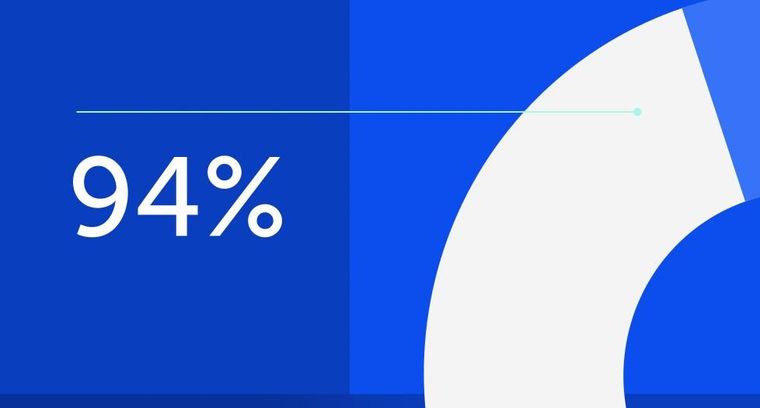
94% of researchers rate our articles as excellent or good
Learn more about the work of our research integrity team to safeguard the quality of each article we publish.
Find out more
ORIGINAL RESEARCH article
Front. Pharmacol., 16 April 2025
Sec. Experimental Pharmacology and Drug Discovery
Volume 16 - 2025 | https://doi.org/10.3389/fphar.2025.1519145
This article is part of the Research TopicInsights in Experimental Pharmacology and Drug Discovery: 2024View all 10 articles
Neurodegenerative disorders (NDs) are typically characterized by progressive loss of neuronal function and the deposition of misfolded proteins in the brain and peripheral organs. They are molecularly classified based on the specific proteins involved, underscoring the critical role of protein-processing systems in their pathogenesis. Alpha-synuclein (α-syn) is a neural protein that is crucial in initiating and progressing various NDs by directly or indirectly regulating other ND-associated proteins. Therefore, reducing the α-syn aggregation can be an excellent option for combating ND initiation and progression. This study presents an in silico phytochemical-based approach for discovering novel neuroprotective agents from bioactive compounds of the Lamiaceae family, highlighting the potential of computational methods such as functional networking, pathway enrichment analysis, molecular docking, and simulation in therapeutic discovery. Functional network and enrichment pathway analysis established the direct or indirect involvement of α-syn in various NDs. Furthermore, molecular docking interaction and simulation studies were conducted to screen 85 major bioactive compounds of the Lamiaceae family against the α-syn aggregation. The results showed that five compounds (α-copaene, γ-eudesmol, carnosol, cedryl acetate, and spathulenol) had a high binding affinity towards α-syn with potential inhibitory activity towards its aggregation. MD simulations validated the stability of the molecular interactions determined by molecular docking. In addition, in silico pharmacokinetic analysis underscores their potential as promising drug candidates, demonstrating excellent blood-brain barrier (BBB) permeability, bioactivity, and reduced toxicity. In summary, this study identifies the most suitable compounds for targeting the α-syn aggregation and recommends these compounds as potential therapeutic agents against various NDs, pending further in vitro and in vivo validation.
Neurodegenerative disorders (NDs), incurable and debilitating diseases of the central and peripheral nervous system (CNS and PNS), are the worldwide leading cause of death and genesis of millions as per disability-adjusted life years (DALYs). NDs are associated with a range of clinical manifestations, including depression, dementia, bradykinesia, and ataxia (Williams-Gray and Worth, 2016). The report on the Global burden of disease (GBD) suggested the number of people affected globally by several NDs such as Alzheimer’s disease (AD), Parkinson’s disease (PD), Huntington’s disease (HD), epilepsy, multiple sclerosis, and others, will rise from 6.2 million to 13 million by 2040 (Feigin et al., 2017). According to the World Health Organisation (WHO), NDs are expected to overtake cancer by 2040 to become the second leading cause of mortality worldwide with the highest prevalence rates and pose a significant financial and health burden globally (Chopade et al., 2023; Dua and Neurology, 2004).
There is neither cure nor prevention available for ND patients; however, NDs can be treated with a variety of synthetic medications such as monoamine oxidase inhibitors, dopamine agonists, acetylcholinesterase inhibitors, adenosine receptor antagonists, L-DOPA replacement therapy, and others (Ellis and Fell, 2017). Considering the epidemiological predictions of NDs and their shortcomings, investigations into natural alternatives with effective and potent preventive actions against acute neurological deterioration are necessary while being well tolerated and free from adverse side effects to patients (Sharifi-Rad et al., 2022). The oxidative stress is a major hallmark of NDs resulting from dysregulated reactive oxygen species (ROS) production. Imbalances in cellular antioxidant defences, coupled with mitochondrial dysfunction, lipid peroxidation, neuroinflammation, and altered dopamine metabolism, contribute to disease pathology. Research focuses on identifying molecules that can modulate ROS pathways and exploring natural polyphenolic phytochemicals as potential therapeutic agents to overcome current treatment limitations (Abd Rashed et al., 2021). Aromatic plants produce essential oils with antiviral, antibacterial, antifungal, and neuroprotective properties. Many studies reported that phytochemicals play a crucial role in maintaining the brain’s chemical balance by modulating the receptor function of specific inhibitory neurotransmitters (Guo et al., 2015; Kolouri et al., 2016; Yadav, 2021). The Lamiaceae family, encompassing over 7,000 species and 236 medicinally significant genera, represents a vast reservoir of bioactive secondary metabolites, including alkaloids, terpenoids, and polyphenols, underpinning its pharmacological significance (Wink, 2020). Among its members, genus Ajuga are particularly noteworthy for their diverse phytochemical profile, comprising iridoids (e.g., harpagide), neo-clerodane diterpenes, and phytoecdysteroids, which contribute to their antimicrobial, anti-inflammatory, and neuroprotective effects (Mimaki et al., 2021). Furthermore, Lamiaceae-derived polyphenols, such as rosmarinic and salvianolic acids, demonstrate acetylcholinesterase inhibition, highlighting their therapeutic relevance in NDs, particularly in AD, while also exhibiting potential in metabolic regulation, including glucose homeostasis in diabetes (Zengin et al., 2018). Collectively, these bioactive constituents position Lamiaceae species as promising candidates for the development of novel interventions in NDs and metabolic disorders (Gupta et al., 2024; Hernandez-Leon et al., 2021). For instance, extracts from Salvia Rosmarinus Spenn., Salvia officinalis L., Otostegia persica (Burm.) Boiss., Ocimum menthiifolium Hochst. ex Benth., and Nepeta menthoides Boiss. & Buhse have been found to exhibit antidepressant and anxiety-reducing properties (Firoozabadi et al., 2015; Kolouri et al., 2016; Mank-Halati et al., 2024; Nematolahi et al., 2018; Sadeghi et al., 2014; Zahran et al., 2021). Proteins like amyloid β-, τ-protein, α-synuclein, and TDP-43 are the most frequently aggregated (misfolded) proteins culpable for NDs. Also, over the years, studies suggested that α-synuclein (α-syn), which is a heat-stable, soluble, and abundant presynaptic brain protein with 140 amino acids encoded by synuclein or SNCA gene, plays a salient role in neurotransmitter release and vesicular trafficking. However, the formation of insoluble forms of α-syn and its inclusion in Lewy bodies is associated with various NDs. It is also directly or indirectly interconnected with various proteins associated with NDs like Parkinson’s disease (PD) and other synucleinopathies (Horne et al., 2024). It disrupts cellular membranes, interferes with protein degradation pathways like the ubiquitin-proteasome system and autophagy, and activates inflammatory processes (Goedert, 2001). This leads to cellular homeostasis disruption and neuronal death, affecting synaptic function. Additionally, secreted α-syn can harm neighboring cells by promoting aggregation, potentially contributing to disease propagation (Shim et al., 2022; Stefanis, 2012). It can also upregulate or downregulate their expression to facilitate different NDs initiation and progression (Davidson et al., 1998; Ghiglieri et al., 2018; Uversky, 2017). Therefore, it can be a potential targeted biomarker for developing precision therapies against NDs (Brundin et al., 2017).
Fortunately, various preclinical reports described phytochemicals belonging to the Lamiaceae family like rosmarinic acid, curcumin, morin, etc., have been reported to specifically target α-syn, which efficiently scavenges oxygen free radicals via different pathways (Hui et al., 2018; Meena et al., 2021), thereby, protecting cells from oxidative damage, confirming the neurogenic potential which prevents loss of neuronal processes (Naoi et al., 2017). Phytochemical-based drug discovery leveraging molecular docking and ADMET analysis presents a robust and cost-effective strategy for identifying novel therapeutic agents. This method facilitates high-throughput screening of natural compounds, detailed insights into molecular interactions, and elucidation of unknown molecular structures, including enzymes and their potential ligands. In silico methods for optimizing the structure of biotherapeutic agents have been reported in several studies, demonstrating the potential of docking and ADMET analysis in novel therapeutic development (Asati et al., 2020; Saha et al., 2023).
Numerous studies have investigated the clinical relationships between proteins implicated in neurodegenerative diseases and phytochemicals (Guo et al., 2015; Lopez-Lopez et al., 2004). However, a unified target linking all neurodegenerative diseases and elucidating their molecular interactions with phytochemical-based bioactive compounds has not yet been identified. Comprehensive research substantiating the association between phytochemicals and neurodegenerative diseases with solid scientific validation is needed to fill this knowledge gap. Therefore, this study aims to elucidate the role of α-synuclein (α-syn) in various neurodegenerative disease pathways, highlighting its potential as a drug development target. Furthermore, it investigates the therapeutic potential of bioactive compounds found in the essential oils of Lamiaceae plants against α-syn aggregation and neurodegenerative disease progression. The research encompasses functional network analysis, pathway enrichment analysis of α-syn, molecular interaction studies with these bioactive compounds, and pharmacokinetic evaluations to assess their viability as promising lead candidates in drug design (Figure 1).
The functional and physical interconnections between α-syn and other proteins involved in neurodegenerative diseases were confirmed using the STRING version 9.1 database (https://string-db.org) (Franceschini et al., 2013), which identifies physical and functional associations between proteins by analyzing co-expressed genes, literature, high-throughput experimental data, and database mining. The neurodegenerative disease-related genes were identified using DisGeNET (https://www.disgenet.org/, accessed on 12 June 2024). For this study, α-syn was queried in the STRING database with Homo sapiens selected as the organism. An interaction score threshold of 0.7 (indicating high confidence) was set. Additionally, cluster analysis was conducted using the K-means clustering algorithm, specifying eight clusters.
