- MeBioS, KU Leuven, Leuven, Belgium
At long time scales, tissue spheroids may flow or appear solid depending on their capacity to reorganize their internal structure. Understanding the relationship between intrinsic mechanical properties at the single cell level, and the tissue spheroids dynamics at the long-time scale is key for artificial tissue constructs, which are assembled from multiple tissue spheroids that over time fuse to form coherent structures. The dynamics of this fusion process are frequently analyzed in the framework of liquid theory, wherein the time scale of coalescence of two droplets is governed by its radius, viscosity and surface tension. In this work, we extend this framework to glassy or jammed cell behavior which can be observed in spheroid fusion. Using simulations of an individual-cell based model, we demonstrate how the spheroid fusion process can be steered from liquid to arrested by varying active cell motility and repulsive energy as established by cortical tension. The divergence of visco-elastic relaxation times indicates glassy relaxation near the transition toward arrested coalescence. Finally, we investigate the role of cell growth in spheroid fusion dynamics. We show that the presence of cell division introduces plasticity in the material and thereby increases coalescence during fusion.
1 Introduction
A general understanding of the rheological properties of multicellular tissues is important to gain insight into the physics of morphogenetic processes during development. Furthermore, robust models of these materials allow for the design and characterization of generic unit operations, such as aggregation, dispersion, and fusion, which are used for the production of artificial tissues. Given its analogy to the merging of two liquid droplets, the fusion of tissue spheroids has received considerable interest as a model for soft tissue rheology. An analytical expression of the onset of coalescence of two equal viscous droplets under influence of their surface tension was first derived by Frenkel [1] and was improved and extended upon so that the dynamics of complete coalescence could be accurately modeled [2, 3]. Furthermore, various extensions to this framework have been proposed to take into account specific properties of multicellular materials, for example differences in spheroid size [4], and the presence of biological processes such as proliferation, differentiation and apoptosis, which may conflict with the assumption of conservation of mass [4, 5].
However, the liquid model cannot consistently reproduce in vitro tissue behavior. For instance, Kosheleva et al. showed that fusion dynamics did not correspond to liquid model predictions based on nano-indentation surface tension measurements [6]. Furthermore, arrested fusion has been observed between spheroids treated with Rho-kinase inhibitor affecting acto-myosin contractility [7, 8]. Arrested fusion was shown to be controlled by cancer cell activity in tumors [9]. To explain these observations, Oriola et al. recently proposed to model spheroids during fusion as visco-elastic materials instead of simple liquid droplets [10]. Such an approach has already been applied to parallel plate compression [11], micro-pipette aspiration [12], and tissue-detachment/fracture assays [13]. In general, a tissue may behave like an elastic solid at short time scales and like a liquid at longer time scales [14]. However, they can also appear solid-like at long time scales. This may occur when tissues change from a fluid-like to a solid-like state by undergoing a jamming transition [15]. In the jammed state, individual cells are caged by their neighbors preventing the cells from rearranging, resulting in glassy rheology, whereas in the unjammed state cells are able to move freely, resembling a fluid-like state.
Multiple computational approaches such as phase field models [16], Monte Carlo based models [17–19], and individual cell-based models [4, 10, 18–22], allow for the characterization of tissue-scale rheological behavior as a function of cell-scale mechanical properties or to simulate fusion of spheroids in more complex geometries. Schötz et al. calibrated an individual cell-based model to better describe the random motion of cells in tissues close to a glass transition. Nevertheless, when investigating fusion with that model, it could still be described by a liquid model. On the other hand, simulations that resulted in a solidification during spheroid fusion have already been mentioned by Kosztin et al. [20]. This observation was attributed to high levels of cell adhesion, but was not further analyzed. Recently, using individual cell-based computational models, it was demonstrated that a divergence of the visco-elastic relaxation time can be observed within a transition region of arrested fusion, indicative of a jammed system [10]. The existence of arrested fusion could thus be traced back to the build up of elastic energy during fusion.
