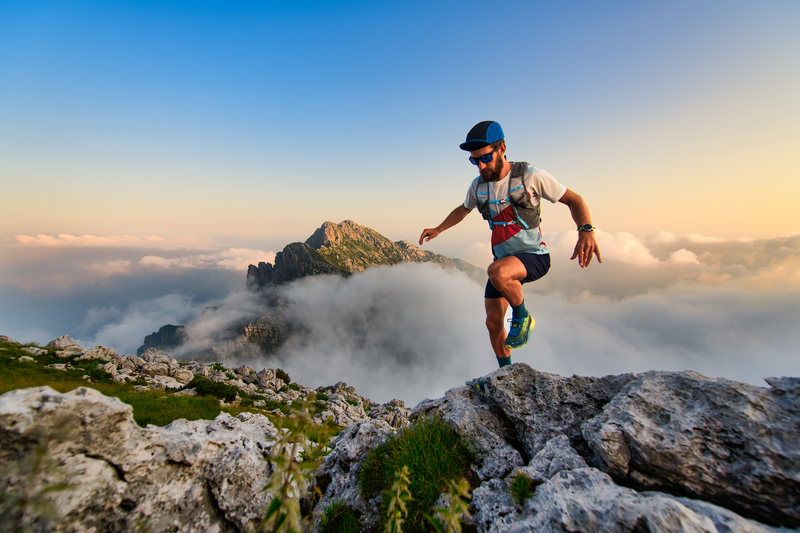
95% of researchers rate our articles as excellent or good
Learn more about the work of our research integrity team to safeguard the quality of each article we publish.
Find out more
ORIGINAL RESEARCH article
Front. Phys. , 17 May 2021
Sec. Social Physics
Volume 9 - 2021 | https://doi.org/10.3389/fphy.2021.664621
This article is part of the Research Topic The Physics of the Law: Legal Systems Through the Prism of Complexity Science View all 14 articles
Do two conventions of international environmental law necessarily endow the same word with the same meaning? A single counterexample is enough to answer in the negative: this is the case of the term “resource” in the United Nations Convention on the Law of the Sea (UNCLOS) and the Convention on Biological Diversity (CBD). Beyond this result, we tackle the questions, raised by the method of analysis implemented, about the semantics of legal texts, a source of interpretative flexibility but also of cognitive amalgamations and confusions of various types. A conceptual graph is associated with each proposition or sentence comprising the term “resource.” Some expressions, especially those of a deontic nature and noun phrases naming a group of interrelated entities or a fact, are encoded in nested graphs. The scope of a term is revealed by the neighbourhood of its uses. Neighbouring expressions, positioned along the paths of conceptual graphs, are ranked owing to their distance from the target expression. Then the neighbours the most contributing to the distributional meaning of the targets are classified in a coarse taxonomy, providing basic ontological traits to “resource” and related expressions in each convention. Although the two conventions rely on the same language, the weak overlap of their respective neighbourhoods of the term “resource” and associated expressions and their contrasted ontological anchorages highlight idiosyncratic meanings and, consequently, divergent orientations and understandings regarding the protection and conservation of resources, especially of living resources. Thus, the complexity of legal texts operates both in the gap between language semantics and cognitive understanding of the concepts used, and in the interpretative flexibility and opportunities for confusion that the texts offer but that the elementary operations of formalisation allow to deconstruct and clarify.
Fifteen years ago, in the continuation of the debate of ideas on the complexity of law and inflation of normative production [1–6] the question arose of defining legal complexity in a way that could be related to the exploration of large corpus of texts available on various online platforms1. The rise in power of network analysis and graph theory [7–11] quite naturally oriented research towards a relatively simple, intuitive and tractable approach which consists in identifying and analysing the networks induced by various types of citations or referencing between textual segments–article, laws, etc. [12, 13]. Physics (and ecology) shows that the structural properties of a complex system depend on the scale at which the system is observed, and thus conditions the choice of the relevant paradigm of analysis. Testing this idea, we highlighted the differentiated statistical properties of texts at the intra-article, article [14] and code level [15], and in a network of tens of codes of French law [16, 17].
Secondly, it became useful to analyse what simple lexicometric indicators can reveal about the emergence of a theme in international environmental law conventions [18]. The current international health situation gives particular relief to our analysis of the emergence of the health-environment theme, from the 90s, and more recently (roughly from 2010) of “One Health”–which links human health, animal health and environmental health, in the Rio conventions [19, 20].
However, these analyses remain far from the centre of the legal forge–at least in its literary expression: the meaning of the texts. Certainly the difficulty of semantic analysis, its disciplinary specificities and the diversity of existing approaches (in particular the logicist vs. distributional currents [21]), or even its links with syntactic analysis and discourse analysis, are a priori discouraging initiatives that would go in such direction2. Yet it is an entire continent that the complex systems approach now sets out to explore. Indeed, the considerable progress made over the past 30 years by Natural Language Processing [23–25] allows rapid and reliable access to the identification and characterisation of various grammatical units that make up lexemes, phrases, sentences, and even texts. Analyses of legal texts based on linguistic concepts have already been proposed ([26–29]; see also the special issue introduced by Robaldo et al. [30]) which open up perspectives that have not yet been explored. The multitude of relationships that it is possible to build between components of texts occurring at various levels of grammatical organisation of utterances deploys network structures. Graph theory, with its algebraic ramifications, offers a battery of more or less standard concepts and tools for analysing these networks.
At one of the most elementary levels of compositional semantics, the analysis proposed here sets out to answer a simple question: Do two conventions of international environmental law necessarily endow the same word with the same meaning? Let us note provisionally that if the answer is positive, then the textual glosses and the dialogues established in various collective arena can be conducted without change. No lexical ambiguity fosters risk of misunderstanding. A negative answer would mean that various legal streams make differentiated uses of the same lexicon. The understanding and use of legal texts then depend on the context of interpretation, or of the intention behind them.
Simple question in the sense that it only touches the lexical layer of texts, contributing to their meaning. This is a non-trivial question, however, insofar as the conventions use the same language, here English, and even belong to the same genre of discourse. Moreover, in addition to the production of appropriate evidence to support an answer, two additional objectives are pursued here: (a) to design an approach capable of highlighting idiosyncratic uses of terms from a restricted textual corpus; (b) identify a first essential property of legal texts that an analysis in context of the linguistic material can reveal, and outline the consequences on the normative level.
After setting the legal context of the study in Section Introduction, Section 3 UNCLOS, CBD and the Resource Issue presents the approach developed. It is inspired by the central hypothesis of distributional semantics (initially proposed by Firth [31]) according to which the meaning of a term or expression emerges from its use in context. By capturing very large textual corpora (composed of billions of words), this approach can legitimately claim to be statistically driven. With two texts in our pocket and at most a few dozen occurrences of the same term, we will not be able to avail ourselves of this advantage. The use of an expression in context amounts to identifying its immediate neighbourhoods in the corpus. We also propose to use the construction of conceptual graphs rather than raw sentences or syntax trees to identify these neighbourhoods. The use of these neighbourhoods constituting the meaning of a term in context is exposed in Section Encoding the Conventions Textual Data.
The neighbourhoods of the target term “resources” (and of associated expressions) as taken from the UNCLOS and CBD conventions, are described in Section Lexical Neighbourhoods and formalised as a lattice relying on a coarse taxonomy. Their comparison leads to favour the hypothesis of an idiosyncratic use of the term “resource” and its associated expressions in these two conventions. Section Meanings of “Resource” in UNCLOS and CBD discusses the limitations, advantages and avenues opened up by the analytical method. Then follow the implications which seem to emerge at the normative level from these results. Section Discussion concludes this exploratory study.
The question addressed by this study corresponds to a testable hypothesis. It would suffice to find a single term or expression that does not have the same meaning in two conventions to answer in the negative. Our candidate is the term “resource” and associated frozen expressions such as “natural resource,” “living resource,” etc. This term has a relatively high number of occurrences in several international environmental law conventions that regulate the management of resources (here “management” includes in particular access to, sharing of, proper management, conservation and protection of resources), but according to the perspectives and objectives specific to each of them. Resource management is a major subject of past, present and likely future tensions in our societies and their differentiated developments, for which the key players on the international scene and for international environmental law are States.
