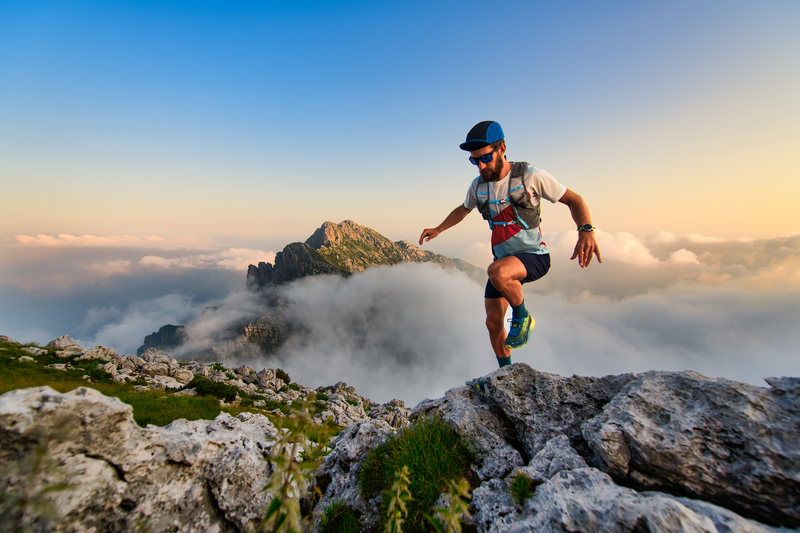
94% of researchers rate our articles as excellent or good
Learn more about the work of our research integrity team to safeguard the quality of each article we publish.
Find out more
BRIEF RESEARCH REPORT article
Front. Phys. , 04 May 2021
Sec. Optics and Photonics
Volume 9 - 2021 | https://doi.org/10.3389/fphy.2021.653353
This article is part of the Research Topic Optical Microscopic and Spectroscopic Techniques Targeting Biological Applications View all 24 articles
We report on the usefulness of digital holographic microscopy (DHM) for the assessment of human neutrophil differentiation from myeloid cells. The cell and nuclear regions have been designated by image segmentation of the optical phase function, and the changes of the cell nucleus morphology in relation to the whole cell morphology have been examined during the process of granulocytic differentiation into mature neutrophils in PLB-985 cell line. Nucleus phase volume and circularity and the ratios between the nucleus and the cell projected area and volume provide a reliable set of parameters to characterize the maturation process. As control, cell differentiation has been monitored in parallel using standard nucleus staining and fluorescence imaging. From this research, it emerged that DHM can be used as a valid label-free solution, alternatively to the standard staining technology.
Human neutrophils are central players in innate immunity, a major component of inflammatory responses, and a leading model for cell motility and chemotaxis. The neutrophils are generated from granulocyte-monocyte progenitors (GMPs) in the bone marrow, where they undergo several stages of maturation [1]. Primary, mature blood neutrophils have a short lifetime and lack proliferation and transfection capacity, thus limiting their ex vivo experimental use in the laboratory [2]. Therefore, protocols using human myeloid leukemia cell lines, such as HL-60 and PLB-985 (which provides higher differentiation efficiency), have been developed for neutrophil differentiation in vivo [3, 4]. In the presence of appropriate inducing agents, these cell lines can undergo granulocyte differentiation into mature neutrophil-like granulocytes [1, 2, 5]. The assessment of the differentiation process is based on cellular morphological parameters, the molecular and functional properties of differentiated cells, defining a neutrophil-like phenotype which closely resembles the mature blood neutrophils [5, 6].
Non-differentiated cells are considered as being at the promyelocytic stage. Activation by chemical agents transforms them along the myeloid pathway into myelocytes (Ms), metamyelocytes (MMs), band neutrophils (BNs) and, lastly, segmented neutrophils (SNs) [1, 2, 7]. This transformation process takes about 4–6 days. Monitoring the changes in nuclear morphology represents one of the most reliable technique to assess different stages and the success of the differentiation process [6, 8, 9]. Thus, cells in SN stage, presenting polymorph nucleus formed usually by two to five nuclear segments, are considered as mature neutrophil-like cells. In the first two stages [promyelocyte (PM) and M], cells have compact ellipsoidal nuclei occupying almost all of the cell body. Nuclei of the MMs begin to be distorted and present indentations. BNs have more distorted nuclei, often with a “horseshoe” shape, while in the final stage, SNs present multi-lobed nuclei connected between them [6, 8, 9].