In this work, we derive a simplified analytical expression for the coalescence of visco-elastic tissue spheroids on the basis of elasticity caused by internal structure of the droplet [23, 24]. Doings so we obtain similar results to [10]. We demonstrate the applicability of this expression by using an individual-cell based model approach to simulate the fusion process and, using this expression, we are able to compare the relaxation dynamics of fusion to the characteristic timescales involved in the preceding aggregation (or compaction) process in which the individual tissue spheroids have been formed. Finally, we extend this simulation framework to include a morphological model of the cell cycle. In analogy to active motility, cell division and growth may introduce excitation that may induce fluidization, as was observed in other systems [25–28]. Here, we investigate whether, in spheroid fusion, there is an additional active contribution of cell division beyond a mere correction for the increase in volume, and assess to what extent this contribution may unjam the cellular material [4, 5] and recover tissue coalescence.
2 Materials and Methods
2.1 Individual Cell-Based Model
We follow an individual cell-based model approach as described in detail in Smeets et al. [29, 30]. This model has already been used to study cell aggregation and compaction dynamics. In this paper we extend the model by taking into account cell proliferation. All details of the individual cell based model can be found in Supplementary Section S1. In brief, the model is based on a simulation framework introduced by Delile et al. [31], in which cells are simulated as self-propelled particles. Conservative active forces are exchanged between neighboring cells, similar to [10]. The connectivity network is based on a Delaunay triangulation of the cell center coordinates. For the edges of this network, a symmetric central potential is calculated, which is parameterized by adhesion
2.2 Simulation Setup
The simulation pipeline is shown in Figure 1 and follows a sequence of generic unit operations to form a small tissue via fusion. In the first step we simulate the seeding and spontaneous aggregation of two aggregates for 24 h, each in their own micro-well, similar to [30]. This guarantees that the shape of each spheroid is consistent with respect to its underlying mechanical properties. It should be noted that, depending on the mechanical properties that govern the aggregation process, this relaxed configuration may have a highly irregular shape. Any (few) remaining cells that did not get incorporated in the main aggregates, are removed from the simulation in analogy to a real fusion experiment. In the second step, the two aggregates are transferred to a larger micro-well and are brought into contact with each other, simulating another 50 h during which the fusion process naturally progresses. In the final step, we extract the spheroid contours and fit two circles to compute the contact angle θ for each simulation, as explained in (Supplementary Section S2). Each realization of a simulated fused spheroid is initialized independently. To calculate the average fusion dynamics, i.e., the average of
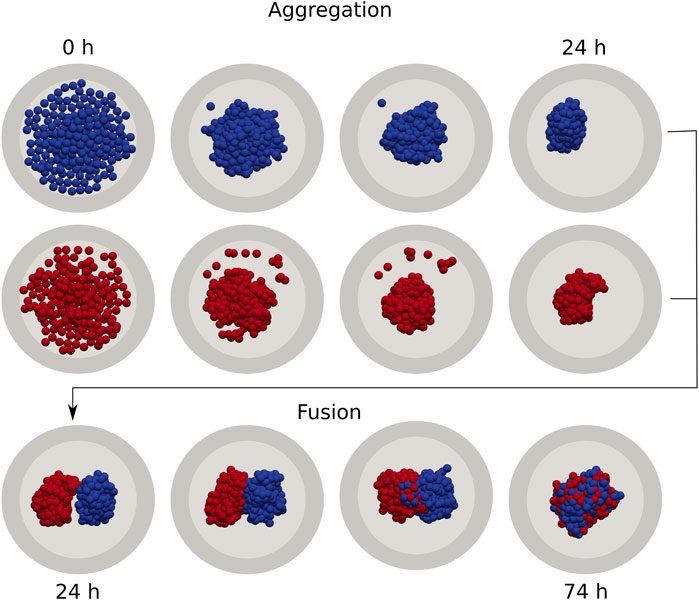
FIGURE 1. Individual cell-based model of cell aggregation and spheroid fusion. First, gravitationally deposited cells aggregate in independent cylindrical wells for 24 h (top). Next, two aggregates are extracted and combined in a single fusion simulation, which continues for a further 50 h (bottom).