Regarding the conventions, we consider the United Nations Convention on the Law of the Sea (hereafter UNCLOS) and the Convention on Biological Diversity (CBD). There are several reasons for this choice. The UNCLOS defines the levels of territorial jurisdiction over the seas and oceans, regulates the passage of vessels, establishes the rules for access to marine resources as well as the conditions for the conduct of activities using these resources, in particular with regard to their impact on the marine environment and its non-living and living resources. The convention stipulates the duties of states in the conservation and management of the resources of the high seas, and establishes the architecture of international ocean governance that has prevailed in recent decades. The CBD regulates the rights and duties of States regarding the use, management, preservation, and conservation of biological resources, including genetic resources. It establishes the rules for cooperation between nations and for sharing the benefits derived from all forms of exploitation of these resources. The marine environment is regulated by CBD, just like other natural environments.
However, marine biodiversity and the environment of the high seas are exposed to increasing threats–physical and chemical modifications of water linked to climate change, various pollution, overexploitation of resources, loss of habitats, etc. directly or indirectly linked to human activities at sea but also on land. The growing needs for resources on a planetary scale, in particular mineral resources (like metals, rare earths) and in connexion with the energy transition [32], on the contrary, are pushing us to increasingly turn to the oceans [33–36] perceived as a kind of immense marine continent, relatively little explored, promise of largely unexploited stocks of mineral and living resources [37, 38]. At the same time, a growing harvest of scientific results warns about the risks that projects of large-scale deep sea mineral resource exploitation pose to living resources and marine biodiversity, and to ecosystems specific to the deep seabed, ocean ridges and seamounts [39]. Therefore, though the UNCLOS and CBD obey the same principles of public international law, the areas of marine activity that the two conventions cover differ, and their goals diverge.
After 10 years of discussions, and the mixed observation regarding the success of the conventions in achieving some objectives for the completion of which they had been designed and implemented, the international community initiated negotiations in 2018 aimed at establish a new binding treaty of the sea3 Under the aegis of the United Nations and under the UNCLOS, this treaty aims at “the conservation and sustainable use of marine biodiversity in areas beyond national jurisdiction.” Resource assessments, environmental baseline studies and assessment studies of the impact of deep seabed exploration and resources exploitation (still underdeveloped) on marine biology, are still limited [40], so that the negotiations around the new treaty must come to terms within a context of high scientific uncertainty [41], or even ignorance as to the vulnerability of the ecosystems that shelter the marine life.
We are therefore at a pivotal moment in the future of the oceans, at least as envisaged by international environmental law, which combines, and potentially, contradicts (at least) two aims of management of marine resources which will have major impacts both on the development of nations and on the marine environment, ecosystems, and life that resides there. In this context it is interesting to return to the source of these regulations and to analyze what these inaugural texts say about resources and how they approach them. Their original versions have shaped the contemporary form of regulation of their respective domains and the initial direction of their developments through Conferences of Parties or works of ad hoc scientific groups to this day.
The UNCLOS (Montego Bay, 10 December 1982) entered into force on 16 November 1994, and the CBD (Rio de Janeiro, 5 June 1992) on 29 December 1993. Both conventions count today 168 Parties4 The UNCLOS text includes a preamble, 320 articles divided into 17 Parts, plus 9 annexes. The CBD is shorter, with a preamble, 42 articles and 2 annexes. The annexes of the two conventions are excluded from our analysis.
Both conventions present sets of definitions gathered under “use of terms” titles. Only two of these sets introduce definitions including the term “resource,” say the UNCLOS Art. 1 of Part I Introduction, and Art. 133 of Part XI The Area; in the CBD, Art. 2 gives definitions related to the “resource.” Lexicology classically distinguishes definitions of the kind “x is a y” from those of the kind “x means y”: the first one is targeting the entity of the world designed by x, while the second one provides information on the term x and about the lexical environment in which the term is inserted, in relation to the elements from which it is distinguished. “Use of terms” sections of legal conventions provide definitions of the second kind. These definitions are worth being included in our analysis, but they are by no way able to render the richness of the meaning of the defined terms, to capture their relations with other concepts or notions, and do not allow a fine distinction of their denotative and connotative uses in context. In addition, they are based on a sort of latent ontology, neither explicit nor explicated in the conventions (this is not their role) but which can be postulated as a minimum representation common to the drafters of legal texts, and linking all the concepts and notions used. Finally, these definitions only concern a very limited number of terms, so that a broader approach must be deployed to look for possible idiosyncratic uses of terms.
For this purpose, we use all 105 occurrences of the term “resource” (singular or plural) in UNCLOS articles, and the 49 occurrences in CBD articles. We do not formalise, by means of a conceptual graph, each complete article in which one or more occurrences of “resource” are inserted, but only the sentences concerned, or even the only propositions having an autonomous meaning (for example when these propositions form a list of options or cases). These restrictions are justified insofar as they reduce the formalisation workload without having any impact on the way in which we define the neighbourhood of a term or of an expression as will be seen.
Conceptual graphs [42, 43] were designed to represent knowledge (assertions, rules or constraints established on domains, queries and answers, etc.) and to translate the various useful manipulations of knowledge in terms of rigorous mathematical operations on graphs [44]. Here we only use–and introduce–the basic properties of conceptual graphs5. Two types of vertices are distinguished–those representing concepts or notions [45] and those representing n-ary relations–connected by edges. In CGs, an edge reifies the link between a conceptual-type node and a relation-type node. For the purposes of our analysis it is enough to define a basic conceptual graph G as a 4-uple (C, R, E, λ) where (C, R, E) is a finite, undirected and bipartite multigraph (that is the possibility to have edges with the same end nodes6), C being the set of concept nodes, R the set of relation nodes, and E the set of edges; λ is a labelling function of the nodes and edges of G. The vocabulary V(G) of graph G is here defined as the set of labels of the only vertices representing entities or concepts (set C).
Each sentence or proposition where the word “resource” occurs is encoded in an elementary conceptual graph. GUNCLOS (respectively, GCBD) is the set of all disconnected elementary graphs formed from the concerned sentences or propositions of the UNCLOS (resp. CBD) convention, and VUNCLOS (resp. VCBD) its vocabulary. A sub-graph of an elementary graph is shown in Figure 1 as illustration. An elementary graph can be as simple as in Figure 1 or count tens of nodes, some of them connected along looping paths (the larger elementary graph we built encodes UNCLOS Art. 150 Policies relating to activities in the Area, and has 67 nodes and 79 edges).
Figure 1. Example of conceptual graph encoding the sentence “coastal States shall give due notice of conservation laws” (extracted from UNCLOS Art.62 §5) with concept node set C = {a, b, c, d}, relation node set R = {A, B, C}, edge set E = {e1, .., e6}, and labelling function λ(a) = ′coastal states′ λ(b) = ′due′, ..., λ(A) = ′shall give′, etc. The edge labels indicate their identifier and arity in parenthesis. V = {coastal states, due notice, conservation laws} (see text).
On Figure 1 concept nodes are represented in rectangles and relation nodes in ellipses. Conceptual nodes can only be connected with relation nodes, and relation nodes only with conceptual nodes. It is often useful to identify some words or expressions as being attributes (relation with label “ATTR”; the label of the attribute is in a hexagonal vertex) of concepts. In such case, the attribute and the concept it modifies are interpreted as a single concept (“due notice” in our example). Several expressions that are more or less “frozen” (linguistic stasis) have this form, whether they include the word “resource” (e. g., “living resources,” “natural resources”) or not (e g., “coastal state,” “country in development”).
When encoding a set of sentences or propositions, it appears that many concepts are occurring several times while the expressions of relations in natural language are much more variable and diverse. Because they are linking two or more concepts, relations are not really contributing to the meaning of a concept. Therefore, the meaning of a target concept like “resource” will be captured only from the neighbouring concepts (a notion to be defined more precisely in Section Lexical Neighbourhoods).
