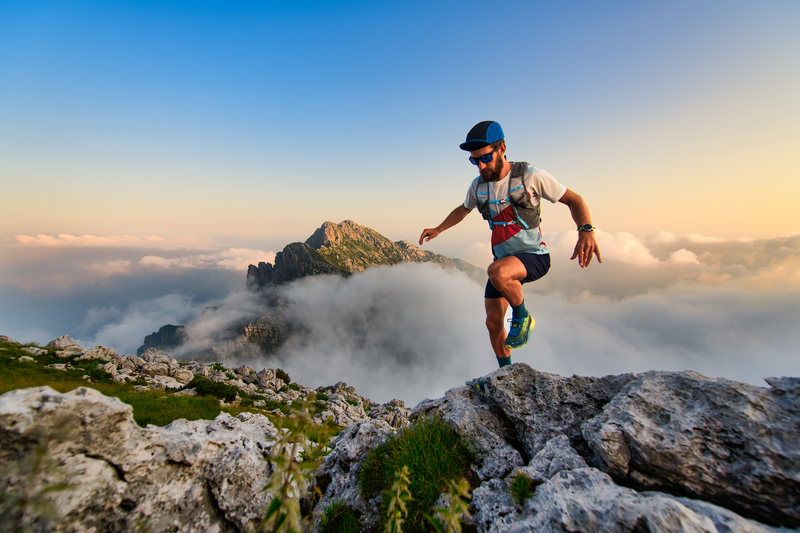
95% of researchers rate our articles as excellent or good
Learn more about the work of our research integrity team to safeguard the quality of each article we publish.
Find out more
ORIGINAL RESEARCH article
Front. Pharmacol. , 19 September 2024
Sec. Gastrointestinal and Hepatic Pharmacology
Volume 15 - 2024 | https://doi.org/10.3389/fphar.2024.1452201
Background: In this retrospective study, we aimed to identify key risk factors and establish an interpretable model for HCC with a diameter ≥ 5 cm using Lasso regression for effective risk stratification and clinical decision-making.
Methods: In this study, 843 patients with advanced hepatocellular carcinoma (HCC) and tumor diameter ≥ 5 cm were included. Using Lasso regression to screen multiple characteristic variables, cox proportional hazard regression and random survival forest models (RSF) were established. By comparing the area under the curve (AUC), the optimal model was selected. The model was visualized, and the order of interpretable importance was determined. Finally, risk stratification was established to identify patients at high risk.
Result: Lasso regression identified 8 factors as characteristic risk factors. Subsequent analysis revealed that the lasso-cox model had AUC values of 0.773, 0.758, and 0.799, while the lasso-RSF model had AUC values of 0.734, 0.695, and 0.741, respectively. Based on these results, the lasso-cox model was chosen as the superior model. Interpretability assessments using SHAP values indicated that the most significant characteristic risk factors, in descending order of importance, were tumor number, BCLC stage, alkaline phosphatase (ALP), ascites, albumin (ALB), and aspartate aminotransferase (AST). Additionally, through risk score stratification and subgroup analysis, it was observed that the median OS of the low-risk group was significantly better than that of the middle- and high-risk groups.
Conclusion: We have developed an interpretable predictive model for middle and late HCC with tumor diameter ≥ 5 cm using lasso-cox regression analysis. This model demonstrates excellent prediction performance and can be utilized for risk stratification.
Hepatocellular carcinoma (HCC), a leading malignancy globally, typically affects individuals with genetic susceptibility who have been exposed to risk factors. Notably, there are significant individual differences in HCC development. Currently, the etiology of HCC is gradually shifting from viral to non-viral origins (Toh et al., 2023).
