- 1Laboratorio de Genética y Biología Molecular (L.G.B.M), Facultad Experimental de Ciencias (F.E.C), Departamento de Biología, Universidad Del Zulia (L.U.Z), Maracaibo, Venezuela
- 2Laboratorio de Caracterización Molecular y Biomolecular, Centro de Investigación y Tecnología de Materiales (CITeMA), Instituto Venezolano de Investigaciones Científicas (IVIC), Maracaibo, Venezuela
- 3Departamento de Física, Escuela Politécnica Nacional, Quito, Ecuador
- 4Facultad de Ciencias Naturales y Matemáticas, Departamento de Química y Ciencias Ambientales, Laboratorio de Análisis Químico Instrumental (LAQUINS), Escuela Superior Politécnica Del Litoral, Guayaquil, Ecuador
SARS-CoV-2 has caused millions of infections and more than 600,000 deaths worldwide. Despite the large number of studies to date, there is no specifically effective treatment available for SARS-CoV-2. However, it has been proposed to target reused drugs with potential antiviral activity to the interface between the angiotensin-converting enzymes 2 (ACE2) and the receptor binding domain (RBD) of SARS-CoV-2 to avoid cell recognition. Some non-steroidal anti-inflammatory drugs (NSAIDs) have been reported to have some type of activity against a wide variety of viruses including SARS-CoV-2. Therefore, we carried out an exhaustive computational biophysical study of various NSAIDs targeting the RBD-ACE2 complex using multiple comparative analysis of docking and molecular dynamics. Only the Ibuprofen (Propionic acid derivative), Aspirin (Salicylate), and the Acetaminophen (p-aminophenol derivative) had a thermodynamically favorable docking with the interface of the RBD-ACE2 complex under the conditions of this study. Although, Ibuprofen was the NSAIDs with the most thermodynamically favorable docking in the shortest simulation time, and was the major inducer of structural changes, conformational changes, and overall changes in the complex throughout the simulation, including disturbances in composition and distribution of cavities at the interface. Results that point to Ibuprofen as an NSAID that, under the conditions outlined in this investigation, may have the highest probability of generating a disturbance in the stability of the RBD-ACE2 complex. This statement, although it could contribute information for the empirical treatment and prevention of COVID-19, represents only a theoretical orientation and approach, and requires its experimental demonstration because our predictions cannot secure a pharmacologically and clinically relevant interaction. However, these results are relevant due that suggest a possible mechanism of action of Ibuprofen against COVID-19 in addition to its anti-inflammatory properties, of which there are no reports in the literature.
Introduction
The SARS-CoV-2 pandemic has required rapid drug searches for the management of COVID-19 disease. COVID-19 is a pathology characterized by an acute viral infection in humans with an average incubation period of 3 days [1] similar to SARS-CoV-1 [2]. The most common characteristics reported during COVID-19 are similar to those described for other coronaviruses and are represented by fever (88%), cough (68%), fatigue (38%), while vomiting (5%) and diarrhea (4%) are less frequent [1, 3]. The development of acute respiratory distress syndrome (ARDS) has been reported in most patients [4–7]. Additionally, patients are prone to a variety of ARDS-related complications, including acute heart injury and secondary infection [8]. Given this, it has been pointed out that the use of anti-inflammatory drugs concomitantly with the standard treatments recommended by the World Health organization (WHO) could be useful against COVID-19 [9] including non-steroidal anti-inflammatory drugs (NSAIDs) [10–14]. NSAIDs have been little considered because although they have a high degree of safety and have been used in adverse respiratory conditions [15] are equally associated with various adverse effects [16–18].
In fact, the use of NSAIDs such as Ibuprofen is highly controversial because it has been reported that in animal models it can mediate the overexpression of the angiotensin-converting enzyme 2 (ACE2) essential for viral recognition and it has been speculated that this could increase the risk of COVID-19 [19–23]. However, in various investigations in patients with COVID-19, the use of Ibuprofen has not been associated with worse clinical outcomes, compared to other NSAIDs such as Acetaminophen [24–26]. On the contrary, it has been described that the chronic use of this type of NSAIDs could even protect against the occurrence and severity of COVID-19 [26, 27]. Therefore, deepening theoretical and experimental in this direction is necessary and is especially justified, because it has been reported that NSAIDs can have a positive effect against ARDS [28] and because the inflammatory process described during SARS-CoV-2 infection has greatly limited the use of other potential drugs [29].
The WHO has indicated that there is no evidence to confirm an aggravation of COVID-19 infection with the administration of NSAIDs [30, 31]. In fact, it has been reported that some NSAIDs could have some type of activity against viruses such as VZV [32], HCMV [32], HSV-1 [33], influenza virus A⁄H1N1 subtype [34, 35], VSV [36, 37], EBOLA [38, 39], HIV [40], JEV [41], CHIKV [42], SARS-CoV-1 [43], and recently against SARS-CoV-2 [35, 44, 45]. Additionally, many studies have been carried out that propose various targets for blocking virus recognition, importation and replication processes through the use of reused drugs, immunotherapies, interference strategies and various inhibitory compounds [46]. But despite the large number of experimental, biophysical, and computational studies that have explored all of these alternatives, to date, there is no specifically confirmed effective treatment available for SARS-CoV-2 [46, 47]. However, important advances have been made in the study of potential compounds targeting the interface between the ACE2 and the receptor binding domain (RBD) of SARS-CoV-2 to prevent cellular recognition of virus [48–50]. Why the RBD domain is known to be part of the SARS-CoV-2 spike receptor with higher affinity for the ACE2 enzyme, a key interaction for infection to establish [48].
Therefore, the theoretical study of candidate compounds for docking and perturbation of the RBD-ACE2 interface could theoretically contribute to this approach in the research and development of COVID-19 treatment alternatives. In this sense, and based on what has been previously described, we propose to perform a computational biophysical characterization of interaction of various NSAID-type compounds at the RBD-ACE2 complex biointerface and the perturbation energy-conformational induce by these drugs on this complex in order to obtain information about possible inhibitory mechanistic routes of these drugs against the SARS-CoV-2 virus, especially since these drugs are not banned to date by the WHO for the symptomatic treatment of COVID-19.
Materials and Methods
Search for Structures in Databases
In this study, the crystal structure of ACE2 and RBD of SARS-CoV-2 (PDB: 6M0J) were considered and obtained from the RCSB protein database (https://www.rcsb.org/). The simulations were carried out using as a target, the entire region of the RBD domain which is bound to the ACE2 receptor by means of 15 residues. Specifically, the amino acids that interact at the ACE2 and RBD interface are in total 15 residues of ACE2 that interact with RBD: these are residues 24 (Q), 27 (T), 30 (D), 31 (K), 34 (H), 35 (E), 37 (E), 38 (D), 41 (Y) and 42 (Q) that are in α1, a residue (residue 82 M) that comes from α2 and residues 353 (K), 354 (G), 355 (D) and 357 (R) that come from the linker between β3 and β4, as reported [49, 50]. At least one member from each of the commonly marketed NSAID types was chosen (see structure of these drugs in Supplementary Figure S1). The 2D structures of the compounds Aspirin_CID_2244 (Salicylates), Celecoxib_CID_2662 (Selective COX-2 inhibitors “coxibs”), Benzydamine_CID_12555 (Indazoles), Metamizole_CID_3111 (Pyrazolone derivate), Diclofenac_CID_3033 (Acetic acid derivatives), Ibuprofen_CID_3672 (Propionic acid derivatives), Indomethacin_CID_3715 (Acetic acid derivatives), Ketoprofen_CID_3825 (Propionic acid derivatives), Meloxicam_CID_54677470 (Enolic acid “oxicam” cSulfonanilides) and Acetaminophen_CID_1983 (p-aminophenol derivative), were obtained from PubChem (https://pubchem.ncbi.nlm.nih.gov/) in SDF format, and the SMILES online converter was used (https://cactus.nci.nih.gov/translate/) to get a PDB format. Acetaminophen is generally not considered an NSAID because it has only minor anti-inflammatory activity but was included as recommended by WHO as part of standard treatment [30, 51]. The Molinspiration server (https://www.molinspiration.com/) was used for the bioactivity calculations (http://www.molinspiration.com/cgi-bin/properties) and tools the Molecular Modeling Group of the Swiss Institute of Bioinformatics [52, 533].
Molecular Docking
To simulate ligand-protein binding the complexes were constructed in DockThor program using the flexibility algorithm, blind docking and calculating the DockT scoring function. To increase accuracy 30 runs were made with 106 evaluations per run. As is usually done, all the water molecules were removed and the PDB files were separated into two different files, one containing the protein and the other containing the ligand structure [54]. The DockThor program is freely available as a Web server (https://dockthor.lncc.br/v2/), which provides to the user the main steps for protein and ligand preparation with PdbThorBox and MMFFLigand and the analyses of the results using DTStatistics. The Web server utilizes the computational facilities of the Brazilian high-performance platform (SINAPAD, https://www.lncc.br/sinapad/) and the supercomputer SDumont (https://sdumont.lncc.br/). The most favored position in the biointerface was analyzed with MMV_2019_7.0.0, calculating the MolDock, Rerank and PLANTS scoring functions [55, 56]. Only the three runs with the most favorable berth were considered in the sampling of the probabilistically most feasible and thermodynamically most favorable positions in the biointerface. This criterion was used to discriminate the complexes that would be subjected to further analysis, including molecular dynamics.
Potential Theoretical Inhibition
The AutoDock Vina (ADV) algorithm estimates the binding constant K from the binding free energy
And the inhibition constant for binding of ligand to proteins (Ki) (in units of M) is obtained as,
where, R is the universal gas constant (1.987 cal/K mol), T is the absolute temperature (298.15 K). The ΔG value used was derived from the mean of the ligand protein binding free energy predicted by each scoring function considered in this study. The equation establishes that Ki can be equaled to the dissociation constant (Kd) of the protein - inhibitor complex. Therefore, Ki and Kd they are equivalent definitions, since Kd is used to describe the strength with which an inhibitor dissociates from the protein, which is equivalent to the strength that the inhibitor would have to inhibit the protein. In this sense, the higher the value of Ki, the weaker the binding of the inhibitor to the protein, and therefore, the protein - inhibitor complex dissociates more easily [57–62]. In our research we assume a competitive inhibition, which is the most extreme case (maximum inhibition among several possible inhibition mechanisms), in which the Ki value can be converted to the Kd value and IC50 (total concentration of inhibitor that reduces these activities by 50%) with the ADV algorithm and following the considerations in the case of competitive inhibition with the equation,
Where P50, is the protein concentration at 50% inhibition; Kd, is the affinity constant of the ligand to the RBD-ACE2 interface; [PL50], is the protein concentration by ligand concentration at 50% inhibition; and L50, is the ligand concentration at 50% inhibition. Another very useful parameter is PI50, which is defined as the concentration of protein-inhibitor complex at 50% inhibition and can be estimated using the equation,
Here IC50, total inhibitor concentration that reduces these activities by 50%; I50 is the inhibitor concentration at 50%. As a positive control for the comparative analysis, concentration of the free protein at 0% inhibition was predicted with the equation,
Where L and P is the ligand concentration and the protein concentration at 0% inhibition, respectively. The IC50-to-Ki web tool was used for all these calculations (https://bioinfo-abcc.ncifcrf.gov/IC50_Ki_Converter/index.php). This tool helps to measure the quality of the parameters used in the calculations of enzyme inhibitors and of binding reactions between macromolecules and ligands that depend on the type of mechanism of action of the inhibitor and the concentrations of the interacting molecular species [63–66].
Molecular Dynamics
Simulations were performed for a docking coup with three purposes: 1) study the relative stability of the ligand residing in the binding pocket; 2) sampling the minimum energy conformations to calculate the disturbance of the thermodynamic and structural stability of the complexes; 3) analyze the pockets distribution and structural deformation coefficients in the minimum energy conformations. For a protein-ligand complex, the MD system was first relaxed through a series of minimization procedures. There were three phases for a MD simulation: the relaxation phase, the equilibrium phase, and the sampling phase, as recommended [67–70]. The MD simulation of the crystal structures was carried out in an explicit water system. Specifically, the solvation of the system was carried out in a solvation box of 8.0 Å. With an Ion-mol/water-mol ratio close to 1 g/cc (equivalent to a molar density of Na+/Cl– = 0.003) to simulate an aqueous medium under physiological conditions. Under these conditions the quantity of water was fixed in 23,886 molecules. Our MD system also consisted of one copy of RBD-ACE2 and one copy of the coupling ligand. An Amber99SB-ILDN force field was applied to the complex, with TIP3P water model. The whole system was neutralized. All model water molecules were treated as rigid bodies, thus allowing a simulation time step of 2 fs. Periodic boundary conditions were applied, and Berendsen’s algorithm for temperature and pressure coupling was adopted. After a first steepest descent to 5,000 steps and conjugated gradient to 5,000 steps energy minimizations with positional restraints on the solute, an initial 100 ps simulation was carried out with the positions of the solute atoms restrained by a force constant of 10 kcal/(mol Å2) to let the water diffuse around the molecule and for equilibration. The method PME was used to calculate the electrostatic contribution to nonbonded interactions with a cutoff of 14.0 Å and a time step of 1 fs. The cutoff distance of the van der Waals interaction was 14.0 Å. After this equilibration run, the NVT production run (100 ns) at 300 K was performed with the cell size remaining the same. The SHAKE algorithm was applied to the system, and the time step was set to 2 fs. Five Snapshot structures were obtained at every 25 ns as the target structures extracted from a trajectory of 100 ns. All MD simulations and the additional settings were performed by using cosgene/myPresto [68–70]. Flexibility and deviation from the initial structure of molecular dynamics was estimated using root mean square deviation (RMSD) from sampling the minimum energy conformations by using cosgene/myPresto. The pair of residues that came into contact with the ligands of interest was considered to determine the folding/unfolding compared to the native structure. The residues were chosen arbitrarily if they are at a distance of ≤6 Å from the ligands. For RMSD calculations, the equation,
Where δi is the distance between atom i and either a reference structure or the mean position of the n equivalent atoms. Additionally, it was compared with the ANM model (Anisotropic Network Model Web Server 2.1) (http://anm.csb.pitt.edu/) to analyze the vibrational movements of the molecular systems built using the Elastic Network (EN) methodology. The network includes all interactions within a cutoff distance, which is the only default parameter in the model. Information about the orientation of each interaction with respect to the global coordinate system is considered within the constant Force matrix and allows the prediction of anisotropic movements. The model was applied to explore the impact of docking on the MD of the protein complex. For protein complexes the default nodes suggested by the server (alpha carbon (Cα) for amino acids) were used. Ligands were explicitly included in the ANM analysis. These were present in the PDB coordinate file, so that their atoms are analyzed and automatically enumerated. Specifically, the structural fluctuation of the scalar type and the root mean square fluctuation (RMSF) were calculated [71]. The ProSA (Protein Structure Analysis) program was also used because it is an established tool for refining and validating experimental protein structures and for predicting and modeling structures. In order to obtain the visualization of scores (Z-scores) and energy graphs that highlight potential variations in the protein structure. In particular, the quality scores of a protein are displayed in the context of a database of known protein structures. To validate the structural disturbance of the protein of interest compared to native models obtained from X-ray analysis, NMR spectroscopy, and theoretical calculations. ProSA-web is accessible at https://prosa.services.came.sbg.ac.at [72, 73].
Analysis of Changes Induced in the Distribution of Pockets of Protein Complex by Binding of Ligand
The PockDrug-Server (http://pockdrug.rpbs.univ-paris-diderot.fr/cgi-bin/index.py?page=Home) site was used to predict the pharmacological characteristics of the pockets, as well as the variations of the pockets after the conformational changes and structural disturbances that the protein complex docking to the ligand may undergo. PockDrug-Server provides consistent and reproducible results using different pocket estimation methods. For this, the Fpocket method was applied, which performs a predicted pocket estimate based on a preliminary detection of all the cavities that can, for example, bind a ligand of sufficient size, but without information on the proximity of the ligand, based on the decomposition of a protein structure in the Voronoi polyhedra. It is robust with respect to pocket limits and estimation uncertainties, therefore it is efficient in using pockets that are difficult to estimate [74]. The AlloSite server (http://mdl.shsmu.edu.cn/AST/Allosite/index.jsp) was used and the AlloSitePro method was applied, which designates a score derived from a logistic regression model, which is based on the topological and physicochemical characteristics of the predicted allosteric site [75].
Results
The results of the molecular docking relative free energies of binding obtained between the various ligands from the group of NSAIDs and the protein complex formed by the Spike Receptor-Binding Domain (RBD) of SARS-CoV-2 bound with ACE2 (RBD- ACE2) are shown in Table 1. The energies were calculated with four scoring functions from the thermodynamically most favorable positions predicted by the sampling algorithm considered. All NSAIDs were thermodynamically bound to the RBD-ACE2 complex. All MolDock bindings showed an average energy of –48.981 kcal/mol, where the minimum energy was exhibited by Ketoprofen_CID_3825 (−22.837 kcal/mol) and the maximum predicted for Celecoxib_CID_2662 (–87.625 kcal/mol). Results coincided with Rerank energies, which average docking energy was –42.985 kcal/mol, with a minimum of –22.339 kcal/mol and a maximum of –71.215 kcal/mol, in the same way for Ketoprofen and Celecoxib, respectively. Furthermore, the average energy of binding according to the scoring function PLANTS was –38.457 kcal/mol, with a minimum of –27.662 kcal/mol for Acetaminophen_CID_1983 and a maximum of –58.674 kcal/mol for Benzydamine_CID_12555. Results that coincide with the position of the ligands with the minimum and maximum energies predicted by the DockT scoring function, specifically for the Acetaminophen and Benzydamine ligands, with energies of –6.323 and –8.370 kcal/mol, respectively, with an average docking energy of –7.163 kcal/mol. Ibuprofen being the NSAID with the most favored thermodynamically docking energy at the interface of the RBD-ACE2 complex, followed by Aspirin and Acetaminophen (see Figure 1).
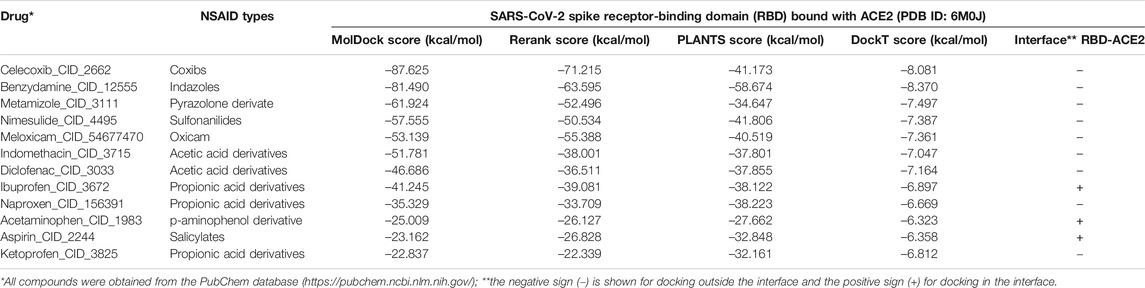
TABLE 1. Results of the binding energies of the NSAIDs considered in this study with the SARS-CoV-2 Spike Receptor-Binding Domain (RBD) complex bound with ACE2 (PDB ID: 6M0J).
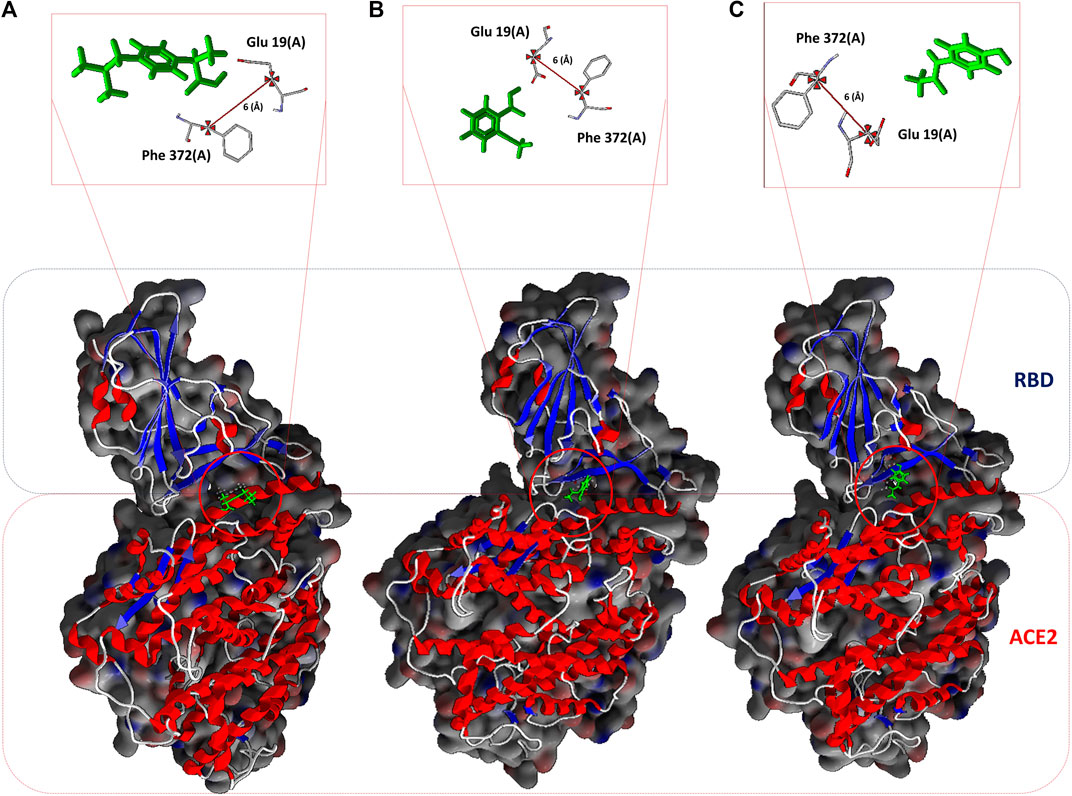
FIGURE 1. NSAIDs docking at the interface of the RBD-ACE2 protein complex. (A) Ibuprofen_CID_3672, (B) Aspirin_CID_2244, and (C) Acetaminophen_CID_1983. In the upper part, the arbitrarily chosen residuals for the subsequent analysis of molecular dynamic are observed, as they are involved in the interaction and at a distance of 6 Å from each other.
