- 1Centro de Física Aplicada y Tecnologia Avanzada, Universidad Nacional Autónoma de México, Juriquilla, Mexico
- 2Instituto de Matemáticas, Universidad Nacional Autonoma de Mexico, Juriquilla, Mexico
The novel coronavirus SARS-CoV-2 has paralyzed our societies, leading to self-isolation and quarantine for several days. As the 10th most populated country in the world, Mexico is on a major threat by COVID-19 due to the limitations of intensive care capacities, about 1.5 hospital beds for every 1,000 citizens. In this paper, we characterize the COVID-19 pandemic in Mexico and projected different scenarios to evaluate sharp or gradual quarantine lifting strategies. Mexican government relaxed strict social distancing regulations on June 1, 2020, deriving to pandemic data with large fluctuations and uncertainties of the tendency of the pandemic in Mexico. Our results suggest that lifting social confinement must be gradually sparse while maintaining a decentralized region strategy among the Mexican states. To substantially lower the number of infections, simulations highlight that a fraction of the population that represents the elderly should remain in social confinement (approximately 11.3% of the population); a fraction of the population that represents the confined working class (roughly 27% of the population) must gradually return in at least four parts in consecutive months; and to the last a fraction of the population that assumes the return of students to schools (about 21.7%). As the epidemic progresses, deconfinement strategies need to be continuously re-adjusting with the new pandemic data. All mathematical models, including ours, are only a possibility of many of the future, however, the different scenarios that were developed here highlight that a gradual decentralized region deconfinement with a significant increase in healthcare capacities is paramount to avoid a high death toll in Mexico.
1. Introduction
Severe Acute Respiratory Syndrome Coronavirus 2 (SARS-CoV-2) is the virus behind the 2019 coronavirus disease (COVID-19) with alarming levels of spread and death tolls worldwide. With more than four million confirmed cases and 292,046 deaths [1], COVID-19 pandemic has spread 212 countries moving the epicentre from China to Europe and consequently to America [1]. While potential vaccines and antiviral drugs are under fast development [2], epidemiological models have underlined the relevance of social distancing interventions as the main weapon so far to mitigate COVID-19 pandemic [3].
Epidemiological models have played a central role to advance our understanding in SARS-CoV-2 transmission [3–6]. In its early stages, the epidemic can double in size every 7.4 days [7]. The case fatality rate for COVID-19 ranges from 0.3 to 1% [1] up to 20% [8]. The basic reproductive number has been computed roughly 2.2 (95% CI, 1.4–3.9) [4, 7]. The lesson learned from China, Italy, and the United States pointed out that COVID-19 can quickly result in high demands of healthcare capacities deriving in the collapsing of hospitals of well-resourced nations [9, 10].
Developing countries with limitations of intensive care beds are highly jeopardized by COVID-19. Mexico ranks as the 10th most populated country in the world with about 127 million people [11]. About 60.4% of the population is economically active whereof 56.2% depends on the informal workforce [11]. Albeit the economic impact and levels of moderate poverty, Mexico adopted on March 15, 2020 social confinement, which was relatively early compared to the confirmed number of cases and deaths [12]. By the cut-off date of May 25, 2020, the public health strategy in Mexico has resulted in 71,105 infected cases and 7,633 deaths by COVID-19 [12].
The public health strategy followed by Mexico for the COVID-19 pandemic is the sentinel model, which was implemented by Mexico in 2009 against the pandemic of influenza H1N1 [13]. The sentinel model consists on three phases. In stage 1 of the outbreak, the model applies case-by-case monitoring. In phase two, the model focuses on places where the epidemic is growing, at this stage a community-based surveillance is applied. In phase three, which is the peak infection phase, the central problem of pandemic surveillance is not to monitor the growth of the pandemic but to ensure that hospital capacities are not exceeded. Mexico has an extremely limited healthcare capacity, approximately 1.5 beds for every 1,000 citizens. Based on early data of the pandemic and assuming social confinement restrictions, the Sub-secretary of Prevention and Health Promotion of Mexico presented mathematical model predictions with a peak of the pandemic Mexico between 8 and 10 May with an end of the pandemic on June 25, 2020 [12]. Mexico is planning to lift the strict social distancing regulations on June 1, 2020. However, an abruptly lifting of social confinement would likely result in new waves of new COVID-19 cases and high death tolls.
