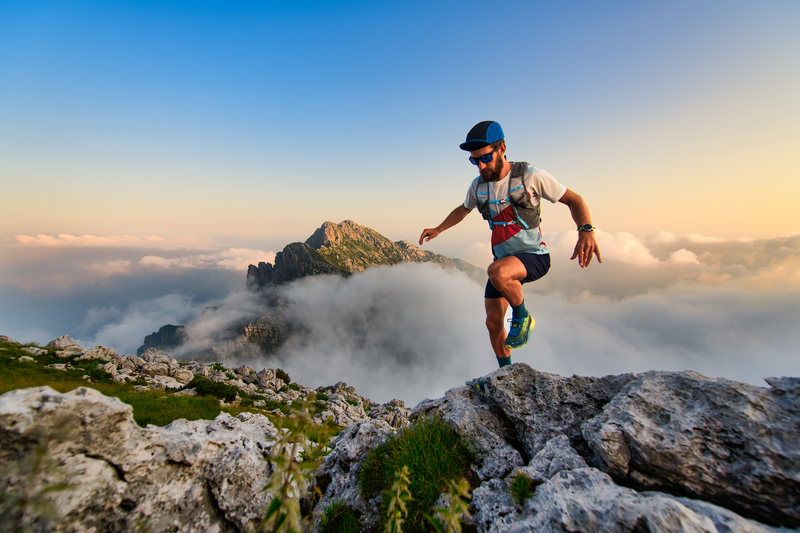
94% of researchers rate our articles as excellent or good
Learn more about the work of our research integrity team to safeguard the quality of each article we publish.
Find out more
ORIGINAL RESEARCH article
Front. Pharmacol. , 21 November 2024
Sec. Pharmacoepidemiology
Volume 15 - 2024 | https://doi.org/10.3389/fphar.2024.1510220
This article is part of the Research Topic Advances in Drug-induced Diseases Volume II View all 42 articles
Objectives: This study aimed to identify the risk factors for pancreatic cancer through machine learning.
Methods: We investigated the relationships between different risk factors and pancreatic cancer using a real-world retrospective cohort study conducted at West China Hospital of Sichuan University. Multivariable logistic regression, with pancreatic cancer as the outcome, was used to identify covariates associated with pancreatic cancer. The machine learning model extreme gradient boosting (XGBoost) was adopted as the final model for its high performance. Shapley additive explanations (SHAPs) were utilized to visualize the relationships between these potential risk factors and pancreatic cancer.
Results: The cohort included 1,982 patients. The median ages for pancreatic cancer and nonpancreatic cancer groups were 58.1 years (IQR: 51.3–64.4) and 57.5 years (IQR: 49.5–64.9), respectively. Multivariable logistic regression indicated that kirsten rats arcomaviral oncogene homolog (KRAS) gene mutation, hyperlipidaemia, pancreatitis, and pancreatic cysts are significantly correlated with an increased risk of pancreatic cancer. The five most highly ranked features in the XGBoost model were KRAS gene mutation status, age, alcohol consumption status, pancreatitis status, and hyperlipidaemia status.
Conclusion: Machine learning algorithms confirmed that KRAS gene mutation, hyperlipidaemia, and pancreatitis are potential risk factors for pancreatic cancer. Additionally, the coexistence of KRAS gene mutation and pancreatitis, as well as KRAS gene mutation and pancreatic cysts, is associated with an increased risk of pancreatic cancer. Our findings offered valuable implications for public health strategies targeting the prevention and early detection of pancreatic cancer.
Pancreatic cancer (PC) is a leading cause of cancer-related death globally, with a 5-year survival rate of approximately 13% (Siegel et al., 2024; Pourshams et al., 2019). PC has an increasing mortality rate and often results in metastasis due to its subtle early symptoms, so most patients are diagnosed at an advanced stage, which limits treatment options (Park et al., 2021). Although computerized tomography (CT) and magnetic resonance imaging (MRI) are effective at diagnosing pancreatic cancer, the cost of these two techniques is relatively high, which limits their wide use (Yang et al., 2021; Diehl et al., 1998; Lu et al., 1997; Sandrasegaran et al., 2013). Seeking potential risk factors could be conducive to early diagnosis and intervention in the risk population.