According to NCCN, the treatment of HCC involves local and systemic therapies. Local regional therapy plays a leading role in the management of HCC in approximately 50%–60% of cases. Radiofrequency ablation (RFA) is the main method for early local treatment, while transarterial chemoembolization (TACE) remains the standard treatment for mid-stage HCC (Llovet et al., 2021). With increasing clinical applications, radiotherapy (RT) has emerged as a reliable and effective treatment option for HCC, depending on the disease severity and patient characteristics. For patients with resectable, single-small hepatitis B virus-associated HCC, there is a high risk of microvascular invasion (MVI), and the response rate to neoadjuvant radiotherapy is promising, with disease-free survival rates of 86.7%, 76.7%, and 60.0% in the first, second, and third years, respectively (Wei et al., 2023). In fact, large hepatocellular carcinoma> 5 cm traditionally requires large hepatectomy. Minor hepatectomy is usually performed with the aim of reducing morbidity and mortality. In NCCN guidelines, non-diffuse HCC with tumor diameter over 5 cm is an indication for external radiotherapy and TACE. Studies from Su suggest that external radiotherapy is better as the primary local treatment for HCC with tumor diameter over 5 cm, especially HCC with tumor diameter 5–10 cm. This discovery laid the foundation for future research on radiotherapy for HCC(4). Drawing from the achievements of the SHARP trial and IMbrave150 trial (Llovet et al., 2008; Liu et al., 2019; Finn et al., 2020), The systemic therapy for HCC has evolved from single-agent targeted therapy (such as sorafenib and lenvatinib) to combination therapy with checkpoint inhibitors (such as atezolizumab combined with bevacizumab) (Yang et al., 2023), and has been recognized by food and Drug Administration (FDA). The updated efficacy and safety data from the IMbrave150 trial demonstrate that the median overall survival (OS) in the atezolizumab combined with bevacizumab group was 19.2 months (95% CI, 17.0–23.7 months), compared to 13.4 months (95% CI, 11.4–16.9 months) in the sorafenib group (Cheng et al., 2022). Previous research has demonstrated that percutaneous acetic acid (PAAI) and RFA are equally effective in the treatment of small (≤5 cm) HCC. However, for HCC tumors ≥ 5 cm, RT and TACE are potential local treatment options. Nevertheless, the optimal local treatment for this tumor size remains controversial. According to Su’s study, the median overall survival (mOS) of the EBRT group was significantly longer than that of the TACE group before and after matching for HCC tumors ≥ 5 cm (14.9 vs 12.3 months, and 16.8 vs 11.4 months, respectively) (Su et al., 2023a).
The prognosis of HCC is complex due to the competing risks of potential cirrhosis and other malignancies. Chronic fibrotic liver disease due to viral or metabolic factors significantly raises HCC risk and often predicts a poorer prognosis (Fujiwara et al., 2018). HCC ranks fifth among cancers in Asia, serving as the second-largest cause of cancer-related deaths, with 72.5% of cases occurring there in 2020 (Zhang et al., 2022). Hepatocellular carcinoma (HCC), prevalent among liver cancers, dominates morbidity and mortality. Key prognostic factors for HCC encompass extrahepatic metastasis and liver function reserves, notably albumin and bilirubin levels (Ho et al., 2023; Schütte et al., 2020). Postoperative HAIC + FOLFOX enhanced DFS in MVI-HCC patients with tolerable side effects. External radiotherapy outcomes for MVI-HCC were unfavorable, but SBRT showed promise, especially in combination with sorafenib. Neoadjuvant radiotherapy yielded favorable responses with low toxicity in resectable, small HBV-HCCs (<5 cm) predicted to have high MVI risk (Wei et al., 2023; Munoz-Schuffenegger et al., 2021). In addition to tumor burden, liver function, and overall physical status, other factors such as tumor biopsy results, serum markers, and more, play significant roles in determining the prognosis of patients with HCC (Kulik and El-Serag, 2019). To establish an effective diagnosis and treatment model for HCC, it is essential to develop risk stratification algorithms and biomarkers to identify individuals at high risk. Additionally, advancements in imaging and blood-based monitoring tests are needed to improve the sensitivity and specificity of early HCC detection. Beyond clinical validation, data are necessary to assess clinical utility, aiming to enhance early tumor detection and ultimately reduce HCC-related mortality (Singal et al., 2023). The assessment of treatment response using the modified Solid Tumour Response Assessment Criteria (mRECIST) category indicated that mRECIST criteria and tumor size could effectively distinguish between patients with a high or low likelihood of recurrence following HCC transplantation (Kim et al., 2014). Cohort studies utilizing serum HCC markers have demonstrated that patients with HCC tumors ≥ 5 cm treated with different interventions exhibit variable survival outcomes. When all three HCC markers are positive, specific interventions can independently predict poor prognosis (Ueno et al., 2015).