While the SIR model has a simple structure, this has been central to understand different epidemiological aspects of COVID-19 such as the reproductive number, number of infected cases in short time scales, and the effects of lifting confinement strategies in different countries [3–5, 14]. Simple models can help us to think clearly and to interpret pandemic data sets on quantitative grounds. Consequently, simulations can infer predictions of different possible scenarios. If the predictions are confirmed, the model has proven to be a justified simplification of the complex reality and may be further used. If the predictions are wrong, the model assumptions can be reconsidered or extended, which is very important during pandemics. In this paper, we fit a mathematical model for COVID-19 [15] using data of the COVID-19 epidemic in Mexico [12] as well as its public health capacities and demographic conditions [11]. Different lifting confinement scenarios are evaluated for the main regions of Mexico in order to inform public makers to tailor decentralized region strategies through the Mexican territory with the ultimate goal to minimize deaths.
2. The Sentinel Model for Tracking COVID-19 in Mexico
Epidemiological models have been proposed to represent COVID-19 pandemic in different countries [15, 16]. These contain many variables resulting in a significant number of parameters difficult to estimate with epidemiological data at the moment of the pandemic. Instead of considering the exposed compartment and then divided into presymptomatic, symptomatic, and asymptomatic, we assume that the exposed hosts to the virus go from susceptible to presymptomatic. Consequently, presymptomatic cases would progress to symptomatic or to recover. In this fashion, we do not increase the complexity of the model. Furthermore, several assumptions would need to be integrated in order to match the sentinel model used by Mexico against COVID-19 [13]. The model writes as follow:
The susceptible population is represented by S, from which the total country population (N) is about 127 million [11]. The susceptible population would pass to the presymptomatic compartment (A). Note that in the sentinel model applied in Mexico is that testing is about 10% of the suspected cases while 100% of hospitalized cases and deaths. Once the infected cases (I) are listed in the epidemiological data sets by the Mexican Government, the infected case should be either hospitalized or at home under confinement. Based on the fact that presymptomatic shedding is very common for COVID-19 [17], it is a reasonable assumption to consider that new infections are mainly driven by the presymptomatic population with the term
The first confirmed case of COVID-19 in Mexico was reported on February 28th, 2020 [12]. On the day of social confinement, March 15, 2020, the number of infected cases (
The compartment C is the confined population which entered with a rate q from the susceptible population. τ the effect of population deconfinement, which can be attributed to government policies as well as resistant/forgetting factor by the population to keep adequately the confinement. Estimations by the COVID-19 community mobility report of Google [23] suggest a reduction in the mobility of about 50–70%. Thus, the ratio between
2.1. Re-sampling Strategy for Parameter Distributions
A re-sampling strategy was employed to fit the parameters β and η. The range of parameter values in Table 1 served to generate 3,000 sets of random parameters with specific statistical distributions as presented in Supplementary Figure S1. This set of parameters consequently was used to fix parameters and perform 3,000 fitting repetitions by minimizing the root mean square (RMS) difference between the model predictive output (
3. Simulation Results
Social confinement started in Mexico on March 15, 2020, which was early compared to the confirmed number of cases and deaths in comparison to other countries [12]. Albeit of this early confinement, Mexico had an exponential growth of infected cases and many deaths, thus confinement needed to be extended. Pandemic data in Mexico is very heterogeneous among regions as well as in time scales. This may be attributed to different social behavior, working class, and public health policies.
3.1. Scenario 1: COVID-19 Pandemic Under Confinement
The first scenario to investigate, Scenario 1, is the reports of infected cases from 15 March till May 25, 2020 [12]. This time period is when a major part of the population remained in social confinement, about 50–70% [23]. The embedded panel in Figure 1 presents the best fit and sampling procedure for the whole country, Mexico City (CMX) and the State of Mexico (MEX), while the other regions with major number of infected cases is shown in Supplementary Figure S2. Simulations show that while the early social confinement would delay the initial expected peak of the pandemic on middle may [12] till July-August, the number of infected per day would reach very high numbers, an order of 104–105.
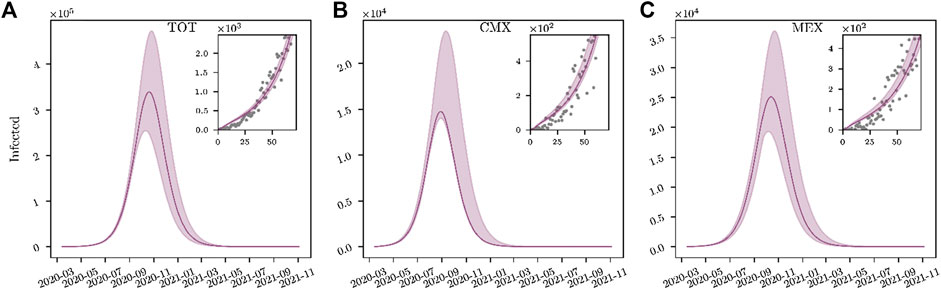
FIGURE 1. Scenario 1: Maintaining COVID-19 Lockdown. Mathematical model (1)–(5) is fitted with pandemic data from 15 March to May 25, 2020. Shadows indicate the range of 3,000 fits of infected cases considering the range of parameters in Table 1. Simulations are performed till November 2021.
