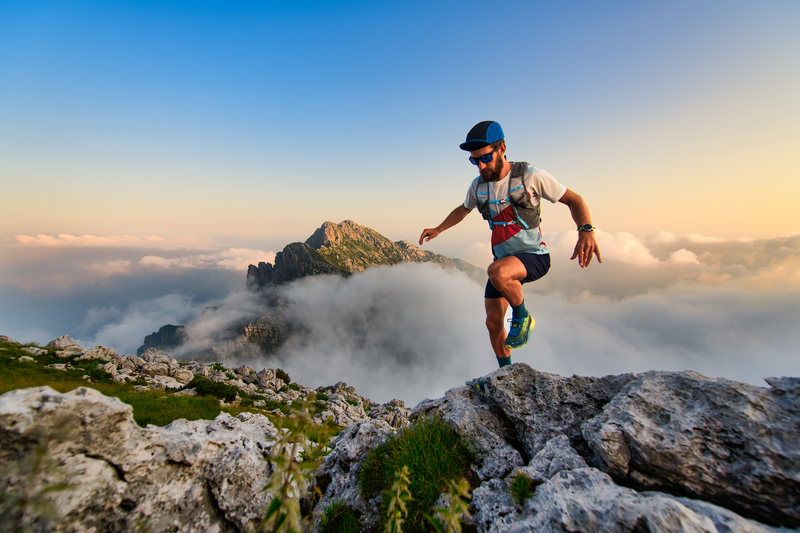
95% of researchers rate our articles as excellent or good
Learn more about the work of our research integrity team to safeguard the quality of each article we publish.
Find out more
ORIGINAL RESEARCH article
Front. Phys. , 23 February 2021
Sec. Social Physics
Volume 9 - 2021 | https://doi.org/10.3389/fphy.2021.639252
Reward is an effective mechanism that promotes cooperation. However, an individual usually reward her opponents in certain cases. Inspired by this, a conditional neutral reward mechanism has been introduced. In detail, an individual will reward his or her neighbors with the same strategy when the payoff of the focal one is higher than that of his or her neighbors. And simulations are conducted to investigate the impact of our mechanism on the evolution of cooperation. Interestingly, cooperation can survive and dominate the system. Nominal antisocial reward that defectors reward each other is rarely because of the greed of defectors. By contrast, cooperators inside the cooperative clusters share the payoff with cooperators on the boundary so that the latter can form shields to protect cooperators.
How cooperation among selfish individuals can emerge and maintain of has been an attractive question in biology, sociology, and many different fields [1–5]. For example, worker ants give up their reproductive capacity to build nests and collect food. And human beings play different roles in social division of labor. In order to explain the widespread phenomenon of cooperation, evolutionary game theory has been proposed and provides a powerful mathematical framework [6–11]. In many game models, PDG (prisoner's dilemma game) is regarded as a paradigm due to capturing the essence of cooperation. In the PDG, two players choose cooperation (C) or defection (D) at the same time without being known by the opponent. If they both choose cooperation or defection, they will both receive the reward (R) or get the punishment (P). However, if one chooses cooperation but the other chooses cooperation, the defector will get the temptation (T) while the cooperator will get the sucker’s payoff (S). For PDG, the ranking rules are T > R > P > S and 2R > T + S. Obviously, the better choice is always defection no matter which strategy the other chooses. But if two individuals both defects, they will receive the less payoff than both cooperating. This is the dilemma.
In the landmark work of Nowak, the mechanism of spatial topology, widely known as spatial reciprocity, has proved to be an effective mechanism to promote cooperative coevolution [12–38]. Inspired by this, many kinds of spatial topologies are applicated to study the cooperative dynamics in evolution, such as square lattice network, ER random network, small-world network, BA scale-free network and so on [39–47]. Besides, to explain cooperation on the spatial topologies, different mechanisms have been proposed, such as reputation, asymmetric interaction, different update rule, co-evolution of dynamical rules, reward or punishment and so on [48–53].