In biological laboratories, the current practice to examine the morphology of the nucleus during neutrophil differentiation involves nuclear staining and epifluorescence microscopy [7, 10–12]. Since these techniques require sample preparation and provide relatively poor information, they are mostly used to evaluate the final stage of the differentiation process. Moreover, the labeling methods have a potential risk of altering cellular functions and phototoxicity from repeated excitation of the dye in the imaging process, which limits the duration of the time-lapse imaging [12]. The use of non-quantitative phase microscopy, which has become a pervasive tool for contrast enhancement and qualitative examination of cellular morphology [13], is limited by the low contrast enhancement for these cells.
Label-free quantitative phase imaging (QPI) [14–16], including digital holographic microscopy (DHM) [17–19], has been recently implemented in different setups to study morphology, structure, and dynamics of unstained living cells. QPI is based on the measurement of the optical phase (OP) shift introduced by the sample cell, which allows to calculate the optical path difference (OPD): OPD = OP·2π/λ, where λ is the wavelength of the laser beam. The OPD combines the physical thickness, h, and the difference between the refractive index (RI) of the cell, nc, and the RI of the medium, nm: OPD = h·Δn, where Δn = (nc − nm). The RI of the cell represents an important parameter which can be used to correlate with other cell biophysical parameters, such as dry and wet mass, protein concentration, elasticity, and conductivity, and to study certain cell metabolic activities, such as cell division and infection [20]. To isolate the RI from the thickness in OPD, several solutions are possible [21]. Assuming that the RI of the suspension medium is known and that cells in suspension are spherical, the simplest and fastest method to determine the integral RI is to approximate the local thickness [22]. This method has been used to show changes in the subcellular structure and the RI of differentiating myeloid precursor cells within 1 day of differentiation induction [23] and to demonstrate that cell nuclei have lower RI than cytoplasm [24]. Another approach is performing two OPD measurements, each of them with a different surrounding medium [25, 26] or a different wavelength [27], thus obtaining two linear equations with two unknowns which enable the decoupling of the integral RI from the thickness. Other solutions for the thickness-RI coupling problem are thoroughly reviewed in Dardikman and Shaked [21].
Nonetheless, various cell morphological features can also be directly extracted from the OPD, e.g., OPD mean/median, phase volume, phase surface area, dry mass, phase sphericity, phase statistical parameters, and energy [28]. Such features have been successfully combined with machine learning algorithms in cytometric classification of cancer cells and blood cells [29], phenotyping of cell lines [30], classification of leukocytes flowing in microfluidics [31], or automatic detection of Plasmodium falciparum [32]. Combining QPI with dye exclusion cell volumetric imaging, the cell volume can be quantified independently of the RI of the cell, enabling high-throughput neutrophil differentiation from other white blood cells flowing in an optofluidic device [33].
Using QPI with a common arrangement for DHM, in this study, we investigate on the usefulness of a set of cell parameters extracted directly from OPD to supervise neutrophil differentiation from the PLB-985 cell line. Since the differentiation protocol includes five stages, namely, PMs, Ms, MMs, BNs, and SNs, and lasts for about 5–6 days, a set of OPD images were taken every day and analyzed. The reconstructed OPD functions for a set of cells in full field were first segmented to separate the cells from the background and then a second segmentation was applied at the single cell level to demarcate the central region with high phase values, designating to the nuclear region. The OPD and a set of nine morphological parameters were calculated for each cell. Comparing the values of the parameters calculated for cells from different stages, we discuss their relevance and show that DHM can be successfully employed to distinguish between different cell stages of neutrophil differentiation.
Neutrophil differentiation from its promyelocytic progenitors was performed following the dimethyl sulfoxide (DMSO) protocol described in Tucker et al. [4]. Briefly, PLB-985 cells (DSMZ, Braunschweig, Germany) were cultured in RPMI 1640 media supplemented with 10% fetal bovine serum (FBS), 1% L-Glutamin, and 100 mg/mL and 100 U/mL (P/S) of streptomycin and penicillin, respectively, at 37°C in a humidified atmosphere with 5% CO2. Cell cultures were passed three times per week, maintaining cell densities between 105 and 2 × 106 cells per mL. The cells were differentiated into a neutrophil-like state by culturing at an initial density of 2 × 105 using RPMI 1640 media supplemented with 10% FBS concentration and 1.3% DMSO for 6 days.
