- 1BioImaging Austria/CMI, Vienna BioCenter Core Facilities, Vienna, Austria
- 2Université de Nantes, CNRS, INSERM, l'institut du thorax, Nantes, France
- 3Nantes Université, CHU Nantes, Inserm, CNRS, SFR Santé, Inserm UMS 016, CNRS UMS 3556, Nantes, France
- 4Upper Austria University of Applied Sciences, Medical Engineering, BioImaging Austria/CMI, Linz, Austria
- 5Center for Advanced Preclinical Imaging (CAPI), First Faculty of Medicine, Charles University, Prague, Czechia
- 6School of Biochemistry, University of Bristol, Bristol, United Kingdom
- 7Department of Preclinical Imaging and Radiopharmacy, Werner Siemens Imaging Center, Eberhard-Karls University Tübingen, Tübingen, Germany
- 8Department of Physics and Astronomy, University of British Columbia, Vancouver, BC, Canada
- 9Cluster of Excellence iFIT (EXC 2180) “Image Guided and Functionally Instructed Tumor Therapies”, University of Tuebingen, Tübingen, Germany
- 10Ludwig Boltzmann Institute for Experimental and Clinical Traumatology, BioImaging Austria/CMI, Vienna, Austria
- 11Center for Medical Physics and Biomedical Engineering, BioImaging Austria/CMI, Medical University of Vienna, Vienna, Austria
- 12Mass Spectrometric Bio- and Polymeranalysis, Institute of Chemical Technologies and Analytics, BioImaging Austria/CMI, TU Wien, Vienna, Austria
- 13Laboratory of MacroMolecular Cancer Therapeutics (MMCT), Department of Pharmaceutical Chemistry, Center of Pharmaceutical Sciences, BioImaging Austria/CMI, University of Vienna, Vienna, Austria
- 14VRVis Zentrum für Virtual Reality und Visualisierung Forschungs GmbH, BioImaging Austria/CMI, Vienna, Austria
- 15Laboratory of Advance Microscopy LAB, Faculty of Engineering, Institute of Nanotechnology and Advanced Materials, Bar Ilan University, Ramat Gan, Israel
- 16Division of Anatomy, Center for Anatomy and Cell Biology, BioImaging Austria/CMI, Medical University of Vienna, Vienna, Austria
- 17VetCore Facility for Research, Imaging Unit, BioImaging Austria/CMI, University of Veterinary Medicine Vienna, Vienna, Austria
- 18Preclinical Molecular Imaging, BioImaging Austria/CMI, AIT Austrian Institute of Technology GmbH, Seibersdorf, Austria
The frontiers of bioimaging are currently being pushed toward the integration and correlation of several modalities to tackle biomedical research questions holistically and across multiple scales. Correlated Multimodal Imaging (CMI) gathers information about exactly the same specimen with two or more complementary modalities that—in combination—create a composite and complementary view of the sample (including insights into structure, function, dynamics and molecular composition). CMI allows to describe biomedical processes within their overall spatio-temporal context and gain a mechanistic understanding of cells, tissues, diseases or organisms by untangling their molecular mechanisms within their native environment. The two best-established CMI implementations for small animals and model organisms are hardware-fused platforms in preclinical imaging (Hybrid Imaging) and Correlated Light and Electron Microscopy (CLEM) in biological imaging. Although the merits of Preclinical Hybrid Imaging (PHI) and CLEM are well-established, both approaches would benefit from standardization of protocols, ontologies and data handling, and the development of optimized and advanced implementations. Specifically, CMI pipelines that aim at bridging preclinical and biological imaging beyond CLEM and PHI are rare but bear great potential to substantially advance both bioimaging and biomedical research. CMI faces three main challenges for its routine use in biomedical research: (1) Sample handling and preparation procedures that are compatible across modalities without compromising data quality, (2) soft- and hardware solutions to relocate the same region of interest (ROI) after transfer between imaging platforms including fiducial markers, and (3) automated software solutions to correlate complex, multiscale, multimodal and volumetric image data including reconstruction, segmentation and visualization. This review goes beyond preclinical imaging and puts accessible information into a broader imaging context. We present a comprehensive overview of the field of CMI from preclinical hybrid imaging to correlative microscopy, highlight requirements for optimization and standardization, present a synopsis of current solutions to challenges of the field and focus on current efforts to bridge the gap between preclinical and biological imaging (from small animals down to single cells and molecules). The review is in line with major European initiatives, such as COMULIS (CA17121), a COST Action to promote and foster Correlated Multimodal Imaging in Life Sciences.
Introduction
The ideal imaging setup would provide both (i) holistic and (ii) multiscale information about the same sample:
Holistic imaging refers to probing all relevant information spaces for the same sample, assessing both structural and functional information (Figure 1). Functional imaging allows to portray dynamic physiological, metabolic and biological processes within the sample, such as diffusion, perfusion or glucose uptake. This requires both sensitivity to low molecular concentrations and specificity, the number of potential molecules resolved per scan (Figure 2). Since these processes occur in a complex tissue environment, ideally, this information is acquired in-vivo or in a close-to-native context without damaging the sample by irradiation. This requires trade-offs in bioimaging using single modalities since usually either structural or functional information is gathered by a single modality, and high-resolution localization with protein or ultrastructural accuracy often requires sectioning the sample and prevents in-vivo studies.
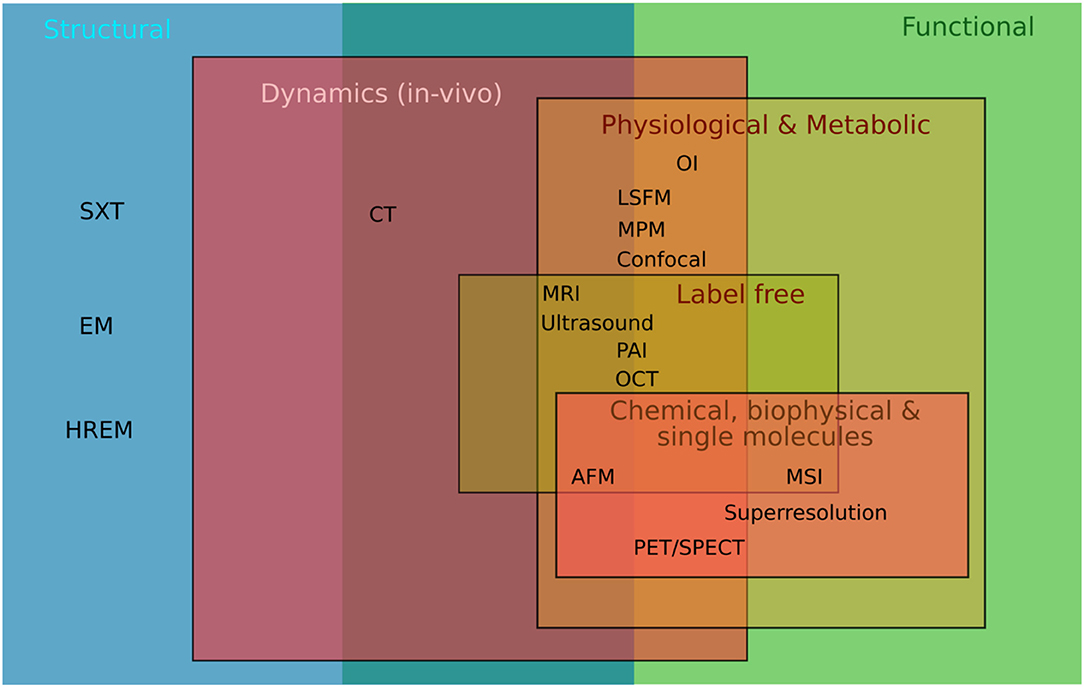
Figure 1. Holistic imaging accessing all relevant information spaces from structural to functional imaging. Representation for the modalities mentioned in the text: SXT, Soft X-ray Tomography; HREM, High Resolution Episcopic Microscopy; EM, Electron Microscopy; CT, Computed Tomography; OI, Optical Imaging including bioluminescence and fluorescence imaging; LSFM, Light Sheet Fluorescence Microscopy; MPM, MultiPhoton Microscopy, Confocal, Confocal Microscopy; MRI, Magnetic Resonance Imaging; Ultrasound, PAI, Photoacoustic Imaging; OCT, Optical Coherence Tomography; AFM, Atomic Force Microscopy; MSI, Mass Spec Imaging; Superresolution, Microscopy such as STORM, PALM, STED; PET/SPECT, Positron Emission Tomography/Single Photon Emission Computed Tomography.
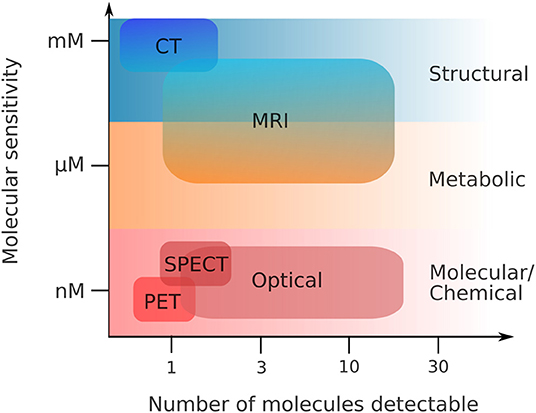
Figure 2. Functional imaging—sensitivity vs. specificity. Same abbreviations as in Figure 1. Adapted from Pogue et al. [1], with permission from the American Journal of Roentgenology (copyright owner).
Multiscale structural imaging visualizes the same sample across all relevant scales. Ideally, it combines high axial and lateral resolution with high penetration depths, and is able to image or scan a wide field of view in a reasonable time that allows the correlation of complementary parameters acquired across the entire sample. However, bioimaging is usually performed using single modalities, which restricts multiscale imaging: Either a large field of view is imaged at low magnification, which provides overview and tissue context but restricts localization, or the specimen is imaged at high resolution, which provides (sub)cellular insights but limits contextual information. Besides, penetration depth comes at the expense of lateral resolution (Figure 3) and is limited due to aberration and attenuation by scattering and absorption (with highly wavelength-dependent elastic (Rayleigh) scattering—the intensity of Rayleigh-scattered light is I ∝1/λ4), and hence restricts 3D in-vivo imaging. The achievable penetration depth is proportional to the scattering mean free path, and strongly depends on the composition of the biological tissue, such as the presence and organization of microvasculature or collagen [2].
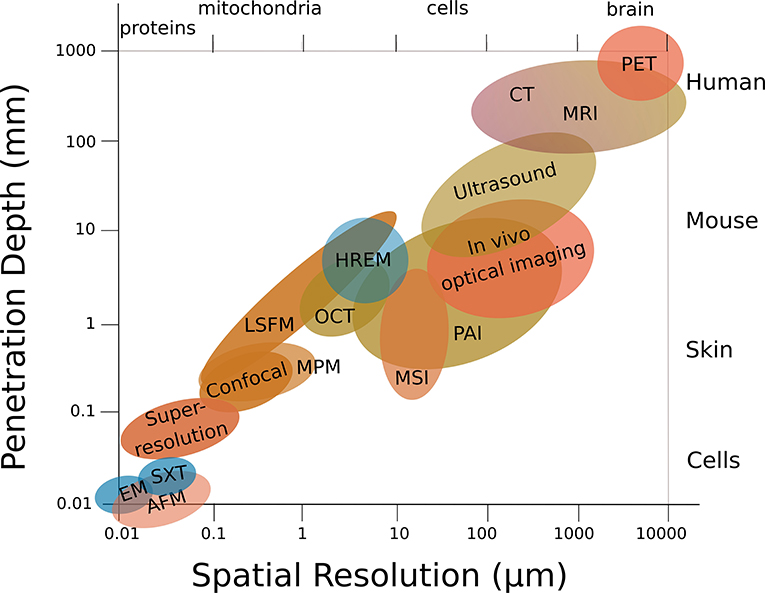
Figure 3. Structural multiscale imaging. Penetration depth usually comes at the expense of lateral resolution. Same abbreviations as in Figure 1.
No single modality can gain multiscale or holistic information and accurately and comprehensively decipher the inner working of cells or entire organisms. Only the combination and—importantly—the multimodal correlation of imaging technologies allow to overcome the limitations mentioned above by integrating the best features of the combined techniques (compare Tables 1, 2, 3). Correlated Multimodal Imaging (CMI) gathers information about the specimen with two or more complementary modalities that—in combination—create a composite view of the sample. It is a holistic multiscale approach that spans the entire resolution range from nano- to millimeters, and provides complementary information about structure, function, dynamics, and molecular composition of the sample. CMI can hence study biomedical processes within their overall spatio-temporal context, and mechanistically analyze pathologies, diseases and organisms down to the underlying molecular events. Correlative Light and Electron Microscopy (CLEM), as a well-established case of CMI, can for example gather both spatial (Electron Microscopy, EM) and functional information about a specific molecule (Fluorescence Microscopy, FM) within its subcellular context, and achieve near-atomic resolution (EM) within a relatively broad field of view (FM). Additionally, due to its complementarity and different contrast mechanisms, CMI allows to validate quantifications and conclusions drawn from any single modality.
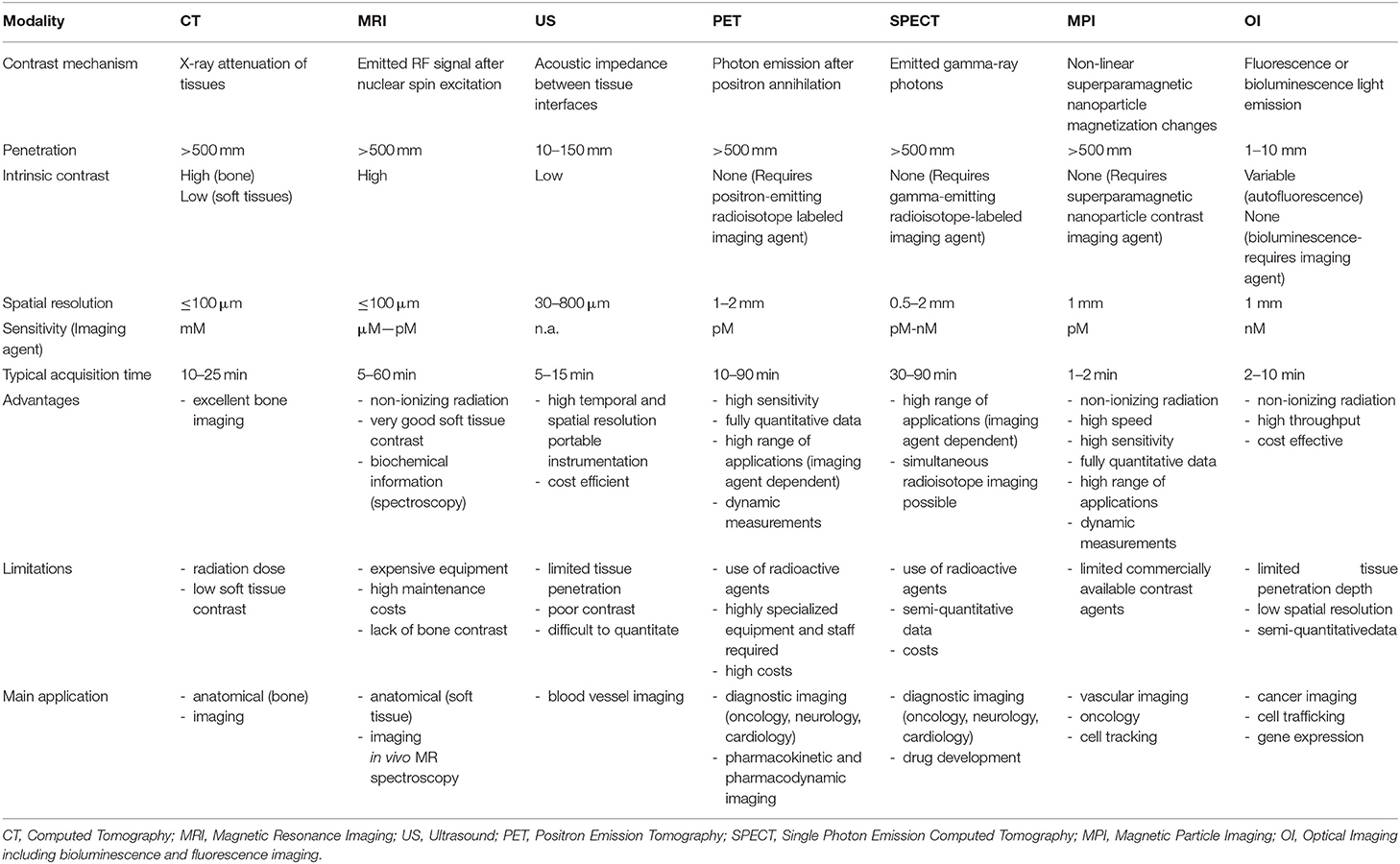
Table 1. Imaging parameters, advantages and limitations of the most used in-vivo preclinical imaging techniques.
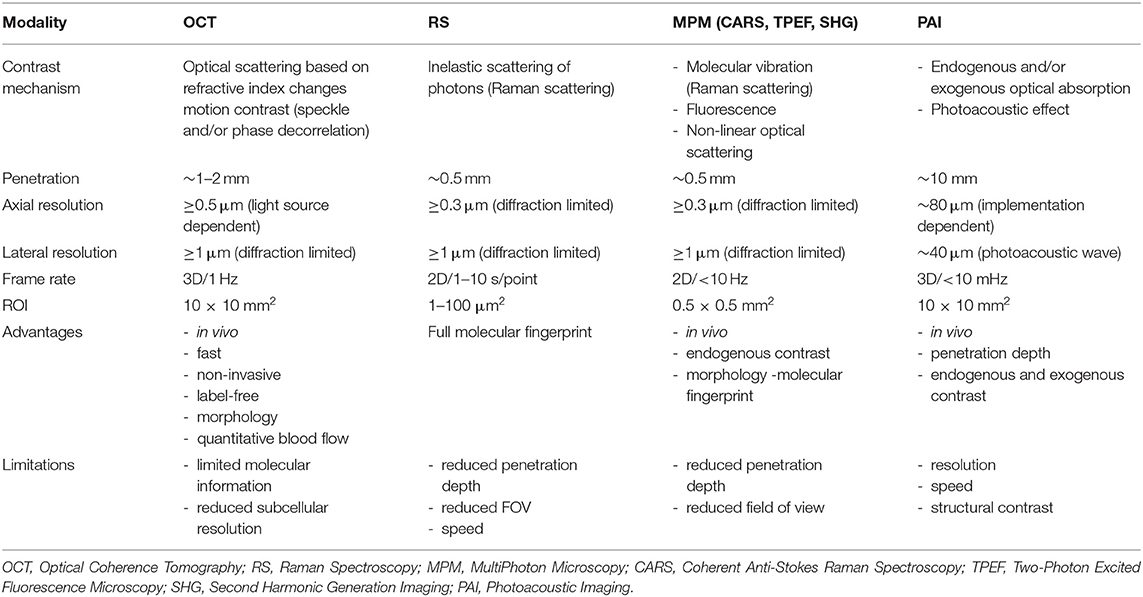
Table 2. Imaging parameters, advantages and limitations of the most used in-vivo microscopy techniques in preclinical research.