The involvement of α-syn interactors in various biological pathways was analyzed using the Enrichr database (https://maayanlab.cloud/Enrichr/enrich#). Enrichr enables users to investigate the roles of query proteins in pathways, ontology, diseases/drugs, and expression across different cell lines. The list of primary interactors of α-syn was submitted as a query to the Enrichr server. Their roles in different pathways were analyzed using the KEGG 2021 Human database, which includes manually curated data on human cell signaling and metabolic pathways (2021 version). A bar graph depicting the top 10 pathways, ranked by p-value, was downloaded from Enrichr. Additionally, a CSV file containing the table format of all pathways associated with α-syn and its interactors was retrieved.
The crystal structure of α-syn (3Q26) implicated in neurodegenerative diseases was obtained from the RCSB PDB (accessed on 12 March 2024) and prepared for docking studies by removing unnecessary chains and residues using UCSF Chimera 1.16. For ligand preparation, 3D SDF structures of 85 major phytochemical compounds belonging to the Lamiaceae family were identified according to previous studies (Ebadollahi et al., 2020; Ramos da Silva et al., 2021) and downloaded from PubChem (accessed on 12 March 2024). These compounds were imported into Avogadro 1.2.0 and subjected to energy minimization using the MMFF94 force field with the steepest descent algorithm. The minimized structures were then converted to PDBQT format for docking.
AutoDock Tools 1.5.7 (Scripps Research Institute, La Jolla, CA, United States) was utilized to predict protein-ligand interactions. Water molecules were removed, and polar hydrogens and Kollman charges were added to the protein structures. Both protein and ligand files were converted to PDBQT format. Active sites were determined using blind-docking and enclosed within a 3D affinity grid centred on the active site residues. Docking was executed via command prompt following the method described previously (Gupta et al., 2023). Binding energies were recorded, and initial visualizations were performed with BIOVIA Discovery Studio Visualizer (BIOVIA, San Diego, CA, United States). The follow-up detailed analysis of amino acid and ligand interaction was also performed using the same. Phytochemical compounds from the Lamiaceae family exhibiting optimal binding affinities to α-synuclein were selected for blood-brain barrier (BBB) permeation analysis. The top five compounds demonstrating both high BBB permeability and superior binding affinity were then chosen for further molecular dynamics simulations and drug-likeness analysis. Additionally, to cross-verify the binding affinities of the ligands with proteins, docking was conducted using the CB-Dock2 online server (https://cadd.labshare.cn/cb-dock2/index.php, accessed on 12 March 2024).
Drug-likeness, bioactivity analysis, and toxicity prediction are crucial for identifying novel drug candidates. Parameters for Lipinski’s rule of five including molecular weight (MW), hydrogen bond acceptors and donors (HBD/HBA), octanol/water partition coefficient (LogP), topological polar surface area (TPSA), bioavailability, and BBB permeability were assessed using SwissADME (http://www.swissadme.ch/, accessed on 14 March 2024). Additionally, the BBB scores of all compounds were confirmed using the Molsoft web server (https://www.molsoft.com/mprop/, accessed on 14 March 2024). The bioactivity scores of the ligands were obtained from the Molinspiration web server (https://www.molinspiration.com/cgi/properties, accessed on 14 March 2024). Candidate molecules were predicted using in silico methods via the pkCSM online tool (https://biosig.lab.uq.edu.au/pkcsm/prediction, accessed on 15 March 2024), evaluating parameters such as AMES toxicity, maximum tolerated dose (human), hERG I and hERG II inhibitory effects, oral rat acute toxicity, hepatotoxicity, skin sensitization, and fathead minnow toxicity.
The five best protein-ligand complexes from the molecular docking study were selected for MD simulation based on the binding energy and optimal docked pose. The macromolecular structure of α-synuclein (3Q26) comprised multiple subunits, which were segregated, and their additional homodomains were removed using Discovery Studio Visualizer v20.1.0.19195 (https://www.3ds.com/products/biovia/discovery-studio). Adjacent heteroatoms were also removed similarly. The modified structures were then further refined to become compatible with in silico virtual screening and molecular dynamics simulation (MDS). A comparison map of the dynamic characteristics of our target proteins and their protein-ligand complexes was generated using GROMACS 2020.4.1, following the methodology described before (Bhattacharya et al., 2024a; Bhattacharya et al., 2024b; Kandasamy et al., 2022). The Protein and protein-ligand complexes were extracted as GROMACS files for 200 ns (nanoseconds) in steps of 2 femtoseconds (fs) from CHRMM-GUI files.
After the successful completion of MD simulation of specific protein-ligand interaction complexes, the following analyses were performed: root mean square deviation (RMSD), solvent accessible surface area (SASA), radius of gyration (Rg), hydrogen bond (HB) and root mean square fluctuations (RMSF). These analyses were performed during the entire 200 ns MD trajectory at intervals of 20 ns. Finally, using Qtgrace (https://qtgrace.sourceforge.io/), all the graphs (HB, Rg, SASA, RMSD, and RMSF) were plotted together.
The recalculating binding free energy of protein-ligand interactions was further assessed using MD simulation-specific binding free energy analysis using the MM-PBSA (Molecular Mechanics Poisson-Boltzmann Surface Area) method. The following equation was used by the GROMACS function “g_mmpbsa” to collect MD trajectory data of protein-ligand interaction complexes at 5-ns intervals.
Where ΔGBA indicates average free energy, ΔEMM indicates average molecular mechanics energy, ΔGPBSA indicates solvation energy, and TΔSMM denotes solute configuration entropy respectively (Kandasamy et al., 2022).
The role of α-syn in various pathways of neurodegenerative diseases was elucidated through functional network analysis. 1,515 genes related to multiple neurodegenerative diseases were used in this study. The interconnection between α-syn (also its encoding gene SNCA) and its 76 interactor proteins are displayed in Figure 2 and Supplementary Table S1. Further, the functional network of α-syn was categorised into distinct 8 clusters to simplify the analyses of their connection in different pathways. The cluster illustrates numerous associations of pathway-related genes with α-syn (Figure 3).
Cluster 1 comprised 44 proteins, including those involved in the Ubiquitin-Proteasome System (UPS), such as F-box protein 7 (FBXO7), Neural precursor cell expressed developmentally downregulated protein 4 (NEDD4), Parkinsonism-associated deglycase (PARK7), PTEN-induced putative kinase 1 (PINK1), Parkin RBR E3 ubiquitin-protein ligase (PRKN), Sequestosome 1 (SQSTM1), Ubiquitin B (UBB), Ubiquitin C (UBC), Ubiquitin C-terminal hydrolase L1 (UCHL1), and Ubiquitin specific peptidase 9, X-linked (USP9X) (Celebi et al., 2020; Han et al., 2023; Huang et al., 2020; Ristic et al., 2014). Additionally, proteins such as Amyloid beta precursor protein (APP), Huntingtin (HTT), Microtubule-associated protein tau (MAPT), Prion protein (PRNP), TAR DNA binding protein (TARDBP), and Transthyretin (TTR) are critical in neurodegeneration and protein aggregation (Gandhi et al., 2019; Goedert, 2015; Wang et al., 2024). Proteins vital for synaptic functions and neurotransmission include Vesicular monoamine transporter 1 (SLC18A1), Vesicular monoamine transporter 2 (SLC18A2), Dopamine transporter (SLC6A3), Synuclein alpha interacting protein (SNCAIP), and Voltage-dependent anion channel 1 (VDAC1) (Courtin et al., 2006; Ning et al., 2023; Shoval et al., 2014). The proteins BCL2 like 1 (BCL2L1), Clusterin (CLU), Monoamine oxidase A (MAO-A), Monoamine oxidase B (MAO-B), and Superoxide dismutase 1 (SOD1) are involved in cell survival and apoptosis (Dabrowska et al., 2011; Suthar and Lee, 2023). For protein folding and stress response, Cathepsin D (CTSD), Gelsolin (GSN), HtrA serine peptidase 2 (HTRA2), and Heat1 shock protein family A member 9 (HSPA9) are key players. In contrast, Leucine-rich repeat kinase 2 (LRRK2) and Fyn proto-oncogene, Src family tyrosine kinase (FYN) are significant for kinase activity (Cai et al., 2022; Garrido et al., 2022; Jin and Youle, 2013). Essential proteins regulating transcriptional and translational processes include the Proteasome 26S subunit, ATPase1 (PSMC1), and GRB10 interacting GYF protein 2 (GIGYF2) (Sathitloetsakun and Heiman, 2024; Yang et al., 2021). Lastly, proteins involved in cellular transport and trafficking, cytoskeletal dynamics, and mitochondrial functions are represented by Vacuolar protein sorting 35 (VPS35), Tubulin polymerization promoting protein (TPPP), and ATPase cation transporting 13A2 (ATP13A2), respectively (Kaur et al., 2021).
Cluster 2 in the functional network analysis included proteins such as ABL proto-oncogene 1, non-receptor tyrosine kinase (ABL1), and epidermal growth factor receptor (EGFR), which are involved in signal transduction and kinase activity (Colicelli, 2010). Neurotrophic factors identified in this cluster include brain-derived neurotrophic factor (BDNF) (Zuccato and Cattaneo, 2009). The calmodulin family, comprising calmodulin 3 (CALM3), calmodulin-like 3 (CALML3), calmodulin-like 4 (CALML4), calmodulin-like 5 (CALML5), and calmodulin-like 6 (CALML6), is associated with calcium signaling (Wang et al., 2019). Proteins involved in metabolism and energy production include glyceraldehyde-3-phosphate dehydrogenase (GAPDH), insulin (INS), and islet amyloid polypeptide (IAPP) (Abedini et al., 2018). Toll-like receptor 2 (TLR2) and toll-like receptor 4 (TLR4) play major roles in the immune response (Zacharowski et al., 2006). Finally, proteins involved in critical functions such as epigenetic regulation, protein degradation, the ubiquitin-proteasome system, nucleic acid binding and processing, synaptic function, and neurotransmission include histone deacetylase 6 (HDAC6), STIP1 homology and U-box containing protein 1 (STUB1), nucleolin (NCL), and neurogranin (NRGN) (Hwang et al., 2021; Seidel et al., 2015; Zhang et al., 2021).
