- Department of General Surgery, Qilu Hospital, Cheeloo College of Medicine, Shandong University, Jinan, China
Background: Hepatocellular carcinoma (HCC) is the most common primary liver cancer and often arises in the context of chronic liver disease, such as hepatitis B or C infection, and cirrhosis. Advanced unresectable HCC (uHCC) presents significant treatment challenges due to its advanced stage and inoperability. One efficient treatment method for advanced uHCC is the use of hepatic arterial infusion chemotherapy (HAIC) combined with transcatheter arterial embolization (TAE).
Patients and Methods: In this study, we conducted a retrospective collection of clinical data, including basic information, radiological data, and blood test parameters, for patients with advanced uHCC who underwent TAE + HAIC treatment from August 2020 to February 2023. A total of 743 cases involving 262 patients were included. Ultimately, the covariates included in the analysis were the Child-Pugh score, extrahepatic metastasis, tumor number, tumor size, and treatment method.
Results: In the study, we performed univariable and multivariable analysis on 23 clinical factors that were screened by LASSO regression, indicating that the five variables aforementionedly were identified as independent factors influencing patient prognosis. Then we developed a nomogram of the sensitive model and calculated concordance indices of prognostic survival models.
Conclusion: Based on the uHCC patient cohort, we have developed a prognostic model for OS in patients who received TAE + HAIC treatment. This model can accurately predict OS and has the potential to assist in personalized clinical decision-making.
Highlights
The prognostic model for OS has been developed specifically for patients with advanced uHCC who have received TAE + HAIC.
The model has the potential to assist in personalized clinical decision-making and prognosis assessment, providing a better net benefit than CNLC or BCLC tumor staging.
Introduction
Malignant hepatic tumors refer to a group of liver cancers. According to the origin of the tumor, malignant hepatic tumors can be divided into primary cancers and metastatic tumors (Shi and Line, 2014; Sunnapwar et al., 2016; Esparza-Baquer et al., 2021). Hepatocellular carcinoma (HCC) is the most common primary malignancy of the liver (Perugorria et al., 2019; An et al., 2022). Advanced unresectable HCC (uHCC) was characterized by strong tumor invasiveness, limited treatment options, a very high patient mortality rate, and an inferior prognosis (Wang et al., 2022; Shi et al., 2024), and the 1-year survival rate for patients is approximately 20% (Gravitz, 2014). Patients with advanced uHCC cannot undergo radical resection treatment due to the challenging surgery and high mortality associated with the disease (Sangro et al., 2012; Galle et al., 2017), and one efficient treatment method for advanced uHCC is the use of TAE combined with HAIC (Chen et al., 2021; An et al., 2022).
TAE + HAIC is an interventional radiology procedure in which chemotherapy drugs and embolic agents are delivered directly into the main blood supply of the advanced uHCC, using a catheter. TAE + HAIC has shown promise in treating advanced uHCC, particularly in cases where the tumor is confined to the hepatic and has not spread extensively to other parts of the body (Lyu et al., 2022).
For advanced uHCC, radical surgical resection can not be performed to obtain visualized tumor data, and it is difficult to conduct predictive analysis based on the Barcelona Clinic Liver Cancer (BCLC) staging system (Chun et al., 2018; Banales et al., 2020). A novel prognostic assessment system needs to be established for predicting the prognosis of patients with advanced uHCC.
In this study, we developed a nomogram of the sensitive model and calculated concordance indices of prognostic survival models. This model, incorporating variables derived from univariable and multivariable regression analyses, can accurately predict the OS of patients with advanced uHCC who have undergone TAE + HAIC. The model has the potential to assist in personalized clinical decision-making and prognosis assessment.
Materials and methods
Patients
Clinical data of 262 patients with advanced uHCC who underwent combined therapy from 2020.08 to 2023.02 in Qilu Hospital of Shandong University, were retrospectively collected. HCC was diagnosed according to the guidelines of the American Association for the Study of Liver Diseases and the European Association for the Study of the Liver. The inclusion criteria were as follows: 1) age 18–80 years; 2) Child-Pugh class A or B liver function; 3) Eastern Cooperative Oncology Group performance score ≤1; 4) lack of preoperative adjuvant treatments for HCC; 5) no presence of other malignancies; 6) availability of complete clinical and follow-up data.