In numerous HCC models, tumor size is recognized as a significant predictor of HCC patient prognosis. In some investigations, larger tumors have been associated with increased aggression and progression (Carr and Guerra, 2016; Yan et al., 2020). This means that patients with HCC tumors ≥ 5 cm often have advanced staging. Large HCC face poor prognosis due to expansion and tissue compression, often linked to delayed liver recovery and higher recurrence. Despite this, research on optimal predictive indices and interpretable ML models remains scarce. This study aims to innovatively address this gap, enhancing our understanding of survival risks in large HCCs through advanced machine learning methods. Currently, there is no established clinical predictive model for HCC tumors of this size, hence the development of an interpretable model for advanced patients with HCC tumors ≥ 5 cm undergoing comprehensive treatment (Pawlik et al., 2005).
The study included 843 patients from three tertiary hospitals in China from May 2017 to December 2023. Inclusion criteria include: a) patients with hepatocellular carcinoma diagnosed pathologically or clinically; b) patients who did not receive any antineoplastic therapy before admission; c) blood routine, biochemistry, electrolytes, and coagulation function measured within 7 days before registration; d) complete clinical data; e) tumordiameter ≥ 5 cm. We excluded patients with other types of malignant tumors. This study was approved by the affiliated Hospital of Ethics Committee of Southwest Medical University (KY2020254). The Ethics Committee abandoned the informed consent form because it was a retrospective study. The data were analyzed anonymously.
The collected patient baselines encompassed the following: 1) Serum laboratory indicators: white blood cell (WBC) count, alpha-fetoprotein (AFP), platelet count (PLT), and prothrombin time (PT). 2) Liver function markers: alanine aminotransferase (ALT), aspartate aminotransferase (AST), alkaline phosphatase (ALP), total bilirubin (TBIL), albumin (ALB), and Child-Pugh score. 3) Tumor burden: portal vein tumor thrombus (PVTT), ascites, extrahepatic metastasis, tumor number, and lymph node metastasis. 4) Tumor staging: Barcelona Clinic Liver Cancer (BCLC) staging system. 5) Additional data: gender, age, hepatitis B virus (HBV) status, diabetes mellitus, alcohol consumption, hypertension, and treatment options. OS was defined as the duration from the start of treatment until death or the last follow-up.
All data were analyzed by SPSS26.0 (SPSS, Chicago, Illinois, United States) and R4.2.2 software. Bilateral P < 0.05 is considered to be statistically significant. For classified variables (χ-2 test). Two predictive models (cox and Random Survival Forest (RSF)) were established, including Lasso regression to screen prognostic characteristics. Finally, the risk score is established based on nomogram, and the cutoff value is determined by X-tile tool; the mOS is estimated by Kaplan-Meier statistics, and the results are compared by Log-rank test. Lasso outperforms Ridge in feature selection occasionally, zeroing out unimportant coefficients via L1 regularization. Ideal for large, irregular feature sets, Lasso generates sparse models with few non-zero coefficients, simplifying and enhancing interpretability. This conciseness is crucial for clinical grafting in medical models, making Lasso the preferred choice.
Among the 843 patients included, there were 709 males, 411patients with HBV infection, 298patients with ascites diagnosed by imaging, 572patients with multiple tumors, 396patients with portal vein thrombosis and 240patients with BCLC stage B. 354 cases had lymph node metastasis, 183cases had distant metastasis. The specific baseline situation is shown in Table 1.
We enrolled patients from May 2017 to December 2023 and divided them into a training set and a verification set at a ratio of 7:3. The training set was utilized to screen features and establish models, utilizing lasso regression to determine the parameters. The variation characteristics of the coefficients of these variables are presented in Figure 1A. Iterative analysis was conducted using cross-validation (Figure 1B). When λ = min, the variables were included as feature variables. Among them, BCLC staging, tumor number, distant metastasis, ascites, age ≥60, aspartate aminotransferase ≥40 ug/L, albumin <35 g/L, alkaline phosphatase ≥125 ug/L were all identified as characteristic factors. Based on the features selected by lasso regression, a multi-factor Cox regression model (Figure 2) was further established. A nomogram visualizes our cox model (Figure 3A), and the correlation heat map shows the correlation between features (Figure 3B).
Figure 1. Variable Screening Based on Lasso Regression: (A) Characteristics of Variable Coefficients and (B) Cross-validation Procedure for Optimal λ Selection.