All NSAIDs exhibited favorable binding energies with the ACE2 receptor. The considered model of the Coxibs group (Celecoxib) and the compound derived from Indazoles (Benzydamine) being the most theoretically most probable and strong binding. Only 3/12 (25%) of the compounds considered in this study had a thermodynamically favorable docking with the interface of the RBD-ACE2 complex. Specifically, the compound Propionic acid derivatives Ibuprofen_CID_3672, the Salicylate Aspirin_CID_2244 model, and the p-aminophenol derivative Acetaminophen_CID_1983. Which do not present any violation of Lipinski’s rules (see Table 2). The predicted mean docking for these compounds at the interface was –31.336 kcal/mol (Ibuprofen), –22.299 kcal/mol (Aspirin) and –21.280 kcal/mol (Acetominophen), respectively. Specifically, the MolDock, Rerank, PLANTS, and DockT scoring functions predicted for Ibuprofen the binding energies of –41.245, –39.081, –38.122, and –6.897 kcal/mol, respectively. All scoring functions in each case predicted a more favorable interface docking for Ibuprofen.
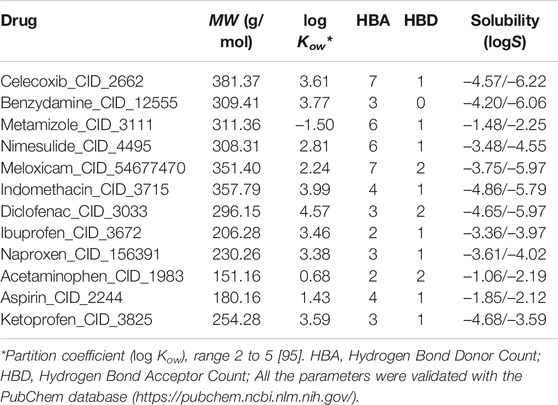
TABLE 2. Characteristics considered associated with the bioactivity and solubility of ligands using the Molinspiration server and the tools of the SIB Molecular Modeling Group | Swiss Institute of Bioinformatics.
The inhibition constant derived from the dissociation model that was applied, using the ADV algorithm for the binding of NSAIDs to the interface of the RBD-ACE2 protein complex is shown in Table 3 in their order of decreasing potency. Also, their thermodynamically favorable medium docking to the interface are also shown. Results that correspond to the amount of free protein assuming the RBD-ACE2 complex at 0 and 50% of theoretical inhibition calculated after predicting the minimum and maximum mean inhibitory concentration, and which favored Ibuprofen with a P0 and P50 of 0.830 and 0.291 M, respectively, followed by 1.100 and 0.355 M for Aspirin, and 1.660 and 0.456 M for Acetaminophen, correspondingly. This allows predicting a possible inhibitory concentration-dependent mechanism of these NSAIDs on the RBD-ACE2 interface in which less Ibuprofen concentration is required to cause some type of competitive antagonism by the region of union between RBD and ACE2. An observation consistent with the theoretical inhibition according to the PI50 and that, although it is very close among the NSAIDs linked to the interface, it also favored Ibuprofen (48.696 M) followed by Aspirin (48.769 M) and Acetaminophen (48.952 M) (see Table 3).
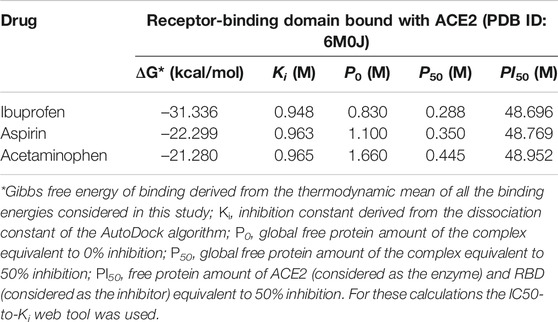
TABLE 3. Results of the amount of free protein assuming a competitive inhibition of the RBD-ACE2 complex in the presence of the NSAIDs bound to the interface.
By studying the theoretical effect of the molecular docking of each of these compounds at the interface, but as a function of time, and based on a simulation of 100 ns molecular dynamics, we found that Ibuprofen was the NSAIDs with the thermodynamically most docking favorable, and it was also the one that achieved the most favorable binding energy in the shortest time of the simulation (see Table 4). This being –47.470 kcal/mol at 75 ns, while aspirin required 100 ns to obtain its most favorable docking (51.711 kcal/mol). Although Acetaminophen achieved its best binding energy at 75 ns, it was the lowest of all the energies calculated in the most optimal simulation period for each docking (–28.634 kcal/mol). Results that correspond in each case with the Ki constants predicted in the same time periods, with 0.922 M being the most optimal for Ibuprofen in the shortest time (75 ns) with respect to the other two compounds. Although the Aspirin Ki was more favorable (0.916 M), it required 100 ns of simulation to obtain this interaction. Once again, Acetaminophen presented its most optimal value for this variable in a time similar to that of Ibuprofen, but with the least favorable Ki of all (0.953 M). In terms of the amount of theoretical free protein predicted by the P0, P50 and PI50 functions, after the docking and relative to the simulation time, we observed that Ibuprofen was able to inhibit the largest amount of complex in any of the conditions, regardless of the points sampled in the simulation used for the prediction and with respect to the rest of the compounds, with a P0, P50 and PI50, of 0.807, 0.280, and 48.693 M, respectively. While for Aspirin the most optimal values were 1.047, 0.333 and 48.760 M; and for Acetaminophen the values are 1.635, 0.440, and 48.947 M, correspondingly. Interesting results because the difference between the amount of theoretical free protein predicted by the P0, P50 and PI50 functions favors Ibuprofen over Aspirin with values of 0.240, 0.053, and 0.067 M; and against Acetaminophen with a difference in favor of Ibuprofen of 0.828, 0.160 and 0.254 M, respectively (see Table 4).
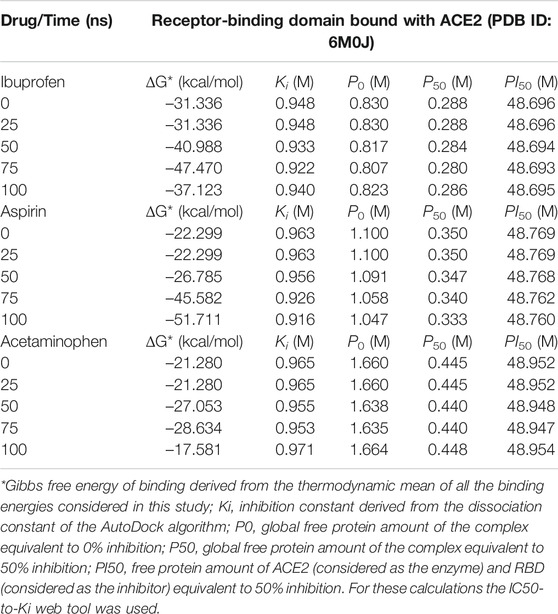
TABLE 4. Results of the binding energy and amount of free protein assuming a competitive inhibition of the RBD-ACE2 complex in the presence of the NSAIDs bound to the interface and as a function of the simulation time.
Interestingly, the three NSAIDs initially docking at the interface established interactions in the same cavity with almost the same number and type of residues of the A chain correspond to the enzyme protein ACE2 and the B chain to the RBD. Ibuprofen and Aspirin coincided in the primary interaction with 7/11 (64%) of the residues predicted in the docking, these being His16 (A), Glu19 (A), Arg71 (B), Tyr173 (B), Ala369 (A), Gln370 (A) and Arg375 (A). While Acetaminophen did so only with 5/11 (45%) of the same residues, except initially with Glu19 (A) and Gln370 (A). Aspirin and Acetaminophen coincided exclusively in the initial docking with other 4/11 (36%) residues other than those predicted in the docking with Ibuprofen, these being Asp73 (B), Glu74 (B), Lys85 (B) and Ala368 (A). The interactions between Aspirin and Acetaminophen being the most similar to each other, while Ibuprofen establishes a greater number of unique interactions, with specifically 4/11 (36%) different residues (see Tables 5–7). Although all compounds showed to induced a change in the native arrangement of the residues, 82% of Ibuprofen interactions were oriented toward the ACE2 protein, followed by Aspirin with 55%, and for Acetaminophen it was 36%. Aspirin was the compound that established the highest number of hydrogen bridges at the interface (36%), followed by Acetaminophen (27%). The greatest number of Ibuprofen interactions were steric (91%), followed by Acetaminophen (72%). These interactions underwent variations throughout the simulation as predicted also with the PockDrug-Server, reflected in the decrease of the hydrophobicity ratio of the associated cavity from –1.40 of the native structure to –1.84, –2.35 and –2.69 in presence of Aspirin, Ibuprofen and Acetaminophen, respectively. Also increasing the number of polar residues with respect to the native structure from 57% to approximately 80%, and aromatic residues from 14% in the native structure to approximately 20% (see Tables 5–7).
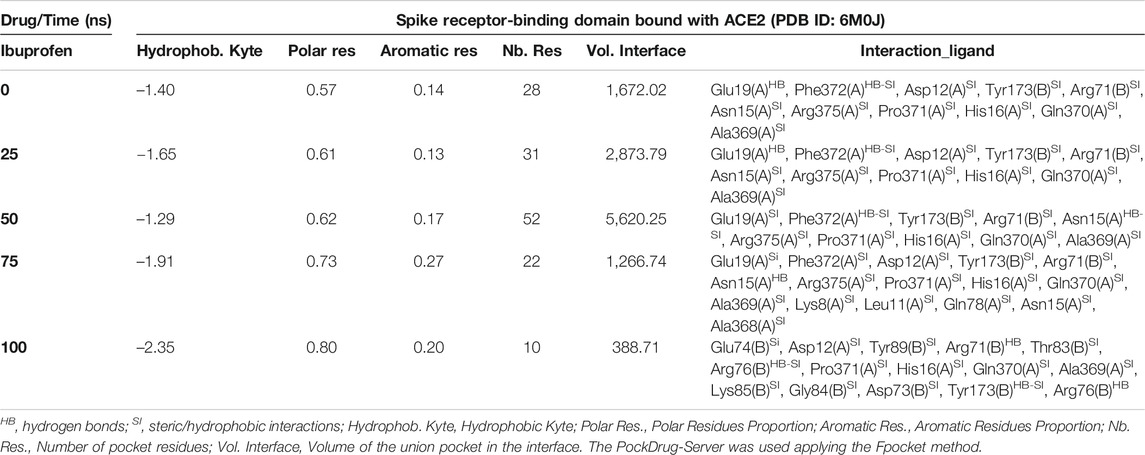
TABLE 5. Biophysical characteristics associated with the amino acid composition of the Ibuprofen binding pocket at the RBD-ACE2 interface and as a function of simulation time.
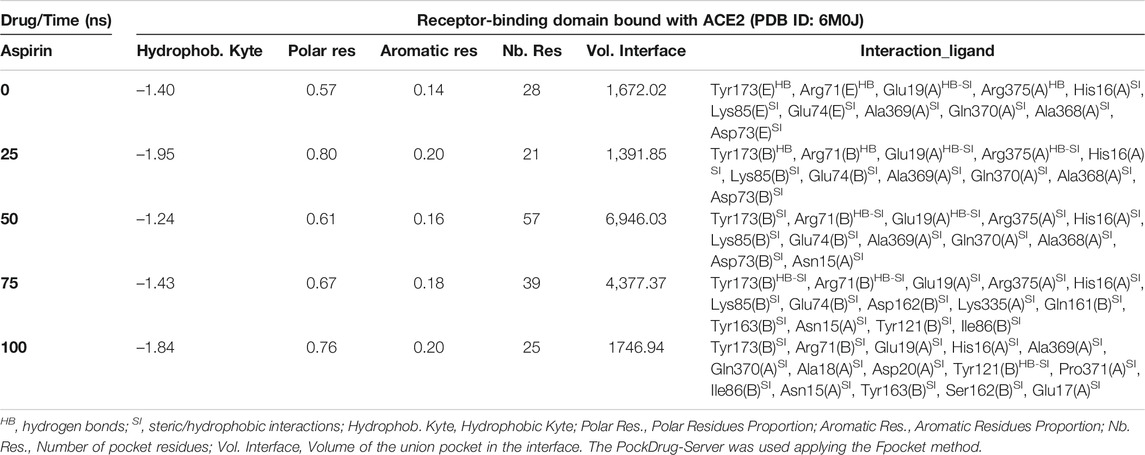
TABLE 6. Biophysical characteristics associated with the amino acid composition of the Aspirin binding pocket at the RBD-ACE2 interface and as a function of simulation time.
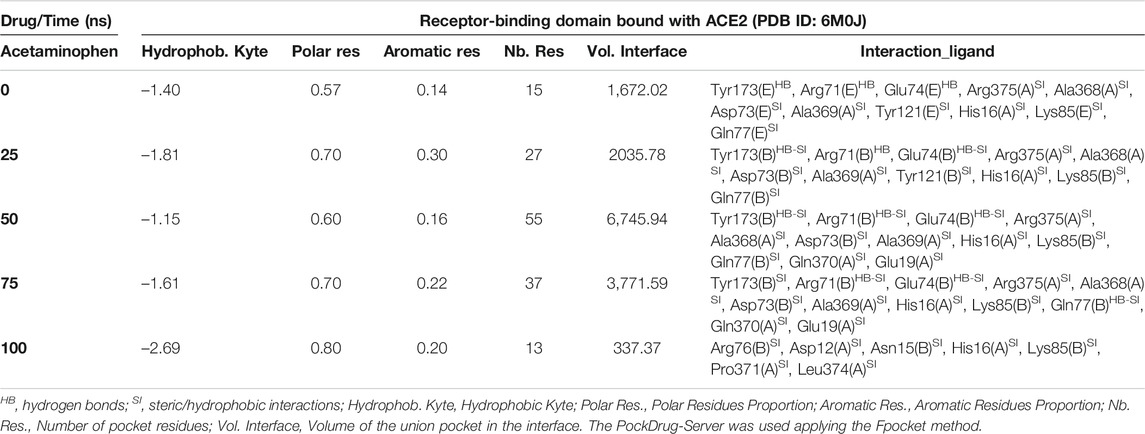
TABLE 7. Biophysical characteristics associated with the amino acid composition of the Acetaminophen binding pocket at the RBD-ACE2 interface and as a function of simulation time.
In fact, Ibuprofen was the only one of the three NSAIDs that presented a greater migration of interactions toward the viral region in our complete simulation cycle, being the only one of these compounds that established twice as many hydrogen bridges during the disruption of its binding pocket, going from 2 to 4. While Aspirin decreased from 4 to 1, and Acetaminophen from 3 to 0 (see Tables 5–7). At the end of the complete simulation cycle, Ibuprofen went from having 80% of its interactions oriented to the ACE2 region to establishing more than 67% of global interactions in the RBD virus region. In contrast, Acetaminophen went from having 64% interactions in the viral region to establishing 57% toward the ACE2 region. While Aspirin went from 55% of interactions oriented to ACE2 to 60% but also in the same region of the complex. Indicative that Aspirin stabilizes its interactions strongly only with the ACE2 region, and that Acetaminophen may lose most of the interactions initially targeting the viral region to have a more stable binding with ACE2.
At the end of the simulation, all ligands altered the volume of the predicted binding cavity for each at the interface (see Figure 2), represented by increases of up to approximately 6,900 Å3 at 50 ns and a decrease of approximately 300 Å3 of the joint pocket at 100 ns with respect to the native cavity. Ibuprofen being the compound that caused the greatest disturbance in terms of decrease in pocket volume within the interface in the shortest time of the simulation, with an approximate volume of 1,200 Å3 at 75 ns, followed by a final decrease at 100 ns of about 300 Å3. In contrast, Aspirin and Acetaminophen induced an increase in the volume of their cavity in the same time period of 75 ns of simulation between 3,700 and 4,300 Å3, Acetaminophen achieved a final decrease at 100 ns similar to Ibuprofen. Only Ibuprofen showed a consistent decrease in the simulation time of the volume of its binding pocket, after the predicted folding in all cases to 50 ns.
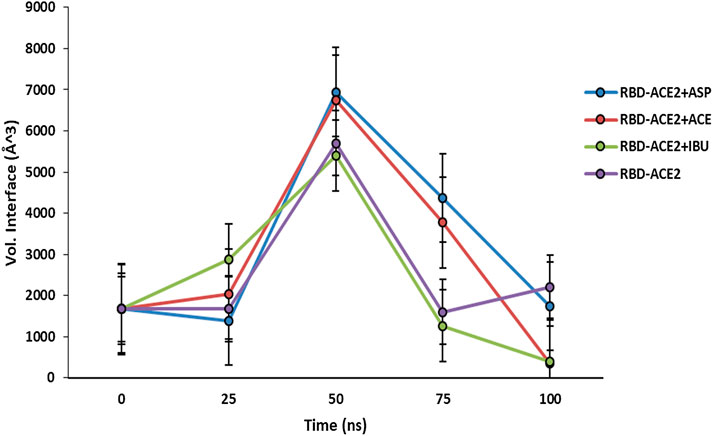
FIGURE 2. Fluctuation of volume of cavity in the interface RBD-ACE2 protein complex in presence of Ibuprofen (RBD-ACE2+IBU), Aspirin (RBD-ACE2+ASP) and Acetaminophen (RBD-ACE2+ACE) at 100 ns. As a control, RBD-ACE2 protein complex without ligands was simulated (RBD-ACE2). Vol. Interface, Volume of the union pocket in the interface. The PockDrug-Server was used applying the Fpocket method.
Both Aspirin and Acetaminophen showed fluctuations in the volume distribution of the cavity located at the interface (see Tables 5–7 and Figure 2). We also examined the main cavities predicted for the complex, which were not always the binding pocket of each ligand, precisely because the volumetric variation of the pockets in each state sampled during simulation is one of the important parameters in the classification of each cavity as main or not. We only considered the three main cavities, finding that for the three NSAIDs the mean volume of the cavity throughout the simulation was approximately 800 Å3 (see Table 8). The algorithm AllositePro predicted that these three NSAIDs bind potentially allosteric regions for RBD-ACE2 system. Furthermore, the structural and conformational changes at the level of the interface that these compounds could induce in the native structure of the complex, are interesting because the AllositePro predicted that these three NSAIDs bind potentially allosteric regions for RBD-ACE2 system. Specifically, during the first 25 ns of simulation Ibuprofen, Aspirin, and Acetaminophen bind to a potentially allosteric region with a solvent-accessible total surface area (SASA) of approximately 1,000 Å2 for Ibuprofen, and approximately 600 Å2 both for Aspirin as Acetaminophen (Table 9).
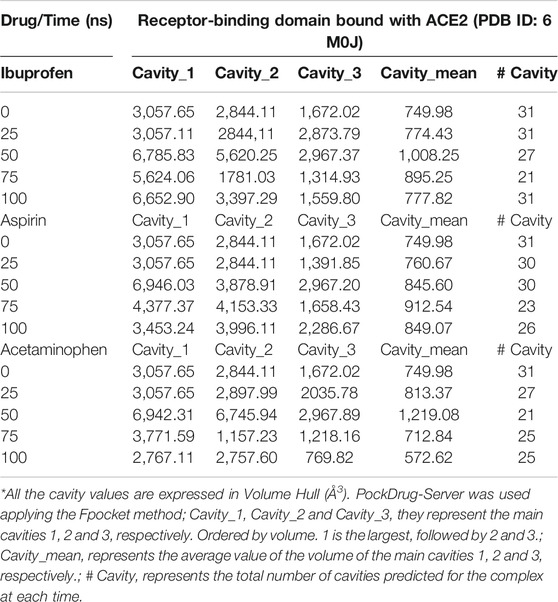
TABLE 8. Distribution of main cavities of the RBD-ACE2 complex in the presence of NSAIDs and as a function of the simulation time.
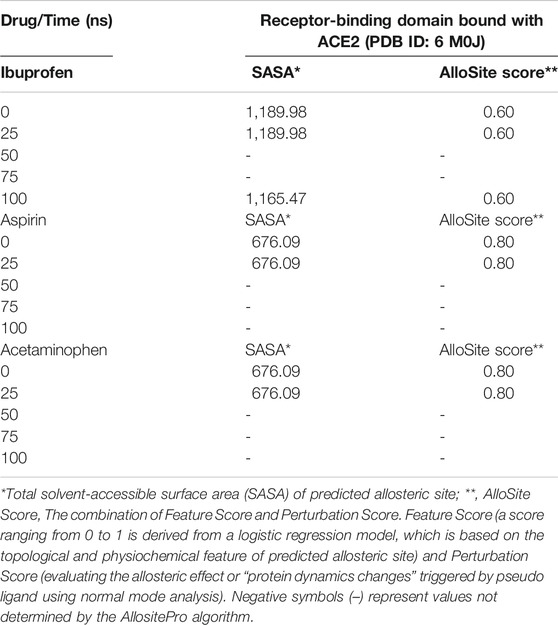
TABLE 9. Prediction of potential allosteric sites in the ligand binding pockets at the interface of the RBD-ACE2 complex as a function of simulation time.
Variations in the thermodynamic stability predicted with the myPresto package showed that all three compounds altered the thermodynamic stability of the complex as a function of time. The structural variations predicted using the algorithms of the ProSA tools, favored Ibuprofen with an optimal Z-score for the disturbance of –8.010 at 100 ns and that represents a difference with respect to the native structure of approximately –1.400. While the difference in terms of variations in the native structure after Aspirin docking was –1.305 as a result of a Z-score of –8.075 at 100 ns, and for Acetaminophen the Z-score was –8.430, representing a difference from –0.950 to 100 ns. The difference between the Z-score of Ibuprofen with Aspirin is –0.065, and with respect to Acetaminophen –0.420. Furthermore, this structural disturbance was calculated and validated with the ANM model, and coincides with ProSA, allowing us to predict that the greatest structural disturbance with the scalar distance model was 0.600 theoretically induced by Ibuprofen, followed by 0.460 for Aspirin and 0.400 for Acetaminophen. Relative to the native scalar dynamics value of 0.300 (see Tables 10 and 11).
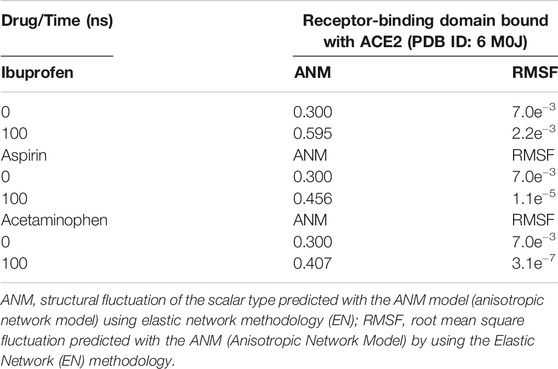
TABLE 11. Structural fluctuation of the RBD-ACE2 complex in the presence of NSAIDs at 100 ns using Anisotropic Network Methodology (ANM).