Conceptual graphs (CGs) represent knowledge in the form of a structure, articulating concepts and their relationships. In a textual corpus these articulations are expressed with the resources of natural language. CGs then make it possible to extract and represent the knowledge carried by the text–which is organised in a structure often qualified as “deep”–without depending on the singular linguistic form chosen to express this knowledge–form designated as “surface structure.”
For the purposes of our study, here we represent only sentences separated from each other. In doing so, a few regularities are observed which guide this rewriting of sentences in a graph, without however making them rigid rules. In CGs, concepts and relationships are represented by a distinct type of node. Two nodes of the same type cannot be directly linked.
Each simple noun phrase is represented by a concept type node. Complex noun phrases, formed by several nested noun phrases, are generally representable by a linear succession of conceptual and relational nodes. From a syntactic point of view, the notion of “resource” which is our target appears in noun phrases. The nature or quality of the resource is specified by the most general addition of an adjective, as in “biological resource” or “mineral resource.” The adjectival modification is represented via the relation “attribute” or in an equivalent way, by making the adjective the component of a frozen expression in a single node. Relationship nodes are most often occupied by verbs, possibly accompanied by an adverb treated as an attribute of the verb. We consider that the distance between an attribute and what it relates to–noun or verb–is zero.
However, sentences do not always have the simple syntactic structure as shown in Figure 1, due in particular to the use of anaphoric relations or of subordinate clauses in complex sentences. Expression in natural language makes frequent use of anaphoric relations and co-references, the resolution of which is essential for a good understanding of the text. This is for example the use of a pronoun which replaces a nominal antecedent, or the use of the referring word (pronoun, verb) which takes the place of a non-nominal antecedent, a source of more complex syntactic structures [47, 48]. Anaphora resolution is performed here by setting a relation node between the antecedent (the replaced term) and the other term syntactically associated to the pronoun. A frequent case is when a possessive pronoun backed by a concept (as in “its exclusive economic zone”) refers to the entity which includes the entity designated by this concept (in our example the “coastal state“). Whatever the overall syntactic structure of the sentence in which these two components appear, a relation is established between them with the label “of” (the result reads “exclusive economic zone of coastal state”). This procedure naturally modifies the paths linking concept nodes and their distance. Authorised here by the small volume of our textual corpus, this simple approach produces reliable results without resorting to complex NLP procedures.
Most expressions of natural language can be attached either to the type of relations, or to a subtype of entities, such as “actors,” “material resources,” “cognitive resources” or “norms.” But let us consider the term “pollution” defined in Art. 1 of UNCLOS as follows:
“‘pollution of the marine environment' means the introduction by man, directly or indirectly, of substances or energy into the marine environment, including estuaries, which results or is likely to result in such deleterious effects as harm to living resources and marine life, hazards to human health, hindrance to marine activities, including fishing and other legitimate uses of the sea, impairment of quality for use of sea water and reduction of amenities.”
“Pollution” is explicitly defined as an action (“introduction of ”) linking some actor (“man”) with material entities (“substances,” “energy”, “marine environment”), through several relations (“result in,” etc.). From this perspective, “pollution” should be represented as a relation node. Elsewhere, “pollution” can design the polluting substances, that is the concept of a material entities, not a relation, and should be an element of the concept set C. In fact, the definition above shows that the concept of pollution is indissolubly a subsystem composed of several other interrelated concepts. In such situation, we encapsulate the fully encoded subsystem in a concept-type node nested in the conceptual graph. The larger node with label “pollution” is in relation with some of its internal conceptual nodes (“man,” “substances,” etc.) if such description is in the sentences.
This way to proceed is relevant because legal norms establish deontic relations (“oblige,” “permit,” “prohibit,” etc.) with other actions (belonging to relation node set) or with concepts derived from actions (like pollution, activities, development, growth, conservation, etc.). Several other expressions—like “growth of international trade,” “fisheries industries,” “development of all countries”—obviously designate complex systems which precise components and relations are neither assignable nor specified. They are represented as an empty concept-like node (with the corresponding label) and nested in the conceptual graph.
It is worth commenting on the anaphoric relations carried by pronouns. Special care must be taken to encode pronouns as they replace a term, an expression or a noun phrase. A relation node is set between the antecedent (the replaced term) and the other term syntactically associated to the pronoun. A frequent case is when a possessive pronoun backed by a concept (as in ”its exclusive economic zone“) refers to the entity which includes the entity designated by this concept (in our example the “coastal state”). Whatever the overall syntactic structure of the sentence in which these two components appear, a relation is established between them with the label “of ” (the result reads “exclusive economic zone of coastal state”). This procedure naturally modifies the paths linking concept nodes and their distance.
At the end, each conceptual graph represents a sentence or proposition as it is interpreted, in the sense that: (a) words or expressions are classified as relations or concepts (a property that will ease the determination of any conceptual neighbourhood); and (b) all syntactic ambiguities are resolved (sentences being often decomposable in several distinct syntactic trees). The encoding of sentence in conceptual graphs is a task of knowledge extraction from natural language that is reputedly difficult to perform automatically (with in particular low recall performances; [49, 50]. Doing it manually provides the required data for achieving our objective and incidentally establishes some kind of standard reference for further work on computer-based knowledge extraction for texts.
Warned of these difficulties and equipped with the procedures described above, we choose to encode by hand each sentence mentioning the target term x as a conceptual graph . These elementary graphs are disconnected from each other as results from the building procedure (which is sentence-based). Their interconnection would be possible, for example based on the concept nodes that they have in common, but this would provide no additional information on the sought neighbourhoods of the target term.
We define the graph of a convention CONV related to term x, GCONV[x], as the set of the k = 1..K elementary graphs . These graphs encode the deep structure of the knowledge carried by the sentences in tree form (without cycle), and sometimes include cycles (for e.g., induced by anaphoric relations and co-references). In all cases, it is possible to follow the paths which pass through each noun phrase which includes the word “resource” or an associated frozen expression, and which, through verbs–or more generally the nodes of relations, link them to other concepts. The vocabulary of GCONV[x], V(GCONV[x]), is defined as the union of the vocabularies of its elementary graphs. GUNCLOS[resource] and GCBD[resource] gather 66 and 20 elementary graphs7, respectively. As we shall see, only a subset of their vocabularies are involved in defining the neighbourhood of the “resource” term in the UNCLOS and CBD conventions.
We define the neighbourhood of the target term x in a given convention as the set of labels of the conceptual nodes belonging to all paths passing through x in the elementary graphs associated with this convention. This definition clearly excludes labels of relation nodes from lexical neighbourhoods. It also leads to discard those terms that are not linked to the target term x through the knowledge representation. In particular terms that are not in the same proposition are not included in the lexical neighbourhood. An example is given in the sentence below, where terms neighbouring target term “resource” are in bold:
“Each Contracting Party [-3] shall take legislative, administrative or policy measures, as appropriate, with the aim [-2] that Contracting Parties [-1], in particular those that are developing countries, which provide genetic resources are provided access [-2] to and transfer [-2] of technology [-1] which makes use of those resources, on mutually agreed terms, including technology protected by patents and other intellectual property rights, where necessary, through the provisions of Articles 20 and 21 and in accordance with international law and consistent with paragraphs 4 and 5 below.” (CBD, Art. 16 Access to and Transfer of Technology, §2).
The number in brackets after each term indicates the distance to the occurrence of the target term (“resource”) to which it relates (a negative sign indicates a predecessor, a single plus a successor). The distance or rank only counts the conceptual nodes of the graph which separates the neighbouring term from the target term along the path. In other words, in a relation of type yRx where x and y are concepts and R a relation, the distance from y to x is −1, or y has rank −1 with regard to the target x.
The example illustrates an important aspect of our method: the neighbours of an expression are identified along paths in the conceptual graph, not as neighbours in the raw sentence as is often done. The neighbours are selected following knowledge representation links captured in conceptual graphs, not positional information provided by the sentence. For this reason, though “developing countries” is a conceptual node of the graph, and near from “genetic resources” in the sentence, it is not in its neighbourhood as it refers to “contracting parties” and as such, occurs on another path of the graph. “access,” “transfer” and “technology” logically refers to “those resources” not directly to “genetic resources,” hence the negative ranks (even if the demonstrative “those” indicates that these resources are genetic resources).