Generally, risk factors can be categorized into genetic and hereditary factors, environmental factors, medical conditions, and demographic Factors. Genetic factors play a significant role in developing pancreatic cancer with about 10% of pancreatic cancer cases attributed to inherited genetic mutations (Mario et al., 2018). In addition, previous studies indicated that smoking, obesity, and alcohol consumption are responsible for pancreatic cancer (Mario et al., 2018). There is also compelling evidence that factors like chronic pancreatitis and age are associated with pancreatic cancer (Mario et al., 2018; Yuan et al., 2022). Kirsten rat sarcoma viral oncogene homolog (KRAS) may influence pancreatic cancer development through various metabolic alterations. These alterations include enhanced glucose uptake, differential channeling of glucose intermediates, reprogramming of glutamine metabolism, increased autophagy, and macropinocytosis (Bryant et al., 2014). Current knowledge about risk factors for developing pancreatic cancer is focused mainly on the impact of specific risk factors (Yuan et al., 2022; Maisonneuve and Lowenfels, 2015; Kirkegård et al., 2017). However, PC is caused by multiple factors, and little is known regarding the relative predictive power of different risk factors. Traditional methods for identifying risk factors rely on case-control studies and logistic regression models. However, logistic regression models have limitations in data processing, particularly when dealing with large-scale high-dimensional clinical data (Oosterhoff et al., 2022; Song et al., 2021). To address these limitations, we designed a retrospective cohort study to reveal the relationships between different risk factors and pancreatic cancer based on machine learning.
A retrospective cohort study was conducted using electronic medical records (EMR) from 1 January 2010, and 31 December 2023, at West China Hospital (WCH), Sichuan University (Chengdu, China). All data were extracted from the hospital EMR. The EMR contains information stored in structured or semistructured formats (e.g., patient demographics, physical examination, laboratory tests, medications, and diagnoses). This study was approved by the Institutional Review Board of WCH in May 2021 (WCH 2021-590), and patient consent was waived.
We included 1,982 patients who had a kirsten rats arcomaviral oncogene homolog (KRAS) gene testing in WCH between 1 January 2010, and 31 December 2023. Patients who met any of the following criteria were excluded: had a history of other malignancies, had incomplete data or missing important information, or had serious complications or illness. Following inclusion, data loss was minimal due to the low rate of missingness in our data source. Given this low rate, statistical methods for handling missing data were not applied.
Patients with pancreatic cancer were defined as individuals who met the diagnostic criteria for pancreatic cancer and had a confirmed diagnosis at West China Hospital. The diagnostic criteria included clinical symptoms (such as abdominal pain and jaundice), radiological assessments (CT and MRI), histopathological examination, and blood tests (serum CA19-9>39 U/mL) (Chan et al., 2014; Goonetilleke and Siriwardena, 2007; Ni et al., 2005). These factors were analyzed comprehensively to establish the diagnosis by the doctor.
Previous studies identified some potential risk factors for pancreatic cancer. Based on clinical evidence and biological rationale (Kamisawa et al., 2016; McGuigan et al., 2018), we compiled an extensive list of variables to identify potential risk factors, classifying them into four groups: demographic characteristics (age and sex), living habits (smoking and drinking), non-pancreatic comorbidities (hypertension, diabetes, uarthritis/hyperuricemia, overweight/obesity and hyperlipidemia) and pancreatic-related diseases (pancreatic cysts and pancreatitis). For statistical analysis, chi-square tests were used for normally distributed categorical variables, while Wilcoxon rank-sum tests were used for continuous variables that did not conform to a normal distribution. A p-value of ≤0.05 was considered statistically significant.
Multivariable logistic regression analyses were performed to calculate the z-value and p-value of the association between each covariate and pancreatic cancer. This initial screening aimed to identify independent variables significantly associated with the disease (p < 0.05).
Significant variables were then included in the multivariable logistic regression model to further evaluate their effects while accounting for potential confounders. The z-value and p-value were computed to estimate the relationship between each variable and pancreatic cancer within the model. Additionally, we performed pairwise multivariable regression with generalized linear models to assess the synergistic effects of KRAS gene mutation and other factors on pancreatic cancer. All statistical analyses were performed using R (version 4.1.3).
