- 1PoreLab, Department of Physics, University of Oslo, Oslo, Norway
- 2PoreLab, Department of Chemistry, Norwegian University of Science and Technology, Trondheim, Norway
- 3PoreLab, Department of Physics, Norwegian University of Science and Technology, Trondheim, Norway
- 4Beijing Computational Sciences Research Center, CSRC, Beijing, China
By means of a particle model that includes interactions only via the local particle concentration, we show that hyperballistic diffusion may result. This is done by findng the exact solution of the corresponding non-linear diffusion equation, as well as by particle simulations. The connection between these levels of description is provided by the Fokker-Planck equation describing the particle dynamics. PACS numbers:
I Introduction
Superdiffusion is characterized by the fact that the root mean square displacement of some kind of particles, increases with time t as
Biological examples may be found in the foraging movement of spider monkeys [5] and the flight paths of albatrosses [6, 7]; in both cases
However, the mere observation that the step length distribution has a fat tail, does not by itself provide any physical model to explain the superdiffusive behavior. The simplest physical example of superdiffusion is perhaps provided by the undamped Langevin equation which describes a random walk in momentum space and a corresponding real space displacement with
Anomalous diffusion of the subdiffusive kind has been studied in a wide range of contexts: It may be observed in compressible gases flowing through porous media [13, 14], a pulse of energy propagating in vacuum [15], or in filtration processes [16]. Another example is heat diffusion at high temperature [17, 18]. Population dynamics gives rise to this kind of behavior [19–21], as does water ingress in zeolites as studied by Azevedo et al. [22, 23] and Fischer et al. [24]. The diffusion of grains in granular media considered by Christov and Stone [25] is yet another example. Pritchard et al., [26] studied gravity-driven fluid flow in layered porous media finding that the fluid motion could be described by a concentration-dependent diffusivity as did Hansen et al. [27] for the spreading of wetting films in wedges. Anomalous diffusion in random geometries, fractals and tree-like structures has been studied for decades [28–32]. Common to all of these examples is subdiffusion,
Hyperballistic diffusion seems almost a contradiction in terms, for how could a random walker move faster than a directed walker that never changes direction? The explanation lies in the fact that the velocity, and thus the step length, keeps increasing with time without limits. This behavior is of course unphysical in the context of the Langevin equation as there will always be dissipative forces that match the fluctuations, but has a physical basis in random potentials. On the other hand, in a hydrodynamic shear-flow that increases without bounds, a random walker will achieve step-lengths that are umlimited too [33, 34], an effect that may give rise to hyper-ballistic diffusion. Without diverging velocities or step lengths, long range time-correlations are required for superdiffusion, an example being the elephant random walk, so named because both the walkers and elephants have long memories, which in the model give rise to (sub-ballistic) superdiffusion [35].
Generally, superdiffusion has been modeled by independent agents interacting with an environment, or possessing a long term memory [36]. The main question of the present article is if superdiffusion, including the hyperballistic case, could result directly from a Markovian description of particle interactions. Such interactive systems could include crowds of people, bacteria swimmers competing for food [37, 38] or the evolution of the porosity in a granular packing. For the purpose of addressing this question we investigate the potentially simplest description of particle interactions, namely, that where a conserved concentration C of particles is governed by Ficks law
II Solution to the Non-Linear Diffusion Equation
Already in 1959 did Pattle [39] solve the diffusion equation
where
where d is the dimension. For negative γ this will always lead to sub-diffusion. We have recently shown that in
To validate the mean field description and provide it with a physical basis, we introduce a particle model that is described by Eq. 1. The step lengths in this model
Following the same lines as in [40] we rewrite Eq. 1 as
Hence, we see that we need
We are free to chose λ such that
where we have introduced
for some dimensionless constant c, which can be absorbed in the definition of
Note That This Form Immediately Gives
with τ given by Eq. 2.