Ibuprofen was the only one of the ligands that was able to maintain a predictable binding with the possible allosteric site after 100 ns of simulation regardless of the induced structural and conformational disturbances (see Table 9). Variations in the aminoacid composition of the residues involved in the binding pockets, and in the interactions established by these with the ligands throughout the simulation time and with respect to the native structure, were also predicted. All compounds induced a change in the native arrangement of the residues corresponding to their binding pockets over time. Specifically, Ibuprofen increased the number of steric interactions throughout the simulation from 10 to 14, and Aspirin from 9 to 15. Unlike Acetaminophen, which exhibited a decrease in the number of such interactions from 10 to 7. This also explains the variations in the relative binding energies at each sampling point. Specifically, Ibuprofen was the NSAID that achieved the lowest energy structure (thermodynamically more stable) by finalizing the simulation with about –8,000 kcal/mol. While the minimum energy induced by Aspirin was –7,900 kcal/mol and by Acetaminophen –7,800 kcal/mol in the same time period. One of the lowest energy steppes reached by Ibuprofen was at 50 ns with –7,800 kcal/mol, the same reached by Acetaminophen but in a longer period of time. The complex in presence of Aspirin reached its minimum energy at 50 ns, inducing a thermodynamically more stable structure than Ibuprofen only at that point (see Table 10 and Figure 3).
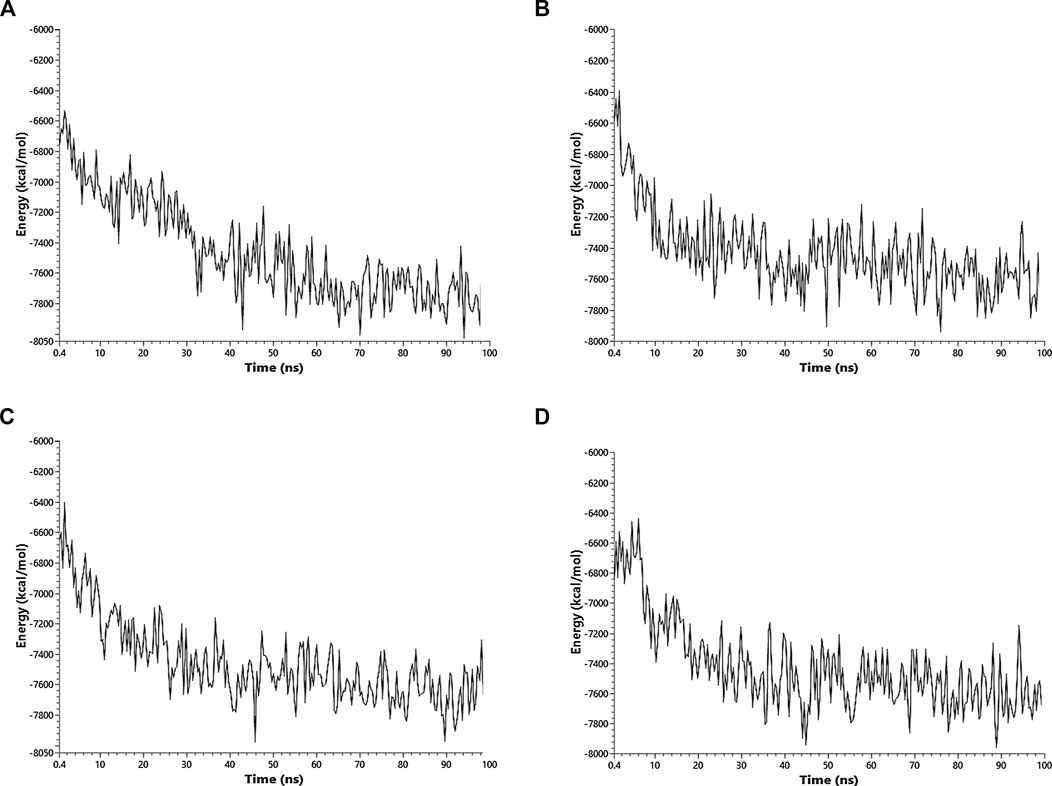
FIGURE 3. Fluctuation of the thermodynamic stability of the RBD-ACE2 complex in the presence of Ibuprofen_CID_3672 (A), Aspirin_CID_2244 (B), Acetaminophen_CID_1983 (C) and the RBD-ACE2 protein complex without ligands (D) was used as a control. Everything was simulated to 100 ns
All the results previously described are related to the structural disturbance using as a reference the pair of residues Glu19 (A) and Phe372 (A), arbitrarily chosen to be close to or involved in interaction with ligands at some point in the docking over time (see Figure 1). We found that Ibuprofen was the only one of the three NSAIDs that could be able to induce a folding in the structure, as determined with the analysis of the volume and distribution of cavities, and as predicted by ProSA and the ANM model. Causing a maximum folding of approximately 13% at 40 ns with respect to the native state and stayed around that value until 100 ns. Followed by Aspirin which induced folding of approximately 5% at 80 ns. Acetaminophen was the only one of the three compounds that did not mediate a folding of the structure, however, it caused a maximum unfolding of approximately 8% at 80 ns. In all cases the results were statistically significant (p < 0.001) (see Table 10 and Figure 4). This folding induced by Ibuprofen reduce the global flexibility (note in Figure 4 the structural flexibility of RBD-ACE2 complex without ligand), which is associate too with the diminution of the size of internal cavities and cavity volume of potentially allosteric region of complex RBD-ACE2. Thus, this conformational change induced stiffness, which has an important effect no favorable upon the energetic of interaction between local aminoacid residues in the potentially allosteric region of RBD-ACE2 complex due to binding of Ibuprofen, which blocks those interactions (see Tables 5–7). Promising results suggesting that Ibuprofen could alter the stability of the RBD-ACE2 complex and affect its formation. Which could compromise the viral infection. A possible alternative mechanism of action that would support the use of this NSAID in the treatment of COVID-19.
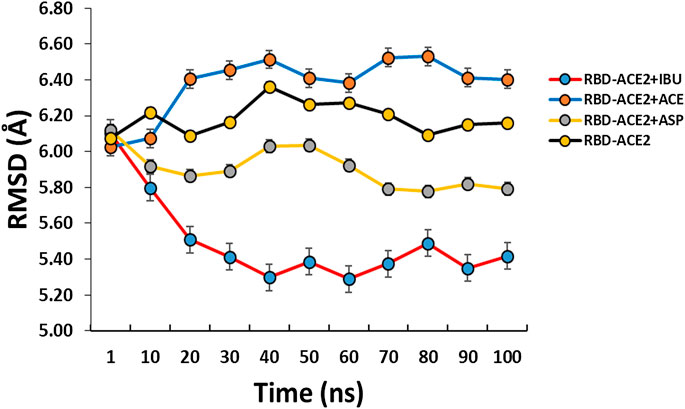
FIGURE 4. Conformational fluctuation and partial unfolding or folding of RBD-ACE2 protein complex in presence of Ibuprofen (RBD-ACE2+IBU), Aspirin (RBD-ACE2+ASP) and Acetaminophen (RBD-ACE2+ACE) at 100 ns. As a control, RBD-ACE2 protein complex without ligands was simulated (RBD-ACE2). Using as a reference the distances between the residues of Glu19(A) and Phe372(A). Arbitrarily chosen because they are at a distance of approximately 6 Å from the ligands.
Discussion
All NSAIDs exhibited favorable binding energies with the ACE2 receptor. Interestingly, Propionic acid derivatives, Salicylates and p-aminophenol derivative exhibited the least favorable binding energy with the ACE2 receptor of the protein complex, but at the same time they presented the most favorable energies for binding to the interface, and with the most probabilistically feasible docking. This is reflected by the fact that these three compounds were favorably sampled at the interface after the reclassified run in each case as the most thermodynamically feasible, but under the sampling conditions of this study (see Table 1 and Figure 1). Although this is the first study that considers the docking of this type of compound at the level of the RBD-ACE2 interface, our results coincide with those obtained by other authors who have found that Ibuprofen can have a docking score considerably better than other NSAIDs compared to proteins associated with SARS-CoV-2 [44], and that some derivatives could effectively interact with protein regions involved in this complex [76].
These NSAIDs drugs exhibited very close Ki. However, Ibuprofen showed the most favorable Ki, followed by Aspirin and Acetaminophen. It is important to note that the Ki, P0, P50 and PI50 values are theoretical predictions calculated to obtain only a rough estimate of the binding affinity and functional strength of the NSAIDs considered in this study, assuming that they have an inhibitory type behavior competitive (see Tables 3 and 4). On the basis that the thermodynamically favorable and probabilistically most feasible docking of these compounds occurs at the interface, which could theoretically compromise the binding of the Spike RBD with ACE2 in a competitive way, as has been described with other compounds capable of binding to the interface [49]. But caution should be exercised in extrapolating these data because these values, especially the experimental Ki (from which the values of P0, P50, and PI50 are derived) tend to be different from the resulting theoretical values for various biophysical parameters involved in enzyme inhibition. In this sense, these parameters should only be considered to designate the probability that any of these particular compounds may have the potential to disturb the binding between the two proteins of interest. Interestingly, these three NSAIDs are among the most studied [77, 78]. Especially since it has been described that Ibuprofen may be a potential inducer of oxidative stress as a result of said intercalation capacity at the biomolecular level [79]. However, although other NSAIDs with antagonistic activity against SARS-CoV-2 with lower inhibitory concentrations have been reported than the one obtained in this study, they are not directed to the RBD-ACE2 interface [80, 81]. Furthermore, the inhibitory activity of Ibuprofen for other unrelated viruses has been reported to be concentration dependent and may require up to 0.500 M [24]. Additionally, it has been pointed out that in the case of NSAIDs the molecular docking values can be much closer to the selectivity through energy terms, but not to the IC50, and it has been indicated that for these compounds the molecular docking represents the descriptor most important for drug-protein interactions [82].
Interestingly, the three NSAIDs initially docking at the interface established interactions in the same cavity with almost the same number and type of residues. These results indication that the residues His16 (A), Glu19 (A), Arg71 (B), Tyr173 (B), Ala369 (A), Gln370 (A) and Arg375 (A) are key in the initial interaction of these compounds on the interface, where 5/7 of them bound to residues correspond to the enzyme protein ACE2 and 2/7 to the RBD (see Tables 5–7). An interesting result because despite being NSAIDs from different groups they share chemical characteristics for this binding. Moreover, one of the few reported compounds capable of interacting with the interface RBD-ACE2 did not share any of the residues involved here [49, 50].
Although all compounds showed to induced a change in the native arrangement of the residues, Ibuprofen indicates a greater binding strength at the interface in the direction of the ACE2 protein, while Aspirin and Acetaminophen established a greater number of interactions toward the viral region. The differences in docking energies and in the inhibitory potentiality of these compounds, and which theoretically favors Ibuprofen, may be associated with the indirect structural and conformational changes that these compounds might be able to induce in the complex after docking in the interface, a phenomenon that could be evidenced with ProSA tools. In fact, we found that Ibuprofen was the only one of the three NSAIDs that increased hydrogen bridges during the disruption of its binding pocket. This is important because each hydrogen bridge can contribute about 2 kcal/mol to the interaction energy, therefore these results are indicative of a more stable interaction of Ibuprofen throughout the simulation, at the same time that this molecule could be capable to induce a disturbance in the native structure without losing its thermodynamically favorable docking. It appears that Acetaminophen is a chemical structure associated with NSAIDs with limited interaction capacity as found in other unrelated docking studies in which it has also been outperformed by Propionic acid derivatives and Salicylates in terms of Gibbs free energy of binding [83]. Also, results in terms of variations in the native structure after Aspirin docking, indicates that Ibuprofen could potentially induce the greatest structural disturbance to the complex once docking at the interface, followed by aspirin, and to a lesser extent Acetaminophen in terms of Z-score prediction and according to the knowledge-based energy function for computational protein studies and the RMSF (see Tables 10 and 11).
Specifically, we found that, at the end of the simulation, all ligands altered the volume of the predicted binding cavity for each at the interface (see Figure 2). The variations in the docking energies and the structural changes that the complex may undergo in the presence of the ligands, could be related to the conformational alterations that occur as a result of changes in the chemical forces involve in the different ligand-protein interactions presents within of the cavity throughout the simulation. In fact, the variability of these interactions is manifested with the change in composition and distribution of cavities obtained at the same simulation time (see Tables 5–7). Ibuprofen being the compound that caused the greatest disturbance in terms of decrease in pocket volume, could be indicative of compaction in that region of the protein complex in the presence of these NSAIDs due to an increase in the specific and non-specific protein-ligand interactions in that dominion. Furthermore, some conformational changes that NSAIDs can induce in other types of unrelated proteins have been shown to be associated with time-dependent inhibition [84]. All compounds induced a change in the native arrangement of the residues corresponding to their binding pockets over time. While Ibuprofen and Aspirin increased the number of steric interactions, Acetaminophen exhibited a decrease. This also explains the variations in the relative binding energies at each sampling point. Furthermore, these are results that provide a theoretical basis to understand a little more the differences observed between these NSAIDs that have been widely studied in search of pharmacological interactions [77]. The ability of Ibuprofen to maintain a predictable binding with its possible allosteric site after the structural and conformational disturbances that induced in their respective binding pockets, is indicative that Ibuprofen possibly behaves as a kind of “allosteric effector”, partially altering the structure of the complex with respect to the native form, especially disrupting the ACE2 protein region in a “competitive” way with RBD and compromising stable binding RBD-ACE2 at the interface level (see Table 9), a phenomenon that has already been evidenced with the interaction of Ibuprofen to other proteins [85–88]. These results are interesting because they show a possible alternative mechanism that has not yet been reported in the literature for these compounds against viruses such as SARS-CoV-2 and, in particular, how they might alter the RBD-ACE2 complex. Although, this represents an approach that has already been explored against SARS-CoV-2 with other compounds [89–91].
The structural compaction induced by Ibuprofen continues to attract attention (see Figure 4). Because cellular membrane is a very crowded medium due to the presence of large amounts of biomolecules in a limited surface area and the crowding play an important role over stability, conformation and dynamic of biomolecular process, then the changes induced in volume and flexibility involve in the biochemical process that occur at membrane are under steric control of important manner, then, the folding of complex induced by Ibuprofen can has an consequence important [92]. Results that show Ibuprofen as one of the few NSAIDs, which, under the conditions described in this research, may have the highest probability of generating an alteration in the stability of the RBD-ACE2 complex and affecting its formation. A result that contributes to the use that has been given to NSAIDs against COVID-19 [93].
This way, our research with a bioinformatics approach allowed us to conclude that all the NSAIDs considered in this study can be docking thermodynamically in a favorable way to the RBD-ACE2 complex. But, only the drugs Ibuprofen (a propionic acid derivative), Aspirin (salicylates) and Acetaminophen (a p-aminophenol derivative) were able to mediate a thermodynamically favorable interaction with the RBD-ACE2 complex interface. Propionic acid derivatives, salicylates and p-aminophenol derivative have the least favorable binding energy with the enzyme receptor ACE2 of the protein complex, but at the same time have the most favorable energies for interface binding and docking more probabilistically feasible. The possibility of a favorable docking under another sampling model of any of the compounds excluded by the conditions of this study is not ruled out. Ibuprofen is the most favored thermodynamically docking NSAID at the interface of the RBD-ACE2 complex, followed by Aspirin and Acetaminophen. Likewise, Ibuprofen is capable of theoretically inhibiting the largest amount of the RBD-ACE2 protein complex in any of the conditions proposed in this study, regardless of time and with respect to the rest of the compounds considered. The differences in the docking energies and in the inhibitory potential of Ibuprofen are related to the indirect structural and conformational changes that this type of compound could induce in the complex after docking at the interface. Ibuprofen was the NSAID capable of causing the greatest disturbance in terms of decreased pocket volume within the interface in the shortest time of the simulation, inducing folding of complex. Ibuprofen is therefore the NSAID with the highest probability of generating an alteration in the stability of the RBD-ACE2 complex. The present study provides information about of the possibility of a new mechanistic route of action anti COVID-19 for Ibuprofen by affect the biomacromolecular docking between RBD and ACE2 which is known as relevant for the proliferation of this virus. However, this statement, although it contributes to the described use of NSAIDs for the empirical treatment and prevention of COVID-19 [93], requires its experimental demonstration because our predictions cannot guarantee a pharmacologically and clinically relevant interaction. Therefore, more studies are needed, especially given the real controversy that exists regarding the use of these drugs against COVID-19 [19–27]. Especially because to date, only one theoretical report of a binding of ligand at the interface of the RBD-ACE2 complex is known [49, 50], but this binding is in a different region than the one found in this work, in our work the binding of Ibuprofen occurs in an allosteric region. Which constitutes another valuable contribution of our work. In addition, we recommended considering different models for water and its effect on protein-protein interaction in the absence and presence of these drugs. Another important aspect is to consider is the energetic and structural disturbance of the RBD-ACE2 complex under different concentration gradients of Ibuprofen on the surface or near the complex, this due to the apparent dependence of the anti-viral activity shown by Ibuprofen with the bioavailability [45, 94]. Why although there are no pharmacological and theoretical results in a similar direction, reflecting the novelty of our study, there is experimental research in a similar direction that has shown that Ibuprofen could contribute to the treatment of COVID-19 [45]. In fact, currently including a clinical trial that seeks to demonstrate the potential antiviral activity against SARS-CoV-2 [94].
Data Availability Statement
The datasets presented in this study can be found in online repositories. The names of the repository/repositories and accession number(s) can be found in the article/Supplementary Material.
Author Contributions
LGP, conceptualization, methodology, software, formal analysis, investigation, writing-reviewing and editing. CL, software, investigation. FFM, preparation of tables and figures, writing and editing. JLP, formal analysis, investigation, writing-reviewing and editing. JV, discussion and analysis of results, reviewing and editing. YJA, conceptualization, methodology, formal analysis, investigation, writing-reviewing and editing.
Funding
This research was funded by the author-members of each of the institutions involved.
Conflict of Interest
The authors declare that the research was conducted in the absence of any commercial or financial relationships that could be construed as a potential conflict of interest.
Acknowledgments
This research was supported by IVIC-VEN and L.G.B.M-F.E.C-LUZ-VEN. JLP acknowledges the financial support provided by the Vice-Rectorado de Investigación, Innovación y Vinculación of Escuela Politécnica Nacional of Ecuador (Grant PII-DFIS-2020-04).
Supplementary Material
The Supplementary Material for this article can be found online at: https://www.frontiersin.org/articles/10.3389/fphy.2020.587606/full#supplementary-material
References
1. Guan W-J, Ni Z-Y, Hu Y, Liang W-H, Ou C-Q, He J-X, et al. Clinical characteristics of 2019 novel coronavirus infection in China. N Engl J Med. (2020). 382:1708–20. doi:10.1056/NEJMoa2002032
2. Chan PKS, Tang JW, Hui DSC SARS: clinical presentation, transmission, pathogenesis and treatment options. Clin Sci. (2006). 110:193–204. doi:10.1042/cs20050188
3. Yang Y, Lu Q, Liu M, Wang Y, Zhang A, Jalali N, et al. Epidemiological and clinical features of the 2019 novel coronavirus outbreak in China. MedRxiv and bioRxiv [Preprints] (2020). Available at: .
10.1101/2020.02.10.20021675CrossRef Full Text | Google Scholar
4. Huang C, Wang Y, Li X, Ren L, Zhao J, Hu Y, et al. Clinical features of patients infected with 2019 novel coronavirus in Wuhan, China. Lancet (2020). 395:497–506. doi:10.1016/S0140-6736(20)30183-5.
5. Xu Z, Shi L, Wang Y, Zhang J, Huang L, Zhang C, et al. Pathological findings of COVID-19 associated with acute respiratory distress syndrome. Lancet Respir Med. (2020). 8:420–2. doi:10.1016/S2213-2600(20)30076-X.
6. Wang D, Hu B, Hu C, Zhu F, Liu X, Zhang J, et al. Clinical characteristics of 138 hospitalized patients with 2019 novel coronavirus-infected pneumonia in Wuhan, China. J Am Med Assoc. (2020). 323:1061–9. doi:10.1001/jama.2020.1585.
7. Matthay MA, Aldrich JM, Gotts JE Treatment for severe acute respiratory distress syndrome from COVID-19. Lancet Respir Med. (2020). 8:433–4. doi:10.1016/S2213-2600(20)30127-2.
8. Chen N, Zhou M, Dong X, Qu J, Gong F, Han Y, et al. Epidemiological and clinical characteristics of 99 cases of 2019 novel coronavirus pneumonia in Wuhan, China: a descriptive study. Lancet (2020). 395:507–13. doi:10.1016/S0140-6736(20)30211-7.
9. Zhang W, Zhao Y, Zhang F, Wang Q, Li T, Liu Z, et al. The use of anti-inflammatory drugs in the treatment of people with severe coronavirus disease 2019 (COVID-19): the experience of clinical immunologists from China. Clin Immunol. (2020). 214:108393. doi:10.1016/j.clim.2020.108393.
10. Vanden Eynde JJ COVID-19: a brief overview of the discovery clinical trial. Pharmaceuticals (2020). 13, E65. doi:10.3390/ph13040065.
11. Shahera U, Sultana N, Sharmin M, Malakar J, Rashedul M, Zafrul K A comprehensive literature review on the pandemic coronavirus disease 2019 (COVID-19): Bangladesh is fighting against it. Am J Biomed Life Sci. (2020). 8, 76–82. doi:10.11648/j.ajbls.20200804.13
12. Hussain Bash S Corona virus drugs: a brief overview of past, present and future. J PeerSci. (2020). 2:1–16. doi:10.5281/zenodo.3747641.
13. Little P Non-steroidal anti-inflammatory drugs and covid-19. Br Med J (2020). 368:m1185. doi:10.1136/bmj.m1185.
14. Giollo A, Adami G, Gatti D, Idolazzi L, Rossini M Coronavirus disease 19 (Covid-19) and non-steroidal anti-inflammatory drugs (NSAID). Ann Rheum Dis. (2020). 0:1. doi:10.1136/annrheumdis-2020-217598.
15. Lands LC, Dauletbaev N High-dose ibuprofen in cystic fibrosis. Pharmaceuticals (2010). 3:2213–24. doi:10.3390/ph3072213.
16. Warden SJ Prophylactic use of NSAIDs by athletes: a risk/benefit assessment. Phys Sportsmed. (2010). 38:132–8. doi:10.3810/psm.2010.04.1770.