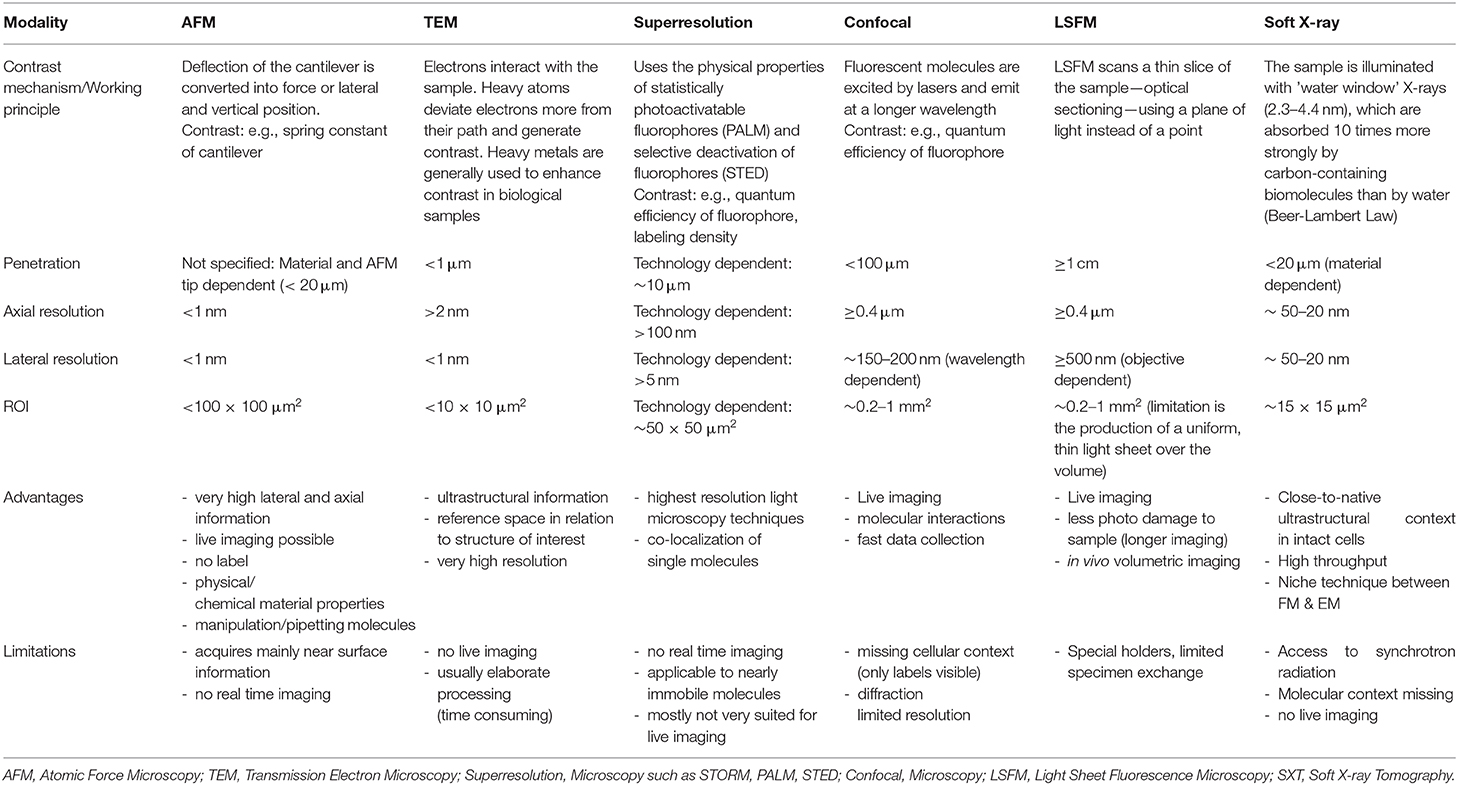
Table 3. Imaging parameters, advantages, and limitations of the most used ex-vivo microscopy techniques in biological research.
For this review, we solely focus and distinguish between preclinical (imaging small animals and molecular processes in-vivo) and biological imaging (largely microscopy, ex-vivo visualization of subcellular processes and molecules, cells or tissues of model organisms). So far, CMI approaches in biological and preclinical research mainly focus on the correlation of two modalities [3]. There is one well-established example for each field: (1) Hardware-fused platforms for Hybrid Imaging [4] in preclinical research and diagnostics (which we refer to as Preclinical Hybrid Imaging, PHI), and (2) Correlative Light and Electron Microscopy (CLEM) in biological research [5]. The most prominent (and commercially available) implementations surely are micro-Positron Emission Tomography and micro-Computed Tomography (PET/CT) and Single Photon Emission Tomography (SPECT)/CT, but there is a large variety of other CMI combinations both in preclinical research and correlative microscopy which will broaden the accessible biomedical information significantly. The field of CMI is highly dynamic and heading toward more complex integrated implementations of multimodal workflows that also include advanced non-commercial setups, such as Soft X-Ray Tomography in biological imaging. Currently, however, there are only very few strategies that aim at bridging biological and preclinical imaging—even though these Novel CMI Pipelines reap the full potential of this approach in tackling biomedical research questions mechanistically. In this context, data handling and Correlation Software for diverse imaging data sets play a crucial role in CMI.
While the benefits of PHI and CLEM are more and more recognized in biomedical research, they lack gold standards for protocols or data handling and limit quantification. This includes for example the quantification of the correlation accuracy in CLEM or biomedical imaging ontologies. Apart from standardization, both PHI and CLEM leave room for optimization and the integration of advanced setups, such volume or super-resolution CLEM [6, 7] or hybrid preclinical multimodal platforms for Optical Coherence Tomography (OCT), Photoacoustic Imaging (PAI), and non-linear in-vivo microscopy [8]. For the routine implementation of CMI in biomedical research, several common bottlenecks need to be overcome, such as sample handling and preparation procedures that are compatible across modalities without compromising data quality, soft- and hardware solutions to relocate the same region of interest (ROI) after transfer between imaging platforms including fiducial markers, and automated software solutions to correlate complex, multiscale, multimodal and volumetric image data including reconstruction, segmentation and visualization. Due to these challenges and lack of gold standards, availability of CMI in routine biomedical research is limited. Specifically for novel CMI pipelines, the involved cutting-edge imaging technologies can be expensive and time-consuming—and simply not available to a single researcher. They require a broad range of interdisciplinary expertise across different imaging modalities from sample preparation to image processing. Besides, it is difficult for the user to keep track of the constantly expanding range of available modalities and their strengths and limitations. The use of CMI in biomedical research is also restricted by the lack of readily accessible commercial solutions that allow to address biomedical research questions without substantial technological R&D.
CMI will play a crucial role in the future of bioimaging and in life sciences, which is reflected by major European initiatives, such as the European Society for Hybrid Imaging or COMULIS, an EU-funded COST network that aims fostering CMI, disseminating its benefits, and accelerating its technological implementation as a versatile tool in biomedical research by addressing the mentioned challenges and bottlenecks.
State-of-the-Art
CLEM and Correlative Microscopy
Ever since the first analysis of a biological sample using EM by Porter et al. [9], the light microscope was used first to target the cell of interest. This highlights one of the hallmarks of the power of CLEM: The identification of a specific event to be analyzed at higher resolution in the electron microscope. Since then, CLEM has been applied to answer specific biological questions, most notably the seminal work by Rieder, using (live) Differential Interference Contrast (DIC) light microscopy to study microtubule organization during cell division [10]. CLEM took off shortly after the groundbreaking use of Green Fluorescent Protein (GFP) [11] that transformed life science research. The groups of Polishchuk et al. [12, 13] used the expression of GFP tagged to a viral protein (VSV-G) to first study the movement of post-Golgi transport carriers and subsequently analyze that exact same carrier at high resolution in EM. This workflow nicely exemplified that by combining the power of each technique, the sum is greater than its parts (1+1 = 3, [14]). Live imaging by FM provided the history of the carrier (originating from the Golgi) and EM not only showed the ultrastructure of the carrier but in addition provided information about its surrounding environment as a bonus, the so-called reference space. One of the great advantages of this workflow is its relative simplicity. It makes use of an imaging dish with a finder pattern embossed in it. The pattern can be recorded in the light microscope (LM) and as the finder pattern stands out from the rest of the glass coverslip, the pattern is also transferred to the resin block. This allows for trimming down the sample to only a very few cells around the cell of interest [15]. In principle, any lab with a light and an electron microscope will be able to perform this technique.
There are many different approaches to a CLEM experiment given the diversity of EM (TEM, SEM, electron tomography) and FM techniques (LSFM, MPM, super-resolution, confocal), which can be roughly classified in chemical fixation and embedding (in-resin) approaches, and cryo approaches (see e.g. Table 3). We have compiled a large number of those in a series of three books in the Methods in Cell Biology series (Volume 111, 124, and 140). Of particular interest for routine use is the preservation of in-resin fluorescence. This method retains the fluorescence (of GFP) after high pressure freezing and freeze substitution to Lowicryl [16–18]. So, after sectioning first, the fluorescence can be recorded with high Z-resolution because the section is only 70–100 nm thick and then can be mapped with high precision (50 nm) onto the underlying ultrastructure. An interesting development here is the integrated light and electron microscope that would allow for even better and more direct correlation as discussed later. It is important to highlight that the development of each of those techniques is driven by the need to answer a biological question and it should always be the case that this biological question is driving what kind of technology will be applied. As an example, we have been studying the formation of membrane tubules emanating from endosomes that transport and recycle cargo back to the plasma membrane. Chemical fixation as done by the pre-embedment approach described earlier [15] causes the tubules to fragment into smaller carriers, thus destroying the very object of study [19]. Hence a cryo-fixation method had to be developed that allows for capturing events observed live in the fluorescence light microscope on a time scale of seconds to be observed down the electron microscopy. This resulted in the development of the EMPACT2 + RTS with Leica Microsystems and allowed us and others to capture short-lived cellular events for study at the ultrastructural level [19–21]. Apart from CLEM, other well-established examples of correlative microscopy include the combination of FM and AFM. Besides, the combination of Soft X-ray Tomography (SXT) with FM and its super-resolution implementations allows to correlate two complementary contrast mechanisms at similar spatial resolution, and was used to study cell infections or the molecular distributions within the ultrastructural architecture [22, 23].
Advanced super-resolution FM circumvents the diffraction barrier with spatial resolution below 200 nm and has become a powerful tool for the observation of specific molecules in living cells, tissues, and even whole organisms. It is a valuable tool to study bio-molecular dynamics, interactions and co-localization via selective and specific labeling of certain components within cells and to provide biological information at the nanoscale by measuring forces of interacting objects. However, even with the recent implementation of high-speed AFM, the temporal resolution of fluorescence-based techniques cannot be reached. Likewise, the introduction of super-resolution microscopy cannot reach the spatial resolution of AFM. However, the combination of “Force-and-Light” allows to watch and simultaneously manipulate or control individual molecules. These two techniques in combination allow probing fundamental biological processes at a previously unrepresented level. In general, it is possible to combine all parameters gained from AFM techniques with those of FM ones (Figure 4). Nevertheless, it needs to be considered which combinations yield meaningful insights into the investigated system, and more importantly, which combinations do not influence each other—such as measuring of interaction forces and interaction kinetics as the kinetics will be directly altered by an applied force. Moreover, synchronized operations of both techniques instead of sequential ones are limited by the individual mechanical stability of each technique (e.g., thermal drift, acoustic disturbance, mechanical, and electronic noise/vibration).
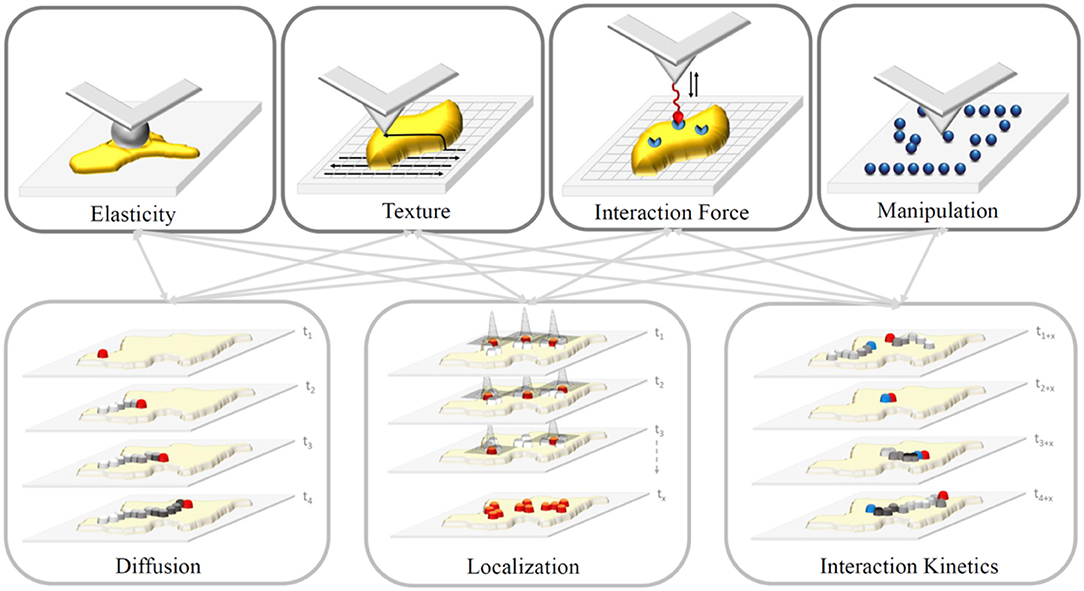
Figure 4. Sketch of properties gained from AFM (upper row) and FM-based (lower row) techniques. Gray arrows depict possible combinations of properties. In general, we can differentiate acquired information premised on AFM, whether they are generated via force spectroscopy (measurement of interaction forces via functionalized tips or elasticity via ball-bearing tips) or by spatial scanning (sample texture or feature manipulation depending on the applied force) of the sample (yellow). In contrast, FM-based techniques study movement (e.g., diffusion) and interaction kinetics of molecules, and localize these particles down to an accuracy of a few nanometers depending on the number of detected photons.
To date, various combinations have been successfully confirmed to characterize previously inaccessible biological information. For example, the AFM tip was used as a nanopipette to supply targeted molecules to bio-membranes and its temporal interaction was described using FM [24, 25]. This approach allows for a so-called touch-and-watch experiment to study the uptake of foreign or active substances for example. Besides, there are a variety of correlative applications, which assessed combinations of the properties depicted in Figure 4: (i) Elasticity and diffusion using for example Förster Resonance Energy Transfer (FRET) between dye molecule [26–30]; (ii) interaction forces and diffusion using single molecule force spectroscopy and Total Internal Reflection Microscopy (TIRF), termed Single Molecule Cut and Paste, to assemble/split nucleotide-based aptamers individually [25, 31, 32]; (iii) interaction forces and localization using super-resolution FM to resolve the architecture of focal adhesion under physiological relevant conditions [33, 34]; and manipulation and localization to assemble single molecules patterns via the AFM to identify blinking parameters and maximal resolvable fluorophore density [35, 36].
Preclinical Hybrid Imaging
Preclinical imaging of small laboratory animals covers all clinically used methods for human in-vivo imaging and also several methods that have not been implemented to humans yet. In-vivo imaging consists of anatomical (structural) imaging and molecular (functional) imaging.
Anatomical Imaging of body structures utilizes X-rays (CT—Computed Tomography), magnetic properties of tissues (MRI—Magnetic Resonance Imaging) or interacting of tissues with sound/pressure waves (US—ultrasonography or ultrasound imaging). CT images correspond to differential attenuation of X-rays depending on the density of interacting structures. CT images are characterized by very good spatial resolution but low contrast—so they are used preferentially for imaging of hard structures (bones). MRI imaging is based on nuclear magnetic resonance of hydrogen nuclei (protons) in oscillating magnetic fields. MRI provides inferior spatial resolution compared to CT but excellent soft tissue resolution [37]. Ultrasound waves penetrate soft tissues and form echoes on the boundary of tissues with different acoustic impedance. This allows for imaging of soft tissues such as muscle, tendon, veins, and inner organs. Higher frequency waves (40 to 70 MHz) penetrate little into the tissue (10–15 mm) but provide excellent spatial resolution down to 30 μm. US imaging is generally 2D but can be acquired and computed to form a 3D and 4D data set [38].
Molecular Imaging localizes a position of accumulated molecules (contrast agents). All three anatomical in-vivo techniques can be enhanced to molecular imaging by the use of contrast agents. Even without the use of contrast agents, MRI can track changes in blood flow and oxygenation of brain tissue connected to increased brain activity after stimulus and thus reveal the brain regions activated by such stimulus [39]. Ultrasound Doppler imaging can also detect functional changes in blood flow without contrast application.
Other pure molecular in-vivo imaging methods utilize radioisotopic, magnetic, optical or optoacoustic contrasts. The obtained images only show regions of contrast accumulation and must be co-registered with anatomical images to validate the exact position of the signal in the body, i.e. always require correlative or hybrid imaging approaches. Radioisotopic imaging methods include PET and SPECT. PET data acquisition is based on positron-emitting radioisotopes, in which the positron travels a short distance in the surrounding tissue, then annihilates with an electron forming a pair of high energy photons (511 keV), which travel in opposite directions and are detected by a ring of detectors surrounding the object of interest. The coincident signals are recorded and the position of radioisotopic contrast lays on the connecting line of the two detected photons. The mean distance of the emission and annihilation positions (positron range) is dependent on the energy of the PET isotope and the attenuation properties of the surrounding tissue [40]. In contrast to PET, SPECT imaging is based on single photon emitting isotopes that are detected by a gamma camera. To determine the direction from where the photon traveled, the collimator (typically made out of lead or tungsten) with single or multiple pinholes or slits must be placed between the imaged object and detector. Only photons that pass the collimator are detected. Based on the trajectory between the collimator and the detector, the position of annihilation can be reconstructed. SPECT isotope energies typically range between 30 and 300 keV and allow multi-isotope imaging when utilizing isotopes with no overlapping energy windows. SPECT and PET imaging have intrinsically different properties in terms of sensitivity and spatial resolution. While in SPECT, the collimator design vastly limits the number of detected photons and hence the sensitivity, PET imaging does not need a collimator and moreover, benefits from the ring design of detectors around the object of interest since the two photons are detected concomitantly by two detectors opposing the site of annihilation. Hence, sensitivity in PET is superior to SPECT sensitivity. Spatial resolution in PET, however, is limited by positron range and crystal size, whereas in SPECT, since the photon originates directly from the nucleus, spatial resolution is theoretically superior to PET resolution. However, spatial resolution and sensitivity are dependent on multiple factors, such as choice of isotope, crystal material, utilized detectors, etc. and especially in SPECT, collimator choice must be adapted based on the desired application. In order to diminish the effects of ionizing radiation, radioisotopes with short half-lives are used for PET and/or SPECT imaging. PET and SPECT scanners are usually constructed as hybrid devices (PET/CT, SPECT/CT, or PET/SPECT/CT). Recently, new PET detector materials compatible with magnetic resonance allowed the construction of PET/MRI hybrid scanners. The advantage of such scanners is excellent soft tissue contrast for precise localization of signal within organs, and the absence of CT imaging allows to diminish the radiation dose accumulated in imaged objects.
Magnetic properties of contrast agents are the basis for magnetic particle imaging (MPI) and electron paramagnetic resonance (EPR) imaging. MPI measures the position of superparamagnetic nanoparticles by detecting their non-linear magnetization response to oscillating magnetic fields [41]. The method ensures positive contrast localization with high spatial and temporal resolution. EPR imaging is similar to nuclear magnetic resonance; electron spins are affected instead of atomic nuclei spins. Different excitation frequencies are used compared to MRI (mostly in the microwave range). Absolute oxygen levels, reactive oxygen species (ROS), oxidative stress or spin probes can be determined in vivo by this method [42]. Magnetic molecular methods are usually co-registered with MRI or CT [43].
