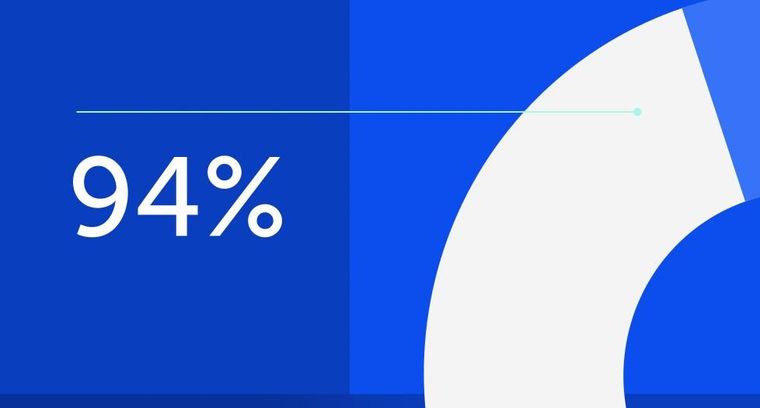
94% of researchers rate our articles as excellent or good
Learn more about the work of our research integrity team to safeguard the quality of each article we publish.
Find out more
ORIGINAL RESEARCH article
Front. Phys., 01 September 2015
Sec. Interdisciplinary Physics
Volume 3 - 2015 | https://doi.org/10.3389/fphy.2015.00065
This article is part of the Research TopicOpinions, Choices and Actions: Applications of Sociophysics to the diffusion of ideasView all 7 articles
The modeling of opinion dynamics in social systems has attracted a good deal of attention in the last decade. Even though based on intuition and observation, the mechanisms behind many of these models need solid empirical grounding. In this work, we investigate the relation among subjective variables (such as the personality), the dynamics of the affinity network dynamics, the communication patterns emerging throughout the social interactions and the opinions dynamics in a series of experiments with five small groups of ten people each. In order to ignite the discussion, the polemic topic of animal experimentation was proposed. The groups essentially polarized in two factions with a set of stubborn individuals (those not changing their opinions in time) playing the role of anchors. Our results suggest that the different layers present in the group dynamics (i.e., individual level, group dynamics and meso-communication) are deeply intermingled, specifically the stubbornness effect appears to be related to the dynamical features of the network topologies, and only in an undirected way to the personality of the participants.
Opinion dynamics modeling has witnessed a surge of interest in the last few years [1]. Most of these models are based on dyadic relations, producing sensible results within the framework of “large” human communities [2–4] including effects such as the influences of the clusterization as well as the minority or the extremist effects [5–7]. However, many real life social interactions take place in the small groups, where the rules of behavior may be more complex that those captured in dyadic models. The relevance of the so-called small group dynamics has generated an interesting scientific production from several domains [8–17]. In particular, the e-democracy applications [18–20], collective social problem solving, and the importance of a specific model for the opinion dynamics within the small group are currently evident.
In cognitive and social sciences, a small group is usually defined by 8–12 individuals, sharing the same environment, where frequent and regular interactions happen. The interaction among the members of the group are often characterized by some cognitive and affective factors, resulting in the merging of individual processes and group processes as well as in the interdependence among the individuals. The size of the small group allows participants to manage a large amount of cognitive and affective information, which leads to a complex prototype construction process prompting individuals to interact effectively with the other members of the group. In this way the relationships among individuals engaged in a small group are strongly linked to the “history” of the previous interactions, which is quite different from what happens in “large” groups where the high number of participants prevent from keeping an accurate track of the many qualities related to interactions.
The dynamics of small groups is thus determined by two scales: the individuals with their own evolution and intrinsic variables, and the group itself for which the interactions between individuals and their history are fundamental. The relation between the two scales follows a feedback loop with the local (i.e., the individual) dynamics shaping the global (i.e., group) dynamics, and vice-versa [21]. The complex interaction among such dynamics can be the key mechanism of several group phenomena, as the emergence of different communication topologies [22–25], the structuring of the social identity, the in-group/out-group effects [26, 27], as well as of the social influence processes [28–31].