Cluster 3 consisted of two proteins, SNAP25 (Synaptosome Associated Protein 25) and SYN1 (Synapsin I), which are involved in synaptic function and neurotransmitter release (Margiotta, 2021). Clusters 4, 5, 6, 7, and 8 are each associated with a single protein: SLC6A2 (Solute Carrier Family 6 Member 2), SLC1A2 (Solute Carrier Family 1 Member 2), NR4A2 (Nuclear Receptor Subfamily 4 Group A Member 2), SNCB (Beta-Synuclein), and RAB1A (Ras-related protein Rab-1A), respectively. These proteins are crucial for various roles, such as neurotransmitter transport, regulation of gene expression, synaptic function, neurodegenerative disorders, and intracellular vesicle trafficking and secretion (McCoy et al., 2015; Goldenring, 2013; Hu et al., 2020).
In addition to the functional network analysis with different clusters, pathway enrichment analysis of α-syn and its interactors was conducted using the Enrichr database. The top 10 pathways involving α-syn and its interactors are highlighted in Figure 4, with p-values and adjusted p-values provided in Supplementary Table S2. α-syn interactors predominantly play significant roles in pathways related to neurodegeneration, PD, lipid and atherosclerosis, amphetamine addiction, alcoholism, dopaminergic synapses, estrogen signaling pathway, AD, amyotrophic lateral sclerosis, and the neurotrophin signaling pathway.
Figure 4. Pathway enrichment analysis of α-syn interactors substantiates α-syn’s involvement in neurodegenerative signaling and metabolic pathways.
To identify the potential α-syn inhibitor, molecular docking was performed with 85 phytochemical compounds (Supplementary Table S3). These selected compounds have previously reported therapeutic potential such as antioxidant, antimicrobial, and anti-inflammatory activity (Nieto, 2017; Ramos da Silva et al., 2021). The binding energies of all selected compounds ranged from −4.2 kcal/mol to −11.4 kcal/mol. Among 85 compounds docked, the top 5 compounds were selected based on the top hit criteria of binding energies and BBB permeability and then further analysed for molecular interactions with α-syn (Figure 5; Table 1). The top hit compounds are α-copaene, carnosol, cedryl acetate, γ-eudesmol, spathulenol. Carnosol demonstrated a strong binding affinity for α-syn, with a binding energy of −9.4 kcal/mol. It formed a hydrogen bond of 4.82 Å with the A chain of Glu45 and exhibited other interactions with the A chains of Ala64, Trp63, Trp231, and Tyr156 (Figures 5I,J). Spathulenol showed −9.1 kcal/mol binding energy against α-syn (Figures 5E,F). No hydrogen bond was found to be involved in the interaction, but other interactions, such as unfavorable Donor-Donor interactions and two 2 groups of hydrophobic interactions (Alkyl and Pi Alkyl) with A chains of Ala64, Pro155, Trp63, Trp341, and Tyr156 were found to be involved. γ-eudesmol showed a high binding affinity towards α-syn with a binding energy of −8.9 kcal/mol. The interaction prediction study showed 1 group of electrostatic and 2 groups of hydrophobic interactions (Pi-Sigma, Alkyl, and Pi-Alkyl) with the A chains amino acids Ala64, Trp63, Trp231, Trp341, and Tyr156 (Figures 5C,D). Cedryl acetate and α-copaene showed a similar binding energy of −8.6 kcal/mol against α-syn. The molecular level interaction prediction study showed a hydrogen bond of length 5.16 Ǻ with the A chain residue Asn151 along with 2 groups of hydrophobic interactions (Alkyl and Pi-Alkyl) with Ala64, Phe157, Trp63, Trp231, and Tyr156 for cedryl acetate (Figures 5A,B). For α-copaene 1 group of electrostatic (Pi-Sigma) and 2 groups of hydrophobic interactions (Alkyl and Pi Alkyl) with the A chains amino acid residues Ala64, Trp63, Trp231, Trp341, and Tyr156 were found to be involved in the interactions (Figures 5G,H).
Figure 5. 3D and 2D interactions of best docked ligands with α-syn. (A) and (B) cedryl acetate, (C) and (D) γ-eudesmol, (E) and (F) spathulenol, (G) and (H) α-copaene, (I) and (J) carnosol.
Compounds’ BBB permeability was determined to understand their ability to penetrate the BBB to be available to the CNS. The BBB is a highly selective permeability barrier that maintains cerebral homeostasis by regulating the transport of nutrients and solutes into the CNS. This barrier presents a significant challenge for the delivery of therapeutics targeting CNS diseases, necessitating that CNS drugs possess the capability to traverse this dynamic and protective membrane (Upadhyay, 2014). The boiled egg diagram, plotting the TPSA against the partition coefficient (cLogP), can predict a molecule’s ability to cross the BBB and its potential for human intestinal absorption (HIA). The egg yolk represents the ability to traverse the BBB, while the egg white (albumin) signifies HIA. Our results suggested that all five compounds can penetrate the BBB to reach the CNS (Figure 6). Further, the BBB score from the molSoft web server confirmed the BBB permeability of all compounds, where a score of 6 indicates the highest permeability and 0 is the lowest (Table 2). Our results indicated that cedryl acetate had the highest BBB permeability, with a score of 4.53, followed by γ-eudesmol (4.39), spathulenol (4.39), and carnosol (3.84). In contrast, α-copaene showed borderline permeability with a score of 1.26, corresponding to our boiled egg diagram results.
Figure 6. BBB permeability analysis using a boiled egg diagram for all top hit compounds. (A)- α-copaene, (B)- γ-eudesmol, (C)- carnosol, (D)-cedryl acetate, (E)- spathulenol.
The drug-likeness of compounds is based on Lipinski’s rule of five (Ro5: HBDs ≤5, HBA≤ 10, MW < 500, LogP ≤5, and no more than one violation), which comprises key molecular properties that affect a drug’s pharmacokinetics in the human body, including absorption, distribution, metabolism, and excretion (ADME) components (Lipinski et al., 1997).
Our results indicated that out of five compounds, α-copaene violates one of Lipinski’s rules as they have a slightly higher logP value greater than 4.15. As a rule, it does not predict pharmacological activity. However, the previously established anti-inflammatory and antioxidant activities should not be overlooked (Acharya et al., 2021; Nascimento et al., 2018; Poeckel et al., 2008; Ramos da Silva et al., 2021). Except for α-copaene, the other four compounds fulfill Lipinski’s Ro5, indicating their potential as active drug candidates (Table 2). Calculating the topological polar surface area (TPSA) provides insights into a drug’s bioavailability and hydrogen bonding potential. All of our compounds have a TPSA range from 0.00 to 66.76 Å (Table 2), lower than the typical upper limit of 160 Å (Abdelrheem et al., 2021). Furthermore, our results indicated an ideal bioavailability score of 0.55 for all tested compounds, suggesting good absorption in the human body (Martin, 2005).
Bioactivity scores for drug compounds can be evaluated based on parameters including kinase inhibition (KI), protease inhibition (PI), enzyme inhibition (EI), binding affinity to G protein-coupled receptors (GPCRs) and nuclear receptors (NRL), as well as ion channel modulation (ICM). A molecule with bioactivity scores above 0.00 is likely to exhibit significant biological activity; scores ranging from −0.50 to 0.00 are considered moderately active, while those below −0.50 are presumed to be inactive (Abdelrheem et al., 2021). All the bioactivity results were tabulated in Table 3. Our results indicated that α-copaene is highly active towards ICM, NRL, and EI and moderately active towards GPCRs and PI. Carnosol is predicted as highly active towards GPCRs, ICM, NRL, and EI and showed moderate activity for KI and PI. Cedryl acetate showed higher activity for GPCRs, ICM, NRL, and EI and moderate activity for PI but remained inactive towards KI. Similarly, γ-Eudesmol showed inactivity towards KI but was highly active towards ICM, NRL, and EI and moderately active for GPCRs and PI. Spathulenol exhibited high activity for NRL and EI and moderately active for GPCRs, ICM, and PI but inactive towards KI.
The toxicity of the drug compounds was assessed using AMES test results, human maximum tolerated dose, oral rat acute toxicity, hepatotoxicity, skin sensitization, minnow toxicity, and human ether-a-go-go gene (hERG) inhibition (Table 4). The AMES results indicated that all tested compounds are non-mutagenic and non-carcinogenic. The Maximum Recommended Tolerance Dose (MRTD) estimates human toxic doses, which are considered low if below log 0.477 (mg/kg/day) (Saha et al., 2021). Hence, the results obtained indicate that all tested compounds are less toxic to humans. The results also showed that none of our tested compounds are non-hERG inhibitors. Cedryl acetate, γ-eudesmol, and spathulenol showed skin sensitivity; however, no hepatotoxic prediction was established for all compounds. According to a report, a higher oral rat-acute toxicity (LD50) indicates less lethality compared to one with a lower LD50 value (Sinha et al., 2022). Our results indicate higher LD50 values ranging from 1.644 to 2.192, indicating less lethality for these compounds. However, if a molecule’s log LC50 (concentration causing 50% fathead minnow mortality) is below 0.5 mM (log LC50 < −0.3), it is considered highly toxic (Sinha et al., 2022). Our findings suggest that all the tested compounds except carnosol are less toxic, with significantly higher scores than the mentioned LC50 threshold. These results suggest that these five phytochemical compounds are less toxic to humans and can be potential drug candidates for treating CNS disorders.
Following a consecutive 200 ns molecular dynamics (MD) simulation, post-MD analysis was conducted to investigate the characteristic trajectories of the reference protein-only system and the respective protein-ligand complexes. This analysis involved the examination of various trajectory characteristics, including root mean square deviation (RMSD), root mean square fluctuation (RMSF), radius of gyration (Rg), solvent accessible surface area (SASA), hydrogen bond (HB) occupancy, and pair distance. These metrics were utilized to evaluate protein-ligand interactions and assess the protein’s stability and folding behavior. The superimposition of ligands on the targeted proteins was shown in Figure 7 to observe the time-dependent protein-drug interaction.
Figure 7. Various image panels extracted from PyMOL™ 2.5.8 illustrate the superimposition of the selected drugs. (A) carnosol (B) cedryl acetate (C) spathulenol (D) α-copaene (E) γ-eudesmol upon the targeted protein (PDB entry 3Q26) in the time-dependent manner of 20 ns interval throughout 200 ns to observe the concomitant protein-drug interactions.
Figure 8A presents the superimposition of all RMSD plots, providing an approximate overview of the significant overlap in the RMSD trajectories of the reference protein-only system and the protein-ligand complexes. This indicates minimal deflection in backbone stability following ligand association. The RMSD, which reflects backbone stability, corroborates the altered protein folding behavior, as validated by the respective Rg trajectories (García and Onuchic, 2003). The concordant fluctuations observed in the Rg and RMSD plots further describe the folding behaviors of the respective systems.
