive with high time and space resolutions. This might be done by coupling the optical microelastography technique with other methods, such as microfluidics with a high throughput [400, 401].
Higher resolution OCE may face computational challenges due to the fact that the speckle decorrelation length scales with the speckle size [402, 403]. This would reduce the maximum displacement that can be measured between frames. Many studies have made progress to further demonstrate substantial improvements in resolution [404, 405]. High resolution OCE systems can be used to assess mechanical properties of cells and a few preliminary studies have showed this potential [404, 406]. These are relatively new developments and the hope remains that OCE would be able to characterize cell aggregates [305], with penetration depth going up to several hundred microns, whilst maintaining a sub-cellular scale resolution.
There has been several studies on elastogram image reconstruction in OCE by inverse problem approaches [407]. Sridhar et al. [408] used an inverse problem approach to understand how stromal tissues affect the broad spectrum of the viscoelastic response [409], by minimizing the mean squared error between computed and measured displacements. Different methods to constrain the optimization algorithm has been summarized by [410], in the context of ultrasound strain elastography. Basic principles are also applicable to OCE. However, one challenge that often prevails in these scenarios is the optimization of the regularization parameter for efficient reconstruction, especially in the context of in vivo experiments.
Another area of interest representing some challenges is the quantitative assessment of tissue viscoelastic properties with OCE. This research is still in its early years acknowledging the fact that the viscosity is not accounted for in the simple approach [411], but hopefully with the development of new models, OCE would be able to convert elastic wave speed and attenuation into quantitative values for clinical diagnosis based on tissue viscoelasticity.
Despite several advancements, very few studies have been done in the area of validation of performance. This would require phantoms that are developed for optics rather than mechanics [305]. Rigorous assessment of sensitivity and specificity for diagnostic applications would be required for translating the method to the clinics.
Photoacoustic elastography imaging is relatively a new development and it still needs to overcome many challenges. The PA signal contrast detected by ultrasound transducers is low due to lower variation in the tissue elasticity distribution in comparison to the optical absorption coefficient. This can potentially be overcome by additionally employing an ultrasound modulation of the laser pulse to provide external mechanical simulation of the tissue [412]. In addition, elasticity can also be estimated from the resonance frequency of the tissue material observed in the measurement of PA signal strength against the operating frequency of the external (ultrasound) mechanical stimulation [412, 413]. Another challenge is the development of quantitative PAE imaging systems, as the first few studies in the field reported only qualitative assessment of elastic properties. However, Hai et al. were the first to develop a quantitative PAE system [414]. It would also be interesting to detect the contrast in the PA signal due to elastic property variation separately from that of other parameters (including the optical absorption coefficient). Grasland-Mongrain et al. generated shear waves in soft tissues in ablative and thermoelastic regimes with a 532 nm Nd:YAG laser [415]. However, it remained a challenge to keep the laser beam energy within safety limits for use in biomedical applications. This can potentially be overcome by use of other types of laser or by emission of the high energy laser beam onto a protective absorbing layer, such as a black sheet, that can cover the tissue externally. In conclusion, PAE is still in the beginning phase of its development compared to ultrasound, MRI or OCT elastography, and there definitely remains scope for many promising improvements to increase its potential for various imaging applications.
HL supervised and structured the review, wrote the introduction and the conclusion, contributed to the section on the generation and detection of shear waves, reviewed, and edited the final document. GF co-supervised and structured the review, wrote the magnetic resonance elastography and the general concepts on shear wave elastography sections, reviewed, and edited the final document. MB wrote the optical coherence elastography imaging and the photoacoustic elastography sections. ZQ wrote the characterization of tissue viscoelasticity section, and contributed to the generation and detection of shear waves section. SG wrote the cellular shear wave elastography section. LY wrote the viscoelasticity reconstruction section and applications in ultrasound. GB wrote the clinical applications of ultrasound shear wave elastography section. IR contributed to the section on ultrasound shear wave elastography. GC received the invitation to provide this review, contributed to the general supervision of all co-authors, did reviewing of all sections of this document for final approval, and provided editing and funding.
Financial support for this research on shear wave elastography was provided by the Canadian Institutes of Health Research (#273738, #301520, #142334, #95288), Natural Sciences and Engineering Research Council of Canada (#503381, #365656), Fonds de Recherche du Québec (#263591), Fonds de Recherche du Québec sur la Nature et les Technologies (#174387), Cancer Research Society (#19075), and Quebec Bioimaging Network (Strategic Program).
The authors declare that the research was conducted in the absence of any commercial or financial relationships that could be construed as a potential conflict of interest.
1. Sarvazyan AP, Skovoroda AR, Emelianov SY, Fowlkes JB, Pipe JG, Adler RS, et al. Biophysical Bases of Elasticity Imaging. Acoust Imaging (1995) 21:223–40. doi:10.1007/978-1-4615-1943-0_23
2. Zhang M, Castaneda B, Wu Z, Nigwekar P, Joseph JV, Rubens DJ, et al. Congruence of Imaging Estimators and Mechanical Measurements of Viscoelastic Properties of Soft Tissues. Ultrasound Med Biol (2007) 33(10):1617–31. doi:10.1016/j.ultrasmedbio.2007.04.012
3. Shahmirzadi D, Bruck HA, Hsieh AH. Measurement of Mechanical Properties of Soft Tissues In Vitro under Controlled Tissue Hydration. Exp Mech (2012) 53(3):405–14. doi:10.1007/s11340-012-9644-y
4. Estermann S-J, Pahr DH, Reisinger A. Hyperelastic and Viscoelastic Characterization of Hepatic Tissue under Uniaxial Tension in Time and Frequency Domain. J Mech Behav Biomed Mater (2020) 112:104038. doi:10.1016/j.jmbbm.2020.104038
5. Vappou J, Breton E, Choquet P, Goetz C, Willinger R, Constantinesco A. Magnetic Resonance Elastography Compared with Rotational Rheometry for In Vitro Brain Tissue Viscoelasticity Measurement. Magn Reson Mater Phy (2007) 20(5):273–8. doi:10.1007/s10334-007-0098-7
6. Klatt D, Friedrich C, Korth Y, Vogt R, Braun J, Sack I. Viscoelastic Properties of Liver Measured by Oscillatory Rheometry and Multifrequency Magnetic Resonance Elastography. Biorheology (2010) 47(2):133–41. doi:10.3233/bir-2010-0565
7. Chatelin S, Oudry J, Périchon N, Sandrin L, Allemann P, Soler L, et al. In Vivo liver Tissue Mechanical Properties by Transient Elastography: Comparison with Dynamic Mechanical Analysis. Biorheology (2011) 48(2):75–88. doi:10.3233/bir-2011-0584
8. Dittmann F, Hirsch S, Tzschätzsch H, Guo J, Braun J, Sack I. In Vivo wideband Multifrequency MR Elastography of the Human Brain and Liver. Magn Reson Med (2016) 76(4):1116–26. doi:10.1002/mrm.26006
9. Lin H, Shen Y, Chen X, Zhu Y, Zheng Y, Zhang X, et al. Viscoelastic Properties of normal Rat Liver Measured by Ultrasound Elastography: Comparison with Oscillatory Rheometry. Biorheology (2016) 53(5-6):193–207. doi:10.3233/BIR-16091
10. Ormachea J, Parker KJ. Elastography Imaging: the 30 Year Perspective. Phys Med Biol (2020) 65:24TR06. doi:10.1088/1361-6560/abca00
11.D Royer, E. Dieulesaint, and SSB Media, editors. Elastic Waves in Solids I: Free and Guided Propagation. Springer Science & Business Media (1999).
12. Skovoroda AR, Emelianov SY, Lubinski MA, Sarvazyan AP, O'Donnell M. Theoretical Analysis and Verification of Ultrasound Displacement and Strain Imaging. IEEE Trans Ultrason Ferroelect., Freq Contr (1994) 41(3):302–13. doi:10.1109/58.285463
13. Sarvazyan AP, Rudenko OV, Swanson SD, Fowlkes JB, Emelianov SY. Shear Wave Elasticity Imaging: a New Ultrasonic Technology of Medical Diagnostics. Ultrasound Med Biol (1998) 24(9):1419–35. doi:10.1016/s0301-5629(98)00110-0
14. Özkaya N, Leger D, Goldsheyder D, Nordin M. Fundamentals of Biomechanics: Equilibrium, Motion, and Deformation. Springer (2016).
15. Konofagou EE, Harrigan TP, Ophir J, Krouskop TA. Poroelastography: Imaging the Poroelastic Properties of Tissues. Ultrasound Med Biol (2001) 27(10):1387–97. doi:10.1016/s0301-5629(01)00433-1
16. Biot MA. Theory of Propagation of Elastic Waves in a Fluid-Saturated Porous Solid. I. Low-Frequency Range. The J Acoust Soc America (1956) 28(2):168–78. doi:10.1121/1.1908239
17. Biot MA. Theory of Propagation of Elastic Waves in a Fluid-Saturated Porous Solid. II. Higher Frequency Range. J Acoust Soc America (1956) 28(2):179–91. doi:10.1121/1.1908241
18. Cheng AHD, Badmus T, Beskos DE. Integral Equation for Dynamic Poroelasticity in Frequency Domain with BEM Solution. J Eng Mech (1991) 117(5):1136–57. doi:10.1061/(asce)0733-9399(1991)117:5(1136)
19. Hirsch S, Braun J, Sack I. Magnetic Resonance Elastography - Physical Background and Medical Applications. Wiley VCH (2017).
20. Romano A, Scheel M, Hirsch S, Braun J, Sack I. In Vivo waveguide Elastography of white Matter Tracts in the Human Brain. Magn Reson Med (2012) 68(5):1410–22. doi:10.1002/mrm.24141
21. Meyers MA, Chawla KK. Mechanical Behavior of Materials. New York: Cambridge University Press (2008). doi:10.1017/cbo9780511810947
22. Schiessel H, Metzler R, Blumen A, Nonnenmacher TF. Generalized Viscoelastic Models: Their Fractional Equations with Solutions. J Phys A: Math Gen (1995) 28(23):6567–84. doi:10.1088/0305-4470/28/23/012
23. Rus G, Faris IH, Torres J, Callejas A, Melchor J. Why Are Viscosity and Nonlinearity Bound to Make an Impact in Clinical Elastographic Diagnosis? Sensors (2020) 20(8):2379. doi:10.3390/s20082379
24. Qiang B, Brigham JC, Aristizabal S, Greenleaf JF, Zhang X, Urban MW. Modeling Transversely Isotropic, Viscoelastic, Incompressible Tissue-like Materials with Application in Ultrasound Shear Wave Elastography. Phys Med Biol (2015) 60(3):1289–306. doi:10.1088/0031-9155/60/3/1289
25. Greenwood MS, Bamberger JA. Measurement of Viscosity and Shear Wave Velocity of a Liquid or Slurry for On-Line Process Control. Ultrasonics (2002) 39(9):623–30. doi:10.1016/s0041-624x(02)00372-4
26. Madsen EL, Sathoff HJ, Zagzebski JA. Ultrasonic Shear Wave Properties of Soft Tissues and Tissuelike Materials. J Acoust Soc America (1983) 74(5):1346–55. doi:10.1121/1.390158
27. Kelly P. Solid Mechanics Part I: An Introduction to Solid Mechanics. Solid mechanics lecture notes (2013).
28. Schmitt C, Hadj Henni A, Cloutier G. Characterization of Blood Clot Viscoelasticity by Dynamic Ultrasound Elastography and Modeling of the Rheological Behavior. J Biomech (2011) 44(4):622–9. doi:10.1016/j.jbiomech.2010.11.015
29. Serra-Aguila A, Puigoriol-Forcada JM, Reyes G, Menacho J. Viscoelastic Models Revisited: Characteristics and Interconversion Formulas for Generalized Kelvin-Voigt and Maxwell Models. Acta Mech Sin (2019) 35(6):1191–209. doi:10.1007/s10409-019-00895-6
30. Greenleaf JF, Fatemi M, Insana M. Selected Methods for Imaging Elastic Properties of Biological Tissues. Annu Rev Biomed Eng (2003) 5:57–78. doi:10.1146/annurev.bioeng.5.040202.121623
31. Parker KJ, Doyley MM, Rubens DJ. Corrigendum: Imaging the Elastic Properties of Tissue: the 20 Year Perspective. Phys Med Biol (2012) 57(16):5359–60. doi:10.1088/0031-9155/57/16/5359
32. Chen S, Fatemi M, Greenleaf JF. Remote Measurement of Material Properties from Radiation Force Induced Vibration of an Embedded Sphere. J Acoust Soc America (2002) 112(3):884–9. doi:10.1121/1.1501276
33. Gao L, Parker KJ, Lerner RM, Levinson SF. Imaging of the Elastic Properties of Tissue-A Review. Ultrasound Med Biol (1996) 22(8):959–77. doi:10.1016/s0301-5629(96)00120-2
34. Li SP, Zhao G, Chen HY. The Relationship between Steady Shear Viscosity and Complex Viscosity. J dispersion Sci Technol (2005) 26(4):415–9. doi:10.1081/dis-200054555
36. Ophir J, Alam SK, Garra B, Kallel F, Konofagou E, Krouskop T, et al. Elastography: Ultrasonic Estimation and Imaging of the Elastic Properties of Tissues. Proc Inst Mech Eng H (1999) 213(3):203–33. doi:10.1243/0954411991534933
37. Lewandowski R, Chorążyczewski B. Identification of the Parameters of the Kelvin–Voigt and the Maxwell Fractional Models, Used to Modeling of Viscoelastic Dampers. Comput Structures (2010) 88(1-2):1–17. doi:10.1016/j.compstruc.2009.09.001
38. Cheeke JDN Fundamentals and Applications of Ultrasonic Waves. CRC Press (2017). doi:10.1201/b12260
39. Lerner RM, Parker KJ, Holen J, Gramiak R, Waag RC. Sono-elasticity: Medical Elasticity Images Derived from Ultrasound Signals in Mechanically Vibrated Targets. Acoust Imaging (1988) 317–27. doi:10.1007/978-1-4613-0725-9_31
40. Yamakoshi Y, Sato J, Sato T. Ultrasonic Imaging of Internal Vibration of Soft Tissue under Forced Vibration. IEEE Trans Ultrason Ferroelect., Freq Contr (1990) 37(2):45–53. doi:10.1109/58.46969
41. Catheline S, Wu F, Fink M. A Solution to Diffraction Biases in Sonoelasticity: the Acoustic Impulse Technique. J Acoust Soc America (1999) 105(5):2941–50. doi:10.1121/1.426907