Cell differentiation was monitored every 24 h for 6 days beginning from day 0. For each measurement, 1 mL of cell suspension was taken from the culture flask. The suspension was then centrifuged, and the supernatant was removed to exclude debris and impurities. Then, the sample was resuspended and incubated for 5 min at 37°C. To measure the cells, 50 μL of cell suspension was placed in a 18 mm diameter glass slide. About 2–3 min was required for the cells to sediment before imaging it. For DHM imaging, the sample was resuspended in RPMI 1640 media, while for fluorescence imaging, the sample was resuspended in a Hoechst 33342 solution of 1:1,000 in RPMI 1640 media as described in the study by Chazotte [11].
We used a simplified version of a previously developed DHM off-axis configuration, based on a Mach-Zehnder interferometer [34, 35]. The laser beam (λ = 520 nm, Thorlabs LP520-SF15) was split into object and reference beams by a fiber optic coupler (Thorlabs TW560R3F1) and recombined by a cube beam splitter (Thorlabs, BS079) to generate the hologram, which was recorded on a sCMOS camera (Thorlabs CS2100M-USB Quantalux). The power P of the laser was set to P < 1 mW, and the exposure time to 1 < t < 5 ms. The magnification of the microscope was 33×, with a lateral spatial resolution d ~ 600 nm. An aspheric lens (Thorlabs C230TMD-A) with the numerical aperture NA = 0.55 was used as the objective lens. The size of the sCMOS sensor was 4.8 × 4.8 μm. The inter-fringe, i, of the interference pattern was adjusted to five pixels on the sensor, corresponding to 750 nm at the sample plane.
The numerical reconstruction of the optical phase was performed using a method based on the spatial filtering at the Fourier plane from the recorded hologram [36]. Thus, the complex amplitude distribution of the transmitted frequency band-pass was recovered by applying a Fourier transform over the recorded hologram while considering a circular spatial filtering mask located at one of the diffraction orders. After the filtering and centering process at the Fourier domain, the complex amplitude was Fourier transformed again to propagate it to the sample plane and retrieve the wrapped OP. The final step, phase unwrapping, is used to get the reconstructed OP function. Since these issues are of particular significance in the reconstruction process, we defined the parameters for spatial filtering, propagation, and phase unwrapping by calibration with silica microbeads of different diameters (3−30 μm) [34]. Microbeads are the perfect sample for calibration purposes since they are microspheres of a single material (silica, polystyerene, etc.) with known value of the RI.
In the first step, the OPD map was segmented to isolate the cells from the background by applying the bimodal Otsu's method [37, 38] implemented in MATLAB, using the following two functions, multitresh (L = 2 levels) and imquantize, from the Image Processing Toolbox. To remove the incongruous cell spots resulting from segmentation, the phase volume of each cell spot was calculated and those spots with the phase volume below a predetermined threshold were discarded.
The designation of the nuclear region was accomplished in a second segmentation applied to each cell, using multitresh with (L = 4). The number of segmentation levels is supported by the Histogram-based Valley Estimation Method presented in the study by Huang et al. [39], where the number of clusters for an image to be properly segmented depends on the number of valleys in the histogram of the image. After segmentation, the first level is assigned to the background pixels, the second and the third levels to the cell cytoplasm region, and the fourth level to cell nucleus. The segmentation method is described in detail in Supplementary Material.
In order to assess Optical phase (OP) imaging by DHM as a free-label technique for characterization and discrimination of the cell stages, we monitored the differentiation of PLB-985 cell line from PMs to SNs and imaged the cells at intervals of 24 h for 6 days during the neutrophil differentiation cycle.
As described in section 2.3, segmentation of the reconstructed OP for an image containing several cells was used to select the cells from the background and delineate the estimated nucleus from the other part of the cell. An example of the image taken at day 5 is illustrated in Figure 1D, showing the presence of multiple types of cells as indicated by the morphology of the cell and the designated nuclear regions, characterized by high values of the OPD function. At a first qualitative inspection, using the morphological phenotypes reported in literature and as already mentioned in the “Introduction” section, we can recognize one SN, three BNs, two MMs, one M. BNs are the most frequent, as expected for day 5. The presence of other types of cells is justified by the various biological times employed by different cells to transform [1]. This observation suggests that OPD and the segmented OPD could be used to characterize different stages during neutrophil differentiation.
