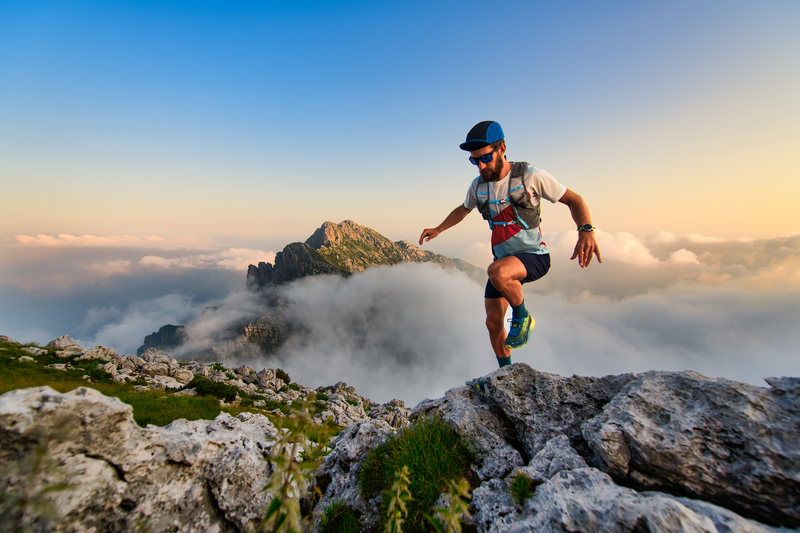
95% of researchers rate our articles as excellent or good
Learn more about the work of our research integrity team to safeguard the quality of each article we publish.
Find out more
ORIGINAL RESEARCH article
Front. Phys. , 15 April 2021
Sec. Soft Matter Physics
Volume 9 - 2021 | https://doi.org/10.3389/fphy.2021.637138
This article is part of the Research Topic 50 years of Statistical Physics in Mexico: Development, State of the Art and Perspectives View all 17 articles
Reversible aggregation of purely short-ranged attractive colloidal particles leads to the formation of clusters with a fractal dimension that only depends on the second virial coefficient. The addition of a long-ranged repulsion to the potential modifies the way in which the particles aggregate into clusters and form intermediate range order structures, and have a strong influence on the dynamical and rheological properties of colloidal dispersions. The understanding of the effect of a long-ranged repulsive potential on the aggregation mechanisms is scientifically and technologically important for a large variety of physical, chemical and biological systems, including concentrated protein solutions. In this work, the equilibrium cluster morphology of particles interacting through a short-ranged attraction plus a long-ranged repulsion is extensively studied by means of Monte Carlo computer simulations. Our findings point out that the addition of the repulsion affects the resulting cluster morphology and allows one to have a full control on the compactness or fractal dimension of the aggregates at a given thermodynamic condition. This allows us to manipulate the reversible aggregation process and, therefore, to finely tune the resulting building blocks of materials at large length scales.
Many body systems composed of particles interacting via a short-range attraction and a long-range repulsion (SALR) have attracted attention in the past decade due to their rich phase behavior not observed for systems interacting with purely attractive potentials. One of these is the clustered fluid phase resulting from the frustration of the gas-liquid separation. This phase has been observed in experiments, as well as in molecular simulations, see, e.g., references [1–14]. Understanding the clustering of particles is necessary to explain changes in the dynamics and rheology of colloidal systems with competing interactions, see [15, 16].
The competition between attraction and repulsion over different length scales makes possible to have different phase diagrams depending on the parameters that control both the range and strength of each potential feature. [17] presented an empirical classification for systems with competing interactions based on the range of the repulsion and attraction. When the attraction range is smaller than
In this work, we focus on the cluster morphology of type I systems, since the features of the interaction are more similar to the estimated potential for colloidal systems and protein solutions, see for example references [2, 4, 5, 20–23]. Besides, the structural properties of clusters have not been studied systematically yet.