17. Bally M, Dendukuri N, Rich B, Nadeau L, Helin-Salmivaara A, Garbe E, et al. Risk of acute myocardial infarction with NSAIDs in real world use: bayesian meta-analysis of individual patient data. Br Med J (2017). 357:j1909. doi:10.1136/bmj.j1909
18. Lanas A, Chan FKL Peptic ulcer disease. Lancet (2017). 390:613–24. doi:10.1016/s0140-6736(16)32404-7.
19. Le Monda Le ministre de la santé déconseille l’ibuprofène contre le coronavirus (2020). Available at: https://www.lemonde.fr/societe/article/2020/03/14/face-au-coronavirus-le-ministre-de-la-sante-recommande-de-ne-pas-prendre-d-Ibuprofene_6033095_3224.html [Accessed March 15, 2018].
20.DGS-urgent. Actualisation recommandations covid 19 (2020). Available at: https://dgs-urgent.sante.gouv.fr/dgsurgent/inter/detailsMessageBuilder.do;jsessionid=21ECACBF8B1ECE6C542B9126E7A8215F.du-dgsurgentc2?id=30500&cmd=visualiserMessage [Accessed June 16, 2020].
21.20 minutes. Coronavirus à Bordeaux: Au cœur de l’unité Covid-19 du service réanimation du CHU Pellegrin (2020). Available at: https://www.20minutes.fr/societe/2742271-20200317-coronavirus-bordeaux-ur-unite-covid-19-service-reanimation-chu-pellegrin [Accessed June 16, 2020].
22. Fang L, Karakiulakis G, Roth M Are patients with hypertension and diabetes mellitus at increased risk for COVID-19 infection? Lancet Respir Med. (2020). 8:e21. doi:10.1016/s2213-2600(20)30116-8
23. Rinott E, Kozer E, Shapira Y, Bar-Haim A, Youngster I Ibuprofen use and clinical outcomes in COVID-19 patients. Clin Microbiol Infect. (2020). 26:e5–7. doi:10.1016/j.cmi.2020.06.003
24. Kutti G, Kotagiri R, Chandiramani VH, Mohan BP, Vegunta R, Rokkam VRP COVID-19 and avoiding ibuprofen. How good is the evidence? Am J Therapeut. (2020). 27:e400–2. doi:10.1097/mjt.0000000000001196
25. Martins‐Filho P, Marques E, Santana V No current evidence supporting risk of using Ibuprofen in patients with COVID‐19. Int J Clin Pract. (2020). 74:e13576. doi:10.1111/ijcp.13576
26. Moore N, Carleton B, Blin P, Bosco-Levy P, Droz C Does ibuprofen worsen COVID-19? Drug Saf. (2020). 43:611–4. doi:10.1007/s40264-020-00953-0
27. Torjesen I Covid-19: ibuprofen can be used for symptoms, says UK agency, but reasons for change in advice are unclear. Br Med J (2020). 367:m1555. doi:10.1136/bmj.m1555
28. Winther B, Mygind N Potential benefits of Ibuprofen in the treatment of viral respiratory infections. Inflammopharmacology (2003). 11:445–52. doi:10.1163/156856003322699627
29. Marzolini C, Stader F, Stoeckle M, Franzeck F, Egli A, Bassetti S, et al. Effect of systemic inflammatory response to SARS-CoV-2 on lopinavir and hydroxychloroquine plasma concentrations. Antimicrob Agents Chemother. (2020). 64:e01177–20. doi:10.1128/aac.01177-20
30.World Health Organization. (2020). The use of non-steroidal anti-inflammatory drugs (NSAIDs) in patients with COVID-19: scientific brief. Available at: https://www.who.int/news-room/commentaries/detail/the-use-of-non-steroidal-anti-inflammatory-drugs-(nsaids)-in-patients-with-covid-19 [Accessed April 19, 2020].
31. Sodhi M, Etminan M Safety of ibuprofen in patients with COVID-19. Chest (2020). 158:55–6. doi:10.1016/j.chest.2020.03.040
32. Wittine K, Benci K, Rajić Z, Zorc B, Kralj M, Marjanović M, et al. The novel phosphoramidate derivatives of NSAID 3-hydroxypropylamides: synthesis, cytostatic and antiviral activity evaluations. Eur J Med Chem. (2009). 44:143–51. doi:10.1016/j.ejmech.2008.03.037
33. Cherrick HM, Li KK, Li S-L, Park N-H Effect of ibuprofen on the in vitro and in vivo reactivation of latent HSV-1. Oral Surg Oral Med Oral Pathol. (1992). 73:321–7. doi:10.1016/0030-4220(92)90129-e
34. Rajic Z, Butula I, Zorc B, Kraljevic S, Hock K, Pavelic K, et al. Cytostatic and antiviral activity evaluations of hydroxamic derivatives of some non-steroidal anti-inflammatory drugs. Chem Biol Drug Des. (2009). 73:328–38. doi:10.1111/j.1747-0285.2009.00774.x
35. Terrier O, Dilly S, Pizzorno A, Henri J, Berenbaum F, Lina B, et al. Broad-spectrum antiviral activity of naproxen: from Influenza A to SARS-CoV-2 Coronavirus. MedRxiv and bioRxiv [Preprints] (2020). Available at: .
10.1101/2020.04.30.069922CrossRef Full Text | Google Scholar
36. Amici C, La Frazia S, Brunelli C, Balsamo M, Angelini M, Santoro MG Inhibition of viral protein translation by indomethacin in vesicular stomatitis virus infection: role of eIF2α kinase PKR. Cell Microbiol. (2015). 17:1391–404. doi:10.1111/cmi.12446
37. Chen N, Warner JL, Reiss CS NSAID treatment suppresses VSV propagation in mouse CNS. Virology (2000). 276:44–51. doi:10.1006/viro.2000.0562
38. Veljkovic V, Goeijenbier M, Glisic S, Veljkovic N, Perovic V, Sencanski M, et al. In silico analysis suggests repurposing of Ibuprofen for prevention and treatment of EBOLA virus disease. F1000 Res. (2015). 1:104. doi:10.12688/f1000research.6436.1
39. Veljkovic V Ibuprofen as a template molecule for drug design against Ebola virus. Front Biosci. (2018). 23:947–53. doi:10.2741/4627
40. Bourinbaiar AS, Lee-Huang S The non-steroidal anti-inflammatory drug, indomethacin, as an inhibitor of HIV replication. FEBS (Fed Eur Biochem Soc) Lett. (1995). 360:85–8. doi:10.1016/0014-5793(95)00057-g
41. Chen CJ, Raung SL, Kuo MD, Wang YM Suppression of Japanese encephalitis virus infection by non-steroidal anti-inflammatory drugs. J Gen Virol. (2002). 83:1897–905. doi:10.1099/0022-1317-83-8-1897
42. Rothan HA, Bahrani H, Abdulrahman AY, Mohamed Z, Teoh TC, Othman S, et al. Mefenamic acid in combination with ribavirin shows significant effects in reducing chikungunya virus infection in vitro and in vivo. Antivir Res. (2016). 127:50–6. doi:10.1016/j.antiviral.2016.01.006
43. Robb CT, Goepp M, Rossi AG, Yao C. Non–steroidal anti‐inflammatory drugs, prostaglandins, and COVID‐19. British J Pharmacol (2020). 177(21):4899--4920. doi:10.1111/bph.15206
44. Lagzian M, Valadan R, Saeedi M, Roozbeh F, Hedayatizadeh-Omran A, Amanlou M, et al. Repurposing naproxen as a potential antiviral agent against SARS-CoV-2. Research Square [Preprint] (2020). Available at: https://www.researchsquare.com/article/rs-21833/v1.
45. García NH, Porta DJ, Alasino RV, Muñoz SE, Beltramo DM Ibuprofen, a traditional drug that may impact the course of COVID-19 New effective formulation in nebulizable solution. Med Hypotheses. (2020). 144:110079. doi:10.1016/j.mehy.2020.110079
46. Liu C, Zhou Q, Li Y, Garner LV, Watkins SP, Carter LJ, et al. Research and development on therapeutic agents and vaccines for COVID-19 and related human coronavirus diseases. ACS Cent Sci. (2020). 6:315–31. doi:10.1021/acscentsci.0c00272
47. Siddiqui AJ, Jahan S, Ashraf SA, Alreshidi M, Ashraf MS, Patel M, et al. Current status and strategic possibilities on potential use of combinational drug therapy against COVID-19 caused by SARS-CoV-2. J Biomol Struct Dyn (2020). 5:1--14. doi:10.1080/07391102.2020.1802345.
48. Ortega JT, Serrano ML, Pujol FH, Rangel HR Role of changes in SARS-CoV-2 spike protein in the interaction with the human ACE2 receptor: an in silico analysis. EXCLI J (2020). 19:410–7. doi:10.17179/excli2020-1167
49. Wu C, Liu Y, Yang Y, Zhang P, Zhong W, Wang Y, et al. Analysis of therapeutic targets for SARS-CoV-2 and discovery of potential drugs by computational methods. Acta Pharm Sin B (2020). 10:766–88. doi:10.1016/j.apsb.2020.02.008
50. Han Y, Král P Computational design of ACE2-based peptide inhibitors of SARS-CoV-2. ACS Nano. (2020). 14:5143–7. doi:10.1021/acsnano.0c02857
51. Hinz B, Cheremina O, Brune K Acetaminophen (Paracetamol) is a selective cyclooxygenase-2 inhibitor in man. Faseb J (2008). 22:383–90. doi:10.1096/fj.07-8506com
52. Zoete V, Daina A, Bovigny C, Michielin O SwissSimilarity: a web tool for low to ultra high throughput ligand-based virtual screening. J Chem Inf Model. (2016). 56:1399–404. doi:10.1021/acs.jcim.6b00174
53. Daina A, Michielin O, Zoete V SwissADME: a free web tool to evaluate pharmacokinetics, drug-likeness and medicinal chemistry friendliness of small molecules. Sci Rep. (2017). 7:42717. doi:10.1038/srep42717
54. Dos Santos K, Guedes I, Karl A, Dardenne L Highly flexible ligand docking: benchmarking of the DockThor program on the LEADS-PEP protein-peptide dataset. J Chem Inf Model. (2020). 60:667–83. doi:10.1021/acs.jcim.9b00905
55. Thomsen R, Christensen MH MolDock: a new technique for high-accuracy molecular docking. J Med Chem. (2006). 49:3315–21. doi:10.1021/jm051197e
56. Velraj M, Chand P Molecular docking approach of potent natural inhibitors against 3D4Z, 4TRO and 5ACS receptors for anti-tubercular activity. Int J Res Pharm Sci. (2019). 10:303–12. doi:10.26452/ijrps.v10i1.1824
57. Umesh , Kundu D, Selvaraj C, Singh SK, Dubey VK Identification of new anti-nCoV drug chemical compounds from Indian spices exploiting SARS-CoV-2 main protease as target. J Biomol Struct Dyn. (2020). 1:1–9. doi:10.1080/07391102.2020.1763202
58. Ardra P, Prachi S, Hariprasad VR, Babu UV, Mohamed R, Raghavendra PR Potential phytochemical inhibitors of the coronavirus rna dependent rna polymerase: a molecular docking study. Research Square [Preprint] (2020). Available at: https://www.researchsquare.com/article/rs-35334/v1.
59. Subramanian S Some fda approved drugs exhibit binding affinity as high as -16.0 kcal/mol against COVID-19 main protease (Mpro): a molecular docking study. Research Square [Preprint] (2020). Available at: https://www.researchsquare.com/article/rs-25649/v1.
60. Tong T Drug targets in severe acute respiratory syndrome (SARS) virus and other coronavirus infections. Infect Disord - Drug Targets. (2009). 9:223–45. doi:10.2174/187152609787847659
61. Kumar Y, Singh H Silico identification and docking-based drug repurposing against the main protease of SARS-CoV-2, causative agent of COVID-19. ChemRxiv [Preprint] (2020). Available at: .
10.26434/chemrxiv.12049590.v1CrossRef Full Text | Google Scholar
62. Subramanian S Some neem leaves extract compounds exhibit very high binding affinity against COVID-19 main protease (Mpro): a molecular docking study. Research Square [Preprint] (2020). Available at: https://www.researchsquare.com/article/rs-25649/v1
63. Popov ME, Karlinsky DM Search for invisible binding sites of low-molecular-weight compounds on protein molecules and prediction of inhibitory activity. Mol Biol. (2013). 47:592–8. doi:10.1134/s0026893313040122
64. Cer RZ, Mudunuri U, Stephens R, Lebeda FJ IC50-to-Ki: a web-based tool for converting IC50 to Ki values for inhibitors of enzyme activity and ligand binding. Nucleic Acids Res. (2009). 37:W441–5. doi:10.1093/nar/gkp253
65. Wang Z, Wang X, Jia Y, Yin H, Feng Y, Jiang L, et al. Inhibition of human UDP‐glucuronosyltransferase enzymes by midostaurin and ruxolitinib: implications for drug‐drug interactions. Biopharm Drug Dispos (2020), 41(6): 231--238. doi:10.1002/bdd.2241
66. Baez-Santos YM, Mielech AM, Deng X, Baker S, Mesecar AD Catalytic function and substrate specificity of the papain-like protease domain of nsp3 from the middle east respiratory syndrome coronavirus. J Virol. (2014). 88:12511–27. doi:10.1128/jvi.01294-14
67. Wang J Fast Identification of possible drug treatment of coronavirus disease-19 (COVID-19) through computational drug repurposing study. J Chem Inf Model. (2020). 60:3277–86. doi:10.1021/acs.jcim.0c00179
68. Kasahara K, Ma B, Goto K, Dasgupta B, Higo J, Fukuda I, et al. myPresto/omegagene: a GPU-accelerated molecular dynamics simulator tailored for enhanced conformational sampling methods with a non-Ewald electrostatic scheme. Biophys Physicobio. (2016). 13:209–16. doi:10.2142/biophysico.13.0_209
69. Wada M, Kanamori E, Nakamura H, Fukunishi Y Selection of in silico drug screening results for G-protein-coupled receptors by using universal active probes. J Chem Inf Model. (2011). 51:2398–407.
70. Yonezawa Y Electrostatic properties of water models evaluated by a long-range potential based solely on the wolf charge-neutral condition. Chem Phys Lett. (2013). 556:308–14.
10.1016/j.cplett.2012.12.028CrossRef Full Text | Google Scholar
71. Eyal E, Lum G, Bahar I The anisotropic network model web server at 2015 (ANM 2.0). Bioinformatics (2015). 31:1487–9. doi:10.1093/bioinformatics/btu84
72. Wiederstein M, Sippl MJ ProSA-web: interactive web service for the recognition of errors in three-dimensional structures of proteins. Nucleic Acids Res. (2007). 35:W407–10. doi:10.1093/nar/gkm290
73. Sippl MJ Recognition of errors in three-dimensional structures of proteins. Proteins (1993). 17:355–62. doi:10.1002/prot.340170404
74. Hussein HA, Borrel A, Geneix C, Petitjean M, Regad L, Camproux AC PockDrug-Server: a new web server for predicting pocket druggability on holo and apo proteins. Nucleic Acids Res. (2015). 43:W436–42. doi:10.1093/nar/gkv462
75. Huang W, Lu S, Huang Z, Liu X, Mou L, Luo Y, et al. Allosite: a method for predicting allosteric sites. Bioinformatics (2013). 29:2357–9. doi:10.1093/bioinformatics/btt399
76. Patel A, Rajendran M, Pakala S, Shah A, Patel H, Karyala P Virtual screening of curcumin and its analogs against the spike surface glycoprotein of SARS-CoV-2 and SARS-CoV. ChemRxiv [Preprint] (2020). Available at: .
10.26434/chemrxiv.12142383.v2CrossRef Full Text | Google Scholar
77. Saxena A, Balaramnavar VM, Hohlfeld T, Saxena AK Drug/drug interaction of common NSAIDs with antiplatelet effect of aspirin in human platelets. Eur J Pharmacol. (2013). 721:215–24. doi:10.1016/j.ejphar.2013.09.032
78. Uchenna F, Chris U, Izuchukwu D, Okafor SN New carboxamides bearing benzenesulphonamides: synthesis, molecular docking and pharmacological properties. Bioorg Chem. (2019). 92:103265. doi:10.1016/j.bioorg.2019.103265
79. Husain MA, Sarwar T, Rehman SU, Ishqi HM, Tabish M Ibuprofen causes photocleavage through ROS generation and intercalates with DNA: a combined biophysical and molecular docking approach. Phys Chem Chem Phys. (2015). 17:13837–50. doi:10.1039/c5cp00272a
80. Wu R, Wang L, Kuo H-CD, Shannar A, Peter R, Chou PJ, et al. An update on current therapeutic drugs treating COVID-19. Curr Pharmacol Rep. (2020). 6:56–70. doi:10.1007/s40495-020-00216-7
81. Gimeno A, Mestres-Truyol J, Ojeda-Montes MJ, Macip G, Saldivar-Espinoza B, Cereto-Massagué A, et al. Prediction of novel inhibitors of the main protease (M-pro) of SARS-CoV-2 through consensus docking and drug reposition. Int J Mol Sci. (2020). 21:3793. doi:10.3390/ijms21113793
82. Mikra C, Rossos G, Hadjikakou S, Kourkoumelis N Molecular docking and structure activity relationship studies of NSAIDs. What do they reveal about IC50? Lett Drug Des Discov. (2017). 14:949–58. doi:10.2174/1570180814666161207143231
83. Azam F, Alabdullah NH, Ehmedat HM, Abulifa AR, Taban I, Upadhyayula S NSAIDs as potential treatment option for preventing amyloid β toxicity in Alzheimer’s disease: an investigation by docking, molecular dynamics, and DFT studies. J Biomol Struct Dyn. (2017). 36:2099–117. doi:10.1080/07391102.2017.1338164
84. Kurumbail RG, Stevens AM, Gierse JK, McDonald JJ, Stegeman RA, Pak JY, et al. Structural basis for selective inhibition of cyclooxygenase-2 by anti-inflammatory agents. Nature (1996). 384:644–8. doi:10.1038/384644a0
85. Di Masi A, Gullotta F, Bolli A, Fanali G, Fasano M, Ascenzi P Ibuprofen binding to secondary sites allosterically modulates the spectroscopic and catalytic properties of human serum heme-albumin. FEBS J (2011). 278:654–62. doi:10.1111/j.1742-4658.2010.07986.x
86. Baroni S, Mattu M, Vannini A, Cipollone R, Aime S, Ascenzi P, et al. Effect of Ibuprofen and warfarin on the allosteric properties of haem-human serum albumin. Eur J Biochem. (2001). 268:6214–20. doi:10.1046/j.0014-2956.2001.02569.x
87. Ascenzi P, Cao Y, Tundo GR, Coletta M, Fanali G, Fasano M Ibuprofen and warfarin modulate allosterically ferrous human serum heme–albumin nitrosylation. Biochem Biophys Res Commun. (2011). 411:185–9. doi:10.1016/j.bbrc.2011.06.130
88. Ascenzi P, di Masi A, De Sanctis G, Coletta M, Fasano M Ibuprofen modulates allosterically NO dissociation from ferrous nitrosylated human serum heme-albumin by binding to three sites. Biochem Biophys Res Commun. (2009). 387:83–6. doi:10.1016/j.bbrc.2009.06.117
89. Ping W, Chun-Mei L, Lu Z, Ying L, Lu-Hua L Substrate binding and homo-dimerization of SARS 3CL proteinase are mutual allosteric effectors. Acta Phys Chim Sin. (2010). 26:1093–8. doi:10.3866/PKU.WHXB20100449
90. Xia B, Kang X Activation and maturation of SARS-CoV main protease. Protein Cell. (2011). 2:282–90. doi:10.1007/s13238-011-1034-1.
91. Olotu FA, Omolabi KF, Soliman MES. Leaving no stone unturned: Allosteric targeting of SARS-CoV-2 Spike protein at putative druggable sites disrupts human angiotensin-converting enzyme interactions at the receptor binding domain. Inform Med unlocked (2020). 21:100451. doi:10.1016/j.imu.2020.100451
92. Gomez D, Huber K, Klumpp S On protein folding in crowded conditions. J Phys Chem Lett. (2019). 10:7650–6. doi:10.1021/acs.jpclett.9b02642
93. Hyoung-Shik S Empirical treatment and prevention of COVID-19. J Infect Chemother. (2020). 52:142–53. doi:10.3947/ic.2020.52.2.142
94.ClinicalTrials.gov. Garcia (MD). National Council of Scientific and Technical Research (Argentina). Extended Compassionate Use Program (UCA) with inhalational ibuprofen in patients with acute respiratory pathology, mediated by COVID-19. (2020) Available in: https://clinicaltrials.gov/ct2/show/NCT04382768.
95. Bhal S Data from: LogP—Making sense of the value. Advanced chemistry development (2007). Available from: https://www.acdlabs.com/download/app/physchem/making_sense.pdf.
Keywords: SARS-COV-2, NSAIDs, molecular docking, molecular dynamics, COVID-19
Citation: González-Paz LA, Lossada CA, Fernández-Materán FV, Paz JL, Vera-Villalobos J and Alvarado YJ (2020) Can Non-steroidal Anti-inflammatory Drugs Affect the Interaction Between Receptor Binding Domain of SARS-COV-2 Spike and the Human ACE2 Receptor? A Computational Biophysical Study. Front. Phys. 8:587606. doi: 10.3389/fphy.2020.587606
Received: 27 July 2020; Accepted: 19 October 2020;
Published: 12 November 2020.
Edited by:
Yong Xu, Northwestern Polytechnical University, ChinaReviewed by:
Antonio Marco Batista, Universidade Estadual de Ponta Grossa, BrazilAnanya Debnath, Indian Institute of Technology Jodhpur, India
Qin Xu, Shanghai Jiao Tong University, China
Copyright © 2020 Gonzalez-Paz, Lossada, Fernández-Materán, Paz, Vera-Villalobos and Alvarado. This is an open-access article distributed under the terms of the Creative Commons Attribution License (CC BY). The use, distribution or reproduction in other forums is permitted, provided the original author(s) and the copyright owner(s) are credited and that the original publication in this journal is cited, in accordance with accepted academic practice. No use, distribution or reproduction is permitted which does not comply with these terms.