The main target of this work is to explore the relationships among personality, communication dynamics, affinity network and opinion dynamics. Starting from the point of view proposed by Lewin in the Field theory [32], the role of the topology of the psychological field, defined as the product of the affinity between participants with their opinion difference, is explored so as to investigate the theorized interaction between personality and environment, where the behavior of people is described by Lewin as a complex function of personality or personal attitude, and the environment. In reference to the first of the two factors proposed by Lewin, the aspects related to the subjective variables, some more crystallized (i.e., structure of personality, age, sex), others more fluid and in some way more context related (i.e., state anxiety, opinion toward a specific topic) are considered. As for the second factor, a virtual environment where the participants interact controlling every feature and aspect of interaction with the community was designed.
According to Festinger [30], group pressures toward the uniformity of opinion have the function to preserve social reality (i.e., the reality built and shared by the members, as a reference point and a way to identify themselves with the group). Moreover, the theory of cognitive dissonance [33] describes the tendency to be coherent in our way of thinking and acting. Festinger's vision can well summarize the intertwined relationship between the individuals and the social environment, between personal opinion and the opinion of the group. The consensus and attitude changes in groups have been in the latest years the target of different approaches ranging from physics to social and cognitive sciences [34–37]. The public opinion and the public debates are today crucial ingredients in modern society policy making [38], and the dynamics of such processes are affected by a several factors ranging from the individual resistance to the change to the collective beliefs effect and the minorities role in the breaking of democratic opinion dynamics [7, 39]. Given the quasi-systematic co-occurrence of the previous described phenomena during a human group interaction, it is easy to understand how important is to consider all of them in the experimental design, as well as in the data interpretation. In our work, such considerations are taken into account by gathering some data about the “hidden” network that can affect the interactions among the participants and the opinion dynamics, therefore, such a network as the affinity among the individuals is considered. Our definition of affinity is the strength of the relationship between people, or their coupling in terms of social closeness [40].
This paper is structured as follows: in Section 2, the experimental design, briefly describing the chat environment where the participants interact is described. Subsequently, the variables gathered and the statistical analysis applied are presented. In Section 3, our main results of the interpretation of the experimental data are presented. In the last section, the conclusions of our work, and the deriving implication from the small group opinion dynamics interpretation are summarized.
The experimental condition and the virtual experimental environment were designed in order to investigate the opinion dynamics of a small group of totally unknown people, gathering their affinity network representations, their opinion at a certain time, and every aspect related to their communication networks (i.e., the public and private).
Every small group was involved in a discussion about animal experimentation within a chat-room environment for 45 min after a 10-min standardized training phase. The chat-room was divided into two parts: a public space (i.e., messages visible to all) and a private space (i.e., messages visible only to the recipient). The participants had to accompany their messages with a positive, neutral or negative mood, thus providing invaluable information about the mood of the textual message [41–43].
Furthermore, the participants had to manipulate two “radars”: a private radar was used by the participants to configure and to represent their personal affinity space, and a public radar which simulated a real room where the interaction took place, affecting the contrast of the font with which the messages were displayed (i.e., the distance between two participants made the message less readable, with less contrast, just as it occurs in a real physical environment where distance affects the loudness of the spoken message). The task required the subject to discuss animal experimentation, to negotiate and to mediate one's own opinion, without having to reach any consensus. The topic of discussion was chosen in order to polarize the opinions of the participants and to force the communication exchange of the participants around a specific topic.
In order to gather information about the structure of personality and the anxiety state of the participants in interaction, two self-report questionnaires before the beginning of the experimental session {i.e., the Five-Factor Adjective Short Test, (5-FasT) [44], and a reduced form of a test for anxiety (STAI) [45]} were administered.
The 5-FasT scoring classifies the subjects by means of 5 personality factors, describing the personality traits of the participants (Table 1).
The STAI measures two types of anxiety: state anxiety or anxiety about a con- text, and trait anxiety or anxiety level as a personal characteristic. It is worth noting that while the anxiety state usually changed frequently depending on the particular context the participants were facing, the anxiety trait was defined as a more stable feature (i.e., a psychological trait changes slowly requiring much more time). In order to appreciate the potential role of anxiety within our framework, only the measure related to the state of anxiety was considered.