The phase diagram of particles with only short-ranged attractions has been studied intensively, see e.g. [24–30]; and it is widely accepted that their thermodynamic behavior is determined by the extended law of corresponding states, [31]. In a similar spirit [10], proposed a generalized phase diagram for particles with competing interactions after studying type I and II systems through Monte Carlo computer simulations. According to the generalized phase diagram, particles at low and moderate concentrations form a dispersed fluid state at high temperatures, and an equilibrium clustered fluid state at temperatures below
The manuscript is organized as follows. In Section 2, the interaction potential, as well as the computer simulation protocol are described. In Section 3, the structural signature of competing interaction systems is discussed and intimately related to the properties of clusters, which are studied in Section 5. Section 4 presents the phase diagram, where the region relevant for this work is explicitly pointed out. Finally, in section 6 the main conclusions of this work and some perspectives are presented.
We have performed extensive Monte Carlo (MC) computer simulations in the canonical ensemble
the range and strength of the attraction are well determined by λ and ϵ, respectively, while the maximum repulsion strength (in units of ϵ) is given by A and the corresponding range is approximately
MC computer simulations were carried out at the same packing fraction,
To characterize the microstructure, we calculate the so-called structure factor,
The hallmark of many SALR systems, is found in the structure factor, [10]. The
[10] defined the clustered fluid state as that thermodynamic state of a system at which the cluster size distribution,
In Figures 1A,B the structure factor for the systems with the lower repulsion, obtained through MC simulation (symbols) and by solving the Ornstein-Zernike equation with the Percus-Yevick closure (solid lines), is shown. In (A) it is also included the
FIGURE 1. Structure factor for competing interaction systems whose repulsion strength is (A)
Figures 1C,D show the cluster size distribution for systems with
The relationship between the low-q peak and the shoulder in
FIGURE 2. Structure factor for competing interaction systems whose repulsion strength is (A)
In Figure 3A the phase diagram for the SW fluid with an interaction range
FIGURE 3. (A) phase diagram for the SW fluid with
The information presented in the previous section allows us to establish the boundary between a disperse fluid, the clustered one and the phase separation. Nonetheless, the exact location of this boundary depends on the specific value of the repulsive potential, see Figure 3B. Competing interaction systems at low temperatures resemble the gas-liquid phase separation for
Figure 3C shows some snapshots of the simulated system at
The cluster morphology of competing interactions systems has been studied in experiments and simulations [13]. found that equilibrium clusters are more compact than those out of equilibrium. Experiments made by [7] indicate that cluster morphology is affected by the attractive contribution. Also [37], found that equilibrium clusters tend to be elongated structures. In a previous publication, [38]; we studied the morphology of clusters made of purely attractive particles at the same ϕ-value and for temperatures above the binodal. There, we found that the morphology of clusters with more than 10 particles is determined by the strength of the attraction via the second virial coefficient, while the morphology of small clusters is insensitive to the state of the system.
In this work, we have performed a systematic study of the cluster morphology. The radius of gyration is fitted using the equation:
FIGURE 4. Radius of gyration for competing interaction systems at
From Figure 1 it was noted the presence of small shoulder at
Figure 4C shows snapshots for systems with
In this work, we investigated the cluster formation for systems with competing interactions. The potential parameters were chosen to represent the interactions between proteins in an aqueous solution at low salinity. The analysis of the structure factor, as well as the cluster morphology, reveals that due to the addition of the long-ranged repulsion, the gas-liquid phase separation is shifted to lower temperatures compared with that of systems with pure attractive potential. For the case
The analysis of the cluster size percolation for systems in the clustered fluid state revealed that there is cluster formation with a preferential size in clustered fluid states. This optimal size depends also on the strength of the repulsive potential. Thus for the type I SALR systems, it is possible to produce clustered states with specific cluster size by controlling the potential parameters.