Figure 3. Construction of nomogram based on lasso-cox regression (A) and correlation of characteristic variables (B).
To further assess the specific impact of each feature variable as a risk factor on OS, we employed the global interpretation method to construct SurvSHAP. This approach quantifies the contribution of each feature to the model and identifies the prediction contribution of the model to a broader range of outcomes. Using SurvSHAP, we calculated the average SHAP value of each feature across all samples and presented the top six risk factors in lasso-cox (Figure 4).
Figure 4. SurvSHAP was used to calculate feature importance and rank the top six features selected by lasso-cox.
By using the features selected via lasso regression, we constructed the RSF model and visualized its feature importance ranking (Figures 5A, B). Through meticulous parameter tuning, we observed that when ntree is set to 200, the error rate of the model tends to stabilize.
Figure 5. Risk analysis of HCC recurrence based on random survival forest. (A) error rate of random survival forest; (B) importance ranking of variables.
The model is verified in the verification set. We establish the timeROC curve under the condition of lasso-cox and lasso-RSF respectively. For the lasso-cox model, the AUC values are 0.773, 0.758, and 0.799 for 1-year, 2-year, and 3-year predictions, respectively,for the lass-RSF model, the AUC values are 0.734, 0.695, and 0.741 for 1-year, 2-year, and 3-year predictions, respectively (Figures 6A, B).
We calculated the risk score for all patients based on the nomogram and utilized the X-tile software to identify the optimal risk stratification demarcation point for dividing the sample into low-risk, middle-risk, and high-risk groups (Supplementary Figure S1). Notably, distinct risk scores were associated with significantly different survival outcomes (Figure 7A).
Figure 7. Survival curve under risk stratification corresponding to overall cohort (A), training set (B), validation set (C), RT set (D), TACE set (E) or targeted combined immunotherapy set (F), respectively.
In the subgroup analysis, we compared survival outcomes across different risk categories between the training and validation datasets (Figures 7B, C). We also evaluated patients who RT,TACE, or targeted combined immunotherapy (TI) as part of their treatment, not only those who received these specific therapies. This enabled us to assess survival disparities among patients at high, medium, and low risk within these three groups (Figures 7D–F).
The survival prognosis for patients with HCC is bleak; however, tumor progression can be monitored by tracking characteristic risk factors. Improving the identification of tumor-specific characteristics can facilitate the implementation of radical treatment (Hong et al., 2018). Since the last official update of BCLC’s HCC prognosis and treatment strategy in 2018, significant progress has been made in the field of HCC treatment. However, the data available for selecting interventions remains insufficiently mature to be included in evidence-based models used by clinicians and researchers. To address this issue, it is imperative to establish models that can determine different clinical prognoses based on various characteristics (Reig et al., 2022).
The study enrolled 843 patients and developed a nomogram, utilizing lasso regression to screen several variables. This established model comprises eight indices for clinical application: BCLC staging, tumor number, presence of distant metastasis, ascites, age ≥60 years, aspartate aminotransferase ≥40 ug/L, albumin <35 g/L, and alkaline phosphatase ≥125 ug/L. Drawing from the characteristic factors identified by Lasso screening, we developed cox and RSF models and compared them in terms of receiver operating characteristic (ROC) and c-index values. We also ranked feature importance using SHAP value or variable importance in projection (VIMP) in these two models. The prediction model based on lasso-cox regression exhibited good performance in predicting 1-, 2-, and 3-year survival. Finally, patients were clearly stratified into three risk groups based on survival, revealing significant survival benefits in the low-risk group compared with the high-risk group.