Figure 8. (A) RMSD (root mean square deviation) plot of protein backbone fluctuations, (B) comparative analysis plot of Rg (radius of gyration) fluctuations, (C) RMSF (root mean square fluctuation) plot of residual fluctuations, (D) comparative analysis plot of SASA (solvent accessible surface area) profiles of protein-only system and protein-docked complexes depicted in specific colour codes.
Individual RMSD plots for all systems show minimal differences in fluctuations, ranging from approximately 0.1–0.55 nm, as depicted in Figure 8. As illustrated in Figure 8, the reference protein-only system (3Q26) and other protein-ligand complexes exhibited erratic RMS deviations up to approximately 20 ns, indicating initial backbone instability. This was followed by stabilization, suggesting a rapid folding process toward the native and thermodynamically favorable conformation (García and Onuchic, 2003; Maruyama et al., 2023). Protein folding is primarily influenced by the interplay of solvation and nonpolar-solvation (de-solvation) effects (García and Onuchic, 2003). Thus, it can be hypothesized that the initial 20 ns interval of erratic RMSD fluctuation observed across all RMSD trajectories is predominantly driven by the solvation effect mediated by TIP3P water molecules in the MD environment. This solvation effect is common to all RMSD profiles. In contrast, the RMSD fluctuations observed in the latter intervals for protein-ligand systems are critically influenced by the de-solvation effect due to ligand interactions.
The radius of gyration (Rg), which signifies molecular and structural compactness, can be calculated as:
Where N is the total number of atoms, rcom is the position vector at the centre of mass and ri is the position vector at the ith atom of respective protein macromolecules (Bronowska, 2011). Rg is a fundamental metric for quantifying the spatial distribution of atoms around the centre of mass, thereby evaluating structural integrity and compactness. As depicted in Figure 8B, the Rg trajectories showed higher fluctuations up to approximately 20 ns for both the protein-only and protein-docked systems, likely due to the solvation effect, similar to the RMSD fluctuations.
As time progressed, the 3Q26-γ-eudesmol complex maintained lower Rg fluctuations around 2.16 nm, while the 3Q26 and 3Q26-spathulenol systems showed higher Rg fluctuations around 2.23 nm. This suggests that the 3Q26-γ-eudesmol complex exhibited more stable protein folding, achieving the native, thermodynamically favored conformation more effectively than the 3Q26 and 3Q26-spathulenol systems.
For specific protein-ligand complexes, such as 3Q26-spathulenol and 3Q26-α-copaene, intermittent unsteady Rg fluctuations were observed during the intervals of approximately 60–83 ns and 90–126 ns. These fluctuations illustrate that the protein tends to optimize its feasible native conformation due to successive ligand accommodations, resulting in slight adjustments to structural integrity as reflected in the Rg trajectories.
The root mean square fluctuation (RMSF) describes the fluctuation of amino acid residues in both protein-only and protein-ligand systems, as depicted in Figure 8C. RMSF is calculated based on the Cα atom fluctuations around their average positions, both in the presence and absence of ligand moieties. Residues with higher fluctuations indicate greater flexibility, while those with lower fluctuations suggest increased rigidity, influenced by weakening or strengthening ligand interactions, respectively (Chaudhary and Aparoy, 2017).
As shown in Figure 10, the protein-only system exhibits significantly higher residue flexibilities in the regions spanning the 165–185th and 334–375th residues, indicative of increased structural instability compared to the protein-ligand systems. This instability is further corroborated by the reduced compactness observed in the protein-only system, confirmed by the less stable radius of the gyration (Rg) trajectory (Ghahremanian et al., 2022). In contrast, the protein-ligand systems demonstrate enhanced stability and structural integrity in their Rg trajectories, supporting the consistency in RMSF residual rigidity throughout the MD simulation.
Among all ligand-docked systems, the 3Q26-spathulenol complex exhibits comparatively higher residue flexibility, particularly in the residues spanning 50–57. This suggests reduced hydrogen bond (HB) formation and the lowest electrostatic energy decomposition, as shown in Figure 9. Other protein-ligand complexes, including 3Q26-carnosol, 3Q26-cedryl acetate, 3Q26-α-copaene, and 3Q26-γ-eudesmol, display significantly constrained residue fluctuations, ranging from approximately 0.06–0.38 nm. This underscores their augmented electrostatic interactions and evident HB occupancy, as indicated in Figure 9.
Figure 9. Illustration of hydrogen bond (HB) occupancy contributed by the interaction between protein and respective docked ligands depicted in specific color codes.
Solvent accessible surface area (SASA) is a critical metric for assessing hydrophobic interactions, often overestimating the effects when modeled explicitly, particularly with encapsulated solvation domains and hydrophobic non-polar molecules (Eisenhaber, 1999). The solvation model rendered by TIP3P water molecules and the nonpolar solvation effect exerted by docked ligand moieties significantly impact protein folding and resultant energy dissipation (Ausaf Ali et al., 2014). A gradual decrease in SASA trajectory indicates slow, stable protein folding dynamics influenced by stronger hydrophobic forces, whereas an increase in SASA values suggests rapid, unstable folding driven by weaker hydrophobic interactions (Duan et al., 2013).
The SASA trajectory plot in Figure 8D reveals that the protein-only system (3Q26) and the protein-docked system with spathulenol exhibit slightly higher SASA values (∼183 nm2). In contrast, other protein-ligand complexes (3Q26-carnosol, 3Q26-cedryl acetate, 3Q26-γ-eudesmol, and 3Q26-α-copaene) show a significant decrease in average SASA values (∼173 nm2). These SASA trajectories highlight distinct protein folding behaviors for the 3Q26 protein with various ligands. Specifically, the 3Q26 and 3Q26-spathulenol systems exhibit rapid and unstable folding patterns, indicative of reduced hydrophobic interactions. Conversely, the 3Q26 complexes with carnosol, cedryl acetate, γ-eudesmol, and α-copaene demonstrate more stable and slower folding dynamics, influenced by stronger hydrophobic interactions, as reflected by reduced SASA fluctuations.
As illustrated in Figure 9, the hydrogen bond (HB) occupancy plot for the 3Q26-docked complexes is dominated by carnosol, followed by γ-eudesmol, cedryl acetate, α-copaene, and spathulenol. This trend aligns with the decreasing order of electrostatic energy dissipation (kJ/mol) shown in Table 5.
Table 5. MM-PBSA (Molecular Mechanics Poisson-Boltzmann Surface Area) based approximate binding free energy (KJ/mol) calculation of top 5 selected small molecules against the reference macromolecule alpha-synuclein chimeric protein (PDB entry 3Q26).
Carnosol exhibited regular intervals of HB formation, starting at the 10th ns with the formation of 1 HB, followed by 2, 3, and 4 HBs at the 20th, 34th, and 47th ns, respectively. The HB formation then decreased, with 3 and 2 HBs observed up to the 169th and 183rd ns, respectively. Similarly, γ-eudesmol showed consistent HB occupancy, starting with 1 and 2 HBs at the 10th and 103rd ns, respectively, and maintaining 1 HB up to the 200th ns.
In contrast, cedryl acetate, α-copaene, and spathulenol displayed intermittent HB formation at specific intervals, as depicted in Figure 9. The pair distance analysis highlights the most stabilized interaction in the 3Q26-γ-eudesmol complex, as shown in panel F of Figure 10, where the proximity of protein 3Q26 and ligand γ-eudesmol is maintained throughout the MD interval of 20–200 ns. Following γ-eudesmol, carnosol maintained proximity during the MD intervals of 25–100 ns and 110–185 ns. Cedryl acetate maintained close pair distance at the MD interval of 50–100 ns. α-Copaene remained within the binding pockets of 3Q26 during the consecutive MD intervals of 5–50 ns, 55–90 ns, and 120–200 ns, constructing a successive interaction dynamic.
Figure 10. Pair distance (PD) analysis between five differential pairs of protein 3Q26 with specific docked ligands – Carnosol, cedryl acetate, spathulenol, α-copaene, and γ-eudesmol represented in respective panels by 5 separate color codes.
In contrast, spathulenol showed intermittent dynamics in maintaining proximity and stable fitting within the binding pocket of 3Q26, supporting its least occupancy in hydrogen bond formation and consequent minimal dissipation of electrostatic energy, as indicated in Table 5.
Binding free energy decomposition was evaluated based on the MM-PBSA method. The total binding free energy (ΔGBA) is the resultant combination of four segregated forms of energy: Van der Waals energy (kJ/mol), electrostatic energy (kJ/mol), polar solvation energy (kJ/mol), and SASA energy (kJ/mol).
Van der Waals energy represents weak interaction energy, primarily arising from permanent and transient hydrophobic, non-polar interaction forces (π−σ, π−alkyl, and π−π). In the MD environment, the de-solvation effect primarily contributes to the rise of van der Waals and electrostatic energy dissipation (Mahmoud et al., 2020; Rasaiah et al., 2008). Therefore, van der Waals forces are directly anti-correlated with polar solvation effects, which are effectively mediated by TIP3P water molecules (Naïm et al., 2007). In Table 5, van der Waals energy is highlighted as a major contributor to the ΔGBA decomposition, except in the case of spathulenol interaction where the ΔGBA is notably influenced by unfavorable polar solvation energy (∼−22.024 kJ/mol). This observation underscores a direct anti-correlation between polar solvation energy and van der Waals energy. Among the five different small molecule drugs studied, γ-eudesmol exhibits the highest dissipation in van der Waals energy (∼−90.186 kJ/mol), leading to the highest ΔGBA decomposition (∼−71.067 kJ/mol) observed among all interactions.
Similarly, the compounds carnosol, cedryl acetate, and α-copaene show substantial van der Waals energy dissipation (approx. −57.564 kJ/mol, approx. −20.145 kJ/mol, approx. −58.857 kJ/mol, respectively), contributing significantly to their respective ΔGBA values. These interactions illustrate how non-polar van der Waals interactions mitigate the polar solvation effect and promote de-solvation effects, thereby enhancing electrostatic interactions to a notable extent. De-solvation or non-polar solvation plays a crucial role in modulating electrostatic energy flow and subsequent HB formation (Dill et al., 2002). Therefore, the intensity of hydrogen bond (HB) formation (Figure 9) corresponds to the order of electrostatic energy dissipation observed in the interactions between 3Q26 and carnosol, cedryl acetate, spathulenol, α-copaene, and γ-eudesmol. The interaction of 3Q26 with carnosol, which dissipates the highest electrostatic energy (approx. −11.084 kJ/mol), exhibits the highest occupancy in HB formation. This is followed by γ-eudesmol (electrostatic energy approx. −3.361 kJ/mol), cedryl acetate (electrostatic energy approx. −2.345 kJ/mol), α-copaene (electrostatic energy approx. −0.730 kJ/mol), and spathulenol (electrostatic energy approx. −0.376 kJ/mol).