42. Wu Z, Taylor LS, Rubens DJ, Parker KJ. Sonoelastographic Imaging of Interference Patterns for Estimation of the Shear Velocity of Homogeneous Biomaterials. Phys Med Biol (2004) 49(6):911–22. doi:10.1088/0031-9155/49/6/003
44. Fatemi M, Greenleaf JF. Ultrasound-stimulated Vibro-Acoustic Spectrography. Science (1998) 280(5360):82–5. doi:10.1126/science.280.5360.82
45. Chen S, Fatemi M, Greenleaf JF. Quantifying Elasticity and Viscosity from Measurement of Shear Wave Speed Dispersion. J Acoust Soc America (2004) 115(6):2781–5. doi:10.1121/1.1739480
46. Chen S, Urban MW, Pislaru C, Kinnick R, Zheng Y, Yao A, et al. Shearwave Dispersion Ultrasound Vibrometry (SDUV) for Measuring Tissue Elasticity and Viscosity. IEEE Trans Ultrason Ferroelectr Freq Control (2009) 56(1):55–62. doi:10.1109/TUFFC.2009.1005
47. Bercoff J, Tanter M, Fink M. Supersonic Shear Imaging: A New Technique for Soft Tissue Elasticity Mapping. IEEE Trans Ultrason Ferroelect., Freq Contr (2004) 51(4):396–409. doi:10.1109/tuffc.2004.1295425
48. McAleavey SA, Menon M, Orszulak J. Shear-modulus Estimation by Application of Spatially-Modulated Impulsive Acoustic Radiation Force. Ultrason Imaging (2007) 29(2):87–104. doi:10.1177/016173460702900202
49. Song P, Zhao H, Manduca A, Urban MW, Greenleaf JF, Chen S. Comb-push Ultrasound Shear Elastography (CUSE): a Novel Method for Two-Dimensional Shear Elasticity Imaging of Soft Tissues. IEEE Trans Med Imaging (2012) 31(9):1821–32. doi:10.1109/tmi.2012.2205586
50. Kazemirad S, Bernard S, Hybois S, Tang A, Cloutier G. Ultrasound Shear Wave Viscoelastography: Model-independent Quantification of the Complex Shear Modulus. IEEE Trans Ultrason Ferroelect., Freq Contr (2016) 63(9):1399–408. doi:10.1109/tuffc.2016.2583785
51. Bhatt M, Moussu MAC, Chayer B, Destrempes F, Gesnik M, Allard L, et al. Reconstruction of Viscosity Maps in Ultrasound Shear Wave Elastography. IEEE Trans Ultrason Ferroelect., Freq Contr (2019) 66(6):1065–78. doi:10.1109/tuffc.2019.2908550
52. Sandrin L, Catheline S, Fink M. Transient Elastography in Biological Tissues. J Acoust Soc America (1999) 105(2):1014–5. doi:10.1121/1.425852
53. Sandrin L, Catheline S, Tanter M, Hennequin X, Fink M. Time-resolved Pulsed Elastography with Ultrafast Ultrasonic Imaging. Ultrason Imaging (1999) 21(4):259–72. doi:10.1177/016173469902100402
54. Zemzemi C, Zorgani A, Daunizeau L, Belabhar S, Souchon R, Catheline S. Super-resolution Limit of Shear-Wave Elastography. EPL (Europhysics Letters) (2020) 129(3). doi:10.1209/0295-5075/129/34002
55. Deffieux T, Gennisson J-L, Bercoff J, Tanter M. On the Effects of Reflected Waves in Transient Shear Wave Elastography. IEEE Trans Ultrason Ferroelect., Freq Contr (2011) 58(10):2032–5. doi:10.1109/tuffc.2011.2052
56. Lipman SL, Rouze NC, Palmeri ML, Nightingale KR. Evaluating the Improvement in Shear Wave Speed Image Quality Using Multidimensional Directional Filters in the Presence of Reflection Artifacts. IEEE Trans Ultrason Ferroelect., Freq Contr (2016) 63(8):1049–63. doi:10.1109/tuffc.2016.2558662
57. Kirby MA, Zhou K, Pitre JJ, Gao L, Li DS, Pelivanov IM, et al. Spatial Resolution in Dynamic Optical Coherence Elastography. J Biomed Opt (2019) 24(9):1–16. doi:10.1117/1.jbo.24.9.096006
58. Chen X, Shen Y, Zheng Y, Lin H, Guo Y, Zhu Y, et al. Quantification of Liver Viscoelasticity with Acoustic Radiation Force: a Study of Hepatic Fibrosis in a Rat Model. Ultrasound Med Biol (2013) 39(11):2091–102. doi:10.1016/j.ultrasmedbio.2013.05.020
59. Gennisson J-L, Deffieux T, Fink M, Tanter M. Ultrasound Elastography: Principles and Techniques. Diagn Interv Imaging (2013) 94(5):487–95. doi:10.1016/j.diii.2013.01.022
60. Nightingale K, McAleavey S, Trahey G. Shear-wave Generation Using Acoustic Radiation Force: In Vivo and Ex Vivo Results. Ultrasound Med Biol (2003) 29(12):1715–23. doi:10.1016/j.ultrasmedbio.2003.08.008
61. Tang A, Cloutier G, Szeverenyi NM, Sirlin CB. Ultrasound Elastography and MR Elastography for Assessing Liver Fibrosis: Part 1, Principles and Techniques. Am J Roentgenology (2015) 205(1):22–32. doi:10.2214/ajr.15.14552
62. Tang A, Cloutier G, Szeverenyi NM, Sirlin CB. Ultrasound Elastography and MR Elastography for Assessing Liver Fibrosis: Part 2, Diagnostic Performance, Confounders, and Future Directions. Am J Roentgenology (2015) 205(1):33–40. doi:10.2214/ajr.15.14553
63. Sandrin L, Fourquet B, Hasquenoph J-M, Yon S, Fournier C, Mal F, et al. Transient Elastography: a New Noninvasive Method for Assessment of Hepatic Fibrosis. Ultrasound Med Biol (2003) 29(12):1705–13. doi:10.1016/j.ultrasmedbio.2003.07.001
64. Arena U, Vizzutti F, Corti G, Ambu S, Stasi C, Bresci S, et al. Acute Viral Hepatitis Increases Liver Stiffness Values Measured by Transient Elastography. Hepatology (2007) 47(2):380–4. doi:10.1002/hep.22007
65. Castéra L, Bernard P-H, Le Bail B, Foucher J, Trimoulet P, Merrouche W, et al. Transient Elastography and Biomarkers for Liver Fibrosis Assessment and Follow-Up of Inactive Hepatitis B Carriers. Aliment Pharmacol Ther (2011) 33(4):455–65. doi:10.1111/j.1365-2036.2010.04547.x
66. Gennisson J-L, Lerouge S, Cloutier G. Assessment by Transient Elastography of the Viscoelastic Properties of Blood during Clotting. Ultrasound Med Biol (2006) 32(10):1529–37. doi:10.1016/j.ultrasmedbio.2006.06.008
67. Castéra L, Vergniol J, Foucher J, Le Bail B, Chanteloup E, Haaser M, et al. Prospective Comparison of Transient Elastography, Fibrotest, APRI, and Liver Biopsy for the Assessment of Fibrosis in Chronic Hepatitis C. Gastroenterology (2005) 128(2):343–50. doi:10.1053/j.gastro.2004.11.018
68. Frulio N, Trillaud H. Ultrasound Elastography in Liver. Diagn Interv Imaging (2013) 94(5):515–34. doi:10.1016/j.diii.2013.02.005
69. Gerber L, Kasper D, Fitting D, Knop V, Vermehren A, Sprinzl K, et al. Assessment of Liver Fibrosis with 2-D Shear Wave Elastography in Comparison to Transient Elastography and Acoustic Radiation Force Impulse Imaging in Patients with Chronic Liver Disease. Ultrasound Med Biol (2015) 41(9):2350–9. doi:10.1016/j.ultrasmedbio.2015.04.014
70. Nightingale K. Acoustic Radiation Force Impulse (ARFI) Imaging: A Review. Curr Med Imaging Rev (2011) 7(4):328–39. doi:10.2174/157340511798038657
71. Cassinotto C, Boursier J, de Lédinghen V, Lebigot J, Lapuyade B, Cales P, et al. Liver Stiffness in Nonalcoholic Fatty Liver Disease: A Comparison of Supersonic Shear Imaging, FibroScan, and ARFI with Liver Biopsy. Hepatology (2016) 63(6):1817–27. doi:10.1002/hep.28394
72. Tanter M, Bercoff J, Athanasiou A, Deffieux T, Gennisson J-L, Montaldo G, et al. Quantitative Assessment of Breast Lesion Viscoelasticity: Initial Clinical Results Using Supersonic Shear Imaging. Ultrasound Med Biol (2008) 34(9):1373–86. doi:10.1016/j.ultrasmedbio.2008.02.002
73. Lee MS, Bae JM, Joo SK, Woo H, Lee DH, Jung YJ, et al. Prospective Comparison Among Transient Elastography, Supersonic Shear Imaging, and ARFI Imaging for Predicting Fibrosis in Nonalcoholic Fatty Liver Disease. PLoS One (2017) 12(11):e0188321. doi:10.1371/journal.pone.0188321
74. Amador C, Urban MW, Chen S, Greenleaf JF. Loss tangent and Complex Modulus Estimated by Acoustic Radiation Force Creep and Shear Wave Dispersion. Phys Med Biol (2012) 57(5):1263–82. doi:10.1088/0031-9155/57/5/1263
75. Rouze NC, Palmeri ML, Nightingale KR. An Analytic, Fourier Domain Description of Shear Wave Propagation in a Viscoelastic Medium Using Asymmetric Gaussian Sources. J Acoust Soc America (2015) 138(2):1012–22. doi:10.1121/1.4927492
76. Chen S, Sanchez W, Callstrom MR, Gorman B, Lewis JT, Sanderson SO, et al. Assessment of Liver Viscoelasticity by Using Shear Waves Induced by Ultrasound Radiation Force. Radiology (2013) 266(3):964–70. doi:10.1148/radiol.12120837
77. Muller M, Gennisson J-L, Deffieux T, Tanter M, Fink M. Quantitative Viscoelasticity Mapping of Human Liver Using Supersonic Shear Imaging: Preliminary In Vivo Feasability Study. Ultrasound Med Biol (2009) 35(2):219–29. doi:10.1016/j.ultrasmedbio.2008.08.018
78. Kijanka P, Urban MW. Local Phase Velocity Based Imaging of Viscoelastic Phantoms and Tissues. IEEE Trans Ultrason Ferroelectr Freq Control (2021) 68:389. doi:10.1109/TUFFC.2020.2968147
79. Orescanin M, Yue Wang Y, Insana MF. 3-D FDTD Simulation of Shear Waves for Evaluation of Complex Modulus Imaging. IEEE Trans Ultrason Ferroelect., Freq Contr (2011) 58(2):389–98. doi:10.1109/tuffc.2011.1816
80. Montagnon E, Hadj-Henni A, Schmitt C, Cloutier G. Rheological Assessment of a Polymeric Spherical Structure Using a Three-Dimensional Shear Wave Scattering Model in Dynamic Spectroscopy Elastography. IEEE Trans Ultrason Ferroelect., Freq Contr (2014) 61(2):277–87. doi:10.1109/tuffc.2014.6722613
81. Bhatt M, Montagnon E, Destrempes F, Chayer B, Kazemirad S, Cloutier G. Acoustic Radiation Force Induced Resonance Elastography of Coagulating Blood: Theoretical Viscoelasticity Modeling and Ex-Vivo Experimentation. Phys Med Biol (2018) 63(6):065018. doi:10.1088/1361-6560/aab46a
82. Bernard S, Cloutier G. Forward and Inverse Viscoelastic Wave Scattering by Irregular Inclusions for Shear Wave Elastography. J Acoust Soc America (2017) 142(4):2346–64. doi:10.1121/1.5007729
83. Ouared A, Kazemirad S, Montagnon E, Cloutier G. Ultrasound Viscoelasticity Assessment Using an Adaptive Torsional Shear Wave Propagation Method. Med Phys (2016) 43(4):1603–14. doi:10.1118/1.4942813
84. Henni AH, Schmitt C, Trop I, Cloutier G. Shear Wave Induced Resonance Elastography of Spherical Masses with Polarized Torsional Waves. Appl Phys Lett (2012) 100(13):1337021–5. doi:10.1063/1.3696300
85. Budelli E, Brum J, Bernal M, Deffieux T, Tanter M, Lema P, et al. A Diffraction Correction for Storage and Loss Moduli Imaging Using Radiation Force Based Elastography. Phys Med Biol (2017) 62(1):91–106. doi:10.1088/1361-6560/62/1/91
86. Nenadic IZ, Urban MW, Heng Z, Sanchez W, Morgan PE, Greenleaf JF, et al. Application of Attenuation Measuring Ultrasound Shearwave Elastography in 8 post-transplant Liver Patients. In: IEEE International Ultrasonics Symposium (2014). p. 987–90.
87. Bernard S, Kazemirad S, Cloutier G. A Frequency-Shift Method to Measure Shear-Wave Attenuation in Soft Tissues. IEEE Trans Ultrason Ferroelect., Freq Contr (2017) 64(3):514–24. doi:10.1109/tuffc.2016.2634329
88. Ormachea J, Parker KJ. Comprehensive Viscoelastic Characterization of Tissues and the Inter-relationship of Shear Wave (Group and Phase) Velocity, Attenuation and Dispersion. Ultrasound Med Biol (2020) 46(12):3448–59. doi:10.1016/j.ultrasmedbio.2020.08.023
89. Wang MH, Palmeri ML, Rotemberg VM, Rouze NC, Nightingale KR. Improving the Robustness of Time-Of-Flight Based Shear Wave Speed Reconstruction Methods Using RANSAC in Human Liver In Vivo. Ultrasound Med Biol (2010) 36(5):802–13. doi:10.1016/j.ultrasmedbio.2010.02.007
90. Engel AJ, Bashford GR. A New Method for Shear Wave Speed Estimation in Shear Wave Elastography. IEEE Trans Ultrason Ferroelect., Freq Contr (2015) 62(12):2106–14. doi:10.1109/tuffc.2015.007282
91. Rouze NC, Deng Y, Trutna CA, Palmeri ML, Nightingale KR. Characterization of Viscoelastic Materials Using Group Shear Wave Speeds. IEEE Trans Ultrason Ferroelect., Freq Contr (2018) 65(5):780–94. doi:10.1109/tuffc.2018.2815505
92. Shiina T, Nightingale KR, Palmeri ML, Hall TJ, Bamber JC, Barr RG, et al. WFUMB Guidelines and Recommendations for Clinical Use of Ultrasound Elastography: Part 1: Basic Principles and Terminology. Ultrasound Med Biol (2015) 41(5):1126–47. doi:10.1016/j.ultrasmedbio.2015.03.009
93. Palmeri ML, Wang MH, Dahl JJ, Frinkley KD, Nightingale KR. Quantifying Hepatic Shear Modulus In Vivo Using Acoustic Radiation Force. Ultrasound Med Biol (2008) 34(4):546–58. doi:10.1016/j.ultrasmedbio.2007.10.009
94. Song P, Manduca A, Zhao H, Urban MW, Greenleaf JF, Chen S. Fast Shear Compounding Using Robust 2-D Shear Wave Speed Calculation and Multi-Directional Filtering. Ultrasound Med Biol (2014) 40(6):1343–55. doi:10.1016/j.ultrasmedbio.2013.12.026
95. Liu H-C, Kijanka P, Urban MW. Four-dimensional (4D) Phase Velocity Optical Coherence Elastography in Heterogeneous Materials and Biological Tissue. Biomed Opt Express (2020) 11(7):3795–817. doi:10.1364/boe.394835
96. Deffieux T, Montaldo G, Tanter M, Fink M. Shear Wave Spectroscopy for In Vivo Quantification of Human Soft Tissues Visco-Elasticity. IEEE Trans Med Imaging (2009) 28(3):313–22. doi:10.1109/tmi.2008.925077
97. Urban MW, Pislaru C, Nenadic IZ, Kinnick RR, Greenleaf JF. Measurement of Viscoelastic Properties of In Vivo Swine Myocardium Using Lamb Wave Dispersion Ultrasound Vibrometry (LDUV). IEEE Trans Med Imaging (2013) 32(2):247–61. doi:10.1109/tmi.2012.2222656
98. Helfenstein-Didier C, Andrade RJ, Brum J, Hug F, Tanter M, Nordez A, et al. In Vivoquantification of the Shear Modulus of the Human Achilles Tendon during Passive Loading Using Shear Wave Dispersion Analysis. Phys Med Biol (2016) 61(6):2485–96. doi:10.1088/0031-9155/61/6/2485
99. Ambrozinski L, Packo P, Pieczonka L, Stepinski T, Uhl T, Staszewski WJ. Identification of Material Properties - Efficient Modelling Approach Based on Guided Wave Propagation and Spatial Multiple Signal Classification. Struct Control Health Monit (2015) 22(7):969–83. doi:10.1002/stc.1728
100. Parker KJ, Ormachea J, Will S, Hah Z. Analysis of Transient Shear Wave in Lossy media. Ultrasound Med Biol (2018) 44(7):1504–15. doi:10.1016/j.ultrasmedbio.2018.03.014
101. Bernal M, Nenadic I, Urban MW, Greenleaf JF. Material Property Estimation for Tubes and Arteries Using Ultrasound Radiation Force and Analysis of Propagating Modes. J Acoust Soc America (2011) 129(3):1344–54. doi:10.1121/1.3533735
102. Alleyne D, Cawley P. A Two-Dimensional Fourier Transform Method for the Measurement of Propagating Multimode Signals. J Acoust Soc America (1991) 89(3):1159–68. doi:10.1121/1.400530