Follow-up
To ensure the accuracy of survival data, follow-up assessments of patients were scheduled every 3 months. All patients were reviewed at the last follow-up in June 2023. Overall survival (OS) was defined as the duration from the initial treatment to either the patient’s death or the last follow-up.
Cohort definition
Patients were randomly divided into training and validation cohorts in a 7:3 ratio to ensure an even distribution of outcome events. The demographic characteristics, laboratory data, and radiological data of patients in the two cohorts were comparable. The training cohort was used to screen variables and construct the nomogram, while the validation cohort was used to validate the results.
Variable selection
As described herein, a total of 23 variables were included in the selection process. To minimize the potential collinearity and over-fitting of variables, the Least Absolute Shrinkage and Selection Operator (LASSO) regression was applied. The variables identified by LASSO regression were then entered into Univariate Cox regression analysis. Relevant variables with a p-value of less than 0.10 were selected as inputs for further analysis. Forward-backward stepwise selection based on the Akaike information criterion (AIC) was used to evaluate variables for the multivariate COX regression model (Collins et al., 2015). Hazard ratios and their corresponding 95% confidence intervals (CIs) were calculated.
Development and validation of nomogram
Significant variables were incorporated into a nomogram to predict the 6-, 12-, and 18-month OS rates after initial treatment. Calibration curves were utilized to assess the agreement between predicted survival probabilities and actual survival proportions at each time point (6, 12, 18 months). Discriminative ability was evaluated using the Concordance index (C-index) and area under the time-dependent receiver operating characteristic curve (time-dependent ROC). Additionally, decision curve analysis was conducted to determine the clinical utility of the nomogram by quantifying the net benefits at different threshold probabilities compared with CNLC or BCLC tumor staging (Vickers et al., 2008).
Statistical analysis
All statistical analysis was conducted using R version 4.3.1 (R Foundation for Statistical Computing). Continuous variables are presented as the mean ± standard deviation or the median with interquartile range (IQR). Categorical variables were presented as frequencies and proportions. Survival curves were generated using the Kaplan-Meier method, and the log-rank test was employed to compare curves. A two-tailed p-value of less than 0.05 was considered statistically significant.
Results
Baseline characteristics of the cohort
A total of 743 cases involving 262 patients with advanced uHCC who have undergone TAE + HAIC were included in this analysis, and 41 patients were excluded primarily due to loss of follow-up and missing clinical data, of which 23 (8.8%) were lost of follow-up. The patients were divided into the training and validation cohorts with a ratio of 7:3 to ensure that outcome events were distributed randomly between the two cohorts (Figure 1A). In an overall perspective, The median overall survival (OS) for 63 patients treated with TAE + HAIC was 14.0 months (95% CI: 11.4–16.2). For 44 patients treated with TAE + HAIC followed by immunotherapy or targeted therapy, the median OS was 19.0 months (95% CI: 11.1–26.9). For 114 patients treated with TAE + HAIC followed by Immune-targeted therapy, the OS rate exceeded 50% at 30 months (Figure 1B). The baseline clinicopathological characteristics of advanced uHCC were obtained from medical records. These clinical factors will be further screened through LASSO regression and then included in Cox regression as foundational factors for developing the nomogram model (Table 1).
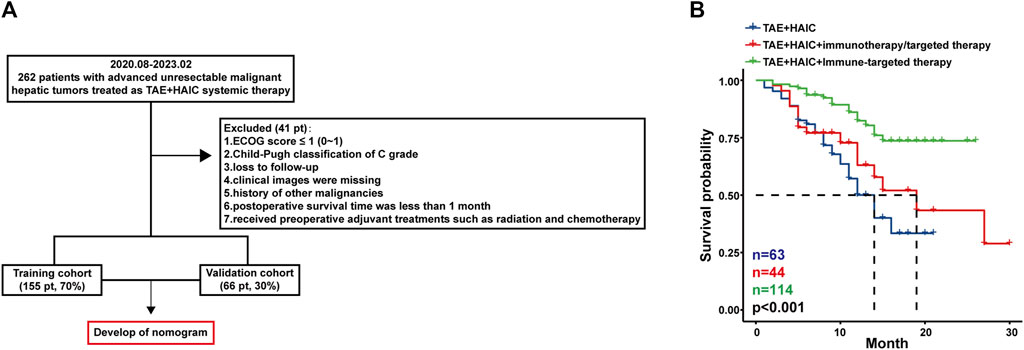
Figure 1. The patient flow of the study. Pt, patients. Flow chart (A) and survival curves (B) of the cohort in patients with advanced uHCC.