In fact, reproductive numbers for the whole country as well as major regions affected with COVID-19 were between 2.3 and 3.6 (Supplementary Table S1). This underlines that even social restrictions SARS-CoV-2 was still spreading among the country and major populated cities. The heterogeneity of the infections and their respective public health necessities varied significantly among different geographic regions of Mexico. Note in Figure 2 that some states had
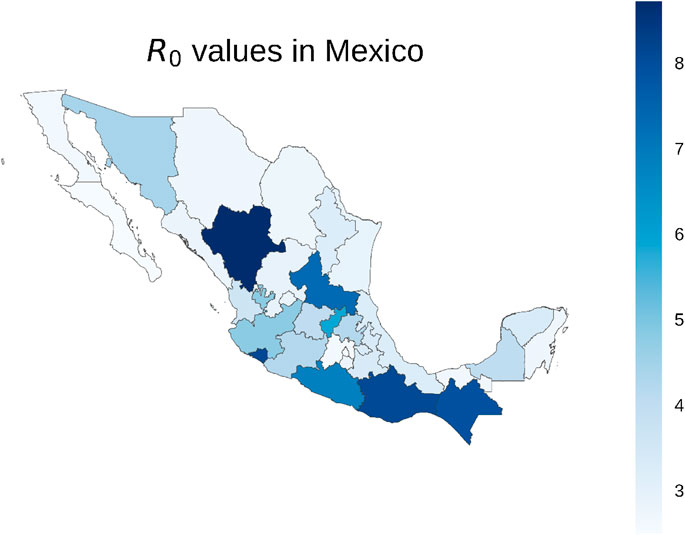
FIGURE 2. Reproductive number (
Considering the reported number of infected cases, Figure 3 presents the percent of the COVID-19 infected cases that require Intensive Care Units beds (ICU), intubation facilities (INT) and hospital beds (HOS) from 12 April till August 15, 2020 [26]. Large box plots imply that the requirements of the state were changing a lot in time e.g., Zacatecas (Zac) and Colima (Col). On the other hand, small box plots highlight that public health requirements for the state were very consistent during the reported period e.g., CMX.
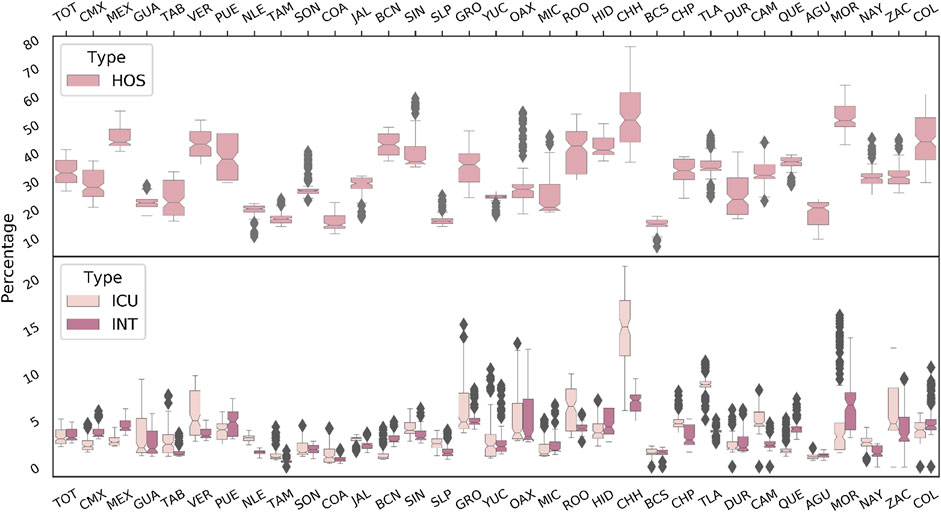
FIGURE 3. National requirements of Intensive Care Units beds (ICU), intubation facilities (INT) and hospital beds (HOS). These percents are based on the reported data of infected cases that require ICU, INT or HOS. Box plots are composed by data sets from 12 april till 15 August. Therefore, those states with small box plots reveal a very stable needs in public health capacities in time (e.g., CMX). Data was extracted from Ref. [26].
Assuming the percents of required hospital beds with respect to the reported infected cases are consistent in the future (about 38% for the whole country, see Figure 3 and Supplementary Table S2), the most optimistic peak of the pandemic presented in Figure 1 could be about 254,174 infected cases, which would result in a saturation of hospitals. That is, the whole country would require to have about 97,180 hospital beds, while the number available of beds for COVID-19 pandemic reported for the whole country is about 49,083 [27]. This is a major public concern as Mexico would only be able to provide approximately 50% for the peak of COVID-19 pandemic, otherwise hospitals capacities for non-COVID-19 patients would be reduced.