2.3 Visco-Elastic Approximation of the Fusion Process
The fusion dynamics of two equal visco-elastic spheres can be approximated by the differential equation
as derived in (Supplementary Section S3). Here, θ is the angle as shown in Figure 2, and is influenced by the surface tension
in which the complex number C (Supplementary Section S3) can be obtained from the initial conditions
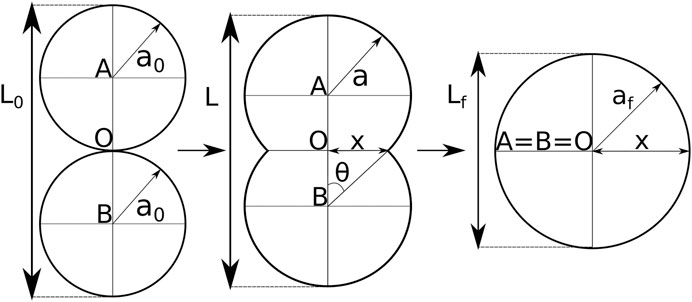
FIGURE 2. Shape evolution during spheroid fusion adapted from [3]. The shape is described by two intersecting circles with equal radius a, and center A and B. O marks the center of the axis system, and depicts the initial contact point. Based on the intersections one defines the neck radius x and the angle θ. During fusion the centers A and B gradually move toward point O, the radius a increases and the doublet length L decreases.
In analogy to previous studies on spheroid fusion, the fusion dynamics are reported as
3 Results
3.1 Arrested Coalescence Dynamics
The average dynamics of simulated tissue spheroid fusion are consistent with the derived visco-elastic material model, expressed in the time evolution of contact angle θ, Eq. 1, as shown in Figure 3B where we varied the cell activity
with initial density
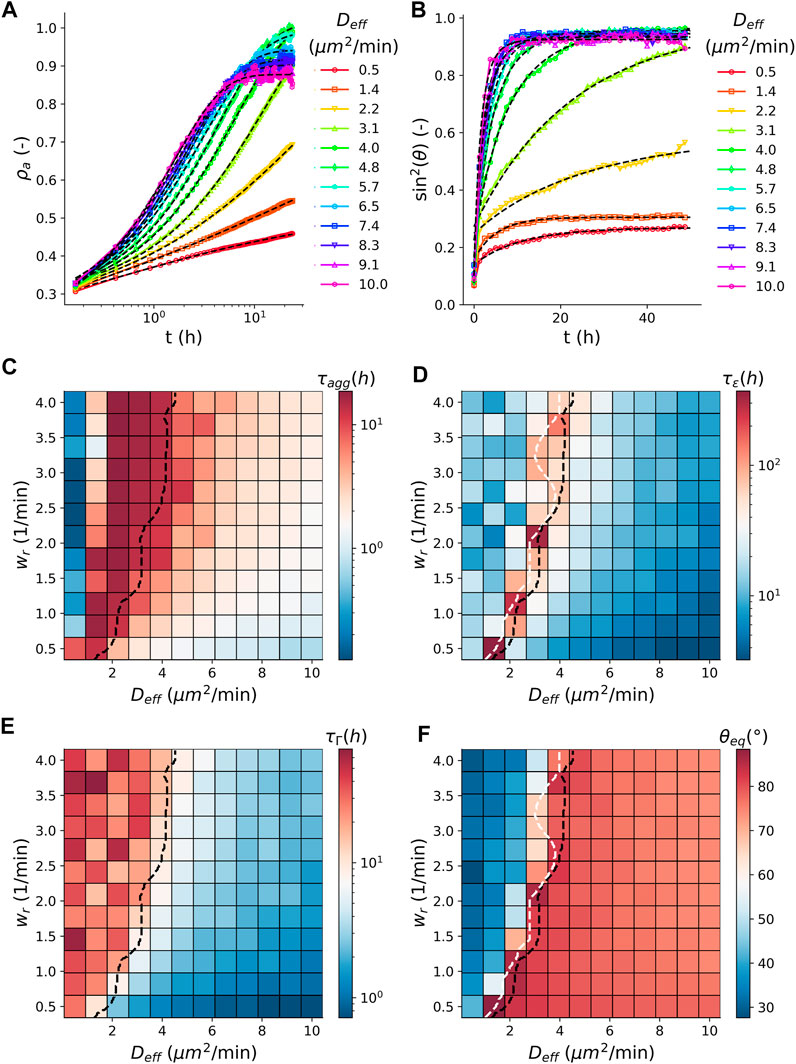
FIGURE 3. Comparison between aggregation and fusion. (A) Time evolution of the average (N = 100) apparent density
3.2 Increase of Coalescence Due to Cell Division
Next, we turn to the role of cell division in the arrest of coalescence. For this, we simulated the fusion dynamics in the presence of cell proliferation, see (Supplementary Section S1.2) Although cell proliferation is not explicitly accounted for in the derivation of our analytical model, Eq. 1 still fits the fusion dynamics of our simulated spheroid fusion in the presence of cell division well, Figure 4A. Figures 4B,C compares the characteristic visco-elastic time constant
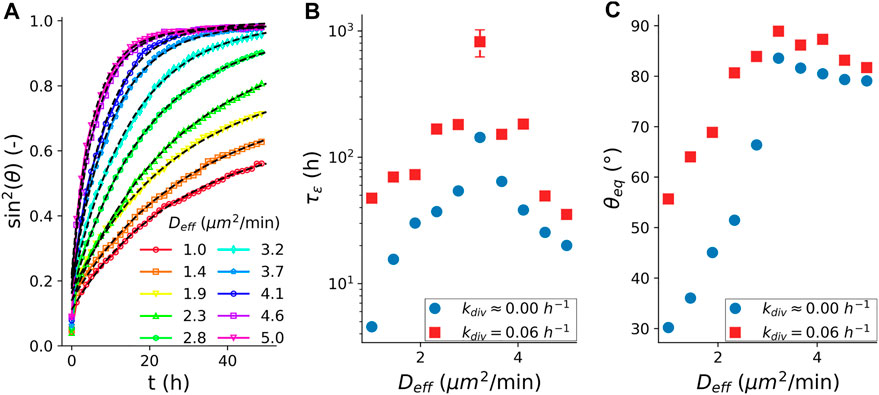
FIGURE 4. Fusion characteristics in the presence of cell division. (A) Average (N = 100) fusion dynamics with simulated cell cycle, quantified as
4 Discussion