Optical imaging (OI) is fast and relatively cheap imaging of fluorescence and/or luminescence signals. Fluorescent probes are excited by matching wavelengths and emit fluorescent signal. The limitation of the method is the low light penetration through the tissues. The measured signal is thus not quantitative with more light loss for deeper probe localization. As hemoglobin (oxy- and deoxy-) absorbs the light in wavelengths below 650 nm, the optimal imaging window opens in the near-infrared (NIR) region (650–1,350 nm). Water absorbs at longer wavelengths [44]. Luminescence based on cellular expression of luciferase enzymes converting substrates to visible light gives superior images because it avoids illumination and corresponding tissue autofluorescence [45]. Fluorescent images are co-registered to hybrid images using brightfield or X-ray anatomical imaging. While OI was initially limited to 2D imaging, several technologies have been developed in recent years which allow 3D tomographic imaging in combination with morphological imaging based on CT. For preclinical OI, firefly luciferase is the most commonly used transgene allowing longitudinal studies on e.g., promoter activity in transgenic mice, growth and dissemination of implanted tumors [46] or biodistribution and proliferation of organisms in infection models [47]. Kuo et al. published the first tomographic imaging setup for luciferase imaging, termed diffuse luminescence imaging tomography (DLIT) [48]. Such systems are now also available with built-in CT capability, where CT data are used to determine surface topology. When implanting luciferase labeled tumor cells, this technology allows proper signal allocation to organs when combined with CT contrast agents. NIR fluorescence imaging (NIR) is not only applied in preclinical but also in clinical applications, e.g., for image guided surgery [49]. For the absorption range of 700–900 nm, several fluorophores, nanoprobes and reporter genes have been developed. Another emerging area in OI is the use of the so-called second NIR window (NIR-II) ranging from 1,000 to 1,700 nm [50]. This wavelength range enables imaging with improved tissue penetration depth and spatial resolution, but also minimized tissue autofluorescence and reduced scattering. Correlative imaging of NIR fluorescence and CT is enabled by applying fluorescence molecular tomography imaging (FMT, [51]). Using a commercialized system, tomographic imaging is achieved by acquiring multiple fluorescence images from different positions in transmission mode.
Another molecular imaging method is photoacoustic imaging (PAI). The laser NIR pulses penetrate into the tissue and deliver energy to photoacoustic contrast molecules which undergo a thermoelastic expansion [52]. This expansion then generates ultrasound waves detected by the ultrasound probe. There are specific endogenous contrasts (oxyhemoglobin, deoxyhemoglobin, melanin) and exogenously delivered photoacoustic contrasts for labeling of cells, vasculature, tumors etc. The contrasts give specific positive signal on the ultrasonic background. While other preclinical molecular imaging methods have a spatial resolution around 1 mm, photoacoustic imaging can produce images with a resolution of 50 μm or less. Nevertheless, the method is limited by the effective light penetration about 10 mm in soft tissues.
While CT has traditionally been used to assess morphologies in bone tissue, it holds more potential to the field of correlative imaging. Going beyond the depiction of mineralized tissues, it provides 3D reference volumes in integrated PET/CT, SPECT/CT or OI/CT devices that readily provide registered, multimodal data sets. Furthermore, CT can be used in a post-mortem, high-resolution, soft-tissue approach. Vascular structures can be visualized at high resolution in 3D via contrast agent perfusion [53], and contrast-enhanced microfocus CT (CE-CT) allows for simultaneous visualization of bone and soft tissues [54]. This makes CT a potent tool for both (a) integrated in-vivo applications providing longitudinal, registered 3D volumes in limited resolution and contrast, and (b) high-resolution post-mortem imaging with soft tissue contrast for 3D anatomical and pathological correlation.
Another important multimodal imaging approach that is gaining importance in preclinical settings as it preserves the tissue is label-free (optical) imaging and non-invasive, aseptic assessment of tissues and cells in-vivo at high resolution. While FM relies on specific contrast or the application of dyes or fluorescent proteins to highlight certain structures, most molecules do not exhibit intrinsic contrast and the application of dyes or fluorescent proteins might interfere with function and is typically limited to three to four colors due to spectral overlap, which makes it difficult to discriminate between the labeled structures or cells. Especially, optical coherence tomography (OCT) has matured over the last three decades to a potent non-invasive, high-resolution, label-free interferometric optical diagnostic imaging modality enabling video-rate in vivo cross-sectional tomographic visualization of structures with resolution comparable to histopathology, serving as in vivo optical biopsy [55, 56]. Despite the large potential of OCT, sensitivity and specificity to detect pathologic tissue is restricted and the correlation with other techniques is required. Raman spectroscopy (RS) complements OCT by giving a quantitative measure of the full molecular fingerprint of biomolecules such as lipids, proteins, carbohydrates, and nucleic acids, but is intrinsically slow. It relies on the effect of inelastic scattering of photons, stimulating molecular vibrations providing specific information on the chemical composition and molecular structure, and is an emerging technique in life sciences owing to its unique capability of generating spectroscopic fingerprints of cells and tissues in a non-destructive and label-free approach. It has been demonstrated that the combination OCT/RS on cancerous tissue can increase diagnostic sensitivity, specificity and accuracy compared to a single modality [57]. Two-photon excited fluorescence (TPEF) microscopy can be used to visualize endogenous fluorophores such as NADH and FAD giving information about redox states. Additionally, second harmonic generation (SHG) imaging is well-suited to image collagen fibers. Since SHG signals arise from induced polarization rather than from absorption, this leads to significantly reduced photobleaching and phototoxicity compared to fluorescence methods. SHG microscopy in combination with TPEF microscopy can monitor collagen structure changes and cellular metabolic activity in vivo during wound healing [58]. Hybrid multimodal multiphoton microscopy (MPM) [59] with single-photon sensitivity and submicron spatial resolution using the response of endogenous chemical biomarkers in skin, such as collagen or lipids acts as fast and label-free in vivo optical biopsy [60]. The synergistically combination of OCT with nonlinear optical imaging techniques such as TPEF, SHG, and CARS (Coherent Anti-Stokes Raman Spectroscopy) provides access to detailed information of tissue structure and molecular composition in a fast, label-free and non-invasive manner [61]. MPM offers high axial resolution with molecular contrast but limited speed and penetration depth. Combining MPM with OCT [62] adds wide-field morphologic information to the chemical fingerprint [63]. As described above, PAI can overcome penetration and scanning range limits of OCT, allowing imaging of deep vasculature [64, 65]. PAI can monitor angiogenesis, map blood oxygenation with sub-100 μm resolution and centimeter penetration depth. In combination with OCT it adds valuable vascular information in depth to the ultrahigh-resolution images [66, 67].
The combination of these optical modalities not only overcomes the limitations of isolated, standard imaging approaches, but also provides unique and complementary information (see Tables 1, 2) which is only achievable through the correlation of these data.
Novel CMI Pipelines
Since CMI allows to gain structural, functional, dynamical and chemical information about a single sample for a well-defined time point or even time lapse series across all relevant length scales and levels of biological organization, it is the most suitable approach to gain otherwise inaccessible insights into a huge variety of intricate biological processes and understand them within their complex (micro)environment. So far, common CMI approaches mainly focus on the combination of two modalities with two prevalent examples in biological imaging (CLEM) and preclinical research (PHI) that allow to combine functional with structural information from a singular event or within a single study (see Introduction). In PHI, two complementary imaging modalities are fused within a single setup, such as PET/CT or PET/MRI. PHI serves as a valuable diagnostic and research tool that can uncover molecular processes and biochemical pathways in living animals non-invasively within their anatomy, and has been used to study wide variety of biomedical questions, for example in cancer biology or brain research [68]. CLEM has become the method of choice to analyze rare and specific processes within tissues or cell lines, and has been used to study a wide variety of biological questions including membrane trafficking and viral pathways [17]. The maturity of the two fields is reflected in several commercial implementations for PHI (e.g., PET/CT or SPECT/CT), and a first commercially available integrated fluorescence and scanning electron microscope and commercial tools, ancillary equipment and software for CLEM to facilitate re-locating of the region of interest across modalities (see section State-of-the-Art). As illustrated in section State-of-the-Art, the limits of CLEM and PHI are currently pushed towards developing advanced implementation. For CLEM, these efforts include for example advanced FM approaches, such as (cryo)super-resolution or FM of thick tissues [6, 7]. Apart from CLEM, more and more other dual-modality combinations of microscopy technologies have been established during the last decade. Examples of these setups include various combinations of AFM with (advanced) FM (cp. section CLEM and Correlative Microscopy); combinations of soft X-ray tomography (SXT) and FM, for example to localize proteins involved in mitochondrial fission within their close-to-native subcellular context [22, 34, 69]; the correlation of mass spectrometry-based imaging (MSI) with EM to combine the inherently lower-resolution chemical images obtained from secondary ion mass spectrometry (SIMS) with the high-resolution ultrastructural images from EM [70]; and the combination of SIMS [71] and matrix-assisted laser desorption/ionization MSI (MALDI MSI) [72] with fluorescence in-situ hybridization (FISH) to link microbial phylogeny to metabolic activity at the single-cell level. Also, the newly developing field of molecular histology has to be mentioned that incorporates findings from MADLI MSI or infrared spectroscopy (IR) in classical histomorphology [73]. Besides, an increasing interest has arisen in research focused on elemental and molecular information that play crucial roles in both physiological and pathological metabolic processes. MALDI MSI was combined with laser-ablation inductively coupled plasma MS (LAICP MS) to study lipid changes colocalized with platinum, sulfur or phosphor distributions [74], and SIMS data were combined with topographical information from AFM to record accurate chemical 3D maps [75]. Advanced PHI includes R&D setups and pipelines that showcase combinations of in-vivo OI, OCT/PAI, US, MRI, CT, or PET [8, 76]. Examples as outlined in section Preclinical Hybrid Imaging include label-free imaging using OCT and RS [57], or the combination of MRI and OI [77].
Novel CMI pipelines go beyond correlative microscopy and PHI setups and usually include more than two complementary modalities. They aim at (1) bridging (preclinical) in-vivo imaging with ex-vivo biological microscopy to zoom in from a living sample to individual cellular structures and/or (2) adding localized spectroscopic [biophysical (e.g., mechanical properties or vibrational modes) or chemical (e.g., molecular or elemental)] information to the acquired structural and functional parameters. With all the electromagnetic spectrum explored for imaging, only incremental improvements in contrast, resolution, or sensitivity are expected for the available spectrum of imaging technologies (see e.g. Tables 1–3). To explore the multiple spatial and temporal scales necessary for a holistic understanding of organisms and their biology, novel CMI pipelines will be the method of choice. However, novel CMI pipelines with more than two modalities are in their infancy due to lack of access to a single researcher, the broad expertise required to oversee several modalities and due to lacking workflows and software solutions to track ROIs across modalities from living 3D tissue down to high lateral molecular resolution. While there are several EU-funded initiatives that aim at improving accessibility of advanced imaging technologies and interdisciplinary imaging expertise (such as Euro-BioImaging or COMULIS), such novel CMI pipelines nevertheless require substantial method development. Due to the diverse plethora of potential combinations of imaging technologies, setting up universal correlation protocols for CMI pipelines is not feasible. Sample preparation procedures for ex-vivo microscopy differ substantially across the technologies and even within a modality; AFM images alone, for example, can be acquired under various conditions (vacuum, atmosphere, and liquid). Correlative imaging usually requires modality-specific preparation and setup trade-offs, such as between preservation of fluorescence and subcellular architecture for CLEM, between the AFM laser and excitation spectra of the used fluorophores to avoid bleaching for correlative AFM [78], or between preservation of fluorescence and X-ray contrast for correlative CT. Dependent on the used technology, the sample preparation needs to be adapted.
In respect to correlation strategies, universal protocols to assess correlation accuracy are not implemented and restrict finding the same ROI after relocation between imaging platforms or co-alignment of data sets. Strategies to improve correlation of different technologies include (1) resolution matching of the technologies and (2) correlative markers that can be visualized in different imaging technologies.
A common approach to improving correlation accuracy in correlative microscopy is to match the FM resolution to that of the microscopy technique with the highest resolution (EM, SXT, AFM) by integrating super-resolution FM. For CMI pipelines that bridge in-vivo with ex-vivo imaging, usually intermediate (mesoscopic) resolution steps need to be implemented—as for example mesoscopic ex-vivo MRI for the integration of macroscopic in-vivo MRI data and microscopic CT data [79]. A common example includes the emerging use of CT in a different context: As an intermediate imaging technology to create a 3D template of the sample after in-vivo imaging and before sectioning of the sample to probe the ROI. CT can visualize thick tissues in 3D at micrometer resolution, tracks distortions and morphological changes of the ROI after embedding and fixation, and allows ROI identification even without (preserving) fluorescence. CT is specifically suited as an intermediate technology between in-vivo optical microscopy and EM since it can also visualize the sample in resin blocks due to the heavy-metal stains used for EM sample preparation. It qualifies for other correlative microscopy approaches as well since it can reveal endogenous landmarks, such as the vasculature, after barium sulfate perfusion.
While there are a variety of fiducial markers that can be used and tracked in correlative microscopy (such as QDs or dye-labeled nanoparticles), there are currently no correlative markers that can be visualized with high accuracy both by microscopy and preclinical imaging technologies. Besides, robust fiducial markers that might withstand electron bombardment or high X-ray doses are also lacking. A common approach to facilitate correlation when using CT as an intermediate modality is near-infrared branding (NIRB). Prior to CT, a pulsed, near-infrared laser is used to create defined 3D marks in the fixed tissue that can be traced by both FM and EM, and hence facilitates dissecting the sample to assess the ROI in a biopsy. In Karreman et al. [80], the position of the ROI was predicted with an accuracy of below 5 μm.
A typical correlation workflow for a CMI pipeline including for example, in-vivo optical microscopy, CT and EM typically might include the following steps, and will need to be adapted for the specific biomedical research question: (1) in-vivo functional imaging of molecular dynamics using FM, such as spinning disk, light sheet or multi-photon microscopy, or in-vivo imaging of metabolic processes using advanced preclinical imaging technologies, such MRI or OCT; (2) (a) near-infrared branding, sample fixation, dissection, and further EM processing or (b) dissection, high pressure freezing, and freeze substitution; (3) resin embedding (lowicryl if fluorescence is to be preserved); (4) CT for identification of ROI; (5) volume EM. This workflow must be adapted according to the desired biomedical outcome. To preserve the native ultrastructure, cryo-fixation (high-pressure freezing) might be desired. This might be followed either by freeze substitution or by a cryo-workflow with the aim to perform cryo-EM. Surely, preserving the fluorescence (either with LR-white or HM20 acrylic resins and adapted EM protocols or by keeping the sample under cryo-conditions) can be of advantage to re-locate ROIs. If considering serial section EM (or on-section CLEM), fiducial markers can be added, and a commercial CLEM system can be used to re-identify the ROI in the fluorescence channel and retrieve it in the EM using e.g., SerialEM.
Several workflows have so far been established that solved the above-mentioned challenges on sample preparation, re-localization of ROIs, and data correlation. Recent examples for multiscale combinations of in-vivo and ex-vivo imaging include the correlation of intravital microscopy, CT and EM to study single tumor cells in the cerebral vasculature [81]; correlation of X-ray holographic nano-tomography, EM and FM to disentangle dense neuronal circuitry in Drosophila melanogaster and mammalian central and peripheral nervous tissue [82]; correlation of local neuronal and capillary responses by two-photon microscopy with mesoscopic responses detected by ultrasound (US) and BOLD-fMRI [83]; or extended CMI pipelines that include the correlation of a variety of imaging technologies, such as non-invasive US, CT and high-resolution episcopic microscopy (HREM) for phenotyping left/right asymmetries of all visceral organs in a mouse model of heterotaxy or combined OCT, PAI and HREM of chick embryos at multiple development stages [8, 84, 85]. Further examples of novel CMI pipelines that uncover biophysical or chemical information include the correlation of FM, molecular (MALDI MSI) and elemental imaging [X-ray fluorescence (XRF)] to analyze lipids and elements relevant to bone structures in the very same sample section of a chicken phalanx without tissue decalcification at the μm scales [86].
Correlation Software
In addition to the experimental elements helping to bridge the different modalities mentioned in the previous sections, analyzing automated software solutions to correlate complex, multiscale, multimodal and volumetric image data including reconstruction, segmentation, and visualization are an essential pillar of CMI. Image processing and image analysis in biomedical imaging is a wide field of research, having their own conferences (such as ISBI, MICCAI, or NEUBIAS) and specialized journals (IEEE TMI or Medical Image Analysis for example), with thousands of new methods published every year. One common aspect defining the field is the cross expertise needed to develop new algorithms and software: The physics of the imaging modality, and the knowledge of the biological model or of the disease and organs beyond studies are usually important elements to be considered when developing an image processing or analysis method, making this field highly pluridisciplinary. Methods tackle different problems such as restoration (denoising and enhancing the quality and resolution of the acquired images), segmentation (identifying and spatially localizing objects in images), registration (aligning different images of the same or similar objects), and visualization (generating a comprehensive, potentially interactive, representation of the acquired imaging data). In this review, we focus on the two latter, in the context of CMI. Other main elements are mostly specific to one modality and we refer the reader to existing reviews for general approaches, for example, for the use of deep learning for all of these main components of image analysis [87] or for specific components for a specific modality (such as for EM image data restoration [88]). Note that one exception can be made regarding segmentation, where aligned volume can be sometimes used for what is called multimodal segmentation where information gathered from the different modalities refine the segmentation of the ROI [89]. This last category is actually one example of the interest of CMI from the image analysis point of view, where CMI helps image analysis and quantification.
Image (2D or 3D) registration is the process of computing the transformation linking two images or volumes to overlay matching structures (Figure 5). It is a prerequisite for joint quantitative evaluation of the data across modalities and scales for any kind of multimodal visualization of imaging data.
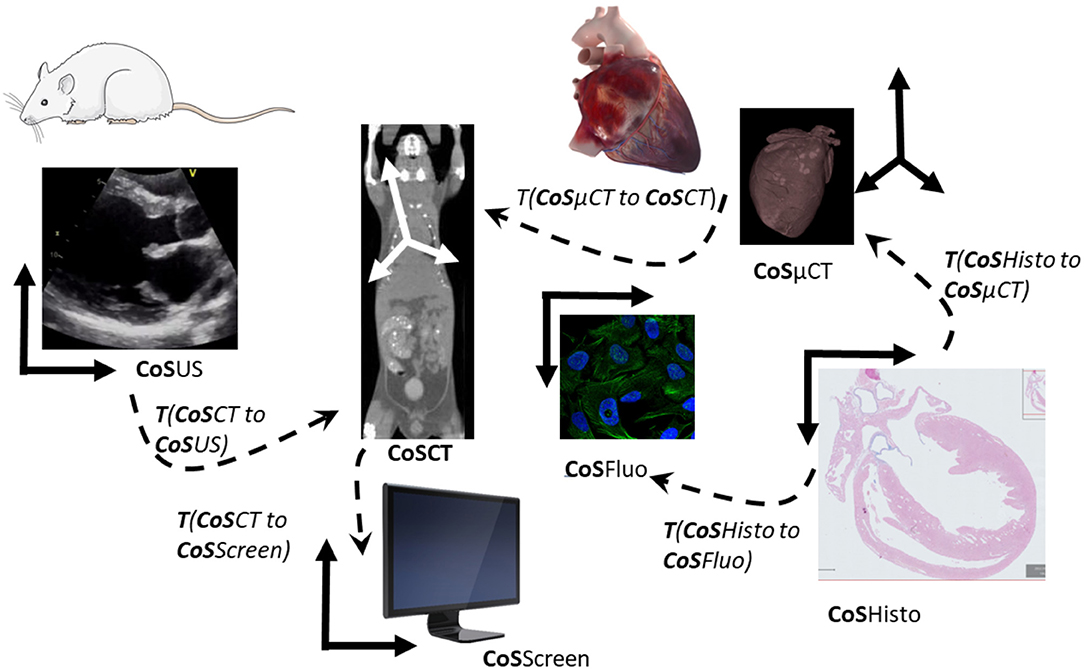
Figure 5. Transformations and coordinate systems involved in the merging and visualization of multimodal data. All transformations between pair of images can be then combined to link all acquisitions and move between scales and modalities. CoS means Coordinate System. T (CoSUS to CoSCT) means the transformation spatially linking the two CoS, allowing for example to locate a US image in the CT volume. To compute the cell in the CT CoS, one can then combine transformations and apply it to the fluorescent cell images (CoSFluo) for example T (CoSFluo to CoSCT) = inverse [T (CoSHisto to CoSFluo)] × T (CoSHisto to CoSμCT) × T (CosμCT to CoSCT). Note that these changes of the coordinate system (or transformations) have to be computed in 3D to take into account possible changes of obliquity, and that they do not take into account deformations induced by sample preparation from one imaging modality to another one.