103. Nightingale K, Rouze N, Rosenzweig S, Wang M, Abdelmalek M, Guy C, et al. Derivation and Analysis of Viscoelastic Properties in Human Liver: Impact of Frequency on Fibrosis and Steatosis Staging. IEEE Trans Ultrason Ferroelect., Freq Contr (2015) 62(1):165–75. doi:10.1109/tuffc.2014.006653
104. Parker KJ, Szabo T, Holm S. Towards a Consensus on Rheological Models for Elastography in Soft Tissues. Phys Med Biol (2019) 64(21):215012. doi:10.1088/1361-6560/ab453d
105. Kijanka P, Urban MW. Local Phase Velocity Based Imaging: A New Technique Used for Ultrasound Shear Wave Elastography. IEEE Trans Med Imaging (2019) 38(4):894–908. doi:10.1109/tmi.2018.2874545
106. Kijanka P, Urban MW. Fast Local Phase Velocity-Based Imaging: Shear Wave Particle Velocity and Displacement Motion Study. IEEE Trans Ultrason Ferroelect., Freq Contr (2020) 67(3):526–37. doi:10.1109/tuffc.2019.2948512
107. Nenadic IZ, Qiang B, Urban MW, Zhao H, Sanchez W, Greenleaf JF, et al. Attenuation Measuring Ultrasound Shearwave Elastography Andin Vivoapplication in post-transplant Liver Patients. Phys Med Biol (2016) 62(2):484–500. doi:10.1088/1361-6560/aa4f6f
108. Rouze NC, Deng Y, Palmeri ML, Nightingale KR. Accounting for the Spatial Observation Window in the 2-D Fourier Transform Analysis of Shear Wave Attenuation. Ultrasound Med Biol (2017) 43(10):2500–6. doi:10.1016/j.ultrasmedbio.2017.06.006
109. Gennisson J-L, Deffieux T, Macé E, Montaldo G, Fink M, Tanter M. Viscoelastic and Anisotropic Mechanical Properties of In Vivo Muscle Tissue Assessed by Supersonic Shear Imaging. Ultrasound Med Biol (2010) 36(5):789–801. doi:10.1016/j.ultrasmedbio.2010.02.013
110. Brum J, Bernal M, Gennisson JL, Tanter M. In Vivoevaluation of the Elastic Anisotropy of the Human Achilles Tendon Using Shear Wave Dispersion Analysis. Phys Med Biol (2014) 59(3):505–23. doi:10.1088/0031-9155/59/3/505
111. Rouze NC, Wang MH, Palmeri ML, Nightingale KR. Finite Element Modeling of Impulsive Excitation and Shear Wave Propagation in an Incompressible, Transversely Isotropic Medium. J Biomech (2013) 46(16):2761–8. doi:10.1016/j.jbiomech.2013.09.008
112. Chatelin S, Gennisson J-L, Bernal M, Tanter M, Pernot M. Modelling the Impulse Diffraction Field of Shear Waves in Transverse Isotropic Viscoelastic Medium. Phys Med Biol (2015) 60(9):3639–54. doi:10.1088/0031-9155/60/9/3639
113. Quan Y, Harris JM. Seismic Attenuation Tomography Using the Frequency Shift Method. Geophysics (1997) 62(3):895–905. doi:10.1190/1.1444197
114. Dines KA, Kak AC. Ultrasonic Attenuation Tomography of Soft Tissues. Ultrason Imaging (1979) 1(1):16–33. doi:10.1177/016173467900100102
115. Fink M, Hottier F, Cardoso JF. Ultrasonic Signal Processing for In Vivo Attenuation Measurement: Short Time Fourier Analysis. Ultrason Imaging (1983) 5(2):117–35. doi:10.1177/016173468300500202
116. Kijanka P, Urban MW. Two-point Frequency Shift Method for Shear Wave Attenuation Measurement. IEEE Trans Ultrason Ferroelect., Freq Contr (2020) 67(3):483–96. doi:10.1109/tuffc.2019.2945620
117. Zaleska-Dorobisz U, Kaczorowski K, Pawluś A, Puchalska A, Inglot M. Ultrasound Elastography - Review of Techniques and its Clinical Applications. Adv Clin Exp Med (2014) 23(4):645–55. doi:10.17219/acem/26301
118. Barr RG. Elastography in Clinical Practice. Radiologic Clin North America (2014) 52(6):1145–62. doi:10.1016/j.rcl.2014.07.002
119. Sigrist RMS, Liau J, Kaffas AE, Chammas MC, Willmann JK. Ultrasound Elastography: Review of Techniques and Clinical Applications. Theranostics (2017) 7(5):1303–29. doi:10.7150/thno.18650
120. El-Serag HB. Hepatocellular Carcinoma and Hepatitis C in the United States. Hepatology (2002) 36(5B):s74–s83. doi:10.1053/jhep.2002.36807
121. Kose S, Ersan G, Tatar B, Adar P, Erturk Sengel B. Evaluation of Percutaneous Liver Biopsy Complications in Patients with Chronic Viral Hepatitis. Eurasian J Med (2015) 47(3):161–4. doi:10.5152/eurasianjmed.2015.107
122. Bamber J, Cosgrove D, Dietrich C, Fromageau J, Bojunga J, Calliada F, et al. EFSUMB Guidelines and Recommendations on the Clinical Use of Ultrasound Elastography. Part 1: Basic Principles and Technology. Ultraschall Med (2013) 34(02):169–84. doi:10.1055/s-0033-1335205
123. Ferraioli G, Tinelli C, Zicchetti M, Above E, Poma G, Di Gregorio M, et al. Reproducibility of Real-Time Shear Wave Elastography in the Evaluation of Liver Elasticity. Eur J Radiol (2012) 81(11):3102–6. doi:10.1016/j.ejrad.2012.05.030
124. Ganne-Carrié N, Ziol M, de Ledinghen V, Douvin C, Marcellin P, Castera L, et al. Accuracy of Liver Stiffness Measurement for the Diagnosis of Cirrhosis in Patients with Chronic Liver Diseases. Hepatology (2006) 44(6):1511–7. doi:10.1002/hep.21420
125. Nahon P, Kettaneh A, Tengher-Barna I, Ziol M, de Lédinghen V, Douvin C, et al. Assessment of Liver Fibrosis Using Transient Elastography in Patients with Alcoholic Liver Disease. J Hepatol (2008) 49(6):1062–8. doi:10.1016/j.jhep.2008.08.011
126. Ferraioli G. Point Shear Wave Elastography Method for Assessing Liver Stiffness. World J Gastroenterol (2014) 20(16). doi:10.3748/wjg.v20.i16.4787
127. Millonig G, Friedrich S, Adolf S, Fonouni H, Golriz M, Mehrabi A, et al. Liver Stiffness Is Directly Influenced by central Venous Pressure. J Hepatol (2010) 52(2):206–10. doi:10.1016/j.jhep.2009.11.018
128. Millonig G, Reimann FM, Friedrich S, Fonouni H, Mehrabi A, Büchler MW, et al. Extrahepatic Cholestasis Increases Liver Stiffness (FibroScan) Irrespective of Fibrosis. Hepatology (2008) 48(5):1718–23. doi:10.1002/hep.22577
129. Takahashi H, Ono N, Eguchi Y, Eguchi T, Kitajima Y, Kawaguchi Y, et al. Evaluation of Acoustic Radiation Force Impulse Elastography for Fibrosis Staging of Chronic Liver Disease: a Pilot Study. Liver Int (2010) 30(4):538–45. doi:10.1111/j.1478-3231.2009.02130.x
130. Boursier J, Isselin G, Fouchard-Hubert I, Oberti F, Dib N, Lebigot J, et al. Acoustic Radiation Force Impulse: a New Ultrasonographic Technology for the Widespread Noninvasive Diagnosis of Liver Fibrosis. Eur J Gastroenterol Hepatol (2010) 22(9):1074–84. doi:10.1097/meg.0b013e328339e0a1
131. Friedrich-Rust M, Nierhoff J, Lupsor M, Sporea I, Fierbinteanu-Braticevici C, Strobel D, et al. Performance of Acoustic Radiation Force Impulse Imaging for the Staging of Liver Fibrosis: a Pooled Meta-Analysis. J Viral Hepat (2012) 19(2):e212–e219. doi:10.1111/j.1365-2893.2011.01537.x
132. Hudson JM, Milot L, Parry C, Williams R, Burns PN. Inter- and Intra-operator Reliability and Repeatability of Shear Wave Elastography in the Liver: A Study in Healthy Volunteers. Ultrasound Med Biol (2013) 39(6):950–5. doi:10.1016/j.ultrasmedbio.2012.12.011
133. Carey E, Carey WD. Noninvasive Tests for Liver Disease, Fibrosis, and Cirrhosis: Is Liver Biopsy Obsolete? Cleve Clin J Med (2010) 77(8):519–27. doi:10.3949/ccjm.77a.09138
134. Ferraioli G, Tinelli C, Dal Bello B, Zicchetti M, Filice G, Filice C. Accuracy of Real-Time Shear Wave Elastography for Assessing Liver Fibrosis in Chronic Hepatitis C: a Pilot Study. Hepatology (2012) 56(6):2125–33. doi:10.1002/hep.25936
135. Dietrich C, Bamber J, Berzigotti A, Bota S, Cantisani V, Castera L, et al. EFSUMB Guidelines and Recommendations on the Clinical Use of Liver Ultrasound Elastography, Update 2017 (Long Version). Ultraschall Med (2017) 38(4):e16–e47. doi:10.1055/s-0043-103952
136. Ferraioli G, Wong VW-S, Castera L, Berzigotti A, Sporea I, Dietrich CF, et al. Liver Ultrasound Elastography: An Update to the World Federation for Ultrasound in Medicine and Biology Guidelines and Recommendations. Ultrasound Med Biol (2018) 44(12):2419–40. doi:10.1016/j.ultrasmedbio.2018.07.008
137. Barry CT, Mills B, Hah Z, Mooney RA, Ryan CK, Rubens DJ, et al. Shear Wave Dispersion Measures Liver Steatosis. Ultrasound Med Biol (2012) 38(2):175–82. doi:10.1016/j.ultrasmedbio.2011.10.019
138. Sugimoto K, Moriyasu F, Oshiro H, Takeuchi H, Yoshimasu Y, Kasai Y, et al. Viscoelasticity Measurement in Rat Livers Using Shear-Wave US Elastography. Ultrasound Med Biol (2018) 44(9):2018–24. doi:10.1016/j.ultrasmedbio.2018.05.008
139. Sugimoto K, Moriyasu F, Oshiro H, Takeuchi H, Yoshimasu Y, Kasai Y, et al. Clinical Utilization of Shear Wave Dispersion Imaging in Diffuse Liver Disease. Ultrasonography (2020) 39(1):3–10. doi:10.14366/usg.19031
140. Ophir J, Céspedes I, Ponnekanti H, Yazdi Y, Li X. Elastography: A Quantitative Method for Imaging the Elasticity of Biological Tissues. Ultrason Imaging (1991) 13(2):111–34. doi:10.1177/016173469101300201
141. Krouskop TA, Wheeler TM, Kallel F, Garra BS, Hall T. Elastic Moduli of Breast and Prostate Tissues under Compression. Ultrason Imaging (1998) 20(4):260–74. doi:10.1177/016173469802000403
142. Athanasiou A, Tardivon A, Tanter M, Sigal-Zafrani B, Bercoff J, Deffieux T, et al. Breast Lesions: Quantitative Elastography with Supersonic Shear Imaging-Preliminary Results. Radiology (2010) 256(1):297–303. doi:10.1148/radiol.10090385
143. Kolb TM, Lichy J, Newhouse JH. Comparison of the Performance of Screening Mammography, Physical Examination, and Breast US and Evaluation of Factors that Influence Them: an Analysis of 27,825 Patient Evaluations. Radiology (2002) 225(1):165–75. doi:10.1148/radiol.2251011667
144. Chiou S-Y, Chou Y-H, Chiou H-J, Wang H-K, Tiu C-M, Tseng L-M, et al. Sonographic Features of Nonpalpable Breast Cancer: A Study Based on Ultrasound-Guided Wire-Localized Surgical Biopsies. Ultrasound Med Biol (2006) 32(9):1299–306. doi:10.1016/j.ultrasmedbio.2006.05.018
145. Evans A, Whelehan P, Thomson K, McLean D, Brauer K, Purdie C, et al. Quantitative Shear Wave Ultrasound Elastography: Initial Experience in Solid Breast Masses. Breast Cancer Res (2010) 12(6):R104. doi:10.1186/bcr2787
146. Goddi A, Bonardi M, Alessi S. Breast Elastography: A Literature Review. J Ultrasound (2012) 15(3):192–8. doi:10.1016/j.jus.2012.06.009
147. Xue Y, Yao S, Li X, Zhang H. Benign and Malignant Breast Lesions Identification through the Values Derived from Shear Wave Elastography: Evidence for the Meta-Analysis. Oncotarget (2017) 8(51):89173–81. doi:10.18632/oncotarget.21124
148. Berg WA, Cosgrove DO, Doré CJ, Schäfer FKW, Svensson WE, Hooley RJ, et al. Shear-wave Elastography Improves the Specificity of Breast US: the BE1 Multinational Study of 939 Masses. Radiology (2012) 262(2):435–49. doi:10.1148/radiol.11110640
149. Lee SH, Chang JM, Kim WH, Bae MS, Seo M, Koo HR, et al. Added Value of Shear-Wave Elastography for Evaluation of Breast Masses Detected with Screening US Imaging. Radiology (2014) 273(1):61–9. doi:10.1148/radiol.14132443
150. Lee SH, Chung J, Choi HY, Choi SH, Ryu EB, Ko KH, et al. Evaluation of Screening US-Detected Breast Masses by Combined Use of Elastography and Color Doppler US with B-Mode US in Women with Dense Breasts: A Multicenter Prospective Study. Radiology (2017) 285(2):660–9. doi:10.1148/radiol.2017162424
151. Kumar V, Denis M, Gregory A, Bayat M, Mehrmohammadi M, Fazzio R, et al. Viscoelastic Parameters as Discriminators of Breast Masses: Initial Human Study Results. PLoS One (2018) 13(10):e0205717. doi:10.1371/journal.pone.0205717
152. Ormachea J, Parker KJ, Barr RG. An Initial Study of Complete 2D Shear Wave Dispersion Images Using a Reverberant Shear Wave Field. Phys Med Biol (2019) 64(14):145009. doi:10.1088/1361-6560/ab2778
153. Nabavizadeh A, Bayat M, Kumar V, Gregory A, Webb J, Alizad A, et al. Viscoelastic Biomarker for Differentiation of Benign and Malignant Breast Lesion in Ultra- Low Frequency Range. Sci Rep (2019) 9(1):5737. doi:10.1038/s41598-019-41885-9
154. Athanasiou A, Latorre-Ossa H, Criton A, Tardivon A, Gennisson J-L, Tanter M. Feasibility of Imaging and Treatment Monitoring of Breast Lesions with Three-Dimensional Shear Wave Elastography. Ultraschall Med (2015) 38:51–9. doi:10.1055/s-0034-1398980
155. Jing H, Cheng W, Li Z-Y, Ying L, Wang Q-C, Wu T, et al. Early Evaluation of Relative Changes in Tumor Stiffness by Shear Wave Elastography Predicts the Response to Neoadjuvant Chemotherapy in Patients with Breast Cancer. J Ultrasound Med (2016) 35(8):1619–27. doi:10.7863/ultra.15.08052
156. Evans A, Armstrong S, Whelehan P, Thomson K, Rauchhaus P, Purdie C, et al. Can Shear-Wave Elastography Predict Response to Neoadjuvant Chemotherapy in Women with Invasive Breast Cancer? Br J Cancer (2013) 109(11):2798–802. doi:10.1038/bjc.2013.660
157. Barr RG, Nakashima K, Amy D, Cosgrove D, Farrokh A, Schafer F, et al. WFUMB Guidelines and Recommendations for Clinical Use of Ultrasound Elastography: Part 2: Breast. Ultrasound Med Biol (2015) 41(5):1148–60. doi:10.1016/j.ultrasmedbio.2015.03.008
158. Woo S, Suh CH, Kim SY, Cho JY, Kim SH. Shear-wave Elastography for Detection of Prostate Cancer: a Systematic Review and Diagnostic Meta-Analysis. Am J Roentgenology (2017) 209(4):806–14. doi:10.2214/ajr.17.18056
159. Zhao C-K, Xu H-X. Ultrasound Elastography of the Thyroid: Principles and Current Status. Ultrasonography (2019) 38(2):106–24. doi:10.14366/usg.18037
160. Hoang P, Wallace A, Sugi M, Fleck A, Pershad Y, Dahiya N, et al. Elastography Techniques in the Evaluation of Deep Vein Thrombosis. Cardiovasc Diagn Ther (2017) 7(Suppl. 3):S238–S245. doi:10.21037/cdt.2017.10.04
161. Rouze NC, Palmeri ML, Nightingale KR. Tractable Calculation of the Green's Tensor for Shear Wave Propagation in an Incompressible, Transversely Isotropic Material. Phys Med Biol (2020) 65(1):015014. doi:10.1088/1361-6560/ab5c2d
162. Correia M, Deffieux T, Chatelin S, Provost J, Tanter M, Pernot M. 3D Elastic Tensor Imaging in Weakly Transversely Isotropic Soft Tissues. Phys Med Biol (2019) 63(15):155005. doi:10.1088/1361-6560/aacfaf
163. Wang M, Byram B, Palmeri M, Rouze N, Nightingale K. Imaging Transverse Isotropic Properties of Muscle by Monitoring Acoustic Radiation Force Induced Shear Waves Using a 2-D Matrix Ultrasound Array. IEEE Trans Med Imaging (2013) 32(9):1671–84. doi:10.1109/tmi.2013.2262948