This approach conforms to the distributional hypothesis of semantics that assumes that terms occurring in similar contexts have similar meaning, but the underlying topology we use is defined from conceptual graphs representing knowledge embedded in a sentence or proposition. It is beyond the scope of this study to decide whether such distributional neighbourhoods authentically define the meaning of a word or phrase, or ultimately only allow the assessment of similarities of meanings (see Sahlgren [51], and references within). But in any case, the comparison of lexical neighbourhoods should allow us to detect possible idiosyncratic uses of the same term.
The above example also shows the importance of distinguishing between frozen expressions. The term “genetic resources” supposedly does not mean the same thing as “living resources,” “natural resources,” or “mineral resource.” An ontology could relate all these terms to the generic class of resources. However, it is obvious that the contexts of use of each of these expressions will differ greatly, depending on the uses that are made of these resources or on the measures and regulations implemented for their management. We can only compare neighbourhoods attached to the same expression or to expressions supposedly referring to the same concept.
Moreover, whether or not to use an expression in a convention is already informative on the field covered by the legal instrument. Likewise, and more significantly, the number of occurrences of an expression provides a first indicator of the lexical–and therefore conceptual–landscape in which the text constructs and moves. Optionally, this number of occurrences can be normalised by the length of the text (evaluated in number of words), then making it possible to compare occurrence densities (remember that the text of the UNCLOS is much longer than that of the CBD). We will therefore compare sets of target terms or expressions (those using the word “resource”) in order to better define the regulated domain, and sets of neighbouring expressions relating to each target term in each convention to detect possible idiosyncratic uses of terminology. We will also use information taken from the rank matrices which values indicate the number of occurrences of a neighbour expression at a given rank.
The use of a neighbour's rank (its distance from the target expression along the path) is justified with the idea that the more distant a term is, the less it contributes to the (distributional) meaning of the target. Another possible use is to identify frozen or semi-frozen expressions (such as for example “resources of the exclusive economic zone”) which appear frequently, or that themselves include frozen expressions, the phrases being often nested (as in “areas beyond national jurisdiction” which already has the acronym ABNJ in use, and now BBNJ for “biodiversity in ABNJ”). Indeed, the occurrence of an expression at a preferential rank from a target (which is a statistically detectable behaviour) suggests the presence of a frozen expression, at least in the analysed corpus.
Let FCONV be the set of the frozen expressions that include the term “resource” found in convention CONV (UNCLOS or CBD), with cardinality |FCONV|. NCONV[x] denotes the set of terms or expressions (labels of conceptual nodes) found in the neighbourhood of target expression x in the convention. We limit the set to terms with rank in the interval [−4, +4]. By construction, NCONV[x] is a subset of the vocabulary V(GCONV[x]) (see Section Encoding Sentences in CGs). From NCONV[x] we derive the set ÑCONV[x] by substituting, when necessary, each single word or word entering in an expression of the set for its lemmatized form (e.g., “states parties” –> “sate party”) using the NLTK lemmatizer [24] based on WordNet [52].
A rank/occurrence index is associated to each neighbour y of target x in convention CONV as given by:
where n(x) [resp. n(y)] is the number of occurrence of target term x (resp. neighbour term y) and rj(y) the rank of the jth occurrence of y. The index is built such that if y occurs only with rank +1 or−1 and whenever x occurs, then . The contribution of each occurrence of y to its rank/occurrence index is inversely proportional to its rank or distance to x: on the average, more distant terms have a lower rank/occurrence index than nearer terms. The rank/occurrence index provides an easy way to compare the contribution of each neighbour expression y to the distributional meaning of target expression x. It is used to identify the most import terms contributing to the meaning of x as it is used in the context of a given convention.
CBD and UNCLOS have four and seven expressions, respectively, using the word “resource”8, forming the following target expression sets:
TCBD ={biological resource, genetic resource, natural resource, resource}
TUNCLOS ={living resource, marine resource, mineral resource, natural resource, non-living resource, resource, resource deposit}
The difference in these two sets results from the difference in the domains covered by the two conventions, as could be expected. But it also suggests that the links or interactions between activities in one domain and the resources of the other domain, are not considered in the conventions. In particular, unless the “living resource” of UNCLOS can be interpreted as an expression synonym to the “biological resource” of CBD, the exploration and exploitation of mineral and/or non-living resource is not considered in relation to the “biological resources” in the UNCLOS; and reversely the protection or conservation of biological diversity is not envisioned in the CBD in relation with the activities regulated by UNCLOS.
For each target expression of the sets TCBD and TUNCLOS, we find the five nearest expressions defined as the neighbour expressions with highest rank/occurrence indexes (see equation 1) in a given convention. These nearest neighbours are listed in Table 1.
Table 1. Target expressions (1st column), convention (2d column), and neighbour expressions ranked (1 to 5) by decreasing rank/occurrence index (given above each expression). In bracket after the convention acronym, the number of neighbour expressions from which the nearest expressions are found.
The vocabulary formed by all expressions close to the targets, found in CBD (resp. in UNCLOS), comprises 81 (resp. 203) expressions or terms, forming 145 (resp. 488) pairs9 with one of the four (resp. seven) target-expressions. Thirty-seven of them appear in Table 1, indicating some partial overlap of the sets of neighbour expressions. ”Genetic resource“ and ”biological resource“ are used in conjunction with the most varied sets of neighbour expressions in the CBD (with, respectively, 51 and 27 neighbours). In this aspect, the expressions “resource” and “living resource” occupy the first places in the UNCLOS (with, respectively, 82 and 80 neighbours).
Most terms in Table 1 refers to actors (State, coastal State, country, Party, etc.), to the geographical zones or territories delimited on a jurisdictional basis (exclusive economic zone, the Area, seabed or subsoil–implied “of the Area” or “of/in the EEZ,” but also explicitly “jurisdiction” and “limit of national jurisdiction”) and to their rights (sovereign right, access). Most of the other expressions concern activities and capabilities, or some resources (polymetallic nodule, natural resource, mineral). These features indicate quite clearly that resources, whatever their type, are well-understood from the angle of law, in the legal genre of discourse.
Now consider the two target expressions shared by the two conventions. The term “natural resource” is very little used in CBD. Its only two neighbours are roughly the same as the two closest neighbours in UNCLOS (although 42 related expressions are identified in this convention): “sovereign right,” and “Sate” in CBD vs. “coastal State” in UNCLOS. The convergence of the distributional meaning of the expression “natural resource” between the two conventions is plausible, even if statistically poorly documented. The alignment, at least partial, of these meanings probably corresponds to an ontologically generic use of this term. In fact, CBD Article 15 §1 indirectly states that genetic resources are natural resources. For its part, UNCLOS Art. 56 §1 includes living and non-living resources under the natural resources, and Article 77 §4 adds mineral resources in the context of Part VI of the convention.
The sets of terms close to the target “resource” found in CBD and UNCLOS are disjointed. No similarities seem to emerge. The conceptual landscape built by the CBD around the term “resource” is based on the notion of actor (the State), of his role and powers. UNCLOS rather stresses on the activities, resources and the location where they both are or take place.
For the reasons explained at the beginning of this section, it is also important to see whether the expressions “biological resource” (CBD) and “living resource” (UNCLOS) designate the same concept or not. The main features of the conceptual landscape of the first expression concerns actors (“contracting party,” “state”) then some cognitive resource, the biological diversity (interpretable as a material resource) and norm (“sovereign right”). The notion of “living resource” in UNCLOS is centred on geographical sets (“EEZ,” “region,” “subregion”) and actors (“coastal State,” “State”). These sets are to be related to the Sates' jurisdiction or location. This comparison shows the importance of State actors in relation with biological and living resource, but diverge on the other determinants, UNCLOS insisting on a geographical mapping of resource locations or of actors' cooperation, while CBD focuses on actor's rights and compatibility of resource uses and preservation of biological diversity. However, this analysis mostly relies on an interpretation of the full sentences.