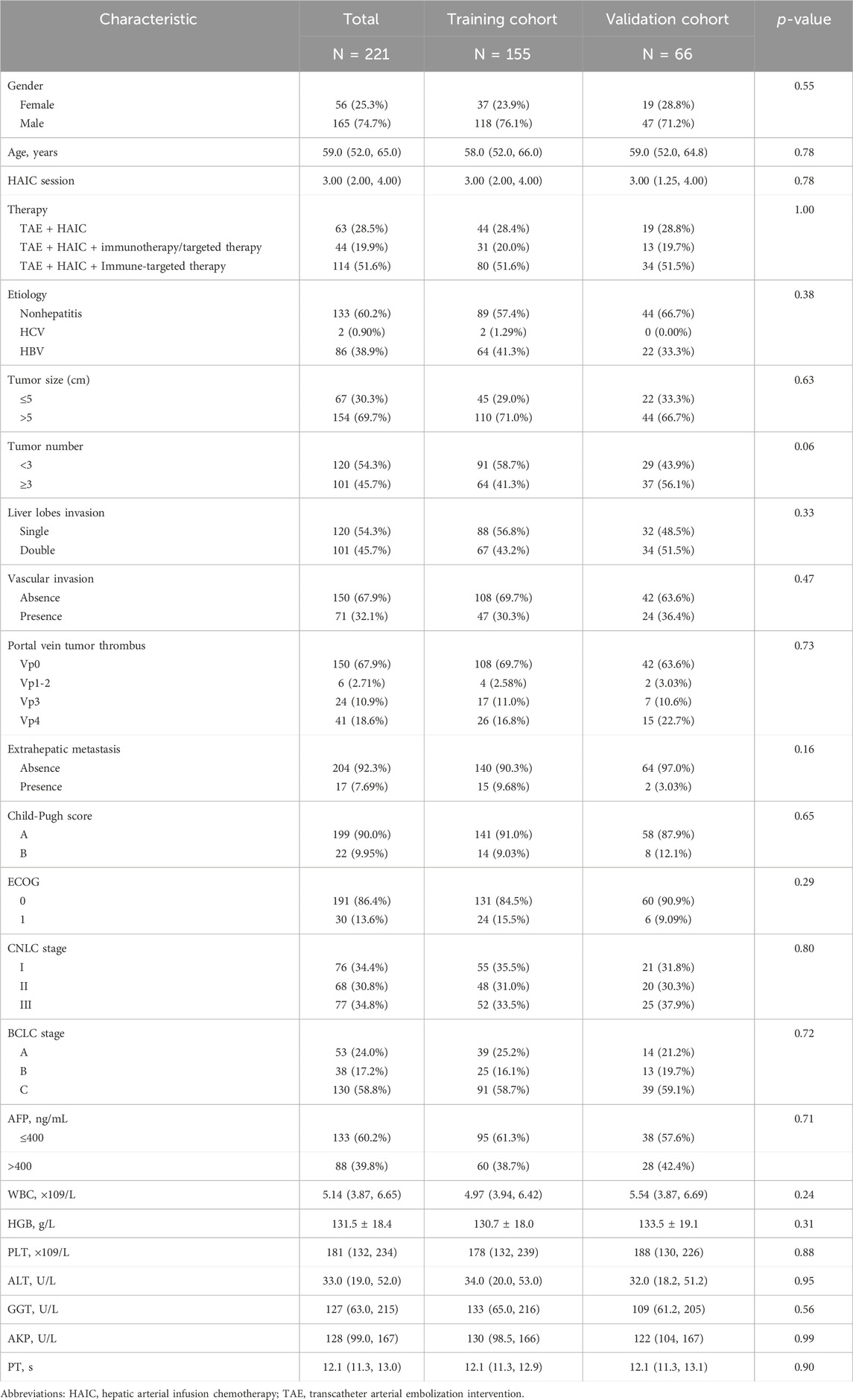
Table 1. Clinicopathological characteristics of patients with advanced unresectable hepatocellular carcinoma who underwent TAE + HAIC.