*Correspondence: Lenin A. González-Paz, bGdvbnphbGV6cGF6QGdtYWlsLmNvbQ==, J. L. Paz, am9zZS5wYXpyQGVwbi5lZHUuZWMuY29t
Can Non-steroidal Anti-inflammatory Drugs Affect the Interaction Between Receptor Binding Domain of SARS-COV-2 Spike and the Human ACE2 Receptor? A Computational Biophysical Study
- 1Laboratorio de Genética y Biología Molecular (L.G.B.M), Facultad Experimental de Ciencias (F.E.C), Departamento de Biología, Universidad Del Zulia (L.U.Z), Maracaibo, Venezuela
- 2Laboratorio de Caracterización Molecular y Biomolecular, Centro de Investigación y Tecnología de Materiales (CITeMA), Instituto Venezolano de Investigaciones Científicas (IVIC), Maracaibo, Venezuela
- 3Departamento de Física, Escuela Politécnica Nacional, Quito, Ecuador
- 4Facultad de Ciencias Naturales y Matemáticas, Departamento de Química y Ciencias Ambientales, Laboratorio de Análisis Químico Instrumental (LAQUINS), Escuela Superior Politécnica Del Litoral, Guayaquil, Ecuador
SARS-CoV-2 has caused millions of infections and more than 600,000 deaths worldwide. Despite the large number of studies to date, there is no specifically effective treatment available for SARS-CoV-2. However, it has been proposed to target reused drugs with potential antiviral activity to the interface between the angiotensin-converting enzymes 2 (ACE2) and the receptor binding domain (RBD) of SARS-CoV-2 to avoid cell recognition. Some non-steroidal anti-inflammatory drugs (NSAIDs) have been reported to have some type of activity against a wide variety of viruses including SARS-CoV-2. Therefore, we carried out an exhaustive computational biophysical study of various NSAIDs targeting the RBD-ACE2 complex using multiple comparative analysis of docking and molecular dynamics. Only the Ibuprofen (Propionic acid derivative), Aspirin (Salicylate), and the Acetaminophen (p-aminophenol derivative) had a thermodynamically favorable docking with the interface of the RBD-ACE2 complex under the conditions of this study. Although, Ibuprofen was the NSAIDs with the most thermodynamically favorable docking in the shortest simulation time, and was the major inducer of structural changes, conformational changes, and overall changes in the complex throughout the simulation, including disturbances in composition and distribution of cavities at the interface. Results that point to Ibuprofen as an NSAID that, under the conditions outlined in this investigation, may have the highest probability of generating a disturbance in the stability of the RBD-ACE2 complex. This statement, although it could contribute information for the empirical treatment and prevention of COVID-19, represents only a theoretical orientation and approach, and requires its experimental demonstration because our predictions cannot secure a pharmacologically and clinically relevant interaction. However, these results are relevant due that suggest a possible mechanism of action of Ibuprofen against COVID-19 in addition to its anti-inflammatory properties, of which there are no reports in the literature.
Introduction
The SARS-CoV-2 pandemic has required rapid drug searches for the management of COVID-19 disease. COVID-19 is a pathology characterized by an acute viral infection in humans with an average incubation period of 3 days [1] similar to SARS-CoV-1 [2]. The most common characteristics reported during COVID-19 are similar to those described for other coronaviruses and are represented by fever (88%), cough (68%), fatigue (38%), while vomiting (5%) and diarrhea (4%) are less frequent [1, 3]. The development of acute respiratory distress syndrome (ARDS) has been reported in most patients [4–7]. Additionally, patients are prone to a variety of ARDS-related complications, including acute heart injury and secondary infection [8]. Given this, it has been pointed out that the use of anti-inflammatory drugs concomitantly with the standard treatments recommended by the World Health organization (WHO) could be useful against COVID-19 [9] including non-steroidal anti-inflammatory drugs (NSAIDs) [10–14]. NSAIDs have been little considered because although they have a high degree of safety and have been used in adverse respiratory conditions [15] are equally associated with various adverse effects [16–18].
In fact, the use of NSAIDs such as Ibuprofen is highly controversial because it has been reported that in animal models it can mediate the overexpression of the angiotensin-converting enzyme 2 (ACE2) essential for viral recognition and it has been speculated that this could increase the risk of COVID-19 [19–23]. However, in various investigations in patients with COVID-19, the use of Ibuprofen has not been associated with worse clinical outcomes, compared to other NSAIDs such as Acetaminophen [24–26]. On the contrary, it has been described that the chronic use of this type of NSAIDs could even protect against the occurrence and severity of COVID-19 [26, 27]. Therefore, deepening theoretical and experimental in this direction is necessary and is especially justified, because it has been reported that NSAIDs can have a positive effect against ARDS [28] and because the inflammatory process described during SARS-CoV-2 infection has greatly limited the use of other potential drugs [29].
The WHO has indicated that there is no evidence to confirm an aggravation of COVID-19 infection with the administration of NSAIDs [30, 31]. In fact, it has been reported that some NSAIDs could have some type of activity against viruses such as VZV [32], HCMV [32], HSV-1 [33], influenza virus A⁄H1N1 subtype [34, 35], VSV [36, 37], EBOLA [38, 39], HIV [40], JEV [41], CHIKV [42], SARS-CoV-1 [43], and recently against SARS-CoV-2 [35, 44, 45]. Additionally, many studies have been carried out that propose various targets for blocking virus recognition, importation and replication processes through the use of reused drugs, immunotherapies, interference strategies and various inhibitory compounds [46]. But despite the large number of experimental, biophysical, and computational studies that have explored all of these alternatives, to date, there is no specifically confirmed effective treatment available for SARS-CoV-2 [46, 47]. However, important advances have been made in the study of potential compounds targeting the interface between the ACE2 and the receptor binding domain (RBD) of SARS-CoV-2 to prevent cellular recognition of virus [48–50]. Why the RBD domain is known to be part of the SARS-CoV-2 spike receptor with higher affinity for the ACE2 enzyme, a key interaction for infection to establish [48].
Therefore, the theoretical study of candidate compounds for docking and perturbation of the RBD-ACE2 interface could theoretically contribute to this approach in the research and development of COVID-19 treatment alternatives. In this sense, and based on what has been previously described, we propose to perform a computational biophysical characterization of interaction of various NSAID-type compounds at the RBD-ACE2 complex biointerface and the perturbation energy-conformational induce by these drugs on this complex in order to obtain information about possible inhibitory mechanistic routes of these drugs against the SARS-CoV-2 virus, especially since these drugs are not banned to date by the WHO for the symptomatic treatment of COVID-19.
Materials and Methods
Search for Structures in Databases
In this study, the crystal structure of ACE2 and RBD of SARS-CoV-2 (PDB: 6M0J) were considered and obtained from the RCSB protein database (https://www.rcsb.org/). The simulations were carried out using as a target, the entire region of the RBD domain which is bound to the ACE2 receptor by means of 15 residues. Specifically, the amino acids that interact at the ACE2 and RBD interface are in total 15 residues of ACE2 that interact with RBD: these are residues 24 (Q), 27 (T), 30 (D), 31 (K), 34 (H), 35 (E), 37 (E), 38 (D), 41 (Y) and 42 (Q) that are in α1, a residue (residue 82 M) that comes from α2 and residues 353 (K), 354 (G), 355 (D) and 357 (R) that come from the linker between β3 and β4, as reported [49, 50]. At least one member from each of the commonly marketed NSAID types was chosen (see structure of these drugs in Supplementary Figure S1). The 2D structures of the compounds Aspirin_CID_2244 (Salicylates), Celecoxib_CID_2662 (Selective COX-2 inhibitors “coxibs”), Benzydamine_CID_12555 (Indazoles), Metamizole_CID_3111 (Pyrazolone derivate), Diclofenac_CID_3033 (Acetic acid derivatives), Ibuprofen_CID_3672 (Propionic acid derivatives), Indomethacin_CID_3715 (Acetic acid derivatives), Ketoprofen_CID_3825 (Propionic acid derivatives), Meloxicam_CID_54677470 (Enolic acid “oxicam” cSulfonanilides) and Acetaminophen_CID_1983 (p-aminophenol derivative), were obtained from PubChem (https://pubchem.ncbi.nlm.nih.gov/) in SDF format, and the SMILES online converter was used (https://cactus.nci.nih.gov/translate/) to get a PDB format. Acetaminophen is generally not considered an NSAID because it has only minor anti-inflammatory activity but was included as recommended by WHO as part of standard treatment [30, 51]. The Molinspiration server (https://www.molinspiration.com/) was used for the bioactivity calculations (http://www.molinspiration.com/cgi-bin/properties) and tools the Molecular Modeling Group of the Swiss Institute of Bioinformatics [52, 533].
Molecular Docking
To simulate ligand-protein binding the complexes were constructed in DockThor program using the flexibility algorithm, blind docking and calculating the DockT scoring function. To increase accuracy 30 runs were made with 106 evaluations per run. As is usually done, all the water molecules were removed and the PDB files were separated into two different files, one containing the protein and the other containing the ligand structure [54]. The DockThor program is freely available as a Web server (https://dockthor.lncc.br/v2/), which provides to the user the main steps for protein and ligand preparation with PdbThorBox and MMFFLigand and the analyses of the results using DTStatistics. The Web server utilizes the computational facilities of the Brazilian high-performance platform (SINAPAD, https://www.lncc.br/sinapad/) and the supercomputer SDumont (https://sdumont.lncc.br/). The most favored position in the biointerface was analyzed with MMV_2019_7.0.0, calculating the MolDock, Rerank and PLANTS scoring functions [55, 56]. Only the three runs with the most favorable berth were considered in the sampling of the probabilistically most feasible and thermodynamically most favorable positions in the biointerface. This criterion was used to discriminate the complexes that would be subjected to further analysis, including molecular dynamics.
Potential Theoretical Inhibition
The AutoDock Vina (ADV) algorithm estimates the binding constant K from the binding free energy
And the inhibition constant for binding of ligand to proteins (Ki) (in units of M) is obtained as,
where, R is the universal gas constant (1.987 cal/K mol), T is the absolute temperature (298.15 K). The ΔG value used was derived from the mean of the ligand protein binding free energy predicted by each scoring function considered in this study. The equation establishes that Ki can be equaled to the dissociation constant (Kd) of the protein - inhibitor complex. Therefore, Ki and Kd they are equivalent definitions, since Kd is used to describe the strength with which an inhibitor dissociates from the protein, which is equivalent to the strength that the inhibitor would have to inhibit the protein. In this sense, the higher the value of Ki, the weaker the binding of the inhibitor to the protein, and therefore, the protein - inhibitor complex dissociates more easily [57–62]. In our research we assume a competitive inhibition, which is the most extreme case (maximum inhibition among several possible inhibition mechanisms), in which the Ki value can be converted to the Kd value and IC50 (total concentration of inhibitor that reduces these activities by 50%) with the ADV algorithm and following the considerations in the case of competitive inhibition with the equation,
Where P50, is the protein concentration at 50% inhibition; Kd, is the affinity constant of the ligand to the RBD-ACE2 interface; [PL50], is the protein concentration by ligand concentration at 50% inhibition; and L50, is the ligand concentration at 50% inhibition. Another very useful parameter is PI50, which is defined as the concentration of protein-inhibitor complex at 50% inhibition and can be estimated using the equation,
Here IC50, total inhibitor concentration that reduces these activities by 50%; I50 is the inhibitor concentration at 50%. As a positive control for the comparative analysis, concentration of the free protein at 0% inhibition was predicted with the equation,
Where L and P is the ligand concentration and the protein concentration at 0% inhibition, respectively. The IC50-to-Ki web tool was used for all these calculations (https://bioinfo-abcc.ncifcrf.gov/IC50_Ki_Converter/index.php). This tool helps to measure the quality of the parameters used in the calculations of enzyme inhibitors and of binding reactions between macromolecules and ligands that depend on the type of mechanism of action of the inhibitor and the concentrations of the interacting molecular species [63–66].
Molecular Dynamics
Simulations were performed for a docking coup with three purposes: 1) study the relative stability of the ligand residing in the binding pocket; 2) sampling the minimum energy conformations to calculate the disturbance of the thermodynamic and structural stability of the complexes; 3) analyze the pockets distribution and structural deformation coefficients in the minimum energy conformations. For a protein-ligand complex, the MD system was first relaxed through a series of minimization procedures. There were three phases for a MD simulation: the relaxation phase, the equilibrium phase, and the sampling phase, as recommended [67–70]. The MD simulation of the crystal structures was carried out in an explicit water system. Specifically, the solvation of the system was carried out in a solvation box of 8.0 Å. With an Ion-mol/water-mol ratio close to 1 g/cc (equivalent to a molar density of Na+/Cl– = 0.003) to simulate an aqueous medium under physiological conditions. Under these conditions the quantity of water was fixed in 23,886 molecules. Our MD system also consisted of one copy of RBD-ACE2 and one copy of the coupling ligand. An Amber99SB-ILDN force field was applied to the complex, with TIP3P water model. The whole system was neutralized. All model water molecules were treated as rigid bodies, thus allowing a simulation time step of 2 fs. Periodic boundary conditions were applied, and Berendsen’s algorithm for temperature and pressure coupling was adopted. After a first steepest descent to 5,000 steps and conjugated gradient to 5,000 steps energy minimizations with positional restraints on the solute, an initial 100 ps simulation was carried out with the positions of the solute atoms restrained by a force constant of 10 kcal/(mol Å2) to let the water diffuse around the molecule and for equilibration. The method PME was used to calculate the electrostatic contribution to nonbonded interactions with a cutoff of 14.0 Å and a time step of 1 fs. The cutoff distance of the van der Waals interaction was 14.0 Å. After this equilibration run, the NVT production run (100 ns) at 300 K was performed with the cell size remaining the same. The SHAKE algorithm was applied to the system, and the time step was set to 2 fs. Five Snapshot structures were obtained at every 25 ns as the target structures extracted from a trajectory of 100 ns. All MD simulations and the additional settings were performed by using cosgene/myPresto [68–70]. Flexibility and deviation from the initial structure of molecular dynamics was estimated using root mean square deviation (RMSD) from sampling the minimum energy conformations by using cosgene/myPresto. The pair of residues that came into contact with the ligands of interest was considered to determine the folding/unfolding compared to the native structure. The residues were chosen arbitrarily if they are at a distance of ≤6 Å from the ligands. For RMSD calculations, the equation,
Where δi is the distance between atom i and either a reference structure or the mean position of the n equivalent atoms. Additionally, it was compared with the ANM model (Anisotropic Network Model Web Server 2.1) (http://anm.csb.pitt.edu/) to analyze the vibrational movements of the molecular systems built using the Elastic Network (EN) methodology. The network includes all interactions within a cutoff distance, which is the only default parameter in the model. Information about the orientation of each interaction with respect to the global coordinate system is considered within the constant Force matrix and allows the prediction of anisotropic movements. The model was applied to explore the impact of docking on the MD of the protein complex. For protein complexes the default nodes suggested by the server (alpha carbon (Cα) for amino acids) were used. Ligands were explicitly included in the ANM analysis. These were present in the PDB coordinate file, so that their atoms are analyzed and automatically enumerated. Specifically, the structural fluctuation of the scalar type and the root mean square fluctuation (RMSF) were calculated [71]. The ProSA (Protein Structure Analysis) program was also used because it is an established tool for refining and validating experimental protein structures and for predicting and modeling structures. In order to obtain the visualization of scores (Z-scores) and energy graphs that highlight potential variations in the protein structure. In particular, the quality scores of a protein are displayed in the context of a database of known protein structures. To validate the structural disturbance of the protein of interest compared to native models obtained from X-ray analysis, NMR spectroscopy, and theoretical calculations. ProSA-web is accessible at https://prosa.services.came.sbg.ac.at [72, 73].
Analysis of Changes Induced in the Distribution of Pockets of Protein Complex by Binding of Ligand
The PockDrug-Server (http://pockdrug.rpbs.univ-paris-diderot.fr/cgi-bin/index.py?page=Home) site was used to predict the pharmacological characteristics of the pockets, as well as the variations of the pockets after the conformational changes and structural disturbances that the protein complex docking to the ligand may undergo. PockDrug-Server provides consistent and reproducible results using different pocket estimation methods. For this, the Fpocket method was applied, which performs a predicted pocket estimate based on a preliminary detection of all the cavities that can, for example, bind a ligand of sufficient size, but without information on the proximity of the ligand, based on the decomposition of a protein structure in the Voronoi polyhedra. It is robust with respect to pocket limits and estimation uncertainties, therefore it is efficient in using pockets that are difficult to estimate [74]. The AlloSite server (http://mdl.shsmu.edu.cn/AST/Allosite/index.jsp) was used and the AlloSitePro method was applied, which designates a score derived from a logistic regression model, which is based on the topological and physicochemical characteristics of the predicted allosteric site [75].
Results
The results of the molecular docking relative free energies of binding obtained between the various ligands from the group of NSAIDs and the protein complex formed by the Spike Receptor-Binding Domain (RBD) of SARS-CoV-2 bound with ACE2 (RBD- ACE2) are shown in Table 1. The energies were calculated with four scoring functions from the thermodynamically most favorable positions predicted by the sampling algorithm considered. All NSAIDs were thermodynamically bound to the RBD-ACE2 complex. All MolDock bindings showed an average energy of –48.981 kcal/mol, where the minimum energy was exhibited by Ketoprofen_CID_3825 (−22.837 kcal/mol) and the maximum predicted for Celecoxib_CID_2662 (–87.625 kcal/mol). Results coincided with Rerank energies, which average docking energy was –42.985 kcal/mol, with a minimum of –22.339 kcal/mol and a maximum of –71.215 kcal/mol, in the same way for Ketoprofen and Celecoxib, respectively. Furthermore, the average energy of binding according to the scoring function PLANTS was –38.457 kcal/mol, with a minimum of –27.662 kcal/mol for Acetaminophen_CID_1983 and a maximum of –58.674 kcal/mol for Benzydamine_CID_12555. Results that coincide with the position of the ligands with the minimum and maximum energies predicted by the DockT scoring function, specifically for the Acetaminophen and Benzydamine ligands, with energies of –6.323 and –8.370 kcal/mol, respectively, with an average docking energy of –7.163 kcal/mol. Ibuprofen being the NSAID with the most favored thermodynamically docking energy at the interface of the RBD-ACE2 complex, followed by Aspirin and Acetaminophen (see Figure 1).
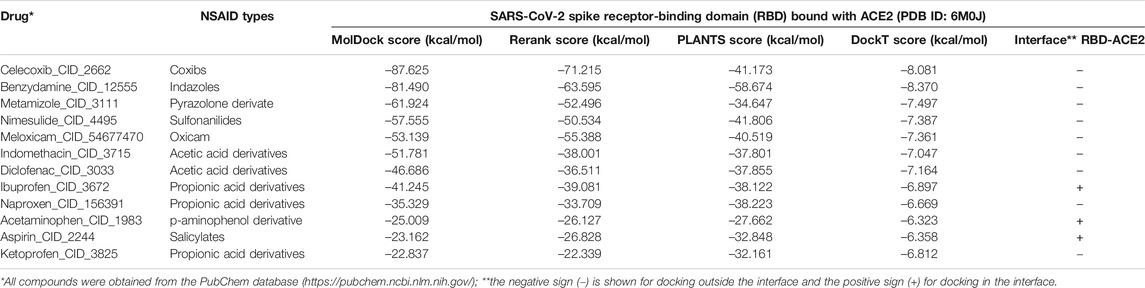
TABLE 1. Results of the binding energies of the NSAIDs considered in this study with the SARS-CoV-2 Spike Receptor-Binding Domain (RBD) complex bound with ACE2 (PDB ID: 6M0J).
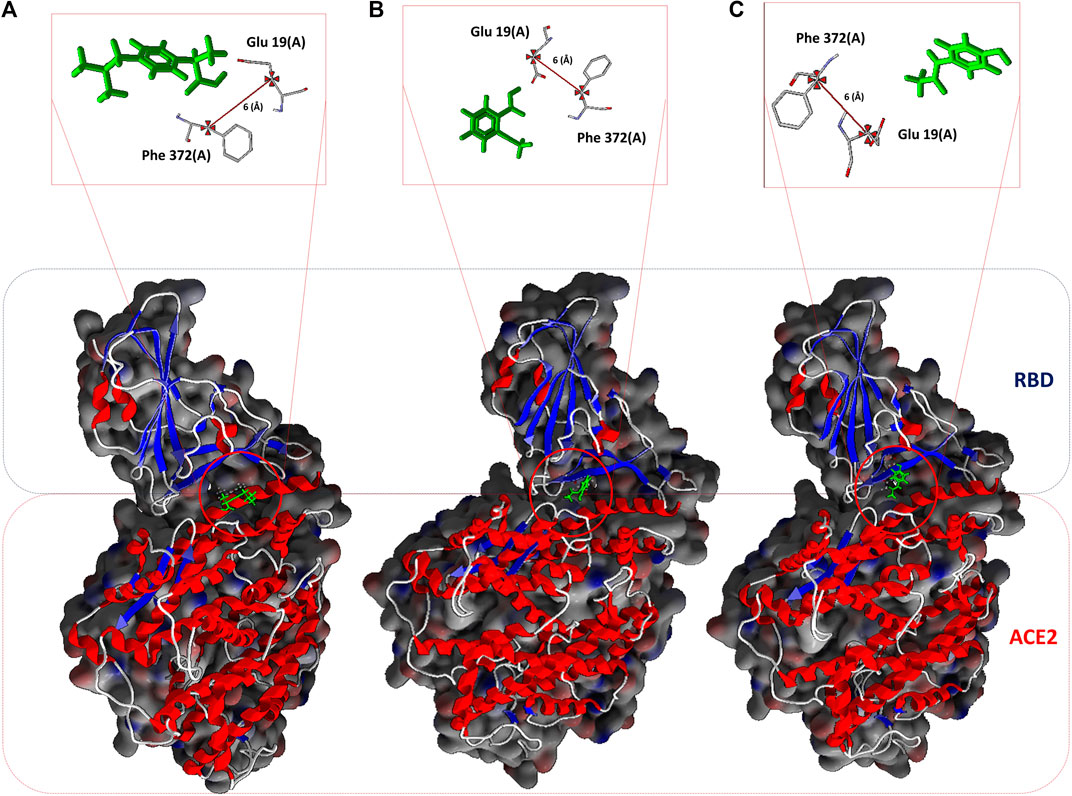
FIGURE 1. NSAIDs docking at the interface of the RBD-ACE2 protein complex. (A) Ibuprofen_CID_3672, (B) Aspirin_CID_2244, and (C) Acetaminophen_CID_1983. In the upper part, the arbitrarily chosen residuals for the subsequent analysis of molecular dynamic are observed, as they are involved in the interaction and at a distance of 6 Å from each other.