The model of transformation, i.e., the number of degrees of freedom allowed between the two images, is an important choice, relying on the knowledge of the physical relationship between the sample or the organ from one modality to the other modality. This transformation can be seen as a change of coordinate system if the organ or sample was not undergoing important deformation or deterioration between the two modalities. In that case, a rigid (rotations and translations), similarity (rigid plus uniform scaling), or affine (similarity plus shearing or reflection) may be sufficient. If there are deformations due to the sample evolution over time or due to the sample preparation step for the second modality, a more complex model allowing global and/or local deformation will have to be used (non-rigid or elastic models), according to the required accuracy. Currently, registration is often done in a semi-automated way in two steps: first, manual or automatic identification of landmarks or whole structures (segmentation) in the images to be correlated; second, manual definition of corresponding pairs of these features by the user. These landmarks serve then as an input to the registration process, that is computing an optimal transformation by maximizing the spatial matching of all defined features pairs.
In CLEM, mainly three software solutions are used to perform landmark- or segmentation-based registration: a plugin for FIJI [90] (distribution of ImageJ including many useful plugins) called BigWarp (initially developed to provide training and validation sets), a plugin for ICY called ec-CLEM [91], and the commercial software AMIRA (Thermofisher, Bordeaux France). Several structures have been used to correlate, including vessel, mitochondria, nuclei, added fiducials such as quantum dots (QDs) in the correlative microscopy field, or specific anatomical landmarks in the medical fields. Several other solutions exist in particular in the medical field, but are very dependent of the medical or biological question and of the workflow of imaging. One method will usually be composed based on a set of existing basic bricks performing one task to achieve the expected results [92].
The challenges in fully automated multimodal registration come from the discrepancy in the appearance of structures by different contrast mechanisms and resolution. While the specimen or sample undergoing imaging is usually kept the same size, imaging can focus on a very different field of view with a very different resolution. Algorithms then have to deal with what is called occlusion effect. The problem is usually tackled in a two-step process: first finding the coarse relationship between images, then doing a more accurate registration, that may take into account local deformation if any [93]. These local deformations are usually due to the sample preparation step (for instance, dehydration in histology, which makes the workflow of Figure 5 very challenging without the use of fiducials). Two main approaches can be considered [94]:
(1) Considering the full content of the images and trying to find a common representation intensity space to be able to use monomodal approaches and metrics, or to define a metric that would take into account the possible discrepancy (a classical one is called mutual information, and is comparing joint histogram rather than intensity itself). These approaches are preferred when the different modalities present potentially similar content but with different aspects, for example, when matching bright field imaging with low magnification electronic images [95–97], when cell or nuclei edges are visible on both modalities, or CT with MRI where most of the anatomical structure will be appearing. An interesting approach in deep learning, rather than learning the common space between images, is to directly learn the transformation parameters linking two modalities by using pre-registered images undergoing a set of different parameters for one given transformation as a training set [98].
(2) Considering elements of interest extracted from both modalities, for example anatomical landmarks (points or shape of interest) or multimodal markers visible in both modalities (such as fluorescent QDs in CLEM). These approaches, generally called feature-based registration, are of particular interest when the relation between content is unknown or cannot be taken as an assumption (for example for the validation of a new probe or a new imaging modality). The method to find the matching and compute the transformation can be done with two main paradigms: transforming the image data in localizations with potential additional features using point-based registration ([89, 91] for the AutoFINDER part of ec-clem) or shape-based registration, potentially with intensity-based machine learning approaches [99]. Note that a plethora of variants exists for point-cloud registration, some of them sounding particularly promising for feature-based multimodal registration [100]. Interesting approaches mixed both feature-based and full registration by restraining the learning data set to registered features [101].
For both approaches one of the commonly used libraries for software implementation is ITK (https://itk.org) usually coupled with its visualization counterpart VTK (https://vtk.org).
Very powerful (command line) tools for landmark based or fully automatic image registration (rigid and deformable) are Elastix (http://elastix.isi.uu.nl), its derivative Simple Elastix (http://simpleelastix.github.io) and ANTs (http://picsl.upenn.edu/software/ants/). They allow the definition of fully parameterizable complex registration pipelines. Both libraries support the creation of so-called templates-standard reference spaces that enable the co-registration, comparison and joint analysis of images related to the same structure as represented on the template. These images can come either from the same or different subjects and, as long as there is enough joint information content to ensure registration, they can come from another modality. Multi-channel imaging, where one channel enables easy registration to the template, can support the integration of imaging data with complementary information to the template, like the integration of anatomical and functional images or spatial gene expression data. One prominent example for such standard spaces are standard brain templates that are used to spatially integrate collections of multi-modal brain data e.g., of humans or rodents like the Allen Brain (https://portal.brain-map.org/) or Human Brain Project (https://ebrains.eu) atlases, or the brain of adult [102] and larval [103] drosophila melanogaster.
Visualizing multimodal data, also referred as image fusion, require the knowledge of the spatial transformation linking the images, obtained by registration as explained above. Once this spatial relationship is known, there are several ways to fuse the image information for its interpretation by the user (Figure 6). Visualization per se is mainly categorized in two areas: image and volume rendering (for example using ray-casting algorithms), and region of interest rendering, using for example surface representation with meshes of polygons to match the ROI outside, after segmentation. One simple surface representation without proper identification is isosurface rendering, where a surface shape is defined by an intensity threshold and a surface mesh generated from it. A third way is to use slicing from a 3D volume and come back to a 2D visualization problem. One of the difficulties in multimodal visualization is the difference of spatial resolution between images, calling for interpolation, e.g., upsampling the images of the modalities with lower resolution to the same resolution as the images with the highest resolution. Most registration algorithms automatically resample the moving image to the resolution of the images to which it is registered (the fixed or target image), meaning pixels not existing in the original image have been created by interpolation.
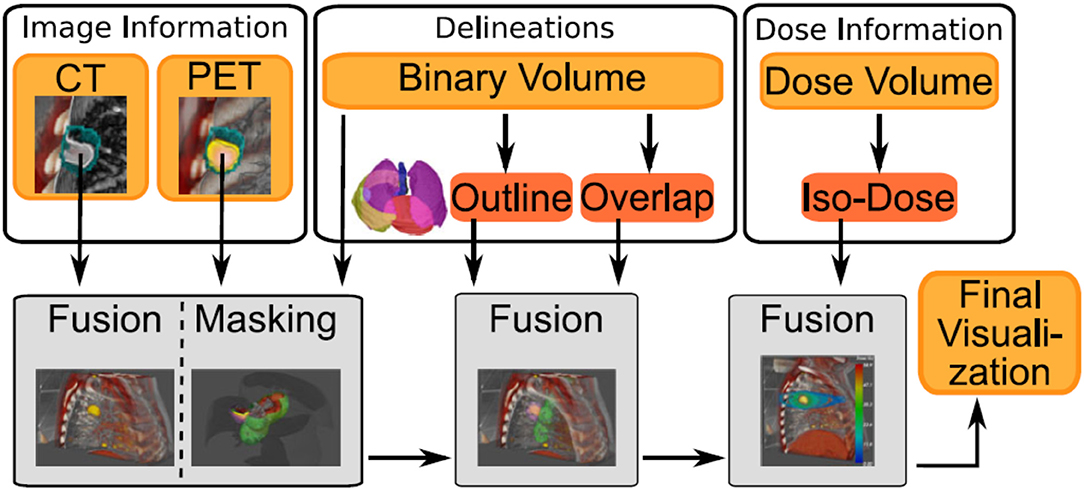
Figure 6. Example of image sources (volume rendering or segmentation) from different modalities, where each type of modalities will be processed differently to provide the final visualization. Figure extracted from Schlachter et al. [104] under the Creative Commons Attribution License.
Efficient visualization of multimodal images will usually propose a combination of different visualization methods [104] and can add an additional channel of information related to the registration itself, such as the error in registration [105, 106]. One of the particular challenges is to deal with data that do not have the same dimensionality, such as time lapse vs. 3D or hyperspectral images, and heterogenous data [107]. To keep the full resolution of the biggest image, with data that can reach several terabytes in size for just one specimen, efforts are ongoing regarding efficient approaches of displaying and manipulating very big data, such as the big data viewer [108] as also used in the BigWarp Fiji Plugin.
For a more exhaustive list of software used in the field, the reader is invited to refer to a constantly updated list of software established in collaboration between the COST actions NEUBIAS (CA15124) and COMULIS (CA17121): www.comulis.eu & www.biii.eu.
Challenges of the Field and Current Solutions
Standardization
CLEM and Correlative Microscopy
As highlighted earlier, every biological question demands its own technological approach. This makes standardization difficult. There will never be one workflow to tackle every single question. It is important however, to try to avoid re-inventing the wheel all over again. Dissemination of established protocols and training the next generation of scientists in these protocols is therefore of the utmost importance. COMULIS is actively promoting such training and standardization where possible.
In CLEM, one of the areas where standardization would be possible is on the correlation precision. Where correlation down to around 50 nm is currently possible (e.g., [16]), for certain approaches an even more precise correlation would open up completely new possibilities. Can we map single fluorophores onto a single protein structure inside a crowded cellular environment? This is currently a dream scenario but will likely be possible in the future (see section CLEM and Correlative Microscopy).
For the moment we will have to do with internal or external added markers that can be used as fiducials for the alignment of the two datasets. If these dual-modality markers are used also to label specific proteins of interest, the first choice are quantum dots as they are fluorescent and their core, generally being made of Cadmium and Selenium, and is made of heavy metals for visualization in EM. Care must be taken however that the proteins coupled to such probes still fulfill its original function. We have shown that Transferrin coupled to QDs does not recycle anymore but, likely due to multiple receptors binding to one QD, is directed down the degradative pathway [109]. An alternative approach, using fluorescent moieties coupled next to a gold particle, has its own issues. It is well-known that fluorescent dyes can be quenched when in close proximity of gold particles [110]. We have recently shown that Alexafluor488 coupled to a 10 nm gold particle is quenched by 95% [111] rendering that particular probe useless as a true CLEM probe. One is probably better off using two individual probes, one tagged with fluorescence and one tagged with gold particles. In our experience a 1:10 ratio works well. Due to technical restraints we have not been able to measure the quenching effect of smaller gold particles yet. One can also add a fiducial marker from the outside. Kukulski et al. [16, 18] reported the use of 50 and 100 nm sized fluorescent beads that are added just before fixation and which are also readily identifiable in the electron microscope. The current methodology allows to correlate the LM and EM images from such experiments down to approximately 50 nm and would at the moment be considered as the standard. With the increasing integration of super-resolution FM technologies into CLEM workflows, this precision is most likely to improve. This does however warrant a remark. Again, it is all down to the underlying question what kind of precision is required. When one is for instance searching for a rare transfected cell amongst a field of untransfected cells all that is required is a correlation precision in the range of micrometers rather than nanometers.
In correlative AFM, first combinations studied the sample first using FM and then transferred it to the AFM [112], which restricted correlation. Early efforts imaged the fluorescence of labeled molecules and topographically imaged the same area. This was misleadingly termed as synchronized operation. The two high-resolution techniques can hardly operate simultaneously due to their reciprocal disturbances. Apart from the mechanical instability of the construction, FM excitation laser(s) can induce disturbances by influencing the detection system of the AFM and/or by short-time heating of the cantilever. Additionally, the AFM laser can lead to photo-bleaching of fluorescently labeled molecules. In general, these problems can be solved by carefully planning the performed experiments and adequately assembling the correlative setup. The biological material itself and the molecule of interest has to be immobile or immobilized as otherwise we would not be able to benefit from the merge. Most immobile samples are studied in combination of AFM with superresolution as both techniques demand immobile samples. Optical microscopy allows studying molecules within transparent samples in contrast to AFM, which is exclusively applicable on surfaces. TIRF excites fluorophores only close to an interface between different optical densities. To limit the influence of the optical excitation to the AFM system, it is convenient to combine these two methods.
Preclinical Hybrid Imaging
Over the last decade, there has been an ongoing discussion about the ability to successfully translate preclinical findings into clinical practice [113–116]. Multiple studies have demonstrated that a bench-to-bedside translation from preclinical results into clinical practice is not as easy as anticipated [113, 115, 117]. Figure 7 illustrates multiple biological, methodologic and technical factors inherently linked to the reproducibility, reliability, and comparability of preclinical imaging data. Each of these factors has a significant impact on the validity of the acquired data and hence can influence reproducibility and reliability of results. Furthermore, it has been shown that replication of already published results is not as straightforward as the scientific community would hope [113, 119, 120]. Hence, standardization of preclinical imaging protocols and techniques to overcome the “replication crisis” has been stated to be of utmost interest [121, 122].
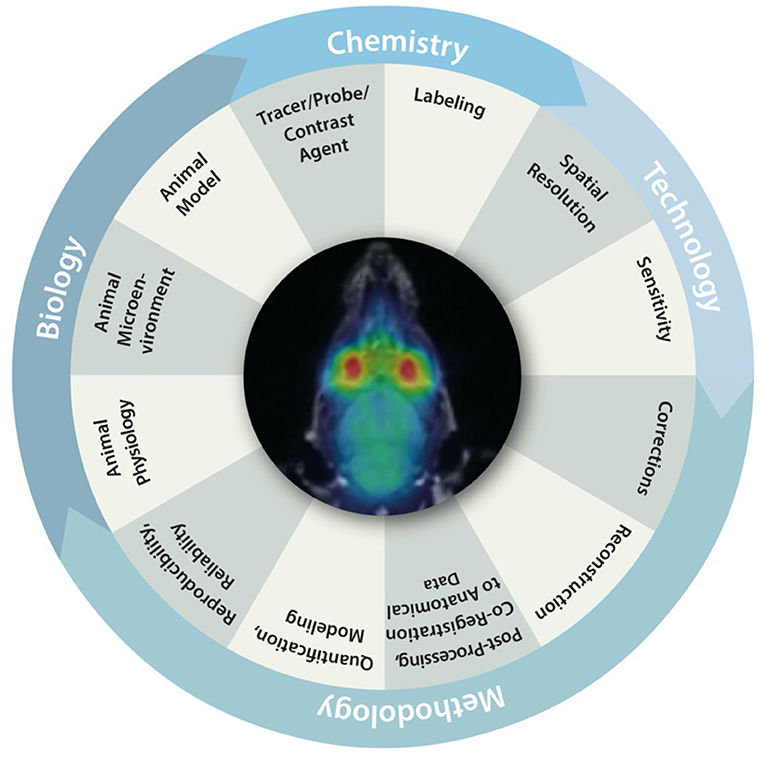
Figure 7. Biological, chemical, methodological and technical factors inherently linked to the reproducibility, reliability and comparability of preclinical imaging data. Figure adapted from Figure 1 from Herfert et al. [118] with reprint permission from Springer according to their creative common license http://creativecommons.org/licenses/by/4.0/.
In sharp comparison to the preclinical research field, clinical standardization is much further advanced and accreditation programs of scanners have been implemented together with unified quality control protocols to ensure reliable, comparable and reproducible results. Furthermore, standardized protocols are in place, which allow multi-center comparison and pooling of the data [123–125]. However, multi-center comparison is still not as easy as anticipated, but up to this point the efforts undertaken have clearly shown its benefit in clinical practice [126, 127].
It is important to emphasize that “over”- standardization in the preclinical environment is not the goal. The fast, dynamic pace of preclinical development is still a strength of one of its kind and should not be outmaneuvered. However, we have to ensure that preclinical findings can be translated more straightforward into clinical research and practice. Therefore, certain techniques, such as anesthesia protocols and animal handling, need to be unified. Furthermore, as has been stated multiple times, precise reporting of methods and techniques is of utmost importance to ensure feasible replication, as well as to facilitate findings from literature and build up based on the existing knowledge [128, 129]. Guidelines, such as the “Animals in Research: Reporting in vivo Experiments (ARRIVE)” guidelines help to improve the quality of reporting, and will consequently maximize the output and validity of published data [129]. In addition, multiple journals have updated their requirements for manuscript submission so that the respective authors either need to upload imaging data as well as all metadata, or to include a data availability statement during submission [130, 131]. An open access of the imaging data and respective metadata is certainly a huge step toward transparency and increased reproducibility and reliability of the data [132], as it has been demonstrated that image analysis is highly user- and software-dependent [133]. Randomized preclinical multi-center studies have been proposed to overcome the lack of reproducibility due to inadequate sample size, low significance, and low confidence of data [134–137]. However, the potential of multi-center studies cannot be fully accessed without proper standardization techniques in place in each participating institute. A recent study focusing on utilizing a basic [18F]fluorodeoxyglucose ([18F]FDG) imaging protocol in 4 different institutes demonstrated that the comparability among multiple institutes might be hampered due to, e.g., animal handling (each institute had different fasting protocols of animals in place; temperature regulation of animals during acquisition differed significantly), and animal facility environment or image analysis [133].
In regards to image analysis, the use of hybrid imaging technologies, with which anatomical co-registration data can be acquired using CT or MRI, significantly enhances the reliability of image analysis since this allows a precise definition of ROIs on the anatomical images that can be overlaid with functional imaging data (e.g., PET or OI data) to ensure the correct placement of ROIs, which is often difficult on functional data only [133]. Multimodal hybrid imaging can enhance reproducibility of results, but nevertheless precise standardized protocols to do so need to be implemented.
There is a huge demand for standardization in preclinical imaging and efforts to implement standardized protocols undertaken by initiatives, such as COMULIS, on a multi-center basis are certainly major steps toward more reproducibility and reliability of preclinical imaging data, as well as increased translation into clinical research and practice.
Correlation Software
As seen from section Correlation Software, different methods have already been proposed in the literature for image registration or multimodal visualization. However, a few of them are actually used by researchers in life sciences, and one of the main reasons for this is the lack of user-friendly implementation and availability as software. In addition, as underlined in the other sections, every biological or medical questions comes with its own image analysis workflow [92]. In order to help with these workflows, but also to help data sharing and open science, a standardization of the representation of the multimodal spatial relationship and content types will be important. In the medical imaging field, such standards are in place, using Digital imaging and communications in medicine (DICOM), which includes guidelines for the representation of spatial transformation between multimodal images [linear (C20.2 DICOM) or deformable (C 20.3 DICOM)]. However, the list of metadata proposed by DICOM is really exhaustive and may prevent users and constructors to actually fill in this information, which represents 15% of major errors as reported in Gueld et al. [138]. In particular, this effort will be important for imaging modalities for which this standard is not in use now and information is not automatically filled in, or for third-party software or home-made methods to compute the spatial transformation that link two images or volumes. For this reason, there is ongoing effort associated with the deployment of public image archive [139–141] to define some minimal metadata requirement, and the one associated with CMI have still to be defined by the community.