Figure 1. Optical phase (OP) reconstruction and cell selection. (A) Recorded hologram, (B) reconstructed OP, (C) segmentation, (D) full field image taken at day 5 showing different types of cells; left: after cell selection, the colors indicate cell height; right: cell and nucleus regions are shown after cell and nucleus selection. Scale bars: 5 μm.
Since the results reported in the literature are based on nuclear staining and fluorescence imaging, we also performed image analysis of the nucleus morphological changes using Hoechst staining and live cell fluorescence imaging (Nikon TE2003-E inverted microscope, 60X, DAPI fluorescence cube). An example of nuclear morphology changes during the five stages of the neutrophil differentiation is shown in Figure 2. The first two rows are brightfield and DIC images, which help to define the cell shape but do not allow to discriminate the nucleus. Complementarily, the fluorescence images illustrated in the third row for the same cell allow the identification of the nucleus. At the beginning of the differentiation, corresponding to PM and M cells, the cell nucleus changes round to oval, occupying more than 50% of the cell projected surface area. The oval shape begins to be preponderant to round shape for M, and the ratio between nucleus and cell area is also slightly smaller than Ms for PMs. As the cell matures, the nucleus begins to be indented and becomes asymmetric with respect to the cell center (MM). A more mature cell presents highly indented nucleus and often a “horseshoes” shape (BN), arriving to the segmentation of the nucleus at the end (SN).
Figure 2. Example of cells in different maturation stages. The first three rows show brightfield, DIC, and fluorescence images, where each column representing the same cell. The last two rows show the reconstructed OP difference (OPD) and cell segmentation. Scale bar: 5 μm.
Free-label DHM imaging for the five stages of the cell differentiation is exemplified in the last two rows of Figure 2. The OPD and the segmented OPD for an isolated cell from each of the five stages are represented, confirming the observations acquired from brightfield and fluorescence imaging on the cell and nucleus configuration during the differentiation cycle. Moreover, the OPD values in the cell and nuclear regions assigned by segmentation provide additional information on the cell morphological features as the OP volume.
We monitored the neutrophil differentiation recording five DHM image fields/day at intervals of 24 h starting from cell plating. Two different differentiation cycles were completed. The OPD was calculated for each field, and a qualitative inspection was performed as discussed above and showed in Figure 1, discarding the cells which did not correspond to the differentiation stage of the respective day. We created then five groups of N > 20 cells, corresponding to the five stages: PM, M, MM, BN, and SN, and calculated the parameters defined above for each cell.
Cellular morphologic parameters as projected surface area (PSA), OP volume (OV), and circularity (C) have been effectively used for the characterization of red blood cells [35, 40, 41] in QPI and DHM. In this study, we propose similar parameters for the nucleus, including the ratios between the values for nucleus and cell as parameters. We define the following morphological parameters:
1. Projected surface area (PSA) of the cell and nuclear regions:
where N is the number of pixels within the cell nucleus region, ps = 4.8 * 4.8 = 23.04 μm2 is the area of a single pixel of the CMOS sensor, and M = 33× is the microscope magnification.
2. Optical Phase Volume (OV) of the cell and nuclear regions:
where OPDi is the optical phase difference of cell/nucleus corresponding to the pixel.
3. Circularity (C) of the cell and nuclear regions:
where Pm is the perimeter of the cell/nucleus. Circularity expresses the roundness of an object, e.g., C = 1 means a perfectly round cell, as a circular disk.
4. PSA and OV nucleus to cell, N/C, aspect ratio:
where N stays for nucleus, C for cell, PSA for projected surface area, and OV for optical volume.
The mean and SD values for each parameter are presented in Figure 3A for each group of cells. This study shows that PSA and OV monotonously decrease as the cells maturate, both for the cell and the nucleus region. The cell C, CC, is preserved close to CC = 1 for PM, N, and MM, while decreasing slightly for the last two stages, BN and SN (CC > 0.9). As the cells present more protrusions in these two stages, the values for C are also more dispersed. The C values for nucleus, CN, decrease more than CN > 0.8 for PM and M, CN = 0.8 ± 0.08 for MM, CN = 0.69 ± 0.13 for BN and CN = 0.63 ± 0.15 for SN, indicating that the nucleus undergoes substantial morphological changes during the differentiation process. The relative morphological changes of the nucleus vs. cell are outlined by the N/C ratio for area and volume, respectively, PSAN/C and OVN/C, in the last row of Figure 3A. Both ratios decrease monotonically during cell maturation, with a more pronounced change in OVN/C.