The BCLC staging system provides clear guidance for prognosis and treatment. Stage B includes asymptomatic patients with multinodular HCC, while Stage C includes patients with symptomatic or invasive tumors. Patients in Stages B and C may potentially benefit from novel treatment approaches investigated in Phase II studies or randomized controlled trials. The prognosis for Stage D patients is exceedingly poor, and the treatment options are more limited (Llovet et al., 1999). The study of tumor diversity has revealed that the genomic characteristics of liver cancer in some patients cannot be fully captured through sequencing individual lesions. Comparative genomic analysis of multiple lesions associated with liver cancer offers valuable insights into the genetic alterations linked to tumor progression. This suggests that a higher number of tumor lesions may carry more prognostic information, emphasizing the importance of studying large tumors (≥5 cm) or multiple tumors (Xue et al., 2016; Lackner et al., 2019). HCC patients with significant ascites exhibited signs of peritoneal infiltration, positive cytological examination results, and elevated fibronectin levels (Martins et al., 2006). Tumor-associated ascites can significantly impact the baseline liver function, and ascites volumes exceeding 500 mL have a notable impact on liver reserve and tumor status (Zhang et al., 2009), Therefore, it can serve as a predictor of survival prior to treatment. Additionally, numerous studies have established tumor number, ascites, age, and distant metastasis as independent risk factors for tumor prognosis, a fact that is widely accepted (Nouso et al., 2010). AST and ALT, primarily in liver cells, elevate in serum upon cell damage. Highly sensitive to liver injury, they’re key indicators for assessing liver health and diagnosing disease. In HCC, their rising levels mirror liver cell deterioration, reflecting disease progression.
Studies have demonstrated that the change in ALP levels is an independent prognostic factor in HCC following partial hepatectomy. ALP elevation serves as a functional biomarker of liver function, rather than a specific biomarker for HCC. The higher regenerative capacity may be associated with the increase in ALP levels postoperatively (Huang et al., 2022). Furthermore, the nomogram utilizing lactate dehydrogenase (LDH) and ALP demonstrates good predictive accuracy for HCC. For HCC patients with elevated ALP or ALP/LDH levels, close monitoring and consideration of adjuvant treatment should be considered (Su et al., 2023b). Wu’s study also revealed that ALP levels can predict the prognosis of patients with HBV-related HCC following radical hepatectomy (Wu et al., 2016). Monitoring these enzymes aids in understanding HCC status and optimizing treatment. ALP, ubiquitous in liver, bone, gut, kidney, and placenta, is an enzyme expelled by the liver to the gallbladder. Elevated serum ALP often signals hepatobiliary disorders, as liver is its primary source in blood.
The Lasso-cox regression seamlessly integrates the strengths of both lasso and cox regressions, ensuring precision in high-dimensional data analysis. By leveraging lasso’s variable selection, the model eliminates unnecessary variables and retains crucial predictors of survival time, yielding a concise yet powerful cox model. This refined approach consistently delivers enhanced accuracy in predicting individual survival durations. Lasso regression mitigates the issue of multi-collinearity among variables, and variable screening significantly reduces overfitting. This study demonstrates that the RSF model exhibits inferior AUC values compared to the Lasso-Cox model. Therefore, a nomogram based on the Lasso-Cox regression model is developed, enhancing the explanation of feature importance using SHAP values. This offers medical professionals valuable insights for intuitive analysis of individual prognostic risks, facilitating the identification of high-risk patients in clinical settings. However, there are limitations to this study. Firstly, the study focuses on HCCs ≥5 cm; future research should explore HCC subtypes between 5 and 10 cm and ≥10 cm. Secondly, more centers are needed to verify the generalizability of external validation datasets. Lastly, future multicenter studies with larger sample sizes are necessary to validate and disseminate these findings in a larger population.
We have developed an interpretable predictive model for middle and late HCC with tumor diameter ≥ 5 cm using lasso-cox regression analysis. This model demonstrates excellent prediction performance and can be utilized for risk stratification.
The raw data supporting the conclusions of this article will be made available by the authors, without undue reservation.
The studies involving humans were approved by the affiliated Hospital of Ethics Committee of Southwest Medical University. The studies were conducted in accordance with the local legislation and institutional requirements. Written informed consent for participation was not required from the participants or the participants legal guardians/next of kin in accordance with the national legislation and institutional requirements. Written informed consent was not obtained from the individual(s) for the publication of any potentially identifiable images or data included in this article because The Ethics Committee abandoned the informed consent form because it was a retrospective study.
HL: Conceptualization, Investigation, Methodology, Validation, Visualization, Writing–original draft. BY: Conceptualization, Project administration, Writing–original draft. CW: Investigation, Supervision, Writing–review and editing. BL: Conceptualization, Data curation, Writing–review and editing. LH: Conceptualization, Data curation, Writing–review and editing. YJ: Conceptualization, Formal Analysis, Writing–review and editing. YS: Data curation, Methodology, Supervision, Writing–review and editing. LW: Conceptualization, Data curation, Writing–review and editing. MR: Data curation, Software, Writing–review and editing. JZ: Conceptualization, Formal Analysis, Writing–review and editing. XL: Data curation, Supervision, Writing–review and editing. KH: Conceptualization, Data curation, Writing–review and editing. YH: Conceptualization, Writing–original draft, Writing–review and editing.