From Eq. 6, we also have an expression for
which can be integrated to give,
For Fick’s law to be valid throughout the domain,
where k is an integration constant. This expression is independent of the dimension d. The value of the constant k can be determined through the normalization,
and yields the concentration field by means of Eq. 5; 11. The mean square displacement is given by
which is limited to the range of γ-values where the integrals in Eq. 8 converge. Since
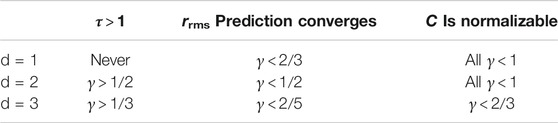
TABLE 1. Behavior with γ in various dimensions d as predicted by Eq. 2.
Interestingly, there exists an alternative route to the solution given in Eq. 5; 11: Working in
III Particle Model that Realizes the Non-Linear Diffusion Equation
We will employ two simulation models, both in
where α is a Cartesian index and the function
Here
where
and requiring equivalence with Eq. 3 thus implies that
where the random variable η is given above.This defines the particle model that is described by Eq. 3.
In the finite interaction range model C is calculated by assuming a maximum interaction range
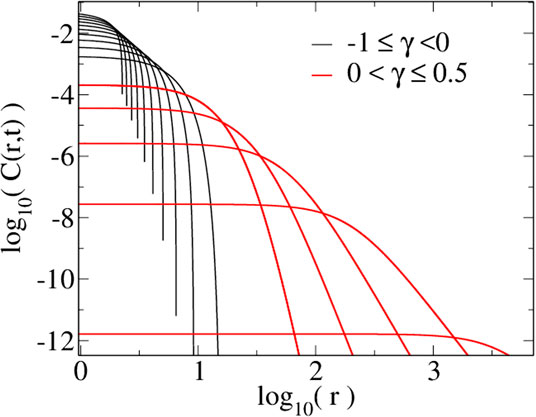
FIGURE 1. (A) Simulations of
The other, infinite interaction range model employs no lattice at all, but evaluates C at any particle position
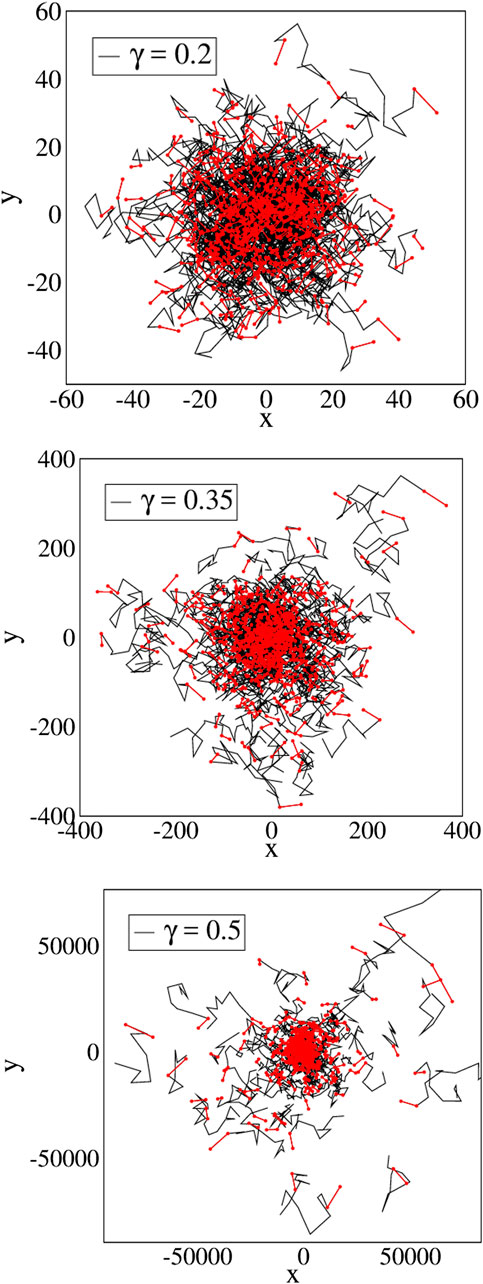
FIGURE 2. The sphere of volume
There is no upper limit to the size of
In Figure 3 the analytic solution of Eq. 11 is plotted for different γ-values. The term “explosive” seems an appropriate label for the behavior of the concentration for two reasons: First, as