At the lowest temperatures for the stronger repulsion, particles are locally organized in very compact structures. Small clusters with less than 12 particles tend to adopt the minimum energy configuration, while intermediate size clusters have elongated configurations when these compact clusters join together with certain specific configurations. This cluster formation is different from that observed in systems made of purely attractive particles, [38]. For the SALR systems studied here, the scales at which the intermediate size clusters are observed can be related with the value at which the IRO peak appears in the structure factor. An interesting future work is the analysis of the dynamics of the clustering process at different concentrations in both the cluster fluid states and the cluster percolated states.
The original contributions presented in the study are included in the article/Supplementary Material, further inquiries can be directed to the corresponding authors.
All authors contributed to the design and implementation of the research, to the analysis of the results and to the writing of the manuscript.
NV-P acknowledges support by CONACyT through the scholarship Retenciones 2019–1. RC-P. also acknowledges the financial support provided by the Consejo Nacional de Ciencia y Tecnología (CONACYT, Mexico) through Grants Nos. 237,425 and 287,067. YL acknowledge the support by the Center for High Resolution Neutron Scattering (CHRNS), a partnership between the National Institute of Standards and Technology and National Science Foundation under Agreement No. DMR-1508249.
The authors declare that the research was conducted in the absence of any commercial or financial relationships that could be construed as a potential conflict of interest.
The Supplementary Material for this article can be found online at: https://www.frontiersin.org/articles/10.3389/fphy.2021.637138/full#supplementary-material.
1. Baglioni P, Fratini E, Lonetti B, Chen SH. Structural arrest in concentrated cytochrome c solutions: the effect of pH and salts. J Phys Condens Matter (2004) 16:S5003. doi:10.1088/0953-8984/16/42/016
2. Stradner A, Sedgwick H, Cardinaux F, Poon WCK, Egelhaaf SU, Schurtenberger P. Equilibrium cluster formation in concentrated protein solutions and colloids. Nature (2004) 432:492–495. doi:10.1038/nature03109
3. Sciortino F, Mossa S, Zaccarelli E, Tartaglia P. Equilibrium cluster phases and low-density arrested disordered states: the role of short-range attraction and long-range repulsion. Phys Rev Lett (2004) 93(5):055701. doi:10.1103/PhysRevLett.93.055701
4. Campbell AI, Anderson VJ, van Duijneveldt JS, Bartlett P. Dynamical arrest in attractive colloids: the effect of long-range repulsion. Phys Rev Lett (2005) 94(20):208301. doi:10.1103/PhysRevLett.94.208301
5. Cardinaux F, Stradner A, Schurtenberger P, Sciortino F, Zaccarelli E. Modeling equilibrium clusters in lysozyme solutions. Europhys Lett (2007) 77:48004. doi:10.1209/0295-5075/77/48004
6. Toledano JCF, Sciortino F, Zaccarelli E. Colloidal systems with competing interactions: from an arrested repulsive cluster phase to a gel. Soft Matter (2009) 5:2390–8. doi:10.1039/B818169A
7. Zhang TH, Klok J, Hans Tromp R, Groenewold J, Kegel WK. Non-equilibrium cluster states in colloids with competing interactions. Soft Matter (2012) 8:667–72. doi:10.1039/C1SM06570J
8. Valadez-Pérez NE, Castañeda Priego R, Liu Y. Percolation in colloidal systems with competing interactions: the role of long-range repulsion. RSC Adv (2013) 3:25110–9. doi:10.1039/C3RA44588G