Figure 3. Cell and nucleus morphological parameters. (A) Projected Surface Area (PSA), Optical Volume (OV), Circularity (C), and ratios of nucleus to cell, NC, of PSA and OV. Mean and SD values for: promyelocytes (PM, N = 22), myelocytes (M,N = 21), metamyelocytes (MM, N = 24), band neutrophils (BN, N = 21), segmented neutrophils (SN, N = 25). (B) Diagram showing the results of the Mann-Whitney U test calculated for nine parameters, for each class pair. A p-value < 0.05 is indicated by blue color. The legend of the parameters is shown top right: PSAN, PSAC—nucleus PSA and cell PSA, respectively; CN, CC—nucleus circularity and cell circularity, respectively; OVN, OVC—nucleus OV and cell OV, respectively; PSAN/C, OVN/C—ratio between the cell and nucleus for PSA and OV; SNG—segmented nucleus.
We note that the values between neighboring stages overlap for some parameters, indicating that a set of parameters should be considered rather than a single parameter to characterize the differentiation stages. In order to test the weight of each parameter, we used the Mann-Whitney U test (or two-sided Wilcoxon rank sum test) for each pair of classes. The results are schematically illustrated in Figure 3B: p < 0.05 (blue box) is considered as a threshold for good distinction between stages, 0.05 < p < 0.1 (gray) as acceptable, and p > 0.1 (white) as non-confident.
It can be seen that all eight parameters are individually suitable to distinguish SN from PM. All parameters except cell PSAC and cell OVC are useful to discriminate SN from M and MM. Only three parameters (OVN/C, PSAN/C, OVN/C) are available to distinguish between SN and BN. Note that all of them were extracted from the OPD, which is provided by DHM only. Additionally, the presence of a segmented nucleus, which is confidently detected from OPD, represents another useful parameter which distinguishes SN from all the other stages (crossed box in Figure 3B).
All eight parameters are suitable to distinguish BN from PM cells, four of the eight parameters to separate BN from M cells, and only two parameters to discriminate BN from neighboring stage, MM: the nucleus and cell circularity, CN and CC. We notice that these two parameters are complementary to the parameters which distinguish BN from the other neighboring stage, SN. Moreover, considering 0.05 < p < 0.1 for an acceptable discrimination, the nucleus area, PSAN, becomes an eligible parameter to distinguish between BN and MM or M.
As regards the MM cells, seven of the eight parameters are useful to distinguish MM from PM cells, but none is adequate using the criterium p < 0.05 to separate MM from M. However, considering p < 0.1, both NA and NC can be used to separate M from MM. Finally, five of eight parameters can be used to separate M from PM cells. These parameters are complementary to the parameters used to distinguish between BN and MM, and only two parameters are in common with the discrimination between BN and SN.
Five parameters can be used to separate M from PM cells. These parameters are complementary to the parameters used to distinguish between BN and MM, and only two parameters are in common with the discrimination between BN and SN.
According to the imposed criterium, p < 0.05, the nearest two classes seem to be MM and M cells. However, by relaxing the criterium to p < 0.1, two more parameters are indicated as suitable for MM and M cells discrimination.
The analysis of the results show that all these parameters are useful to discriminate between cells in different differentiation stages. Three of these parameters, OVN, OVC, OVN/C, which have a central role for cell characterization and discrimination between differentiation stages, are extracted from the OPD, which is specific for DHM. These preliminary results suggest that a combination of the parameters derived by means of DHM in a multiparameter testing scheme would enable the characterization and monitoring of the different stages of cell differentiation.
We note that the OPD function was used directly, without decoupling the RI from the cell height. Although, the designation of the nuclear region by segmentation cannot be claimed as precise identification of the nucleus, the method appears useful to monitor the neutrophil differentiation cycle. Considering the recent findings showing that the nuclear RI is lower than the cytoplasm [24, 42], this affects the OV parameter. We calculated the OV of the value which corresponds to a physical volume bigger for the nucleus relative to the same value of the OV for the cell. Although the absolute values of the volume change, the relative differences between stages are not expected to be altered considerably because the changes are uniformly applied.
Another interesting point is the possible change of the cellular RI during the HL60 cells differentiation [23], indicating that the cells become less dense during differentiation. Since we measured only the OPD, we could not confirm that this observation also applied for PLB-985 cells cultured on substrate, but we observed a significant decrease of the PSAN/C, which was associated with the decrease in the RI in this study.