All the covariates were included in the machine learning models. Twelve machine-learning methods were tested: extreme gradient boosting (XGBoost), random forest (RF), classification and regression tree (CART), support vector classifier (SVC), adaptive boosting (AdaBoost), gradient boosting, neural network (NN), extremely randomized trees (ExtraTrees), balanced bagging classifier, balanced random forest classifier (BalancedRF), random undersampling boosting (RUSBoost) and easyensemble. All variables were included in these models. A training: testing (80:20) approach was used to compute the final set of model-fit-parameters. RandomizedSearchCV was used to search for the optimal hyperparameters for the 12 models. All machine learning models were constructed using 5-fold cross-validation. The accuracy, precision, F1 score, recall, and area under the receiver operating characteristic (AUROC) curve were used to evaluate model performance. Additionally, SHAP values are a powerful tool for interpreting the predictive outcomes of machine learning models by quantifying the impact of each feature on the model’s predictions. In this study, the SHAP technique was utilized to visualize the relationships between these potential risk factors and pancreatic cancer. We included only positive SHAP values, as our goal was to identify potential risk factors for pancreatic cancer. Positive SHAP values specifically indicate contributions toward an increased risk of pancreatic cancer, aligning with our study’s focus.
The study involved 1,982 patients in the cohort during the study period. As shown in Table 1, we divided the patients into two groups: a pancreatic cancer group and a nonpancreatic cancer group. The median ages for pancreatic cancer and nonpancreatic cancer groups were 58.1 years (IQR: 51.3–64.4) and 57.5 years (IQR: 49.5–64.9), respectively. The gender imbalance observed in this study was statistically significant (p = 0.002), with a greater proportion of males being found in the nonpancreatic group. Additionally, the pancreatic cancer group exhibited a significantly lower median body mass index (BMI) (22.58, IQR: 20.70–24.07) compared to the nonpancreatic cancer group (23.34, IQR: 20.90–25.39) (p = 0.004507). We also found a greater prevalence of smoking in the nonpancreatic cancer group, whereas alcohol consumption did not differ significantly between the groups. Notably, the pancreatic cancer group had a greater frequency of KRAS gene mutation (83.7% vs. 51.3%, p < 0.001) and a greater prevalence of pancreatitis (18.6% vs. 0.9%, p < 0.001) and pancreatic cysts (6.2% vs. 0.2%, p < 0.001) compared to the nonpancreatic cancer group.
Table 2 presents the results of multivariable logistic regression analyses assessing the associations between baseline variables and pancreatic cancer status. The receiver operating characteristic (ROC) curve of the multivariable logistic regression model revealed that the AUC of the integrated factors was 0.829 (Supplementary Figure S1). KRAS gene mutation (OR = 9.09, 95% CI: 5.50–15.75, p < 0.001), hyperlipidaemia (OR = 3.37, 95% CI: 1.35–7.86, p = 0.006), pancreatitis (OR = 29.97, 95% CI: 12.93–72.27, p < 0.001), and pancreatic cysts (OR = 17.29, 95% CI: 3.85–97.69, p < 0.001) were significantly correlated with an increased risk of pancreatic cancer. After the screening, KRAS gene mutation, hyperlipidaemia, pancreatitis, and pancreatic cysts were entered into the model as independent variables, and KRAS gene mutation (OR = 8.99, 95% CI: 5.48–15.46, p < 0.001), hyperlipidaemia (OR = 3.46, 95% CI: 1.45–7.65, p = 0.003), pancreatitis (OR = 25.30, 95% CI: 11.46–57.79 p < 0.001), and pancreatic cysts (OR = 21.12, 95% CI: 4.71–119.03, p = 0.0001) were significantly associated with the risk of developing pancreatic cancer (Supplementary Table S1).