164. Miyamoto N, Hirata K, Kanehisa H, Yoshitake Y. Validity of Measurement of Shear Modulus by Ultrasound Shear Wave Elastography in Human Pennate Muscle. PLoS One (2015) 10(4):e0124311. doi:10.1371/journal.pone.0124311
165. Aubry S, Nueffer J-P, Tanter M, Becce F, Vidal C, Michel F. Viscoelasticity in Achilles Tendonopathy: Quantitative Assessment by Using Real-Time Shear-Wave Elastography. Radiology (2015) 274(3):821–9. doi:10.1148/radiol.14140434
166. Muthupillai R, Lomas D, Rossman P, Greenleaf J, Manduca A, Ehman R. Magnetic Resonance Elastography by Direct Visualization of Propagating Acoustic Strain Waves. Science (1995) 269(5232):1854–7. doi:10.1126/science.7569924
167. Kolipaka A, McGee KP, Araoz PA, Glaser KJ, Manduca A, Romano AJ, et al. MR Elastography as a Method for the Assessment of Myocardial Stiffness: Comparison with an Established Pressure-Volume Model in a Left Ventricular Model of the Heart. Magn Reson Med (2009) 62(1):135–40. doi:10.1002/mrm.21991
168. Perriñez PR, Kennedy FE, Van Houten EE, Weaver JB, Paulsen KD. Magnetic Resonance Poroelastography: An Algorithm for Estimating the Mechanical Properties of Fluid-Saturated Soft Tissues. IEEE Trans Med Imaging (2010) 29(3):746–55. doi:10.1109/TMI.2009.2035309
169. Mariappan YK, Glaser KJ, Hubmayr RD, Manduca A, Ehman RL, McGee KP. MR Elastography of Human Lung Parenchyma: Technical Development, Theoretical Modeling and In Vivo Validation. J Magn Reson Imaging (2011) 33(6):1351–61. doi:10.1002/jmri.22550
170. Kolipaka A, McGee KP, Manduca A, Anavekar N, Ehman RL, Araoz PA. In Vivo assessment of MR Elastography-Derived Effective End-Diastolic Myocardial Stiffness under Different Loading Conditions. J Magn Reson Imaging (2011) 33(5):1224–8. doi:10.1002/jmri.22531
171. Yin M, Kolipaka A, Woodrum DA, Glaser KJ, Romano AJ, Manduca A, et al. Hepatic and Splenic Stiffness Augmentation Assessed with MR Elastography in an In Vivo Porcine portal Hypertension Model. J Magn Reson Imaging (2013) 38(4):809–15. doi:10.1002/jmri.24049
172. Klatt D, Johnson CL, Magin RL. Simultaneous, Multidirectional Acquisition of Displacement fields in Magnetic Resonance Elastography of the In Vivo Human Brain. J Magn Reson Imaging (2015) 42(2):297–304. doi:10.1002/jmri.24806
173. Low G, Owen NE, Joubert I, Patterson AJ, Graves MJ, Glaser KJ, et al. Reliability of Magnetic Resonance Elastography Using Multislice Two-Dimensional Spin-echo echo-planar Imaging (SE-EPI) and Three-Dimensional Inversion Reconstruction for Assessing Renal Stiffness. J Magn Reson Imaging (2015) 42(3):844–50. doi:10.1002/jmri.24826
174. Numano T, Mizuhara K, Hata J, Washio T, Homma K. A Simple Method for MR Elastography: A Gradient-echo Type Multi-echo Sequence. Magn Reson Imaging (2015) 33(1):31–7. doi:10.1016/j.mri.2014.10.002
175. Fattahi N, Arani A, Perry A, Meyer F, Manduca A, Glaser K, et al. MR Elastography Demonstrates Increased Brain Stiffness in normal Pressure Hydrocephalus. AJNR Am J Neuroradiol (2016) 37(3):462–7. doi:10.3174/ajnr.a4560
176. Anderson AT, Van Houten EEW, McGarry MDJ, Paulsen KD, Holtrop JL, Sutton BP, et al. Observation of Direction-dependent Mechanical Properties in the Human Brain with Multi-Excitation MR Elastography. J Mech Behav Biomed Mater (2016) 59:538–46. doi:10.1016/j.jmbbm.2016.03.005
177. Klatt D, Hamhaber U, Asbach P, Braun J, Sack I. Noninvasive Assessment of the Rheological Behavior of Human Organs Using Multifrequency MR Elastography: A Study of Brain and Liver Viscoelasticity. Phys Med Biol (2007) 52(24):7281–94. doi:10.1088/0031-9155/52/24/006
178. Asbach P, Klatt D, Hamhaber U, Braun J, Somasundaram R, Hamm B, et al. Assessment of Liver Viscoelasticity Using Multifrequency MR Elastography. Magn Reson Med (2008) 60(2):373–9. doi:10.1002/mrm.21636
179. Sack I, Rump J, Elgeti T, Samani A, Braun J. MR Elastography of the Human Heart: Noninvasive Assessment of Myocardial Elasticity Changes by Shear Wave Amplitude Variations. Magn Reson Med (2009) 61(3):668–77. doi:10.1002/mrm.21878
180. Asbach P, Klatt D, Schlosser B, Biermer M, Muche M, Rieger A, et al. Viscoelasticity-based Staging of Hepatic Fibrosis with Multifrequency MR Elastography. Radiology (2010) 257(1):80–6. doi:10.1148/radiol.10092489
181. Murphy MC, Huston J, Jack CR, Glaser KJ, Manduca A, Felmlee JP, et al. Decreased Brain Stiffness in Alzheimer's Disease Determined by Magnetic Resonance Elastography. J Magn Reson Imaging (2011) 34(3):494–8. doi:10.1002/jmri.22707
182. Streitberger KJ, Sack I, Krefting D, Pfüller C, Braun J, Paul F, et al. Brain Viscoelasticity Alteration in Chronic-Progressive Multiple Sclerosis. PLoS ONE (2012) 7(1):e29888. doi:10.1371/journal.pone.0029888
183. Johnson CL, Holtrop JL, McGarry MDJ, Weaver JB, Paulsen KD, Georgiadis JG, et al. 3D Multislab, Multishot Acquisition for Fast, Whole-Brain MR Elastography with High Signal-To-Noise Efficiency. Magn Reson Med (2014) 71(2):477–85. doi:10.1002/mrm.25065
184. McGarry MDJ, Johnson CL, Sutton BP, Georgiadis JG, Van Houten EEW, Pattison AJ, et al. Suitability of Poroelastic and Viscoelastic Mechanical Models for High and Low Frequency MR Elastography. Med Phys (2015) 42(2):947–57. doi:10.1118/1.4905048
185. Sinkus R, Tanter M, Xydeas T, Catheline S, Bercoff J, Fink M. Viscoelastic Shear Properties of In Vivo Breast Lesions Measured by MR Elastography. Magn Reson Imaging (2005) 23(2):159–65. doi:10.1016/j.mri.2004.11.060
186. Rump J, Klatt D, Braun J, Warmuth C, Sack I. Fractional Encoding of Harmonic Motions in MR Elastography. Magn Reson Med (2007) 57(2):388–95. doi:10.1002/mrm.21152
187. Green MA, Bilston LE, Sinkus R. In Vivobrain Viscoelastic Properties Measured by Magnetic Resonance Elastography. NMR Biomed (2008) 21(7):755–64. doi:10.1002/nbm.1254
188. Riek K, Klatt D, Nuzha H, Mueller S, Neumann U, Sack I, et al. Wide-range Dynamic Magnetic Resonance Elastography. J Biomech (2011) 44(7):1380–6. doi:10.1016/j.jbiomech.2010.12.031
189. Zhang J, Green MA, Sinkus R, Bilston LE. Viscoelastic Properties of Human Cerebellum Using Magnetic Resonance Elastography. J Biomech (2011) 44(10):1909–13. doi:10.1016/j.jbiomech.2011.04.034
190. Garteiser P, Sahebjavaher RS, Ter Beek LC, Salcudean S, Vilgrain V, Van Beers BE, et al. Rapid Acquisition of Multifrequency, Multislice and Multidirectional MR Elastography Data with a Fractionally Encoded Gradient echo Sequence. NMR Biomed (2013) 26(10):1326–35. doi:10.1002/nbm.2958
191. Braun J, Guo J, Lützkendorf R, Stadler J, Papazoglou S, Hirsch S, et al. High-resolution Mechanical Imaging of the Human Brain by Three-Dimensional Multifrequency Magnetic Resonance Elastography at 7T. NeuroImage (2014) 90:308–14. doi:10.1016/j.neuroimage.2013.12.032
192. Sahebjavaher RS, Frew S, Bylinskii A, ter Beek L, Garteiser P, Honarvar M, et al. Prostate MR Elastography with Transperineal Electromagnetic Actuation and a Fast Fractionally Encoded Steady-State Gradient echo Sequence. NMR Biomed (2014) 27(7):784–94. doi:10.1002/nbm.3118
193. Hirsch S, Guo J, Reiter R, Papazoglou S, Kroencke T, Braun J, et al. MR Elastography of the Liver and the Spleen Using a Piezoelectric Driver, Single-Shot Wave-Field Acquisition, and Multifrequency Dual Parameter Reconstruction. Magn Reson Med (2014) 71(1):267–77. doi:10.1002/mrm.24674
194. Tzschätzsch H, Guo J, Dittmann F, Hirsch S, Barnhill E, Jöhrens K, et al. Tomoelastography by Multifrequency Wave Number Recovery from Time-Harmonic Propagating Shear Waves. Med Image Anal (2016) 30:1–10. doi:10.1016/j.media.2016.01.001
195. Guo J, Hirsch S, Fehlner A, Papazoglou S, Scheel M, Braun J, et al. Towards an Elastographic Atlas of Brain Anatomy. PLoS ONE (2013) 8(8):e71807. doi:10.1371/journal.pone.0071807
196. Strasser J, Haindl MT, Stollberger R, Fazekas F, Ropele S. Magnetic Resonance Elastography of the Human Brain Using a Multiphase DENSE Acquisition. Magn Reson Med (2019) 81(6):3578–87. doi:10.1002/mrm.27672
197. Doyley MM, VanHouten EE, Weaver JB, Poplack S, Duncan L, Kennedy F, et al. Shear Modulus Estimation Using Parallelized Partial Volumetric Reconstruction. IEEE Trans Med Imaging (2004) 23(11):1404–16. doi:10.1109/tmi.2004.834624
198. Clayton EH, Garbow JR, Bayly PV. Frequency-dependent Viscoelastic Parameters of Mouse Brain Tissue Estimated by MR Elastography. Phys Med Biol (2011) 56(8):2391–406. doi:10.1088/0031-9155/56/8/005
199. Yin Z, Kearney SP, Magin RL, Klatt D. Concurrent 3D Acquisition of Diffusion Tensor Imaging and Magnetic Resonance Elastography Displacement Data ( DTI-MRE ): Theory and In Vivo Application. Magn Reson Med (2017) 77(1):273–84. doi:10.1002/mrm.26121
200. Papazoglou S, Hirsch S, Braun J, Sack I. Multifrequency Inversion in Magnetic Resonance Elastography. Phys Med Biol (2012) 57(8):2329–46. doi:10.1088/0031-9155/57/8/2329
201. Streitberger KJ, Reiss-Zimmermann M, Freimann FB, Bayerl S, Guo J, Arlt F, et al. High-resolution Mechanical Imaging of Glioblastoma by Multifrequency Magnetic Resonance Elastography. PLoS ONE (2014) 9(10):e110588. doi:10.1371/journal.pone.0110588
202. Streitberger K-J, Diederichs G, Guo J, Fehlner A, Hamm B, Braun J, et al. In Vivo multifrequency Magnetic Resonance Elastography of the Human Intervertebral Disk. Magn Reson Med (2015) 74(5):1380–7. doi:10.1002/mrm.25505
203. Testu J, McGarry MDJ, Dittmann F, Weaver JB, Paulsen KD, Sack I, et al. Viscoelastic Power Law Parameters of In Vivo Human Brain Estimated by MR Elastography. J Mech Behav Biomed Mater (2017) 74:333–41. doi:10.1016/j.jmbbm.2017.06.027
204. Neumann W, Schad LR, Zöllner FG. A Novel 3D-Printed Mechanical Actuator Using Centrifugal Force for Magnetic Resonance Elastography. In: 2017 39th Annual International Conference of the IEEE Engineering in Medicine and Biology SocietyEMBC (2017). p. 3541–4.
205. Numano T, Kawabata Y, Mizuhara K, Washio T, Nitta N, Homma K. Magnetic Resonance Elastography Using an Air ball-actuator. Magn Reson Imaging (2013) 31(6):939–46. doi:10.1016/j.mri.2013.02.001
206. Runge JH, Hoelzl SH, Sudakova J, Dokumaci AS, Nelissen JL, Guenthner C, et al. A Novel Magnetic Resonance Elastography Transducer Concept Based on a Rotational Eccentric Mass: Preliminary Experiences with the Gravitational Transducer. Phys Med Biol (2019) 64(4):045007. doi:10.1088/1361-6560/aaf9f8
207. Wu T, Felmlee JP, Greenleaf JF, Riederer SJ, Ehman RL. MR Imaging of Shear Waves Generated by Focused Ultrasound. Magn Reson Med (2000) 43(1):111–5. doi:10.1002/(sici)1522-2594(200001)43:1<111::aid-mrm13>3.0.co;2-d
208. Weaver JB, Pattison AJ, McGarry MD, Perreard IM, Swienckowski JG, Eskey CJ, et al. Brain Mechanical Property Measurement Using MRE with Intrinsic Activation. Phys Med Biol (2012) 57(22):7275–87. doi:10.1088/0031-9155/57/22/7275
209. Hirsch S, Klatt D, Freimann F, Scheel M, Braun J, Sack I. In Vivo measurement of Volumetric Strain in the Human Brain Induced by Arterial Pulsation and Harmonic Waves. Magn Reson Med (2013) 70(3):671–83. doi:10.1002/mrm.24499
210. Zorgani A, Souchon R, Dinh A-H, Chapelon J-Y, Ménager J-M, Lounis S, et al. Brain Palpation from Physiological Vibrations Using MRI. Proc Natl Acad Sci USA (2015) 112(42):12917–21. doi:10.1073/pnas.1509895112
211. Solamen LM, McGarry MDJ, Fried J, Weaver JB, Lollis SS, Paulsen KD. Poroelastic Mechanical Properties of the Brain Tissue of normal Pressure Hydrocephalus Patients during Lumbar drain Treatment Using Intrinsic Actuation MR Elastography. Acad Radiol (2020) 28(4):457–66. doi:10.1016/j.acra.2020.03.009
212. Guenthner C, Kozerke S. Encoding and Readout Strategies in Magnetic Resonance Elastography. NMR Biomed (2018) 31(10):1–29. doi:10.1002/nbm.3919
213. Hahn EL. Detection of Sea-Water Motion by Nuclear Precession. J Geophys Res (1960) 65(2):776–7. doi:10.1029/jz065i002p00776
214. Moran PR. A Flow Velocity Zeugmatographic Interlace for NMR Imaging in Humans. Magn Reson Imaging (1982) 1(4):197–203. doi:10.1016/0730-725x(82)90170-9
215. Haacke EM, Brown RW, Thompson MR, Venkatesan R Magnetic Resonance Imaging: Physical Principles and Sequence Design. John Wiley & Sons (2014).
217. Muthupillai R, Rossman PJ, Lomas DJ, Greenleaf JF, Riederer SJ, Ehman RL. Magnetic Resonance Imaging of Transverse Acoustic Strain Waves. Magn Reson Med (1996) 36(2):266–74. doi:10.1002/mrm.1910360214
218. Sinkus R, Daire JL, Van Beers BE, Vilgrain V. Elasticity Reconstruction: Beyond the assumption of Local Homogeneity. Comptes Rendus - Mecanique (2010) 338(7-8):474–9. doi:10.1016/j.crme.2010.07.014
219. Boulet T, Kelso ML, Othman SF. Microscopic Magnetic Resonance Elastography of Traumatic Brain Injury Model. J Neurosci Methods (2011) 201(2):296–306. doi:10.1016/j.jneumeth.2011.08.019
220. Okamoto RJ, Clayton EH, Bayly PV. Viscoelastic Properties of Soft Gels: Comparison of Magnetic Resonance Elastography and Dynamic Shear Testing in the Shear Wave Regime. Phys Med Biol (2011) 56(19):6379–400. doi:10.1088/0031-9155/56/19/014
221. Yin Z, Magin RL, Klatt D. Simultaneous MR Elastography and Diffusion Acquisitions: Diffusion-MRE (dMRE). Magn Reson Med (2014) 71(5):1682–8. doi:10.1002/mrm.25180
222. Sack I, McGowan CK, Samani A, Luginbuhl C, Oakden W, Plewes DB. Observation of Nonlinear Shear Wave Propagation Using Magnetic Resonance Elastography. Magn Reson Med (2004) 52(4):842–50. doi:10.1002/mrm.20238
223. Clayton EH, Genin GM, Bayly PV. Transmission, Attenuation and Reflection of Shear Waves in the Human Brain. J R Soc Interf (2012) 9(76):2899–910. doi:10.1098/rsif.2012.0325
224. Bernstein MA, King KF, Zhou XJ Handbook of MRI Pulse Sequences. Burlington: Academic Press (2004). p. iv.
225. Manduca A, Muthupillai R, Rossman PJ, Greenleaf JF, Ehman RL. Local Wavelength Estimation for Magnetic Resonance Elastography. Proc 3rd IEEE Int Conf Image Process (1996) 3:527–30.