Recent research has shown that rewarding is an effective way to promote cooperation. Various rewards are often given to those who perform well, which is very common in the real society. In this paper, we consider a reward mechanism that the individual could pay a cost to reward the neighbors who has the same strategy. Meanwhile, he could be rewarded by his neighbors. We find that rewards have a positive effect on the maintenance of cooperation, which is manifested in the fact that the weakened cooperators are supported against the invasion of defectors by the population of their kinds, in the form of rewards, while it is opposite for defectors. This creates a unique boundary structure. The reminder of this paper is organized as follows. First, we describe our model detailly. Then, we show the simulation results with figures and try to give an explanation. Finally, we summarize and give the discussion about the conclusions.
We introduce social reward in the PDG (prisoner’s dilemma game) on L*L square lattices, where each player occupies one and is surrounded by four neighbors. Each player will be initialized as either C (cooperator) or D (defector) with the same possibility. We use the standard PDG by setting T = b (1 < b < 2), R = 1, P = 0, and S = 0. The value of b specifies the strength of the dilemma [54–56]. Hence, the payoff matrix of PDG is described as follows:
A player x is chosen randomly at the beginning of each time step, whose payoff
where
If payoff of player x is higher than his neighbors’ or equal, he will pay a cost to reward each his neighbors who have the same strategy. Otherwise, his payoff remains the same. Meanwhile, his four neighbors follow the same procedure. The accumulated payoff of player x at current time step is:
where
Finally, player x updates his strategy. A neighbor y is chosen and player x learns the strategy of y randomly with the probability as following:
where K indicates the amplitude of noise that also called intensity of selection [57–62]. Without loss of generality, we set K = 0.1.
The Monte Carlo simulation is carried out with setting L = 200, and the number of all step is set to
In order to verify the impact of our reward mechanism on cooperation, we give a contour plot as Figure 1, where the simulation result of fraction of cooperation
FIGURE 1. Fraction of cooperation on the b-r parameter space when c = 0.01. (A) and (B) give the results simulated on square lattice and WS small world network.
Figure 2 shows the impact of both cost
FIGURE 3. Schematic of the boundary structure. Cooperator (defectors) are presented in red (blue). We show the payoffs of the nodes in the dotted boxes.
In Figure 3, we show the payoffs of a cooperator and a defector on the boundary, which are marked with dotted boxes. For the defector, first, he gets payoff
As mentioned before, the supports of cooperation clusters to cooperators on the boundaries, and on the contrary for defectors on the boundaries of defection clusters, may be the potential reasons for promoting cooperation. To confirm that, we show the characteristic snapshots in Figure 4, where different types of nodes called cooperator (C), cooperative rewarder (CR), defector (D) and defective rewarder (DR), are marked in four different colors. Here we fixed cost = 0.1 and from top to bottom, the reward is set as 0.1, 0.3, 0.6 respectively. Obviously, the evolution of game is very different under different values of reward when the value of cost is fixed. It is worth mentioning that distributions CR-C-DR-D as shown in Figure 3 indeed appear on the boundaries among cluster of cooperators and cluster of defectors at any value of reward. However, different
FIGURE 4. Initial evolution of the prepared scenario. The top, medium and bottom correspond to r values of 0.1, 0.3, 0.6 respectively. From left to right, the snapshots correspond to MCS = 0, 1, 10, 100, and 50,000. Cooperator (C), cooperative rewarder (CR), defector (D) and defective rewarder (DR) are shown in color cyan, blue, magenta, and red respectively.