All NSAIDs exhibited favorable binding energies with the ACE2 receptor. The considered model of the Coxibs group (Celecoxib) and the compound derived from Indazoles (Benzydamine) being the most theoretically most probable and strong binding. Only 3/12 (25%) of the compounds considered in this study had a thermodynamically favorable docking with the interface of the RBD-ACE2 complex. Specifically, the compound Propionic acid derivatives Ibuprofen_CID_3672, the Salicylate Aspirin_CID_2244 model, and the p-aminophenol derivative Acetaminophen_CID_1983. Which do not present any violation of Lipinski’s rules (see Table 2). The predicted mean docking for these compounds at the interface was –31.336 kcal/mol (Ibuprofen), –22.299 kcal/mol (Aspirin) and –21.280 kcal/mol (Acetominophen), respectively. Specifically, the MolDock, Rerank, PLANTS, and DockT scoring functions predicted for Ibuprofen the binding energies of –41.245, –39.081, –38.122, and –6.897 kcal/mol, respectively. All scoring functions in each case predicted a more favorable interface docking for Ibuprofen.
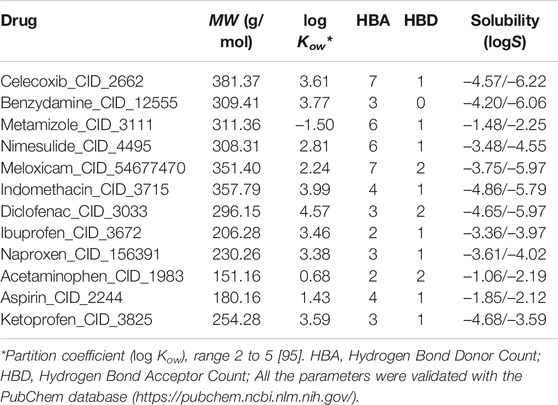
TABLE 2. Characteristics considered associated with the bioactivity and solubility of ligands using the Molinspiration server and the tools of the SIB Molecular Modeling Group | Swiss Institute of Bioinformatics.
The inhibition constant derived from the dissociation model that was applied, using the ADV algorithm for the binding of NSAIDs to the interface of the RBD-ACE2 protein complex is shown in Table 3 in their order of decreasing potency. Also, their thermodynamically favorable medium docking to the interface are also shown. Results that correspond to the amount of free protein assuming the RBD-ACE2 complex at 0 and 50% of theoretical inhibition calculated after predicting the minimum and maximum mean inhibitory concentration, and which favored Ibuprofen with a P0 and P50 of 0.830 and 0.291 M, respectively, followed by 1.100 and 0.355 M for Aspirin, and 1.660 and 0.456 M for Acetaminophen, correspondingly. This allows predicting a possible inhibitory concentration-dependent mechanism of these NSAIDs on the RBD-ACE2 interface in which less Ibuprofen concentration is required to cause some type of competitive antagonism by the region of union between RBD and ACE2. An observation consistent with the theoretical inhibition according to the PI50 and that, although it is very close among the NSAIDs linked to the interface, it also favored Ibuprofen (48.696 M) followed by Aspirin (48.769 M) and Acetaminophen (48.952 M) (see Table 3).
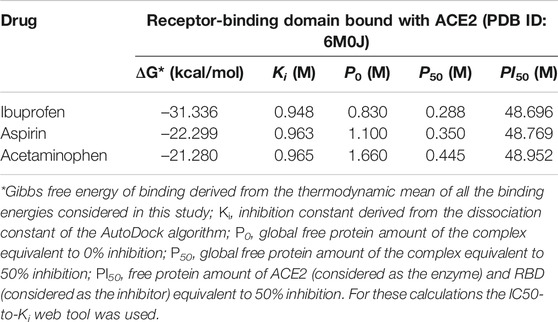
TABLE 3. Results of the amount of free protein assuming a competitive inhibition of the RBD-ACE2 complex in the presence of the NSAIDs bound to the interface.
By studying the theoretical effect of the molecular docking of each of these compounds at the interface, but as a function of time, and based on a simulation of 100 ns molecular dynamics, we found that Ibuprofen was the NSAIDs with the thermodynamically most docking favorable, and it was also the one that achieved the most favorable binding energy in the shortest time of the simulation (see Table 4). This being –47.470 kcal/mol at 75 ns, while aspirin required 100 ns to obtain its most favorable docking (51.711 kcal/mol). Although Acetaminophen achieved its best binding energy at 75 ns, it was the lowest of all the energies calculated in the most optimal simulation period for each docking (–28.634 kcal/mol). Results that correspond in each case with the Ki constants predicted in the same time periods, with 0.922 M being the most optimal for Ibuprofen in the shortest time (75 ns) with respect to the other two compounds. Although the Aspirin Ki was more favorable (0.916 M), it required 100 ns of simulation to obtain this interaction. Once again, Acetaminophen presented its most optimal value for this variable in a time similar to that of Ibuprofen, but with the least favorable Ki of all (0.953 M). In terms of the amount of theoretical free protein predicted by the P0, P50 and PI50 functions, after the docking and relative to the simulation time, we observed that Ibuprofen was able to inhibit the largest amount of complex in any of the conditions, regardless of the points sampled in the simulation used for the prediction and with respect to the rest of the compounds, with a P0, P50 and PI50, of 0.807, 0.280, and 48.693 M, respectively. While for Aspirin the most optimal values were 1.047, 0.333 and 48.760 M; and for Acetaminophen the values are 1.635, 0.440, and 48.947 M, correspondingly. Interesting results because the difference between the amount of theoretical free protein predicted by the P0, P50 and PI50 functions favors Ibuprofen over Aspirin with values of 0.240, 0.053, and 0.067 M; and against Acetaminophen with a difference in favor of Ibuprofen of 0.828, 0.160 and 0.254 M, respectively (see Table 4).
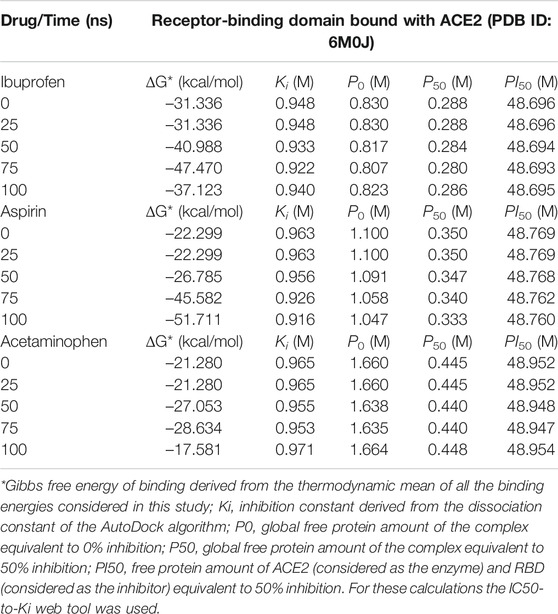
TABLE 4. Results of the binding energy and amount of free protein assuming a competitive inhibition of the RBD-ACE2 complex in the presence of the NSAIDs bound to the interface and as a function of the simulation time.
Interestingly, the three NSAIDs initially docking at the interface established interactions in the same cavity with almost the same number and type of residues of the A chain correspond to the enzyme protein ACE2 and the B chain to the RBD. Ibuprofen and Aspirin coincided in the primary interaction with 7/11 (64%) of the residues predicted in the docking, these being His16 (A), Glu19 (A), Arg71 (B), Tyr173 (B), Ala369 (A), Gln370 (A) and Arg375 (A). While Acetaminophen did so only with 5/11 (45%) of the same residues, except initially with Glu19 (A) and Gln370 (A). Aspirin and Acetaminophen coincided exclusively in the initial docking with other 4/11 (36%) residues other than those predicted in the docking with Ibuprofen, these being Asp73 (B), Glu74 (B), Lys85 (B) and Ala368 (A). The interactions between Aspirin and Acetaminophen being the most similar to each other, while Ibuprofen establishes a greater number of unique interactions, with specifically 4/11 (36%) different residues (see Tables 5–7). Although all compounds showed to induced a change in the native arrangement of the residues, 82% of Ibuprofen interactions were oriented toward the ACE2 protein, followed by Aspirin with 55%, and for Acetaminophen it was 36%. Aspirin was the compound that established the highest number of hydrogen bridges at the interface (36%), followed by Acetaminophen (27%). The greatest number of Ibuprofen interactions were steric (91%), followed by Acetaminophen (72%). These interactions underwent variations throughout the simulation as predicted also with the PockDrug-Server, reflected in the decrease of the hydrophobicity ratio of the associated cavity from –1.40 of the native structure to –1.84, –2.35 and –2.69 in presence of Aspirin, Ibuprofen and Acetaminophen, respectively. Also increasing the number of polar residues with respect to the native structure from 57% to approximately 80%, and aromatic residues from 14% in the native structure to approximately 20% (see Tables 5–7).
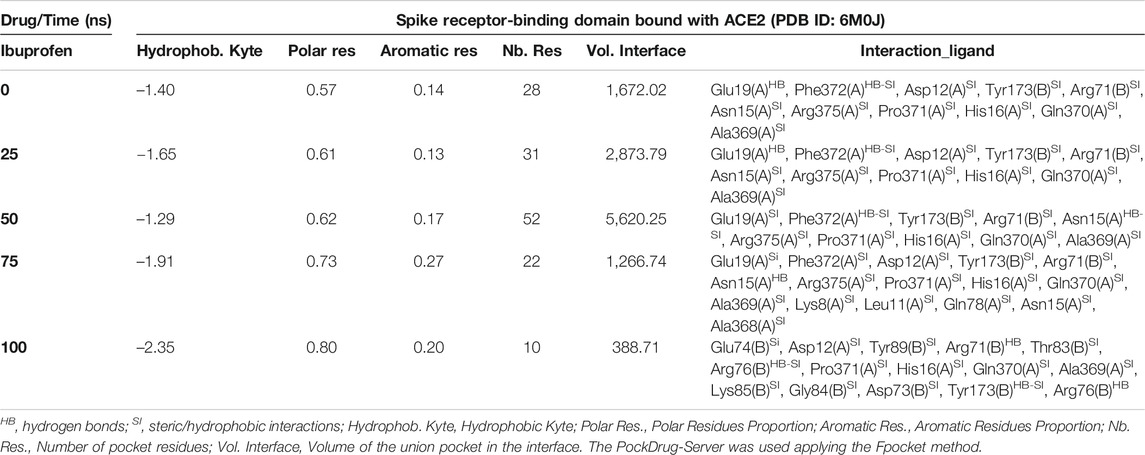
TABLE 5. Biophysical characteristics associated with the amino acid composition of the Ibuprofen binding pocket at the RBD-ACE2 interface and as a function of simulation time.
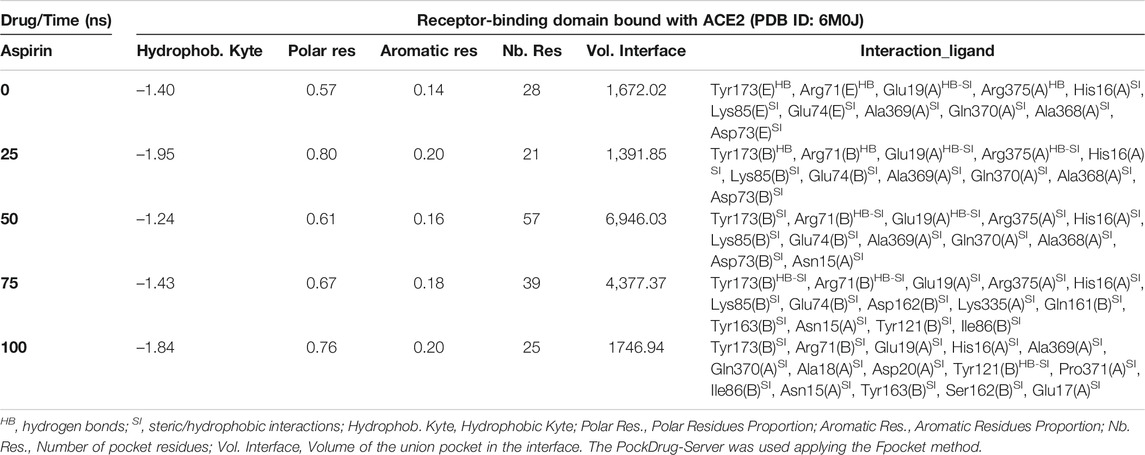
TABLE 6. Biophysical characteristics associated with the amino acid composition of the Aspirin binding pocket at the RBD-ACE2 interface and as a function of simulation time.
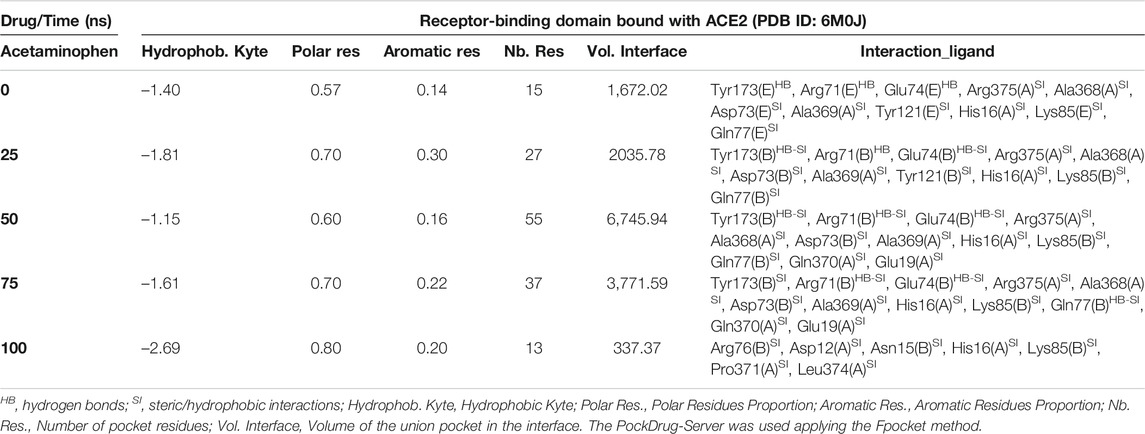
TABLE 7. Biophysical characteristics associated with the amino acid composition of the Acetaminophen binding pocket at the RBD-ACE2 interface and as a function of simulation time.
In fact, Ibuprofen was the only one of the three NSAIDs that presented a greater migration of interactions toward the viral region in our complete simulation cycle, being the only one of these compounds that established twice as many hydrogen bridges during the disruption of its binding pocket, going from 2 to 4. While Aspirin decreased from 4 to 1, and Acetaminophen from 3 to 0 (see Tables 5–7). At the end of the complete simulation cycle, Ibuprofen went from having 80% of its interactions oriented to the ACE2 region to establishing more than 67% of global interactions in the RBD virus region. In contrast, Acetaminophen went from having 64% interactions in the viral region to establishing 57% toward the ACE2 region. While Aspirin went from 55% of interactions oriented to ACE2 to 60% but also in the same region of the complex. Indicative that Aspirin stabilizes its interactions strongly only with the ACE2 region, and that Acetaminophen may lose most of the interactions initially targeting the viral region to have a more stable binding with ACE2.
At the end of the simulation, all ligands altered the volume of the predicted binding cavity for each at the interface (see Figure 2), represented by increases of up to approximately 6,900 Å3 at 50 ns and a decrease of approximately 300 Å3 of the joint pocket at 100 ns with respect to the native cavity. Ibuprofen being the compound that caused the greatest disturbance in terms of decrease in pocket volume within the interface in the shortest time of the simulation, with an approximate volume of 1,200 Å3 at 75 ns, followed by a final decrease at 100 ns of about 300 Å3. In contrast, Aspirin and Acetaminophen induced an increase in the volume of their cavity in the same time period of 75 ns of simulation between 3,700 and 4,300 Å3, Acetaminophen achieved a final decrease at 100 ns similar to Ibuprofen. Only Ibuprofen showed a consistent decrease in the simulation time of the volume of its binding pocket, after the predicted folding in all cases to 50 ns.
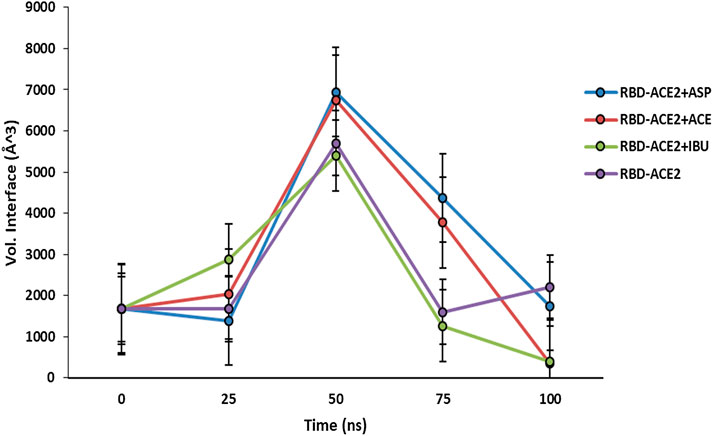
FIGURE 2. Fluctuation of volume of cavity in the interface RBD-ACE2 protein complex in presence of Ibuprofen (RBD-ACE2+IBU), Aspirin (RBD-ACE2+ASP) and Acetaminophen (RBD-ACE2+ACE) at 100 ns. As a control, RBD-ACE2 protein complex without ligands was simulated (RBD-ACE2). Vol. Interface, Volume of the union pocket in the interface. The PockDrug-Server was used applying the Fpocket method.
Both Aspirin and Acetaminophen showed fluctuations in the volume distribution of the cavity located at the interface (see Tables 5–7 and Figure 2). We also examined the main cavities predicted for the complex, which were not always the binding pocket of each ligand, precisely because the volumetric variation of the pockets in each state sampled during simulation is one of the important parameters in the classification of each cavity as main or not. We only considered the three main cavities, finding that for the three NSAIDs the mean volume of the cavity throughout the simulation was approximately 800 Å3 (see Table 8). The algorithm AllositePro predicted that these three NSAIDs bind potentially allosteric regions for RBD-ACE2 system. Furthermore, the structural and conformational changes at the level of the interface that these compounds could induce in the native structure of the complex, are interesting because the AllositePro predicted that these three NSAIDs bind potentially allosteric regions for RBD-ACE2 system. Specifically, during the first 25 ns of simulation Ibuprofen, Aspirin, and Acetaminophen bind to a potentially allosteric region with a solvent-accessible total surface area (SASA) of approximately 1,000 Å2 for Ibuprofen, and approximately 600 Å2 both for Aspirin as Acetaminophen (Table 9).
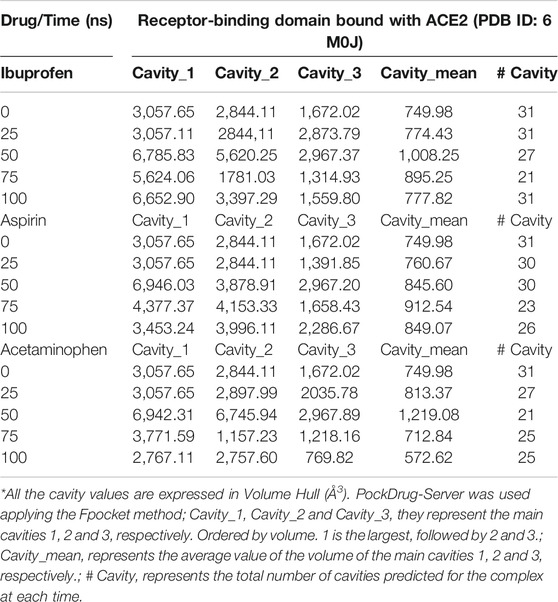
TABLE 8. Distribution of main cavities of the RBD-ACE2 complex in the presence of NSAIDs and as a function of the simulation time.
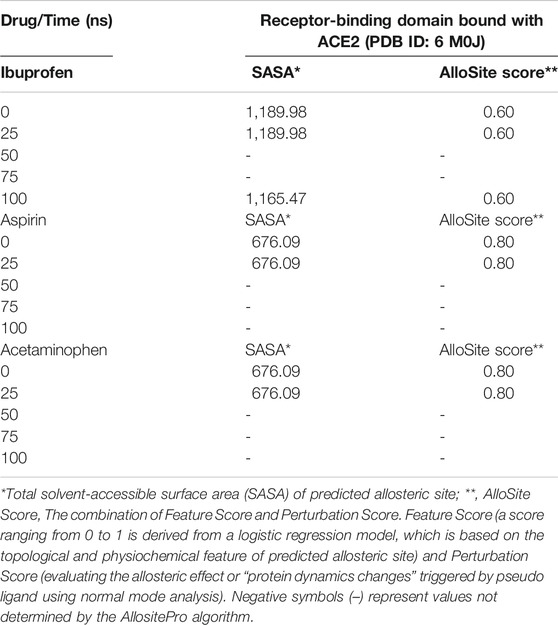
TABLE 9. Prediction of potential allosteric sites in the ligand binding pockets at the interface of the RBD-ACE2 complex as a function of simulation time.
Variations in the thermodynamic stability predicted with the myPresto package showed that all three compounds altered the thermodynamic stability of the complex as a function of time. The structural variations predicted using the algorithms of the ProSA tools, favored Ibuprofen with an optimal Z-score for the disturbance of –8.010 at 100 ns and that represents a difference with respect to the native structure of approximately –1.400. While the difference in terms of variations in the native structure after Aspirin docking was –1.305 as a result of a Z-score of –8.075 at 100 ns, and for Acetaminophen the Z-score was –8.430, representing a difference from –0.950 to 100 ns. The difference between the Z-score of Ibuprofen with Aspirin is –0.065, and with respect to Acetaminophen –0.420. Furthermore, this structural disturbance was calculated and validated with the ANM model, and coincides with ProSA, allowing us to predict that the greatest structural disturbance with the scalar distance model was 0.600 theoretically induced by Ibuprofen, followed by 0.460 for Aspirin and 0.400 for Acetaminophen. Relative to the native scalar dynamics value of 0.300 (see Tables 10 and 11).
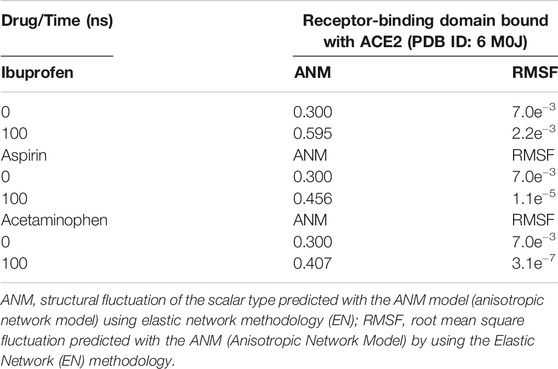
TABLE 11. Structural fluctuation of the RBD-ACE2 complex in the presence of NSAIDs at 100 ns using Anisotropic Network Methodology (ANM).