226. Latta P, Gruwel MLH, Debergue P, Matwiy B, Sboto-Frankenstein UN, Tomanek B. Convertible Pneumatic Actuator for Magnetic Resonance Elastography of the Brain. Magn Reson Imaging (2011) 29(1):147–52. doi:10.1016/j.mri.2010.07.014
227. Sack I, Beierbach B, Wuerfel J, Klatt D, Hamhaber U, Papazoglou S, et al. The Impact of Aging and Gender on Brain Viscoelasticity. NeuroImage (2009) 46(3):652–7. doi:10.1016/j.neuroimage.2009.02.040
228. Wuerfel J, Paul F, Beierbach B, Hamhaber U, Klatt D, Papazoglou S, et al. MR-elastography Reveals Degradation of Tissue Integrity in Multiple Sclerosis. NeuroImage (2010) 49(3):2520–5. doi:10.1016/j.neuroimage.2009.06.018
229. Sack I, Streitberger KJ, Krefting D, Paul F, Braun J. The Influence of Physiological Aging and Atrophy on Brain Viscoelastic Properties in Humans. PLoS ONE (2011) 6(9):e23451. doi:10.1371/journal.pone.0023451
230. Solanas PS, Tse K, Koon V, Ratiney H, Millioz F, Caussy C, et al. Harmonic Wideband Simultaneous Dual-Frequency MR Elastography. NMR Biomed (2020) e4442.
231. Gordon-Wylie SW, Solamen LM, McGarry MDJ, Zeng W, VanHouten E, Gilbert G, et al. MR Elastography at 1 Hz of Gelatin Phantoms Using 3D or 4D Acquisition. J Magn Reson (2018) 296:112–20. doi:10.1016/j.jmr.2018.08.012
232. Herzka DA, Kotys MS, Sinkus R, Pettigrew RI, Gharib AM. Magnetic Resonance Elastography in the Liver at 3 Tesla Using a Second Harmonic Approach. Magn Reson Med (2009) 62(2):284–91. doi:10.1002/mrm.21956
233. Elgeti T, Steffen IG, Knebel F, Hättasch R, Hamm B, Braun J, et al. Time-resolved Analysis of Left Ventricular Shear Wave Amplitudes in Cardiac Elastography for the Diagnosis of Diastolic Dysfunction. Invest Radiol (2016) 51(1):1–6. doi:10.1097/rli.0000000000000198
234. Elgeti T, Knebel F, Hättasch R, Hamm B, Braun J, Sack I. Shear-wave Amplitudes Measured with Cardiac MR Elastography for Diagnosis of Diastolic Dysfunction. Radiology (2014) 271(3):681–7. doi:10.1148/radiol.13131605
235. Rump J, Warmuth C, Braun J, Sack I. Phase Preparation in Steady-State Free Precession MR Elastography. Magn Reson Imaging (2008) 26(2):228–35. doi:10.1016/j.mri.2007.07.009
236. Elgeti T, Tzschätzsch H, Hirsch S, Krefting D, Klatt D, Niendorf T, et al. Vibration-synchronized Magnetic Resonance Imaging for the Detection of Myocardial Elasticity Changes. Magn Reson Med (2012) 67(4):919–24. doi:10.1002/mrm.24185
237. Bieri O, Maderwald S, Ladd ME, Scheffler K. Balanced Alternating Steady-State Elastography. Magn Reson Med (2006) 55(2):233–41. doi:10.1002/mrm.20812
238. Klatt D, Asbach P, Rump J, Papazoglou S, Somasundaram R, Modrow J, et al. In Vivo determination of Hepatic Stiffness Using Steady-State Free Precession Magnetic Resonance Elastography. Invest Radiol (2006) 41(12):841–8. doi:10.1097/01.rli.0000244341.16372.08
239. Doyley MM. Model-based Elastography: A Survey of Approaches to the Inverse Elasticity Problem. Phys Med Biol (2012) 57(3):R35–R73. doi:10.1088/0031-9155/57/3/r35
240. Knutsson H, Westin CF, Granlund G. Local Multiscale Frequency and Bandwidth Estimation. In: International Conference on Image Processing (1994). p. 36–40.
241. Braun J, Buntkowsky G, Bernarding J, Tolxdorff T, Sack I. Simulation and Analysis of Magnetic Resonance Elastography Wave Images Using Coupled Harmonic Oscillators and Gaussian Local Frequency Estimation. Magn Reson Imaging (2001) 19(5):703–13. doi:10.1016/s0730-725x(01)00387-3
242. McGee KP, Lake D, Mariappan Y, Hubmayr RD, Manduca A, Ansell K, et al. Calculation of Shear Stiffness in Noise Dominated Magnetic Resonance Elastography Data Based on Principal Frequency Estimation. Phys Med Biol (2011) 56(14):4291–309. doi:10.1088/0031-9155/56/14/006
243. Manduca A, Oliphant TE, Dresner MA, Mahowald JL, Kruse SA, Amromin E, et al. Magnetic Resonance Elastography: Non-invasive Mapping of Tissue Elasticity. Med Image Anal (2001) 5(4):237–54. doi:10.1016/s1361-8415(00)00039-6
244. Oliphant TE, Manduca A, Ehman RL, Greenleaf JF. Complex-valued Stiffness Reconstruction for Magnetic Resonance Elastography by Algebraic Inversion of the Differential Equation. Magn Reson Med (2001) 45(2):299–310. doi:10.1002/1522-2594(200102)45:2<299::aid-mrm1039>3.0.co;2-o
245. Manduca A, Lake DS, Kruse SA, Ehman RL. Spatio-temporal Directional Filtering for Improved Inversion of MR Elastography Images. Med Image Anal (2003) 7(4):465–73. doi:10.1016/s1361-8415(03)00038-0
246. Manduca A, Oliphant TE, Dresner MA, Lake DS, Greenleaf JF, Ehman RL. Comparative Evaluation of Inversion Algorithms for Magnetic Resonance Elastography. In: Proceedings IEEE International Symposium on Biomedical Imaging (2002). p. 997–1000.
247. Sinkus R, Tanter M, Catheline S, Lorenzen J, Kuhl C, Sondermann E, et al. Imaging Anisotropic and Viscous Properties of Breast Tissue by Magnetic Resonance-Elastography. Magn Reson Med (2005) 53(2):372–87. doi:10.1002/mrm.20355
248. Reiter R, Majumdar S, Kearney S, Kajdacsy-Balla A, Macias V, Crivellaro S, et al. Prostate Cancer Assessment Using MR Elastography of Fresh Prostatectomy Specimens at 9.4 T. Magn Reson Med (2020) 84(1):396–404. doi:10.1002/mrm.28127
249. Fovargue D, Kozerke S, Sinkus R, Nordsletten D. Robust MR Elastography Stiffness Quantification Using a Localized Divergence Free Finite Element Reconstruction. Med Image Anal (2018) 44:126–42. doi:10.1016/j.media.2017.12.005
250. Antonio Sánchez C, Drapaca CS, Sivaloganathan S, Vrscay ER. Elastography of Biological Tissue: Direct Inversion Methods that Allow for Local Shear Modulus Variations. In: International Conference Image Analysis and Recognition (2010). p. 195–206. doi:10.1007/978-3-642-13775-4_20
251. Barnhill E, Davies PJ, Ariyurek C, Fehlner A, Braun J, Sack I. Heterogeneous Multifrequency Direct Inversion (HMDI) for Magnetic Resonance Elastography with Application to a Clinical Brain Exam. Med Image Anal (2018) 46:180–8. doi:10.1016/j.media.2018.03.003
252. Babuška I, Suri M. Locking Effects in the Finite Element Approximation of Elasticity Problems. Numerische Mathematik (1992) 62(1):439–63.
253. Park E, Maniatty AM. Shear Modulus Reconstruction in Dynamic Elastography: Time Harmonic Case. Phys Med Biol (2006) 51(15):3697–721. doi:10.1088/0031-9155/51/15/007
254. Guo Z, You S, Wan X, Bićanić N. A FEM-Based Direct Method for Material Reconstruction Inverse Problem in Soft Tissue Elastography. Comput Structures (2010) 88(23-24):1459–68. doi:10.1016/j.compstruc.2008.06.004
255. Eskandari H, Salcudean SE, Rohling R, Bell I. Real-time Solution of the Finite Element Inverse Problem of Viscoelasticity. Inverse Probl (2011) 27(8):085002. doi:10.1088/0266-5611/27/8/085002
256. Honarvar M, Sahebjavaher R, Sinkus R, Rohling R, Salcudean SE. Curl-based Finite Element Reconstruction of the Shear Modulus without Assuming Local Homogeneity: Time Harmonic Case. IEEE Trans Med Imaging (2013) 32(12):2189–99. doi:10.1109/tmi.2013.2276060
257. Honarvar M, Rohling R, Salcudean SE. A Comparison of Direct and Iterative Finite Element Inversion Techniques in Dynamic Elastography. Phys Med Biol (2016) 61(8):3026–48. doi:10.1088/0031-9155/61/8/3026
258. Hansen PC. Getting to Business: Discretizations of Linear Inverse Problems. Discrete Inverse Probl (2010) 23–51.
259. Kallel F, Bertrand M. Tissue Elasticity Reconstruction Using Linear Perturbation Method. IEEE Trans Med Imaging (1996) 15(3):299–313. doi:10.1109/42.500139
260. Samani A, Bishop J, Plewes DB. A Constrained Modulus Reconstruction Technique for Breast Cancer Assessment. IEEE Trans Med Imaging (2001) 20(9):877–85. doi:10.1109/42.952726
261. Zhang Y, Hall LO, Goldgof DB, Sarkar S. A Constrained Genetic Approach for Computing Material Property of Elastic Objects. IEEE Trans Evol Comput (2006) 10(3):341–57.
262. Oberai AA, Gokhale NH, Feij o GR. Solution of Inverse Problems in Elasticity Imaging Using the Adjoint Method. Inverse Probl (2003) 19(2):297–313. doi:10.1088/0266-5611/19/2/304
263. Van Houten EEW, Paulsen KD, Miga MI, Kennedy FE, Weaver JB. An Overlapping Subzone Technique for MR-Based Elastic Property Reconstruction. Magn Reson Med (1999) 42(4):779–86. doi:10.1002/(sici)1522-2594(199910)42:4<779::aid-mrm21>3.0.co;2-z
264. McGarry MDJ, Van Houten EEW, Johnson CL, Georgiadis JG, Sutton BP, Weaver JB, et al. Multiresolution MR Elastography Using Nonlinear Inversion. Med Phys (2012) 39(10):6388–96. doi:10.1118/1.4754649
265. McGarry MDJ, Van Houten E, Guertler C, Okamoto RJ, Smith DR, Sowinski DR, et al. A Heterogenous, Time Harmonic, Nearly Incompressible Transverse Isotropic Finite Element Brain Simulation Platform for MR Elastography. Phys Med Biol (2020) 66. doi:10.1088/1361-6560/ab9a84
266. McGarry MDJ, Van Houten EEW, Perrĩez PR, Pattison AJ, Weaver JB, Paulsen KD. An Octahedral Shear Strain-Based Measure of SNR for 3D MR Elastography. Phys Med Biol (2011) 56(13):N153. doi:10.1088/0031-9155/56/13/n02
267. Van Houten EEW, Viviers Dv., McGarry MDJ, Perriñez PR, Perreard II, Weaver JB, et al. Subzone Based Magnetic Resonance Elastography Using a Rayleigh Damped Material Model. Med Phys (2011) 38(4):1993–2004. doi:10.1118/1.3557469
268. Van Houten EEW, Doyley MM, Kennedy FE, Paulsen KD, Weaver JB. A Three-Parameter Mechanical Property Reconstruction Method for MR-Based Elastic Property Imaging. IEEE Trans Med Imaging (2005) 24(2):311–24. doi:10.1109/tmi.2004.842451
269. Van Houten EEW, Weaver JB, Miga MI, Kennedy FE, Paulsen KD. Elasticity Reconstruction from Experimental MR Displacement Data: Initial Experience with an Overlapping Subzone Finite Element Inversion Process. Med Phys (2000) 27(1):101–7. doi:10.1118/1.598861
270. Tan L, McGarry MDJ, Van Houten EEW, Ji M, Solamen L, Weaver JB, et al. Gradient-based Optimization for Poroelastic and Viscoelastic MR Elastography. IEEE Trans Med Imaging (2017) 36(1):236–50. doi:10.1109/tmi.2016.2604568
271. Perriñez PR, Kennedy FE, Van Houten EEW, Weaver JB, Paulsen KD. Modeling of Soft Poroelastic Tissue in Time-Harmonic MR Elastography. IEEE Trans Biomed Eng (2009) 56(3):598–608. doi:10.1109/tbme.2008.2009928
272. Solamen LM, McGarry MD, Tan L, Weaver JB, Paulsen KD. Phantom Evaluations of Nonlinear Inversion MR Elastography. Phys Med Biol (2018) 63(14):145021. doi:10.1088/1361-6560/aacb08
273. McGarry M, Van Houten E, Solamen L, Gordon-Wylie S, Weaver J, Paulsen K. Uniqueness of Poroelastic and Viscoelastic Nonlinear Inversion MR Elastography at Low Frequencies. Phys Med Biol (2019) 64(7):075006. doi:10.1088/1361-6560/ab0a7d
274. Singh S, Venkatesh SK, Wang Z, Miller FH, Motosugi U, Low RN, et al. Diagnostic Performance of Magnetic Resonance Elastography in Staging Liver Fibrosis: A Systematic Review and Meta-Analysis of Individual Participant Data. Clin Gastroenterol Hepatol (2015) 13(3):440–51.e6. doi:10.1016/j.cgh.2014.09.046
275. Singh S, Venkatesh SK, Loomba R, Wang Z, Sirlin C, Chen J, et al. Magnetic Resonance Elastography for Staging Liver Fibrosis in Non-alcoholic Fatty Liver Disease: a Diagnostic Accuracy Systematic Review and Individual Participant Data Pooled Analysis. Eur Radiol (2016) 26(5):1431–40. doi:10.1007/s00330-015-3949-z
276. Huwart L, Peeters F, Sinkus R, Annet L, Salameh N, ter Beek LC, et al. Liver Fibrosis: Non-invasive Assessment with MR Elastography. NMR Biomed (2006) 19(2):173–9. doi:10.1002/nbm.1030
277. Huwart L, Sempoux C, Salameh N, Jamart J, Annet L, Sinkus R, et al. Liver Fibrosis: Noninvasive Assessment with MR Elastography versus Aspartate Aminotransferase-To-Platelet Ratio Index. Radiology (2007) 245(2):458–66. doi:10.1148/radiol.2452061673
278. Salameh N, Larrat B, Abarca-Quinones J, Pallu S, Dorvillius M, Leclercq I, et al. Early Detection of Steatohepatitis in Fatty Rat Liver by Using MR Elastography. Radiology (2009) 253(1):90–7. doi:10.1148/radiol.2523081817
279. Ronot M, Lambert SA, Wagner M, Garteiser P, Doblas S, Albuquerque M, et al. Viscoelastic Parameters for Quantifying Liver Fibrosis: Three-Dimensional Multifrequency MR Elastography Study on Thin Liver Rat Slices. Plos One (2014) 9(4):e94679. doi:10.1371/journal.pone.0094679
280. Hiscox LV, Johnson CL, Barnhill E, McGarry MDJ, Huston J, Van Beek EJR, et al. Magnetic Resonance Elastography (MRE) of the Human Brain: Technique, Findings and Clinical Applications. Phys Med Biol (2016) 61(24):401–37. doi:10.1088/0031-9155/61/24/r401
281. Hiscox LV, Johnson CL, McGarry MDJ, Perrins M, Littlejohn A, van Beek EJR, et al. High-resolution Magnetic Resonance Elastography Reveals Differences in Subcortical gray Matter Viscoelasticity between Young and Healthy Older Adults. Neurobiol Aging (2018) 65:158–67. doi:10.1016/j.neurobiolaging.2018.01.010
282. Hiscox LV, Schwarb H, McGarry MDJ, Johnson CL. Aging Brain Mechanics: Progress and Promise of Magnetic Resonance Elastography. NeuroImage (2021) 232:117889. doi:10.1016/j.neuroimage.2021.117889
283. Schregel K, Nazari N, Nowicki MO, Palotai M, Lawler SE, Sinkus R, et al. Characterization of Glioblastoma in an Orthotopic Mouse Model with Magnetic Resonance Elastography. NMR Biomed (2018) 31(10):e3840. doi:10.1002/nbm.3840