Is there a vector space where this kind of analysis can be done from the sole information of Table 1? A simple approach to word embedding is to associate a dimension of vector space with each term (here, each neighbouring expression). In such configuration, the target expression is represented by a vector in the subspace spanned by its neighbouring expressions, each value of the rank / occurrence index being a coordinate. The cardinality of the union of the sets of neighbouring lemmatized expressions of the two conventions (see Table 1) is given by:
Thus, the set of all neighbouring expressions defines a vector space of dimension 37, populated by 11 vectors, each representing a target expression (the same expression considered in two conventions is represented by two vectors). Since none of these vectors occupy exactly the same subspace, they are two-by-two orthogonal. Calculating the cosine of the angle between two vectors then provides no information on the similarity of the (distributional) meaning of the expressions they represent. Vector space reduction techniques10 that would allow these angles between word or expression vectors to be calculated are of little interest here: they are relevant for large sets of vectors. Moreover, after reduction, the new dimensions of the embedding space do not correspond to lexical elements and are therefore no longer interpretable.
We propose the following approach. Each neighbouring expression is attached to a generic ontological class. All of these classes is a kind of coarse taxonomy, which induces a partition of all 37 related expressions. We distinguish the following classes11: actor (“AC” label), material resource (“MR” label), cognitive resource (“CR” label) and process or activity (“PA” label). As the analysis relates to international law conventions, all entities relating to a type of legal norm (understood in a loose sense) are attached to a fifth class “norm” (label “NO”). The class relevant to each expression is given in Table 1.
No longer considering neighbouring expressions but their classes, Table 1 corresponds to another matrix: each row is a target expression in the context of a convention, and each column indicates the class of an expression of its lexical neighbourhood. The corresponding mathematical structure is a lattice, similar to those used in Formal Concept Analysis (FCA [54–56]). The lattice presents a double nested hierarchy established between the 11 target expressions (or “objects” in the FCA language) and the five classes (“attributes” in FCA) to which the neighbouring expressions belong. Some line diagrams representing this lattice are presented in Figure 2. Any target expression of a vertex which is on a descending path from a vertex with an attribute (class) have this attribute (and is an element of the extent of the attribute). Conversely, any attribute of a vertex lying on an ascending path starting from a target expression, is the class of one of the target's neighbour expressions (and is an element of the intent of the expression). To simplify the figures, the reduced representation of the lattice is used here: class (resp. target expression) labels are given only in the node occupying the higher (resp. lower) position they appear in the hierarchy (therefore, some nodes do not have an apparent label). The lattice immediately shows that none of the four attributes is an attribute of all target expressions, and that none of the target expressions collect all the attributes in its profile (set of its attributes).
Figure 2. Reduced representation (see text) of the expressions/classes lattice. (Top) Part of the lattice linked with the CBD “biological resource” expression (label BR_B); (bottom) Part of the lattice linked with the UNCLOS “living resource” expression (label LR_S). Labels combine the final B (from “biodiversity”) for CBD or S (from “sea”) for UNCLOS (sea) and the following sub-labels: GR, genetic resource; MaR, marine resource; MiR, mineral resource; NLR, non-living resource; NR, natural resource; R, resource; RD, resource deposit. Generic ontological classes are “actor” (AC label), “material resource” (MR label), “cognitive resource” (CR label), “process or activity” (PA label), and “norm” (NO label). Grey nodes and blue dashed links do not belong to the sub-lattice (but to the overall lattice) (figures built with the free software Concept Explorer 1.2; [57]).
Let us go back to the comparison of the terms “biological resource” of CBD and “living resource” of UNCLOS. The two sub-lattices linked to each of these targets are highlighted in Figure 2. Note first that the living resource and non-living resource of UNCLOS have, in this rough taxonomy, the same profile (they occupy the same vertex). This profile (actor AC and material resource MR) is a subset of the biological resource profile of the CBD, the latter also requesting the classes “norm” (NO) and “process and activity” (PA) in its lexical neighbourhood. Considering that we have restricted these neighbourhoods to the only 5 expressions with highest rank/occurrence indices, this difference between profiles is a significant feature of the semantic difference of the two expressions: the biological resources of the CBD cannot be assimilated. to the living resources of UNCLOS. Moreover, under the aspect of this taxonomy of neighbours, biological resources dominate the three other kinds of resources (“genetic resources,” “natural resources,” and “resources”) regulated by the CBD. Surprisingly, it is the “resource deposit” of UNCLOS which presents the same profile as “biological resources” of CBD while dominating “non-living resource” (and its paired expression “living resource”) and “resource,” expressions used in UNCLOS.
The sub-lattices corresponding to CBD “resource” (label R_B) and UNCLOS “resource” (label R_S) are exhibited on Figure 3. On the CBD diagram, “resource” is subsumed by “biological resource,” and have a larger profile (subsumes) than “natural resource.” In UNCLOS, “resource deposit” subsumes “resource.” Both CBD and UNCLOS “resource” have the attributes “process and activity” and “norm.” CBD “resource” adds the “actor” attribute while UNCLOS “resource” adds “material resource.” It must be kept in mind that this kind of subsumption relationships (or hyponym-hypernym relationship) is only valid in the context set by the coarse taxonomy used in this study. These differences emerging from the analysis of the distributional meaning established on the basis of a somewhat minimalist neighbourhood of expressions, they apparently express very distinct cognitive orientations as to what are resources, for the one or the other of these conventions.
Figure 3. (Top) Part of the lattice linked with the CBD “resource” expression (label R_B); (bottom) Part of the lattice linked with the UNCLOS “resource” expression (label LR_S). Reduced representation, see Figure 2 (figures built with the free software Concept Explorer 1.2; [57]).
The sub-lattice associated to the attribute “norm” is shown in Figure 4. The extent of “norm” is the union of the sets {“resource,” “resource deposit,” “natural resource”} of UNCLOS expressions and {“natural resource,” “resource,” “biological resource”} of CBD expressions. All other target expressions, “genetic resource” from CBD, “living resource,” “non-living resource,” “mineral resource,” and “marine resource” from UNCLOS, are not connected to the “norm” class in this simple taxonomy.
Figure 4. Part of the lattice linked with the attribute “norm” (label NO). Reduced representation, see Figure 2 (figure built with the free software Concept Explorer 1.2; [57]).
The link with the concepts attached to normativity is made in relation to the relatively general expressions involving the resources, rather than with their derivations of a more technical or specialised character.
Where has the search for a plausible answer to the original question led us? The experimental study argues for a notable and observable difference in the meaning of the same expression in two conventions of international environmental law. Legal language is not free from internal lexical idiosyncrasies, within the legal genre itself. Definitions are not sufficient to contain the meaning of legal terms or expressions. Distributional semantics provides complementary analysis tools, sensitive to the different contexts of use of these expressions. This result could moreover constitute only the first cog in a progression showing that the emergence of meaning, going up the levels of segmentation of texts until the constitution of a legal discourse, accumulates epistemological divergences between distinct legal currents.
Certainly this first conclusion needs to be confirmed on the basis of a more extensive textual corpus and diversified sources of rights. The analytical method outlined here can be replicated and supported by the use of various NLP and knowledge extraction tools. However, some features and some consequences of our analysis deserve further discussion.
Objectively, the description of the distributional meanings of the term “resource” and associated expressions rests on a statistically fragile basis. This situation is not a flaw in the approach but rather reflects the essential condition for analysing small corpora. The study made it possible to explicitly describe the contexts of use of each target expression and their contributions to its distributional meaning. As such, the results are likely to warn against any approach to normative texts which would dispense with a reflection on the variability of the meaning of words and on the dependence of this meaning on the regulatory context, at the risk of misinterpreting texts and depart from the intentions of legislators. The differentiated meanings of the same word used in different conventions reveal blind spots in international environmental law that need to be addressed.
These observations also have implications for the methodological aspect of the analyses. Any physical or informational measure (e.g., posterior probabilities, information functions, entropy) based on the frequency of occurrence of phrases or words is potentially affected. Indeed, counting of occurrences implicitly presupposes that the meaning attributed to an expression does not vary in the corpus. This hypothesis is not always valid. In these shifts in meaning, legal and political cultures specific to the regulated field and to the agents who design and promote legal instruments are expressed. The estimate of the frequencies of occurrences and the derived statistical measures remain valid, but on condition that they are applied to sets of semantically or ontologically homogeneous utterances, sets whose limits are traceable via the analysis of the deep structures of language and identification of the professional or “epistemological affiliation” of the authors.