In this work, we derived an expression for the arrested coalescence of tissue spheroid fusion, based on visco-elastic material properties. Simulations of a minimal individual cell-based model of the fusion process showed that this expression is able to describe the transition of liquid-like to arrested coalescence dynamics. Furthermore, a divergence in the visco-elastic relaxation time indicates the presence of jammed or glassy relaxation behavior near the transition toward arrested coalescence. These findings are highly similar to recent work from Oriola et al. [10], hence a brief comparison between these contemporary results is appropriate. First, the analytical expressions for the dynamics of θ (Eq. 1 in [10], compared to Eq. 1) are based on somewhat different assumptions. Our model is an extension of the model of Pokluda [3] by adding an elastic energy term in the equation which was suggested by [23, 24]. This has the advantage that in the absence of elasticity, the model of Pokluda is retrieved. The downside of our approach is that this leads to an inconsistency in the strain and strain rate for viscous energy dissipation rate and the elastic energy rate (we note this difference as ε and ε). For the viscous dissipation term, we have, according to Pokluda [3]
The glassy relaxation dynamics during fusion mirror the dynamics of the aggregation process during which the initial tissue spheroids are formed. A direct comparison between these two unit processes shows that there is a clear correspondence between, on the one hand, the transition from granular compaction to liquid dewetting during the aggregation phase, and on the other hand, the transition from arrested coalescence to liquid behavior during the fusion phase. However, this transition occurs for slightly smaller values of cell activity in the case of aggregate formation. Experimental confirmation of this correspondence can be found in studies involving Rho kinase inhibitor, which has been observed to cause arrested dynamics during aggregate formation of human periosteum-derived cells [30], as well as inhibit fusion of spheroids from human mesenchymal stem cells [8] and of embryonal chicken organoids [7]. The role of cell activity in fluidization has been experimentally shown [9]. They related cell activity as obtained from cell tracking to the outcome of fusion. The fusion of low cell activity tumor spheroids appears like a jammed tissue and results in arrested coalescence, while in high cell activity tumor spheroids, fusion is completed. They also noted that the dynamics of this arrested fusion process could not be captured with the classical model. The equation, as derived in this paper will therefore contribute to a better understanding of spheroid fusion in general.