Figure 5 shows how the size of the largest cluster of all kinds of cooperators (including cooperators and cooperative rewarders)
FIGURE 5. The size of the largest cluster of all kinds of cooperators (including cooperators and cooperative rewarders)
In the real world, individuals are more willing to reward other participants according to certain conditions rather than directly reward them. Hence, we explore the effects of neutral and conditional rewards in structural groups. By numerical simulation, we find that cooperation can be greatly promoted, while conditional antisocial reward does not prevent the evolution of cooperation. From the micro perspective, we provide some evidence to prove that our mechanism enhances the spatial reciprocity and is conducive to the formation of cooperation clusters. In our model, the individuals in the cooperative cluster reward the same kind of individuals on the boundary, so that the latter can form a shield to protect the former. On the contrary, defectors on the border will gradually reduce themselves after rewarding similar individuals inside. By and large, Social reward rather than antisocial reward shapes the direction of collective behavior when an individual rewards others under the condition that her payoff is higher. We hope our work is helpful to resolve the social dilemmas in real society.
The original contributions presented in the study are included in the article/Supplementary Material, further inquiries can be directed to the corresponding authors.
YT designed the research, YT and YY performed the research, MJ and YY analyzed the results, YT and MJ wrote the manuscript. All authors reviewed and approved the manuscript.
This work was supported by the Science Foundation of Yunnan Province under Grant No. 202001BB050063 and the Open Foundation of Key Laboratory in Software Engineering of Yunnan Province under Grant No. 2020SE315.
The authors declare that the research was conducted in the absence of any commercial or financial relationships that could be construed as a potential conflict of interest.
1. Axelrod R, Hamilton WD. The evolution of cooperation. Science (1981) 211(80):1390–6. doi:10.1126/science.7466396
2. Wingreen NS, Levin SA. Cooperation among microorganisms. Plos Biol (2006) 4:e299–1488. doi:10.1371/journal.pbio.0040299
3. Morgenstern O, von Neumann J. Theory of games and economic behavior. commemorative edition. Princeton, NJ: Princeton University Press (2007).
4. Nowak MA, Sasaki A, Taylor C, Fudenberg D. Emergence of cooperation and evolutionary stability in finite populations. Nature (2004) 428:646–50. doi:10.1038/nature02414
5. Castellano C, Fortunato S, Loreto V. Statistical physics of social dynamics. Rev Mod Phys (2009) 81:591–646. doi:10.1103/RevModPhys.81.591
6. Jm S. Evolution and the theory of games. Cambridge, United Kingdom: Cambridge university press (1982) doi:10.1017/CBO9780511806292
7. Ritzberger K, Weibull JW. Evolutionary selection in normal-form games. Econometrica (1995) 63:1371. doi:10.2307/2171774
8. Hofbauer J, Sigmund K. Evolutionary games and population dynamics. Cambridge, United Kingdom: Cambridge University Press (1998). doi:10.1017/cbo9781139173179
9. Szabó G, Tőke C. Evolutionary prisoner’s dilemma game on a square lattice. Phys Rev E - Stat Physics, Plasmas Fluids Relat Interdiscip Top (1998) 58:69–73. doi:10.1103/PhysRevE.58.69
10. Hill K. Altruistic cooperation during foraging by the Ache, and the evolved human predisposition to cooperate. Hum Nat (2002) 13:105–28. doi:10.1007/s12110-002-1016-3