9. Godfrin PD, Castañeda Priego R, Liu Y, Wagner NJ. Intermediate range order and structure in colloidal dispersions with competing interactions. J Chem Phys (2013) 139:154904. doi:10.1063/1.4824487
10. Godfrin PD, Valadez-Pérez NE, Castañeda Priego R, Wagner NJ, Liu Y. Generalized phase behavior of cluster formation in colloidal dispersions with competing interactions. Soft Matter (2014) 10:5061–71. doi:10.1039/C3SM53220H
11. Mani E, Lechner W, Kegel WK, Bolhuis PG. Equilibrium and non-equilibrium cluster phases in colloids with competing interactions. Soft Matter (2014) 10:4479–86. doi:10.1039/C3SM53058B
12. Sweatman MB, Fartaria R, Lue L. Cluster formation in fluids with competing short-range and long-range interactions. J Chem Phys (2014) 140:124508. doi:10.1063/1.4869109
13. Jadrich RB, Bollinger JA, Johnston KP, Truskett TM. Origin and detection of microstructural clustering in fluids with spatial-range competitive interactions. Phys Rev E (2015) 91:042312. doi:10.1103/PhysRevE.91.042312
14. Santos AP, Pękalski J, Panagiotopoulos AZ. Thermodynamic signatures and cluster properties of self-assembly in systems with competing interactions. Soft Matter (2017) 13:8055–63. doi:10.1039/C7SM01721A
15. Riest J, Nägele G, Liu Y, Wagner NJ, Godfrin PD. Short-time dynamics of lysozyme solutions with competing short-range attraction and long-range repulsion: experiment and theory. J Chem Phys (2018) 148:065101. doi:10.1063/1.5016517
16. Ruiz-Franco J, Camerin F, Gnan N, Zaccarelli E. Tuning the rheological behavior of colloidal gels through competing interactions. Phys Rev Mater (2020) 4:045601. doi:10.1103/PhysRevMaterials.4.045601
17. Liu Y, Xi Y. Colloidal systems with a short-range attraction and long-range repulsion: phase diagrams, structures, and dynamics. Curr Opin Colloid Interf Sci (2019) 39:123–36. doi:10.1016/j.cocis.2019.01.016
18. Archer AJ, Wilding NB. Phase behavior of a fluid with competing attractive and repulsive interactions. Phys Rev E (2007) 76:031501. doi:10.1103/PhysRevE.76.031501
19. Zhuang Y, Charbonneau P. Equilibrium phase behavior of the square-well linear microphase-forming model. J Phys Chem B (2016) 120:6178–88. doi:10.1021/acs.jpcb.6b02167
20. Shukla A, Mylonas E, Di Cola E, Finet S, Timmins P, Narayanan T. Absence of equilibrium cluster phase in concentrated lysozyme solutions. Pnas (2008) 105:5075–80. doi:10.1073/pnas.0711928105
21. Godfrin PD, Hudson SD, Hong K, Porcar L, Falus P, Wagner NJ. Short-time glassy dynamics in viscous protein solutions with competing interactions. Phys Rev Lett (2015) 115:228302. doi:10.1103/PhysRevLett.115.228302
22. Liu Y, Porcar L, Chen J, Chen WR, Falus P, Faraone A. Lysozyme protein solution with an intermediate range order structure. J Phys Chem B 115 (2011) 7238–47. doi:10.1021/jp109333c
23. Baumketner A, Cai W. Clusters of lysozyme in aqueous solutions. Phys Rev E (2018) 98:032419. doi:10.1103/PhysRevE.98.032419
24. Dijkstra M. Phase behavior of hard spheres with a short-range yukawa attraction. Phys Rev E (2002) 66:021402. doi:10.1103/PhysRevE.66.021402
25. Schöll-Paschinger E, Benavides AL, Castañeda-Priego R. Vapor-liquid equilibrium and critical behavior of the square-well fluid of variable range: a theoretical study. J Chem Phys (2005) 123:234513. doi:10.1063/1.2137713
26. Largo J, Solana JR, Yuste SB, Santos A. Pair correlation function of short-ranged square-well fluids. J Chem Phys (2005) 122:084510. doi:10.1063/1.1855312
27. Pagan DL, Gunton JD. Phase behavior of short-range square-well model. J Chem Phys (2005) 122:184515. doi:10.1063/1.1890925
28. Espíndola-Heredia R, del Río F, Malijevsky A. Optimized equation of the state of the square-well fluid of variable range based on a fourth-order free-energy expansion. J Chem Phys (2009) 130:024509. doi:10.1063/1.3054361