In this study, we performed cell morphological analysis during promyelocytic differentiation into SN for both cell and nuclear regions using DHM as free-label optical imaging technique. We defined a set of eight morphological parameters and investigated their contribution to characterize cells belonging to one of the five maturation stages: PMs, Ms, MMs, BNs, and SNs.
We proved that DHM provides additional information with respect to the standard fluorescence imaging of stained nucleus. This information is related to the volumes of cells and nucleus and allows a better discrimination between different stages of neutrophil differentiation. We believe that DHM has been employed for the first time for monitoring neutrophil differentiation in PLB 945 cells. The results suggest DHM is a suitable free-label technique providing better cell characterization and discrimination between differentiation stages.
The original contributions presented in the study are included in the article/Supplementary Material; further inquiries can be directed to the corresponding author/s.
DC and ML conceived the project, analyzed and interpreted the data, and wrote the manuscript. DC designed and implemented the DHM. ML performed the experiments. All authors contributed to the article and approved the submitted version.
The authors declare that the research was conducted in the absence of any commercial or financial relationships that could be construed as a potential conflict of interest.
The authors thanks Dr. Marta Stefania Semrau and Dr. Valentina Masciotti for the training in cell culture basics.
The Supplementary Material for this article can be found online at: https://www.frontiersin.org/articles/10.3389/fphy.2021.653353/full#supplementary-material
1. Ng LG, Ostuni R, Hidalgo A. Heterogeneity of neutrophils. Nat Rev Immunol. (2019) 19:255–65. doi: 10.1038/s41577-019-0141-8
2. Summers C, Rankin SM, Condliffe AM, Singh N, Peters AM, Chilvers ER. Neutrophil kinetics in health and disease. Trends Immunol. (2010) 31:318–24. doi: 10.1016/j.it.2010.05.006
3. Dalton WJ, Ahearn MJ, McCredie KB, Freireich EJ, Stass SA, Trujillo JM. HL-60 cell line was derived from a patient with FAB-M2 and not FAB-M3. Blood. (1988) 71:242–7. doi: 10.1182/blood.V71.1.242.242
4. Tucker KA, Lilly MB, Heck LJ, Rado TA. Characterization of a new human diploid myeloid leukemia cell line (PLB-985) with granulocytic and monocytic differentiating capacity. Blood. (1987) 70:372–8. doi: 10.1182/blood.V70.2.372.372
5. Boulven I, Levasseur S, Marois S, Paré G, Rollet-Labelle E, Naccache PH. Class IA phosphatidylinositide 3-kinases, rather than p110γ, regulate formyl-methionyl-leucyl-phenylalanine-stimulated chemotaxis and superoxide production in differentiated neutrophil-like PLB-985 cells. J Immunol. (2006) 176:7621–7. doi: 10.4049/jimmunol.176.12.7621
6. Shehu S. Regulation of Apoptosis of Myeloid Immune Cells: Implication for Cancer Therapy and Inflammation. Liverpool: University of Liverpool (2017).
7. Bjerregaard MD, Jurlander J, Klausen P, Borregaard N, Cowland JB. The in vivo profile of transcription factors during neutrophil differentiation in human bone marrow. Blood. (2003) 101:4322–32. doi: 10.1182/blood-2002-03-0835
8. Iwasaki H, Akashi K. Myeloid lineage commitment from the hematopoietic stem cell. Immunity. (2007) 26:726–40. doi: 10.1016/j.immuni.2007.06.004
9. Lieber JG, Webb S, Suratt BT, Young SK, Johnson GL, Keller GM, et al. The in vitro production and characterization of neutrophils from embryonic stem cells. Blood. (2004) 103:852–9. doi: 10.1182/blood-2003-04-1030
10. Bezrukov A. Romanowsky staining, the Romanowsky effect and thoughts on the question of scientific priority. Biotech Histochem. (2017) 92:29–35. doi: 10.1080/10520295.2016.1250285
11. Chazotte B. Labeling nuclear DNA with Hoechst 33342. Cold Spring Harbor Protoc. (2011) 2011:pdb-prot5557. doi: 10.1101/pdb.prot5557
12. Siemann DW, Keng PC. Cell cycle specific toxicity of the Hoechst 33342 stain in untreated or irradiated murine tumor cells. Cancer Res. (1986) 46:3556–9.