On March 24, an official communication [27] reported that 40% of the available hospital beds in the country were dedicated to the COVID-19 epidemic. That is, a total of 49,083 hospital beds, 256 intensive care units, 5,523 ventilators and 2,446 intensive care beds. Furthermore, a re-conversion program was in process, to increase the health capacity [27]. In spite of social confinement, simulations pointed out a high threat to Mexico (Figure 1), that is for the most optimistic scenario, the pandemic peak would be about 338 thousand infected people. If the ratios of hospitalization observed so far are consistent in the next months (about 38% of infected cases, see Figure 3), Mexico would be almost at the maximum capacity, approximately 98% hospital bed occupancy, although beds of non-COVID-19 patients are considered for COVID-19 patients. Therefore, social confinement lifting would very likely result in a hospital system breakdown.
3.2. Scenario 2: Complete Lifting Confinement on June 1, 2020
Mexico was planning to lift social distancing restrictions on June 1, 2020, however, due to the continuous increase in infected cases, confinement has been irregular [12]. Based on the nominal values presented in Table 1, we develop different possible scenarios to gradually lift social confinement and explain the on-going pandemic. To this end, we will study first the hypothetical case of a complete lifting confinement on June 1, 2020 (Scenario 2). This would help to understand what has been the implication of social confinement on the initial estimations. Panels (a), 2) and 3) in Figure 4 present a simulation scenario with a sharp and massive return of activities on June 1, 2020. This would result in a major increase of infected cases, about two orders of magnitude higher than the scenario with social confinement in Figure 1.
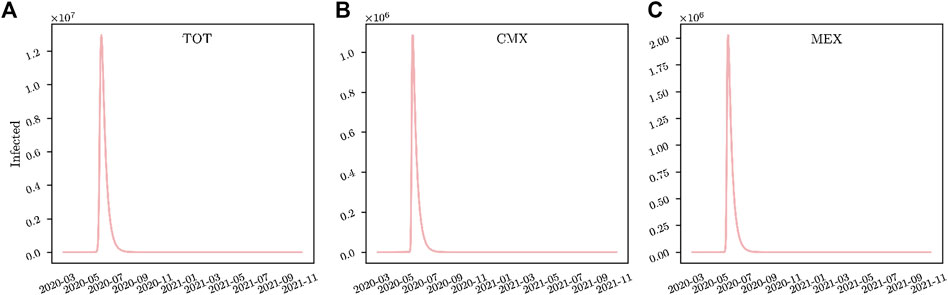
FIGURE 4. Scenario 2: Complete lifting Confinement on June 1, 2020. Panels (A) Present a sharp and massive return of people for social deconfinement on June 1, 2020 for the whole country; (B) CMX, and; (C) MEX, respectively.
3.3. Scenario 3: Orderly Gradual Lifting Confinement on June 1, 2020
Considering that the confinement in Mexico was about 60% of the population [23], we evaluate a step-wise social confinement lifting based on a gradual deconfinement of different age groups. Based on Mexican reports by the INEGI [11], Mexican population can be divided into three major age groups:
Note that our model does not include any differentiation in infection risk and contact patterns among elderly, working class and students. As a result, it is not able to distinguish those sub-populations. Therefore, any conclusion about the order for lifting confinement of any sub-populations is purely based on assumptions. What can be represented in our model is the fraction of population lifted in each phase.
Thus, we consider fractions of the population that would represent the elderly, group
The initiation of activities of a fraction of the population proportional to the working class and students is tailored to reduce the peak of the pandemic. Figure 5A–C shows a step-wise deconfinement strategy starting at different months (from June till November), returning a fraction proportional to the working class in four consecutive months (or three for CMX and MEX) and at the last the returning of students to schools. Independently of the initiation time of deconfinement, simulations in Figure 5 highlight that confinement lifting must be avoided before passing the peak of the pandemic. If the initiation of activities is before the first peak, this would result in a much higher number of infected cases, and consequently death tolls.