284. Fehlner A, Behrens JR, Streitberger K-J, Papazoglou S, Braun J, Bellmann-Strobl J, et al. Higher-resolution MR Elastography Reveals Early Mechanical Signatures of Neuroinflammation in Patients with Clinically Isolated Syndrome. J Magn Reson Imaging (2016) 44(1):51–8. doi:10.1002/jmri.25129
285. Schwarb H, Johnson CL, McGarry MDJ, Cohen NJ. Medial Temporal Lobe Viscoelasticity and Relational Memory Performance. NeuroImage (2016) 132:534–41. doi:10.1016/j.neuroimage.2016.02.059
286. Schwarb H, Johnson CL, Daugherty AM, Hillman CH, Kramer AF, Cohen NJ, et al. Aerobic Fitness, Hippocampal Viscoelasticity, and Relational Memory Performance. NeuroImage (2017) 153:179–88. doi:10.1016/j.neuroimage.2017.03.061
287. Sandroff BM, Johnson CL, Motl RW. Exercise Training Effects on Memory and Hippocampal Viscoelasticity in Multiple Sclerosis: a Novel Application of Magnetic Resonance Elastography. Neuroradiology (2017) 59(1):61–7. doi:10.1007/s00234-016-1767-x
288. Johnson CL, Schwarb H, Horecka KM, McGarry MDJ, Hillman CH, Kramer AF, et al. Double Dissociation of Structure-Function Relationships in Memory and Fluid Intelligence Observed with Magnetic Resonance Elastography. NeuroImage (2018) 171:99–106. doi:10.1016/j.neuroimage.2018.01.007
289. Schwarb H, Johnson CL, Dulas MR, McGarry MDJ, Holtrop JL, Watson PD, et al. Structural and Functional MRI Evidence for Distinct Medial Temporal and Prefrontal Roles in Context-dependent Relational Memory. J Cogn Neurosci (2019) 31(12):1857–72. doi:10.1162/jocn_a_01454
290. Hiscox LV, Johnson CL, McGarry MDJ, Schwarb H, van Beek EJR, Roberts N, et al. Hippocampal Viscoelasticity and Episodic Memory Performance in Healthy Older Adults Examined with Magnetic Resonance Elastography. Brain Imaging Behav (2020) 14(1):175–85. doi:10.1007/s11682-018-9988-8
291. McIlvain G, Clements RG, Magoon EM, Spielberg JM, Telzer EH, Johnson CL. Viscoelasticity of Reward and Control Systems in Adolescent Risk Taking. NeuroImage (2020) 215:116850. doi:10.1016/j.neuroimage.2020.116850
292. Sinkus R, Lorenzen J, Schrader D, Lorenzen M, Dargatz M, Holz D. High-resolution Tensor MR Elastography for Breast Tumour Detection. Phys Med Biol (2000) 45(6):1649–64. doi:10.1088/0031-9155/45/6/317
293. Qin EC, Sinkus R, Geng G, Cheng S, Green M, Rae CD, et al. Combining MR Elastography and Diffusion Tensor Imaging for the Assessment of Anisotropic Mechanical Properties: A Phantom Study. J Magn Reson Imaging (2013) 37(1):217–26. doi:10.1002/jmri.23797
294. Schmidt JL, Tweten DJ, Badachhape AA, Reiter AJ, Okamoto RJ, Garbow JR, et al. Measurement of Anisotropic Mechanical Properties in Porcine Brain white Matter Ex Vivo Using Magnetic Resonance Elastography. J Mech Behav Biomed Mater (2018) 79:30–7. doi:10.1016/j.jmbbm.2017.11.045
295. Lilaj L, Fischer T, Guo J, Braun J, Sack I, Hirsch S. Separation of Fluid and Solid Shear Wave fields and Quantification of Coupling Density by Magnetic Resonance Poroelastography. Magn Reson Med (2021) 85(3):1655–68. doi:10.1002/mrm.28507
296. Yin M, Talwalkar JA, Glaser KJ, Manduca A, Grimm RC, Rossman PJ, et al. Assessment of Hepatic Fibrosis with Magnetic Resonance Elastography. Clin Gastroenterol Hepatol (2007) 5(10):1207–13. doi:10.1016/j.cgh.2007.06.012
297. Patel BK, Samreen N, Zhou Y, Chen J, Brandt K, Ehman R, et al. MR Elastography of the Breast: Evolution of Technique, Case Examples, and Future Directions. Clin Breast Cancer (2021) 21(1):e102–e111. doi:10.1016/j.clbc.2020.08.005
298. Kennedy BF, Wijesinghe P, Sampson DD. The Emergence of Optical Elastography in Biomedicine. Nat Photon (2017) 11(4):215–21. doi:10.1038/nphoton.2017.6
299. Ingber D. Mechanobiology and Diseases of Mechanotransduction. Ann Med (2003) 35(8):564–77. doi:10.1080/07853890310016333
300. Di Carlo D. A Mechanical Biomarker of Cell State in Medicine. J Lab Autom (2012) 17(1):32–42. doi:10.1177/2211068211431630
301. Akinyi T, Grasland-Mongrain P, Bhatt M, Catheline S, Cloutier G. Recent Advances in Imaging of Cell Elasticity. Viscoelasticity and Collective Cell Migration (2021) 257–96. doi:10.1016/b978-0-12-820310-1.00010-0
302. Grasland-Mongrain P, Zorgani A, Nakagawa S, Bernard S, Paim LG, Fitzharris G, et al. Ultrafast Imaging of Cell Elasticity with Optical Microelastography. Proc Natl Acad Sci USA (2018) 115(5):861–6. doi:10.1073/pnas.1713395115
303. Poree J, Garcia D, Chayer B, Ohayon J, Cloutier G. Noninvasive Vascular Elastography with Plane Strain Incompressibility assumption Using Ultrafast Coherent Compound Plane Wave Imaging. IEEE Trans Med Imaging (2015) 34(12):2618–31. doi:10.1109/tmi.2015.2450992
304. Gallot T, Catheline S, Roux P, Brum J, Benech N, Negreira C. Passive Elastography: Shear-Wave Tomography from Physiological-Noise Correlation in Soft Tissues. IEEE Trans Ultrason Ferroelect., Freq Contr (2011) 58(6):1122–6. doi:10.1109/tuffc.2011.1920
305. Larin KV, Sampson DD. Optical Coherence Elastography - OCT at Work in Tissue Biomechanics [Invited]. Biomed Opt Express (2017) 8(2):1172–202. doi:10.1364/BOE.8.001172
306. Liang X, Boppart SA. Biomechanical Properties of In Vivo Human Skin from Dynamic Optical Coherence Elastography. IEEE Trans Biomed Eng (2010) 57(4):953–9. doi:10.1109/TBME.2009.2033464
307. Sun C, Standish B, Yang VX. Optical Coherence Elastography: Current Status and Future Applications. J Biomed Opt (2011) 16(4):043001. doi:10.1117/1.3560294
308. Kennedy KM, Ford C, Kennedy BF, Bush MB, Sampson DD. Analysis of Mechanical Contrast in Optical Coherence Elastography. J Biomed Opt (2013) 18(12):121508. doi:10.1117/1.jbo.18.12.121508
309. Yang VXD, Munce N, Pekar J, Gordon ML, Lo S, Marcon NE, et al. Micromachined Array Tip for Multifocus Fiber-Based Optical Coherence Tomography. Opt Lett (2004) 29:1754–6. doi:10.1364/ol.29.001754
310. Kennedy BF, McLaughlin RA, Kennedy KM, Chin L, Curatolo A, Tien A, et al. Optical Coherence Micro-elastography: Mechanical-Contrast Imaging of Tissue Microstructure. Biomed Opt Express (2014) 5(7):2113–24. doi:10.1364/BOE.5.002113
311. Wang S, Singh M, Tran TT, Leach J, Aglyamov SR, Larina IV, et al. Biomechanical Assessment of Myocardial Infarction Using Optical Coherence Elastography. Biomed Opt Express (2018) 9(2):728–42. doi:10.1364/boe.9.000728
312. Qu Y, Ma T, He Y, Zhu J, Dai C, Yu M, et al. Acoustic Radiation Force Optical Coherence Elastography of Corneal Tissue. IEEE J Select Top Quan Electron. (2016) 22(3):288–94. doi:10.1109/jstqe.2016.2524618
313. Rogowska J, Patel NA, Fujimoto JG, Brezinski ME. Optical Coherence Tomographic Elastography Technique for Measuring Deformation and Strain of Atherosclerotic Tissues. Heart (2004) 90(5):556–62. doi:10.1136/hrt.2003.016956
314. Chan RC, Chau AH, Karl WC, Nadkarni S, Khalil AS, Iftimia N, et al. OCT-based Arterial Elastography: Robust Estimation Exploiting Tissue Biomechanics. Opt Express (2004) 12(19):4558–457. doi:10.1364/opex.12.004558
315. Wang RK, Ma Z, Kirkpatrick SJ. Tissue Doppler Optical Coherence Elastography for Real Time Strain Rate and Strain Mapping of Soft Tissue. Appl Phys Lett (2006) 89(14):144103. doi:10.1063/1.2357854
316. Crecea V, Oldenburg AL, Liang X, Ralston TS, Boppart SA. Magnetomotive Nanoparticle Transducers for Optical Rheology of Viscoelastic Materials. Opt Express (2009) 17(25):23114–22. doi:10.1364/oe.17.023114
317. Li C, Huang Z, Wang RK. Elastic Properties of Soft Tissue-Mimicking Phantoms Assessed by Combined Use of Laser Ultrasonics and Low Coherence Interferometry. Opt Express (2011) 19(11):10153–63. doi:10.1364/oe.19.010153
318. Razani M, Mariampillai A, Sun C, Luk TWH, Yang VXD, Kolios MC. Feasibility of Optical Coherence Elastography Measurements of Shear Wave Propagation in Homogeneous Tissue Equivalent Phantoms. Biomed Opt Express (2012) 3(5):972–80. doi:10.1364/boe.3.000972
319. Nahas A, Bauer M, Roux S, Boccara AC. 3D Static Elastography at the Micrometer Scale Using Full Field OCT. Biomed Opt Express (2013) 4(10):2138–49. doi:10.1364/boe.4.002138
320. Song S, Huang Z, Wang RK. Tracking Mechanical Wave Propagation within Tissue Using Phase-Sensitive Optical Coherence Tomography: Motion Artifact and its Compensation. J Biomed Opt (2013) 18(12):121505. doi:10.1117/1.jbo.18.12.121505
321. Wang S, Larin KV. Optical Coherence Elastography for Tissue Characterization: a Review. J Biophoton (2015) 8(4):279–302. doi:10.1002/jbio.201400108
322. Zhu J, Qi L, Miao Y, Ma T, Dai C, Qu Y, et al. 3D Mapping of Elastic Modulus Using Shear Wave Optical Micro-elastography. Scientific Rep (2016) 6(1):1–9. doi:10.1038/srep35499
323. Song S, Le NM, Huang Z, Shen T, Wang RK. Quantitative Shear-Wave Optical Coherence Elastography with a Programmable Phased Array Ultrasound as the Wave Source. Opt Lett (2015) 40(21):5007–10. doi:10.1364/ol.40.005007
324. Zvietcovich F, Ge GR, Mestre H, Giannetto M, Nedergaard M, Rolland JP, et al. Longitudinal Shear Waves for Elastic Characterization of Tissues in Optical Coherence Elastography. Biomed Opt Express (2019) 10(7):3699–718. doi:10.1364/BOE.10.003699
325. Nahas A, Tanter M, Nguyen T-M, Chassot J-M, Fink M, Claude Boccara A. From Supersonic Shear Wave Imaging to Full-Field Optical Coherence Shear Wave Elastography. J Biomed Opt (2013) 18(12):121514. doi:10.1117/1.jbo.18.12.121514
326. Zhu J, Qu Y, Ma T, Li R, Du Y, Huang S, et al. Imaging and Characterizing Shear Wave and Shear Modulus under Orthogonal Acoustic Radiation Force Excitation Using OCT Doppler Variance Method. Opt Lett (2015) 40(9):2099–102. doi:10.1364/ol.40.002099
327. Nguyen T-M, Song S, Arnal B, Huang Z, O’Donnell M, Wang RK. Visualizing Ultrasonically Induced Shear Wave Propagation Using Phase-Sensitive Optical Coherence Tomography for Dynamic Elastography. Opt Lett (2014) 39(4):838–41. doi:10.1364/ol.39.000838
328. Qi W, Li R, Ma T, Li J, Kirk Shung K, Zhou Q, et al. Resonant Acoustic Radiation Force Optical Coherence Elastography. Appl Phys Lett (2013) 103(10):103704. doi:10.1063/1.4820252
329. Nguyen TM, Arnal B, Song S, Huang Z, Wang RK, O'Donnell M. Shear Wave Elastography Using Amplitude-Modulated Acoustic Radiation Force and Phase-Sensitive Optical Coherence Tomography. J Biomed Opt (2015) 20(1):016001. doi:10.1117/1.jbo.20.1.016001
330. Zhu J, Miao Y, Qi L, Qu Y, He Y, Yang Q, et al. Longitudinal Shear Wave Imaging for Elasticity Mapping Using Optical Coherence Elastography. Appl Phys Lett (2017) 110(20):201101. doi:10.1063/1.4983292
331. Zhu J, Yu J, Qu Y, He Y, Li Y, Yang Q, et al. Coaxial Excitation Longitudinal Shear Wave Measurement for Quantitative Elasticity Assessment Using Phase-Resolved Optical Coherence Elastography. Opt Lett (2018) 43(10):2388–91. doi:10.1364/ol.43.002388
332. Ambrozinski L, Song S, Yoon SJ, Pelivanov I, Li D, Gao L, et al. Acoustic Micro-tapping for Non-contact 4D Imaging of Tissue Elasticity. Sci Rep (2016) 6(1):38967. doi:10.1038/srep38967
333. Wang S, Larin KV. Shear Wave Imaging Optical Coherence Tomography (SWI-OCT) for Ocular Tissue Biomechanics. Opt Lett (2014) 39(1):41–4. doi:10.1364/ol.39.000041
334. Wang S, Lopez AL, Morikawa Y, Tao G, Li J, Larina IV, et al. Noncontact Quantitative Biomechanical Characterization of Cardiac Muscle Using Shear Wave Imaging Optical Coherence Tomography. Biomed Opt Express (2014) 5(7):1980–92. doi:10.1364/BOE.5.001980
335. Wang S, Larin KV. Noncontact Depth-Resolved Micro-scale Optical Coherence Elastography of the Cornea. Biomed Opt Express (2014) 5(11):3807–21. doi:10.1364/boe.5.003807
336. Zvietcovich F, Nair A, Ambekar YS, Singh M, Aglyamov SR, Twa MD, et al. Confocal Air-Coupled Ultrasonic Optical Coherence Elastography Probe for Quantitative Biomechanics. Opt Lett (2020) 45(23):6567–70. doi:10.1364/ol.410593
337. Pitre JJ, Kirby MA, Li DS, Shen TT, Wang RK, O'Donnell M, et al. Nearly-incompressible Transverse Isotropy (NITI) of Cornea Elasticity: Model and Experiments with Acoustic Micro-tapping OCE. Sci Rep (2020) 10(1):12983. doi:10.1038/s41598-020-69909-9
338. Pelivanov I, Gao L, Pitre J, Kirby MA, Song S, Li D, et al. Does Group Velocity Always Reflect Elastic Modulus in Shear Wave Elastography. J Biomed Opt (2019) 24(7):076003. doi:10.1117/1.jbo.24.7.076003
339. Kirby MA, Pelivanov I, Song S, Ambrozinski Ł, Yoon SJ, Gao L, et al. Optical Coherence Elastography in Ophthalmology. J Biomed Opt (2017) 22(12):1–28. doi:10.1117/1.jbo.22.12.121720
340. Alonso-Caneiro D, Karnowski K, Kaluzny BJ, Kowalczyk A, Wojtkowski M. Assessment of Corneal Dynamics with High-Speed Swept Source Optical Coherence Tomography Combined with an Air Puff System. Opt Express (2011) 19(15):14188–99. doi:10.1364/oe.19.014188
341. Wang S, Li J, Manapuram RK, Menodiado FM, Ingram DR, Twa MD, et al. Noncontact Measurement of Elasticity for the Detection of Soft-Tissue Tumors Using Phase-Sensitive Optical Coherence Tomography Combined with a Focused Air-Puff System. Opt Lett (2012) 37(24):5184–6. doi:10.1364/ol.37.005184
342. Ambrozinski L, Pelivanov I, Song S, Yoon SJ, Li D, Gao L, et al. Air-coupled Acoustic Radiation Force for Non-contact Generation of Broadband Mechanical Waves in Soft media. Appl Phys Lett (2016) 109(4):043701. doi:10.1063/1.4959827