Computing the accuracy and assessing the quality of the registration is one of the central problems of correlative microscopies or more generally CMI, in particular because the structure of interest is usually not marked in both modalities, and so it is essential to confirm the correlation is correct and not biased by user assumptions. This is of particular importance when dealing with largely multiscale approaches, since one pixel can be matched with a structure of hundreds of pixels in another modality, and then an error of one pixel could lead to erroneous conclusions. In previous publications [16, 142], an iterative leave-one-out method was used to assess the accuracy of the registration, where the registration error was computed as the average error of localization of beads not used for the registration and was therefore empirical. Recent work tried to find a theoretical estimation of the error, using the Cramer Rao limits [143] to estimate the transform error by taking into account the high resolution limit of accuracy. In ec-clem, the error is estimated using a formalism from the medical field for 2D and 3D rigid registration [144], originally developed for image guided surgery. It can be applied to correlative microscopy but is limited to a rigid-transformation point-based framework, and has the advantage of not requiring any ground truth matching in the images. In addition, this method may lack a proper mathematical formalism to demonstrate their usability in the fields. Note that metrics to compare images based on intensity may not be adapted in most of the case due to the discrepancy of image content (structures not present in both images). In the case of the absence of fiducials or anatomical landmarks to validate the registration, another currently used method to validate the accuracy of a registration is to use segmentation quality metrics, such as DICE metrics, to assess the overlap between known segmented structure.
Challenges are the competition of algorithms on a given dataset, where the ground truth is known in order to rank the algorithms according to a set of metrics designed for the challenge. Some challenges have been organized for the multimodal field, but usually focused on one particular problem (Anhir focused on multiplexed histological data or Curious for US to MRI brain images registration [145]). These challenges are of great interest since they provide a way to identify the actual level of accuracy state of the art and give direction for future research. They will also help define common and standard ways to assess the accuracy of the alignment of multimodal data, by defining community accepted stand error metrics.
Requirements for Optimization and Challenges to the Field
CLEM and Correlative Microscopy
In the recently published book “Correlative Imaging: Focusing on the Future,” there was one over-arching theme that was highlighted in almost all chapters: Data. If handling data from a single modality can already create headaches for the data analysis and IT people, how about trying to combine datasets from different imaging modalities. Developments and possible solutions will be discussed in other parts of this review.
On the hardware side there are also clear trends visible and they almost seem to diverge from each other. On the one hand, there are the cryo-CLEM approaches trying to map protein structures in ultrastructural data, on the other, volume CLEM (a collection of techniques including FIB-SEM, SBF-SEM, array tomography, electron tomography) is more focused on large scale structures and mainly looks at connectivity between cells.
As with the integration of GFP in CLEM from 2000, the resolution revolution in cryoEM has now also been integrated into CLEM workflows, especially aided by the development of LM stages that can work under Liquid Nitrogen conditions [146–148]. These devices allow for the observation of fluorescent structures of plunge frozen samples and record their location for further studying in a cryo-TEM. Whereas, these stages are now fairly commonly in use, the development of cryo-CLEM workflows is still improving; e.g., super-resolution cryo-fluorescence has been recently shown [149]. One of the issues that needs to be dealt with in cellular cryoEM is the thickness of the sample. In most cases, only the outer edges of a cell can be directly imaged. Anything more inside will be too thick to image directly. Cryo-FIB-milling is currently the only way to acquire thin slices of frozen material for cryo-electron tomography (ET) [150]. Targeting the correct area in Z-height, the depth of the sample, is still one of the bottlenecks but acquiring fluorescence data in 3D using confocal cryo-fluorescence will further aid with this targeting problem.
Life is 3D, so also techniques falling under the quiet revolution banner (FIB-SEM, SBF-SEM, Array tomography, electron tomography) are being integrated more and more into CLEM approaches. Especially in these cases, finding the structure of interest adds another dimension of complexity. Resolution in z is generally lower than in x, y so targeting is even more difficult. Also acquisition of z-stacks acquired before processing for EM can be useful and essential but the coordinates may change during the processing. The addition of fiducials or endogenous tissue-existing landmarks, such as blood vessels, can be useful. In addition, a bridging step with intermediate resolution such as CT are relatively new additions to the CLEM workflows [80].
Fully integrated light and electron microscopes should be able to provide the best correlation between the two modalities and both integrated LM-TEM [151] and LM-SEM [111, 152] have been developed and are still improving. Of note here is that all these systems can only work with fixed samples and one of the hallmarks of light microscopy, live imaging, is lost. As before, it is the biological question that will determine what workflow and technology fits best.
In correlative AFM, correlative challenges and opportunities are faced by recent technological advancements, improving temporal and spatial resolution of already established techniques. The combination of these highly sophisticated setups is far from trivial and highly challenging. High-Speed Atomic Force Microscope, for example, allows studying dynamic processes at sub-molecular and sub-second scale and can be combined with STED to track molecular movement at nanometer resolution and in the millisecond range.
To enable a precise and simultaneous superposition, disturbances in correlative AFM must be sufficiently shielded. An acoustically shielded chamber around the FM including the AFM measuring head is suitable for this purpose. All components that can cause electronic or acoustic interference must be removed from the isolation chamber. Typically, water-cooled EM-CCD cameras are used here to minimize interference from the outset. During simultaneous applications, the problem arises that the measuring tip of the AFM is heated by the excitation laser. This problem cannot be prevented; in this case, combinations with TIRF or confocal microscopy are usually used. It is important to keep the excitation energy at the position of the cantilever low. If both techniques are used at the same time, a real time superposition of the images is currently not possible. In most cases, the images have to be adapted to each other by mathematically forced imaging errors. For this purpose, a fluorescent grid is suitable which is taken before the actual measurement with both techniques at the same position and subsequently adapted to each other. Currently, the AFM manufacturers already offer their own software for superimposing the images. The measuring tip is moved to several different positions, which are recorded simultaneously on the microscope and then superimposed directly. However, the measuring tip in the fluorescence image cannot be superimposed more precisely than the resolution of the microscope. Of course, the resolution can be increased by fitting techniques, known from super-resolution microscopy, but the resolution of the AFM itself can never be achieved.
Further challenges will be the combination and further development of additional microscopy technologies, such as correlative SXT. Since imaging under cryo-conditions preserves a close-to-native environment (as for cryoEM) and in certain cases (as for SXT due to its operation in the water window) is the only possible implementation, the development of cryo-FM will play a crucial role in correlative microscopy. For correlative SXT, superresolution FM is specifically appealing since it matches the achievable spatial resolution, and further efforts will focus on its cryo-implementation [23].
Preclinical Hybrid Imaging
One of the current bottlenecks for PHI lays in radiation doses for X-ray imaging together with radioisotopic imaging methods. CT, PET, and SPECT imaging delivers substantial radiation dose into the animal, and, of course, to the patient as well in clinics. The prolonged CT scanning times along with high doses of radioisotopes applied to the animal may interfere with the immune system, tumor growth and rapidly proliferating tissues (bone marrow, intestine) renewal. There are estimates that up to one percent of patients repeatedly scanned for possible metastases by whole body CT, PET/CT or SPECT/CT during a 5-years follow-up period may die because of new tumors induced by the imaging process [153, 154]. The significantly lower radiation load to the patient brings PET/MRI imaging; nevertheless, the high dose coming from high energy PET isotope injection cannot be avoided. Moreover, CT is excellent for hard tissue (bone) and contrast (e.g., angiography) imaging but soft tissue discrimination is rather poor. Several companies brought to the market so called spectral CT devices. They are usually based on dual energy X-ray sources, and the comparison of the energy-dependent attenuation of signal can improve the soft tissue recognition and distinguish contrasts and the bone, which is not possible in classical CT devices [155]. These CT machines can utilize slightly lower radiation dose compared to previous generation. Due to the recent progress in the development of novel radiation detectors, there occurred a possibility to introduce a completely new radiation detection approach. Under international collaboration in CERN, the photon counting TimePix detectors were developed. The current generation of TimePix3 detectors allows a simultaneous detection of the exact position, energy and time of the photon interaction. These properties can be used for true spectral CT detection. Novel detectors are much more sensitive and have a high spatial resolution of 55 μm. The CT image can be obtained very fast (in seconds compared to 20 to 30 min for high-resolution standard scans), thus significantly reducing the absorbed radiation dose. The filtering of noise allows to increase the signal-to-noise ratio. According to different attenuation tissue patterns, soft tissue recognition is easier even without contrast [156]. The high speed of TimePix3 detectors (1,700 images per second) and energy resolution is suitable for coincident event registration also in PET imaging [157]. The proof-of-principle of the use of the TimePix3 detectors has been published [158]. Several groups are testing the use of Compton cameras for SPECT imaging instead of collimated SPECT detection [159, 160]. Compton cameras allow to calculate the trajectory of incoming photons from original hit position and Compton scattering detected by a second detector layer. This allows to increase the sensitivity of SPECT from <0.1 to 80% for the most used SPECT isotope 99mTc. SPECT imaging thus only requires a fraction of currently used radiation activity, and the absence of collimator facilitates the construction of combined CT/PET/SPECT devices with just one ring of detectors that can simultaneously record fast fully trimodal hybrid whole body imaging with very low radiation load. The main limitation is still the high price of detectors which could be in future substantially lowered by demand for production in large series.
Most of the optical imagers allow to detect fluorophores from visible light up to the NIR region with the longest wavelengths around 850 to 900 nm. This covers the close NIR region with relatively good light penetration. The signal loss in deeper tissues does not permit quantitative data nor fully tomographic imaging. Mouse tissues are much more transparent for shortwave infrared light (SWIR) with wavelengths between 1,000 and 2,000 m. No autofluorescence, reduced light scattering and lack of absorption by blood are the main advantages of imaging in the SWIR region [161]. The first commercial in vivo optical scanner allowing imaging of contrasts with longer excitation wavelengths than 1,000 nm appeared on the market in 2019. The method is still limited by the low availability of fluorescent contrast agents for use in the SWIR region.
Besides, there is the current challenge of correlating additional beneficial but not yet readily/commercially available imaging modalities (same challenge as faced by the biological microscopy community). For example, while fusion of optical tomography data (DLIT, FLIT, or FMT) with CT can be achieved using commercial imaging systems, co-registration of OI and MRI is still in the developmental state. As OI and MRI are recorded in separate systems, relocations of animals between the recording sessions have to be conducted with great diligence to avoid anatomical distortion and positional changes. Chehade developed a shuttle made of CT- and MRI- compatible material, which allowed the relocations of mice while keeping them properly in place [77].
These challenges hold also true for in-vivo microscopy—despite its impressive advances. It is still challenging to synergistically combine optical technologies in one platform since they do not match in imaging speed, size, resolution and contrast, and proper imaging pipelines have to be established. In this context, CMI platforms for label-free sample screening bear great potential, and working combinations of these modalities (such as OCT, RS, MPM, SHG—STATE-OF-THE-ART/Preclinical Hybrid Imaging) will need to be identified on the basis of their added value in tackling specific biomedical research questions.
Novel CMI Pipelines
Novel CMI pipelines will continue to bridge in-vivo (preclinical) and ex-vivo (biological) imaging and allow to zoom in from physiological native tissue context to subcellular molecular resolution. This comes with several challenges to be tackled: (1) sample preparation that is compatible across imaging modalities without compromising data quality, (2) hard- and software solutions to relocate the same ROI after changing the imaging platform, (3) robust markers that can be detected in different imaging technologies, (4) lack of high throughput and automatization, (5) software solutions to correlate the imaging data and standards for data handling and storage, (6) availability of research infrastructure (i.e., cutting-edge imaging technologies from PET scanners down to cryo-EM).
(1) Sample preparation procedures are mainly an issue across ex-vivo imaging technologies since these require specific fixation and embedding that might be incompatible with other techniques. Typically, most in-vivo imaging protocols such as MRI, CT or US do not interfere with downstream processing for histologic and ultrastructural observation. This is true even when routine contrast agents are administered for in-vivo imaging. Typical example of incompatibility are the quenching of fluorophores by standard EM preparation protocols (compare section CLEM and Correlative Microscopy) or incompatibility of glutaraldehyde fixation or JB4 resin embedding with many protocols for immunohistochemistry. In general, fixation is a critical parameter in correlative workflows and requires optimization since it usually comes with sample-distorting artifacts, such as tissue shrinkage, swelling, hardening, and color change. Besides, it is of highest importance to fix the tissue or organism right after euthanasia to prevent autolysis and degradation of cellular structures [162]. To improve the penetration of fixatives, the tissue or sample might need to be incised. The sample should then be incubated in at least 20 times the sample volume, and, to facilitate correlation, be pre-embedded in 1% agarose using a casting mold prior to processing [163]. Ideally, both macroscopic and microscopic morphologies are preserved by the simultaneous stabilization of all cellular components as achieved by cryofixation. However, while preserving the close-to-native morphology, the drawback of cryofixation in comparison to chemical fixation is its limited depth to which samples can be well-frozen. High-pressure freezing allows to fix a thickness of maximally 0.6 mm. Continuing to work under cryo-conditions ensures close-to-native architecture, but poses additional challenges to potential follow-up microscopy technologies such as FM—since current cryo-objectives are limited in their optical performance (such as low numerical aperture) [164].
Importantly, all destructive staining needs to be avoided: An example is the perfusion of vasculature with heparin, formalin, NaCl and barium sulfate with gelatin to stain blood vessels via the ventricles after anesthesia in mice. Vasculature staining can be replaced in certain cases by post-mortem staining with Lugol's solution, a mixture of one part iodine and two parts potassium iodide in water [165].
Imaging thick tissues or entire organisms in vivo and subsequently zooming-in into the subcellular ultrastructure is facilitated by current advances in FM of non-transparent organisms, such as longer wavelengths for deeper penetrations depths [166], development of improved near-infrared probes [167], or photoacoustics [168]. While studying even thicker tissue—though in-vitro—will be facilitated by further advancements in clearing larger samples using lipid extraction to reduce light scattering, it is questionable whether this will also facilitate correlative microscopy approaches since clearance of larger samples might interfere with ultrastructural preservation.
(2) Identifying the same ROI across diverse in- and ex-vivo imaging platforms is currently an inherent bottleneck of novel CMI approaches. Specifically, the relocation of a ROI in thick living tissue at high subcellular or molecular resolution presents the biggest challenge faced by CMI when bridging in-vivo preclinical imaging and ex-vivo biological microscopy. As outlined in 2.3, current strategies focus on NIRB as an intermediate step for volume CLEM. If no additional processing step is foreseen between modalities (which might induce distortions or even require reduction of the volume by sectioning), the straightforward approach to correlate the ROI across modalities is to use a joint transferable coordinating system. A well-established example is the annotation of FM to define ROIs with a dedicated CLEM module and import and relocate these coordination lists into the cryo-EM microscope using SerialEM [169]. Other approaches focus on using the same holder for different imaging modalities. Examples include immobilization beds for preclinical imaging in mice using PET and CT (where additionally 22Na fiducial markers can be placed into stationary pegs at defined depths to provide a 3D references to simplify image registration), or the combination of x-ray spectromicroscopy with electron tomography, where Allende meteorite grains were deposited on a TEM grid and transferred between the electron microscope and the COSMIC soft x-ray beamline [170]. First “plug-and-play” holder solutions are being described that are compatible and even commercially available, which can fit in a variety of microscopes for correlative imaging without changing the holder. While it facilitates relocation of ROIs tremendously, this approach cannot overcome the main limits or CMI pipelines: To assess a ROI in thick tissue, the tissue will still need to be cut due to the limited penetration depths of most high-resolution ex-vivo microscopy techniques.
To facilitate ROI relocation, CMI also aims at setting up hardware-fused hybrid setups that inherently co-localize the same ROI due to their joint coordination system. Apart from well-known commercially available PHI scanners (such as PET/CT, SPECT/CT, or PET/MRI), as described in section State-of-the-Art, examples include (i) a variety of hybrid AFM and FM setups [30, 171, 172], (ii) first implementations of integrated EM-FM setups [111, 173], (iii) several combined setups of OCT with photoacoustics or non-linear microscopy [174], and (iv) diverse hardware-based approaches to combine OI techniques with CT or MRI [175]. Nevertheless, in certain cases, relocation across single modality systems using cross-platform transport beds for CMI may provide superior performance compared to hybrid systems where compromises may have been made in the integration process.
If hybrid setups are not available (as is mostly the case), correlation can be facilitated by imaging the exact same sample without intermediate processing steps. For example, instead of performing FM before EM fixation and embedding, EM sections could be imaged directly with FM if preserving fluorescence or immunolabeling them.
(3) There is a plethora of multimodal probes for correlative microscopy and preclinical imaging, but there are hardly any robust markers that can be detected across modalities when combining various contrast mechanism with high microscopic accuracy. For correlative microscopy, most commonly used markers include QDs or polymer beads. Other examples include biocompatible nanosized, fluorescent and electron-dense intracellular nanodiamonds (internalized in living cells via endocytosis) as probes for 3D CLEM [176]. For the combination of preclinical imaging modalities, mainly CT, MRI, and optical approaches, there is a variety of CMI probes: (1) Lipid-based markers, such as liposomes or lipoproteins as carriers; (2) macromolecular carriers where different contrast agents are attached to a common macromolecule (and its reactive amines, thiols, or carboxyls); (3) nanoparticles, such as QDs, iron oxide nanoparticles or nanoparticle carriers; or (4) small molecules where two or more probes are directly fused together with minimal intervening bonds [177]. Further examples include high-contrast, non-radioactive tungsten-based fiducial markers for multimodal brain imaging with MRI, PET and CT that are attached outside of the sample in close proximity of the ROI, and numerous dual PET and NIR fluorescence imaging probes [178, 179], such as fluorescence-labeled monoclonal antibodies (mAbs) and systemic applications of both mAbs and peptides in PET/SPECT in-vivo. While 64Cu the prevalent isotope for systemic mAb imaging, 18F and 68Ga isotopes better match the targeting half-lives of peptides. Although dual agents for PET and NIR imaging are in its infancy and no agent has been approved by the FDA so far, several preclinical applications have been reported [179]. Examples for markers for advanced CMI pipelines include photo- or chemically-convertible tags (such as miniSOG or APEX) that can be detected in FM, CT and EM and were used to identify the ROI across multiple imaging modalities [180–182].
Most multimodal probes are exogenous. The ultimate goal is to have the organism or cells express their own probes after transfection. Fusions of GFP for fluorescence imaging and herpes simplex virus thymidine kinase (HSV-TK) for PET have been reported in several studies. HSV-TK can also be fused to other optical reporters and constructs of luciferase. With QDs and (NIR) fluorophores being used across preclinical and biological imaging modalities, these two markers appear most promising for advanced CMI pipelines. While a single CMI marker will guarantee the same pharmacokinetics and colocalization of the signal for each modality and reduces the stress on the blood clearance mechanisms of small animals (as induced by multiple doses of agents), the variations of sensitivities of different imaging modalities need to be considered when aiming for the detection of a single probe correlatively. In certain cases, it may not be practical to simply add all functionalities to one molecule [177].
For a rough alignment of untreated samples between relocation of imaging platforms, registration marks such as gridded coverslips or finder grids [183], or deposition of metal structures or engraving of the surface are commonly used. To provide rough orientation when dissecting ROIs from living organisms for subsequent ex-vivo analysis, the margins of adjacent tissue are demarcated in their bodily orientation with surgical ink or notches on the skin. For advanced CMI pipelines from thick tissue to 2D sections, endogenous landmarks or NIRB can be used. In volume CLEM, blood vessels, nuclei or myelinated axons can be used as endogenous fiducials since they show sufficient contrast both in light and electron microscopy and are distinctive in size and shape—as demonstrated for mouse brain imaging using a CMI pipeline with in-vivo 2-photon microscopy and FIB/SEM [6].