Ibuprofen was the only one of the ligands that was able to maintain a predictable binding with the possible allosteric site after 100 ns of simulation regardless of the induced structural and conformational disturbances (see Table 9). Variations in the aminoacid composition of the residues involved in the binding pockets, and in the interactions established by these with the ligands throughout the simulation time and with respect to the native structure, were also predicted. All compounds induced a change in the native arrangement of the residues corresponding to their binding pockets over time. Specifically, Ibuprofen increased the number of steric interactions throughout the simulation from 10 to 14, and Aspirin from 9 to 15. Unlike Acetaminophen, which exhibited a decrease in the number of such interactions from 10 to 7. This also explains the variations in the relative binding energies at each sampling point. Specifically, Ibuprofen was the NSAID that achieved the lowest energy structure (thermodynamically more stable) by finalizing the simulation with about –8,000 kcal/mol. While the minimum energy induced by Aspirin was –7,900 kcal/mol and by Acetaminophen –7,800 kcal/mol in the same time period. One of the lowest energy steppes reached by Ibuprofen was at 50 ns with –7,800 kcal/mol, the same reached by Acetaminophen but in a longer period of time. The complex in presence of Aspirin reached its minimum energy at 50 ns, inducing a thermodynamically more stable structure than Ibuprofen only at that point (see Table 10 and Figure 3).
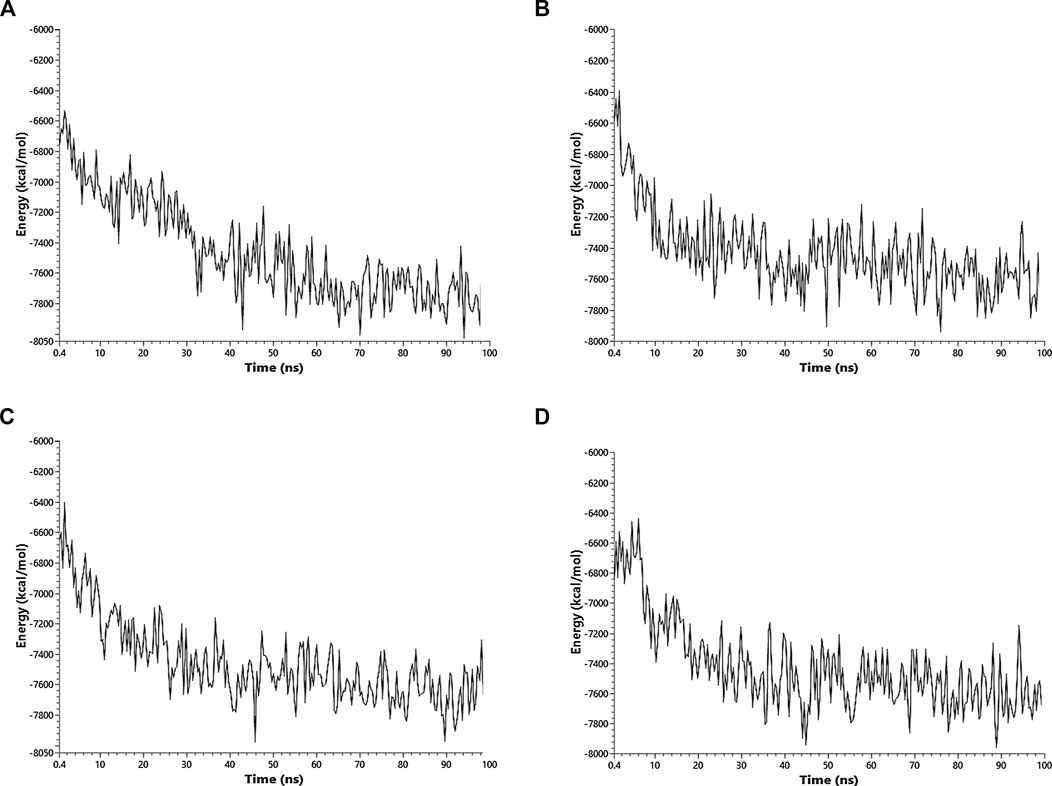
FIGURE 3. Fluctuation of the thermodynamic stability of the RBD-ACE2 complex in the presence of Ibuprofen_CID_3672 (A), Aspirin_CID_2244 (B), Acetaminophen_CID_1983 (C) and the RBD-ACE2 protein complex without ligands (D) was used as a control. Everything was simulated to 100 ns
All the results previously described are related to the structural disturbance using as a reference the pair of residues Glu19 (A) and Phe372 (A), arbitrarily chosen to be close to or involved in interaction with ligands at some point in the docking over time (see Figure 1). We found that Ibuprofen was the only one of the three NSAIDs that could be able to induce a folding in the structure, as determined with the analysis of the volume and distribution of cavities, and as predicted by ProSA and the ANM model. Causing a maximum folding of approximately 13% at 40 ns with respect to the native state and stayed around that value until 100 ns. Followed by Aspirin which induced folding of approximately 5% at 80 ns. Acetaminophen was the only one of the three compounds that did not mediate a folding of the structure, however, it caused a maximum unfolding of approximately 8% at 80 ns. In all cases the results were statistically significant (p < 0.001) (see Table 10 and Figure 4). This folding induced by Ibuprofen reduce the global flexibility (note in Figure 4 the structural flexibility of RBD-ACE2 complex without ligand), which is associate too with the diminution of the size of internal cavities and cavity volume of potentially allosteric region of complex RBD-ACE2. Thus, this conformational change induced stiffness, which has an important effect no favorable upon the energetic of interaction between local aminoacid residues in the potentially allosteric region of RBD-ACE2 complex due to binding of Ibuprofen, which blocks those interactions (see Tables 5–7). Promising results suggesting that Ibuprofen could alter the stability of the RBD-ACE2 complex and affect its formation. Which could compromise the viral infection. A possible alternative mechanism of action that would support the use of this NSAID in the treatment of COVID-19.
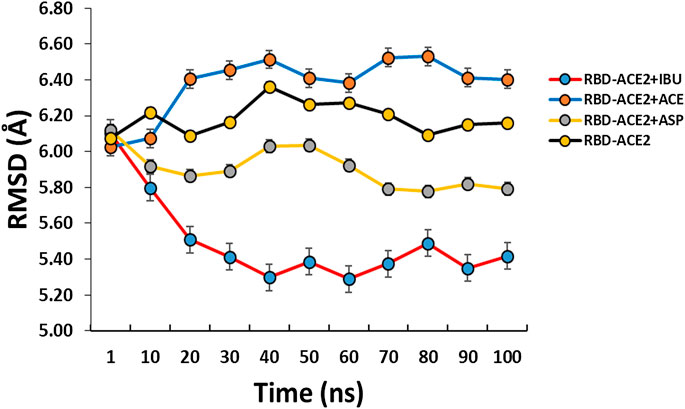
FIGURE 4. Conformational fluctuation and partial unfolding or folding of RBD-ACE2 protein complex in presence of Ibuprofen (RBD-ACE2+IBU), Aspirin (RBD-ACE2+ASP) and Acetaminophen (RBD-ACE2+ACE) at 100 ns. As a control, RBD-ACE2 protein complex without ligands was simulated (RBD-ACE2). Using as a reference the distances between the residues of Glu19(A) and Phe372(A). Arbitrarily chosen because they are at a distance of approximately 6 Å from the ligands.
Discussion
All NSAIDs exhibited favorable binding energies with the ACE2 receptor. Interestingly, Propionic acid derivatives, Salicylates and p-aminophenol derivative exhibited the least favorable binding energy with the ACE2 receptor of the protein complex, but at the same time they presented the most favorable energies for binding to the interface, and with the most probabilistically feasible docking. This is reflected by the fact that these three compounds were favorably sampled at the interface after the reclassified run in each case as the most thermodynamically feasible, but under the sampling conditions of this study (see Table 1 and Figure 1). Although this is the first study that considers the docking of this type of compound at the level of the RBD-ACE2 interface, our results coincide with those obtained by other authors who have found that Ibuprofen can have a docking score considerably better than other NSAIDs compared to proteins associated with SARS-CoV-2 [44], and that some derivatives could effectively interact with protein regions involved in this complex [76].
These NSAIDs drugs exhibited very close Ki. However, Ibuprofen showed the most favorable Ki, followed by Aspirin and Acetaminophen. It is important to note that the Ki, P0, P50 and PI50 values are theoretical predictions calculated to obtain only a rough estimate of the binding affinity and functional strength of the NSAIDs considered in this study, assuming that they have an inhibitory type behavior competitive (see Tables 3 and 4). On the basis that the thermodynamically favorable and probabilistically most feasible docking of these compounds occurs at the interface, which could theoretically compromise the binding of the Spike RBD with ACE2 in a competitive way, as has been described with other compounds capable of binding to the interface [49]. But caution should be exercised in extrapolating these data because these values, especially the experimental Ki (from which the values of P0, P50, and PI50 are derived) tend to be different from the resulting theoretical values for various biophysical parameters involved in enzyme inhibition. In this sense, these parameters should only be considered to designate the probability that any of these particular compounds may have the potential to disturb the binding between the two proteins of interest. Interestingly, these three NSAIDs are among the most studied [77, 78]. Especially since it has been described that Ibuprofen may be a potential inducer of oxidative stress as a result of said intercalation capacity at the biomolecular level [79]. However, although other NSAIDs with antagonistic activity against SARS-CoV-2 with lower inhibitory concentrations have been reported than the one obtained in this study, they are not directed to the RBD-ACE2 interface [80, 81]. Furthermore, the inhibitory activity of Ibuprofen for other unrelated viruses has been reported to be concentration dependent and may require up to 0.500 M [24]. Additionally, it has been pointed out that in the case of NSAIDs the molecular docking values can be much closer to the selectivity through energy terms, but not to the IC50, and it has been indicated that for these compounds the molecular docking represents the descriptor most important for drug-protein interactions [82].
Interestingly, the three NSAIDs initially docking at the interface established interactions in the same cavity with almost the same number and type of residues. These results indication that the residues His16 (A), Glu19 (A), Arg71 (B), Tyr173 (B), Ala369 (A), Gln370 (A) and Arg375 (A) are key in the initial interaction of these compounds on the interface, where 5/7 of them bound to residues correspond to the enzyme protein ACE2 and 2/7 to the RBD (see Tables 5–7). An interesting result because despite being NSAIDs from different groups they share chemical characteristics for this binding. Moreover, one of the few reported compounds capable of interacting with the interface RBD-ACE2 did not share any of the residues involved here [49, 50].
Although all compounds showed to induced a change in the native arrangement of the residues, Ibuprofen indicates a greater binding strength at the interface in the direction of the ACE2 protein, while Aspirin and Acetaminophen established a greater number of interactions toward the viral region. The differences in docking energies and in the inhibitory potentiality of these compounds, and which theoretically favors Ibuprofen, may be associated with the indirect structural and conformational changes that these compounds might be able to induce in the complex after docking in the interface, a phenomenon that could be evidenced with ProSA tools. In fact, we found that Ibuprofen was the only one of the three NSAIDs that increased hydrogen bridges during the disruption of its binding pocket. This is important because each hydrogen bridge can contribute about 2 kcal/mol to the interaction energy, therefore these results are indicative of a more stable interaction of Ibuprofen throughout the simulation, at the same time that this molecule could be capable to induce a disturbance in the native structure without losing its thermodynamically favorable docking. It appears that Acetaminophen is a chemical structure associated with NSAIDs with limited interaction capacity as found in other unrelated docking studies in which it has also been outperformed by Propionic acid derivatives and Salicylates in terms of Gibbs free energy of binding [83]. Also, results in terms of variations in the native structure after Aspirin docking, indicates that Ibuprofen could potentially induce the greatest structural disturbance to the complex once docking at the interface, followed by aspirin, and to a lesser extent Acetaminophen in terms of Z-score prediction and according to the knowledge-based energy function for computational protein studies and the RMSF (see Tables 10 and 11).
Specifically, we found that, at the end of the simulation, all ligands altered the volume of the predicted binding cavity for each at the interface (see Figure 2). The variations in the docking energies and the structural changes that the complex may undergo in the presence of the ligands, could be related to the conformational alterations that occur as a result of changes in the chemical forces involve in the different ligand-protein interactions presents within of the cavity throughout the simulation. In fact, the variability of these interactions is manifested with the change in composition and distribution of cavities obtained at the same simulation time (see Tables 5–7). Ibuprofen being the compound that caused the greatest disturbance in terms of decrease in pocket volume, could be indicative of compaction in that region of the protein complex in the presence of these NSAIDs due to an increase in the specific and non-specific protein-ligand interactions in that dominion. Furthermore, some conformational changes that NSAIDs can induce in other types of unrelated proteins have been shown to be associated with time-dependent inhibition [84]. All compounds induced a change in the native arrangement of the residues corresponding to their binding pockets over time. While Ibuprofen and Aspirin increased the number of steric interactions, Acetaminophen exhibited a decrease. This also explains the variations in the relative binding energies at each sampling point. Furthermore, these are results that provide a theoretical basis to understand a little more the differences observed between these NSAIDs that have been widely studied in search of pharmacological interactions [77]. The ability of Ibuprofen to maintain a predictable binding with its possible allosteric site after the structural and conformational disturbances that induced in their respective binding pockets, is indicative that Ibuprofen possibly behaves as a kind of “allosteric effector”, partially altering the structure of the complex with respect to the native form, especially disrupting the ACE2 protein region in a “competitive” way with RBD and compromising stable binding RBD-ACE2 at the interface level (see Table 9), a phenomenon that has already been evidenced with the interaction of Ibuprofen to other proteins [85–88]. These results are interesting because they show a possible alternative mechanism that has not yet been reported in the literature for these compounds against viruses such as SARS-CoV-2 and, in particular, how they might alter the RBD-ACE2 complex. Although, this represents an approach that has already been explored against SARS-CoV-2 with other compounds [89–91].
The structural compaction induced by Ibuprofen continues to attract attention (see Figure 4). Because cellular membrane is a very crowded medium due to the presence of large amounts of biomolecules in a limited surface area and the crowding play an important role over stability, conformation and dynamic of biomolecular process, then the changes induced in volume and flexibility involve in the biochemical process that occur at membrane are under steric control of important manner, then, the folding of complex induced by Ibuprofen can has an consequence important [92]. Results that show Ibuprofen as one of the few NSAIDs, which, under the conditions described in this research, may have the highest probability of generating an alteration in the stability of the RBD-ACE2 complex and affecting its formation. A result that contributes to the use that has been given to NSAIDs against COVID-19 [93].
This way, our research with a bioinformatics approach allowed us to conclude that all the NSAIDs considered in this study can be docking thermodynamically in a favorable way to the RBD-ACE2 complex. But, only the drugs Ibuprofen (a propionic acid derivative), Aspirin (salicylates) and Acetaminophen (a p-aminophenol derivative) were able to mediate a thermodynamically favorable interaction with the RBD-ACE2 complex interface. Propionic acid derivatives, salicylates and p-aminophenol derivative have the least favorable binding energy with the enzyme receptor ACE2 of the protein complex, but at the same time have the most favorable energies for interface binding and docking more probabilistically feasible. The possibility of a favorable docking under another sampling model of any of the compounds excluded by the conditions of this study is not ruled out. Ibuprofen is the most favored thermodynamically docking NSAID at the interface of the RBD-ACE2 complex, followed by Aspirin and Acetaminophen. Likewise, Ibuprofen is capable of theoretically inhibiting the largest amount of the RBD-ACE2 protein complex in any of the conditions proposed in this study, regardless of time and with respect to the rest of the compounds considered. The differences in the docking energies and in the inhibitory potential of Ibuprofen are related to the indirect structural and conformational changes that this type of compound could induce in the complex after docking at the interface. Ibuprofen was the NSAID capable of causing the greatest disturbance in terms of decreased pocket volume within the interface in the shortest time of the simulation, inducing folding of complex. Ibuprofen is therefore the NSAID with the highest probability of generating an alteration in the stability of the RBD-ACE2 complex. The present study provides information about of the possibility of a new mechanistic route of action anti COVID-19 for Ibuprofen by affect the biomacromolecular docking between RBD and ACE2 which is known as relevant for the proliferation of this virus. However, this statement, although it contributes to the described use of NSAIDs for the empirical treatment and prevention of COVID-19 [93], requires its experimental demonstration because our predictions cannot guarantee a pharmacologically and clinically relevant interaction. Therefore, more studies are needed, especially given the real controversy that exists regarding the use of these drugs against COVID-19 [19–27]. Especially because to date, only one theoretical report of a binding of ligand at the interface of the RBD-ACE2 complex is known [49, 50], but this binding is in a different region than the one found in this work, in our work the binding of Ibuprofen occurs in an allosteric region. Which constitutes another valuable contribution of our work. In addition, we recommended considering different models for water and its effect on protein-protein interaction in the absence and presence of these drugs. Another important aspect is to consider is the energetic and structural disturbance of the RBD-ACE2 complex under different concentration gradients of Ibuprofen on the surface or near the complex, this due to the apparent dependence of the anti-viral activity shown by Ibuprofen with the bioavailability [45, 94]. Why although there are no pharmacological and theoretical results in a similar direction, reflecting the novelty of our study, there is experimental research in a similar direction that has shown that Ibuprofen could contribute to the treatment of COVID-19 [45]. In fact, currently including a clinical trial that seeks to demonstrate the potential antiviral activity against SARS-CoV-2 [94].
Data Availability Statement
The datasets presented in this study can be found in online repositories. The names of the repository/repositories and accession number(s) can be found in the article/Supplementary Material.
Author Contributions
LGP, conceptualization, methodology, software, formal analysis, investigation, writing-reviewing and editing. CL, software, investigation. FFM, preparation of tables and figures, writing and editing. JLP, formal analysis, investigation, writing-reviewing and editing. JV, discussion and analysis of results, reviewing and editing. YJA, conceptualization, methodology, formal analysis, investigation, writing-reviewing and editing.
Funding
This research was funded by the author-members of each of the institutions involved.
Conflict of Interest
The authors declare that the research was conducted in the absence of any commercial or financial relationships that could be construed as a potential conflict of interest.
Acknowledgments
This research was supported by IVIC-VEN and L.G.B.M-F.E.C-LUZ-VEN. JLP acknowledges the financial support provided by the Vice-Rectorado de Investigación, Innovación y Vinculación of Escuela Politécnica Nacional of Ecuador (Grant PII-DFIS-2020-04).
Supplementary Material
The Supplementary Material for this article can be found online at: https://www.frontiersin.org/articles/10.3389/fphy.2020.587606/full#supplementary-material
References
1. Guan W-J, Ni Z-Y, Hu Y, Liang W-H, Ou C-Q, He J-X, et al. Clinical characteristics of 2019 novel coronavirus infection in China. N Engl J Med. (2020). 382:1708–20. doi:10.1056/NEJMoa2002032
2. Chan PKS, Tang JW, Hui DSC SARS: clinical presentation, transmission, pathogenesis and treatment options. Clin Sci. (2006). 110:193–204. doi:10.1042/cs20050188
3. Yang Y, Lu Q, Liu M, Wang Y, Zhang A, Jalali N, et al. Epidemiological and clinical features of the 2019 novel coronavirus outbreak in China. MedRxiv and bioRxiv [Preprints] (2020). Available at: .
10.1101/2020.02.10.20021675CrossRef Full Text | Google Scholar
4. Huang C, Wang Y, Li X, Ren L, Zhao J, Hu Y, et al. Clinical features of patients infected with 2019 novel coronavirus in Wuhan, China. Lancet (2020). 395:497–506. doi:10.1016/S0140-6736(20)30183-5.
5. Xu Z, Shi L, Wang Y, Zhang J, Huang L, Zhang C, et al. Pathological findings of COVID-19 associated with acute respiratory distress syndrome. Lancet Respir Med. (2020). 8:420–2. doi:10.1016/S2213-2600(20)30076-X.
6. Wang D, Hu B, Hu C, Zhu F, Liu X, Zhang J, et al. Clinical characteristics of 138 hospitalized patients with 2019 novel coronavirus-infected pneumonia in Wuhan, China. J Am Med Assoc. (2020). 323:1061–9. doi:10.1001/jama.2020.1585.
7. Matthay MA, Aldrich JM, Gotts JE Treatment for severe acute respiratory distress syndrome from COVID-19. Lancet Respir Med. (2020). 8:433–4. doi:10.1016/S2213-2600(20)30127-2.
8. Chen N, Zhou M, Dong X, Qu J, Gong F, Han Y, et al. Epidemiological and clinical characteristics of 99 cases of 2019 novel coronavirus pneumonia in Wuhan, China: a descriptive study. Lancet (2020). 395:507–13. doi:10.1016/S0140-6736(20)30211-7.
9. Zhang W, Zhao Y, Zhang F, Wang Q, Li T, Liu Z, et al. The use of anti-inflammatory drugs in the treatment of people with severe coronavirus disease 2019 (COVID-19): the experience of clinical immunologists from China. Clin Immunol. (2020). 214:108393. doi:10.1016/j.clim.2020.108393.
10. Vanden Eynde JJ COVID-19: a brief overview of the discovery clinical trial. Pharmaceuticals (2020). 13, E65. doi:10.3390/ph13040065.
11. Shahera U, Sultana N, Sharmin M, Malakar J, Rashedul M, Zafrul K A comprehensive literature review on the pandemic coronavirus disease 2019 (COVID-19): Bangladesh is fighting against it. Am J Biomed Life Sci. (2020). 8, 76–82. doi:10.11648/j.ajbls.20200804.13
12. Hussain Bash S Corona virus drugs: a brief overview of past, present and future. J PeerSci. (2020). 2:1–16. doi:10.5281/zenodo.3747641.
13. Little P Non-steroidal anti-inflammatory drugs and covid-19. Br Med J (2020). 368:m1185. doi:10.1136/bmj.m1185.
14. Giollo A, Adami G, Gatti D, Idolazzi L, Rossini M Coronavirus disease 19 (Covid-19) and non-steroidal anti-inflammatory drugs (NSAID). Ann Rheum Dis. (2020). 0:1. doi:10.1136/annrheumdis-2020-217598.
15. Lands LC, Dauletbaev N High-dose ibuprofen in cystic fibrosis. Pharmaceuticals (2010). 3:2213–24. doi:10.3390/ph3072213.