13. Shaked NT, Zalevsky Z, Satterwhite LL. Biomedical Optical Phase Microscopy and Nanoscopy. Amsterdam; Boston, MA; Heidelberg; London; New York, NY; Oxford, Paris, San Diego, CA; San Francisco, CA; Singapore; Sidney: Academic Press (2013).
14. Park Y, Depeursinge C, Popescu G. Quantitative phase imaging in biomedicine. Nat Photon. (2018) 12:578–89. doi: 10.1038/s41566-018-0253-x
15. Popescu G. Quantitative Phase Imaging of Cells and Tissues. New York, NY; Chicago, San Francisco, CA; Lisbon; London; Madrid; Mexico City; Milan; New Delhi; San Juan; Seoul; Singapore; Sydney, NSW; Toronto, ON: Graw-Hill Education (2011).
16. Ferraro P, Wax A, Zalevsky Z. Coherent Light Microscopy: Imaging and Quantitative Phase Analysis. Vol. 46. Berlin: Heidelberg: Springer-Verlag (2011). doi: 10.1007/978-3-642-15813-1
17. Marquet P, Depeursinge C, Magistretti PJ. Review of quantitative phase-digital holographic microscopy: promising novel imaging technique to resolve neuronal network activity and identify cellular biomarkers of psychiatric disorders. Neurophotonics. (2014) 1:020901. doi: 10.1117/1.NPh.1.2.020901
18. Micó V, Zheng J, Garcia J, Zalevsky Z, Gao P. Resolution enhancement in quantitative phase microscopy. Adv Opt Photon. (2019) 11:135–214. doi: 10.1364/AOP.11.000135
19. Marquet P, Rappaz B, Magistretti PJ, Cuche E, Emery Y, Colomb T, et al. Digital holographic microscopy: a noninvasive contrast imaging technique allowing quantitative visualization of living cells with subwavelength axial accuracy. Opt Lett. (2005) 30:468–70. doi: 10.1364/OL.30.000468
20. Liu PY, Chin L, Ser W, Chen H, Hsieh CM, Lee CH, et al. Cell refractive index for cell biology and disease diagnosis: past, present and future. Lab Chip. (2016) 16:634–44. doi: 10.1039/C5LC01445J
21. Dardikman G, Shaked NT. Review on methods of solving the refractive index-thickness coupling problem in digital holographic microscopy of biological cells. Opt Commun. (2018) 422:8–16. doi: 10.1016/j.optcom.2017.11.084
22. Kemper B, Kosmeier S, Langehanenberg P, von Bally G, Bredebusch I, Domschke W, et al. Integral refractive index determination of living suspension cells by multifocus digital holographic phase contrast microscopy. J Biomed Opt. (2007) 12:054009. doi: 10.1117/1.2798639
23. Chalut KJ, Ekpenyong AE, Clegg WL, Melhuish IC, Guck J. Quantifying cellular differentiation by physical phenotype using digital holographic microscopy. Integr Biol. (2012) 4:280–4. doi: 10.1039/c2ib00129b
24. Schürmann M, Scholze J, Müller P, Guck J, Chan CJ. Cell nuclei have lower refractive index and mass density than cytoplasm. J Biophoton. (2016) 9:1068–76. doi: 10.1002/jbio.201500273
25. Rappaz B, Marquet P, Cuche E, Emery Y, Depeursinge C, Magistretti PJ. Measurement of the integral refractive index and dynamic cell morphometry of living cells with digital holographic microscopy. Opt Express. (2005) 13:9361–73. doi: 10.1364/OPEX.13.009361
26. Cardenas N, Mohanty S. Decoupling of geometric thickness and refractive index in quantitative phase microscopy. Opt Lett. (2013) 38:1007–9. doi: 10.1364/OL.38.001007
27. Boss D, Kühn J, Jourdain P, Depeursinge CD, Magistretti PJ, Marquet PP. Measurement of absolute cell volume, osmotic membrane water permeability, and refractive index of transmembrane water and solute flux by digital holographic microscopy. J Biomed Opt. (2013) 18:036007. doi: 10.1117/1.JBO.18.3.036007
28. Roitshtain D, Wolbromsky L, Bal E, Greenspan H, Satterwhite LL, Shaked NT. Quantitative phase microscopy spatial signatures of cancer cells. Cytometry A. (2017) 91:482–93. doi: 10.1002/cyto.a.23100
29. Nissim N, Dudaie M, Barnea I, Shaked NT. Real-time stain-free classification of cancer cells and blood cells using interferometric phase microscopy and machine learning. Cytometry A. (2020). doi: 10.1002/cyto.a.24227. [Epub ahead of print].