In this study, we developed 12 machine-learning models to identify risk factors for pancreatic cancer (Supplementary Table S2). Five-fold cross-validation was used to evaluate the performance of the constructed models, and we found that RF, CART, and XGBoost outperformed models of data imbalance processing technology (BalanceBagging, BalanceRF, RUSBoost, and EasyEnsemble) (Supplementary Figure S2). We also assessed their performance using metrics such as the area under the curve (AUC), accuracy, precision, recall, and F1 score. As shown in Supplementary Table S3, XGBoost was the best-performing model (AUC = 0.999, accuracy = 0.994, precision = 1.000, recall = 0.909, F1 score = 0.952). The recall and precision scores of RF and CART models are low, as these models often prioritize achieving higher accuracy by classifying the majority of samples as negative cases. According to the above assessment, XGBoost was chosen as the final machine learning model.
SHAP values indicate the importance of each feature to the prediction of individual instances. We assessed the contributions of different factors in the XGBoost models using SHAP values. Figure 1 displays the importance scores of the different factors. The study identified KRAS gene mutation, age, alcohol consumption status, pancreatitis status, and hyperlipidaemia status as the five most common potential risk factors.
Pairwise multivariable regression analyses were conducted to investigate the synergistic effects of KRAS gene mutation and other factors. We found a significant association between the coexistence of KRAS gene mutation and pancreatitis (OR = 14.18, 95% CI: 2.78–105.26, P < 0.01), as well as between KRAS gene mutation and pancreatic cysts (OR = 20.62, 95% CI: 7.56–60.30, P = 0.0026), with an increased risk of pancreatic cancer (Figure 2).
The results of this retrospective cohort study showed that KRAS gene mutation, hyperlipidaemia, pancreatitis, and pancreatic cysts are significantly associated with the risk of developing pancreatic cancer. A machine learning model utilizing demographic characteristics, living habits, nonpancreatic diseases, and pancreatic disease had strong predictive performance (XGBoost, AUC = 0.999). The greatest predictors for pancreatic cancer included KRAS gene mutation, age, alcohol consumption status, pancreatitis, and hyperlipidemia. Both logistic regression and machine learning confirmed that KRAS gene mutation, hyperlipidaemia and pancreatitis are potential risk factors for pancreatic cancer. Additionally, the coexistence of KRAS gene mutation and pancreatitis, as well as KRAS gene mutation and pancreatic cysts, is associated with an increased risk of pancreatic cancer.
The study is among the first to apply advanced machine learning algorithms, specifically XGBoost, to real-world clinical data for the identification of pancreatic cancer risk factors. While previous studies have relied on traditional statistical methods, such as logistic regression, the use of machine learning allows for the handling of high-dimensional data and complex interactions between variables, providing more robust risk prediction models. We also identified the synergistic effects between KRAS mutation and other risk factors, offering new insights into the genetic and biological mechanisms of pancreatic cancer development. Additionally, machine learning models trained on real-world data enable promising applications for improving pancreatic cancer risk assessment, early detection, and diagnosis. However, further validation in diverse populations and prospective clinical studies will be crucial before widespread implementation.
Bryant, Kirsten L. et al. have demonstrated that oncogenic KRAS plays a central role in regulating tumor metabolism, orchestrating diverse metabolic changes such as enhanced glucose uptake, selective channeling of glucose intermediates, reprogrammed glutamine metabolism, increased autophagy, and macropinocytosis (Bryant et al., 2014). Several prior studies have shown similar results: KRAS mutation is related to PC and is found in almost all pancreatic ductal adenocarcinomas (PDACs) (Cox et al., 2014; Luo, 2021). Kamisawa, Terumi et al. reported that KRAS mutation and alterations in CDKN2A are early events in pancreatic tumorigenesis (Kamisawa et al., 2016). Bannoura SF et al. suggested that oncogenic KRAS signaling is critical for both the initiation and maintenance of pancreatic cancer; therefore, it is an ideal target for therapy (Bannoura et al., 2021). Although KRAS is a critical oncogene and therefore an important therapeutic target, its therapeutic inhibition is challenging. Recently, specific mutant KRAS inhibitors have been discovered (Bannoura et al., 2021).