16. Warden SJ Prophylactic use of NSAIDs by athletes: a risk/benefit assessment. Phys Sportsmed. (2010). 38:132–8. doi:10.3810/psm.2010.04.1770.
17. Bally M, Dendukuri N, Rich B, Nadeau L, Helin-Salmivaara A, Garbe E, et al. Risk of acute myocardial infarction with NSAIDs in real world use: bayesian meta-analysis of individual patient data. Br Med J (2017). 357:j1909. doi:10.1136/bmj.j1909
18. Lanas A, Chan FKL Peptic ulcer disease. Lancet (2017). 390:613–24. doi:10.1016/s0140-6736(16)32404-7.
19. Le Monda Le ministre de la santé déconseille l’ibuprofène contre le coronavirus (2020). Available at: https://www.lemonde.fr/societe/article/2020/03/14/face-au-coronavirus-le-ministre-de-la-sante-recommande-de-ne-pas-prendre-d-Ibuprofene_6033095_3224.html [Accessed March 15, 2018].
20.DGS-urgent. Actualisation recommandations covid 19 (2020). Available at: https://dgs-urgent.sante.gouv.fr/dgsurgent/inter/detailsMessageBuilder.do;jsessionid=21ECACBF8B1ECE6C542B9126E7A8215F.du-dgsurgentc2?id=30500&cmd=visualiserMessage [Accessed June 16, 2020].
21.20 minutes. Coronavirus à Bordeaux: Au cœur de l’unité Covid-19 du service réanimation du CHU Pellegrin (2020). Available at: https://www.20minutes.fr/societe/2742271-20200317-coronavirus-bordeaux-ur-unite-covid-19-service-reanimation-chu-pellegrin [Accessed June 16, 2020].
22. Fang L, Karakiulakis G, Roth M Are patients with hypertension and diabetes mellitus at increased risk for COVID-19 infection? Lancet Respir Med. (2020). 8:e21. doi:10.1016/s2213-2600(20)30116-8
23. Rinott E, Kozer E, Shapira Y, Bar-Haim A, Youngster I Ibuprofen use and clinical outcomes in COVID-19 patients. Clin Microbiol Infect. (2020). 26:e5–7. doi:10.1016/j.cmi.2020.06.003
24. Kutti G, Kotagiri R, Chandiramani VH, Mohan BP, Vegunta R, Rokkam VRP COVID-19 and avoiding ibuprofen. How good is the evidence? Am J Therapeut. (2020). 27:e400–2. doi:10.1097/mjt.0000000000001196
25. Martins‐Filho P, Marques E, Santana V No current evidence supporting risk of using Ibuprofen in patients with COVID‐19. Int J Clin Pract. (2020). 74:e13576. doi:10.1111/ijcp.13576
26. Moore N, Carleton B, Blin P, Bosco-Levy P, Droz C Does ibuprofen worsen COVID-19? Drug Saf. (2020). 43:611–4. doi:10.1007/s40264-020-00953-0
27. Torjesen I Covid-19: ibuprofen can be used for symptoms, says UK agency, but reasons for change in advice are unclear. Br Med J (2020). 367:m1555. doi:10.1136/bmj.m1555
28. Winther B, Mygind N Potential benefits of Ibuprofen in the treatment of viral respiratory infections. Inflammopharmacology (2003). 11:445–52. doi:10.1163/156856003322699627
29. Marzolini C, Stader F, Stoeckle M, Franzeck F, Egli A, Bassetti S, et al. Effect of systemic inflammatory response to SARS-CoV-2 on lopinavir and hydroxychloroquine plasma concentrations. Antimicrob Agents Chemother. (2020). 64:e01177–20. doi:10.1128/aac.01177-20
30.World Health Organization. (2020). The use of non-steroidal anti-inflammatory drugs (NSAIDs) in patients with COVID-19: scientific brief. Available at: https://www.who.int/news-room/commentaries/detail/the-use-of-non-steroidal-anti-inflammatory-drugs-(nsaids)-in-patients-with-covid-19 [Accessed April 19, 2020].
31. Sodhi M, Etminan M Safety of ibuprofen in patients with COVID-19. Chest (2020). 158:55–6. doi:10.1016/j.chest.2020.03.040
32. Wittine K, Benci K, Rajić Z, Zorc B, Kralj M, Marjanović M, et al. The novel phosphoramidate derivatives of NSAID 3-hydroxypropylamides: synthesis, cytostatic and antiviral activity evaluations. Eur J Med Chem. (2009). 44:143–51. doi:10.1016/j.ejmech.2008.03.037
33. Cherrick HM, Li KK, Li S-L, Park N-H Effect of ibuprofen on the in vitro and in vivo reactivation of latent HSV-1. Oral Surg Oral Med Oral Pathol. (1992). 73:321–7. doi:10.1016/0030-4220(92)90129-e
34. Rajic Z, Butula I, Zorc B, Kraljevic S, Hock K, Pavelic K, et al. Cytostatic and antiviral activity evaluations of hydroxamic derivatives of some non-steroidal anti-inflammatory drugs. Chem Biol Drug Des. (2009). 73:328–38. doi:10.1111/j.1747-0285.2009.00774.x
35. Terrier O, Dilly S, Pizzorno A, Henri J, Berenbaum F, Lina B, et al. Broad-spectrum antiviral activity of naproxen: from Influenza A to SARS-CoV-2 Coronavirus. MedRxiv and bioRxiv [Preprints] (2020). Available at: .
10.1101/2020.04.30.069922CrossRef Full Text | Google Scholar
36. Amici C, La Frazia S, Brunelli C, Balsamo M, Angelini M, Santoro MG Inhibition of viral protein translation by indomethacin in vesicular stomatitis virus infection: role of eIF2α kinase PKR. Cell Microbiol. (2015). 17:1391–404. doi:10.1111/cmi.12446
37. Chen N, Warner JL, Reiss CS NSAID treatment suppresses VSV propagation in mouse CNS. Virology (2000). 276:44–51. doi:10.1006/viro.2000.0562
38. Veljkovic V, Goeijenbier M, Glisic S, Veljkovic N, Perovic V, Sencanski M, et al. In silico analysis suggests repurposing of Ibuprofen for prevention and treatment of EBOLA virus disease. F1000 Res. (2015). 1:104. doi:10.12688/f1000research.6436.1
39. Veljkovic V Ibuprofen as a template molecule for drug design against Ebola virus. Front Biosci. (2018). 23:947–53. doi:10.2741/4627
40. Bourinbaiar AS, Lee-Huang S The non-steroidal anti-inflammatory drug, indomethacin, as an inhibitor of HIV replication. FEBS (Fed Eur Biochem Soc) Lett. (1995). 360:85–8. doi:10.1016/0014-5793(95)00057-g
41. Chen CJ, Raung SL, Kuo MD, Wang YM Suppression of Japanese encephalitis virus infection by non-steroidal anti-inflammatory drugs. J Gen Virol. (2002). 83:1897–905. doi:10.1099/0022-1317-83-8-1897
42. Rothan HA, Bahrani H, Abdulrahman AY, Mohamed Z, Teoh TC, Othman S, et al. Mefenamic acid in combination with ribavirin shows significant effects in reducing chikungunya virus infection in vitro and in vivo. Antivir Res. (2016). 127:50–6. doi:10.1016/j.antiviral.2016.01.006
43. Robb CT, Goepp M, Rossi AG, Yao C. Non–steroidal anti‐inflammatory drugs, prostaglandins, and COVID‐19. British J Pharmacol (2020). 177(21):4899--4920. doi:10.1111/bph.15206
44. Lagzian M, Valadan R, Saeedi M, Roozbeh F, Hedayatizadeh-Omran A, Amanlou M, et al. Repurposing naproxen as a potential antiviral agent against SARS-CoV-2. Research Square [Preprint] (2020). Available at: https://www.researchsquare.com/article/rs-21833/v1.
45. García NH, Porta DJ, Alasino RV, Muñoz SE, Beltramo DM Ibuprofen, a traditional drug that may impact the course of COVID-19 New effective formulation in nebulizable solution. Med Hypotheses. (2020). 144:110079. doi:10.1016/j.mehy.2020.110079
46. Liu C, Zhou Q, Li Y, Garner LV, Watkins SP, Carter LJ, et al. Research and development on therapeutic agents and vaccines for COVID-19 and related human coronavirus diseases. ACS Cent Sci. (2020). 6:315–31. doi:10.1021/acscentsci.0c00272
47. Siddiqui AJ, Jahan S, Ashraf SA, Alreshidi M, Ashraf MS, Patel M, et al. Current status and strategic possibilities on potential use of combinational drug therapy against COVID-19 caused by SARS-CoV-2. J Biomol Struct Dyn (2020). 5:1--14. doi:10.1080/07391102.2020.1802345.
48. Ortega JT, Serrano ML, Pujol FH, Rangel HR Role of changes in SARS-CoV-2 spike protein in the interaction with the human ACE2 receptor: an in silico analysis. EXCLI J (2020). 19:410–7. doi:10.17179/excli2020-1167
49. Wu C, Liu Y, Yang Y, Zhang P, Zhong W, Wang Y, et al. Analysis of therapeutic targets for SARS-CoV-2 and discovery of potential drugs by computational methods. Acta Pharm Sin B (2020). 10:766–88. doi:10.1016/j.apsb.2020.02.008
50. Han Y, Král P Computational design of ACE2-based peptide inhibitors of SARS-CoV-2. ACS Nano. (2020). 14:5143–7. doi:10.1021/acsnano.0c02857
51. Hinz B, Cheremina O, Brune K Acetaminophen (Paracetamol) is a selective cyclooxygenase-2 inhibitor in man. Faseb J (2008). 22:383–90. doi:10.1096/fj.07-8506com
52. Zoete V, Daina A, Bovigny C, Michielin O SwissSimilarity: a web tool for low to ultra high throughput ligand-based virtual screening. J Chem Inf Model. (2016). 56:1399–404. doi:10.1021/acs.jcim.6b00174
53. Daina A, Michielin O, Zoete V SwissADME: a free web tool to evaluate pharmacokinetics, drug-likeness and medicinal chemistry friendliness of small molecules. Sci Rep. (2017). 7:42717. doi:10.1038/srep42717
54. Dos Santos K, Guedes I, Karl A, Dardenne L Highly flexible ligand docking: benchmarking of the DockThor program on the LEADS-PEP protein-peptide dataset. J Chem Inf Model. (2020). 60:667–83. doi:10.1021/acs.jcim.9b00905
55. Thomsen R, Christensen MH MolDock: a new technique for high-accuracy molecular docking. J Med Chem. (2006). 49:3315–21. doi:10.1021/jm051197e
56. Velraj M, Chand P Molecular docking approach of potent natural inhibitors against 3D4Z, 4TRO and 5ACS receptors for anti-tubercular activity. Int J Res Pharm Sci. (2019). 10:303–12. doi:10.26452/ijrps.v10i1.1824
57. Umesh , Kundu D, Selvaraj C, Singh SK, Dubey VK Identification of new anti-nCoV drug chemical compounds from Indian spices exploiting SARS-CoV-2 main protease as target. J Biomol Struct Dyn. (2020). 1:1–9. doi:10.1080/07391102.2020.1763202
58. Ardra P, Prachi S, Hariprasad VR, Babu UV, Mohamed R, Raghavendra PR Potential phytochemical inhibitors of the coronavirus rna dependent rna polymerase: a molecular docking study. Research Square [Preprint] (2020). Available at: https://www.researchsquare.com/article/rs-35334/v1.
59. Subramanian S Some fda approved drugs exhibit binding affinity as high as -16.0 kcal/mol against COVID-19 main protease (Mpro): a molecular docking study. Research Square [Preprint] (2020). Available at: https://www.researchsquare.com/article/rs-25649/v1.
60. Tong T Drug targets in severe acute respiratory syndrome (SARS) virus and other coronavirus infections. Infect Disord - Drug Targets. (2009). 9:223–45. doi:10.2174/187152609787847659
61. Kumar Y, Singh H Silico identification and docking-based drug repurposing against the main protease of SARS-CoV-2, causative agent of COVID-19. ChemRxiv [Preprint] (2020). Available at: .
10.26434/chemrxiv.12049590.v1CrossRef Full Text | Google Scholar
62. Subramanian S Some neem leaves extract compounds exhibit very high binding affinity against COVID-19 main protease (Mpro): a molecular docking study. Research Square [Preprint] (2020). Available at: https://www.researchsquare.com/article/rs-25649/v1
63. Popov ME, Karlinsky DM Search for invisible binding sites of low-molecular-weight compounds on protein molecules and prediction of inhibitory activity. Mol Biol. (2013). 47:592–8. doi:10.1134/s0026893313040122
64. Cer RZ, Mudunuri U, Stephens R, Lebeda FJ IC50-to-Ki: a web-based tool for converting IC50 to Ki values for inhibitors of enzyme activity and ligand binding. Nucleic Acids Res. (2009). 37:W441–5. doi:10.1093/nar/gkp253
65. Wang Z, Wang X, Jia Y, Yin H, Feng Y, Jiang L, et al. Inhibition of human UDP‐glucuronosyltransferase enzymes by midostaurin and ruxolitinib: implications for drug‐drug interactions. Biopharm Drug Dispos (2020), 41(6): 231--238. doi:10.1002/bdd.2241
66. Baez-Santos YM, Mielech AM, Deng X, Baker S, Mesecar AD Catalytic function and substrate specificity of the papain-like protease domain of nsp3 from the middle east respiratory syndrome coronavirus. J Virol. (2014). 88:12511–27. doi:10.1128/jvi.01294-14
67. Wang J Fast Identification of possible drug treatment of coronavirus disease-19 (COVID-19) through computational drug repurposing study. J Chem Inf Model. (2020). 60:3277–86. doi:10.1021/acs.jcim.0c00179
68. Kasahara K, Ma B, Goto K, Dasgupta B, Higo J, Fukuda I, et al. myPresto/omegagene: a GPU-accelerated molecular dynamics simulator tailored for enhanced conformational sampling methods with a non-Ewald electrostatic scheme. Biophys Physicobio. (2016). 13:209–16. doi:10.2142/biophysico.13.0_209
69. Wada M, Kanamori E, Nakamura H, Fukunishi Y Selection of in silico drug screening results for G-protein-coupled receptors by using universal active probes. J Chem Inf Model. (2011). 51:2398–407.
70. Yonezawa Y Electrostatic properties of water models evaluated by a long-range potential based solely on the wolf charge-neutral condition. Chem Phys Lett. (2013). 556:308–14.
10.1016/j.cplett.2012.12.028CrossRef Full Text | Google Scholar
71. Eyal E, Lum G, Bahar I The anisotropic network model web server at 2015 (ANM 2.0). Bioinformatics (2015). 31:1487–9. doi:10.1093/bioinformatics/btu84
72. Wiederstein M, Sippl MJ ProSA-web: interactive web service for the recognition of errors in three-dimensional structures of proteins. Nucleic Acids Res. (2007). 35:W407–10. doi:10.1093/nar/gkm290
73. Sippl MJ Recognition of errors in three-dimensional structures of proteins. Proteins (1993). 17:355–62. doi:10.1002/prot.340170404
74. Hussein HA, Borrel A, Geneix C, Petitjean M, Regad L, Camproux AC PockDrug-Server: a new web server for predicting pocket druggability on holo and apo proteins. Nucleic Acids Res. (2015). 43:W436–42. doi:10.1093/nar/gkv462
75. Huang W, Lu S, Huang Z, Liu X, Mou L, Luo Y, et al. Allosite: a method for predicting allosteric sites. Bioinformatics (2013). 29:2357–9. doi:10.1093/bioinformatics/btt399
76. Patel A, Rajendran M, Pakala S, Shah A, Patel H, Karyala P Virtual screening of curcumin and its analogs against the spike surface glycoprotein of SARS-CoV-2 and SARS-CoV. ChemRxiv [Preprint] (2020). Available at: .
10.26434/chemrxiv.12142383.v2CrossRef Full Text | Google Scholar
77. Saxena A, Balaramnavar VM, Hohlfeld T, Saxena AK Drug/drug interaction of common NSAIDs with antiplatelet effect of aspirin in human platelets. Eur J Pharmacol. (2013). 721:215–24. doi:10.1016/j.ejphar.2013.09.032
78. Uchenna F, Chris U, Izuchukwu D, Okafor SN New carboxamides bearing benzenesulphonamides: synthesis, molecular docking and pharmacological properties. Bioorg Chem. (2019). 92:103265. doi:10.1016/j.bioorg.2019.103265
79. Husain MA, Sarwar T, Rehman SU, Ishqi HM, Tabish M Ibuprofen causes photocleavage through ROS generation and intercalates with DNA: a combined biophysical and molecular docking approach. Phys Chem Chem Phys. (2015). 17:13837–50. doi:10.1039/c5cp00272a
80. Wu R, Wang L, Kuo H-CD, Shannar A, Peter R, Chou PJ, et al. An update on current therapeutic drugs treating COVID-19. Curr Pharmacol Rep. (2020). 6:56–70. doi:10.1007/s40495-020-00216-7
81. Gimeno A, Mestres-Truyol J, Ojeda-Montes MJ, Macip G, Saldivar-Espinoza B, Cereto-Massagué A, et al. Prediction of novel inhibitors of the main protease (M-pro) of SARS-CoV-2 through consensus docking and drug reposition. Int J Mol Sci. (2020). 21:3793. doi:10.3390/ijms21113793
82. Mikra C, Rossos G, Hadjikakou S, Kourkoumelis N Molecular docking and structure activity relationship studies of NSAIDs. What do they reveal about IC50? Lett Drug Des Discov. (2017). 14:949–58. doi:10.2174/1570180814666161207143231
83. Azam F, Alabdullah NH, Ehmedat HM, Abulifa AR, Taban I, Upadhyayula S NSAIDs as potential treatment option for preventing amyloid β toxicity in Alzheimer’s disease: an investigation by docking, molecular dynamics, and DFT studies. J Biomol Struct Dyn. (2017). 36:2099–117. doi:10.1080/07391102.2017.1338164
84. Kurumbail RG, Stevens AM, Gierse JK, McDonald JJ, Stegeman RA, Pak JY, et al. Structural basis for selective inhibition of cyclooxygenase-2 by anti-inflammatory agents. Nature (1996). 384:644–8. doi:10.1038/384644a0
85. Di Masi A, Gullotta F, Bolli A, Fanali G, Fasano M, Ascenzi P Ibuprofen binding to secondary sites allosterically modulates the spectroscopic and catalytic properties of human serum heme-albumin. FEBS J (2011). 278:654–62. doi:10.1111/j.1742-4658.2010.07986.x
86. Baroni S, Mattu M, Vannini A, Cipollone R, Aime S, Ascenzi P, et al. Effect of Ibuprofen and warfarin on the allosteric properties of haem-human serum albumin. Eur J Biochem. (2001). 268:6214–20. doi:10.1046/j.0014-2956.2001.02569.x
87. Ascenzi P, Cao Y, Tundo GR, Coletta M, Fanali G, Fasano M Ibuprofen and warfarin modulate allosterically ferrous human serum heme–albumin nitrosylation. Biochem Biophys Res Commun. (2011). 411:185–9. doi:10.1016/j.bbrc.2011.06.130
88. Ascenzi P, di Masi A, De Sanctis G, Coletta M, Fasano M Ibuprofen modulates allosterically NO dissociation from ferrous nitrosylated human serum heme-albumin by binding to three sites. Biochem Biophys Res Commun. (2009). 387:83–6. doi:10.1016/j.bbrc.2009.06.117
89. Ping W, Chun-Mei L, Lu Z, Ying L, Lu-Hua L Substrate binding and homo-dimerization of SARS 3CL proteinase are mutual allosteric effectors. Acta Phys Chim Sin. (2010). 26:1093–8. doi:10.3866/PKU.WHXB20100449
90. Xia B, Kang X Activation and maturation of SARS-CoV main protease. Protein Cell. (2011). 2:282–90. doi:10.1007/s13238-011-1034-1.
91. Olotu FA, Omolabi KF, Soliman MES. Leaving no stone unturned: Allosteric targeting of SARS-CoV-2 Spike protein at putative druggable sites disrupts human angiotensin-converting enzyme interactions at the receptor binding domain. Inform Med unlocked (2020). 21:100451. doi:10.1016/j.imu.2020.100451
92. Gomez D, Huber K, Klumpp S On protein folding in crowded conditions. J Phys Chem Lett. (2019). 10:7650–6. doi:10.1021/acs.jpclett.9b02642
93. Hyoung-Shik S Empirical treatment and prevention of COVID-19. J Infect Chemother. (2020). 52:142–53. doi:10.3947/ic.2020.52.2.142
94.ClinicalTrials.gov. Garcia (MD). National Council of Scientific and Technical Research (Argentina). Extended Compassionate Use Program (UCA) with inhalational ibuprofen in patients with acute respiratory pathology, mediated by COVID-19. (2020) Available in: https://clinicaltrials.gov/ct2/show/NCT04382768.
95. Bhal S Data from: LogP—Making sense of the value. Advanced chemistry development (2007). Available from: https://www.acdlabs.com/download/app/physchem/making_sense.pdf.
Keywords: SARS-COV-2, NSAIDs, molecular docking, molecular dynamics, COVID-19
Citation: González-Paz LA, Lossada CA, Fernández-Materán FV, Paz JL, Vera-Villalobos J and Alvarado YJ (2020) Can Non-steroidal Anti-inflammatory Drugs Affect the Interaction Between Receptor Binding Domain of SARS-COV-2 Spike and the Human ACE2 Receptor? A Computational Biophysical Study. Front. Phys. 8:587606. doi: 10.3389/fphy.2020.587606
Received: 27 July 2020; Accepted: 19 October 2020;
Published: 12 November 2020.
Edited by:
Yong Xu, Northwestern Polytechnical University, ChinaReviewed by:
Antonio Marco Batista, Universidade Estadual de Ponta Grossa, BrazilAnanya Debnath, Indian Institute of Technology Jodhpur, India
Qin Xu, Shanghai Jiao Tong University, China
Copyright © 2020 Gonzalez-Paz, Lossada, Fernández-Materán, Paz, Vera-Villalobos and Alvarado. This is an open-access article distributed under the terms of the Creative Commons Attribution License (CC BY). The use, distribution or reproduction in other forums is permitted, provided the original author(s) and the copyright owner(s) are credited and that the original publication in this journal is cited, in accordance with accepted academic practice. No use, distribution or reproduction is permitted which does not comply with these terms.
*Correspondence: Lenin A. González-Paz, bGdvbnphbGV6cGF6QGdtYWlsLmNvbQ==, J. L. Paz, am9zZS5wYXpyQGVwbi5lZHUuZWMuY29t