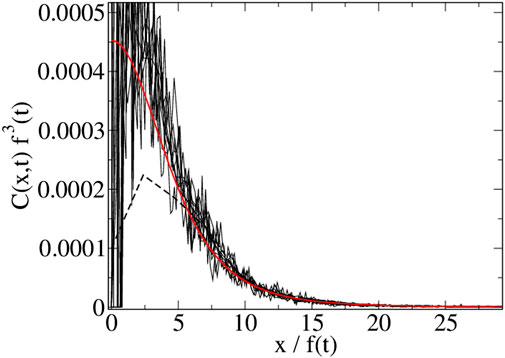
FIGURE 3. The predicted/theoretical concentration field at different γ-values when
Figure 4 show simulations using dimensionless spatial and time coordinates. If units were assigned to them the background diffusivity
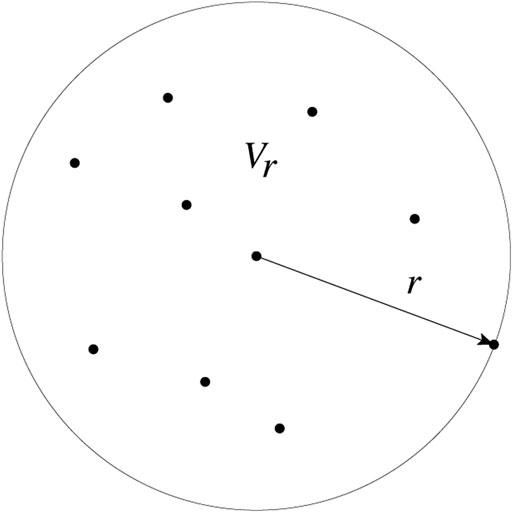
FIGURE 4. Projections into the xy-plane of particle trajectories for different values of γ, using the infinite-interaction-range model. The last 10 time steps are shown in black the last step in red. All simulations are run for a time
In Figure 5 the data collapse anticipated in Eq. 5 is seen to be satisfied. Figures 1A,B demonstrate that the particle displacement is in fact characterized by Eq. 13, the difference between Figures 1A,B, being that the first figure compares simulations and the full analytic prediction of Eq. 13, while the hyperballistic transport shown in Figures 1B, only confirms the prediction of the τ exponent, Eq. 2. Note that in Figures 1A the convergence to the prediction of Eq. 13, happens over a time that increases with γ, signaling the end of the regime where
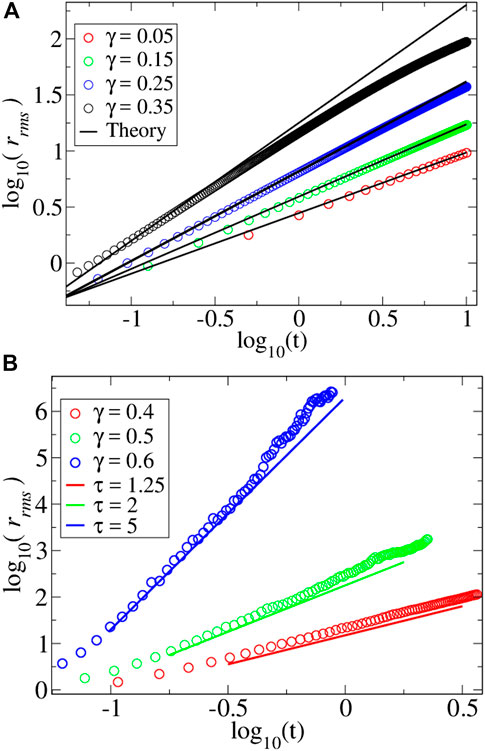
FIGURE 5. Simulations, sampled at equispaced time intervals (the stapled curve shows the first time) using
Figure 6 summarizes this comparison for the full range of relevant γ-values, using the finite-range model for the smaller- and the infinite range model for the larger γ-values.