Polar solvation energy, regulated by the aqueous nature of the MD environment, significantly influences the interactions of drug molecules with macromolecules. Positive (+ve) polar solvation energy dissipation is favorable for enhancing electrostatic, hydrophobic, and van der Waals interactions, thereby improving the binding affinity of protein-ligand interactions. Conversely, negative (-ve) polar solvation energy dissipation hinders significant protein-ligand interactions and stable protein folding dynamics.
Spathulenol, which exhibits the highest negative polar solvation energy dissipation (approx. −22.024 kJ/mol) among the selected drugs, also shows the lowest dissipation of electrostatic and SASA (solvent accessible surface area) energy (approx.−1.373 kJ/mol). This correlates with its least occupancy in HB formation and the most unstable pair distance (Figure 10) throughout the 200 ns MD simulation interval.
The present study investigated the role of the neuronal protein α-syn in neurodegenerative diseases using functional networking and pathway enrichment analysis. Additionally, it evaluated the neuroprotective potential of Lamiaceae family bioactive compounds against α-syn aggregation through molecular docking and simulation approach. The functional network and enrichment analysis demonstrated the association of α-syn with various proteins involved in NDs, which α-syn may directly or indirectly regulate. Additionally, α-syn was found to be involved in several neurodegenerative signaling pathways such as neurodegeneration, PD, lipid and atherosclerosis, amphetamine addiction, alcoholism, dopaminergic synapses, estrogen signaling pathway, AD, amyotrophic lateral sclerosis, and the neurotrophin signaling pathway, underscoring its impact on the initiation and progression of various NDs. It is well known that α-syn is associated with PD and is a potential molecular drug target for its therapeutic solutions (Horne et al., 2024). However, our findings suggest that α-syn is not only a potential target for PD but also a viable target for other ND therapeutics.
As α-syn is a potential target molecule for NDs, in this study we found five compounds such as α-copaene, carnosol, cedryl acetate, γ-eudesmol, and spathulenol that exhibited strong binding interactions with α-syn. Strong interactions of these five selected phytochemical compounds with α-syn indicate their potency as probable inhibitors. However, only a limited number of α-syn inhibitors have been identified to date, such as Anle138b, NPT200-11, SynuClean-D, ZPD-2, and CLR01 (De Luca et al., 2022; Fields et al., 2019). Consequently, there is a critical need for further development of α-syn inhibitors. Our findings suggest that these phytochemicals could serve as effective agents to prevent α-syn aggregation, enhance α-syn degradation, inhibit α-syn expression, or disrupt α-syn interactions with other proteins implicated in NDs. Moreover, α-copaene, carnosol, cedryl acetate, γ-eudesmol, and spathulenol have been identified as potent antioxidants and anti-inflammatory agents (Acharya et al., 2021; Nascimento et al., 2018; Poeckel et al., 2008; Ramos da Silva et al., 2021) that attenuate cellular oxidative stress and neural inflammation associated with various NDs, thereby acting as potential neuroprotective agents. These results underscore the potential of these compounds as promising candidates for α-syn-targeted drug development.
The BBB’s protective nature makes it challenging for drug molecules to cross, posing a major hurdle for CNS drug candidates that should be addressed early in drug discovery. Drugs targeting peripheral organs should also be checked for BBB permeability to prevent potential CNS side effects (Alavijeh et al., 2005). Therefore, our results elucidated the BBB permeation ability of these five phytochemical compounds, which can be further utilised as potential CNS drugs to combat various NDs. Depending on the docking analysis, further pharmacokinetic study was performed to analyze these top-hit compounds as potent drugs. The present investigation demonstrated that these compounds have excellent CNS bioavailability, lower toxicity levels, and significant biological activities.
Moreover, MD simulation analysis elucidated the interaction stability of these five compounds with α-syn. A precise examination of backbone stability during the MD interval of approximately 30–200 ns reveals that notable RMS deviations in the protein-ligand systems (PDB entry 3Q26 complexed with cedryl acetate, spathulenol, and α-Copaene) align closely with the RMSD trajectory of the protein-only system (3Q26 without ligand). This indicates that the aqueous environment-driven solvation effect mitigates the de-solvation effect, as the respective ligand interactions play a minor role. This is evidenced by lower hydrogen bond occupancy (Figure 9) and electrostatic energy decomposition. Therefore, this hypothesis supports the notion that the increased backbone fluctuations (RMSD ∼0.4 nm) in the 3Q26 complexes with cedryl acetate, spathulenol, and α-Copaene lead to faster and less stable protein folding.
Conversely, the dynamics of 3Q26 interactions with carnosol and γ-eudesmol demonstrate comparatively stable backbone fluctuations, indicating a slower, more stable protein folding behavior. This stability is due to the significant influence of ligand interactions and the prevailing solvation effect, where ligand-mediated de-solvation and electrostatic interactions dominate over the solvation effect, as observed in the hydrogen bond occupancy plot. Similarly, A stable Rg trajectory indicates a well-packed protein conformation, with minimal fluctuations suggesting retention of the native fold. In contrast, an unfolded protein shows a higher, unsteady Rg. Rg and RMSD plots often correlate, offering insights into how structural compactness and backbone flexibility affect protein-ligand interactions (Kim et al., 2015; Rosilan et al., 2024; Yamamoto et al., 2021). The Rg values of proteins and protein-ligand complexes reflect the compactness and structural integrity of proteins in the presence of the tested compounds. Simultaneously, comprehensive SASA analysis aligns with RMSD and Rg results, validating the observed protein folding dynamics. The SASA data also delineate the simultaneous interplay of solvation and de-solvation effects in stabilizing protein-ligand interactions, providing a holistic view of SASA and the protein-folding pathways. The HB formation between protein-ligand indicates short-range electrostatic interactions occurring through intra- or intermolecular covalent bonds between hydrogen and electronegative atom(s). Intermolecular HB formation strengthens the stabilized ligand-protein interaction, resulting in significant electrostatic energy dissipation, as detailed in Table 5 of the binding free energy decomposition (Choudhary et al., 2023). In protein-ligand interaction dynamics, the pair distance defines the specific MD intervals during which the ligand stays within the protein’s binding pocket. Pair distance also illustrates the role of interacting forces in driving protein-ligand interactions, as shown in the HB occupancy plot in Figure 9 (Kandasamy et al., 2022). At the same time, SASA energy, influenced by the hydrophobicity and amphiphilicity of the ligands, plays a crucial role in determining the effective binding affinity and protein folding dynamics of protein-ligand complexes (Eisenberg and McLachlan, 1986). Table 5 shows that the van der Waals, electrostatic, and SASA energy of different protein-ligand complexes exhibit a consistent increasing or decreasing order. This correlation underscores the intricate interplay between ligand-mediated and de-solvation effects in SASA energy decomposition and subsequent protein folding dynamics. Among all five interactions studied, the γ-eudesmol and spathulenol interactions with 3Q26 show maximum (approx. −11.055 kJ/mol) and minimum (approx.−1.373 kJ/mol) SASA energy flows, respectively. This validates their highest and lowest dominance in HB formation (Figure 9) and the most stable and unstable maintenance of pair distances (Figure 10, respectively). This pattern also supports the corresponding order of electrostatic energy dissipation in these interactions. Ultimately, it can be postulated that the backbone stability, hydrophobic/hydrophilic surface nature of proteins, and subsequent altered protein folding dynamics of native proteins are significantly influenced by ligand interactions. These interactions dictate the subsequent de-solvation and solvation effects, thereby affecting the substantial dynamics of ΔGBA decomposition. Therefore, ΔGBA decomposition alone may not always indicate strong protein-ligand interactions, as the dissipation of van der Waals energy, SASA energy, and electrostatic energy can vary, reflecting the complex mechanisms of protein-ligand interactions challenged by the inherent solvation model of the MD environment.
Moreover, these findings suggest that α-copaene, γ-eudesmol, carnosol, cedryl acetate, and spathulenol have the potential to counteract α-syn as an alternative neuroprotective solution. However, phytochemical-based drug discovery using molecular docking and ADMET analysis offers a cost-effective approach to identify novel therapeutic compounds. However, in silico predictions are limited by structural data and may not accurately capture pharmacokinetics or toxicity. Based on the data presented, the compounds investigated in this study could be considered for further in vitro, in vivo experimental validation, and clinical studies for the potential treatment of NDs.
The relevant information on docking for all tested compounds as discussed in the main text is provided in a separate archive at https://zenodo.org/records/13192341. Software used in this manuscript are third-party software and packages; versions are described in detail in the Materials and Methods section. All the data are provided in the supplementary file and above mentioned link. Further inquiries can be directed to the corresponding author(s).
SB: Conceptualization, Data curation, Formal Analysis, Investigation, Methodology, Visualization, Writing – original draft, Writing – review and editing. NG: Formal Analysis, Visualization, Writing – review and editing. AD: Formal Analysis, Methodology, Visualization, Writing – review and editing. PK: Formal Analysis, Methodology, Visualization, Writing – review and editing. RD: Formal Analysis, Visualization, Writing – review and editing. JZ: Funding acquisition, Supervision, Writing – review and editing. NT: Project administration, Supervision, Writing – review and editing. LS: Funding acquisition, Writing – review and editing. LK: Funding acquisition, Writing – review and editing. LM: Formal Analysis, Supervision, Writing – review and editing. EF-C: Formal Analysis, Supervision, Writing – review and editing.
The author(s) declare that financial support was received for the research and/or publication of this article. This research was funded by the Faculty of Economics and Management, Czech University of Life Sciences in Prague, grant number 2022B0004, Internal Grant Agency, grant number 20223101, Faculty of Tropical AgriSciences, Czech University of Life Sciences in Prague and KEGA 001SPU-4/2025 Internationalization of teaching texts of genetic and molecular safety subjects in the context of activating education.
The authors declare that the research was conducted in the absence of any commercial or financial relationships that could be construed as a potential conflict of interest.
The author(s) declare that no Gen AI was used in the creation of this manuscript.