29. Valadez-Pérez NE, Benavides AL, Schöll-Paschinger E, Castañeda Priego R. Phase behavior of colloids and proteins in aqueous suspensions: theory and computer simulations. J Chem Phys (2012) 137:084905. doi:10.1063/1.4747193
30. Orea P, Romero-Martínez A, Basurto E, Vargas CA, Odriozola G. Corresponding states law for a generalized Lennard-Jones potential. J Chem Phys (2015) 143:024504. doi:10.1063/1.4926464
31. Noro MG, Frenkel D. Extended corresponding-states behavior for particles with variable range attractions. J Chem Phys (2000) 113:2941–4. doi:10.1063/1.1288684
32. Heyes DM. Monte Carlo simulations of continuum percolation of 3d well fluids. J Phys Condens Matter (1990) 2:2241–9. doi:10.1088/0953-8984/2/9/013
33. Wei J, Xu L, Song F. Range effect on percolation threshold and structural properties for short-range attractive spheres. J Chem Phys (2015) 142:034504. doi:10.1063/1.4906084
34. Hansen JP, McDonald IR. Static properties of liquids: thermodynamics and structure. 4th ed. Oxford: Academic Press (2013). 61–104 p.
35. Stauffer D. Scaling theory of percolation clusters. Phys Rep (1979) 54:1–74. doi:10.1016/0370-1573(79)90060-7
36. Benavides AL, Gil-Villegas A. The thermodynamics of molecules with discrete potentials. Mol Phys (1999) 97:1225–32. doi:10.1080/00268979909482924
37. Kowalczyk P, Ciach A, Gauden P, Terzyk A. Equilibrium clusters in concentrated lysozyme protein solutions. J Colloid Interf Sci (2011) 363:579–84. doi:10.1016/j.jcis.2011.07.043
38. Valadez-Pérez NE, Liu Y, Castañeda Priego R. Reversible aggregation and colloidal cluster morphology: the importance of the extended law of corresponding states. Phys Rev Lett (2018) 120:248004. doi:10.1103/PhysRevLett.120.248004
39. Sciortino F, Tartaglia P, Zaccarelli E. One-dimensional cluster growth and branching gels in colloidal systems with short-range depletion attraction and screened electrostatic repulsion. J Phys Chem B (2005) 109:21942–53. doi:10.1021/jp052683g
Keywords: competing interactions, reversible aggregation, clustering, cluster morphology, fractal dimension
Citation: Valadez-Pérez NE, Liu Y and Castañeda-Priego R (2021) Cluster Morphology of Colloidal Systems With Competing Interactions. Front. Phys. 9:637138. doi: 10.3389/fphy.2021.637138
Received: 02 December 2020; Accepted: 03 February 2021;
Published: 15 April 2021.
Edited by:
Giancarlo Ruocco, Italian Institute of Technology (IIT), ItalyReviewed by:
Emanuela Zaccarelli, National Research Council (CNR), ItalyCopyright © 2021 Valadez-Pérez, Liu and Castañeda-Priego. This is an open-access article distributed under the terms of the Creative Commons Attribution License (CC BY). The use, distribution or reproduction in other forums is permitted, provided the original author(s) and the copyright owner(s) are credited and that the original publication in this journal is cited, in accordance with accepted academic practice. No use, distribution or reproduction is permitted which does not comply with these terms.
*Correspondence: Néstor E. Valadez-Pérez, bmVzdG9yLnZhbGFkZXpAdW5hY2gubXg=, ZW5leHR2QGdtYWlsLmNvbQ==
Disclaimer: All claims expressed in this article are solely those of the authors and do not necessarily represent those of their affiliated organizations, or those of the publisher, the editors and the reviewers. Any product that may be evaluated in this article or claim that may be made by its manufacturer is not guaranteed or endorsed by the publisher.
Research integrity at Frontiers
Learn more about the work of our research integrity team to safeguard the quality of each article we publish.