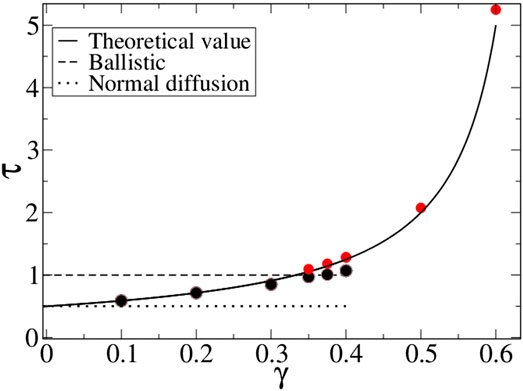
FIGURE 6. Simulation results for τ using the finite range
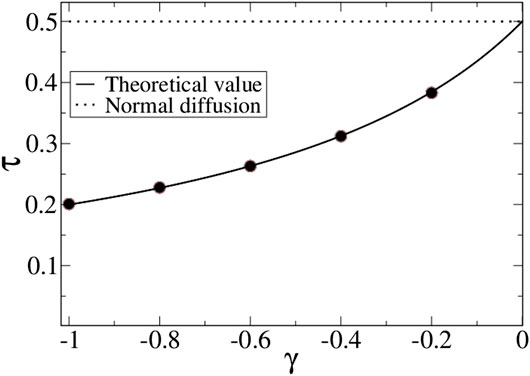
FIGURE 7. Simulation results for τ using the finite range
IV Conclusion
In conclusion, we have shown that particle interactions described entirely in terms of their local concentration may yield superdiffusion, and even hyperballistic diffusion. This was done by solving the diffusion equation with the diffusivity
Data Availability Statement
The raw data supporting the conclusions of this article will be made available by the authors, without undue reservation.
Author Contributions
All authors contributed to the analytic work and discussions. EGF did the simulations and the writing of the paper.
Funding
This work was partly supported by the Research Council of Norway through its Centers of Excellence funding scheme, project number 262644.
Conflict of Interest
The authors declare that the research was conducted in the absence of any commercial or financial relationships that could be construed as a potential conflict of interest.
Appendix
In Pattles classical 1959 paper [39] the
where we have used the isotropic nature of the problem to perform the angular integration and thus introduced the geometric factor
We see from Eq. 11 that, for
yielding the normalization constant
for
We now combine results, using Eqs. 5, 7; 11. to find the concentration field,
where
By comparison, for
with k given by Eq. 12 now. Finally we find that for
In Figure 7 this behavior is confirmed by simulations using the finite-range model.
References
1. Bouchaud J, Georges A. Anomalous diffusion in disordered media: statisticalmechanisms, models, and physical applications. Phys Rep (1990) 195:125. doi:10.1016/0370-1573(90)90099-n
2. Gosh SK, Cherstvy AG, Grebenkov D, Metzler R. Anomalous non-gaussiantracer diffusion in crowded two-dimensional environments. New J Phys (2016) 18:013027. 10.1088/1367-2630/18/1/013027
3. Richardson L. Atmospheric diffusion shown on a distance-neighbour graph. Proc Roy Soc London A (1926) 100:709. 10.1098/rspa.1926.0043
4. Schlesinger M, West B, Klafter J. Levy dynamics of enhanced diffusion: application to turbulence. Rev Geophys (1987) 58:1101. 10.1103/PhysRevLett.58.1100
5. Ramos-Fernundez G, Mateos J, Miramontes O, Cocho G, Larralde H, Ayala-Orozco B. Levy walk patterns in the foraging movements of spidermonkeys (Ateles geoffroyi). Behav Ecol Sociobiol (2004) 55:223. 10.1007/s00265-003-0700-6
6. Viswanathan GM, Afanasyev V, Buldyrev SV, Murphy EJ, Prince PA, Stanley HE. Lévy flight search patterns of wandering albatrosses. Nature (1996) 381:413. doi:10.1038/381413a0
7. Viswanathan GM, Buldyrev SV, Havlin S, da Luz MGE, Raposo EP, Stanley HE. Optimizing the success of random searches. Nature (1999) 401:911914. doi:10.1038/44831
8. Jayannavar AM, Kumar N. Nondiffusive quantum transport in a dynamically disordered medium. Phys Rev Lett (1982) 48:553. doi:10.1103/physrevlett.48.553
9. Golubovic L, Feng S, Zeng FA. Classical and quantum superdiffusion in a time-dependent random potential. Phys Rev Lett (1991) 67:2115. doi:10.1103/PhysRevLett.67.2115
10. Levi L, Krivolapov Y, Fishman S, Segev M. Hyper-transport of light and stochastic acceleration by evolving disorder. Nat Phys (2012) 8:912. doi:10.1038/nphys2463
11. Sagi Y, Brook M, Almog I, Davidson N. Observation of anomalous diffusion and fractional self-similarity in one dimension. Phys Rev Lett (2012) 108:093002. doi:10.1103/physrevlett.108.093002
12. Anderson PW. Absence of diffusion in certain random lattices. Phys Rev (1958) 109:1492. doi:10.1103/physrev.109.1492
13. Muskat M. The flow of fluids through porous media. J Appl Physics (1937) 8:274. doi:10.1063/1.1710292
14. Barenblatt GI, Entov VM, Ryzhik VM. Theory of fluid flows through natural rocks, Vol. ber90. Dordrecht, Netherland: Kluwer (1990). 396 p.