All claims expressed in this article are solely those of the authors and do not necessarily represent those of their affiliated organizations, or those of the publisher, the editors and the reviewers. Any product that may be evaluated in this article, or claim that may be made by its manufacturer, is not guaranteed or endorsed by the publisher.
The Supplementary Material for this article can be found online at: https://www.frontiersin.org/articles/10.3389/fphar.2025.1519145/full#supplementary-material
NDs, Neurodegenerative Disorders; CNS, Central Nervous System; PNS, Peripheral Nervous System; DALYs, Disability Adjusted Life Years; AD, Alzheimer’s Disease; PD, Parkinson’s Disease; HD, Huntington’s Disease; ADMET, Absorption, Distribution, Metabolism, Excretion, Toxicity; α-syn, α-synuclein; ROS, Reactive Oxygen Species.
Abdelrheem, D. A., Rahman, A. A., Elsayed, K. N. M., Abd El-Mageed, H. R., Mohamed, H. S., and Ahmed, S. A. (2021). Isolation, characterization, in vitro anticancer activity, dft calculations, molecular docking, bioactivity score, drug-likeness and admet studies of eight phytoconstituents from brown alga sargassum platycarpum. J. Mol. Struct. 1225, 129245. doi:10.1016/j.molstruc.2020.129245
Abd Rashed, A., Abd Rahman, A. Z., and Rathi, D. N. G. (2021). Essential oils as a potential neuroprotective Remedy for Age-related neurodegenerative diseases: a review. Molecules 26, 1107. doi:10.3390/molecules26041107
Abedini, A., Cao, P., Plesner, A., Zhang, J., He, M., Derk, J., et al. (2018). RAGE binds preamyloid IAPP intermediates and mediates pancreatic β cell proteotoxicity. J. Clin. Invest. 128, 682–698. doi:10.1172/JCI85210
Acharya, B., Chaijaroenkul, W., and Na-Bangchang, K. (2021). Therapeutic potential and pharmacological activities of β-eudesmol. Chem. Biol. Drug Des. 97, 984–996. doi:10.1111/cbdd.13823
Alavijeh, M. S., Chishty, M., Qaiser, M. Z., and Palmer, A. M. (2005). Drug metabolism and pharmacokinetics, the blood-brain barrier, and central nervous system drug discovery. Neurotherapeutics 2, 554–571. doi:10.1602/neurorx.2.4.554
Asati, V., Agarwal, S., Mishra, M., Das, R., and Kashaw, S. K. (2020). Structural prediction of novel pyrazolo-pyrimidine derivatives against PIM-1 kinase: in-silico drug design studies. J. Mol. Struct. 1217, 128375. doi:10.1016/j.molstruc.2020.128375
Ausaf Ali, S., Imtaiyaz Hassan, Md., Islam, A., and Ahmad, F. (2014). A review of methods available to estimate solvent-accessible surface areas of soluble proteins in the folded and unfolded States. Curr. Protein Pept. Sci. 15, 456–476. doi:10.2174/1389203715666140327114232
Bhattacharya, S., Dutta, A., Khanra, P. K., Gupta, N., Dutta, R., Tzvetkov, N. T., et al. (2024a). In silico exploration of 4(α-l-rhamnosyloxy)-benzyl isothiocyanate: a promising phytochemical-based drug discovery approach for combating multi-drug resistant Staphylococcus aureus. Comput. Biol. Med. 179, 108907. doi:10.1016/j.compbiomed.2024.108907
Bhattacharya, S., Khanra, P. K., Dutta, A., Gupta, N., Aliakbar Tehrani, Z., Severová, L., et al. (2024b). Computational screening of T-Muurolol for an alternative antibacterial solution against Staphylococcus aureus Infections: an in silico approach for phytochemical-based drug discovery. Int. J. Mol. Sci. 25, 9650. doi:10.3390/ijms25179650
Bronowska, A. K. (2011). “Thermodynamics of ligand-protein interactions: implications for molecular design,” in Thermodynamics - interaction studies - Solids, Liquids and Gases (London, United Kingdom: IntechOpen). doi:10.5772/19447
Brundin, P., Dave, K. D., and Kordower, J. H. (2017). Therapeutic approaches to target alpha-synuclein pathology. Exp. Neurol. 298, 225–235. doi:10.1016/j.expneurol.2017.10.003
Cai, Z., Hu, W., Wu, R., Zheng, S., and Wu, K. (2022). Bioinformatic analyses of hydroxylated polybrominated diphenyl ethers toxicities on impairment of adrenocortical secretory function. Environ. health Prev. Med. 27, 38. doi:10.1265/ehpm.22-00023
Celebi, G., Kesim, H., Ozer, E., and Kutlu, O. (2020). The effect of dysfunctional ubiquitin enzymes in the pathogenesis of most common diseases. Int. J. Mol. Sci. 21, 6335. doi:10.3390/ijms21176335
Chaudhary, N., and Aparoy, P. (2017). Deciphering the mechanism behind the varied binding activities of COXIBs through Molecular Dynamic Simulations, MM-PBSA binding energy calculations and per-residue energy decomposition studies. J. Biomol. Struct. Dyn. 35, 868–882. doi:10.1080/07391102.2016.1165736
Chopade, P., Chopade, N., Zhao, Z., Mitragotri, S., Liao, R., and Chandran Suja, V. (2023). Alzheimer’s and Parkinson’s disease therapies in the clinic. Bioeng. Transl. Med. 8, e10367. doi:10.1002/btm2.10367
Choudhary, S., Kesavan, A. K., Juneja, V., and Thakur, S. (2023). Molecular modeling, simulation and docking of Rv1250 protein from Mycobacterium tuberculosis. Front. Bioinform 3, 1125479. doi:10.3389/fbinf.2023.1125479
Colicelli, J. (2010). ABL tyrosine kinases: Evolution of function, regulation, and Specificity. Sci. Signal. 3, re6. doi:10.1126/scisignal.3139re6
Courtin, C., Crete, D., Canestrelli, C., Noble, F., and Marie-Claire, C. (2006). Regulation of genes involved in dopamine transporter modulation by acute cocaine in rat striatum. Neurosci. Lett. 398, 235–240. doi:10.1016/j.neulet.2006.01.001
Dabrowska, M., Skoneczny, M., and Rode, W. (2011). Functional gene expression profile underlying methotrexate-induced senescence in human colon cancer cells. Tumor Biol. 32, 965–976. doi:10.1007/s13277-011-0198-x
Davidson, W. S., Jonas, A., Clayton, D. F., and George, J. M. (1998). Stabilization of α-synuclein secondary structure upon binding to synthetic membranes. J. Biol. Chem. 273, 9443–9449. doi:10.1074/jbc.273.16.9443
De Luca, L., Vittorio, S., Peña-Díaz, S., Pitasi, G., Fornt-Suñé, M., Bucolo, F., et al. (2022). Ligand-based discovery of a small molecule as inhibitor of α-synuclein amyloid formation. Int. J. Mol. Sci. 23, 14844. doi:10.3390/ijms232314844
Dill, K. A. (2002). Dominant forces in protein folding. ACS Publ. 29, 7133–7155. doi:10.1021/bi00483a001
Nascimento, K. F., Moreira, F. M. F., Alencar Santos, J., Kassuya, C. A. L., Croda, J. H. R., Cardoso, C. A. L., et al. (2018). Antioxidant, anti-inflammatory, antiproliferative and antimycobacterial activities of the essential oil of Psidium guineense Sw. and spathulenol. J. Ethnopharmacol. 210, 351–358. doi:10.1016/j.jep.2017.08.030
Dua, T., and Neurology, W. F. (2004). Atlas: Country Resources for neurological disorders 2004: results of a Collaborative study of the World health organization and the World Federation of Neurology. Geneva, Switzerland: World Health Organization.