In addition, we considered the effect of cell division on the dynamics of the fusion process. In the framework of arrested coalescence, we showed that the presence of cell division may recover coalescence of fusion. Other studies on the role of cell growth in biological active matter systems, for example in simulations of two-dimensional epithelial tissues [27], or in growing 3D cell aggregates [25], observed an increase of fluidization induced by cell division. More generally, an overview of the role of cell division in tissue rheology and mechanics is provided in [32]. However, in our simulations, the effect of cell division on the location of the fluidization transition was limited, at least for realistic cell division rates compared to the timescale of fusion. Instead, cell division appeared to increase plasticity of the glassy material and thereby improves coalescence in the arrested phase. However, it should be noted that the absence of fluidization as a result of cell division in simulations could be partly due to the minimal representation of cell shape, which introduces artificial energy barriers and thereby overly penalizes neighbor exchanges. This shortcoming could be addressed in more detailed tissue models, such as vertex models [33] or deformable cell models [34, 35]. On the other hand, our representation of the cell cycle was limited to cell growth and cell division, while fluidization is often investigated in the presence of apoptosis when the number of cells is stationary or at a homeostatic pressure [28]. We expect that including apoptosis will further fluidize the tissue as this creates random vacancies around caged cells, offering a low energy route for local relaxation. Additional complexities on fusion dynamics could arise as cells enter a pressure-dependent dormant state, increasing tissue heterogeneity. For example, the increased pressure in the core of tumor spheroids results in a jammed stage with dormant cells, while cells at the periphery grow and motility is super-diffusive [36].
In practice, technologies that involve the production of artificial tissues frequently incorporate subsequent steps of micro-aggregation and tissue assembly, where the latter often relies on the (partial) fusion of spheroids to create larger tissue constructs [37]. To complicate matters, all these steps are typically accompanied by biological processes such as cell division, production of extracellular matrix, cell differentiation or apoptosis. However, since the physical description of the underlying aggregation and fusion dynamics is highly generic, each of these steps may be parameterized in terms of its characteristic material properties, allowing for the comparison within and between different culture conditions and production formats. As such, continued efforts toward the characterization of structure, rheology and mechanics of these artificial tissues will become indispensable.
Data Availability Statement
The datasets presented in this study can be found in online repositories. The names of the Zenodo repository/repositories and accession number(s) can be found in the article/Supplementary Material.
Author Contributions
BS and HR conceived the project, SO and BS designed and conducted the simulations, SO performed the mathematical analysis, SO performed data analysis. SO, MC, JV, and BS wrote the manuscript. All authors commented on the manuscript.
Funding
This work is part of Prometheus, the KU Leuven R&D Division for Skeletal Tissue Engineering. SO acknowledges support from KU Leuven internal funding C14/18/055. MC acknowledges support form the Research Foundation Flanders (FWO), grant 1S46817N. BS acknowledges support from the Research Foundation Flanders (FWO) grant 12Z6118N.