Smoking is recognized as a risk factor for many types of cancer (Sasco et al., 2004; Scherübl, 2023). A review and meta-analysis concluded that cigarette smoking causes a 75% increase in the risk of pancreatic cancer compared to nonsmokers, and the risk persists for a minimum of 10 years after smoking cessation (Iodice et al., 2008). Similarly, a meta-analysis indicated that pancreatic cancer risk increases sharply with a low number of cigarettes smoked or after a 5 years of smoking and that it rapidly decreases a few years after cessation, although it takes almost 20 years to reach that of nonsmokers (Lugo et al., 2018). However, we did not find the same result, probably because of bias and the limited study population.
A growing body of evidence suggests that longstanding preexisting chronic pancreatitis is a strong risk factor for pancreatic cancer (Kamisawa et al., 2007; Dítĕ et al., 2010; Kudo et al., 2011). Although there is a strong link between chronic pancreatitis and pancreatic cancer, over 20 years, only approximately five percent of patients with chronic pancreatitis will develop pancreatic cancer (Raimondi et al., 2010). Lin et al. confirmed that hyperlipidaemia can promote tumor growth and subcutaneous tumor formation in mice, and Roy et al. described a two-way relationship between pancreatic cancer and diabetes, which might indicate that there is a complicated relationship between metabolic disease and pancreatic cancer (Qin et al., 2023; Roy et al., 2021).
Identifying risk factors for pancreatic cancer offers significant benefits in clinical and public health contexts. Early detection and targeted screening of high-risk populations can improve the proportion of early-stage diagnoses, which is associated with increased survival rates (Grigorescu et al., 2024). Additionally, understanding modifiable risk factors facilitates the development of targeted public health initiatives, such as lifestyle modification programs and genetic counseling, aimed at mitigating risk in susceptible populations.
A potential weakness of this study is the retrospective nature of this cohort. Since retrospective studies rely on existing records that were not originally collected for research purposes, key information is often missing or incomplete (Talari and Goyal, 2020). There may be variations in diagnostic criteria, treatment protocols, or data entry practices that are difficult to account for retrospectively. Medical records may lack detailed information on confounding variables or precise measurements necessary for robust analysis. These limitations can introduce potential biases and restrict the validity and generalizability of study conclusions. Several statistical methods were used to control for confounding factors; however, some unmeasured residual confounding factors were likely present. Furthermore, due to data inaccuracies and incomplete data, misclassification bias was not uncommon in retrospective database studies. The strength of inference on causality was thus weakened given the retrospective nature of the study. Future studies could address these limitations by implementing strategies such as improving data collection processes, refining study designs, and employing advanced analytical approaches. These enhancements may help to mitigate data gaps, reduce bias, and strengthen the reliability of study findings (Popovic and Huecker, 2024; Jager et al., 2020).
We confirmed that KRAS gene mutation, hyperlipidaemia, pancreatitis, and pancreatic cysts are significantly correlated with an increased risk of pancreatic cancer. KRAS gene mutation, age, alcohol consumption status, pancreatitis status, and hyperlipidaemia status are the strongest predictors of pancreatic cancer. Both logistic regression and machine learning algorithms confirmed that KRAS gene mutation, hyperlipidaemia and pancreatitis are potential risk factors for pancreatic cancer. Additionally, the coexistence of KRAS gene mutation and pancreatitis, as well as KRAS gene mutation and pancreatic cysts, is associated with an increased risk of pancreatic cancer.
The original contributions presented in the study are included in the article/Supplementary Material, further inquiries can be directed to the corresponding author.
The studies involving humans were approved by the Institutional Review Board of WCH (WCH 2021-590). The studies were conducted in accordance with the local legislation and institutional requirements. The participants provided their written informed consent to participate in this study. Written informed consent was obtained from the individual(s) for the publication of any potentially identifiable images or data included in this article.
NS: Conceptualization, Formal Analysis, Methodology, Software, Writing–original draft, Writing–review and editing. RT: Formal Analysis, Software, Writing–original draft. YZ: Conceptualization, Validation, Visualization, Writing–original draft. JN: Supervision, Writing–review and editing. YH: Supervision, Validation, Writing–review and editing. CL: Data curation, Writing–original draft. YX: Formal Analysis, Writing–original draft. BZ: Formal Analysis, Writing–original draft. YZ: Conceptualization, Formal Analysis, Funding acquisition, Methodology, Software, Writing–original draft.