11. Hammond RA, Axelrod R. Evolution of contingent altruism when cooperation is expensive. Theor Popul Biol (2006) 69:333–8. doi:10.1016/j.tpb.2005.12.002
12. Hardin G. The tragedy of the commons. Science (1968) 162(80):1243–8. doi:10.1126/science.162.3859.1243
13. Nowak MA, Sigmund K. Evolution of indirect reciprocity by image scoring. Nature (1998) 393:573–7. doi:10.1038/31225
14. Perc M, Szolnoki A. Social diversity and promotion of cooperation in the spatial prisoner’s dilemma game. Phys Rev E Stat Nonlin Soft Matter Phys (2008) 77:011904. doi:10.1103/PhysRevE.77.011904
15. Szolnoki A, Perc M. Emergence of multilevel selection in the prisoner’s dilemma game on coevolving random networks. New J Phys (2009) 11:93033. doi:10.1088/1367-2630/11/9/093033
16. Zhang H, Small M, Yang H, Wang B. Adjusting learning motivation to promote cooperation. Phys A Stat Mech Its Appl (2010) 389:4734–9. doi:10.1016/j.physa.2010.06.023
17. Wang Z, Wang Z, Zhu X, Arenzon JJ. Cooperation and age structure in spatial games. Phys Rev E Stat Nonlin Soft Matter Phys (2012) 85:011149. doi:10.1103/PhysRevE.85.011149
18. Shen C, Chu C, Shi L, Perc M, Wang Z. Aspiration-based coevolution of link weight promotes cooperation in the spatial prisoner's dilemma game. R Soc Open Sci (2018) 5:180199. doi:10.1098/rsos.180199
19. Wang Z, Szolnoki A, Perc M. Evolution of public cooperation on interdependent networks: the impact of biased utility functions. Epl (2012) 97:48001. doi:10.1209/0295-5075/97/48001
20. Liu C, Shi J, Li T, Liu J. Aspiration driven coevolution resolves social dilemmas in networks. Appl Math Comput (2019) 342:247–54. doi:10.1016/j.amc.2018.09.034
21. Shen C, Chu C, Guo H, Shi L, Duan J. Coevolution of vertex weights resolves social dilemma in spatial networks. Sci Rep (2017) 7:15213. doi:10.1038/s41598-017-15603-2
22. Shen C, Chu C, Geng Y, Jin J, Chen F, Shi L. Cooperation enhanced by the coevolution of teaching activity in evolutionary prisoner’s dilemma games with voluntary participation. PLoS One (2018) 13:e0193151. doi:10.1371/journal.pone.0193151
23. Perc M, Wang Z. Heterogeneous aspirations promote cooperation in the prisoner’s dilemma game. PLoS One (2010) 5:e15117. doi:10.1371/journal.pone.0015117
24. Wang Z, Perc M. Aspiring to the fittest and promotion of cooperation in the prisoner’s dilemma game. Phys Rev E Stat Nonlin Soft Matter Phys (2010) 82:021115. doi:10.1103/PhysRevE.82.021115
25. Barabási AL, Albert R. Emergence of scaling in random networks. Science (1999) 80-286:509–12. doi:10.1126/science.286.5439.509
26. Chu C, Mu C, Liu J, Liu C, Boccaletti S, Shi L, et al. Aspiration-based coevolution of node weights promotes cooperation in the spatial prisoner’s dilemma game. New J Phys (2019) 21:63024. doi:10.1088/1367-2630/ab0999
27. Watts DJ, Strogatz SH. Collective dynamics of “small-world” networks. Nature (1998) 393:440–2. doi:10.1038/30918
28. Wang Z, Wang L, Szolnoki A, Perc M. Evolutionary games on multilayer networks: a colloquium. Eur Phys J B (2015) 88:124. doi:10.1140/epjb/e2015-60270-7
29. Nowak MA. Five rules for the evolution of cooperation. Science (2006) 314(80):1560–3. doi:10.1126/science.1133755
30. Shi J, Hu D, Tao R, Peng Y, Li Y, Liu J. Interaction between populations promotes cooperation in voluntary prisoner’s dilemma. Appl Math Comput (2021) 392:125728. doi:10.1016/j.amc.2020.125728
31. Liu J, Meng H, Wang W, Li T, Yu Y. Synergy punishment promotes cooperation in spatial public good game. Chaos, Solitons & Fractals (2018) 109:214–8. doi:10.1016/j.chaos.2018.01.019
32. Wang Z, Wang L, Perc M. Degree mixing in multilayer networks impedes the evolution of cooperation. Phys Rev E Stat Nonlin Soft Matter Phys (2014) 89:052813. doi:10.1103/physreve.89.052813