After a standardized training phase, where instructions were administered to the participants, the opinions of each participant were recorded through a self-placement within the values 0–100 (totally unfavorable—totally favorable). This information was gathered at the beginning and at the end of every session, and after 15′, 25′, 35′, and 45′ of the interaction. In order to further the individuals' profiling, before the discussion, the participants were asked about their feelings of empathy toward animals, their perceived trust in science and their absolute final opinion (contrary or favorable) to animal experimentation. The absolute final opinion request had the role to force the participants to adopt only one of two possible votes, respectively labeled as favorable and unfavorable (see Table 2).
The data collected before, during and at the end of each experimental session was used to examine the trend of the order parameters describing the evolution of the system, both from the local (i.e., individual and dyadic communication and affinity dynamics) and the global (i.e., opinion distribution and global group dynamics) point of view. In the present study only the frequencies and the directions of the messages exchanged, the mood information and the moves on the two radars were considered. The first step of data analysis was to obtain the probability to observe an interaction between participants:
where is the sum of the messages sent by i to j at time window t. As a consequence we can define the activity of a subject i as:
As for the study of the several communication networks considered, the centrality degree was used, that produced the sum of the probabilities of connection between a subject i at time t and the rest of the network.
In addition to the centrality degree (), the betweenness degree , that provides some indications regarding the structural relevance of the node with respect to the configuration of the network [46, 47] were considered:
where is the shortest path passing trough i and connecting j and k, while is the total number of shortest paths connecting j and k.
As presented in our previous works [43], the different communicative dimensions shown in Table 3 for a precise and focused analysis of the communication networks were used. Moreover, the relationship between the opinion gathered and summarized in Table 2 and the characterizing parameters of the system (i.e., activity, centrality degree and betweenness degree) were explored. The degree centrality corresponds directly to the number of connections of each node, while the betweeness of a node i is related to the number of shortest paths joining every pair of nodes and crossing through i.
The experiments were conducted at the Psychology Department of the University of Florence, utilizing the voluntary collaboration of 50 participants (28 males and 22 females). The participants presented an average age of 25.88 (std.dev. 6.29), and were divided into 5 small groups of 10 people. Every small group was made up of individuals who were complete strangers, and the number of males and females for each group was balanced in order to have the same distribution for each small group.
A classical statistical approach was used to describe and define the weight of the subjective factors on the dynamics of communication, opinion and affinity. A discriminant function analysis [48] was applied in order to evaluate which parameters allowed us to classify a dyad as coherent or incoherent (i.e., a couple of participants equipped with the same or two different opinions), or to identify the best predicting factors of the individual's opinion changing (i.e., the change of the opinion with respect to the initial one). Discriminant function analysis is a statistical analysis able to forecast a categorical dependent variable (i.e., grouping variable) starting by one or more continuous or binary independent variables (i.e., predictor variables). The discriminant functions explained variance is revealed by means of the canonical correlation index. The canonical correlation reveals the association between each variables in the model and the discriminant functions. It allows us to compare correlations and see how closely a variable is related to each function [49]. Given the relative reduced size of our datasets, we are not considering more involved (generalized, information theory or non-linear) correlation measures. Simpler estimators such as the canonical correlations should be able to capture better the signal.
Generally, any variables with a correlation of 0.3 or more is considered to be important. The larger the canonical correlation index is, the more amount of variance shared the linear combination of variables. The eigenvalues are sorted in descending order of importance. So the first one always explains that majority of variance in the relationship. Bravais Pearson's (r) [50] product-moment correlation was utilized to explore the relationships between the personality factors, collected by the 5-FasT and STAI, and the order parameters within the different communication networks. Similarly, the correlations between the personality traits and the opinion dynamics (Table 2) were considered. A linear regression method in order to define the best linear predicting model of the individual's opinion shifts were also considered. In order to characterize the participants who did not change their opinions during the virtual interaction, their differences compared to the other experimental participants using the independent sample student-t test [51] were analyzed.
The investigation stemmed from the assumption that certain local dynamics happen among individuals, and that their subjective factors (e.g., personality) affect their mental representations of the social environment, their adopted communicative behavior, as well as their opinion dynamics. Therefore, the correlations among the 5-FasT FasT personality factors and the order parameters related to the communicative dimensions shown in Table 3 were analyzed. Such a procedure was adopted in order to assess the “local” variables that demonstrated a relevant impact on the communication dynamics.