(4) Nanometer-resolution of the subcellular architecture of tissues usually requires time-intensive scanning of the sample (as for FIB/SEM or AFM) since lateral resolution often comes at the expense of penetration depth and field of view. The selection of a volume of interest several orders of magnitude smaller than the sample imaged by FM is hence both crucial and challenging. Solutions to studying big volumes at high resolution and with high throughput include the use of multi-beam setups (such as multi-beam SEM [184]) with parallelized data collection, or the automation of the identification of ROIs and image acquisition [185]. Since advanced CMI setups require tedious protocol optimization, time-intensive image acquisitions and intermediate processing steps, in general, novel CMI pipelines suffer from lack of throughput, which restricts reproducibility and statistics. Currently, the focus of CMI pipelines is rather on identifying working combinations to address previously inaccessible biomedical research than on fostering throughput. Once those correlations have been showcased and are proven feasible by substantial R&D efforts, CMI will enter further automatization and simplification to generate throughput.
(5) Advances and current trends in correlation software are discussed in sections Correlation Software and Correlation Software. To expedite automated multimodal image registrations and quantification, data handling specific to multimodality needs to be established, including universal imaging formats, ontologies, and data storage and repositories. While there is currently no universally established microscopy format with additional diversity between preclinical and biological imaging approaches, repositories and public archives for diverse imaging data are being implemented—from single molecules (EMPIAR) to tissues (Tissue-IDR). Correlative data sets (such as CLEM data to link functional information across spatial and temporal scales) will be included in the so-called added-value databases that are developed around the archive. They aim at gathering a greater understanding for specific biological areas through systematic integration of images [141]. Integration of such multimodal data sets and their interoperability will be facilitated by universal large-scale multi-granular imaging ontologies, whose need is being described in first publications [186, 187].
(6) Novel CMI pipelines require access to diverse imaging technologies. All these technologies and the necessary expertise are unlikely to be found in a single laboratory, which restricts the development and implementation of CMI. To facilitate access to complementary imaging technologies and exchange of knowledge, several initiatives have been established. Two prominent examples are (1) COMULIS (Correlated Multimodal Imaging in Life Sciences), a COST Action (CA17121) to foster CMI, and (2) Euro-BioImaging, a European Research Infrastructure Consortium providing open access to imaging technologies in biological and biomedical research.
Correlation Software
As already underlined, most of the automated methods are developed ad hoc for a particular multimodal problem. Machine learning and deep learning are definitely moving the image analysis field a step forward [87] since their main interest is to create an ideal method of processing based on training data sets, translating the effort of developing ad-hoc computer vision or signal processing methods to the effort of annotating data and formalizing the problem as input/output. Note that deep learning is still in progress and a lot of research is still going on to optimize these methods and reduce the number of training datasets required, as well as taking into account the errors in annotations in training data sets [87]. Based on these approaches, a universal solution without any user input is not envisioned per se, but rather a universal framework for multimodal registration, or the creation of a giant bank of pretrained models. It could be envisioned to be set up with minimal user input, i.e., by providing registered data sets or at least identifying on both modalities what should be used for matching. There are ongoing approaches for a universal segmenting tool, based on deep learning, trained on different data, for example, for nuclei segmentation [188]. Interestingly, it has been shown that some of the models trained could be applied in a new different modality (even if all at the same microscopic scale), for example, with different staining without the need of further training.
Another challenge in the field is the integration of very heterogeneous data, such as CMI with very different dimensions (multiplexing or spectral data with hundreds of outputs for one spatial localization, temporal vs. static) with non-imaging data such as -omics data. This effort could be facilitated by two approaches: the single cells approaches, and the development of spatially localized proteomics or genomics which are now starting to appear as commercial platform and would facilitate these links. But then the analysis of this largely heterogeneous data still requires to develop new statistical tools.
Another trend is to use multimodal aligned images as training sets to construct inference models to reduce the needs for one or the other modality. For example, Li et al. [189] used a deep learning approach to generate PET images from MRI and demonstrated similar classification results using the generated PET images than the true PET images for Alzheimer Disease and Mild Cognitive impairment. In this preliminary study, a 3D convolutional neural network was trained with MRI patches as input and matching PET patches as output using half of a database of patients having both exams. The parameters of the network capture a relationship between both modalities. This trained network was then used to generate the predicted PET images from MRI images, and validated against the remaining half of the database. The same principles have been applied also to microscopy images, where for example restoration based on deep learning have shown impressive results [190], and even predicting fluorescent labeling from transmitted light images [191].
These approaches in the long term could then reduce the number of modalities required to answer a particular question. To achieve such a goal, sharing well-annotated aligned multimodal data is of particular importance. Efforts are on-going in this direction to share repositories and public archives ([141], Empiar, IDR).
Conclusions and Outlook: Future of the Field
To have an even bigger impact and to become a basic life science technology as FM is nowadays, it will be crucial for correlative microscopy to develop and disseminate automated workflows that can deal with huge amounts of data and seamlessly merge diverse data sets. This process will be facilitated by a number of factors: the improved capabilities of integrated systems, the adaption of standard file formats, and the deposition and sharing of these information-rich datasets.
For PHI, preclinical molecular in-vivo whole-body imaging is fully dependent on hybrid imaging and co-registration with anatomical images. Some devices are already multimodal in their hardware settings, but images from different techniques are taken sequentially and implemented automated co-registration often requires manual intervention to obtain the best results. Multimodal animal beds allow to scan the same anesthetized animal in different devices, co-register multiple imaging methods and obtain enhanced molecular information about the in-vivo processes. The implementation of new methods and contrast agents broadens the spectrum of imaging possibilities, and simultaneously acquired multiple hybrid images facilitate proper visualization. Besides, OI has seen significant expansion in biomedical and diagnostic applications, which go beyond simple visualization of the sample. Novel modalities allow label-free mapping of biomolecules in vivo providing a way to determine a stage of disease progression or enable tomographic assessment of deep tissue layers. Nevertheless, current research efforts are indicating that in many biomedical applications, a single modality is inadequate to provide a comprehensive picture of a disease. Instead a targeted combination of modalities, which give access to a set of (label-free) parameters is necessary.
In summary, CMI is a field under construction, relying on broad expertise. In particular, data processing, analysis and management need to be incorporated in the initial reflections leading to a project, and a continuous and iterative dialog has to take place during the whole project with image data analysts such that the communities can understand the requirements and needs of each other. Setting up standard approaches and sharing protocols and generated data will definitely be the key elements for achieving a smooth communication. A real holistic view will be achieved when other type of data will be also correlated with imaging data, but to reach such a goal the CMI community needs to develop its own solid ground.
Ideally, CMI will lead to multimodal platforms that allow to functionally and morphologically characterize the entire sample in-vivo, fast and non-destructively at high axial and lateral resolution and high penetration to gain a mechanistic understanding of organisms and diseases. By synergistically fusing complementary imaging techniques, CMI platforms can give insights into a variety of tissue properties during a single image acquisition, and better tissue characterization can be achieved than by the separate imaging modalities alone. Complementary information provided by the fused imaging modalities and machine-learning-assisted data analysis will ultimately yield novel biomarkers by a multi-dimensional classification accelerating the discovery and translation of novel therapeutic strategies. Such hybrid platforms of high accuracy will correlate the modalities instantly without the need for post-processing correlation software. Surely, 3D cellular, ultrastructural and molecular tissue maps as acquired by CMI will substantially transform biomedical research and diagnostics in the future.
Author Contributions
AW initiated, coordinated, and supervised the manuscript and wrote all CMI sections, Introduction, Conclusion, and Abstract. LS wrote main parts of the PHI sections. PP-G wrote all software sections. PV and BP main parts of the correlative microscopy sessions. JM, MO, BP, PS, AU, SH, DF, and MM-D contributed to each section. KB, SG, MG, TW, and WW provided feedback and edits.
Funding
This work has been supported by the Federal State of Upper Austria and “Land OÖ Basisfinanzierung” and Timed Center Upper Austria TC-HyperChol.
Conflict of Interest
The authors declare that the research was conducted in the absence of any commercial or financial relationships that could be construed as a potential conflict of interest.
Acknowledgments
PP-G acknowledges ANR-18-CE45-0015 and the France-BioImaging infrastructure supported by the French National Research Agency (ANR-10-INBS-04). LS acknowledges the large RI Project LM2015062 Czech-BioImaging funded by MEYS, Czech Republic. KB acknowledges the FFG COMET center project 854174. All authors acknowledge their collaboration facilitated by COST as members of COMULS (CA17121).
Abbreviations
AFM, Atomic Force Microscopy; CARS, Coherent Anti-Stokes Raman Spectroscopy; CLEM, Correlative Light and Electron Microscopy; CMI, Correlated Multimodal Imaging; CT, Computed Tomography; EM, Electron Microscopy; FIB, Focused Ion Beam; FM, Fluorescence Microscopy; HREM, High Resolution Episcopic Microscopy; LM, Light Microscopy; LSFM, Light Sheet Fluorescence Microscopy; MPI, Magnetic Particle Imaging; MPM, MultiPhoton Microscopy; MRI, Magnetic Resonance Imaging; MSI, Mass Spec Imaging; OCT, Optical Coherence Tomography; OI, Optical Imaging; PAI, Photoacoustic Imaging; PET, Positron Emission Tomography; PHI, Preclinical Hybrid Imaging; RS, Raman Spectroscopy; SEM, Scanning Electron Microscopy; SHG, Second Harmonic Generation Microscopy; SPECT, Single Photon Emission Computed Tomography; Superresolution, Superresolution Microscopy (such as STORM, PALM, STED); SXT, Soft X-ray Tomography; TEM, Transmission Electron Microscopy; TPEF, Two-Photon Excited Fluorescence Microscopy; US, Ultrasound.
References
1. Pogue BW, Leblond F, Krishnaswamy V, Paulsen KD. Radiologic and near-infrared/optical spectroscopic imaging: where is the synergy? Am J Roentgenol. (2010) 195:321–32. doi: 10.2214/AJR.10.5002
2. Helmchen F, Denk W. Deep tissue two-photon microscopy. Nat Methods. (2005) 2:932–40. doi: 10.1038/nmeth818
3. Marti-Bonmati L, Sopena R, Bartumeus P, Sopena P. Multimodality imaging techniques. Contrast Media Mol Imaging. (2010) 5:180–9. doi: 10.1002/cmmi.393
4. Beyer T, Freudenberg LS, Townsend DW, Czernin J. The future of hybrid imaging-part 1: hybrid imaging technologies and SPECT/CT. Insights Imaging. (2011) 2:161–9. doi: 10.1007/s13244-010-0063-2
5. de Boer P, Hoogenboom JP, Giepmans BN. Correlated light and electron microscopy: ultrastructure lights up! Nat Methods. (2015) 12:503–13. doi: 10.1038/nmeth.3400
6. Luckner M, Burgold S, Filser S, Scheungrab M, Niyaz Y, Hummel E, et al. Label-free 3D-CLEM using endogenous tissue landmarks. iScience. (2018) 6:92–101. doi: 10.1016/j.isci.2018.07.012
7. Johnson E, Seiradake E, Jones EY, Davis I, Grunewald K, Kaufmann R. Correlative in-resin super-resolution and electron microscopy using standard fluorescent proteins. Sci Rep. (2015) 5:9583. doi: 10.1038/srep09583
8. Liu M, Chen Z, Zabihian B, Sinz C, Zhang E, Beard PC, et al. Combined multi-modal photoacoustic tomography, optical coherence tomography (OCT) and OCT angiography system with an articulated probe for in vivo human skin structure and vasculature imaging. Biomed Opt Express. (2016) 7:3390–402. doi: 10.1364/BOE.7.003390
9. Porter KR, Claude A, Fullam EF. A study of tissue culture cells by electron microscopy : methods and preliminary observations. J Exp Med. (1945) 81:233–46. doi: 10.1084/jem.81.3.233
10. Rieder CL, Cole RW, Khodjakov A, Sluder G. The checkpoint delaying anaphase in response to chromosome monoorientation is mediated by an inhibitory signal produced by unattached kinetochores. J Cell Biol. (1995) 130:941–8. doi: 10.1083/jcb.130.4.941
11. Heim R, Cubitt AB, Tsien RY, Porter KR, Claude A, Fullam EF. Improved green fluorescence. Nature. (1995) 373:663–4. doi: 10.1038/373663b0
12. Polishchuk RS, Polishchuk EV, Marra P, Alberti S, Buccione R, Luini A, et al. Correlative light-electron microscopy reveals the tubular-saccular ultrastructure of carriers operating between Golgi apparatus and plasma membrane. J Cell Biol. (2000) 148:45–58. doi: 10.1083/jcb.148.1.45
13. Polishchuk EV, Di Pentima A, Luini A, Polishchuk RS. Mechanism of constitutive export from the golgi: bulk flow via the formation, protrusion, and en bloc cleavage of large trans-golgi network tubular domains. Mol Biol Cell. (2003) 14:4470–85. doi: 10.1091/mbc.e03-01-0033
14. Lees RM, Peddie CJ, Collinson LM, Ashby MC, Verkade P, Miles BT, et al. Correlative two-photon and serial block face scanning electron microscopy in neuronal tissue using 3D near-infrared branding maps. Methods Cell Biol. (2017) 140:245–76. doi: 10.1016/bs.mcb.2017.03.007
15. Olmos Y, Hodgson L, Mantell J, Verkade P, Carlton JG. ESCRT-III controls nuclear envelope reformation. Nature. (2015) 522:236–9. doi: 10.1038/nature14503
16. Kukulski W, Schorb M, Welsch S, Picco A, Kaksonen M, Briggs JA. Correlated fluorescence and 3D electron microscopy with high sensitivity and spatial precision. J Cell Biol. (2011) 192:111–9. doi: 10.1083/jcb.201009037
17. Kukulski W, Schorb M, Kaksonen M, Briggs JA. Plasma membrane reshaping during endocytosis is revealed by time-resolved electron tomography. Cell. (2012) 150:508–20. doi: 10.1016/j.cell.2012.05.046
18. Kukulski W, Schorb M, Welsch S, Picco A, Kaksonen M, Briggs JA. Precise, correlated fluorescence microscopy and electron tomography of lowicryl sections using fluorescent fiducial markers. Methods Cell Biol. (2012) 111:235–57. doi: 10.1016/B978-0-12-416026-2.00013-3
19. Brown E, Van Weering J, Sharp T, Mantell J, Verkade P. Capturing endocytic segregation events with HPF-CLEM. Methods Cell Biol. (2012) 111:175–201. doi: 10.1016/B978-0-12-416026-2.00010-8
20. Spiegelhalter C, Tosch V, Hentsch D, Koch M, Kessler P, Schwab Y, et al. From dynamic live cell imaging to 3D ultrastructure: novel integrated methods for high pressure freezing and correlative light-electron microscopy. PLoS ONE. (2010) 5:e9014. doi: 10.1371/journal.pone.0009014
21. Verkade P. Moving EM: the rapid transfer system as a new tool for correlative light and electron microscopy and high throughput for high-pressure freezing. J Microsc. (2008) 230(Pt. 2):317–28. doi: 10.1111/j.1365-2818.2008.01989.x
22. Elgass KD, Smith EA, LeGros MA, Larabell CA, Ryan MT. Analysis of ER-mitochondria contacts using correlative fluorescence microscopy and soft X-ray tomography of mammalian cells. J Cell Sci. (2015) 128:2795–804. doi: 10.1242/jcs.169136
23. Pereiro E. Correlative cryo-soft X-ray tomography of cells. Biophys Rev. (2019) 11:529–30. doi: 10.1007/s12551-019-00560-z
24. Axmann M, Sezgin E, Karner A, Novacek J, Brodesser MD, Rohrl C, et al. Receptor-Independent transfer of low density lipoprotein cargo to biomembranes. Nano Lett. (2019) 19:2562–7. doi: 10.1021/acs.nanolett.9b00319
25. Plochberger B, Rohrl C, Preiner J, Rankl C, Brameshuber M, Madl J, et al. HDL particles incorporate into lipid bilayers - a combined AFM and single molecule fluorescence microscopy study. Sci Rep. (2017) 7:15886. doi: 10.1038/s41598-017-15949-7
26. Spedden E, White JD, Naumova EN, Kaplan DL, Staii C. Elasticity maps of living neurons measured by combined fluorescence and atomic force microscopy. Biophys J. (2012) 103:868–77. doi: 10.1016/j.bpj.2012.08.005
27. Kashef J, Franz CM. Quantitative methods for analyzing cell-cell adhesion in development. Dev Biol. (2015) 401:165–74. doi: 10.1016/j.ydbio.2014.11.002
28. Christenson W, Yermolenko I, Plochberger B, Camacho-Alanis F, Ros A, Ugarova TP, et al. Combined single cell AFM manipulation and TIRFM for probing the molecular stability of multilayer fibrinogen matrices. Ultramicroscopy. (2014) 136:211–5. doi: 10.1016/j.ultramic.2013.10.009
29. Staunton JR, Doss BL, Lindsay S, Ros R. Correlating confocal microscopy and atomic force indentation reveals metastatic cancer cells stiffen during invasion into collagen I matrices. Sci Rep. (2016) 6:19686. doi: 10.1038/srep19686
30. Chaudhuri O, Parekh SH, Lam WA, Fletcher DA. Combined atomic force microscopy and side-view optical imaging for mechanical studies of cells. Nat Methods. (2009) 6:383–7. doi: 10.1038/nmeth.1320
31. Chtcheglova LA, Hinterdorfer P. Simultaneous AFM topography and recognition imaging at the plasma membrane of mammalian cells. Semin Cell Dev Biol. (2018) 73:45–56. doi: 10.1016/j.semcdb.2017.08.025
32. Cordes T, Strackharn M, Stahl SW, Summerer W, Steinhauer C, Forthmann C, et al. Resolving single-molecule assembled patterns with superresolution blink-microscopy. Nano Lett. (2010) 10:645–51. doi: 10.1021/nl903730r
33. Franz CM, Muller DJ. Analyzing focal adhesion structure by atomic force microscopy. J Cell Sci. (2005) 118:5315–23. doi: 10.1242/jcs.02653
34. Harke B, Chacko JV, Haschke H, Canale C, Diaspro A. A novel nanoscopic tool by combining AFM with STED microscopy. Opt Nanoscopy. (2012) 1:3. doi: 10.1186/2192-2853-1-3
35. Rief M, Gautel M, Gaub HE. Unfolding forces of titin and fibronectin domains directly measured by AFM. Adv Exp Med Biol. (2000) 481:129–41. doi: 10.1007/978-1-4615-4267-4_8
36. Bek A, Jansen R, Ringler M, Mayilo S, Klar TA. Fluorescence enhancement in hot spots of AFM-designed gold nanoparticle sandwiches. Nano Lett. (2008) 8:485–90. doi: 10.1021/nl072602n
37. Caldemeyer KS, Buckwalter KA. The basic principles of computed tomography and magnetic resonance imaging. J Am Acad Dermatol. (1999) 41:768–71. doi: 10.1016/S0190-9622(99)70015-0
38. Damen FW, Berman AG, Soepriatna AH, Ellis JM, Buttars SD, Aasa KL, et al. High-frequency 4-Dimensional Ultrasound (4DUS): a reliable method for assessing murine cardiac function. Tomography. (2017) 3:180–7. doi: 10.18383/j.tom.2017.00016
39. Chow MSM, Wu SL, Webb SE, Gluskin K, Yew DT. Functional magnetic resonance imaging and the brain: a brief review. World J Radiol. (2017) 9:5–9. doi: 10.4329/wjr.v9.i1.5
40. Carter LM, Kesner AL, Pratt EC, Sanders VA, Massicano AVF, Cutler CS, et al. The impact of positron range on PET resolution, evaluated with phantoms and PHITS monte carlo simulations for conventional and non-conventional radionuclides. Mol Imaging Biol. (2020) 22:73–84. doi: 10.1007/s11307-019-01337-2
41. Knopp T, Gdaniec N, Moddel M. Magnetic particle imaging: from proof of principle to preclinical applications. Phys Med Biol. (2017) 62:R124–78. doi: 10.1088/1361-6560/aa6c99
42. Khramtsov VV. In vivo molecular electron paramagnetic resonance-based spectroscopy and imaging of tumor microenvironment and redox using functional paramagnetic probes. Antioxid Redox Signal. (2018) 28:1365–77. doi: 10.1089/ars.2017.7329
43. Gonet M, Epel B, Halpern HJ, Elas M. Merging preclinical EPR tomography with other imaging techniques. Cell Biochem Biophys. (2019) 77:187–96. doi: 10.1007/s12013-019-00880-7
44. Jiang Y, Pu K. Molecular fluorescence and photoacoustic imaging in the second near-infrared optical window using organic contrast agents. Adv Biosyst. (2018) 2:1700262. doi: 10.1002/adbi.201700262
45. Yao Z, Zhang BS, Prescher JA. Advances in bioluminescence imaging: new probes from old recipes. Curr Opin Chem Biol. (2018) 45:148–56. doi: 10.1016/j.cbpa.2018.05.009
46. Manni I, de Latouliere L, Gurtner A, Piaggio G. Transgenic animal models to visualize cancer-related cellular processes by bioluminescence imaging. Front Pharmacol. (2019) 10:235. doi: 10.3389/fphar.2019.00235
47. Dorsaz S, Coste AT, Sanglard D. Red-shifted firefly luciferase optimized for Candida albicans In vivo bioluminescence imaging. Front Microbiol. (2017) 8:1478. doi: 10.3389/fmicb.2017.01478
48. Kuo C, Coquoz O, Troy TL, Xu H, Rice BW. Three-dimensional reconstruction of in vivo bioluminescent sources based on multispectral imaging. J Biomed Opt. (2007) 12:024007. doi: 10.1117/1.2717898
49. Vahrmeijer AL, Hutteman M, van der Vorst JR, van de Velde CJ, Frangioni JV. Image-guided cancer surgery using near-infrared fluorescence. Nat Rev Clin Oncol. (2013) 10:507–18. doi: 10.1038/nrclinonc.2013.123
50. Kenry Duan Y, Liu B. Recent advances of optical imaging in the second near-infrared window. Adv Mater. (2018) 30:e1802394. doi: 10.1002/adma.201802394
51. Ale A, Ermolayev V, Herzog E, Cohrs C, de Angelis MH, Ntziachristos V. FMT-XCT: in vivo animal studies with hybrid fluorescence molecular tomography-X-ray computed tomography. Nat Methods. (2012) 9:615–20. doi: 10.1038/nmeth.2014
52. Xu M, Wang LV. Photoacoustic imaging in biomedicine. Rev Sci Instruments. (2006) 77:041101. doi: 10.1063/1.2195024
53. Blery P, Pilet P, Bossche AV, Thery A, Guicheux J, Amouriq Y, et al. Vascular imaging with contrast agent in hard and soft tissues using microcomputed-tomography. J Microsc. (2016) 262:40–9. doi: 10.1111/jmi.12339
54. Kerckhofs G, Stegen S, van Gastel N, Sap A, Falgayrac G, Penel G, et al. Simultaneous three-dimensional visualization of mineralized and soft skeletal tissues by a novel microCT contrast agent with polyoxometalate structure. Biomaterials. (2018) 159:1–12. doi: 10.1016/j.biomaterials.2017.12.016
55. Huang D, Swanson EA, Lin CP, Schuman JS, Stinson WG, Chang W, et al. Optical coherence tomography. Science. (1991) 254:1178–81. doi: 10.1126/science.1957169
56. Drexler W, Fujimoto JG. Optical Coherence Tomography: Technology and Applications. Basel: Springer International Publishing Switzerland. (2015).