Conflict of Interest
The authors declare that the research was conducted in the absence of any commercial or financial relationships that could be construed as a potential conflict of interest.
Acknowledgments
We thank Jiří Pešek for proofreading the derivations.
Supplementary Material
The Supplementary Material for this article can be found online at: https://www.frontiersin.org/articles/10.3389/fphy.2021.649821/full#supplementary-material
References
1. Frenkel JJ. Viscous Flow of Crystalline Bodies under the Action of Surface Tension. J Phys (1945) 9:385. doi:10.1007/BF03398399
2. Eshelby J. Discussion,’ in a.J. Shuler, ‘seminar on the Kinetics of Sintering’. Met Trans (1949) 185:806.
3. Pokluda O, Bellehumeur CT, Vlachopoulos J. Modification of Frenkel's Model for Sintering. Aiche J (1997) 43:3253–6. doi:10.1002/aic.690431213
4. McCune M, Shafiee A, Forgacs G, Kosztin I. Predictive Modeling of post Bioprinting Structure Formation. Soft matter (2014) 10:1790–800. doi:10.1039/c3sm52806e
5. Dechristé G, Fehrenbach J, Griseti E, Lobjois V, Poignard C. Viscoelastic Modeling of the Fusion of Multicellular Tumor Spheroids in Growth Phase. J Theor Biol 454 (2018) 102 – 9. doi:10.1016/j.jtbi.2018.05.005
6. Kosheleva NV, Efremov YM, Shavkuta BS, Zurina IM, Zhang D, Zhang Y, et al. Cell Spheroid Fusion: beyond Liquid Drops Model. Sci Rep (2020) 10:12614. doi:10.1038/s41598-020-69540-8
7. Pierzchalska M, Panek M, Grabacka M. The Migration and Fusion Events Related to Rock Activity Strongly Influence the Morphology of Chicken Embryo Intestinal Organoids. Protoplasma (2019) 256:575–81. doi:10.1007/s00709-018-1312-3
8. Tsai A-C, Liu Y, Yuan X, Ma T. Compaction, Fusion, and Functional Activation of Three-Dimensional Human Mesenchymal Stem Cell Aggregate. Tissue Eng Part APart A (2015) 21:1705–19. doi:10.1089/ten.TEA.2014.0314
9. Grosser S, Lippoldt J, Oswald L, Merkel M, Sussman DM, Renner F, et al. Cell and Nucleus Shape as an Indicator of Tissue Fluidity in Carcinoma. Phys Rev X (2021) 11:011033. doi:10.1103/physrevx.11.011033
10. Oriola D, Marin-Riera M, Aalderink G, Anlas K, Gritti N, Sharpe J, et al. Arrested Coalescence of Multicellular Aggregates (2020) arXiv:2012.01455.