Regression analysis and nomogram construction in the advanced uHCC cohort
As described herein, we performed LASSO regression to screen the collected 23 variables and calculated the regression coefficients for each clinical factor (Figure 2A). Nine resolving variables were selected for further analysis, including Child-Pugh score, extrahepatic metastasis, tumor number, tumor size, and treatment method, etiology, portal vein tumor thrombus, age, HAIC session (Figure 2B). After conducting univariable and multivariable analysis on the selected nine clinical factors, we included variables that exhibited a significant association with OS to identify independent predictors of OS (Table 2).
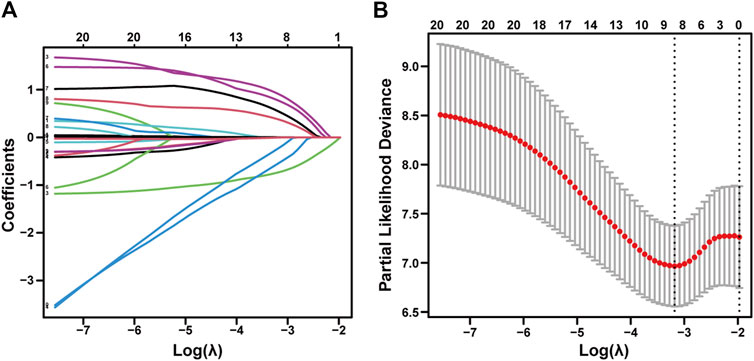
Figure 2. LASSO regression of advanced uHCC cohort. Feature selection using the least absolute shrinkage and selection operator. (A) LASSO coefficient profiles of the 23 baseline features. (B) The selection process of the optimum value of the parameter λ in the Lasso regression model by a 10-fold cross-validation method.
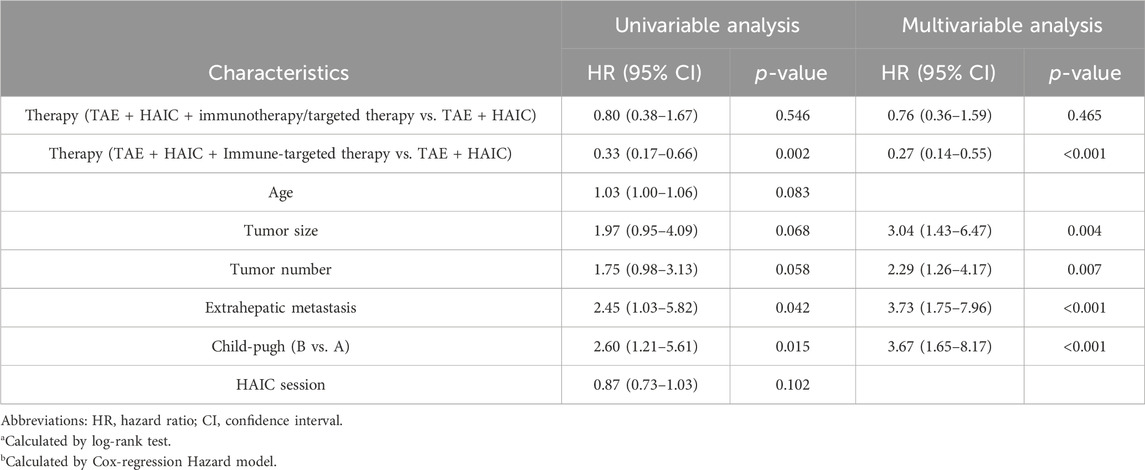
Table 2. Cox regression analysis of predictive factors for overall survival in patients with advanced unresectable hepatocellular carcinoma.
Subsequently, we developed nomograms using the outcomes of the multivariable analysis, aiming to forecast 6-month, 12-month, and 18-month OS after interventional surgery involving HAIC plus TAE for advanced uHCC (Figure 3). A higher total score was correlated with poorer OS, and the nomogram demonstrated a concordance index (C-index) of 0.74 (training cohort, 95% CI: 0.66–0.82) and 0.76 (validation cohort, 95% CI: 0.64–0.89) for OS prediction.