343. Ramier A, Tavakol B, Yun SH. Measuring Mechanical Wave Speed, Dispersion, and Viscoelastic Modulus of the Cornea Using Optical Coherence Elastography. Opt Express (2019) 27(12):16635–49. doi:10.1364/OE.27.016635
344. Han Z, Li J, Singh M, Wu C, Liu C-h., Raghunathan R, et al. Optical Coherence Elastography Assessment of Corneal Viscoelasticity with a Modified Rayleigh-Lamb Wave Model. J Mech Behav Biomed Mater (2017) 66:87–94. doi:10.1016/j.jmbbm.2016.11.004
345. Jin Z, Zhou Y, Shen M, Wang Y, Lu F, Zhu D. Assessment of Corneal Viscoelasticity Using Elastic Wave Optical Coherence Elastography. J Biophotonics (2020) 13(1):e201960074. doi:10.1002/jbio.201960074
346. Liu HC, Abbasi M, Ding YH, Roy T, Capriotti M, Liu Y, et al. Characterizing Blood Clots Using Acoustic Radiation Force Optical Coherence Elastography and Ultrasound Shear Wave Elastography. Phys Med Biol (2021) 66(3):035013. doi:10.1088/1361-6560/abcb1e
347. Liu HC, Kijanka P, Urban MW. Acoustic Radiation Force Optical Coherence Elastography for Evaluating Mechanical Properties of Soft Condensed Matters and its Biological Applications. J Biophotonics (2020) 13(3):e201960134. doi:10.1002/jbio.201960134
348. Wang LV, Hu S. Photoacoustic Tomography: In Vivo Imaging from Organelles to Organs. Science (2012) 335(6075):1458–62. doi:10.1126/science.1216210
349. Hai P, Zhou Y, Liang J, Li C, Wang LV. Photoacoustic Tomography of Vascular Compliance in Humans. J Biomed Opt (2015) 20(12):126008. doi:10.1117/1.jbo.20.12.126008
350. Singh MS, Thomas A. Photoacoustic Elastography Imaging: a Review. J Biomed Opt (2019) 24(4):040902. doi:10.1117/1.jbo.24.4.040902
351. Hai P, Yao J, Li G, Li C, Wang LV. Photoacoustic Elastography. Opt Lett (2016) 41(4):725–8. doi:10.1364/ol.41.000725
352. Gao G, Yang S, Xing D. Viscoelasticity Imaging of Biological Tissues with Phase-Resolved Photoacoustic Measurement. Opt Lett (2011) 36(17):3341–3. doi:10.1364/ol.36.003341
353. Wadamori N. Non-restrained Measurement of Young’s Modulus for Soft Tissue Using a Photoacoustic Technique. Appl Phys Lett (2014) 105(10):103707. doi:10.1063/1.4893725
354. Liu Y, Yuan Z. Multi-spectral Photoacoustic Elasticity Tomography. Biomed Opt Express (2016) 7(9):3323–34. doi:10.1364/BOE.7.003323
355. Upputuri PK, Pramanik M. Recent Advances toward Preclinical and Clinical Translation of Photoacoustic Tomography: a Review. J Biomed Opt (2017) 22(4):41006. doi:10.1117/1.JBO.22.4.041006
356. Singh MS, Jiang H. Elastic Property Attributes to Photoacoustic Signals: an Experimental Phantom Study. Opt Lett (2014) 39(13):3970–3. doi:10.1364/OL.39.003970
357. Singh MS, Jiang H. Ultrasound (US) Transducer of Higher Operating Frequency Detects Photoacoustic (PA) Signals Due to the Contrast in Elastic Property. AIP Adv (2016) 6(2):025210. doi:10.1063/1.4942106
358. Zhao Y, Yang S, Chen C, Xing D. Simultaneous Optical Absorption and Viscoelasticity Imaging Based on Photoacoustic Lock-In Measurement. Opt Lett (2014) 39(9):2565–8. doi:10.1364/OL.39.002565
359. Wang Q, Shi Y, Yang F, Yang S. Quantitative Photoacoustic Elasticity and Viscosity Imaging for Cirrhosis Detection. Appl Phys Lett (2018) 112(21):211902. doi:10.1063/1.5021675
360. Scholz M, Noack V, Pechlivanis I, Engelhardt M, Fricke B, Linstedt U, et al. Vibrography during Tumor Neurosurgery. J Ultrasound Med (2005) 24(7):985–92. doi:10.7863/jum.2005.24.7.985
361. Lee W-N, Larrat B, Pernot M, Tanter M. Ultrasound Elastic Tensor Imaging: Comparison with MR Diffusion Tensor Imaging in the Myocardium. Phys Med Biol (2012) 57(16):5075–95. doi:10.1088/0031-9155/57/16/5075
362. Islam MT, Chaudhry A, Tang S, Tasciotti E, Righetti R. A New Method for Estimating the Effective Poisson's Ratio in Ultrasound Poroelastography. IEEE Trans Med Imaging (2018) 37(5):1178–91. doi:10.1109/tmi.2018.2792437
363. Righetti R, Ophir J, Srinivasan S, Krouskop TA. The Feasibility of Using Elastography for Imaging the Poisson's Ratio in Porous media. Ultrasound Med Biol (2004) 30(2):215–28. doi:10.1016/j.ultrasmedbio.2003.10.022
364. Chaudhry A, Kim N, Unnikrishnan G, Nair S, Reddy JN, Righetti R. Effect of Interstitial Fluid Pressure on Ultrasound Axial Strain and Axial Shear Strain Elastography. Ultrason Imaging (2017) 39(2):137–46. doi:10.1177/0161734616671713
365. Islam MT, Tasciotti E, Righetti R. Estimation of Vascular Permeability in Irregularly Shaped Cancers Using Ultrasound Poroelastography. IEEE Trans Biomed Eng (2020) 67(4):1083–96. doi:10.1109/tbme.2019.2929134
366. Drakonaki EE, Allen GM, Wilson DJ. Ultrasound Elastography for Musculoskeletal Applications. Br J Radiol (2012) 85(1019):1435–45. doi:10.1259/bjr/93042867
367. Bhatia KSS, Cho CCM, Tong CSL, Yuen EHY, Ahuja AT. Shear Wave Elasticity Imaging of Cervical Lymph Nodes. Ultrasound Med Biol (2012) 38(2):195–201. doi:10.1016/j.ultrasmedbio.2011.10.024
368. Strachinaru M, Bosch JG, van Dalen BM, van Gils L, van der Steen AFW, de Jong N, et al. Cardiac Shear Wave Elastography Using a Clinical Ultrasound System. Ultrasound Med Biol (2017) 43(8):1596–606. doi:10.1016/j.ultrasmedbio.2017.04.012
369. Zhou J, Zhan W, Chang C, Zhang J, Yang Z, Dong Y, et al. Role of Acoustic Shear Wave Velocity Measurement in Characterization of Breast Lesions. J Ultrasound Med (2013) 32(2):285–94. doi:10.7863/jum.2013.32.2.285
370. Palmeri ML, Wang MH, Rouze NC, Abdelmalek MF, Guy CD, Moser B, et al. Noninvasive Evaluation of Hepatic Fibrosis Using Acoustic Radiation Force-Based Shear Stiffness in Patients with Nonalcoholic Fatty Liver Disease. J Hepatol (2011) 55(3):666–72. doi:10.1016/j.jhep.2010.12.019
371. Golatta M, Schweitzer-Martin M, Harcos A, Schott S, Gomez C, Stieber A, et al. Evaluation of Virtual Touch Tissue Imaging Quantification, a New Shear Wave Velocity Imaging Method, for Breast Lesion Assessment by Ultrasound. Biomed Res Int (2014) 2014:960262. doi:10.1155/2014/960262
372. Deffieux T, Gennisson J-L, Bousquet L, Corouge M, Cosconea S, Amroun D, et al. Investigating Liver Stiffness and Viscosity for Fibrosis, Steatosis and Activity Staging Using Shear Wave Elastography. J Hepatol (2015) 62(2):317–24. doi:10.1016/j.jhep.2014.09.020
373. Castera L, Denis J, Babany G, Roudot-Thoraval F. Evolving Practices of Non-invasive Markers of Liver Fibrosis in Patients with Chronic Hepatitis C in France: Time for New Guidelines? J Hepatol (2007) 46(3):528–9. doi:10.1016/j.jhep.2006.12.002
374. Arnal B, Pernot M, Tanter M. Monitoring of thermal Ablation Therapy Based on Shear Modulus Changes: Shear Wave Thermometry and Shear Wave Lesion Imaging. In: 2010 IEEE International Ultrasonics Symposium (2010). p. 1522–5.
375. Tanter M, Fink M. Ultrafast Imaging in Biomedical Ultrasound. IEEE Trans Ultrason Ferroelect., Freq Contr (2014) 61(1):102–19. doi:10.1109/tuffc.2014.2882
376. Song P, Macdonald MC, Behler RH, Lanning JD, Wang MH, Urban MW, et al. Shear Wave Elastography on the GE LOGIQ E9 with Comb-Push Ultrasound Shear Elastography (CUSE) and Time Aligned Sequential Tracking (TAST). In: 2014 IEEE International Ultrasonics Symposium (2014). p. 1101–4.
377. Aristizabal S, Amador Carrascal C, Nenadic IZ, Greenleaf JF, Urban MW. Application of Acoustoelasticity to Evaluate Nonlinear Modulus in Ex Vivo Kidneys. IEEE Trans Ultrason Ferroelect., Freq Contr (2018) 65(2):188–200. doi:10.1109/tuffc.2017.2781654
378. Gennisson J-L, Aristizabal S. Nonlinear Shear Elasticity. In: Ultrasound Elastography for Biomedical Applications and Medicine (2018). p. 451–69. doi:10.1002/9781119021520.ch29
379. Caenen A, Pernot M, Peirlinck M, Mertens L, Swillens A, Segers P. An In Silico Framework to Analyze the Anisotropic Shear Wave Mechanics in Cardiac Shear Wave Elastography. Phys Med Biol (2018) 63(7):075005. doi:10.1088/1361-6560/aaaffe
380. Chen YL, Gao Y, Chang C, Wang F, Zeng W, Chen JJ. Ultrasound Shear Wave Elastography of Breast Lesions: Correlation of Anisotropy with Clinical and Histopathological Findings. Cancer Imaging (2018) 18(1):1–11. doi:10.1186/s40644-018-0144-x
382. Islam MT, Chaudhry A, Unnikrishnan G, Reddy JN, Righetti R. An Analytical Model of Tumors with Higher Permeability Than Surrounding Tissues for Ultrasound Elastography Imaging. J Eng Sci Med Diagn Ther (2018) 1(3):031006. doi:10.1115/1.4040145
383. Islam MT, Reddy JN, Righetti R. An Analytical Poroelastic Model of a Nonhomogeneous Medium under Creep Compression for Ultrasound Poroelastography Applications—Part I. J Biomechanical Eng (2019) 141(6):060902. doi:10.1115/1.4040604
384. Mariani A, Kwiecinski W, Pernot M, Balvay D, Tanter M, Clement O, et al. Real Time Shear Waves Elastography Monitoring of thermal Ablation: In Vivo Evaluation in Pig Livers. J Surg Res (2014) 188(1):37–43. doi:10.1016/j.jss.2013.12.024
385. Sapin-de Brosses E, Gennisson J-L, Pernot M, Fink M, Tanter M. Temperature Dependence of the Shear Modulus of Soft Tissues Assessed by Ultrasound. Phys Med Biol (2010) 55(6):1701–18. doi:10.1088/0031-9155/55/6/011
386. Venkatesh SK, Yin M, Ehman RL. Magnetic Resonance Elastography of Liver: Technique, Analysis, and Clinical Applications. J Magn Reson Imaging (2013) 37(3):544–55. doi:10.1002/jmri.23731
387. Bohte AE, Nelissen JL, Runge JH, Holub O, Lambert SA, de Graaf L, et al. Breast Magnetic Resonance Elastography: a Review of Clinical Work and Future Perspectives. NMR Biomed (2018) 31(10):e3932. doi:10.1002/nbm.3932
388. Davids M, Guérin B, vom Endt A, Schad LR, Wald LL. Prediction of Peripheral Nerve Stimulation Thresholds of MRI Gradient Coils Using Coupled Electromagnetic and Neurodynamic Simulations. Magn Reson Med (2019) 81(1):686–701. doi:10.1002/mrm.27382
389. Souchon R, Salomir R, Beuf O, Milot L, Grenier D, Lyonnet D, et al. Transient MR Elastography (T-MRE) Using Ultrasound Radiation Force: Theory, Safety, and Initial Experiments In Vitro. Magn Reson Med (2008) 60(4):871–81. doi:10.1002/mrm.21718
390. Grasland-Mongrain P, Souchon R, Cartellier F, Zorgani A, Chapelon JY, Lafon C, et al. Imaging of Shear Waves Induced by Lorentz Force in Soft Tissues. Phys Rev Lett (2014) 113(3):1–5. doi:10.1103/physrevlett.113.038101
391. Grasland-Mongrain P, Miller-Jolicoeur E, Tang A, Catheline S, Cloutier G. Contactless Remote Induction of Shear Waves in Soft Tissues Using a Transcranial Magnetic Stimulation Device. Phys Med Biol (2016) 61(6):2582–93. doi:10.1088/0031-9155/61/6/2582
392. Vappou J, Bour P, Marquet F, Ozenne V, Quesson B. MR-ARFI-based Method for the Quantitative Measurement of Tissue Elasticity: Application for Monitoring HIFU Therapy. Phys Med Biol (2018) 63(9):095018. doi:10.1088/1361-6560/aabd0d
393. Yuan L, Glaser KJ, Rouviere O, Gorny KR, Chen S, Manduca A, et al. Preliminary Assessment of One-Dimensional MR Elastography for Use in Monitoring Focused Ultrasound Therapy. Phys Med Biol (2007) 52(19):5909–19. doi:10.1088/0031-9155/52/19/012
394. Corbin N, Vappou J, Breton E, Boehler Q, Barbé L, Renaud P, et al. Interventional MR Elastography for MRI-Guided Percutaneous Procedures. Magn Reson Med (2016) 75(3):1110–8. doi:10.1002/mrm.25694
395. Chen J, Woodrum DA, Glaser KJ, Murphy MC, Gorny K, Ehman R. Assessment of In Vivo Laser Ablation Using MR Elastography with an Inertial Driver. Magn Reson Med (2014) 72(1):59–67. doi:10.1002/mrm.24891
396. Wu T, Felmlee JP, Greenleaf JF, Riederer SJ, Ehman RL. Assessment of thermal Tissue Ablation with MR Elastography. Magn Reson Med (2001) 45(1):80–7. doi:10.1002/1522-2594(200101)45:1<80::aid-mrm1012>3.0.co;2-y
397. Guenthner C, Sethi S, Troelstra M, Gorkum RJH, Gastl M, Sinkus R, et al. Unipolar MR Elastography: Theory, Numerical Analysis and Implementation. NMR Biomed (2020) 33(1):e4138. doi:10.1002/nbm.4138
398. Wu P-H, Aroush DR-B, Asnacios A, Chen W-C, Dokukin ME, Doss BL, et al. A Comparison of Methods to Assess Cell Mechanical Properties. Nat Methods (2018) 15(7):491–8. doi:10.1038/s41592-018-0015-1
399. Girardo S, Träber N, Wagner K, Cojoc G, Herold C, Goswami R, et al. Standardized Microgel Beads as Elastic Cell Mechanical Probes. J Mater Chem B (2018) 6(39):6245–61. doi:10.1039/c8tb01421c
400. Guck J. Some Thoughts on the Future of Cell Mechanics. Biophys Rev (2019) 11(5):667–70. doi:10.1007/s12551-019-00597-0
401. Hao Y, Cheng S, Tanaka Y, Hosokawa Y, Yalikun Y, Li M. Mechanical Properties of Single Cells: Measurement Methods and Applications. Biotechnol Adv (2020) 45:107648. doi:10.1016/j.biotechadv.2020.107648
402. Fu J, Pierron F, Ruiz PD. Elastic Stiffness Characterization Using Three-Dimensional Full-Field Deformation Obtained with Optical Coherence Tomography and Digital Volume Correlation. J Biomed Opt (2013) 18(12):121512. doi:10.1117/1.jbo.18.12.121512
403. Chin L, Curatolo A, Kennedy BF, Doyle BJ, Munro PR, McLaughlin RA, et al. Analysis of Image Formation in Optical Coherence Elastography Using a Multiphysics Approach. Biomed Opt Express (2014) 5(9):2913–30. doi:10.1364/BOE.5.002913
404. Leroux C-E, Palmier J, Boccara AC, Cappello G, Monnier S. Elastography of Multicellular Aggregates Submitted to Osmo-Mechanical Stress. New J Phys (2015) 17(7):073035. doi:10.1088/1367-2630/17/7/073035