33. Wang Z, Wang L, Yin ZY, Xia CY. Inferring reputation promotes the evolution of cooperation in spatial social dilemma games. PLoS One (2012) 7:e40218. doi:10.1371/journal.pone.0040218
34. Jin Q, Wang L, Xia CY, Wang Z. Spontaneous symmetry breaking in interdependent networked game. Sci Rep (2014) 4:4095. doi:10.1038/srep04095
35. Wang Z, Andrews MA, Wu ZX, Wang L, Bauch CT. Coupled disease-behavior dynamics on complex networks: a review. Phys Life Rev (2015) 15:1–29. doi:10.1016/j.plrev.2015.07.006
36. Li X, Sun S, Xia C. Reputation-based adaptive adjustment of link weight among individuals promotes the cooperation in spatial social dilemmas. Appl Math Comput (2019) 361:810–20. doi:10.1016/j.amc.2019.06.038
37. Zhang Y, Wang J, Ding C, Xia C. Impact of individual difference and investment heterogeneity on the collective cooperation in the spatial public goods game. Knowl-based Syst (2017) 136:150–8. doi:10.1016/j.knosys.2017.09.011
38. Wang J, Lu W, Liu L, Li L, Xia C. Utility evaluation based on one-to-N mapping in the prisoner's dilemma game for interdependent networks. PLoS One (2016) 11:e0167083. doi:10.1371/journal.pone.0167083
39. Huang K, Chen X, Yu Z, Yang C, Gui W. Heterogeneous cooperative belief for social dilemma in multi-agent system. Appl Math Comput (2018) 320:572–9. doi:10.1016/j.amc.2017.10.018
40. Szolnoki A, Perc M. Promoting cooperation in social dilemmas via simple coevolutionary rules. Eur Phys J B (2009) 67:337–44. doi:10.1140/epjb/e2008-00470-8
41. Droz M, Szwabiński J, Szabó G. Motion of influential players can support cooperation in prisoner’s dilemma. Eur Phys J B (2009) 71:579–85. doi:10.1140/epjb/e2009-00160-1
42. Perc M, Szolnoki A, Szabó G. Restricted connections among distinguished players support cooperation. Phys Rev E Stat Nonlin Soft Matter Phys (2008) 78:066101. doi:10.1103/PhysRevE.78.066101
43. Raihani NJ, Bshary R. The evolution of punishment in n-player public goods games: a volunteer's dilemma. Evolution (2011) 65:2725–8. doi:10.1111/j.1558-5646.2011.01383.x
44. Chu C, Liu J, Shen C, Jin J, Tang Y, Shi L. Coevolution of game strategy and link weight promotes cooperation in structured population. Chaos, Solitons and Fractals (2017) 104:28–32. doi:10.1016/j.chaos.2017.07.023
45. Chu C, Hu X, Shen C, Li T, Boccaletti S, Shi L, et al. Self-organized interdependence among populations promotes cooperation by means of coevolution. Chaos (2019) 29:13139. doi:10.1063/1.5059360
46. Dai X, Zhu P, Guo Y, Wang Z. Coevolution of vaccination opinions and awareness affecting the spread of epidemics. IEEE Access (2019) 7:61558–69. doi:10.1109/ACCESS.2019.2902856
47. Huang K, Wang Z, Jusup M. Incorporating latent constraints to enhance inference of network structure. IEEE Trans Netw Sci Eng (2020) 7:466–75. doi:10.1109/TNSE.2018.2870687
48. Huang K, Liu Y, Zhang Y, Yang C, Wang Z. Understanding cooperative behavior of agents with heterogeneous perceptions in dynamic networks. Phys A Stat Mech Its Appl (2018) 509:234–40. doi:10.1016/j.physa.2018.06.043
49. Wang Z, Jusup M, Shi L, Lee JH, Iwasa Y, Boccaletti S. Exploiting a cognitive bias promotes cooperation in social dilemma experiments. Nat Commun (2018) 9:2954. doi:10.1038/s41467-018-05259-5
50. Wang Z, Jusup M, Wang RW, Shi L, Iwasa Y, Moreno Y, et al. Onymity promotes cooperation in social dilemma experiments. Sci Adv (2017) 3:e1601444. doi:10.1126/sciadv.1601444
51. Chu C, Liu J, Shen C, Jin J, Shi L. Win-stay-lose-learn promotes cooperation in the prisoner's dilemma game with voluntary participation. PLoS One (2017) 12:e0171680. doi:10.1371/journal.pone.0171680