57. Bovenkamp D, Sentosa R, Rank E, Erkkilä M, Placzek F, Püls J, et al. Combination of high-resolution optical coherence tomography and raman spectroscopy for improved staging and grading in bladder cancer. Appl Sci. (2018) 8:2371. doi: 10.3390/app8122371
58. Li J, Bower AJ, Vainstein V, Gluzman-Poltorak Z, Chaney EJ, Marjanovic M, et al. Effect of recombinant interleukin-12 on murine skin regeneration and cell dynamics using in vivo multimodal microscopy. Biomed Opt Express. (2015) 6:4277–87. doi: 10.1364/BOE.6.004277
59. König K. Hybrid multiphoton multimodal tomography of in vivo human skin. IntraVital. (2012) 1:11–26. doi: 10.4161/intv.21938
60. Hanson KM, Bardeen CJ. Application of nonlinear optical microscopy for imaging skin. Photochem Photobiol. (2009) 85:33–44. doi: 10.1111/j.1751-1097.2008.00508.x
61. Andreana M, Sentosa R, Erkkilä MT, Drexler W, Unterhuber A. Depth resolved label-free multimodal optical imaging platform to study morpho-molecular composition of tissue. Photochem Photobiol Sci. (2019) 18:997–1008. doi: 10.1039/C8PP00410B
62. Alex A, Weingast J, Weinigel M, Kellner-Höfer M, Nemecek R, Binder M, et al. Three-dimensional multiphoton/optical coherence tomography for diagnostic applications in dermatology. J Biophotonics. (2013) 6:352–62. doi: 10.1002/jbio.201200085
63. Drexler W, Liu M, Kumar A, Kamali T, Unterhuber A, Leitgeb RA. Optical coherence tomography today: speed, contrast, and multimodality. J Biomed Opt. (2014) 19:071412. doi: 10.1117/1.JBO.19.7.071412
64. Jun X, Wang LV. Small-animal whole-body photoacoustic tomography: a review. IEEE Trans Biomed Eng. (2014) 61:1380–9. doi: 10.1109/TBME.2013.2283507
65. Zhou Y, Yao J, Wang LV. Tutorial on photoacoustic tomography. J Biomed Opt. (2016) 21:061007. doi: 10.1117/1.JBO.21.6.061007
66. Zhang W, Li Y, Nguyen VP, Huang Z, Liu Z, Wang X, et al. High-resolution, in vivo multimodal photoacoustic microscopy, optical coherence tomography, and fluorescence microscopy imaging of rabbit retinal neovascularization. Light Sci Appl. (2018) 7:103. doi: 10.1038/s41377-018-0093-y
67. Liu M, Drexler W. Optical coherence tomography angiography and photoacoustic imaging in dermatology. Photochem Photobiol Sci. (2019) 18:945–62. doi: 10.1039/C8PP00471D
68. Cai W, Chen X. Multimodality molecular imaging of tumor angiogenesis. J Nucl Med. (2008) 49(Suppl. 2):113–28S. doi: 10.2967/jnumed.107.045922
69. Labernadie A, Thibault C, Vieu C, Maridonneau-Parini I, Charriere GM. Dynamics of podosome stiffness revealed by atomic force microscopy. Proc Natl Acad Sci USA. (2010) 107:21016–21. doi: 10.1073/pnas.1007835107
70. Vollnhals F, Audinot J-N, Wirtz T, Mercier-Bonin M, Fourquaux I, Schroeppel B, et al. Correlative microscopy combining secondary ion mass spectrometry and electron microscopy: comparison of intensity-hue-saturation and laplacian pyramid methods for image fusion. Anal Chem. (2017) 89:10702–10. doi: 10.1021/acs.analchem.7b01256
71. Behrens S, Lösekann T, Pett-Ridge J, Weber PK, Ng WO, Stevenson BS, et al. Linking microbial phylogeny to metabolic activity at the single-cell level by using enhanced element labeling-catalyzed reporter deposition fluorescence in situ hybridization (EL-FISH) and NanoSIMS. Appl Environ Microbiol. (2008) 74:3143–50. doi: 10.1128/AEM.00191-08
72. Geier BK, Sogin E, Michellod D, Janda M, Kompauer M, Spengler B, et al. Spatial metabolomics of in situ, host-microbe interactions. (2020) bioRxiv 2019:555045. doi: 10.1101/555045
73. Vachet RW. Molecular histology: More than a picture. Nat Nanotechnol. (2015) 10:103–4. doi: 10.1038/nnano.2015.4.
74. Holzlechner M, Bonta M, Lohninger H, Limbeck A, Marchetti-Deschmann M. Multi-sensor Imaging - from sample preparation to integrated multimodal interpretation of LA-ICP-MS and MALDI MS imaging data. Anal Chem. (2018) 90:8831–7. doi: 10.1021/acs.analchem.8b00816
75. Fleming Y, Wirtz T. High sensitivity and high resolution element 3D analysis by a combined SIMS-SPM instrument. Beilstein J Nanotechnol. (2015) 6:1091–9. doi: 10.3762/bjnano.6.110
76. Menendez MI, Hettlich B, Wei L, Knopp MV. Preclinical multimodal molecular imaging using (18)F-FDG PET/CT and MRI in a phase i study of a knee osteoarthritis in In vivo canine Model. Mol Imaging. (2017) 16:1536012117697443. doi: 10.1177/1536012117697443
77. Chehade M, Srivastava AK, Bulte JW. Co-registration of bioluminescence tomography, computed tomography, and magnetic resonance imaging for multimodal in vivo stem cell tracking. Tomography. (2016) 2:159–65. doi: 10.18383/j.tom.2016.00160
78. Zhou L, Cai M, Tong T, Wang H. Progress in the correlative atomic force microscopy and optical microscopy. Sensors. (2017) 17:938. doi: 10.3390/s17040938
79. Cebulla J, Kim E, Rhie K, Zhang J, Pathak AP. Multiscale and multi-modality visualization of angiogenesis in a human breast cancer model. Angiogenesis. (2014) 17:695–709. doi: 10.1007/s10456-014-9429-2
80. Karreman MA, Mercier L, Schieber NL, Shibue T, Schwab Y, Goetz JG. Correlating intravital multi-photon microscopy to 3D electron microscopy of invading tumor cells using anatomical reference points. PLoS ONE. (2014) 9:e114448. doi: 10.1371/journal.pone.0114448
81. Karreman MA, Mercier L, Schieber NL, Solecki G, Allio G, Winkler F, et al. Fast and precise targeting of single tumor cells in vivo by multimodal correlative microscopy. J Cell Sci. (2016) 129:444–56. doi: 10.1242/jcs.181842
82. Pacureanu A, Maniates-Selvin J, Kuan AT, Thomas LA, Chen C-L, Cloetens P, et al. Dense neuronal reconstruction through X-ray holographic nano-tomography. bioRxiv. (2019) 653188. doi: 10.1101/653188
83. Boido D, Rungta RL, Osmanski BF, Roche M, Tsurugizawa T, Le Bihan D, et al. Mesoscopic and microscopic imaging of sensory responses in the same animal. Nat Commun. (2019) 10:1110. doi: 10.1038/s41467-019-09082-4
84. Desgrange A, Lokmer J, Marchiol C, Houyel L, Meilhac SM. Standardised imaging pipeline for phenotyping mouse laterality defects and associated heart malformations, at multiple scales and multiple stages. Dis Model Mech. (2019) 12:dmm038356. doi: 10.1101/516039
85. Liu M, Maurer B, Hermann B, Zabihian B, Sandrian MG, Unterhuber A, et al. Dual modality optical coherence and whole-body photoacoustic tomography imaging of chick embryos in multiple development stages. Biomed Opt Express. (2014) 5:3150–9. doi: 10.1364/BOE.5.003150
86. Svirkova A, Turyanskaya A, Perneczky L, Streli C, Marchetti-Deschmann M. Multimodal imaging of undecalcified tissue sections by MALDI MS and muXRF. Analyst. (2018) 143:2587–95. doi: 10.1039/C8AN00313K
87. Litjens G, Kooi T, Bejnordi BE, Setio AAA, Ciompi F, Ghafoorian M, et al. A survey on deep learning in medical image analysis. Med Image Anal. (2017) 42:60–88. doi: 10.1016/j.media.2017.07.005
88. Roels J, Aelterman J, Luong HQ, Lippens S, Pižurica A, Saeys Y, et al. An overview of state-of-the-art image restoration in electron microscopy. J Microsc. (2018) 271:239–54. doi: 10.1111/jmi.12716
89. Akselrod-Ballin A, Dafni H, Addadi Y, Biton I, Avni R, Brenner Y, et al. Multimodal correlative preclinical whole body imaging and segmentation. Sci Rep. (2016) 6:27940. doi: 10.1038/srep27940
90. Schindelin J, Arganda-Carreras I, Frise E, Kaynig V, Longair M, Pietzsch T, et al. Fiji: an open-source platform for biological-image analysis. Nat Methods. (2012) 9:676–82. doi: 10.1038/nmeth.2019
91. Heiligenstein X, Paul-Gilloteaux P, Raposo G, Salamero J. eC-CLEM: a multidimension, multimodel software to correlate intermodal images with a focus on light and electron microscopy. Methods Cell Biol. 140:335–52. doi: 10.1016/bs.mcb.2017.03.014
92. Miura K, Paul-Gilloteaux P, Tosi S, Colombelli J. Workflows and components of bioimage analysis. In: Miura K, Sladoje N, editors. Bioimage Data Analysis Workflows. Learning Materials in Biosciences. Cham: Springer (2020) p. 1–7.
93. Paul Gilloteaux P, Schorb M. Correlating data from imaging modalities. In: Verkade P, Collinson L, editors. Correlative Imaging, Focusing on the Future. Wiley (2019) p. 191–210.
94. Sotiras A, Davatzikos C, Paragios N. Deformable medical image registration: a survey. IEEE Trans Med Imaging. (2013) 32:1153–90. doi: 10.1109/TMI.2013.2265603
95. Acosta BMT, Bouthemy P, Kervrann C. A common image representation and a patch-based search for correlative light-electron-microscopy (CLEM) registration. In: IEEE 13th International Symposium on Biomedical Imaging (ISBI). Prague (2016). p. 257–260.
96. Acosta BMT, Heiligenstein X, Malandain G, Bouthemy P. Intensity-based matching and registration for 3D correlative microscopy with large discrepancies. In: IEEE 15th International Symposium on Biomedical Imaging (ISBI 2018). Washington, DC (2018). p. 493–496.
97. Cao T, Zach C, Modla S, Powell D, Czymmek K, Niethammer M. Multi-modal registration for correlative microscopy using image analogies. Med Image Anal. (2014) 18:914–26. doi: 10.1016/j.media.2013.12.005
98. Gutierrez-Becker B, Mateus D, Peter L, Navab N. Guiding multimodal registration with learned optimization updates. Med Image Anal. (2017) 41:2–17. doi: 10.1016/j.media.2017.05.002
99. Blendowski M, Bouteldja N, Heinrich MP. Multimodal 3D medical image registration guided by shape encoder-decoder networks. Int J Comput Assist Radiol Surg. (2019) 15:1–8. doi: 10.1007/s11548-019-02089-8
100. Huang X, Zhang J, Fan L, Wu Q, Yuan C. A systematic approach for cross-source point cloud registration by preserving macro and micro structures. IEEE Trans Image Process. (2017) 26:3261–76. doi: 10.1109/TIP.2017.2695888
101. Hu Y, Modat M, Gibson E, Li W, Ghavami N, Bonmati E, et al. Weakly-supervised convolutional neural networks for multimodal image registration. Med Image Anal. (2018) 49:1–13. doi: 10.1016/j.media.2018.07.002
102. Arganda-Carreras I, Manoliu T, Mazuras N, Schulze F, Iglesias JE, Buhler K, et al. A statistically representative atlas for mapping neuronal circuits in the Drosophila adult brain. Front Neuroinform. (2018) 12:13. doi: 10.3389/fninf.2018.00013
103. Muenzing SEA, Strauch M, Truman JW, Buhler K, Thum AS, Merhof D. larvalign: aligning gene expression patterns from the larval brain of Drosophila melanogaster. Neuroinformatics. (2018) 16:65–80. doi: 10.1007/s12021-017-9349-6
104. Schlachter M, Fechter T, Adebahr S, Schimek-Jasch T, Nestle U, Bühler K. Visualization of 4D multimodal imaging data and its applications in radiotherapy planning. J Appl Clin Med Phys. (2017) 18:183–93. doi: 10.1002/acm2.12209
105. Schlachter M, Fechter T, Jurisic M, Schimek-Jasch T, Oehlke O, Adebahr S, et al. Visualization of deformable image registration quality using local image dissimilarity. IEEE Trans Med Imaging. (2016) 35:2319–28. doi: 10.1109/TMI.2016.2560942
106. Heiligenstein X, Paul-Gilloteaux P, Belle M, Raposo G, Salamero J. eC-CLEM: flexible multidimensional registration software for correlative microscopies with refined accuracy mapping. Microsc Microanal. (2017) 23:360–1. doi: 10.1017/S1431927617002483
107. Ganglberger F, Swoboda N, Frauenstein L, Kaczanowska J, Haubensak W, Bühler K. BrainTrawler: a visual analytics framework for iterative exploration of heterogeneous big brain data. Comput Graphics. (2019) 82:304–20. doi: 10.1016/j.cag.2019.05.032
108. Pietzsch T, Saalfeld S, Preibisch S, Tomancak P. BigDataViewer: visualization and processing for large image data sets. Nat Methods. (2015) 12:481–3. doi: 10.1038/nmeth.3392
109. Brown E, Verkade P. The use of markers for correlative light electron microscopy. Protoplasma. (2010) 244:91–7. doi: 10.1007/s00709-010-0165-1
110. Kandela IK, Albrecht RM. Fluorescence quenching by colloidal heavy metals nanoparticles: implications for correlative fluorescence and electron microscopy studies. Scanning. (2007) 29:152–61. doi: 10.1002/sca.20055
111. Gorelick S, Buckley G, Gervinskas G, Johnson TK, Handley A, Caggiano MP, et al. PIE-scope, integrated cryo-correlative light and FIB/SEM microscopy. Elife. (2019) 8:e45919. doi: 10.7554/eLife.45919
112. Pfister G, Stroh CM, Perschinka H, Kind M, Knoflach M, Hinterdorfer P, et al. Detection of HSP60 on the membrane surface of stressed human endothelial cells by atomic force and confocal microscopy. J Cell Sci. (2005) 118:1587–94. doi: 10.1242/jcs.02292
113. Prinz F, Schlange T, Asadullah K. Believe it or not: how much can we rely on published data on potential drug targets? Nat Rev Drug Discov. (2011) 10:712. doi: 10.1038/nrd3439-c1
114. Begley CG, Ellis LM. Drug development: raise standards for preclinical cancer research. Nature. (2012) 483:531–3. doi: 10.1038/483531a
115. Begley CG, Ioannidis JP. Reproducibility in science: improving the standard for basic and preclinical research. Circ Res. (2015) 116:116–26. doi: 10.1161/CIRCRESAHA.114.303819
116. Peers IS, Ceuppens PR, Harbron C. In search of preclinical robustness. Nat Rev Drug Discov. (2012) 11:733–4. doi: 10.1038/nrd3849
117. Lieu CH, Tan AC, Leong S, Diamond JR, Eckhardt SG. From bench to bedside: lessons learned in translating preclinical studies in cancer drug development. J Natl Cancer Inst. (2013) 105:1441–56. doi: 10.1093/jnci/djt209
118. Herfert K, Mannheim JG, Kuebler L, Marciano S, Amend M, Parl C, et al. Quantitative rodent brain receptor imaging. Mol Imaging Biol. (2020) 22:223–44. doi: 10.1007/s11307-019-01368-9
119. Roy Choudhury K, Gibson R. Reproducible research in medical imaging. Mol Imaging Biol. (2012) 14:395–6. doi: 10.1007/s11307-012-0569-8
120. Hackam DG, Redelmeier DA. Translation of research evidence from animals to humans. JAMA. (2006) 296:1731–2. doi: 10.1001/jama.296.14.1731
121. Llovera G, Liesz A. The next step in translational research: lessons learned from the first preclinical randomized controlled trial. J Neurochem. (2016) 139(Suppl. 2):271–9. doi: 10.1111/jnc.13516
122. McNutt M. Journals unite for reproducibility. Science. (2014) 346:679. doi: 10.1126/science.aaa1724
123. Zhu Y, Geng C, Huang J, Liu J, Wu N, Xin J, et al. Measurement and evaluation of quantitative performance of PET/CT images before a multicenter clinical trial. Sci Rep. 2018. 8:9035. doi: 10.1038/s41598-018-27143-4
124. Hristova I, Boellaard R, Galette P, Shankar LK, Liu Y, Stroobants S, et al. Guidelines for quality control of PET/CT scans in a multicenter clinical study. EJNMMI Phys. (2017) 4:23. doi: 10.1186/s40658-017-0190-7
125. Aide N, Lasnon C, Veit-Haibach P, Sera T, Sattler B, Boellaard R. EANM/EARL harmonization strategies in PET quantification: from daily practice to multicentre oncological studies. Eur J Nucl Med Mol Imaging. (2017) 44(Suppl 1):17–31. doi: 10.1007/s00259-017-3740-2
126. Murphy P, Koh DM. Imaging in clinical trials. Cancer Imaging. (2010) 10:S74–82. doi: 10.1102/1470-7330.2010.9027
127. van Horn JD, Toga AW. Multisite neuroimaging trials. Curr Opin Neurol. (2009) 22:370–8. doi: 10.1097/WCO.0b013e32832d92de
128. Stout D, Berr SS, LeBlanc A, Kalen JD, Osborne D, Price J, et al. Guidance for methods descriptions used in preclinical imaging papers. Mol Imaging. (2013) 12:1–15. doi: 10.2310/7290.2013.00055
129. Kilkenny C, Browne WJ, Cuthill IC, Emerson M, Altman DG. Improving bioscience research reporting: the ARRIVE guidelines for reporting animal research. PLoS Biol. (2010) 8:e1000412. doi: 10.1371/journal.pbio.1000412
130. Data Availability. (2019). Available online at: https://journals.plos.org/plosone/s/data-availability (accessed November 16, 2019).