11. Yu M, Mahtabfar A, Beelen P, Demiryurek Y, Shreiber DI, Zahn JD, et al. Coherent Timescales and Mechanical Structure of Multicellular Aggregates. Biophysical J 114 (2018) 2703 – 16. doi:10.1016/j.bpj.2018.04.025
12. Guevorkian K, Colbert M-J, Durth M, Dufour S, Brochard-Wyart F, Aspiration of Biological Viscoelastic Drops. Phys Rev Lett (2010) 104:218101. doi:10.1103/PhysRevLett.104.218101
13. Gonzalez-Rodriguez D, Bonnemay L, Elgeti J, Dufour S, Cuvelier D, Brochard-Wyart F. Detachment and Fracture of Cellular Aggregates. Soft Matter (2013) 9:2282–90. doi:10.1039/C2SM26648B
14. Forgacs G, Foty RA, Shafrir Y, Steinberg MS. Viscoelastic Properties of Living Embryonic Tissues: a Quantitative Study. Biophysical J 74 (1998) 2227–34. doi:10.1016/S0006-3495(98)77932-9
15. Mongera A, Rowghanian P, Gustafson HJ, Shelton E, Kealhofer DA, Carn EK, et al. A Fluid-To-Solid Jamming Transition Underlies Vertebrate Body axis Elongation. Nature (2018) 561:401–5. doi:10.1038/s41586-018-0479-2
16. Yang X, Mironov V, Wang Q. Modeling Fusion of Cellular Aggregates in Biofabrication Using Phase Field Theories. J Theor Biol 303 (2012) 110–8. doi:10.1016/j.jtbi.2012.03.003
17. Fleming PA, Argraves WS, Gentile C, Neagu A, Forgacs G, Drake CJ. Fusion of Uniluminal Vascular Spheroids: A Model for Assembly of Blood Vessels. Dev Dyn (2010) 239:398–406. doi:10.1002/dvdy.22161
18. Flenner E, Janosi L, Barz B, Neagu A, Forgacs G, Kosztin I. Kinetic Monte Carlo and Cellular Particle Dynamics Simulations of Multicellular Systems. Phys Rev E (2012) 85:031907. doi:10.1103/PhysRevE.85.031907
19. Flenner E, Marga F, Neagu A, Kosztin I, Forgacs G. Relating Biophysical Properties across Scales. Schnell S, Maini PK, Newman SA, and Newman TJ, editors. Relating Biophysical Properties across Scales. Multiscale Modeling of Developmental SystemsCurrent Topics in Developmental Biology, 81. Academic Press (2008). p. 461–83. doi:10.1016/S0070-2153(07)81016-7
20. Kosztin I, Vunjak-Novakovic G, Forgacs G. Colloquium: Modeling the Dynamics of Multicellular Systems: Application to Tissue Engineering. Rev Mod Phys (2012) 84:1791–805. doi:10.1103/RevModPhys.84.1791
21. Shafiee A, McCune M, Forgacs G, Kosztin I. Post-deposition Bioink Self-Assembly: a Quantitative Study. Biofabrication (2015) 7:045005. doi:10.1088/1758-5090/7/4/045005
22. Schötz E-M, Lanio M, Talbot JA, Manning ML. Glassy Dynamics in Three-Dimensional Embryonic Tissues. J R Soc Interf (2013) 10:20130726. doi:10.1098/rsif.2013.0726
23. Pawar AB, Caggioni M, Hartel RW, Spicer PT. Arrested Coalescence of Viscoelastic Droplets with Internal Microstructure. Faraday Discuss (2012) 158:341–35. doi:10.1039/c2fd20029e
24. Dahiya P, Caggioni M, Spicer PT. Arrested Coalescence of Viscoelastic Droplets: Polydisperse Doublets. Phil Trans R Soc A (2016) 374:20150132. doi:10.1098/rsta.2015.0132
25. Ranft J, Basan M, Elgeti J, Joanny J-F, Prost J, Jülicher F. Fluidization of Tissues by Cell Division and Apoptosis. Proc Natl Acad Sci (2010) 107:20863–8. doi:10.1073/pnas.1011086107
26. Malmi-Kakkada AN, Li X, Samanta HS, Sinha S, Thirumalai D. Cell Growth Rate Dictates the Onset of Glass to Fluidlike Transition and Long Time Superdiffusion in an Evolving Cell colony. Phys Rev X (2018) 8:021025. doi:10.1103/PhysRevX.8.021025
27. Matoz-Fernandez DA, Martens K, Sknepnek R, Barrat JL, Henkes S. Cell Division and Death Inhibit Glassy Behaviour of Confluent Tissues. Soft Matter (2017) 13:3205–12. doi:10.1039/C6SM02580C