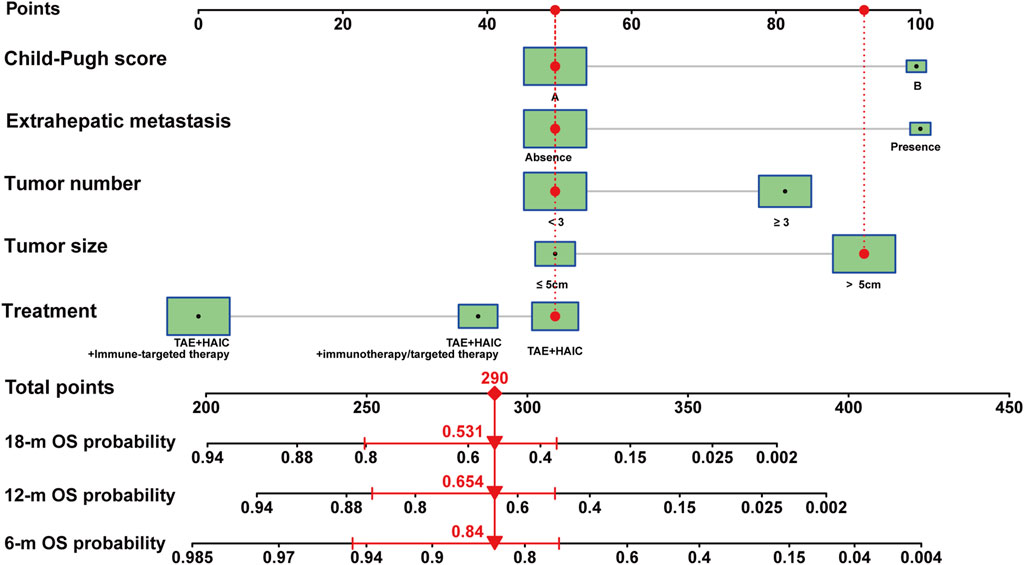
Figure 3. Nomogram model of advanced uHCC cohort. Nomogram for prognostic prediction of a patient with advanced uHCC. The patient had a single, local tumor of 6.1 cm, with liver function is Child-pugh A, without extrahepatic metastasis, and underwent HAIC therapy. For category variables, their distributions are reflected by the size of the box. The importance of each variable was ranked according to the standard deviation along nomogram scales. To use the nomogram, the specific points (black dots) of individual patients are located on each variable axis. Red lines and dots are drawn upward to determine the points received by each variable; the sum (290) of these points is located on the Total Points axis, and a line is drawn downward to the survival axes to determine the probability of 6- month (84.0%), 12-month (65.4%) and 18-month (53.1%) overall survival.
Performance analysis of nomogram
To assess the performance of the nomogram model, calibration curves for 6-month, 12-month, and 18-month were generated, which showed strong concordance between the predicted and observed probabilities of OS (Figure 4A). In addition, AUC curves for training and validation cohorts were generated for OS status, considering cumulative sensitivity (Figure 4B).
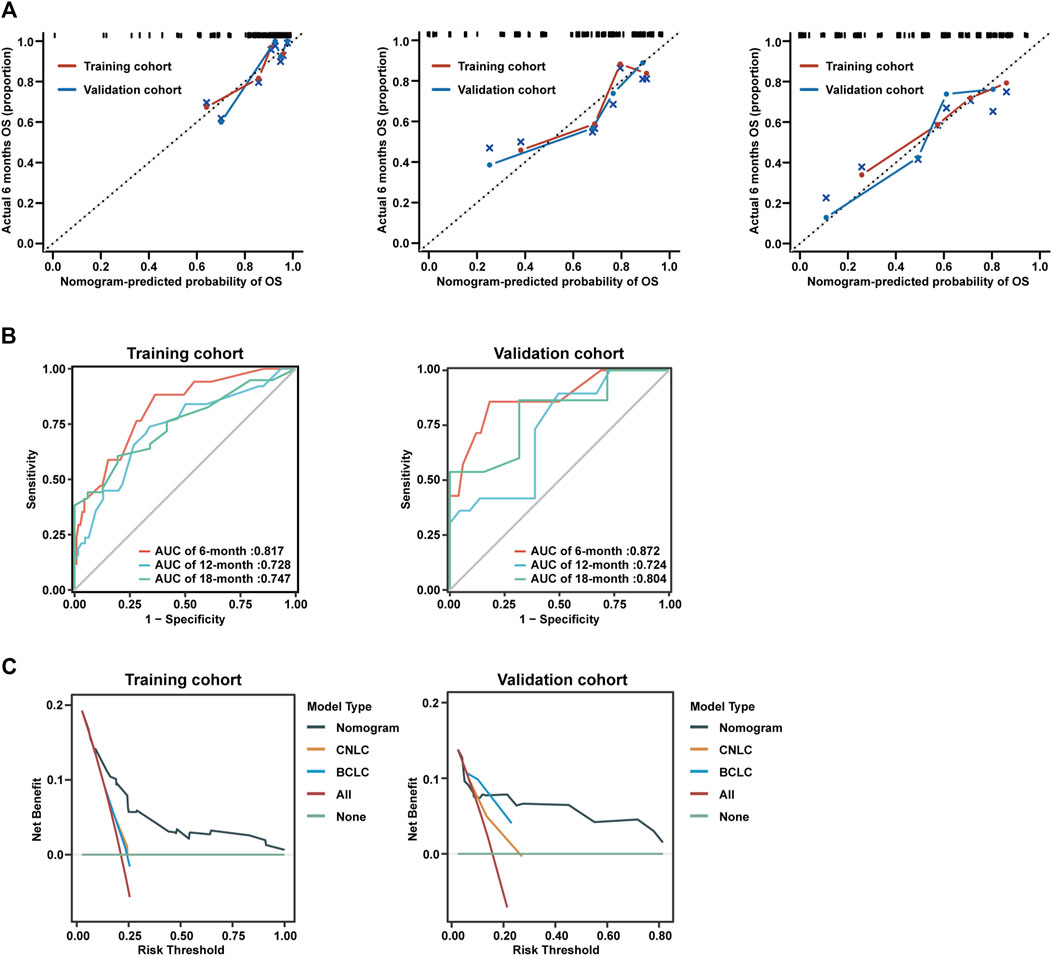
Figure 4. Calibration curves and decision curve analysis of nomogram model. (A) Calibration curves of 6-month (left), 12-month (middle), and 18-month (right) OS for uHCC patients in the training cohort and validation cohort. (B) AUC of using the nomogram to predict overall survival probability in the training cohort and validation cohorts. (C) Decision curve analysis of the nomogram, CNLC tumor staging, and BCLC tumor staging for the survival prediction of patients with advanced uHCC.