The scores of personality factors respectively, related to the surgency scale (5-FasT Su) and to the conscientiousness scale (5-FasT Co), show significant correlations with several communicative observables (Table 4). The 5-FasT Su predicts the participants that produced several messages in community or in private chat. Furthermore, such personality factors correlate with the probability of sending positive messages within the community. The 5-FasT Co positively correlates with number of messages sent in the community sphere. As expected, the 5-Fast Ne shows a positive correlation with the STAI scoring. Besides this, such personality factors are negatively correlated with a centrality degree and a betweenness degree in the community positive messages network (). During the first 30′ min of interaction, the participants with a high score in neuroticismin neuroticism appear to be less involved in positive discussions within the community. The other two personality factors (i.e., 5-FasT Ag and 5-FasT Cl) did not show any significant correlation with the communicative variables, except for the correlation between the 5-FasT Cl and the betweenness degree in the public radar.
Table 4. Significant correlations between the 5-FasT personality factors and the communicative observables.
Examining the correlations between the 5-FasT factors and the opinion's related variables, no 5-FasT factors show a significant correlation with any opinion's dimension collected during the experiments (i.e., final opinion shifts, final decision). Such findings suggest the existence of no trivial relationships between the structure of the personality and what emerges from the opinion dynamics, nevertheless, such apparent independence seems to be related to the dynamics of the communicative topology. Likewise, the local dynamics focusing on the dyadic relationships as well as on the emerging opinion dynamics were examined. A discriminant function analysis to mine the best model in order to distinguish the coherent from the incoherent dyads was applied. A coherent dyad was defined as a couple of participants sharing the same absolute opinion.
The discriminant function reached a canonical correlation of 0.76 and a relative reliability equal to 96.9%. The analysis produced the first linear approximation of a model characterized by seven parameters, as shown in Table 5. The probability of observing a coherent dyad decreased depending on the number of neutral private messages in the first 15′, on the distance in the private radar in the first 15′, on the difference in age, and on the difference in the 5-FasT Su and 5-FasT Ag. On the contrary, such probability increased with the difference in 5-FasT Cl as well as with the difference in the shift in opinion. Only two observables, related to the dynamics among participants, appear relevant in order to discriminate a coherent dyad. In short, the best model takes into account three different traits of personality, the difference of age between participants, the amount of the opinion changing, the private neutral messages and the affinity representations in the early stages (i.e., 15 min) of interaction. Consequently taking the parameters in Table 5, into account, it appeared to be possible to forecast whether two individuals would share the same final opinion or not within a dyad in a small group engaged in a virtual discussion.
Then, our attention was directed to the global dynamics shown by each experiment. In order to explore the dynamics of the average opinion within every experimental session, the opinion centroid of each small group was defined as the average opinion of their members. As it can be observed in Figure 1, the average opinion trends of the different experiments show peculiar evolutions. The opinion centroids follow different pathways, showing a certain consistency between the initial average value of opinion and the final one, passing through a series of fluctuations due to the interactions among the participants, and their shifts in opinion.
Figure 1. Time evolution of the average opinion within the 5 small group experiments. On the X axis are reported the time steps in which the opinions were collected (i.e., OpIn, Op15′, Op25′, Op35′, Op45′, OpFi), while on the Y axis is reported the average opinion for each experiment, from adverse (0) to prone (100). In order to facilitate the lecture of the slope and the trend of the trajectories, in the Y axis we took as extremes values 25 for the adverse and 75 for the prone. The colored lines indicate the different experimental sessions.
Upon further exploration of the opinion dynamics, a discriminant function in order to highlight what parameters would allow those participants that move away from their initial opinion to discrimate were applied. The discriminant function is described by the parameters in Table 6, and shows a canonical correlation of the model of 0.8 and a relative reliability of 88%.
In fact, 50% (25 subjects) of the entire sample had not changed opinion after 45′ of interaction. As shown in Table 6, only the variables related to the groups interaction are involved in the discriminant function. Very interestingly, the parameters related to subjective variables, such as personality factors, anxiety, their opinions or their values/beliefs (i.e., trust in science, empathy for animals) are not involved in such a function. This finding seems to identify the emergence of a group phenomenon due to its independence by the subjective dimensions. If a person changes or not, albeit slightly, his final opinion based only on his dynamics of communication can be determined. The best model considers the frequency of the interactions (i.e., activity in public negative messages), the importance of the individuals within the structure of the communication detected (i.e., betweenness parameters) and the topology of the network revealed by the radars (i.e., centrality in private radar, betweenness in public radar).