131. Data availability statements and data citations policy: guidance for authors. Available online at: https://www.nature.com/documents/nr-data-availability-statements-data-citations.pdf. (accessed November 16, 2019)
132. Wilkinson MD, Dumontier M, Aalbersberg IJ, Appleton G, Axton M, Baak A, et al. The FAIR guiding principles for scientific data management and stewardship. Sci Data. (2016) 3:160018. doi: 10.1038/sdata.2016.18
133. Mannheim JG, Mamach M, Reder S, Traxl A, Mucha N, Disselhorst JA, et al. Reproducibility and comparability of preclinical PET imaging data: a multicenter small-animal PET study. J Nucl Med. (2019) 60:1483–91. doi: 10.2967/jnumed.118.221994
134. Voelkl B, Vogt L, Sena ES, Wurbel H. Reproducibility of preclinical animal research improves with heterogeneity of study samples. PLoS Biol. (2018) 16:e2003693. doi: 10.1371/journal.pbio.2003693
135. Bath PM, Macleod MR, Green AR. Emulating multicentre clinical stroke trials: a new paradigm for studying novel interventions in experimental models of stroke. Int J Stroke. (2009). 4:471–9. doi: 10.1111/j.1747-4949.2009.00386.x
136. Dirnagl U, Hakim A, Macleod M, Fisher M, Howells D, Alan SM, et al. A concerted appeal for international cooperation in preclinical stroke research. Stroke. (2013) 44:1754–60. doi: 10.1161/STROKEAHA.113.000734
137. Lefer DJ, Bolli R. Development of an NIH consortium for preclinicAl AssESsment of CARdioprotective therapies (CAESAR): a paradigm shift in studies of infarct size limitation. J Cardiovasc Pharmacol Ther. (2011). 16:332–9. doi: 10.1177/1074248411414155
138. Gueld MO, Kohnen M, Keysers D, Schubert H, Wein BB, Bredno J, et al. Quality of DICOM header information for image categorization. Proc. SPIE 4685, Medical Imaging 2002: PACS and Integrated Medical Information Systems: Design and Evaluation, p. 280–7. doi: 10.1117/12.467017
139. Iudin A, Korir PK, Salavert-Torres J, Kleywegt GJ, Patwardhan A. EMPIAR: a public archive for raw electron microscopy image data. Nat Methods. (2016) 13:387–8. doi: 10.1038/nmeth.3806
140. Bourne RM, Bailey C, Johnston EW, Pye H, Heavey S, Whitaker H, et al. Apparatus for histological validation of in vivo and ex vivo magnetic resonance imaging of the human prostate. Front Oncol. (2017) 7:47. doi: 10.3389/fonc.2017.00047
141. Ellenberg J, Swedlow JR, Barlow M, Cook CE, Sarkans U, Patwardhan A, et al. A call for public archives for biological image data. Nat Methods. (2018) 15:849–54. doi: 10.1038/s41592-018-0195-8
142. Schellenberger P, Kaufmann R, Siebert CA, Hagen C, Wodrich H, Grunewald K. High-precision correlative fluorescence and electron cryo microscopy using two independent alignment markers. Ultramicroscopy. (2014) 143:41–51. doi: 10.1016/j.ultramic.2013.10.011
143. Cohen EAK, Ober RJ. Analysis of Point based image registration errors with applications in single molecule microscopy. IEEE Trans Signal Process. (2013) 61:6291–306. doi: 10.1109/TSP.2013.2284154
144. Fitzpatrick JM, West JB. The distribution of target registration error in rigid-body point-based registration. IEEE Trans Med Imaging. (2001) 20:917–27. doi: 10.1109/42.952729
145. Xiao Y, Rivaz H, Chabanas M, Fortin M, Machado I, Ou Y, et al. Evaluation of MRI to ultrasound registration methods for brain shift correction: the CuRIOUS2018 challenge. IEEE Trans Med Imaging. (2020) 39:777–86. doi: 10.1109/TMI.2019.2935060
146. Sartori A, Gatz R, Beck F, Rigort A, Baumeister W, Plitzko JM. Correlative microscopy: bridging the gap between fluorescence light microscopy and cryo-electron tomography. J Struct Biol. (2007) 160:135–45. doi: 10.1016/j.jsb.2007.07.011
147. Schwartz CL, Sarbash VI, Ataullakhanov FI, McIntosh JR, Nicastro D. Cryo-fluorescence microscopy facilitates correlations between light and cryo-electron microscopy and reduces the rate of photobleaching. J Microsc. (2007) 227:98–109. doi: 10.1111/j.1365-2818.2007.01794.x
148. Schorb M, Briggs JA. Correlated cryo-fluorescence and cryo-electron microscopy with high spatial precision and improved sensitivity. Ultramicroscopy. (2014) 143:24–32. doi: 10.1016/j.ultramic.2013.10.015
149. Tuijtel MW, Koster AJ, Jakobs S, Faas FGA, Sharp TH. Correlative cryo super-resolution light and electron microscopy on mammalian cells using fluorescent proteins. Sci Rep. (2019) 9:1369. doi: 10.1038/s41598-018-37728-8
150. Arnold J, Mahamid J, Lucic V, de Marco A, Fernandez JJ, Laugks T, et al. Site-specific Cryo-focused ion beam sample preparation guided by 3D correlative microscopy. Biophys J. (2016) 110:860–9. doi: 10.1016/j.bpj.2015.10.053
151. Agronskaia AV, Valentijn JA, van Driel LF, Schneijdenberg CT, Humbel BM, van Bergen en Henegouwen PM, et al. Integrated fluorescence and transmission electron microscopy. J Struct Biol. (2008) 164:183–9. doi: 10.1016/j.jsb.2008.07.003
152. Peddie CJ, Liv N, Hoogenboom JP, Collinson LM. Integrated light and scanning electron microscopy of GFP-expressing cells. Methods Cell Biol. (2014) 124:363–89. doi: 10.1016/B978-0-12-801075-4.00017-3
153. Fabritius G, Brix G, Nekolla E, Klein S, Popp HD, Meyer M, et al. Cumulative radiation exposure from imaging procedures and associated lifetime cancer risk for patients with lymphoma. Sci Rep. (2016) 6:35181. doi: 10.1038/srep35181
154. Salminen E, Niiniviita H, Jarvinen H, Heinavaara S. Cancer death risk related to radiation exposure from computed tomography scanning among testicular cancer patients. Anticancer Res. (2017) 37:831–4. doi: 10.21873/anticanres.11385
155. Clark DP, Badea CT. Hybrid spectral CT reconstruction. PLoS ONE. (2017) 12:e0180324. doi: 10.1371/journal.pone.0180324
156. Trojanova E, Schyns L, Ludwig D, Jakubek J, Le Pape A, Sefc L, et al. Tissue sensitive imaging and tomography without contrast agents for small animals with Timepix based detectors. J Instrum. (2017) 12:C01056. doi: 10.1088/1748-0221/12/01/C01056
157. Turecek D, Jakubek J, Trojanova E, Sefc L, Kolarova V. Application of timepix3 based CdTe spectral sensitive photon counting detector for PET imaging. Nucl Instrum Methods Phys Res A. (2018) 895:84–9. doi: 10.1016/j.nima.2018.04.007
158. Trojanova E, Jakubek J, Turecek D, Sykora V, Francova P, Kolarova V, et al. Evaluation of Timepix3 based CdTe photon counting detector for fully spectroscopic small animal SPECT imaging. J Instrum. (2018) 13:C01001. doi: 10.1088/1748-0221/13/01/C01001
159. Turecek D, Jakubek J, Trojanova E, Sefc L. Compton camera based on Timepix3 technology. J Instrum. (2018) 13:C11022. doi: 10.1088/1748-0221/13/11/C11022
160. Sakai M, Yamaguchi M, Nagao Y, Kawachi N, Kikuchi M, Torikai K, et al. In vivo simultaneous imaging with (99m)Tc and (18)F using a Compton camera. Phys Med Biol. (2018) 63:205006. doi: 10.1088/1361-6560/aae1d1
161. Bruns OT, Bischof TS, Harris DK, Franke D, Shi Y, Riedemann L, et al. Next-generation in vivo optical imaging with short-wave infrared quantum dots. Nat Biomed Eng. (2017) 1:0056. doi: 10.1038/s41551-017-0056
162. George J, Van Wettere AJ, Michaels BB, Crain D, Lewbart GA. Histopathologic evaluation of postmortem autolytic changes in bluegill (Lepomis macrohirus) and crappie (Pomoxis anularis) at varied time intervals and storage temperatures. PeerJ. (2016) 4:e1943. doi: 10.7717/peerj.1943
163. Copper JE, Budgeon LR, Foutz CA, van Rossum DB, Vanselow DJ, Hubley MJ, et al. Comparative analysis of fixation and embedding techniques for optimized histological preparation of zebrafish. Comp Biochem Physiol C Toxicol Pharmacol. (2018) 208:38–46. doi: 10.1016/j.cbpc.2017.11.003
164. Kaufmann R, Hagen C, Grunewald K. Fluorescence cryo-microscopy: current challenges and prospects. Curr Opin Chem Biol. (2014) 20:86–91. doi: 10.1016/j.cbpa.2014.05.007
165. Heimel P, Swiadek NV, Slezak P, Kerbl M, Schneider C, Nurnberger S, et al. Iodine-enhanced micro-CT imaging of soft tissue on the example of peripheral nerve regeneration. Contrast Media Mol Imaging. (2019) 2019:7483745. doi: 10.1155/2019/7483745
166. Ouzounov DG, Wang T, Wang M, Feng DD, Horton NG, Cruz-Hernandez JC, et al. In vivo three-photon imaging of activity of GCaMP6-labeled neurons deep in intact mouse brain. Nat Methods. (2017) 14:388–90. doi: 10.1038/nmeth.4183
167. Ding F, Zhan Y, Lu X, Sun Y. Recent advances in near-infrared II fluorophores for multifunctional biomedical imaging. Chem Sci. (2018) 9:4370–80. doi: 10.1039/C8SC01153B
168. van den Berg PJ, Daoudi K, Steenbergen W. Review of photoacoustic flow imaging: its current state and its promises. Photoacoustics. (2015) 3:89–99. doi: 10.1016/j.pacs.2015.08.001
169. Schorb M, Gaechter L, Avinoam O, Sieckmann F, Clarke M, Bebeacua C, et al. New hardware and workflows for semi-automated correlative cryo-fluorescence and cryo-electron microscopy/tomography. J Struct Biol. (2017) 197:83–93. doi: 10.1016/j.jsb.2016.06.020
170. Lo YH, Liao C-T, Zhou J, Rana A, Bennett C, Murnane M, et al. Multimodal x-ray and electron microscopy of the Allende meteorite. Sci Adv. (2019) 5:eaax3009. doi: 10.1126/sciadv.aax3009
171. Colom A, Casuso I, Rico F, Scheuring S. A hybrid high-speed atomic force-optical microscope for visualizing single membrane proteins on eukaryotic cells. Nat Commun. (2013) 4:2155. doi: 10.1038/ncomms3155
172. Handschuh-Wang S, Wang T, Zhou X. Recent advances in hybrid measurement methods based on atomic force microscopy and surface sensitive measurement techniques. RSC Adv. (2017) 7:47464–47499. doi: 10.1039/C7RA08515J
173. Haring MT, Liv N, Zonnevylle AC, Narvaez AC, Voortman LM, Kruit P, et al. Automated sub-5 nm image registration in integrated correlative fluorescence and electron microscopy using cathodoluminescence pointers. Sci Rep. (2017) 7:43621. doi: 10.1038/srep43621
174. Leitgeb RA, Baumann B. Multimodal optical medical imaging concepts based on optical coherence tomography. Front Phys. (2018) 6:00114. doi: 10.3389/fphy.2018.00114
175. Stuker F, Ripoll J, Rudin M. Fluorescence molecular tomography: principles and potential for pharmaceutical research. Pharmaceutics. (2011) 3:229–74. doi: 10.3390/pharmaceutics3020229
176. Prabhakar N, Belevich I, Peurla M, Heiligenstein X, Chang H-C, Sahlgren C, et al. Dynamic-ultrastructural cell volume (3D) correlative microscopy facilitated by intracellular fluorescent nanodiamonds as multi-modal probes. bioRxiv. (2019) 823278. doi: 10.1101/823278
177. Louie A. Multimodality imaging probes: design and challenges. Chem Rev. (2010) 110:3146–95. doi: 10.1021/cr9003538
178. Ose T, Autio JA, Ohno M, Nishigori K, Tanki N, Igesaka A, et al. A novel Tungsten-based fiducial marker for multi-modal brain imaging. J Neurosci Methods. (2019) 323:22–31. doi: 10.1016/j.jneumeth.2019.04.014
179. An FF, Chan M, Kommidi H, Ting R. Dual PET and near-infrared fluorescence imaging probes as tools for imaging in oncology. AJR Am J Roentgenol. (2016) 207:266–73. doi: 10.2214/AJR.16.16181
180. Bushong EA, Johnson DD Jr, Kim KY, Terada M, Hatori M, et al. X-ray microscopy as an approach to increasing accuracy and efficiency of serial block-face imaging for correlated light and electron microscopy of biological specimens. Microsc Microanal. (2015) 21:231–8. doi: 10.1017/S1431927614013579
181. Shu X, Lev-Ram V, Deerinck TJ, Qi Y, Ramko EB, Davidson MW, et al. A genetically encoded tag for correlated light and electron microscopy of intact cells, tissues, and organisms. PLoS Biol. (2011) 9:e1001041. doi: 10.1371/journal.pbio.1001041
182. Ariotti N, Hall TE, Parton RG. Correlative light and electron microscopic detection of GFP-labeled proteins using modular APEX. Methods Cell Biol. (2017) 140:105–21. doi: 10.1016/bs.mcb.2017.03.002
183. Rijnsoever Cv, Oorschot V, Klumperman J. Correlative light-electron microscopy (CLEM) combining live-cell imaging and immunolabeling of ultrathin cryosections. Nature Methods. (2008) 5:973–80. doi: 10.1038/nmeth.1263
184. Eberle AL, Mikula S, Schalek R, Lichtman J, Tate MLK, Zeidler D. High-resolution, high-throughput imaging with a multibeam scanning electron microscope. J Microsc. (2015) 259:114–20. doi: 10.1111/jmi.12224
185. Collinson LM, Carroll EC, Hoogenboom JP. Correlating 3D light to 3D electron microscopy for systems biology. Curr Opin Biomed Eng. (2017) 3:49–55. doi: 10.1016/j.cobme.2017.10.006
186. Hoehndorf R, Schofield PN, Gkoutos GV. The role of ontologies in biological and biomedical research: a functional perspective. Brief Bioinform. (2015) 16:1069–80. doi: 10.1093/bib/bbv011
187. Smith B, Arabandi S, Brochhausen M, Calhoun M, Ciccarese P, Doyle S, et al. Biomedical imaging ontologies: a survey and proposal for future work. J Pathol Inform. (2015) 6:37. doi: 10.4103/2153-3539.159214
188. Caicedo JC, Goodman A, Karhohs KW, Cimini BA, Ackerman J, Haghighi M, et al. Nucleus segmentation across imaging experiments: the 2018 data science bowl. Nat Methods. (2019) 16:1247–53. doi: 10.1038/s41592-020-0733-z
189. Li R, Zhang W, Suk H-I, Wang L, Li J, Shen D, et al. Deep learning based imaging data completion for improved brain disease diagnosis. Med Image Comput Comput Assist Interv. (2014) 17(Pt. 3):305–12. doi: 10.1007/978-3-319-10443-0_39
190. Weigert M, Schmidt U, Boothe T, Müller A, Dibrov A, Jain A, et al. Content-aware image restoration: pushing the limits of fluorescence microscopy. Nat Methods. (2018) 15:1090–7. doi: 10.1038/s41592-018-0216-7
Keywords: bioimaging, correlated multimodal imaging, CMI, COMULIS, CLEM, correlative microscopy, hybrid imaging, correlation software
Citation: Walter A, Paul-Gilloteaux P, Plochberger B, Sefc L, Verkade P, Mannheim JG, Slezak P, Unterhuber A, Marchetti-Deschmann M, Ogris M, Bühler K, Fixler D, Geyer SH, Weninger WJ, Glösmann M, Handschuh S and Wanek T (2020) Correlated Multimodal Imaging in Life Sciences: Expanding the Biomedical Horizon. Front. Phys. 8:47. doi: 10.3389/fphy.2020.00047
Received: 29 November 2019; Accepted: 20 February 2020;
Published: 09 April 2020.
Edited by:
Andreas Hess, University of Erlangen Nuremberg, GermanyReviewed by:
Maurits A. Jansen, University of Edinburgh, United KingdomMichael D. Noseworthy, McMaster University, Canada
Copyright © 2020 Walter, Paul-Gilloteaux, Plochberger, Sefc, Verkade, Mannheim, Slezak, Unterhuber, Marchetti-Deschmann, Ogris, Bühler, Fixler, Geyer, Weninger, Glösmann, Handschuh and Wanek. This is an open-access article distributed under the terms of the Creative Commons Attribution License (CC BY). The use, distribution or reproduction in other forums is permitted, provided the original author(s) and the copyright owner(s) are credited and that the original publication in this journal is cited, in accordance with accepted academic practice. No use, distribution or reproduction is permitted which does not comply with these terms.
*Correspondence: Andreas Walter, andreas.walter@vbcf.ac.at