Duan, L. L., Zhu, T., Mei, Y., Zhang, Q. G., Tang, B., and Zhang, J. Z. H. (2013). An implementation of hydrophobic force in implicit solvent molecular dynamics simulation for packed proteins. J. Mol. Model 19, 2605–2612. doi:10.1007/s00894-013-1798-8
Ebadollahi, A., Ziaee, M., and Palla, F. (2020). Essential oils extracted from different species of the Lamiaceae plant family as Prospective Bioagents against several Detrimental Pests. Molecules 25, 1556. doi:10.3390/molecules25071556
Eisenberg, D., and McLachlan, A. D. (1986). Solvation energy in protein folding and binding. Nature 319, 199–203. doi:10.1038/319199a0
Eisenhaber, F. (1999). Hydrophobic regions on protein surfaces. Perspect. Drug Discov. Des. 17, 27–42. doi:10.1023/A:1008766422140
Ellis, J. M., and Fell, M. J. (2017). Current approaches to the treatment of Parkinson’s Disease. Bioorg. Med. Chem. Lett. 27, 4247–4255. doi:10.1016/j.bmcl.2017.07.075
Feigin, V. L., Abajobir, A. A., Abate, K. H., Abd-Allah, F., Abdulle, A. M., Abera, S. F., et al. (2017). Global, regional, and national burden of neurological disorders during 1990–2015: a systematic analysis for the Global Burden of Disease Study 2015. Lancet Neurol. 16, 877–897. doi:10.1016/S1474-4422(17)30299-5
Fields, C. R., Bengoa-Vergniory, N., and Wade-Martins, R. (2019). Targeting alpha-synuclein as a therapy for Parkinson’s disease. Front. Mol. Neurosci. 12, 299. doi:10.3389/fnmol.2019.00299
Firoozabadi, A., Zarshenas, M. M., Salehi, A., Jahanbin, S., and Mohagheghzadeh, A. (2015). Effectiveness of Cuscuta planiflora ten. And Nepeta menthoides Boiss. & Buhse in major depression: a Triple-blind randomized controlled trial study. J. Evid. Based Complement. Altern. Med. 20, 94–97. doi:10.1177/2156587214557359
Franceschini, A., Szklarczyk, D., Frankild, S., Kuhn, M., Simonovic, M., Roth, A., et al. (2013). STRING v9.1: protein-protein interaction networks, with increased coverage and integration. Nucleic Acids Res. 41, D808–D815. doi:10.1093/nar/gks1094
Gandhi, J., Antonelli, A. C., Afridi, A., Vatsia, S., Joshi, G., Romanov, V., et al. (2019). Protein misfolding and aggregation in neurodegenerative diseases: a review of pathogeneses, novel detection strategies, and potential therapeutics. Rev. Neurosci. 30, 339–358. doi:10.1515/revneuro-2016-0035
García, A. E., and Onuchic, J. N. (2003). Folding a protein in a computer: an atomic description of the folding/unfolding of protein A. Proc. Natl. Acad. Sci. U.S.A. 100, 13898–13903. doi:10.1073/pnas.2335541100
Garrido, A., Santamaría, E., Fernández-Irigoyen, J., Soto, M., Simonet, C., Fernández, M., et al. (2022). Differential Phospho-Signatures in blood cells identify G2019S Carriers in Parkinson’s disease. Mov. Disord. 37, 1004–1015. doi:10.1002/mds.28927
Ghahremanian, S., Rashidi, M. M., Raeisi, K., and Toghraie, D. (2022). Molecular dynamics simulation approach for discovering potential inhibitors against SARS-CoV-2: a structural review. J. Mol. Liq. 354, 118901. doi:10.1016/j.molliq.2022.118901
Ghiglieri, V., Calabrese, V., and Calabresi, P. (2018). Alpha-synuclein: from early synaptic dysfunction to neurodegeneration. Front. Neurol. 9, 295. doi:10.3389/fneur.2018.00295
Goedert, M. (2001). Alpha-synuclein and neurodegenerative diseases. Nat. Rev. Neurosci. 2, 492–501. doi:10.1038/35081564
Goedert, M. (2015). NEURODEGENERATION. Alzheimer's and Parkinson's diseases: the prion concept in relation to assembled Aβ, tau, and α-synuclein. Science 349, 1255555. doi:10.1126/science.1255555
Goldenring, J. R. (2013). A central role for vesicle trafficking in epithelial neoplasia: intracellular highways to carcinogenesis. Nat. Rev. Cancer 13, 813–820. doi:10.1038/nrc3601
Guo, M., Suo, Y., Gao, Q., Du, H., Zeng, W., Wang, Y., et al. (2015). The protective mechanism of Ginkgolides and Ginkgo flavonoids on the TNF-α induced apoptosis of rat hippocampal neurons and its mechanisms in vitro. Heliyon 1, e00020. doi:10.1016/j.heliyon.2015.e00020
Gupta, N., Bhattacharya, S., Dutta, A., Tauchen, J., Landa, P., Urbanová, K., et al. (2024). Synthetic polyploidization induces enhanced phytochemical profile and biological activities in Thymus vulgaris L. essential oil. Sci. Rep. 14, 5608. doi:10.1038/s41598-024-56378-7
Gupta, N., Bhattacharya, S., Urbanová, K., Dutta, A., Hazra, A. K., Fernández-Cusimamani, E., et al. (2023). Systematic analysis of antimicrobial activity, phytochemistry, and in silico molecular interaction of selected essential oils and their formulations from different Indian spices against foodborne bacteria. Heliyon 9, e22480. doi:10.1016/j.heliyon.2023.e22480
Han, R., Liu, Y., Li, S., Li, X.-J., and Yang, W. (2023). PINK1-PRKN mediated mitophagy: differences between in vitro and in vivo models. Autophagy 19, 1396–1405. doi:10.1080/15548627.2022.2139080
Hernandez-Leon, A., Moreno-Pérez, G. F., Martínez-Gordillo, M., Aguirre-Hernández, E., Valle-Dorado, M. G., Díaz-Reval, M. I., et al. (2021). Lamiaceae in Mexican species, a Great but Scarcely explored source of secondary metabolites with potential pharmacological effects in Pain Relief. Molecules 26, 7632. doi:10.3390/molecules26247632
Horne, R. I., Andrzejewska, E. A., Alam, P., Brotzakis, Z. F., Srivastava, A., Aubert, A., et al. (2024). Discovery of potent inhibitors of α-synuclein aggregation using structure-based iterative learning. Nat. Chem. Biol. 20, 634–645. doi:10.1038/s41589-024-01580-x
Hu, C., Tao, L., Cao, X., and Chen, L. (2020). The solute carrier transporters and the brain: Physiological and pharmacological implications. Asian J. Pharm. Sci. 15, 131–144. doi:10.1016/j.ajps.2019.09.002
Huang, S., Hsu, L., and Chang, N. (2020). Functional role of WW domain-containing proteins in tumor biology and diseases: Insight into the role in ubiquitin-proteasome system. FASEB Bioadv 2, 234–253. doi:10.1096/fba.2019-00060
Hui, Y., Chengyong, T., Cheng, L., Haixia, H., Yuanda, Z., and Weihua, Y. (2018). Resveratrol attenuates the Cytotoxicity induced by amyloid-β1–42 in PC12 cells by upregulating Heme Oxygenase-1 via the PI3K/Akt/Nrf2 pathway. Neurochem. Res. 43, 297–305. doi:10.1007/s11064-017-2421-7
Hwang, H., Szucs, M. J., Ding, L. J., Allen, A., Ren, X., Haensgen, H., et al. (2021). Neurogranin, encoded by the Schizophrenia Risk gene NRGN, Bidirectionally modulates synaptic Plasticity via calmodulin-dependent regulation of the neuronal Phosphoproteome. Biol. Psychiatry 89, 256–269. doi:10.1016/j.biopsych.2020.07.014
Jin, S. M., and Youle, R. J. (2013). The accumulation of misfolded proteins in the mitochondrial matrix is sensed by PINK1 to induce PARK2/Parkin-mediated mitophagy of polarized mitochondria. Autophagy 9, 1750–1757. doi:10.4161/auto.26122
Kandasamy, T., Sen, P., and Ghosh, S. S. (2022). Multi-targeted drug Repurposing approach for Breast cancer via Integrated functional network analysis. Mol. Inf. 41, 2100300. doi:10.1002/minf.202100300
Kaur, I., Behl, T., Sehgal, A., Singh, S., Sharma, N., Aleya, L., et al. (2021). Connecting the dots between mitochondrial dysfunction and Parkinson’s disorder: focus mitochondria-targeting therapeutic paradigm in mitigating the disease severity. Environ. Sci. Pollut. Res. 28, 37060–37081. doi:10.1007/s11356-021-14619-6
Kim, S. B., Dsilva, C. J., Kevrekidis, I. G., and Debenedetti, P. G. (2015). Systematic characterization of protein folding pathways using diffusion maps: Application to Trp-cage miniprotein. J. Chem. Phys. 142, 085101. doi:10.1063/1.4913322
Kolouri, S., Firoozabadi, A., Salehi, A., Zarshenas, M. M., Dastgheib, S. A., Heydari, M., et al. (2016). Nepeta menthoides Boiss. & Buhse freeze-dried aqueous extract versus sertraline in the treatment of major depression: a double blind randomized controlled trial. Complement. Ther. Med. 26, 164–170. doi:10.1016/j.ctim.2016.03.016
Lipinski, C. A., Lombardo, F., Dominy, B. W., and Feeney, P. J. (1997). Experimental and computational approaches to estimate solubility and permeability in drug discovery and development settings. Adv. Drug Deliv. Rev. 23, 3–26. doi:10.1016/s0169-409x(00)00129-0
López-López, G., Moreno, L., Cogolludo, A., Galisteo, M., Ibarra, M., Duarte, J., et al. (2004). Nitric Oxide (NO) scavenging and NO protecting effects of Quercetin and their biological significance in Vascular Smooth Muscle. Mol. Pharmacol. 65, 851–859. doi:10.1124/mol.65.4.851
Mahmoud, A. H., Masters, M. R., Yang, Y., and Lill, M. A. (2020). Elucidating the multiple roles of hydration for accurate protein-ligand binding prediction via deep learning. Commun. Chem. 3, 19–13. doi:10.1038/s42004-020-0261-x
Mank-Halati, M. S., Rezaei, M., Farzaei, M. H., and Khatony, A. (2024). Comparing the effects of rosemary aromatherapy and music therapy on anxiety levels in patients undergoing general surgery: a randomized controlled clinical trial. EXPLORE 20 (5), 102976. doi:10.1016/j.explore.2024.01.002
Margiotta, A. (2021). Role of SNAREs in neurodegenerative diseases. Cells 10, 991. doi:10.3390/cells10050991
Maruyama, Y., Igarashi, R., Ushiku, Y., and Mitsutake, A. (2023). Analysis of protein folding simulation with Moving root mean square deviation. J. Chem. Inf. Model. 63, 1529–1541. doi:10.1021/acs.jcim.2c01444
McCoy, J. M., Walkenhorst, D. E., McCauley, K. S., Elaasar, H., Everett, J. R., and Mix, K. S. (2015). Orphan nuclear receptor NR4A2 induces transcription of the immunomodulatory peptide hormone prolactin. J. Inflamm. 12, 13. doi:10.1186/s12950-015-0059-2
Meena, V. K., Kumar, V., and Karalia, S. (2021). Inhibitory effect of naturally occurring Ocimum sanctum extract on α-Synuclein aggregation in aqueous solution. J. Mol. Liq. 336, 116176. doi:10.1016/j.molliq.2021.116176
Mimaki, Y., Kuroda, M., Sashida, Y., Falkinham, J. O., Hemby, S. E., and Oberlies, N. H. (2021). Phytochemical and pharmacological studies on the Ajuga genus. Phytochemistry 189, 112830. doi:10.1016/j.phytochem.2021.112830
Naïm, M., Bhat, S., Rankin, K. N., Dennis, S., Chowdhury, S. F., Siddiqi, I., et al. (2007). Solvated interaction energy (SIE) for scoring Protein−Ligand binding affinities. 1. Exploring the parameter Space. J. Chem. Inf. Model. 47, 122–133. doi:10.1021/ci600406v
Naoi, M., Inaba-Hasegawa, K., Shamoto-Nagai, M., and Maruyama, W. (2017). Neurotrophic function of phytochemicals for neuroprotection in aging and neurodegenerative disorders: modulation of intracellular signaling and gene expression. J. Neural Transm. 124, 1515–1527. doi:10.1007/s00702-017-1797-5
Nematolahi, P., Mehrabani, M., Karami-Mohajeri, S., and Dabaghzadeh, F. (2018). Effects of Rosmarinus officinalis L. on memory performance, anxiety, depression, and sleep quality in university students: a randomized clinical trial. Complement. Ther. Clin. Pract. 30, 24–28. doi:10.1016/j.ctcp.2017.11.004
Nieto, G. (2017). Biological activities of three essential oils of the Lamiaceae family. Medicines 4, 63. doi:10.3390/medicines4030063
Ning, X., Li, X., Ma, K., Pang, H., Tian, T., Hao, H., et al. (2023). VDAC1 protein regulation of oxidative damage and mitochondrial dysfunction-mediated Cytotoxicity by Silica Nanoparticles in SH-SY5Y cells. Mol. Neurobiol. 60, 6542–6555. doi:10.1007/s12035-023-03491-9
Poeckel, D., Greiner, C., Verhoff, M., Rau, O., Tausch, L., Hörnig, C., et al. (2008). Carnosic acid and carnosol potently inhibit human 5-lipoxygenase and suppress pro-inflammatory responses of stimulated human polymorphonuclear leukocytes. Biochem. Pharmacol. 76, 91–97. doi:10.1016/j.bcp.2008.04.013
Ramos da Silva, L. R., Ferreira, O. O., Cruz, J. N., de Jesus Pereira Franco, C., Oliveira dos Anjos, T., Cascaes, M. M., et al. (2021). Lamiaceae essential oils, phytochemical profile, antioxidant, and biological activities. Evidence-based Complement. Altern. Med. 2021, e6748052. doi:10.1155/2021/6748052
Rasaiah, J. C., Garde, S., and Hummer, G. (2008). Water in nonpolar Confinement: from Nanotubes to proteins and beyond. Annu. Rev. Phys. Chem. 59, 713–740. doi:10.1146/annurev.physchem.59.032607.093815
Ristic, G., Tsou, W.-L., and Todi, S. V. (2014). An optimal ubiquitin-proteasome pathway in the nervous system: the role of deubiquitinating enzymes. Front. Mol. Neurosci. 7, 72. doi:10.3389/fnmol.2014.00072
Rosilan, N. F., Jamali, M. A. M., Sufira, S. A., Waiho, K., Fazhan, H., Ismail, N., et al. (2024). Molecular docking and dynamics simulation studies uncover the host-pathogen protein-protein interactions in Penaeus vannamei and Vibrio parahaemolyticus. PLOS ONE 19, e0297759. doi:10.1371/journal.pone.0297759
Sadeghi, Z., Akaberi, M., and Valizadeh, J. (2014). Otostegia persica (Lamiaceae): a review on its ethnopharmacology, phytochemistry, and pharmacology. Avicenna J. Phytomed 4, 79–88. Available online at: https://www.ncbi.nlm.nih.gov/pmc/articles/PMC4103708/ (Accessed July 24, 2024).