By only considering the 25 individuals who changed their opinion, a linear regression model to explore the relationships between the amount of the opinion shift of a subject with all the other observables collected was designed. In order to select the best model, and considering the limited size of the experimental sample, we adopted the corrected Akaike Information Criterion (AICc), to estimate the normalized relative likelihood values for all the significant models resulting from the linear regression [52]. First we computed the AICc for all the models using the Equation (4):
with n equal to the number of observations (i.e., 25), k reporting the number of parameters of the model considering the constant and the error coefficients of the equation, and RSS indicating the residuals sum of squares of the model:
To compare the models we first estimated the relative distance between the models' AICc (Equation 5), and then we calculated the normalized relative likelihood of the models (wi) by means of Equation (6):
with r indicating the number of models we obtained from the multiple regression. The results of the model comparison are reported in Table 7, considering only the significant model extracted by the standard multiple linear regeression analysis. The wi can be interpreted as the probability that i is the best model, given the data and set of candidate models [52].
The best linear regression model appears to explain the 79% of variance of the data (Table 8) and involved five parameters (Equation 7, Table 9). Four parameters refer to the variables detectable through the participants interaction (i.e., the centrality degree in the community positive messages network at the end of experiment, the activity of the participants in the private neutral messages network at the end of discussion, the centrality degree in the community messages network, and in the public radar within the first 15′ min of interaction). Only one personality factor, the 5-FasT Co (β2), is included in the best regression model:
All of the coefficients of the parameters involved in the model are negative. The negative sign of the communicative parameters indicates that a greater active participation to the discussion is related to a minor change in opinion. The more a person tended to send and receive messages (e.g., how much more one is involved in the discussion), the smaller his shift in opinion was. It is also interesting to note that the parameters involved affect all areas of communication (i.e., community and private chat) for the entire duration of the interaction. Such a finding identified an opinion dynamics affected by both the dyadic communications (i.e., private messages), as well as by the social side of the communication (i.e., community messages).
The behavior of the participants who did not change their opinion is deserves also attention. These individuals have been defined as “Stubborn people.” By comparing the stubborn people with the participants who changed their opinion (Table 10), it was discovered that the stubbornness effect is related to a favorable initial opinion to scientific research. The stubborn individuals posted far fewer negative messages within the community, they modified their position on the public radar in the first 15′ less and they used their private radar in the first 15′ less. The stubbornness effect is also related to a lower degree of betweenness in the community negative messages network in the first 15′, and to a higher degree of betweenness in the public radar in the first 15′. No significant differences between stubborn people and participants who changed their opinion were found as far as age, gender, personality structure, anxiety, final absolute opinion, empathy with animals and the trust in science is concerned. This latter discovery may suggest a crucial role of the communication network topology in the process of opinion negotiation of the individuals in our experimental condition (i.e., within a group size of 10 people).
The results of our work indicate that the opinion dynamics produced by the interaction of a small group of individuals, engaged in a virtual discussion about a specific topic, is affected by different factors as suggested by the Lewin field theory. First of all, the data from the perspective of the participants, analysing the correlations among their personality factors and their communicative features were examined. Then, quite a rich characterizability of the personality of the participants in terms of adopted communicative behavior was observed. The neurocitism factor negatively correlates with the centrality degree, and the betweenness degree, in the community positive messages network exchanged during the first 30 min. The participants with a higher score on the personality traits related to melancholy, worry and dissatisfaction, appear to be less involved in the community positive discussions. Conversely, the surgency and the conscientiousness positively correlate with the average number of sent messages. The surgency, that indicates how much an individual is energetic, active and original, affects the centrality degree of a subject within the private communication space in a coherent way. The closeness, that describes how much a subject is distant, close, elusive, appears to affect the betweenness in the public radar, and consequently the position assumed by the participants in the affinity space.