15. Hurtado PI, Krapivsky PL. Compact waves in microscopic nonlinear diffusion. Phys Rev E (2012) 85:060103. doi:10.1103/physreve.85.060103
16. Kamenomostskaya SK. Similar solutions and the asymptotics of filtration equations. Arch Rational Mech Anal (1976) 60, 171. doi:10.1007/bf00250678
17. Zeldovich IB, Kompaneez AS. “On the theory of heat propagation with heatconduction depending on temperature,” in Lectures dedicated on the 70th anniversary of A. F. Joffe. Moscow, Russia: Akad. Nauk SSSR (1950). p. 61–71.
18. Zeldovich Y, Raizer YP. Physics of shock waves and high-temperature hydrodynamic phenomena, Vol. 2. New York, NY: Academic Press (1968). p. 826–7.
19. Gurtin ME, MacCamy RC. On the diffusion of biological populations. Math Biosci (1977) 33:35. doi:10.1016/0025-5564(77)90062-1
20. Newman WI. Some exact solutions to a non-linear diffusion problem in population genetics and combustion. J Theor Biol (1980) 85:325. doi:10.1016/0022-5193(80)90024-7
22. de Azevedo EN, de Sousa PL, de Souza RE, Engelsberg M, Miranda MDNDN, Silva MA. Concentration-dependent diffusivity and anomalous diffusion: a magnetic resonance imaging study of water ingress in porous zeolite. Phys Rev E (2006a) 73:011204. doi:10.1103/physreve.73.011204
23. de Azevedo EN, da Silvaandde Souzaand DV, Engelsberg RE, Engelsberg M. Water ingress in y-type zeolite: anomalous moisture-dependent transport diffusivity. Phys Rev E(2006b) 74:041108. doi:10.1103/physreve.74.041108
24. Fischer D, Finger T, Angenstein FRSR. Diffusive and subdiffusive axial transport of granular material in rotating mixers. Phys Rev E (2009) 80:061302. doi:10.1103/physreve.80.061302
25. Stannarius IC, Has HA. Resolving a paradox of anomalous scalings in the diffusion of granular materials. Proc Natl Acad Sci (2012) 109:16012. doi:10.1073/pnas.1211110109
26. Stone D, Woods AW, Hogg AJ. On the slow draining of a gravity current moving through a layered permeable medium. J Fluid Mech (2001) 444:23. doi:10.1017/s002211200100516x
27. Hansen A, Skagerstam B-S, Tørå G. Anomalous scaling and solitary waves in systems with nonlinear diffusion. Phys Rev E (2011) 83:056314. doi:10.1103/physreve.83.056314
28. Shlesinger MF. Asymptotic solutions of continuous-time random walks. J Stat Phys (1974) 10:421. doi:10.1007/bf01008803
29. Havlin S, Kiefer JE, Weiss GH. Anomalous diffusion on a random comblike structure. Phys Rev A (1987) 36:1403. doi:10.1103/physreva.36.1403
30. Havlin S, Djordjevic ZV, Majid I, Stanley HE, Weiss GH. Relation between dynamic transport properties and static topological structure for the lattice-animal model of branched polymers. Phys Rev Lett (1984) 53:178. doi:10.1103/physrevlett.53.178
31. de Gennes PG. La percolation un concept unificateur, La Recherche. Singapore: World Scientific Publishing (1976). 919 p.