405. Curatolo A, Villiger M, Lorenser D, Wijesinghe P, Fritz A, Kennedy BF, et al. Ultrahigh-resolution Optical Coherence Elastography. Opt Lett (2016) 41(1):21–4. doi:10.1364/ol.41.000021
406. Pokharel D, Wijesinghe P, Oenarto V, Lu JF, Sampson DD, Kennedy BF, et al. Deciphering Cell-To-Cell Communication in Acquisition of Cancer Traits: Extracellular Membrane Vesicles Are Regulators of Tissue Biomechanics. OMICS: A J Integr Biol (2016) 20(8):462–9. doi:10.1089/omi.2016.0072
407. Karimi R, Zhu T, Bouma BE, Kaazempur Mofrad MR. Estimation of Nonlinear Mechanical Properties of Vascular Tissues via Elastography. Cardiovasc Eng (2008) 8(4):191–202. doi:10.1007/s10558-008-9061-0
408. Sridhar M, Liu J, Insana MF. Elasticity Imaging of Polymeric media. J Biomech Eng (2007) 129(2):259–72. doi:10.1115/1.2540804
409. Banerjee B, Roy D, Vasu RM. Efficient Implementations of a Pseudodynamical Stochastic Filtering Strategy for Static Elastography. Med Phys (2009) 36(8):3470–6. doi:10.1118/1.3158808
410. Le Floc'h S, Ohayon J, Tracqui P, Finet G, Gharib AM, Maurice RL, et al. Vulnerable Atherosclerotic Plaque Elasticity Reconstruction Based on a Segmentation-Driven Optimization Procedure Using Strain Measurements: Theoretical Framework. IEEE Trans Med Imaging (2009) 28(7):1126–37. doi:10.1109/tmi.2009.2012852
412. Singh MS, Yalavarthy PK, Vasu RM, Rajan K. Assessment of Ultrasound Modulation of Near Infrared Light on the Quantification of Scattering Coefficient. Med Phys (2010) 37(7):3744–51. doi:10.1118/1.3456441
413. Singh MS, Rajan K, Vasu RM, Roy D. Quantitative Estimation of Mechanical and Optical Properties from Ultrasound Assisted Optical Tomography Data. J Biomed Opt (2012) 17(10):101507. doi:10.1117/1.jbo.17.10.101507
414. Hai P, Zhou Y, Gong L, Wang LV. Quantitative Photoacoustic Elastography in Humans. J Biomed Opt (2016) 21(6):66011. doi:10.1117/1.JBO.21.6.066011
415. Grasland-Mongrain P, Lu Y, Lesage F, Catheline S, Cloutier G. Generation of Shear Waves by Laser in Soft media in the Ablative and Thermoelastic Regimes. Appl Phys Lett (2016) 109(22):1–5. doi:10.1063/1.4968538
416. Ferraioli G, Parekh P, Levitov AB, Filice C. Shear Wave Elastography for Evaluation of Liver Fibrosis. J Ultrasound Med (2014) 33(2):197–203. doi:10.7863/ultra.33.2.197
417. Crombé A, Hurtevent-Labrot G, Asad-Syed M, Palussière J, MacGrogan G, Kind M, et al. Shear-wave Elastography Quantitative Assessment of the Male Breast: Added Value to Distinguish Benign and Malignant Palpable Masses. Br J Radiol (2018) 91(1082):20170676. doi:10.1259/bjr.20170676
418. Barr RG, Zhang Z. Shear-wave Elastography of the Breast: Value of a Quality Measure and Comparison with Strain Elastography. Radiology (2015) 275(1):45–53. doi:10.1148/radiol.14132404
419. Cantisani V, Lodise P, Grazhdani H, Mancuso E, Maggini E, Di Rocco G, et al. Ultrasound Elastography in the Evaluation of Thyroid Pathology. Current Status. Eur J Radiol (2014) 83(3):420–8. doi:10.1016/j.ejrad.2013.05.008
420. Gu J, Du L, Bai M, Chen H, Jia X, Zhao J, et al. Preliminary Study on the Diagnostic Value of Acoustic Radiation Force Impulse Technology for Differentiating between Benign and Malignant Thyroid Nodules. J Ultrasound Med (2012) 31(5):763–71. doi:10.7863/jum.2012.31.5.763
421. Magri F, Chytiris S, Capelli V, Alessi S, Nalon E, Rotondi M, et al. Shear Wave Elastography in the Diagnosis of Thyroid Nodules: Feasibility in the Case of Coexistent Chronic Autoimmune Hashimoto's Thyroiditis. Clin Endocrinol (2012) 76(1):137–41. doi:10.1111/j.1365-2265.2011.04170.x
422. Zhan J, Jin J-M, Diao X-H, Chen Y. Acoustic Radiation Force Impulse Imaging (ARFI) for Differentiation of Benign and Malignant Thyroid Nodules-A Meta-Analysis. Eur J Radiol (2015) 84(11):2181–6. doi:10.1016/j.ejrad.2015.07.015
423. Dong F-J, Li M, Jiao Y, Xu JF, Xiong Y, Zhang L, et al. Acoustic Radiation Force Impulse Imaging for Detecting Thyroid Nodules: a Systematic Review and Pooled Meta-Analysis. Med Ultrason (2015) 17(2):192–9. doi:10.11152/mu.2013.2066.172.hyr
424. Slapa RZ, Piwowonski A, Jakubowski WS, Bierca J, Szopinski KT, Slowinska-Srzednicka J, et al. Shear Wave Elastography May Add a New Dimension to Ultrasound Evaluation of Thyroid Nodules: Case Series with Comparative Evaluation. J Thyroid Res (2012) 2012:657147. doi:10.1155/2012/657147
425. Sebag F, Vaillant-Lombard J, Berbis J, Griset V, Henry JF, Petit P, et al. Shear Wave Elastography: a New Ultrasound Imaging Mode for the Differential Diagnosis of Benign and Malignant Thyroid Nodules. J Clin Endocrinol Metab (2010) 95(12):5281–8. doi:10.1210/jc.2010-0766
426. Szczepanek-Parulska E, Woliński K, Stangierski A, Gurgul E, Biczysko M, Majewski P, et al. Comparison of Diagnostic Value of Conventional Ultrasonography and Shear Wave Elastography in the Prediction of Thyroid Lesions Malignancy. PLoS One (2013) 8(11):e81532. doi:10.1371/journal.pone.0081532
427. Hu L, He NA, Xie L, Ye X, Liu X, Pei C, et al. Evaluation of the Perinodular Stiffness Potentially Predicts the Malignancy of Thyroid Nodules. J Ultrasound Med (2020) 39(11):2183–93. doi:10.1002/jum.15329
428. You J, Chen J, Xiang F, Song Y, Khamis S, Lu C, et al. The Value of Quantitative Shear Wave Elastography in Differentiating the Cervical Lymph Nodes in Patients with Thyroid Nodules. J Med Ultrason (2018) 45(2):251–9. doi:10.1007/s10396-017-0819-0
429. Veyrieres J-B, Albarel F, Lombard JV, Berbis J, Sebag F, Oliver C, et al. A Threshold Value in Shear Wave Elastography to Rule Out Malignant Thyroid Nodules: a Reality? Eur J Radiol (2012) 81(12):3965–72. doi:10.1016/j.ejrad.2012.09.002
430. Samir AE, Dhyani M, Anvari A, Prescott J, Halpern EF, Faquin WC, et al. Shear-wave Elastography for the Preoperative Risk Stratification of Follicular-Patterned Lesions of the Thyroid: Diagnostic Accuracy and Optimal Measurement Plane. Radiology (2015) 277(2):565–73. doi:10.1148/radiol.2015141627
431. Eby SF, Cloud BA, Brandenburg JE, Giambini H, Song P, Chen S, et al. Shear Wave Elastography of Passive Skeletal Muscle Stiffness: Influences of Sex and Age throughout Adulthood. Clin Biomech (2015) 30(1):22–7. doi:10.1016/j.clinbiomech.2014.11.011
432. Koo TK, Guo J-Y, Cohen JH, Parker KJ. Quantifying the Passive Stretching Response of Human Tibialis Anterior Muscle Using Shear Wave Elastography. Clin Biomech (2014) 29(1):33–9. doi:10.1016/j.clinbiomech.2013.11.009
434. Cortes DH, Suydam SM, Silbernagel KG, Buchanan TS, Elliott DM. Continuous Shear Wave Elastography: A New Method to Measure Viscoelastic Properties of Tendons In Vivo. Ultrasound Med Biol (2015) 41(6):1518–29. doi:10.1016/j.ultrasmedbio.2015.02.001
435. Aubry S, Risson J-R, Kastler A, Barbier-Brion B, Siliman G, Runge M, et al. Biomechanical Properties of the Calcaneal Tendon In Vivo Assessed by Transient Shear Wave Elastography. Skeletal Radiol (2013) 42(8):1143–50. doi:10.1007/s00256-013-1649-9
436. Chen X-M, Cui L-G, He P, Shen W-W, Qian Y-J, Wang J-R. Shear Wave Elastographic Characterization of Normal and Torn Achilles Tendons. J Ultrasound Med (2013) 32(3):449–55. doi:10.7863/jum.2013.32.3.449
438. Schmitt C, Hadj Henni A, Cloutier G. Characterization of Blood Clot Viscoelasticity by Dynamic Ultrasound Elastography and Modeling of the Rheological Behavior. J Biomech (2011) 44(4):622–9. doi:10.1016/j.jbiomech.2010.11.015
439. Mfoumou E, Tripette J, Blostein M, Cloutier G. Time-dependent Hardening of Blood Clots Quantitatively Measured In Vivo with Shear-Wave Ultrasound Imaging in a Rabbit Model of Venous Thrombosis. Thromb Res (2014) 133(2):265–71. doi:10.1016/j.thromres.2013.11.001
440. Mercado-Shekhar KP, Kleven RT, Aponte Rivera H, Lewis R, Karani KB, Vos HJ, et al. Effect of Clot Stiffness on Recombinant Tissue Plasminogen Activator Lytic Susceptibility In Vitro. Ultrasound Med Biol (2018) 44(12):2710–27. doi:10.1016/j.ultrasmedbio.2018.08.005
441. Zheng X, Ji P, Mao H, Hu J. A Comparison of Virtual Touch Tissue Quantification and Digital Rectal Examination for Discrimination between Prostate Cancer and Benign Prostatic Hyperplasia. Radiol Oncol (2012) 46(1):69–74. doi:10.2478/v10019-011-0026-3
442. Ahmad S, Cao R, Varghese T, Bidaut L, Nabi G. Transrectal Quantitative Shear Wave Elastography in the Detection and Characterisation of Prostate Cancer. Surg Endosc (2013) 27(9):3280–7. doi:10.1007/s00464-013-2906-7
443. Rouvière O, Melodelima C, Hoang Dinh A, Bratan F, Pagnoux G, Sanzalone T, et al. Stiffness of Benign and Malignant Prostate Tissue Measured by Shear-Wave Elastography: a Preliminary Study. Eur Radiol (2017) 27(5):1858–66. doi:10.1007/s00330-016-4534-9
444. Boehm K, Salomon G, Beyer B, Schiffmann J, Simonis K, Graefen M, et al. Shear Wave Elastography for Localization of Prostate Cancer Lesions and Assessment of Elasticity Thresholds: Implications for Targeted Biopsies and Active Surveillance Protocols. J Urol (2015) 193(3):794–800. doi:10.1016/j.juro.2014.09.100
445. Woo S, Kim SY, Lee MS, Cho JY, Kim SH. Shear Wave Elastography Assessment in the Prostate: an Intraobserver Reproducibility Study. Clin Imaging (2015) 39(3):484–7. doi:10.1016/j.clinimag.2014.11.013
446. Barr RG, Memo R, Schaub CR. Shear Wave Ultrasound Elastography of the Prostate. Ultrasound Q (2012) 28(1):13–20. doi:10.1097/ruq.0b013e318249f594
447. Correas J-M, Tissier A-M, Khairoune A, Vassiliu V, Méjean A, Hélénon O, et al. Prostate Cancer: Diagnostic Performance of Real-Time Shear-Wave Elastography. Radiology (2015) 275(1):280–9. doi:10.1148/radiol.14140567
448. Majeed W, Kalra P, Kolipaka A. Simultaneous Multislice Rapid Magnetic Resonance Elastography of the Liver. NMR Biomed (2020) 33(4):1–15. doi:10.1002/nbm.4252
US ultrasound
MR magnetic resonance
SW shear wave
ARF acoustic radiation force
ARFI acoustic radiation force imaging
SMURF spatially modulated ultrasound radiation force
SSI supersonic shear imaging
TOF time-of-flight
TTP time-to-peak
2D-F two-dimensional Fourier transform
LPVI local phase velocity imaging
AMUSE attenuation-measuring ultrasound shear wave elastography
E Young’s modulus
K Bulk modulus
ν Poisson’s ratio
|µ| real shear modulus
G* magnetic field gradient complex shear modulus
G′ shear storage modulus
G′′ shear loss modulus
T Stress tensor
S Deformation tensor
λ First Lamé’s coefficient
ω angular frequency
vs shear wave speed
αs shear wave attenuation
η shear viscosity
B0 static magnetic field in MRI
T1 longitudinal magnetisation regrowth time constant
T2 transverse magnetisation decay time constant due to spin-spin interaction
MRI magnetic resonance imaging
RF radiofrequency
MEGs motion encoding gradients
MRE magnetic resonance elastography
FID free induction decay
EPI echo planar imaging
SE spin echo
GRE gradient recalled echo
LFE local frequency estimation
MDEV multi-frequency dual-elasto-visco inversion
HMDI heterogeneous multifrequency direct inversion
FE finite element
T2* transverse magnetisation decay time constant due to spin-spin interaction and magnetic field inhomogeneity
TE echo time
TR repetition time
ϕp material porosity
p complex time harmonic pressure field
ρf pore fluid density
κ hydraulic conductivity
ρa apparent mass density
P0 tissue initial pressure
β thermal expansion coefficient
νL speed of sound
CP specific heat capacity at constant pressure
є strain
єA complex strain amplitude
δ phase delay
μa intrinsic absorption coefficient
ϕ spatial resolution of optical fluence
Keywords: viscoelasticity (linear), elastography, mechanical shear waves, ultrasound imaging, magnetic resonance imaging, optical imaging, optical coherence tomography, photoacoustic imaging
Citation: Li H, Flé G, Bhatt M, Qu Z, Ghazavi S, Yazdani L, Bosio G, Rafati I and Cloutier G (2021) Viscoelasticity Imaging of Biological Tissues and Single Cells Using Shear Wave Propagation. Front. Phys. 9:666192. doi: 10.3389/fphy.2021.666192
Received: 09 February 2021; Accepted: 04 June 2021;
Published: 21 June 2021.
Edited by:
Ivana Dusan Pajic-Lijakovic, University of Belgrade, SerbiaReviewed by:
Ivan Pelivanov, University of Washington, United StatesCopyright © 2021 Li, Flé, Bhatt, Qu, Ghazavi, Yazdani, Bosio, Rafati and Cloutier. This is an open-access article distributed under the terms of the Creative Commons Attribution License (CC BY). The use, distribution or reproduction in other forums is permitted, provided the original author(s) and the copyright owner(s) are credited and that the original publication in this journal is cited, in accordance with accepted academic practice. No use, distribution or reproduction is permitted which does not comply with these terms.
*Correspondence: Guy Cloutier, Z3V5LmNsb3V0aWVyQHVtb250cmVhbC5jYQ==
†These authors share first authorship
Disclaimer: All claims expressed in this article are solely those of the authors and do not necessarily represent those of their affiliated organizations, or those of the publisher, the editors and the reviewers. Any product that may be evaluated in this article or claim that may be made by its manufacturer is not guaranteed or endorsed by the publisher.
Research integrity at Frontiers
Learn more about the work of our research integrity team to safeguard the quality of each article we publish.