The author(s) declare that financial support was received for the research, authorship, and/or publication of this article. This work was supported by the planned project of Luzhou Science and Technology Bureau (2022-JYJ-123).
The authors declare that the research was conducted in the absence of any commercial or financial relationships that could be construed as a potential conflict of interest.
All claims expressed in this article are solely those of the authors and do not necessarily represent those of their affiliated organizations, or those of the publisher, the editors and the reviewers. Any product that may be evaluated in this article, or claim that may be made by its manufacturer, is not guaranteed or endorsed by the publisher.
The Supplementary Material for this article can be found online at: https://www.frontiersin.org/articles/10.3389/fphar.2024.1452201/full#supplementary-material
Carr, B. I., and Guerra, V. (2016). Hepatocellular carcinoma extrahepatic metastasis in relation to tumor size and alkaline phosphatase levels. Oncology 90 (3), 136–142. doi:10.1159/000443480
Cheng, A. L., Qin, S., Ikeda, M., Galle, P. R., Ducreux, M., Kim, T. Y., et al. (2022). Updated efficacy and safety data from IMbrave150: atezolizumab plus bevacizumab vs. sorafenib for unresectable hepatocellular carcinoma. J. Hepatol. 76 (4), 862–873. doi:10.1016/j.jhep.2021.11.030
Finn, R. S., Qin, S., Ikeda, M., Galle, P. R., Ducreux, M., Kim, T. Y., et al. (2020). Atezolizumab plus bevacizumab in unresectable hepatocellular carcinoma. N. Engl. J. Med. 382 (20), 1894–1905. doi:10.1056/NEJMoa1915745
Fujiwara, N., Friedman, S. L., Goossens, N., and Hoshida, Y. (2018). Risk factors and prevention of hepatocellular carcinoma in the era of precision medicine. J. Hepatol. 68 (3), 526–549. doi:10.1016/j.jhep.2017.09.016
Ho, S. Y., Liu, P. H., Hsu, C. Y., Tseng, H. T., Huang, Y. H., Su, C. W., et al. (2023). Albumin-based liver reserve models vs. MELD 3.0 in prognostic prediction for hepatocellular carcinoma patients with renal insufficiency. Int. J. Mol. Sci. 24 (23), 16987. doi:10.3390/ijms242316987
Hong, T. P., Gow, P. J., Fink, M., Dev, A., Roberts, S. K., Nicoll, A., et al. (2018). Surveillance improves survival of patients with hepatocellular carcinoma: a prospective population-based study. Med. J. Aust. 209 (8), 348–354. doi:10.5694/mja18.00373
Huang, C. W., Wu, T. H., Hsu, H. Y., Pan, K. T., Lee, C. W., Chong, S. W., et al. (2022). Reappraisal of the role of alkaline phosphatase in hepatocellular carcinoma. J. Pers. Med. 12 (4), 518. doi:10.3390/jpm12040518
Kim, D. J., Clark, P. J., Heimbach, J., Rosen, C., Sanchez, W., Watt, K., et al. (2014). Recurrence of hepatocellular carcinoma: importance of mRECIST response to chemoembolization and tumor size. Am. J. Transpl. 14 (6), 1383–1390. doi:10.1111/ajt.12684
Kulik, L., and El-Serag, H. B. (2019). Epidemiology and management of hepatocellular carcinoma. Gastroenterology 156 (2), 477–491. doi:10.1053/j.gastro.2018.08.065
Lackner, C., Quagliata, L., Cross, W., Ribi, S., Heinimann, K., Paradiso, V., et al. (2019). Convergent evolution of copy number alterations in multi-centric hepatocellular carcinoma. Sci. Rep. 9 (1), 4611. doi:10.1038/s41598-019-40843-9
Liu, Z., Lin, Y., Zhang, J., Zhang, Y., Li, Y., Liu, Z., et al. (2019). Molecular targeted and immune checkpoint therapy for advanced hepatocellular carcinoma. J. Exp. Clin. Cancer Res. 38 (1), 447. doi:10.1186/s13046-019-1412-8