32. ben-Avraham D, Havlin S. Diffusion and reactions in fractals and disordered systems. London, United Kingdom: Cambridge University Press (2000). 316 p.
33. ben-Avraham D, Leyvraz F, Redner S. Superballistic motion in a “random-walk” shear flow. Phys Rev A (1992) 45:2315. doi:10.1103/physreva.45.2315
34. ben-Naim E, Redner S, ben-Avraham D. Bimodal diffusion in power-law shear flows. Phys Rev A (1992) 45:7207. doi:10.1103/physreva.45.7207
35. Schütz GM, Trimper S. Elephants can always remember: exact long-range memory effects in a non-markovian random walk. Phys Rev E (2004) 70:045101. doi:10.1103/physreve.70.045101
36. Morgado R, Oliveira FA, Batrouni GG, Hansen A. Relation between anomalous and normal diffusion in systems with memory. Phys Rev Lett (2002) 89:100601. doi:10.1103/physrevlett.89.100601
37. Wei X, Bauer W. Starvation-induced changes in motility, chemotaxis, and flagellation of rhizobium melilot. Appl Abd Environ Microbiol (1998) 65:1708–14. doi:10.1128/AEM.64.5.1708-1714.1998
38. Wu X-L, Libchaber A. Particle diffusion in a quasi-two-dimensional bacterial bath. Phys Rev Lett (2000) 84:3017. doi:10.1103/physrevlett.84.3017
39. Pattle RE. Diffusion from an instantaneous point source with a concentration-dependent coefficient. Q J Mech Appl Math (1959) 12:407. doi:10.1093/qjmam/12.4.407
40. Hansen A, Flekkøy EG, Baldelli B. Anomalous diffusion in systems with concentration-dependent diffusivity: exact solutions and particle simulations. Front Phys (2020) 8:519624. doi:10.3389/fphy.2020.519624
41. Plastino AR, Plastino A. Non-extensive statistical mechanics and generalized Fokker-Planck equation. Physica A (1995) 222:347. doi:10.1016/0378-4371(95)00211-1
42. Tsallis C, Bukman DJ. Anomalous diffusion in the presence of external forces: exact time-dependent solutions and their thermostatistical basis. Phys Rev E (1996) 54:R2197. doi:10.1103/physreve.54.r2197
43. van Kampen N. Stochastic processes in physics and chemistry. 3rd ed, Vol. k07. Amsterdam: North Holland (2007). 464 p.
Keywords: anomalous diffusion, concentration-dependent diffusivity, non-linear diffusion equation, brownian motion (wiener process), random walks
Citation: Flekkøy EG, Hansen A and Baldelli B (2021) Hyperballistic Superdiffusion and Explosive Solutions to the Non-Linear Diffusion Equation. Front. Phys. 9:640560. doi: 10.3389/fphy.2021.640560
Received: 11 December 2020; Accepted: 18 January 2021;
Published: 17 March 2021.
Edited by:
Fernando A. Oliveira, University of Brasilia, BrazilReviewed by:
Marie-Christine Firpo, Center National de la Recherche Scientifique (CNRS), FranceHaroldo V. Ribeiro, State University of Maringá, Brazil
Copyright © 2021 Flekkøy, Hansen and Baldelli. This is an open-access article distributed under the terms of the Creative Commons Attribution License (CC BY). The use, distribution or reproduction in other forums is permitted, provided the original author(s) and the copyright owner(s) are credited and that the original publication in this journal is cited, in accordance with accepted academic practice. No use, distribution or reproduction is permitted which does not comply with these terms.
*Correspondence: Eirik G. Flekkøy, Zmxla2tveUBmeXMudWlvLm5v