28. Basan M, Prost J, Joanny J-F, Elgeti J. Dissipative Particle Dynamics Simulations for Biological Tissues: Rheology and Competition. Phys Biol (2011) 8:026014. doi:10.1088/1478-3975/8/2/026014
29. Smeets B, Pešek J, Deckers T, Hall GN, Cuvelier M, Ongenae S, et al. Compaction Dynamics during Progenitor Cell Self-Assembly Reveal Granular Mechanics. Matter 2 (2020) 1283 – 95. doi:10.1016/j.matt.2020.02.016
30. Smeets B, Pešek J, Deckers T, Hall GN, Cuvelier M, Ongenae S, et al. Compaction Dynamics during Progenitor Cell Self-Assembly Reveal Granular Mechanics. Matter 2 (2020) 1283 – 95. doi:10.1016/j.matt.2020.02.016
31. Delile J, Herrmann M, Peyriéras N, Doursat R. A Cell-Based Computational Model of Early Embryogenesis Coupling Mechanical Behaviour and Gene Regulation. Nat Commun (2017) 8:13929. doi:10.1038/ncomms13929
32. Godard BG, Heisenberg C-P. Cell Division and Tissue Mechanics. Curr Opin Cel Biol 60 (2019) 114 – 20. doi:10.1016/j.ceb.2019.05.007
33. Krajnc M, Dasgupta S, Ziherl P, Prost J. Fluidization of Epithelial Sheets by Active Cell Rearrangements. Phys Rev E (2018) 98:022409. doi:10.1103/PhysRevE.98.022409
34. Van Liedekerke P, Neitsch J, Johann T, Alessandri K, Nassoy P, Drasdo D. Quantitative Cell-Based Model Predicts Mechanical Stress Response of Growing Tumor Spheroids over Various Growth Conditions and Cell Lines. Plos Comput Biol (2019) 15:e1006273. doi:10.1371/journal.pcbi.1006273
35. Smeets B, Cuvelier M, Pešek J, Ramon H. The Effect of Cortical Elasticity and Active Tension on Cell Adhesion Mechanics. Biophysical J (2019) 116:930–7. doi:10.1016/j.bpj.2019.01.015
36. Sinha S, Malmi-Kakkada AN, Li X, Samanta HS, Thirumalai D. Spatially Heterogeneous Dynamics of Cells in a Growing Tumor Spheroid: Comparison between Theory and Experiments. Soft Matter (2020) 16:5294–304. doi:10.1039/C9SM02277E
37. De Moor L, Fernandez S, Vercruysse C, Tytgat L, Asadian M, De Geyter N, et al. Hybrid Bioprinting of Chondrogenically Induced Human Mesenchymal Stem Cell Spheroids. Front Bioeng Biotechnol (2020) 8:484. doi:10.3389/fbioe.2020.00484
Keywords: spheroid fusion, arrested coalescence, tissue rheology, visco-elastic model, individual cell-based model, glass transition, tissue engineering, organoids
Citation: Ongenae S, Cuvelier M, Vangheel J, Ramon H and Smeets B (2021) Activity-Induced Fluidization and Arrested Coalescence in Fusion of Cellular Aggregates. Front. Phys. 9:649821. doi: 10.3389/fphy.2021.649821
Received: 05 January 2021; Accepted: 21 May 2021;
Published: 09 June 2021.
Edited by:
Karine Guevorkian, UMR168 Unite Physico-Chimie Curie (PCC), FranceReviewed by:
Jens Elgeti, Helmholtz-Verband Deutscher Forschungszentren (HZ), GermanyAndrej Vilfan, Max Planck Society (MPG), Germany
Copyright © 2021 Ongenae, Cuvelier, Vangheel, Ramon and Smeets. This is an open-access article distributed under the terms of the Creative Commons Attribution License (CC BY). The use, distribution or reproduction in other forums is permitted, provided the original author(s) and the copyright owner(s) are credited and that the original publication in this journal is cited, in accordance with accepted academic practice. No use, distribution or reproduction is permitted which does not comply with these terms.
*Correspondence: Bart Smeets, YmFydC5zbWVldHNAa3VsZXV2ZW4uYmU=