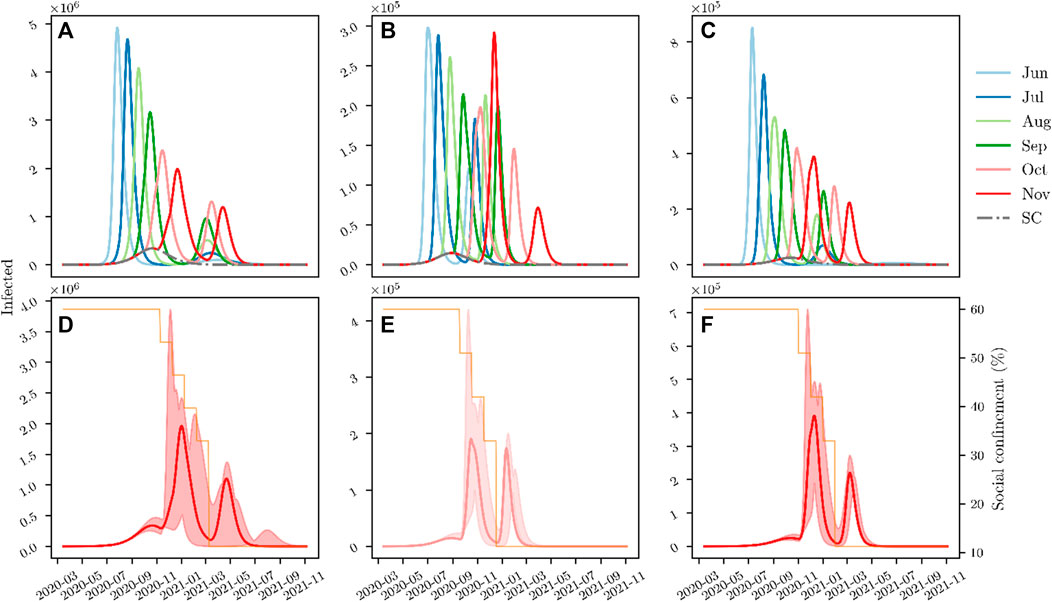
FIGURE 5. Scenario 3: Gradual lifting Confinement on June 1, 2020. Panels (A) shows a step-wize social confinement lifting at different time points for the whole country; (B) CMX, and; (C) MEX, respectively. Panels (D) underlined the best scenario for confinement lifting in the whole country; (E) CMX, and; (F) MEX, respectively. This consists on keeping in confinement a fraction of the population equivalent to the size of the elderly, while a fraction representing the confined working force of Mexico (about 27%) returns to activity in four parts (three steps for CMX and MEX), each one in consecutive months, while a fraction assuming the students would come back to school one month after the working group.
Similar outcomes are obtained for CMX and MEX (Figure 5B, C) as well as in major regions of Mexico (Supplementary Figure S3). Figure 5D–F underlines that the best time to initiate deconfinement in CMX and MEX would be between October and November. While this step-wise confinement lifting strategy is the best alternative to mitigate the peak of the pandemic, it would still derive a second wave of the pandemic equivalent to the first one under social restrictions. Estimations of hospital beds requirements for deconfinement strategies for different Mexican regions are presented in Supplementary Table S2.
3.4. Scenario 4: Compliance of the Population Under Social Restrictions and Gradual Lifting Confinement on June 1, 2020
Using the model (1)–(5) to recapitulate the pandemic data from June 1 till August 15, 2020 was not possible to achieve changing only the social confinement (%C). Therefore, the model (1)–(5) was extended with the Eq. 6, which would mimic the deconfinement and social behavior dynamics in Mexico. Deconfinement initiates at day
Note that Eq. 6 is a possible scenario among many others to explain the epidemiological data in Mexico. Of course this is a hypothetical scenario, but in principle any damped oscillating function would represent the pandemic data.
To further adapt to the pandemic data, we incorporate social distance (
The dynamics of social distance and deconfinement is illustrated in the lower panel in Figure 6 with parameter values in Table 2. Figure 6 shows the pandemic data and model simulations. We would like to remark this is just a possible scenario among possibly many others that recapitulate the pandemic data of Mexico.
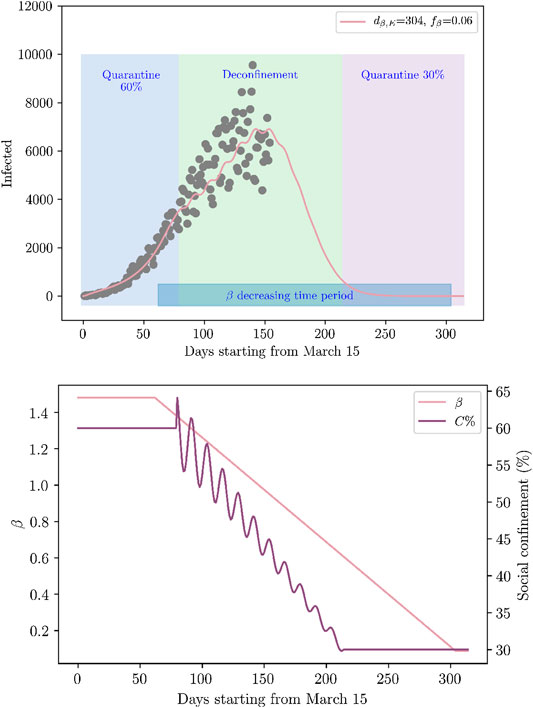
FIGURE 6. Possible scenario to represent COVID-19 pandemic data. Pandemic data and model simulation (upper panel). Confinement lifting scheme and ß modification (lower panel). The percent of the population in confinement (%C) with Eq. 6 and social distance measures affecting the value of ß with Eq. 7. These equations with parameter values at Table 2 represent a possible scenario to reconcile the pandemic data from June 1 till August 15.