Moreover, it is interesting to note that neither the personality factor, nor any individual variables such as age, or sex, correlates with the opinions dynamics detected (i.e., the trajectories of the participants on the opinion space). This suggests that there is no direct (or simple) relationship between the structure of personality and what was found in the opinion dynamics of the participants. The opinion dynamics within a local dynamics dimension (i.e., dyadic relationships) was explored, and a discriminant function was applied in order to distinguish the coherent dyads from the incoherent dyads. A couple of participants that had the same final vote in absolute terms as a coherent dyad were considered. The parameters of the resulting discriminant function involve the personality factors, some subjective variables and the communicative parameters. In particular, a coherent dyad can be found by observing the exchange of private neutral messages, the distances in the affinity space between two participants that compose the dyad in the early stage of the experiments, the differences in agreeableness and closeness between two participants in the surgency. Additionally, even some subjective variables, age difference, a type of openness to the opinion changing allow us to foresee whether a dyad shares the same final opinion or not. This result suggests that regarding the opinion dynamics within a dyadic relationship, the difference related to the personality structure between two participants, as well as their distance in affinity, appears to be decisive, while the communication variables appear not to be so relevant. Specifically, within the dyadic interaction, the subjective variables showed to have much relevance, and it seemed to prevail the effect of cognitive dissonance on the opinion dynamics with respect to the social pressure factors.
A discriminant function analysis was applied to distinguish the best set of variables describing the participants who changed their final opinions, and those who did not. It is very interesting to note that the resulting discriminant function only suggests that the parameters are related to the interactions within the group. No subjective parameter such as age, sex, personality, anxiety, attitudes toward animal experimentation, empathy for the animals or the trust in science influenced the identification of who would change their opinions during the interaction. In general the stubborn people appeared to be more favorable to animal experimentation, and less involved in negative mood conversation in the community, less central within the affinity space (i.e., private radar) and less oriented to modify their position in the public radar. The majority of the features found as defining the stubborn behavior refers to the first 15′ of interaction, therefore within the small group the stubborn individuals can be recognizable by considering the early stage of a virtual discussion. Furthermore, we brought to light 5 parameters involved in a linear regression model explaining the shift in opinion. Such a model explained 79% of variance of the data, and presented only one parameter related to the personality, while the other parameters depend on the dynamics of communication among the participants. Such a result suggests that it is possible to forecast the shift in opinion, once the individuals who have changed their opinion are identified, taking into account the amount of positive messages exchanged in the community sphere at the end of the session, the messages sent and received in the community, and the centrality degree in the public radar. Above all, the activity in private messages with neutral mood seemed to be related to the total shift in opinion, as well as the personality trait of conscientiousness, which is related to the precision and the meticulousness of the individuals.
In conclusion, the main insights of our work indicate that within the small groups engaged in a virtual interaction, and facing a structured discussion around a polarizing topic, part of the opinion dynamics is mediated, if not dominated, by the group structure and its evolution (i.e., topology of the “socio-psychological field”). The stubbornness effect appears to be related only to the topology of the network of communication and affinity, and demonstrates the complexity of forecasting small human dynamics (e.g., workgroup decision making, governance of commissions, etc.). In other words, the group seems to require or impose a certain “location” (role) to the individuals, regardless of their personal features.
The authors declare that the research was conducted in the absence of any commercial or financial relationships that could be construed as a potential conflict of interest.
This work was funded by the European Commission under the FET-AWARENESS RECOGNITION (257756). AG and FB acknowledges partial financial support from the EU projects 288021 (EINS – Network of Excellence in Internet Science) and 611299 (FP7 Programme on Collective-Awareness Platforms SciCafe2.0).