The author(s) declare that financial support was received for the research, authorship, and/or publication of this article. NS was supported by grants from the Sichuan Province Science and Technology Support Program (grant number 2023JDR0243) and the Health Commission Program (grant number 2020-111). This research was supported by the National Key Clinical Specialties Construction Program.
We acknowledge support from the West China School of Pharmacy, State Key Laboratory of Biotherapy and Cancer Center, West China Hospital, West China Medical School, and Collaborative Innovation Center for Biotherapy, Sichuan University. We also thank ZS for his contribution to the paper.
The authors declare that the research was conducted in the absence of any commercial or financial relationships that could be construed as a potential conflict of interest.
The author(s) declare that no Generative AI was used in the creation of this manuscript.
The Supplementary Material for this article can be found online at: https://www.frontiersin.org/articles/10.3389/fphar.2024.1510220/full#supplementary-material
All claims expressed in this article are solely those of the authors and do not necessarily represent those of their affiliated organizations, or those of the publisher, the editors and the reviewers. Any product that may be evaluated in this article, or claim that may be made by its manufacturer, is not guaranteed or endorsed by the publisher.
Bannoura, S. F., Uddin, M. H., Nagasaka, M., Fazili, F., Al-Hallak, M. N., Philip, P. A., et al. (2021). Targeting KRAS in pancreatic cancer: new drugs on the horizon. Cancer Metastasis Rev. 40, 819–835. doi:10.1007/s10555-021-09990-2
Bryant, K. L., Mancias, J. D., Kimmelman, A. C., and Der, C. J. (2014). KRAS: feeding pancreatic cancer proliferation. Trends Biochem. Sci. 39, 91–100. doi:10.1016/j.tibs.2013.12.004
Chan, A., Prassas, I., Dimitromanolakis, A., Brand, R. E., Serra, S., Diamandis, E. P., et al. (2014). Validation of biomarkers that complement CA19.9 in detecting early pancreatic cancer. Clin. Cancer Res. 20, 5787–5795. doi:10.1158/1078-0432.CCR-14-0289
Cox, A. D., Fesik, S. W., Kimmelman, A. C., Luo, J., and Der, C. J. (2014). Drugging the undruggable RAS: mission possible? Nat. Rev. Drug Discov. 13, 828–851. doi:10.1038/nrd4389
Diehl, S. J., Lehmann, K. J., Sadick, M., Lachmann, R., and Georgi, M. (1998). Pancreatic cancer: value of dual-phase helical CT in assessing resectability. Radiology 206, 373–378. doi:10.1148/radiology.206.2.9457188
Dítĕ, P., Novotný, I., Precechtĕlová, M., Růzicka, M., Záková, A., Hermanová, M., et al. (2010). Incidence of pancreatic carcinoma in patients with chronic pancreatitis. Hepatogastroenterology 57, 957–960.
Goonetilleke, K. S., and Siriwardena, A. K. (2007). Systematic review of carbohydrate antigen (CA 19-9) as a biochemical marker in the diagnosis of pancreatic cancer. Eur. J. Surg. Oncol. 33, 266–270. doi:10.1016/j.ejso.2006.10.004
Grigorescu, R. R., Husar-Sburlan, I. A., and Gheorghe, C. (2024). Pancreatic cancer: a review of risk factors. Life 14, 980. doi:10.3390/life14080980
Iodice, S., Gandini, S., Maisonneuve, P., and Lowenfels, A. B. (2008). Tobacco and the risk of pancreatic cancer: a review and meta-analysis. Langenbecks Arch. Surg. 393, 535–545. doi:10.1007/s00423-007-0266-2
Jager, K. J., Tripepi, G., Chesnaye, N. C., Dekker, F. W., Zoccali, C., and Stel, V. S. (2020). Where to look for the most frequent biases? Nephrol. Carlt. Vic. 25, 435–441. doi:10.1111/nep.13706
Kamisawa, T., Tu, Y., Egawa, N., Nakajima, H., Tsuruta, K., and Okamoto, A. (2007). The incidence of pancreatic and extrapancreatic cancers in Japanese patients with chronic pancreatitis. Hepatogastroenterology 54, 1579–1581.