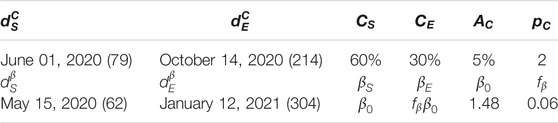
TABLE 2. Parameter values for confinement lifting scheme and social distance measures in Eqs 6, 7.
4. Discussion
The year 2020 has revealed one of the biggest pandemics reported in history, the novel coronavirus SARS-CoV-2 causes a severe and potentially fatal acute respiratory syndrome (COVID-19). Epidemiological reports by countries with strong public health capacities have uncovered the potential of COVID-19 to saturate hospitals in short time [1]. Therefore, COVID-19 is a major threat to developing countries because of the limitations of intensive care beds. While no vaccine or antiviral drug is likely to be available soon, the only remaining tool against COVID-19 is social confinement. However, a prolonged lock-down will hugely affect societies, education, and economy.
In this paper, we investigate the dynamics of the COVID-19 pandemic in Mexico, which adopted on March 15 social confinement to avoid the fast spread of the virus and the eventual collapse of the public health services [12]. Mexico is a highly populated country with major levels of moderate poverty. A strict social confinement has been extremely difficult to apply in a country with 56.2% of the population working informally [11]. Mexico originally plan to lift the social confinement on June 1, 2020, however, the high death tolls in a limited public health system forced to extend the confinement.
While keeping the elderly in social confinement until a vaccine is available, the best strategy to contain the epidemic in Mexico would be to return social activities after the first peak of the pandemic, returning a fraction of the population equivalent to the confined working class (about 27% of the population) in four equal parts, and at the last the returning of students to schools (see Figure 5). Considering the best deconfinement strategy, Mexico would need at least a three fold increase in current hospital capacities. Note that this is an upper view of the pandemic at country level, however, the fate decision of pandemic evolution has shown great differences not only among countries but also among states inside of countries. Therefore, social confinement lifting should be tailored for each city in Mexico. Mexican Government may need to consider in deconfinement strategies not only on the age factor in COVID-19 patients Supplementary Figure S4 but also people with chronic diseases such as diabetes, COPD and obesity as shown in Supplementary Figure S5. This is also a main concern, as in Mexico 65% of the population is overweight, 30% of the population is obese, and about 10% of the population had diabetes [28].
The mathematical model (1)–(5) was fitted with data from 15 March to May 25, 2020 (Figure 3). For validation the model was used to predict an independent data set from May 25 till June 1st, Supplementary Figure S6 shows how the model can predict the tendency of the pandemic. However, on June 1, 2020, confinement restrictions were relaxed in a growing phase of new infected cases and deaths. However, data sets from June 1 till 15 shows a deceleration of the infected cases. Simulations in Figure 6 shows that the dispersion of data sets of infected cases could be attributed to changes in social behavior to confinement as well as social distance measures. A key aspect to represent pandemic data in Figure 6 was the combination of deconfinement from 60% to 30% and a decrease in ß starting two weeks before deconfinement initiation.
In the same spirit of the epic phrase by Goerge E.P. Box that “All models are wrong, but some are useful”, our model as well as others for COVID-19 has several limitations. In particular, mathematical models that are fitted during the emerging phase, exponential growth, of an outbreak that have potential sources of bias [29]. Furthermore, the data of infected cases in Mexico is very limited because of the implementation of the sentinel model. While sampling is based on 10% of the suspected cases while 100% of hospitalized cases and deaths, mass testing for stages 2 and 3 is considered irrelevant. In fact, reports by the OECD (COVID-19) [30] underlines that Mexico was only testing 0.6–0.8 per every thousand, occupying in this way Mexico the last place among countries with high number of infected cases. With 5,623 new tests on May 4, 2020, Mexico reported the highest number of samples per day [30]. Therefore, with this sample rate Mexico will be unable to register the dimension of the epidemic, actually, it is expected that Mexico will have a flat region as soon as the number of infected cases per day passes over 7,560. At the peak of the pandemic Mexico would need to test at least 18 per every thousand to provide a better vision of the problem. The summary of activities to reduce the COVID-19 Pandemic in Mexico would be:
• Return social activities after the first peak of the pandemic.
• Keep the elderly and citizens with chronic diseases such as diabetes, COPD and obesity in social confinement until a vaccine is available.