On a technical level, the purpose of using conceptual graphs is generally to build up a knowledge base that can then be queried (to answer questions or produce new knowledge) via machines. Our posture is different: the work of formalising legal proposals, sentences or articles via conceptual graphs creates the conditions for an interrogation in direct contact with the legal matter (data), on mechanisms, artifices and techniques–implicit or explicit, intentional or unconscious, known or hidden–used by ”the legislator“ in the production of normative texts. Even if the theory of conceptual graphs cannot claim the universality of its capacities to transcribe any text into natural language and therefore presents limits of applicability, the formalisation exercise offers the opportunity to explain a part of the latent cognitive options which govern the choice of expressions in natural language and their conceptual underpinning. In this process, the nature of these revealed choices makes it possible to question the clarity and distinction of the concepts used and, admittedly a more adventurous steps, to try to understand the consequences of these choices.
The language used by the various international conventions is the same: for example, the English versions of the textual corpus. The lexicon, except for any technical terms specific to each legal (or related scientific) field, is also the same. But the use of certain key terms is differentiated according to conventions. A term appears in a convention in one or two types of occurrence: (a) as a word or part of a phrase in sentences or clauses; (b) as an entry of a definition. A definition is a short text, usually presented in intention, and positions itself between hypo and hyper-specificity. Thus, regardless of the method used, the comparison of the definitions by two conventions of the same given term, provides little insight into the analysis. On the other hand, the notional context in which the term is inserted through its uses is rich in lessons. If the notional contexts of use of a term in two conventions differ significantly as we have seen, the hypothesis of idiosyncratic conceptions attached to each convention is necessary. Such variations of the meaning of a term convey a semantic meaning specific to a given text.
But the analysis shows yet another thing. The terms of the notional neighbourhood suitable for the use of a target term in a convention can be related to a taxonomy, as we have done. However, a comparison shows a disparity in the ontological anchoring of expressions more or less frozen around the same central term (here the term “resource”). Belonging to a community of lexical expressions therefore in no way guarantees an alignment of the ontological bases for the design of the signified things. The ontological anchors of terms are established implicitly and along with a quasi-fortuitous lexical choice of particular terms in the development of legal discourse 12.
The text of a convention finalises a process of consultation and negotiation between actors (delegations) duly mandated and having not only different aims, but also different implicit knowledge or backgrounds (this also between members of the same delegation or group). However, this same text is also the starting point for new phases of negotiations, amendments or extensions. It constitutes the linguistic and cognitive reference for these developments carried out during conferences of the Parties or brought by ad hoc working groups. Even if, as we can see, the corpus of texts produced during these developments and attached to the source convention is enriched with new notions, the base on which these notional expansions necessarily rest, remains the inaugural notional and relational landscape established by the convention.
While staying mostly confined to the ”cognitive cone“ projected by the convention, the subsequent work carried out under its aegis reinforces (in the sense of learning) the significance of this landscape, freezing its contours and internal structuring. The use of a particular expression that was specific in the beginning, in a distinctive context, becomes idiosyncratic. Its fictitious neighbourhood is fully functioning, at the cost of increasing relegation in an implicit and unthinkable context, therefore sheltered from possible questioning. Thus, the normativity of the convention is intentionally expressed in the legal instruments that it establishes, but also, and in a latent and perhaps more profound way, in the structuring of the interrelationships between concepts that it explicitly invokes and whose idiosyncratic meanings are made to be reproduced and to persist over time.
The follow-up to the negotiations on the new sea treaty shows the difficulty of reaching a consensus capable of both meeting the contrasting expectations of States and of building a new governance of the oceans [58] while preserving some older institutional structures, and to produce an effectively binding and efficient instrument, responding to the urgent need for BBNJ regulation [59]. On the level of words and concepts alone, the terminology of the treatise has also been debated [60], but the most remarkable fact is the shift towards a new lexical set linked to “resources.” Indeed, in the “Revised draught text of an agreement under the United Nations Convention on the Law of the Sea on the conservation and sustainable use of marine biological diversity of areas beyond national jurisdiction”13, after exclusion of expressions “financial resources” and “human resources,” “marine genetic resources” glean about 90% of the occurrences (more than 70 occurrences), against about 10% for the term “resources,” and a single occurrence of the term “biological resources.”
It will be understood: the new treaty regulates marine genetic resources in the ABNJ. All aspects of the management of other types of resources, especially living or biological resources–not even to speak more prosaically of marine life–are left to the discretion of earlier treaties. No reinforcement of an ontological anchoring of the concepts used is made explicit14. The regulatory framework for these other resources and for activities having an impact on these resources remains that set by the UNCLOS and CBD and their Conferences of the Parties, with their terminological and conceptual dissonances, or blind spots.
The abandonment of the development of a rigorous conceptual framework that can support the normative discourse, in favour of lexical choices emerging in a fortuitous way from the development of the text may constitute the price to pay for obtaining a soft consensus around a treaty, an additional piece to a kind of “diplomatic” law [61]. However, the deleterious and irreversible effects on living resources, marine biodiversity and marine life, which an ineffective law would allow to slip through its nets, should not be added to this bill.
The analysis of expressions including the term “resource” in the CBD and UNCLOS, shows that the two conventions do not use these expressions in the same conceptual landscape. In this sense, they associate them with different meanings. The meaning of an expression is established according to the distributional hypothesis that the meaning of a word mainly emerges from the lexical environment in which it is inserted, from its use in a particular context. Rather than considering the simple alignment of words, we go here through a formalisation of sentences or propositions in the form of conceptual graphs, a step which imposes, among other things, to remove syntactic ambiguities. The neighbourhood of a target expression is then extracted along the paths of the graph which pass through the vertex whose label is this expression. We also take into consideration the distance of each neighbouring concept or notion to the target, in order to penalise the contribution of the most distant expressions in the distributional meaning of the target expressions.
The comparison of the rank/occurrence matrices of the neighbours then makes it possible to evaluate the similarity or disparity of the distributional meanings of the expressions frozen with the word “resource.” For this purpose, only the most contributory expressions to the target meaning are retained. The diversity of neighbouring expressions then requires their classification in a partition induced by a coarse taxonomy (with only five classes: actors, material resources, cognitive resources, norms, processes and activities). The neighbourhood comparison structure is a lattice that reveals relationships of subsumption or non-comparability between target expressions considered in the context of each convention.
Beyond highlighting idiosyncratic uses of expressions linked to the notion of resource in international law conventions, the developed method is potentially applicable to a large set of lexical entries. It also shows the disparity in the ontological anchors of lexically similar expressions in legal texts, anchors that are both implicit and constructed along with a random lexical choice of particular terms in the development of legal discourse. The normativity of conventions is then expressed at the lexical level in the reinforcement, reproduction and persistence of these distributional meanings and of their fortuitous ontological basis.
Publicly available datasets were analysed in this study. The data include the texts of the two conventions available on their respective sites (CBD: https://www.cbd.int/; UNCLOS: https://www.un.org/depts/los/convention_agreements/texts/unclos/unclos_e.pdf).
The author confirms being the sole contributor of this work and has approved it for publication.
With funding from United Kingdom Research and Innovation (UKRI) Global Challenges Research Fund (GCRF) One Ocean Hub (Grant Ref: NE/S008950/1).
The author declares that the research was conducted in the absence of any commercial or financial relationships that could be construed as a potential conflict of interest.
I would like to thank professors P. Vivo, D. M. Katz, and J. B. Ruhl for giving me the opportunity to present this work within the framework of the conference The Physics of Law, November 12–13, 2020. The manuscript has been greatly improved thanks to discussions with Dr C. Lajaunie (environmental lawyer, INSERM - France), for which I am very grateful. My thanks also to Prof. E. Morgera (SCELG, University of Strathclyde) PI of the One Ocean Hub UKRI project, for the possibility of taking part into an international project on the oceans. I thank the two anonymous reviewers for allowing me to improve this manuscript through comments which represented a challenge that I had to take up.