To evaluate the prognostic performance and clinical utility, we conducted a comparative analysis of the nomogram model and CNLC or BCLC tumor staging using Decision Curve Analysis (DCA). The findings indicated that the nomogram model consistently provided a superior net benefit compared to CNLC or BCLC tumor staging across various thresholds for advanced uHCC (Figure 4C).
Discussion
Advanced uHCC exhibited high tumor heterogeneity, poor prognosis, and an exceedingly high treatment risk (Wang et al., 2022). As the primary treatment approach for advanced uHCC (Zhou and Song, 2021), the effectiveness and postoperative prognosis prediction model of interventional therapy should be studied.
Advanced uHCC typically has a plentiful blood supply, but embolization can help reduce the supply to the tumor. White ball embolization can also increase the duration that chemotherapy drugs interact with the tumor, ultimately improving their effectiveness. While there was a consensus that TAE + HAIC provided benefits to patients with advanced uHCC (Forner et al., 2018; Yang et al., 2023), there has been no research on the construction of a predictive model for the postoperative prognosis of TAE + HAIC-treated patients. In consideration of this circumstance, we conducted a retrospective data collection and established a cohort comprising patients with advanced uHCC who underwent TAE + HAIC intervention surgery, subsequently tracking their survival data. Through LASSO regression screening and logistic regression analysis of the gathered clinical data, we once again validated that specific clinical factors act as independent risk factors for patient prognosis.