1. Castellano C, Fortunato S, Loreto V. Statistical physics of social dynamics. Rev Mod Phys. (2009) 81:591–646. doi: 10.1103/RevModPhys.81.591
2. Weisbuch G, Deffuant G, Amblard F, Nadal JP. Meet, discuss, and segregate. Complexity (2002) 3:55–63. doi: 10.1002/cplx.10031
4. Bagnoli F, Carletti T, Fanelli D, Guarino A, Guazzini A. Dynamical affinity in opinion dynamics modeling. Phys Rev E (2008) 76:66105. doi: 10.1103/PhysRevE.76.066105
5. Amblard F, Deffuant G. The role of network topology on extremism propagation with the relative agreement opinion dynamics. Physica A (2004) 343:725–38. doi: 10.1016/j.physa.2004.06.102
6. Galam S. Heterogeneous beliefs, segregation, and extremism in the making of public opinions. Phys Rev E (2005) 71:046123. doi: 10.1103/PhysRevE.71.046123
7. Galam S, Jacobs F. The role of inflexible minorities in the breaking of democratic opinion dynamics. Physica A (2007) 381:366–76. doi: 10.1016/j.physa.2007.03.034
9. Bowles S, Gintis H. Social capital and community governance. Econ J. (2002) 112:F419–36. doi: 10.1111/1468-0297.00077
10. Fine GA, Harrington B. Tiny publics: small groups and civil society. Sociol Theory (2004) 22:341–56. doi: 10.1111/j.0735-2751.2004.00223.x
11. Burkhalter S, Gastil J, Kelshaw T. A conceptual definition and theoretical model of public deliberation in small face to face groups. Commun Theory (2002) 12:398–422. doi: 10.1111/j.1468-2885.2002.tb00276.x
12. Ryfe DM. Narrative and deliberation in small group forums. J Appl Commun Res. (2006) 34:72–93. doi: 10.1080/00909880500420226
13. Fraser SW, Greenhalgh T. Complexity science: coping with complexity: educating for capability. Br Med J (2001) 323:799. doi: 10.1136/bmj.323.7316.799
14. Beebe SA, Masterson JT. Communication in Small Groups: Principles and Practice. Boston, MA: Allyn and Bacon (2009).
15. Springer L, Stanne ME, Donovan SS. Effects of small-group learning on undergraduates in science, mathematics, engineering, and technology: a meta-analysis. Rev Educ Res. (1999) 69:21–51. doi: 10.3102/00346543069001021
16. Kerr NL, Tindale RS. Group performance and decision making. Annu Rev Psychol. (2004) 55:623–55. doi: 10.1146/annurev.psych.55.090902.142009
17. Van Rooy DA. Connectionist Abm of social categorization processes. Adv Complex Syst. (2012) 15:1250077–98. doi: 10.1142/S0219525912500774
18. Gastil J, Levine P. The Deliberative Democracy Handbook: Strategies for Civic Engagement in the 21st Century. San Francisco, CA: Jossey Bass (2005).
19. Macintosh AEA. Technology to Support Participatory Democracy. Hershey, PA: Idea Group Publishing (2002).
20. Jaeger PT. Deliberative democracy and the conceptual foundations of electronic government. Gov Infor Q (2005) 22:702–19. doi: 10.1016/j.giq.2006.01.012
21. Arrow H, McGrath JE, Berdahl JL. Small Groups as Complex Systems: Formation, Coordination, Development, and Adaptation. Thousand Oaks, CA: APA; Sage (2000).
22. Bavelas A. Communication in task-oriented groups. J Acoust Soc Am. (1950) 22:271282. doi: 10.1121/1.1906679
23. Luce RD. Connectivity and generalized cliques in sociometric group structure. Psychometrika (1950) 15:169–90. doi: 10.1007/BF02289199
24. Shaw ME. Communication networks. Adv Exp Soc Psychol. (1964) 1:111147. doi: 10.1016/S0065-2601(08)60050-7
25. Leavitt HJ. Some effects of communication patterns on group performance. J Abnorm Soc Psychol. (1950) 46:38–50. doi: 10.1037/h0057189
26. Isenber DJ. Group polarization: a critical review and meta-analysis. J Pers Soc Psychol. (1986) 50:1141. doi: 10.1037/0022-3514.50.6.1141
27. Tajfel H. Social identity and intergroup behaviour. Soc Sci Inform. (1974) 13:65–93. doi: 10.1177/053901847401300204
28. Asch SE. Studies of independence and conformity: I. A minority of one against a unanimous majority. Psychol Monogr Gen Appl. (1956) 9:1–70.
29. Sherif M, Hovland CI. Social Judgment: Assimilation and Contrast Effects in Communication and Attitude Change. New Haven, CT: Yale University Press (1961).