1. ^Like the European Eur Lex platform https://eur-lex.europa.eu/homepage.html, the French Legifrance https://www.legifrance.gouv.fr/, US codes at the Legal Information Institute of Cornell Law School https://www.law.cornell.edu/uscode/text, the IUCN gateway to environmental law ECOLEX https://www.iucn.org/theme/environmental-law/resources/ecolex, the UN Treaty collection https://treaties.un.org/, WTO legal texts https://www.wto.org/english/docs_e/legal_e/legal_e.htm, just to cite a few of them.
2. ^“The little existing research on legal language suggests that, more than by a specialized vocabulary, it is characterized by overly complex sentences, the overuse of passives, whiz-deletion and unclear pronoun reference, archaic and misplaced prepositional phrases, and its own set of articles and demonstrative pronouns. The historical development of legal language is unique, paralleling but independent of the development of the rest of English. Legal language is both the medium of communication and the primary tool of the legal profession, and is powerful because it carries the force of law.,” cited from Crandall and Charrow [22].
3. ^See Available online at: https://www.un.org/bbnj/content/background.
4. ^See the UN Treaty Collection, chap. XXI for UNCLOS and chap. XVII for CBD at https://treaties.un.org/.
5. ^In particular, we will adopt a definition of a “vocabulary” that does not conform to that used for conceptual graphs, but simpler and better suited to our needs; where appropriate we will also use directed graphs (oriented edges).
6. ^Since the CGs are multigraphs some application may require modelling them as weighted graph (see e g., Bellingeri et al. [46]). However, the sentence-by-sentence analysis performed here does not require resorting to it.
7. ^Graphs containing only the expressions “human resources” or “financial resources” are omitted.
8. ^The two expressions ≪financial resource≫ and ≪human resource≫ are discarded.
9. ^For example, the pair “natural resource” (target expression) and “exclusive economic zone” (rank +2) appearing from UNCLOS Art. 56 where are mentioned the “natural resources (...) of seabed”–(under understood) “of the exclusive economic zone,” the latter indication coming from the beginning of the article.
10. ^Like singular value decomposition.
11. ^This taxonomy is inspired by the meta-model developed for the modelling of socio-ecological systems by Sibertin-B1anc et al. [53] to which we add here the “norm” class.
12. ^It is not in the scope of this study to know if this state of affairs is a good or a bad, a defect or a quality.
13. ^See Available online at: https://digitallibrary.un.org/record/3847798?ln=en (accessed January 15, 2021).
14. ^Except for ”marine genetic resources“ which are the subject of a definition in the draft Article 1 ”use of terms" of the treaty (with the reservations made in Section UNCLOS, CBD, and the Resource Issue on any definition).
1. Schuck PH. Legal complexity: some causes, consequences, and cures. Duke Law J. (1992) 42:1–52. doi: 10.2307/1372753
2. White M. Legal complexity and lawyers' benefit from litigation. Int Rev Law Econ. (1992) 12:381–3. doi: 10.1016/0144-8188(92)90016-K
4. Conseil d'Etat. Rapport Public 2006 - Sécurité Juridique et Complexité du Droit, Jurisprudence et Avis de 2005. Paris: Etudes et Documents du Conseil d'Etat, n. 57 (2006).
5. Doat M, Le Goff J, Pédrot PH. Droit et Complexité – Pour une Nouvelle Intelligence du Droit Vivant. Rennes: Presse University (2007).
6. Ruhl JB. Law's complexity: a primer. Geor State Univ Law Rev. (2008) 24:885–912. Available online at: https://readingroom.law.gsu.edu/gsulr/vol24/iss4/9
7. Jungnickel D. Graphs, Networks and Algorithms. Algorithms and Computation in Math. Vol. 5. Berlin: Springer (1999). doi: 10.1007/978-3-662-03822-2
8. Watts DJ. Small Worlds: The Dynamics of Networks between Order and Randomness. Princeton, NY: Princeton University Press (2003).
9. Newman M, Barabási AL, Watts JD (eds). The Structure and Dynamics of Networks. Princeton, NJ: Princeton University Press (2006).
10. Brandes U, Erlebach T. Network Analysis - Methodological Foundations. Berlin: Springer (2005). doi: 10.1007/b106453
12. Bourcier D, Mazzega P. Toward measures of legal complexity. In: Artificial Intelligence and Law, Stanford Law School. New York, NY: ACM Press (2007). p. 211–5.
13. Bommarito MJ, Katz DM. A mathematical approach to the study of the United States code (March 25, 2010). Phys A. (2010) 389:4195–200. doi: 10.1016/j.physa.2010.05.057
14. Bourcier D, Mazzega P. Codification law article graphs. In: Lodder AR, Mommers L, editors. Legal Knowledge Information Systems. Amsterdam: IOS Press (2007). p. 29–38.
15. Boulet R, Mazzega P, Bourcier D. Network analysis of the french environmental code. In: Casanovas P, editor. AI Approches to the Complexity of Legal Systems. Heidelberg: LNASpringer (2010). doi: 10.1007/978-3-642-16524-5_4
16. Boulet R, Mazzega P, Bourcier D. A network approach to the french system of legal codes- part i: analysis of a dense network. Artif Intell Law. (2011) 19:333–55. doi: 10.1007/s10506-011-9116-1
17. Boulet R, Mazzega P, Bourcier D. Network approach to the French system of legal codes. Part II: the role of the weights in a network. Artif Intell Law. (2018) 26:23–47. doi: 10.1007/s10506-017-9204-y
18. Lajaunie C, Mazzega P. Mining CBD. Braz J Int Law. (2016) 13:277–92. doi: 10.5102/rdi.v13i2.4058
19. Lajaunie C, Mazzega P. One health and biodiversity conventions. The emergence of health issues in biodiversity conventions. IUCN Acad Environ Law J. (2016) 7:105–21. Available online at: http://www.iucnael.org/en/e-journal/current-issue-
20. Lajaunie C, Mazzega P, Boulet R. Health in biodiversity-related conventions: analysis of a multiplex terminological network (1973-2016). In: Chen SH, editor. Big Data in Computational Social Science and Humanities. Springer (2018). p. 165–82. doi: 10.1007/978-3-319-95465-3_7
21. Boleda G, Herbelot A. Formal distributional semantics: introduction to the special issue. Comput Linguist. (2016) 42:619–35. doi: 10.1162/COLI_a_00261
22. Crandall J, Charrow VR. Linguistic Aspects of Legal Language. (1990). Available online at: https://eric.ed.gov/?id=ED318248
23. Feldman R, Sanger J. The Text Mining Handbook: Advanced Approaches in Analyzing Unstructured Data. Cambridge: Cambridge University Press (2007). doi: 10.1017/CBO9780511546914
24. Bird S, Loper E, Klein E. Natural Language Processing with Python. Sebastopol, CA: O'Reilly Media Inc (2009).
25. Maynard D, Bontcheva K, Augenstein I. Natural Language Processing for the Semantic Web. San Rafael, CA: Morgan & Claypool Publishers (2017).
26. Francesconi E, Montemagni S, Peters W, Tiscornia D. Integrating a bottom-up top down methodology for building semantic resources for a multilingual legal domain. In Francesconi E, Montemagni S, Peters W, Tiscornia D, editors. Semantic Processing of Legal Texts. Where the Language of Law Meets the Law of Language. Vol. 6036. Berlin: Springer (2010). p. 95–121. doi: 10.1007/978-3-642-12837-0_6
27. Wagh RS. Knowledge discovery from legal documents dataset using text mining techniques. Intern J Comput Applicat. (2013) 66:32–34. doi: 10.5120/11258-6501
28. Wyner AZ, Peters W, Price D. Argument discovery and extraction with the argument workbench. In: Proceedings of the 2nd Workshop on Argumentation Mining. New York, NY (2015). doi: 10.3115/v1/W15-0510
29. Peters W, Wyner A. Legal Text Interpretation: Identifying Hohfeldian Relations From Text. In: Proceeding of LREC (2016). Available online at: http://www.lrec-conf.org/proceedings/lrec2016/summaries/253.html
30. Robaldo L, Villata S, Wyner AZ, And M. Grabmair. Introduction for artificial intelligence and law: special issue “natural language processing for legal texts”. Artif Intell Law. (2019) 27:113–5. doi: 10.1007/s10506-019-09251-2
31. Firth JR. A synopsis of linguistic theory 1930-1955. In: Palmer FR, editor. Selected Papers of JR. Bloomingtaon: Indiana University Press(1957) 168–205.