30. Lam VK, Nguyen T, Phan T, Chung BM, Nehmetallah G, Raub CB. Machine learning with optical phase signatures for phenotypic profiling of cell lines. Cytometry A. (2019) 95:757–68. doi: 10.1002/cyto.a.23774
31. Ugele M, Weniger M, Stanzel M, Bassler M, Krause SW, Friedrich O, et al. Label-free high-throughput leukemia detection by holographic microscopy. Adv Sci. (2018) 5:1800761. doi: 10.1002/advs.201800761
32. Park HS, Rinehart MT, Walzer KA, Chi JTA, Wax A. Automated detection of P. falciparum using machine learning algorithms with quantitative phase images of unstained cells. PLoS ONE. (2016) 11:e0163045. doi: 10.1371/journal.pone.0163045
33. Schonbrun E, Di Caprio G. Differentiating neutrophils using the optical coulter counter. J Biomed Opt. (2015) 20:111205. doi: 10.1117/1.JBO.20.11.111205
34. Picazo-Bueno JÁ, Cojoc D, Iseppon F, Torre V, Micó V. Single-shot, dual-mode, water-immersion microscopy platform for biological applications. Appl Opt. (2018) 57:A242–9. doi: 10.1364/AO.57.00A242
35. Merola F, Miccio L, Memmolo P, Di Caprio G, Galli A, Puglisi R, et al. Digital holography as a method for 3D imaging and estimating the biovolume of motile cells. Lab Chip. (2013) 13:4512–6. doi: 10.1039/c3lc50515d
36. Micó V, Zalevsky Z, Ferreira C, García J. Superresolution digital holographic microscopy for three-dimensional samples. Opt Express. (2008) 16:19260–70. doi: 10.1364/OE.16.019260
37. Otsu N. A threshold selection method from gray-level histograms. IEEE Trans Syst Man Cybernet. (1979) 9:62–6. doi: 10.1109/TSMC.1979.4310076
38. Gonzalez RC, Woods RE. Digital Image Processing. 3rd ed. Upper Saddle River, NJ: Prentice-Hall, Inc. (2006).
39. Huang DY, Lin TW, Hu WC. Automatic multilevel thresholding based on two-stage Otsu's method with cluster determination by valley estimation. Int J Innov Comput Inform Control. (2011) 7:5631–44.
40. Jaferzadeh K, Moon I. Quantitative investigation of red blood cell three-dimensional geometric and chemical changes in the storage lesion using digital holographic microscopy. J Biomed Opt. (2015) 20:111218. doi: 10.1117/1.JBO.20.11.111218
41. Park H, Lee S, Ji M, Kim K, Son Y, Jang S, et al. Measuring cell surface area and deformability of individual human red blood cells over blood storage using quantitative phase imaging. Sci Rep. (2016) 6:34257. doi: 10.1038/srep34257
Keywords: digital holography microscopy, neutrophil differentiation, PLB-985 cell line, cell and nucleus morphology, label-free
Citation: do R. B. F. Lima MA and Cojoc D (2021) Monitoring Human Neutrophil Differentiation by Digital Holographic Microscopy. Front. Phys. 9:653353. doi: 10.3389/fphy.2021.653353
Received: 14 January 2021; Accepted: 26 March 2021;
Published: 04 May 2021.
Edited by:
Vicente Micó, University of Valencia, SpainReviewed by:
Björn Kemper, University of Münster, GermanyCopyright © 2021 do R. B. F. Lima and Cojoc. This is an open-access article distributed under the terms of the Creative Commons Attribution License (CC BY). The use, distribution or reproduction in other forums is permitted, provided the original author(s) and the copyright owner(s) are credited and that the original publication in this journal is cited, in accordance with accepted academic practice. No use, distribution or reproduction is permitted which does not comply with these terms.
*Correspondence: Dan Cojoc, Y29qb2NAaW9tLmNuci5pdA==
Disclaimer: All claims expressed in this article are solely those of the authors and do not necessarily represent those of their affiliated organizations, or those of the publisher, the editors and the reviewers. Any product that may be evaluated in this article or claim that may be made by its manufacturer is not guaranteed or endorsed by the publisher.
Research integrity at Frontiers
Learn more about the work of our research integrity team to safeguard the quality of each article we publish.