52. Liu J, Meng H, Wang W, Xie Z, Yu Q. Evolution of cooperation on independent networks: the influence of asymmetric information sharing updating mechanism. Appl Math Comput (2019) 340:234–41. doi:10.1016/j.amc.2018.07.004
53. Shen C, Lu J, Shi L. Does coevolution setup promote cooperation in spatial prisoner’s dilemma game?. Appl Math Comput (2016) 290:201–7. doi:10.1016/j.amc.2016.05.044
54. Tanimoto J, Sagara H. Relationship between dilemma occurrence and the existence of a weakly dominant strategy in a two-player symmetric game. BioSystems (2007) 90:105–14. doi:10.1016/j.biosystems.2006.07.005
55. Wang Z, Kokubo S, Jusup M, Tanimoto J. Dilemma strength as a framework for advancing evolutionary game theory: reply to comments on “Universal scaling for the dilemma strength in evolutionary games”. Phys Life Rev (2015) 14:56–8. doi:10.1016/j.plrev.2015.07.012
56. Ito H, Tanimot J. Scaling the phase-planes of social dilemma strengths shows game-class changes in the five rules governing the evolution of cooperation. R Soc Open Sci (2018) 5:181085. doi:10.1098/rsos.181085
58. Tanimoto J. Fundamentals of evolutionary game theory and its applications. Singapore: Springer (2015) Available from: http://link.springer.com/10.1007/978-4-431-54962-8 (Accessed October 7, 2020).
59. Alam M, Nagashima K, Tanimoto J. Various error settings bring different noise-driven effects on network reciprocity in spatial prisoner’s dilemma. Chaos, Solitons and Fractals (2018) 114:338–46. doi:10.1016/j.chaos.2018.07.014
60. Alam M, Kuga K, Tanimoto J. Three-strategy and four-strategy model of vaccination game introducing an intermediate protecting measure. Appl Math Comput (2019) 346:408–22. doi:10.1016/j.amc.2018.10.015
61. Alam M, Tanaka M, Tanimoto J. A game theoretic approach to discuss the positive secondary effect of vaccination scheme in an infinite and well-mixed population. Chaos, Solitons and Fractals (2019) 125:201–13. doi:10.1016/j.chaos.2019.05.031
Keywords: prisoner’s dilemma game, game theory, cooperation, neutral reward, complex network
Citation: Tang Y, Jing M and Yu Y (2021) Conditional Neutral Reward Promotes Cooperation in the Spatial Prisoner’s Dilemma Game. Front. Phys. 9:639252. doi: 10.3389/fphy.2021.639252
Received: 08 December 2020; Accepted: 01 February 2021;
Published: 23 February 2021.
Edited by:
Hui-Jia Li, Beijing University of Posts and Telecommunications (BUPT), ChinaReviewed by:
Lin Wang, University of Cambridge, United KingdomCopyright © 2021 Tang, Jing and Yu. This is an open-access article distributed under the terms of the Creative Commons Attribution License (CC BY). The use, distribution or reproduction in other forums is permitted, provided the original author(s) and the copyright owner(s) are credited and that the original publication in this journal is cited, in accordance with accepted academic practice. No use, distribution or reproduction is permitted which does not comply with these terms.
*Correspondence: Ming Jing, cHJvb2ZsZUAxNjMuY29t; Yong Yu, eXV5MTIxOUAxNjMuY29t
Disclaimer: All claims expressed in this article are solely those of the authors and do not necessarily represent those of their affiliated organizations, or those of the publisher, the editors and the reviewers. Any product that may be evaluated in this article or claim that may be made by its manufacturer is not guaranteed or endorsed by the publisher.
Research integrity at Frontiers
Learn more about the work of our research integrity team to safeguard the quality of each article we publish.