Saha, M., Gupta, S., Dhiman, S., Asati, V., Ali, A., and Ali, A. (2023). Field and atom-based 3D-QSAR models of chromone (1-benzopyran-4-one) derivatives as MAO inhibitors. J. Biomol. Struct. Dyn. 41, 12171–12185. doi:10.1080/07391102.2023.2166122
Saha, S., Nandi, R., Vishwakarma, P., Prakash, A., and Kumar, D. (2021). Discovering potential RNA dependent RNA Polymerase inhibitors as Prospective drugs against COVID-19: an in silico approach. Front. Pharmacol. 12, 634047. doi:10.3389/fphar.2021.634047
Sathitloetsakun, S., and Heiman, M. (2024). “Chapter 16 - Unbiased genome-wide approaches to identify vulnerability factors in Huntington’s disease,” in Huntington’s disease. Editors X. W. Yang, L. M. Thompson, and M. Heiman (Academic Press), 397–410. doi:10.1016/B978-0-323-95672-7.00017-0
Seidel, C., Schnekenburger, M., Dicato, M., and Diederich, M. (2015). Histone deacetylase 6 in health and disease. Epigenomics 7, 103–118. doi:10.2217/epi.14.69
Sharifi-Rad, J., Quispe, C., Bouyahya, A., El Menyiy, N., El Omari, N., Shahinozzaman, M., et al. (2022). Ethnobotany, phytochemistry, biological activities, and health-promoting effects of the genus Bulbophyllum. Evidence-Based Complement. Altern. Med. 2022, 6727609. doi:10.1155/2022/6727609
Shim, K. H., Kang, M. J., Youn, Y. C., An, S. S. A., and Kim, S. (2022). Alpha-synuclein: a pathological factor with Aβ and tau and biomarker in Alzheimer’s disease. Alzheimer’s Res. Ther. 14, 201. doi:10.1186/s13195-022-01150-0
Shoval, G., Bar-Shira, O., Zalsman, G., John Mann, J., and Chechik, G. (2014). Transitions in the transcriptome of the serotonergic and dopaminergic systems in the human brain during adolescence. Eur. Neuropsychopharmacol. 24, 1123–1132. doi:10.1016/j.euroneuro.2014.02.009
Sinha, M., Jagadeesan, R., Kumar, N., Saha, S., Kothandan, G., and Kumar, D. (2022). In-silico studies on Myo inositol-1-phosphate synthase of Leishmania donovani in search of anti-leishmaniasis. J. Biomol. Struct. Dyn. 40, 3371–3384. doi:10.1080/07391102.2020.1847194
Stefanis, L. (2012). α-Synuclein in Parkinson’s disease. Cold Spring Harb. Perspect. Med. 2, a009399. doi:10.1101/cshperspect.a009399
Suthar, S. K., and Lee, S.-Y. (2023). The role of Superoxide dismutase 1 in amyotrophic lateral sclerosis: Identification of signaling pathways, Regulators, molecular interaction networks, and biological functions through Bioinformatics. Brain Sci. 13, 151. doi:10.3390/brainsci13010151
Upadhyay, R. K. (2014). Drug delivery systems, CNS protection, and the blood brain barrier. Biomed. Res. Int. 2014, 869269. doi:10.1155/2014/869269
Uversky, V. N. (2017). Looking at the recent advances in understanding α-synuclein and its aggregation through the proteoform prism. F1000Res 6, 525. doi:10.12688/f1000research.10536.1
Wang, L., Li, J., Liu, E., Kinnebrew, G., Zhang, X., Stover, D., et al. (2019). Identification of Alternatively-Activated pathways between primary Breast cancer and Liver Metastatic cancer using Microarray data. Genes 10, 753. doi:10.3390/genes10100753
Wang, X., Hu, Y., and Xu, R. (2024). The pathogenic mechanism of TAR DNA-binding protein 43 (TDP-43) in amyotrophic lateral sclerosis. Neural Regen. Res. 19, 800–806. doi:10.4103/1673-5374.382233
Williams-Gray, C. H., and Worth, P. F. (2016). Parkinson’s disease. Medicine 44, 542–546. doi:10.1016/j.mpmed.2016.06.001
Wink, M. (2020). Medicinal plants: a source of anti-parasitic secondary metabolites. Int. J. Parasitol. Drugs Drug Resist. 12, 15–34. doi:10.1016/j.ijpddr.2020.07.001
Yadav, D. K. (2021). Potential therapeutic strategies of phytochemicals in neurodegenerative disorders. Curr. Top. Med. Chem. 21, 2814–2838. doi:10.2174/1568026621666211201150217
Yamamoto, E., Akimoto, T., Mitsutake, A., and Metzler, R. (2021). Universal relation between Instantaneous Diffusivity and radius of gyration of proteins in aqueous solution. Phys. Rev. Lett. 126, 128101. doi:10.1103/PhysRevLett.126.128101
Yang, B., Zhang, D., Shi, X., Shen, C., Hao, Y., Zhang, T., et al. (2021). Construction, Identification and analysis of the interaction network of African Swine fever virus MGF360-9L with host proteins. Viruses 13, 1804. doi:10.3390/v13091804
Zacharowski, K., Zacharowski, P. A., Koch, A., Baban, A., Tran, N., Berkels, R., et al. (2006). Toll-like receptor 4 plays a crucial role in the immune–adrenal response to systemic inflammatory response syndrome. Proc. Natl. Acad. Sci. U.S.A. 103, 6392–6397. doi:10.1073/pnas.0601527103
Zahran, E. M., Abdelmohsen, U. R., Kolkeila, A., Salem, M. A., Khalil, H. E., Desoukey, S. Y., et al. (2021). Anti-epileptic potential, metabolic profiling and in silico studies of the aqueous fraction from Ocimum menthiifolium benth, family Lamiaceae. Nat. Prod. Res. 35, 5972–5976. doi:10.1080/14786419.2020.1809396
Zengin, G., Mahomoodally, M. F., and Mollica, A. (2018). Lamiaceae plants: a new source of innovative drugs for Alzheimer’s and diabetes? J. Ethnopharmacol. 213, 156–173. doi:10.1016/j.jep.2017.11.022
Zhang, Y., Hou, P., He, D. C., Wang, H., and He, H. (2021). RACK1 degrades MAVS to promote bovine ephemeral fever virus replication via upregulating E3 ubiquitin ligase STUB1. Vet. Microbiol. 257, 109096. doi:10.1016/j.vetmic.2021.109096
Keywords: functional network analysis, molecular docking, MD simulation, neuroprotective agent, pathway enrichment analysis, phytochemical
Citation: Bhattacharya S, Gupta N, Dutta A, Khanra PK, Dutta R, Žiarovská J, Tzvetkov NT, Severová L, Kopecká L, Milella L and Fernández-Cusimamani E (2025) Repurposing major metabolites of lamiaceae family as potential inhibitors of α-synuclein aggregation to alleviate neurodegenerative diseases: an in silico approach. Front. Pharmacol. 16:1519145. doi: 10.3389/fphar.2025.1519145
Received: 29 October 2024; Accepted: 01 April 2025;
Published: 16 April 2025.
Edited by:
Anil K. Giri, University of Helsinki, FinlandReviewed by:
Francisca Pérez-Severiano, Instituto Nacional de Neurología y Neurocirugía MVS y, MexicoCopyright © 2025 Bhattacharya, Gupta, Dutta, Khanra, Dutta, Žiarovská, Tzvetkov, Severová, Kopecká, Milella and Fernández-Cusimamani. This is an open-access article distributed under the terms of the Creative Commons Attribution License (CC BY). The use, distribution or reproduction in other forums is permitted, provided the original author(s) and the copyright owner(s) are credited and that the original publication in this journal is cited, in accordance with accepted academic practice. No use, distribution or reproduction is permitted which does not comply with these terms.
*Correspondence: Luigi Milella, bHVpZ2kubWlsZWxsYUB1bmliYXMuaXQ=
Disclaimer: All claims expressed in this article are solely those of the authors and do not necessarily represent those of their affiliated organizations, or those of the publisher, the editors and the reviewers. Any product that may be evaluated in this article or claim that may be made by its manufacturer is not guaranteed or endorsed by the publisher.
Research integrity at Frontiers
Learn more about the work of our research integrity team to safeguard the quality of each article we publish.