• Lift confinement to a fraction of the population equivalent to the confined working class (about 27% of the population) in four equal parts after the first peak during confinement.
• Returning of students to schools by February 2021.
• Testing for COVID-19 should be at least 18 per every thousand.
• Increase at least three fold hospital capacities dedicated for COVID-19.
• Continuous social distance and mouth cover use to decrease the rate of new infections.
Traveling is having a key aspect in COVID-19 pandemic. Previous studies explored the consequences for travel [6], particularly focusing on airplane transportation but attempting to give a gross approximation to terrestrial movement since this is the main form of population movement in Mexico. Given the large population size of migrants in Mexico, the transmission risk of this population is high. Thus, airports should test passengers arriving from certain countries when they enter Mexican territory, potentially allowing them to spend less time in quarantine if they test negative.
Note that this study does not consider the economic effects but it was only based to have enough hospital capacities. With the progressing of information of COVID-19 pandemic as well as new data of infected cases in Mexico, our simulations would be more accurate and hopefully less drastic scenarios. Nevertheless, our model predictions highlight critical scenarios and suggest tailored public health strategies for social confinement lifting in combination with a significant increase in the health care system capacities.
Author’s Note
This manuscript has been released as a preprint at medRxiv (31).
Data Availability Statement
Publicly available datasets were analyzed in this study. This data can be found here: https://serendipia.digital/2020/03/datos-abiertos-sobre-casos-de-coronavirus-covid-19-en-mexico.
Author Contributions
CR performed the simulations. EH-V envisaged the project. All the authors discussed and wrote the paper.
Funding
This research was funded by the Universidad Nacional Autonoma de Mexico (UNAM), CONACYT, and the Alfons und Gertrud Kassel-Stiftung. This work received support from Luis Aguilar of the Laboratorio Nacional de Visualización Cientifica Avanzada (LAVIS) at UNAM-Juriquilla.
Disclaimer
The results expressed in this report should not be construed to represent the views of any agencies or the Mexican government.
Conflict of Interest
The authors declare that the research was conducted in the absence of any commercial or financial relationships that could be construed as a potential conflict of interest.
Supplementary Material
The Supplementary Material for this article can be found online at: https://www.frontiersin.org/articles/10.3389/fphy.2020.573322/full#supplementary-material.
References
2. Lowe D A close look at the frontrunning coronavirus vaccines as of may 1 (updated)—in the pipeline. (2020). Tech. Rep.
3. Anderson RM, Heesterbeek H, Klinkenberg D, Hollingsworth TD. How will country-based mitigation measures influence the course of the COVID-19 epidemic? Lancet (2020) 395:931–934. doi:10.1016/S0140-6736(20)30567-5
4. Kucharski AJ, Russell TW, Diamond C, Liu Y, Edmunds J, Funk S, et al. Early dynamics of transmission and control of COVID-19: a mathematical modelling study. Lancet Infect Dis (2020) 3099:1–7. doi:10.1016/s1473-3099(20)30144-4
5. Weitz JS, Beckett SJ, Coenen AR, Demory D, Dominguez-Mirazo M, Dushoff J, et al. Modeling shield immunity to reduce COVID-19 epidemic spread. Nat Med (2020) 26:849–854. doi:10.1038/s41591-020-0895-3
6. Acuña-Zegarra MA, Santana-Cibrian M, Velasco-Hernandez JX. Modeling behavioral change and COVID-19 containment in Mexico: a trade-off between lockdown and compliance. Math Biosci (2020) 325:108370. doi:10.1016/j.mbs.2020.108370
7. Li Q, Guan X, Wu P, Wang X, Zhou L, Tong Y, et al. Early transmission dynamics in wuhan, China, of novel coronavirus–infected pneumonia. N Engl J Med (2020) 382:1199–1207. doi:10.1056/NEJMoa2001316
8. Baud D, Qi X, Nielsen-Saines K, Musso D, Pomar L, Favre G. Real estimates of mortality following COVID-19 infection. Lancet Infect Dis (2020) 3099:30195. doi:10.1016/s1473-3099(20)30195-x
9. Li R, Rivers C, Tan Q, Murray MB, Toner E, Lipsitch M. The demand for inpatient and ICU beds for COVID-19 in the US: lessons from Chinese cities. medRxiv (2020) 16:20033241. doi:03.0910.1101/2020.03.09.20033241
10. Kissler SM, Tedijanto C, Goldstein E, Grad YH, Lipsitch M. Projecting the transmission dynamics of SARS-CoV-2 through the postpandemic period. Science (2020) 368(6493):860–8. doi:10.1126/SCIENCE.ABB5793
11.INEGI. Encuesta Nacional de Ocupación y Empleo (ENOE), población de 15 años y más de edad. (2019). Tech Rep.