Llovet, J. M., Brú, C., and Bruix, J. (1999). Prognosis of hepatocellular carcinoma: the BCLC staging classification. Semin. Liver Dis. 19 (3), 329–338. doi:10.1055/s-2007-1007122
Llovet, J. M., De Baere, T., Kulik, L., Haber, P. K., Greten, T. F., Meyer, T., et al. (2021). Locoregional therapies in the era of molecular and immune treatments for hepatocellular carcinoma. Nat. Rev. Gastroenterol. Hepatol. 18 (5), 293–313. doi:10.1038/s41575-020-00395-0
Llovet, J. M., Ricci, S., Mazzaferro, V., Hilgard, P., Gane, E., Blanc, J. F., et al. (2008). Sorafenib in advanced hepatocellular carcinoma. N. Engl. J. Med. 359 (4), 378–390. doi:10.1056/NEJMoa0708857
Martins, A., Cortez-Pinto, H., Marques-Vidal, P., Mendes, N., Silva, S., Fatela, N., et al. (2006). Treatment and prognostic factors in patients with hepatocellular carcinoma. Liver Int. 26 (6), 680–687. doi:10.1111/j.1478-3231.2006.001285.x
Munoz-Schuffenegger, P., Barry, A., Atenafu, E. G., Kim, J., Brierley, J., Ringash, J., et al. (2021). Stereotactic body radiation therapy for hepatocellular carcinoma with Macrovascular invasion. Radiother. Oncol. 156, 120–126. doi:10.1016/j.radonc.2020.11.033
Nouso, K., Kobayashi, Y., Nakamura, S., Kobayashi, S., Toshimori, J., Kuwaki, K., et al. (2010). Evolution of prognostic factors in hepatocellular carcinoma in Japan. Aliment. Pharmacol. Ther. 31 (3), 407–414. doi:10.1111/j.1365-2036.2009.04179.x
Pawlik, T. M., Delman, K. A., Vauthey, J. N., Nagorney, D. M., Ng, I. O., Ikai, I., et al. (2005). Tumor size predicts vascular invasion and histologic grade: implications for selection of surgical treatment for hepatocellular carcinoma. Liver Transpl. 11 (9), 1086–1092. doi:10.1002/lt.20472
Reig, M., Forner, A., Rimola, J., Ferrer-Fàbrega, J., Burrel, M., Garcia-Criado, Á., et al. (2022). BCLC strategy for prognosis prediction and treatment recommendation: the 2022 update. J. Hepatol. 76 (3), 681–693. doi:10.1016/j.jhep.2021.11.018
Schütte, K., Schinner, R., Fabritius, M. P., Möller, M., Kuhl, C., Iezzi, R., et al. (2020). Impact of extrahepatic metastases on overall survival in patients with advanced liver dominant hepatocellular carcinoma: a subanalysis of the soramic trial. Liver Cancer 9 (6), 771–786. doi:10.1159/000510798
Singal, A. G., Sanduzzi-Zamparelli, M., Nahon, P., Ronot, M., Hoshida, Y., Rich, N., et al. (2023). International Liver Cancer Association (ILCA) white paper on hepatocellular carcinoma risk stratification and surveillance. J. Hepatol. 79 (1), 226–239. doi:10.1016/j.jhep.2023.02.022
Su, K., Huang, W., Li, X., Xu, K., Gu, T., Liu, Y., et al. (2023b). Evaluation of lactate dehydrogenase and alkaline phosphatase as predictive biomarkers in the prognosis of hepatocellular carcinoma and development of a new nomogram. J. Hepatocell. Carcinoma 10, 69–79. doi:10.2147/JHC.S398632
Su, K., Wang, F., Li, X., Chi, H., Zhang, J., He, K., et al. (2023a). Effect of external beam radiation therapy versus transcatheter arterial chemoembolization for non-diffuse hepatocellular carcinoma (≥5 cm): a multicenter experience over a ten-year period. Front. Immunol. 14, 1265959. doi:10.3389/fimmu.2023.1265959
Toh, M. R., Wong, E. Y. T., Wong, S. H., Ng, A. W. T., Loo, L. H., Chow, P. K., et al. (2023). Global epidemiology and genetics of hepatocellular carcinoma. Gastroenterology 164 (5), 766–782. doi:10.1053/j.gastro.2023.01.033
Ueno, M., Hayami, S., Shigekawa, Y., Kawai, M., Hirono, S., Okada, K., et al. (2015). Prognostic impact of surgery and radiofrequency ablation on single nodular HCC ⩽5 cm: cohort study based on serum HCC markers. J. Hepatol. 63 (6), 1352–1359. doi:10.1016/j.jhep.2015.07.013