32. World Bank Group. The Growing Role of Minerals and Metals for a Low Carbon Future. Washington, DC: World Bank Group/EGPS (2017).
33. Jones N. Sea holds treasure trove of rare-earth elements. Nature. (2011). doi: 10.1038/news.2011.393
34. Hein JR, Mizell K, Koschinsky A, Conrad T. Deep-ocean mineral deposits as a source of critical metals for high- and green-technology applications: comparison with land-based resources. Ore Geol Rev. (2013) 51:1–14. doi: 10.1016/j.oregeorev.2012.12.001
35. McLellan BC, Yamasue E, Tezuka T, Corder G, Golev A, Giurco D. Critical minerals and energy–impacts and limitations of moving to unconventional resources. Resources. (2016) 5:19. doi: 10.3390/resources5020019
36. Sharma R. Deep-Sea mining: current status future considerations. In: Sharma R, editors. Deep-Sea Mining. Berlin: Springer (2017). doi: 10.1007/978-3-319-52557-0
37. Ifremer. Les Ressources Minerals Marines Profondes. Synthèse d'une Etude Prospective à L'horizon 2030. Issy les Moulineaux (2011).
38. World Ocean Review. Marine Resources – Opportunities and Risks. Future Ocean. Mare: The International Ocean Institute (2014).
39. Jones DOB, Kaiser S, Sweetman AK, Smith CR, Menot L, Vink A, et al. Biological responses to disturbance from simulated deep-sea polymetallic nodule mining. PLoS ONE. (2017) 12:e0171750. doi: 10.1371/journal.pone.0171750
40. Madureira P, Brekke H, Cherkashov G, Rovere M. Exploration of polymetallic nodules in the area: reporting practices, data management and transparency. Marine Policy. (2016) 70:101–7. doi: 10.1016/j.marpol.2016.04.051
41. Miller KA, Thompson KF, Johnston P, Santillo D. An overview of seabed mining including the current state of development, environmental impacts, knowledge gaps. Front Marine Sci. (2018) 4:418. doi: 10.3389/fmars.2017.00418
43. Sowa JF. Conceptual Structures: Information Processing in Mind and Machine. Boston, MA: Addison-Wesley (1984).
45. Khairullina D. Concept vs notion and lexical meaning: what is the difference? J Hist Cult Art Res. (2018) 7:303–13. doi: 10.7596/taksad.v7i5.1929
46. Bellingeri M, Bevacqua D, Scotognella F, Alfieri R, Nguyen Q, Montepietra D, et al. Link and node removal in real social networks: a review. Front Phys. (2020) 8:228. doi: 10.3389/fphy.2020.00228
47. Sukthanker R, Poria S, Cambria E, Thirunavukarasu R. Anaphora and coreference resolution: a review. Inform Fus. (2020) 59:139–62. doi: 10.1016/j.inffus.2020.01.010
48. Kolhatkar V, Roussel A, Dipper S, Zinsmeister H. Anaphora with non-nominal antecedents in computational linguistics: a survey. Comput Linguist. (2018) 44:547–612. doi: 10.1162/coli_a_00327
49. Khodak M, Risteski A, Fellbaum C, Arora S. AutomatedWordNet construction usingword embeddings. In: Proceedings of the 1st Workshop on Sense, Concept and Entity Representations and their Applications. Valencia: Association for Computational Linguistics (2017). doi: 10.18653/v1/W17-1902
50. Neale S. A survey on automatically-constructed wordnets and their evaluation: lexical and word embedding-based approaches. In: Proceedings of the Eleventh International Conference on Language Resources and Evaluation (LREC 2018). Miyazaki (2018). Available online at: https://www.aclweb.org/anthology/L18-1270.pdf
51. Sahlgren M. The distributional hypothesis. Rivis Linguist. (2008) 20:33–53. Available online at: http://www.italian-journal-linguistics.com/wp-content/uploads/Sahlgren-1.pdf
52. Fellbaum C editor. WordNet: an electronic lexical database. In: Bradford Books. Cambridge, MA: MIT press (1998). doi: 10.7551/mitpress/7287.001.0001
53. Sibertin-Blanc CH, Therond O, Monteil C, Mazzega P. The entity-process framework for integrated agent-based modeling of social-ecological systems. In: Boulet R, Lajaunie C, Mazzega P, editors. Law, Public Policies and Complex Systems: Networks in Action, Law, Governance and Technology Series. Berlin: Springer (2019). p. 57–87. doi: 10.1007/978-3-030-11506-7_4
55. Poelmans J, Elzinga P, Viaene S, Dedene G. Formal concept analysis in knowledge discovery: a survey. In: Croitoru M, Ferré S, and Lukose D, editors. Conceptual Structures: From Information to Intelligence, LNCS. Vol. 6208. Berlin: Springer (2010). p. 139–153. doi: 10.1007/978-3-642-14197-3_15
56. Codocedo V, Napoli A. Formal concept analysis and information retrieval—a survey. In: Sacarea JB, Ojeda-Aciego M. International Conference in Formal Concept Analysis. Nerja: Springer; LNCS. (2015). p. 61–77. doi: 10.1007/978-3-319-19545-2_4
57. Yevtushenko SA. System of data analysis “concept explorer.” In: Proceedings of the 7th National Conference on Artificial Intelligence KII-2000. (2000). p. 127–34.
58. Clark NA. Institutional arrangements for the new BBNJ agreement: moving global, regional and hybrid. Marine Policy. (2020) 122:104143. doi: 10.1016/j.marpol.2020.104143
59. Mendenhall E, Elizabeth De Santo E, Elizabeth Nyman E, Tiller R. A soft treaty, hard to reach: the second inter-governmental conference for biodiversity beyond national jurisdiction. Marine Policy. (2019) 108:103664. doi: 10.1016/j.marpol.2019.103664
60. Leary D. Agreeing to disagree on what we have or have not agreed on: the current state of play of the BBNJ negotiations on the status of marine genetic resources in areas beyond national jurisdiction. Marine Policy. (2019) 99:21–9. doi: 10.1016/j.marpol.2018.10.031
61. Flückiger A. Le principe de Clarté de la loi ou L'ambiguïté d'un Ideal. Cahiers du Conseil Constitutionnel No 21 Dossier: la Normativité (Janvier 2007). (2007). Available online at: https://www.conseil-constitutionnel.fr/nouveaux-cahiers-du-conseil-constitutionnel/cahiers-du-conseil-constitutionnel-n-21-janvier-2007 (accessed January 15, 2021).
Keywords: environmental law, conceptual graph, lexicon, distributional semantics, lattice, idiosyncrasy
Citation: Mazzega P (2021) Conceptual Graphs and Terminological Idiosyncrasy in UNCLOS and CBD. Front. Phys. 9:664621. doi: 10.3389/fphy.2021.664621
Received: 05 February 2021; Accepted: 09 April 2021;
Published: 17 May 2021.
Edited by:
Daniel Martin Katz, Illinois Institute of Technology, United StatesReviewed by:
Michele Bellingeri, University of Parma, ItalyCopyright © 2021 Mazzega. This is an open-access article distributed under the terms of the Creative Commons Attribution License (CC BY). The use, distribution or reproduction in other forums is permitted, provided the original author(s) and the copyright owner(s) are credited and that the original publication in this journal is cited, in accordance with accepted academic practice. No use, distribution or reproduction is permitted which does not comply with these terms.
*Correspondence: Pierre Mazzega, cG1henplZ2FAZ21haWwuY29t
Disclaimer: All claims expressed in this article are solely those of the authors and do not necessarily represent those of their affiliated organizations, or those of the publisher, the editors and the reviewers. Any product that may be evaluated in this article or claim that may be made by its manufacturer is not guaranteed or endorsed by the publisher.
Research integrity at Frontiers
Learn more about the work of our research integrity team to safeguard the quality of each article we publish.