Kamisawa, T., Wood, L. D., Itoi, T., and Takaori, K. (2016). Pancreatic cancer. Lancet 388, 73–85. doi:10.1016/S0140-6736(16)00141-0
Kirkegård, J., Mortensen, F. V., and Cronin-Fenton, D. (2017). Chronic pancreatitis and pancreatic cancer risk: a systematic review and meta-analysis. Am. J. Gastroenterol. 112, 1366–1372. doi:10.1038/ajg.2017.218
Kudo, Y., Kamisawa, T., Anjiki, H., Takuma, K., and Egawa, N. (2011). Incidence of and risk factors for developing pancreatic cancer in patients with chronic pancreatitis. Hepatogastroenterology 58, 609–611.
Lu, D. S., Reber, H. A., Krasny, R. M., Kadell, B. M., and Sayre, J. (1997). Local staging of pancreatic cancer: criteria for unresectability of major vessels as revealed by pancreatic-phase, thin-section helical CT. AJR Am. J. Roentgenol. 168, 1439–1443. doi:10.2214/ajr.168.6.9168704
Lugo, A., Peveri, G., Bosetti, C., Bagnardi, V., Crippa, A., Orsini, N., et al. (2018). Strong excess risk of pancreatic cancer for low frequency and duration of cigarette smoking: a comprehensive review and meta-analysis. Eur. J. Cancer 104, 117–126. doi:10.1016/j.ejca.2018.09.007
Luo, J. (2021). KRAS mutation in pancreatic cancer. Semin. Oncol. 48, 10–18. doi:10.1053/j.seminoncol.2021.02.003
Maisonneuve, P., and Lowenfels, A. B. (2015). Risk factors for pancreatic cancer: a summary review of meta-analytical studies. Int. J. Epidemiol. 44, 186–198. doi:10.1093/ije/dyu240
Mario, C., Marilisa, F., Kryssia, I. R. C., Pellegrino, C., Ginevra, C., Chiara, M., et al. (2018). Epidemiology and risk factors of pancreatic cancer. Acta Bio Medica Atenei Parm. 89, 141–146. doi:10.23750/abm.v89i9-S.7923
McGuigan, A., Kelly, P., Turkington, R. C., Jones, C., Coleman, H. G., and McCain, R. S. (2018). Pancreatic cancer: a review of clinical diagnosis, epidemiology, treatment and outcomes. World J. Gastroenterol. 24, 4846–4861. doi:10.3748/wjg.v24.i43.4846
Ni, X. G., Bai, X. F., Mao, Y. L., Shao, Y. F., Wu, J. X., Shan, Y., et al. (2005). The clinical value of serum CEA, CA19-9, and CA242 in the diagnosis and prognosis of pancreatic cancer. Eur. J. Surg. Oncol. 31, 164–169. doi:10.1016/j.ejso.2004.09.007
Oosterhoff, J. H. F., Gravesteijn, B. Y., Karhade, A. V., Jaarsma, R. L., Kerkhoffs, GMMJ, Ring, D., et al. (2022). Feasibility of machine learning and logistic regression algorithms to predict outcome in orthopaedic trauma surgery. J. Bone Jt. Surg. Am. 104, 544–551. doi:10.2106/JBJS.21.00341
Park, W., Chawla, A., and O’Reilly, E. M. (2021). Pancreatic cancer: a review. JAMA 326 (326), 851–862. doi:10.1001/jama.2021.13027
Popovic, A., and Huecker, M. R. (2024). “Study bias,” in StatPearls (Treasure Island (FL): StatPearls Publishing).