Wei, X., Jiang, Y., Feng, S., Lu, C., Huo, L., Zhou, B., et al. (2023). Neoadjuvant intensity modulated radiotherapy for a single and small (≤5 cm) hepatitis B virus-related hepatocellular carcinoma predicted to have high risks of microvascular invasion: a randomized clinical trial. Int. J. Surg. 109 (10), 3052–3060. doi:10.1097/JS9.0000000000000574
Wu, S. J., Lin, Y. X., Ye, H., Xiong, X. Z., Li, F. Y., and Cheng, N. S. (2016). Prognostic value of alkaline phosphatase, gamma-glutamyl transpeptidase and lactate dehydrogenase in hepatocellular carcinoma patients treated with liver resection. Int. J. Surg. 36 (Pt A), 143–151. doi:10.1016/j.ijsu.2016.10.033
Xue, R., Li, R., Guo, H., Guo, L., Su, Z., Ni, X., et al. (2016). Variable intra-tumor genomic heterogeneity of multiple lesions in patients with hepatocellular carcinoma. Gastroenterology 150 (4), 998–1008. doi:10.1053/j.gastro.2015.12.033
Yan, B., Bai, D. S., Zhang, C., Qian, J. J., Jin, S. J., and Jiang, G. Q. (2020). Characteristics and risk differences of different tumor sizes on distant metastases of hepatocellular carcinoma: a retrospective cohort study in the SEER database. Int. J. Surg. 80, 94–100. doi:10.1016/j.ijsu.2020.06.018
Yang, C., Zhang, H., Zhang, L., Zhu, A. X., Bernards, R., Qin, W., et al. (2023). Evolving therapeutic landscape of advanced hepatocellular carcinoma. Nat. Rev. Gastroenterol. Hepatol. 20 (4), 203–222. doi:10.1038/s41575-022-00704-9
Zhang, C. H., Cheng, Y., Zhang, S., Fan, J., and Gao, Q. (2022). Changing epidemiology of hepatocellular carcinoma in Asia. Liver Int. 42 (9), 2029–2041. doi:10.1111/liv.15251
Keywords: LASSO-COX, nomogram, interpretable, hepatocellular carcinoma, radiotherapy
Citation: Li H, Yang B, Wang C, Li B, Han L, Jiang Y, Song Y, Wen L, Rao M, Zhang J, Li X, He K and Han Y (2024) Construction of an interpretable model for predicting survival outcomes in patients with middle to advanced hepatocellular carcinoma (≥5 cm) using lasso-cox regression. Front. Pharmacol. 15:1452201. doi: 10.3389/fphar.2024.1452201
Received: 20 June 2024; Accepted: 04 September 2024;
Published: 19 September 2024.
Edited by:
David Sacerdoti, University of Verona, ItalyReviewed by:
Shivani Srivastava, Yale University, United StatesCopyright © 2024 Li, Yang, Wang, Li, Han, Jiang, Song, Wen, Rao, Zhang, Li, He and Han. This is an open-access article distributed under the terms of the Creative Commons Attribution License (CC BY). The use, distribution or reproduction in other forums is permitted, provided the original author(s) and the copyright owner(s) are credited and that the original publication in this journal is cited, in accordance with accepted academic practice. No use, distribution or reproduction is permitted which does not comply with these terms.
*Correspondence: Kun He, aGt0b25namlAc3dtdS5lZHUuY24=; Yunwei Han, bGFucGFveGlhbnNoZW5nQDEyNi5jb20=
†These authors have contributed equally to this work
Disclaimer: All claims expressed in this article are solely those of the authors and do not necessarily represent those of their affiliated organizations, or those of the publisher, the editors and the reviewers. Any product that may be evaluated in this article or claim that may be made by its manufacturer is not guaranteed or endorsed by the publisher.
Research integrity at Frontiers
Learn more about the work of our research integrity team to safeguard the quality of each article we publish.