30. Festinger L. A theory of social comparison processes. Hum Relat. (1954) 7:117–40. doi: 10.1177/001872675400700202
33. Festinger L. A Theory of Cognitive Dissonance. Redwood City, CA: Stanford University Press (1962).
34. Galam S, Moscovici S. Towards a theory of collective phenomena: consensus and attitude changes in groups. Eur J Soc Psychol. (1991) 21:49–74. doi: 10.1002/ejsp.2420210105
35. Turner JC, Oakes PJ, Haslam SA, McGarty C. Self and collective: cognition and social context. Pers Soc Psychol Bull. (1994) 20:454–4. doi: 10.1177/0146167294205002
37. Luhtanen R, Crocker J. A collective self-esteem scale: self-evaluation of one's social identity. Pers Soc Psychol Bull. (1992) 18:302–18. doi: 10.1177/0146167292183006
38. Galam S. Public debates driven by incomplete scientific data: the cases of evolution theory, global warming and H1N1 pandemic influenza. Physica A (2010) 389:3619–31. doi: 10.1016/j.physa.2010.04.039
39. Galam S. Collective beliefs versus individual inflexibility: the unavoidable biases of a public debate. Physica A (2011) 390:3036–54. doi: 10.1016/j.physa.2011.03.021
40. Dunbar RIM, Duncan NDC, Nettle D. Size and structure of freely forming conversational groups. Hum Nat. (1995) 6:67–78. doi: 10.1007/BF02734136
41. Guazzini AEA. Cognitive network dynamics in chatlines. Procedia Comput Sci. (2010) 1:2355–62. doi: 10.1016/j.procs.2010.04.265
42. Guazzini A, Bagnoli F, Carletti T, Vilone D, Lauro Grotto R. Cognitive network structure: an experimental study. Adv Complex Sci. (2012) 15:12584–99. doi: 10.1142/S0219525912500841
43. Cini A, Guazzini A. Human virtual communities: affinity and communication dynamics. Adv Complex Sci. (2013) 16:1350034-1–24. doi: 10.1142/S0219525913500343
44. Giannini M, Pannocchia L, Grotto LP, Gori A. A measure for counseling: the five factor adjective short test (5-fast). Couns G Ital Ric Appl. (2012) 3:384.
46. Freeman LC. Centrality in social networks conceptual clarification. Soc Netw. (1979) 1:215–39. doi: 10.1016/0378-8733(78)90021-7
47. Freeman LC, Borgatti SP, White DR. Centrality in valued graphs: a measure of betweenness based on network flow. Soc Netw. (1991) 13:141–54. doi: 10.1016/0378-8733(91)90017-N
48. Cohen J, Cohen P. Applied multiple regression/correlation analysis for the behavioral sciences. Mahwah, NJ: Lawrence Erlbaum (1975).
50. Rodgers JL, Nicewander WA. Thirteen ways to look at the correlation coefficient. Am Stat. (1988) 42:59–66. doi: 10.2307/2685263
51. Box GE, Hunter JS, Hunter WG. Statistics for Experimenters: Design, Innovation, and Discovery. Hoboken, NJ: John Wiley & Sons, Inc. (2005).
Keywords: small group dynamics, cyber psychology, opinion dynamics, stubborness effect, web based human interaction
Citation: Guazzini A, Cini A, Bagnoli F and Ramasco JJ (2015) Opinion dynamics within a virtual small group: the stubbornness effect. Front. Phys. 3:65. doi: 10.3389/fphy.2015.00065
Received: 16 February 2015; Accepted: 11 August 2015;
Published: 01 September 2015.
Edited by:
Serge Galam, Centre National de la Recherche Scientifique, FranceReviewed by:
Salvatore Micciche', Università degli Studi di Palermo, ItalyCopyright © 2015 Guazzini, Cini, Bagnoli and Ramasco. This is an open-access article distributed under the terms of the Creative Commons Attribution License (CC BY). The use, distribution or reproduction in other forums is permitted, provided the original author(s) or licensor are credited and that the original publication in this journal is cited, in accordance with accepted academic practice. No use, distribution or reproduction is permitted which does not comply with these terms.
*Correspondence: Andrea Guazzini, Department of Psychology, Center for the Study of Complex Dynamics, University of Florence, Via di San Salvi 12, 50135 Firenze, Italy,YW5kcmVhLmd1YXp6aW5pQHVuaWZpLml0
Disclaimer: All claims expressed in this article are solely those of the authors and do not necessarily represent those of their affiliated organizations, or those of the publisher, the editors and the reviewers. Any product that may be evaluated in this article or claim that may be made by its manufacturer is not guaranteed or endorsed by the publisher.
Research integrity at Frontiers
Learn more about the work of our research integrity team to safeguard the quality of each article we publish.