Pourshams, A., Sepanlou, S. G., Ikuta, K. S., Bisignano, C., Safiri, S., Roshandel, G., et al. (2019). The global, regional, and national burden of pancreatic cancer and its attributable risk factors in 195 countries and territories, 1990–2017: a systematic analysis for the Global Burden of Disease Study 2017. Lancet Gastroenterology and Hepatology 4 (4), 934–947. doi:10.1016/S2468-1253(19)30347-4
Qin, L., Sun, K., Shi, L., Xu, Y., and Zhang, R. (2023). High-fat mouse model to explore the relationship between abnormal lipid metabolism and enolase in pancreatic cancer. Mediat. Inflamm. 2023, 4965223. doi:10.1155/2023/4965223
Raimondi, S., Lowenfels, A. B., Morselli-Labate, A. M., Maisonneuve, P., and Pezzilli, R. (2010). Pancreatic cancer in chronic pancreatitis; aetiology, incidence, and early detection. Best. Pract. Res. Clin. Gastroenterol. 24, 349–358. doi:10.1016/j.bpg.2010.02.007
Roy, A., Sahoo, J., Kamalanathan, S., Naik, D., Mohan, P., and Kalayarasan, R. (2021). Diabetes and pancreatic cancer: exploring the two-way traffic. World J. Gastroenterol. 27, 4939–4962. doi:10.3748/wjg.v27.i30.4939
Sandrasegaran, K., Nutakki, K., Tahir, B., Dhanabal, A., Tann, M., and Cote, G. A. (2013). Use of diffusion-weighted MRI to differentiate chronic pancreatitis from pancreatic cancer. AJR Am. J. Roentgenol. 201, 1002–1008. doi:10.2214/AJR.12.10170
Sasco, A. J., Secretan, M. B., and Straif, K. (2004). Tobacco smoking and cancer: a brief review of recent epidemiological evidence. Lung Cancer 45 (Suppl. 2), S3–S9. doi:10.1016/j.lungcan.2004.07.998
Scherübl, H. (2023). Tobacco smoking and cancer risk. Pneumologie 77, 27–32. doi:10.1055/a-1916-1466
Siegel, R. L., Giaquinto, A. N., and Jemal, A. (2024). Cancer statistics, 2024. CA Cancer J. Clin. 74, 12–49. doi:10.3322/caac.21820
Song, X., Liu, X., Liu, F., and Wang, C. (2021). Comparison of machine learning and logistic regression models in predicting acute kidney injury: a systematic review and meta-analysis. Int. J. Med. Inf. 151, 104484. doi:10.1016/j.ijmedinf.2021.104484
Talari, K., and Goyal, M. (2020). Retrospective studies - utility and caveats. J. R. Coll. Physicians Edinb 50, 398–402. doi:10.4997/JRCPE.2020.409
Yang, J., Xu, R., Wang, C., Qiu, J., Ren, B., and You, L. (2021). Early screening and diagnosis strategies of pancreatic cancer: a comprehensive review. Cancer Commun. (Lond). 41, 1257–1274. doi:10.1002/cac2.12204
Keywords: pancreatic cancer, machine learning, multivariable logistic regression, risk factors, KRAS gene mutation
Citation: Su N, Tang R, Zhang Y, Ni J, Huang Y, Liu C, Xiao Y, Zhu B and Zhao Y (2024) Using machine learning to identify risk factors for pancreatic cancer: a retrospective cohort study of real-world data. Front. Pharmacol. 15:1510220. doi: 10.3389/fphar.2024.1510220
Received: 12 October 2024; Accepted: 11 November 2024;
Published: 21 November 2024.
Edited by:
Linan Zeng, McMaster University, CanadaCopyright © 2024 Su, Tang, Zhang, Ni, Huang, Liu, Xiao, Zhu and Zhao. This is an open-access article distributed under the terms of the Creative Commons Attribution License (CC BY). The use, distribution or reproduction in other forums is permitted, provided the original author(s) and the copyright owner(s) are credited and that the original publication in this journal is cited, in accordance with accepted academic practice. No use, distribution or reproduction is permitted which does not comply with these terms.
*Correspondence: Yinglan Zhao, emhhb3lpbmdsYW5Ac2N1LmVkdS5jbg==
Disclaimer: All claims expressed in this article are solely those of the authors and do not necessarily represent those of their affiliated organizations, or those of the publisher, the editors and the reviewers. Any product that may be evaluated in this article or claim that may be made by its manufacturer is not guaranteed or endorsed by the publisher.
Research integrity at Frontiers
Learn more about the work of our research integrity team to safeguard the quality of each article we publish.