12.Secretaria de Salud MEXICO. Coronavirus (COVID-19)-Comunicado técnico diario—secretaría de Salud—gobierno—gob.mx. (2020). Tech Rep.
13. de Salud S. Manual para la vigilancia epidemiológica de Influenza. Dirección General de Epidemiologia (2014) 1–84.
14. López L, Rodó X. The end of social confinement and COVID-19 re-emergence risk. Nat Human Behav (2020) 4:746–55. doi:10.1038/s41562-020-0908-8
15. Peng L, Yang W, Zhang D, Zhuge C, Hong L Epidemic analysis of COVID-19 in China by dynamical modeling. arXiv (2020) 1–12. doi:10.1101/2020.02.16.2002.06563
16. Zhao H, Feng Z. Staggered release policies for covid-19 control: costs and benefits of relaxing restrictions by age and risk. Math Biosci (2020) 326:108405. doi:10.1016/j.mbs.2020.108405
17. He X, Lau EHY, Wu P, Deng X, Wang J, Hao X, et al. Temporal dynamics in viral shedding and transmissibility of COVID-19. Nat Med (2020) 26:672–5. doi:10.1038/s41591-020-0869-5
18. LeungLau R, Okel LC, Dorigatt I, Winskill P, Whittaker C, Imai N, Cuomo-Dannenburg G, et al. Estimates of the severity of coronavirus disease 2019: a model-based analysis. Lancet Infect Dis (2020) 20(6):669–77. doi:10.1016/S1473-3099(20)30243-7
19. Wölfel R, Corman VM, Guggemos W, Seilmaier M, Zange S, Müller MA, et al. Virological assessment of hospitalized patients with COVID-2019. Nature (2020) 581(7809):465–9. doi:10.1038/s41586-020-2196-x
20.WHO. WHO Director-General’s opening remarks at the media briefing on COVID-19–24 February 2020 (2020).
21. Phua J, Weng L, Ling L, Egi M, Lim C-M, Divatia JV, et al. Intensive care management of coronavirus disease 2019 (COVID-19): challenges and recommendations. Lancet Respir Med (2020) 8:506–17. doi:10.1016/s2213-2600(20)30161-2
22.Reporte de la Secretaria de Salud MEXICO (8 de Abril 2020). Versión estenográfica. Conferencia de prensa. Informe diario sobre coronavirus COVID-19 en México (2020).
24. DuWeng R, Price K. Differential Evolution–a simple and efficient adaptive scheme for global optimization over continuous spaces. J Global Optim (1997) 11:341–59. doi:10.1023/A:1008202821328
25. Parra-Rojas C, Hernandez-Vargas EAPDE. Parameter fitting toolbox for partial differential equations in python. Bioinformatics (2019) 36(8):2618–9. doi:10.1093/bioinformatics/btz938
26. General de Epidemiología D. Datos Abiertos para COVID-19–dirección General de Epidemiología |Secretaría de Salud | Gobierno | gob.mx.
27. Velázquez Ramírez MDC. Capacidad instalada en México para enfrentar al coronavirus COVID-19 (2020).
28. Levaillant M, Lièvre G, Baert G. Ending diabetes in Mexico. Lancet (2019) 394:467–8. doi:10.1016/S0140-6736(19)31662-9
29. Britton T, Tomba GS. Estimation in emerging epidemics: biases and remedies. J R Soc Interface (2019) 16:20180670. doi:10.1098/RSIF.2018.0670
30.OECD. Testing for COVID-19. A way to lift confinement restrictions. OECD (2020). Tech. Rep. April.
Keywords: COVID-19, confinement, control strategies, epidemiology, risk, Mexico
Citation: Azanza Ricardo CL and Hernandez-Vargas EA (2020) Epidemiological Characteristics of COVID-19 in Mexico and the Potential Impact of Lifting Confinement Across Regions. Front. Phys. 8:573322. doi: 10.3389/fphy.2020.573322
Received: 16 June 2020; Accepted: 15 September 2020;
Published: 21 December 2020.
Edited by:
Lin Wang, University of Cambridge, United KingdomReviewed by:
Sen Pei, Columbia University, United StatesLin Yang, Hong Kong Polytechnic University, Hong Kong
Gaoxi Xiao, Nanyang Technological University, Singapore
Copyright © Azanza Ricardo and Hernandez-Vargas. This is an open-access article distributed under the terms of the Creative Commons Attribution License (CC BY). The use, distribution or reproduction in other forums is permitted, provided the original author(s) and the copyright owner(s) are credited and that the original publication in this journal is cited, in accordance with accepted academic practice. No use, distribution or reproduction is permitted which does not comply with these terms.
*Correspondence: Esteban A. Hernandez-Vargas, esteban@im.unam.mx