Accurate prognostic models are crucial for forecasting patient outcomes, guiding adjuvant therapy, and informing postoperative monitoring in cancer patients (Groot Koerkamp et al., 2015; Burkhart and Pawlik, 2017). At present, there exists no established effective model for predicting the survival situation of post-TAE + HAIC patients (Chen et al., 2021). Therefore, we have developed a nomogram of the sensitive model to anticipate the expected survival outcomes for post-TAE + HAIC patients. Advanced uHCC patients cannot undergo radical resection treatment (Sangro et al., 2012; Galle et al., 2017), it is therefore hard to obtain pathological data and conduct predictive analysis based on the BCLC staging system (Anwanwan et al., 2020). To the best of our abilities, we gathered general clinical information and various clinical data from malignant uHCC patients and predicted variables that could impact the outcomes. We conducted stepwise regression analysis on these variables and ultimately chose the essential clinical factors for nomogram model construction. This improved the model’s sensitivity, accuracy, and stability in predicting post-TAE + HAIC patient survival rates, consequently guiding clinical treatment. The data analysis, which included assessing the C-index and calibration plots, confirmed that the nomogram demonstrated a high level of prediction accuracy. Furthermore, we observed a high level of accuracy in predicting OS using this nomogram. The nomogram model exhibited a superior net benefit to CNLC or BCLC tumor staging following the TAE + HAIC intervention. This discovery suggests that the nomogram can serve as a valuable addition to clinical practice, enabling prognosis assessment for patients with advanced uHCC.
Our study has several noteworthy advantages. Firstly, the sample cohort of advanced uHCC patients is relatively large. Before excluding patients, a total of 743 cases involving 262 patients were included. This significant sample size enhances the confidence and precision of the predicted OS. Secondly, we established comprehensive clinical information, such as gender, age, history of hepatitis B, maximum tumor diameter, number of intrahepatic tumors, tumor distribution differentiation, the situation of invasion and metastasis, ascites, WBC, HGB, PLT, ALB, TBIL, DBIL, ALT, GGT, AKP, PT, and AFP, and accurate follow-up data for the included patients. The selection of multiple variables enhanced the scientific rigor of nomogram construction, making the research results more representative and reliable. Certainly, this study also exhibits several weaknesses. Firstly, this study is a single-center retrospective study, which may introduce potential selection bias. Secondly, further confirmation of the results’ applicability is required through large-scale prospective studies conducted across multiple centers, and the sample size of the uHCC cohort should be further expanded. In summary, although this study has a retrospective design, it provides valuable insights that can enhance the management of patients with advanced uHCC following TAE + HAIC and promote personalized treatment.
Data availability statement
The datasets presented in this article are not readily available due to privacy reasons. Requests to access the datasets should be directed to Z-lZ, enpsenpsMTkwMEAxNjMuY29t.
Ethics statement
The studies involving humans were approved by Ethics Committee of Qilu Hospital, Shandong University. The studies were conducted in accordance with the local legislation and institutional requirements. The participants provided their written informed consent to participate in this study.
Author contributions
L-xD: Data curation, Formal Analysis, Methodology, Software, Validation, Visualization, Writing–original draft, Writing–review and editing. G-lS: Data curation, Formal Analysis, Methodology, Resources, Software, Validation, Writing–review and editing. A-dS: Conceptualization, Data curation, Formal Analysis, Methodology, Validation, Visualization, Writing–original draft, Writing–review and editing. K-sL: Investigation, Methodology, Resources, Supervision, Writing–original draft. Zl-L: Data curation, Formal Analysis, Investigation, Resources, Writing–original draft. Y-CT: Data curation, Investigation, Software, Validation, Writing–original draft. YL: Conceptualization, Funding acquisition, Project administration, Resources, Supervision, Writing–review and editing. Z-lZ: Conceptualization, Funding acquisition, Methodology, Project administration, Resources, Supervision, Writing–review and editing.
Funding
The author(s) declare that financial support was received for the research, authorship, and/or publication of this article. This study was supported by Shandong Province Key Research and Development Project (Grant No. 2021CXGC011105), National Natural Science Foundation of China (Grant No. 82172791), Clinical Research Foundation of Shandong University (Grant No. 2020SDUCRCA018), Natural Science Foundation of Shandong Province (ZR2019MH008, ZR2021QH153), Key Research and Development Program of Shandong Province (Grant No. 2019GSF108254).
Acknowledgments
This retrospective study protocol has received approval from the Ethics Committee of Qilu Hospital, Shandong University. Informed consent allowing the use of retrospective data at the time of admission was obtained from all patients included in the study.
Conflict of interest
The authors declare that the research was conducted in the absence of any commercial or financial relationships that could be construed as a potential conflict of interest.
Publisher’s note
All claims expressed in this article are solely those of the authors and do not necessarily represent those of their affiliated organizations, or those of the publisher, the editors and the reviewers. Any product that may be evaluated in this article, or claim that may be made by its manufacturer, is not guaranteed or endorsed by the publisher.
Abbreviations
BCLC, Barcelona Clinic Liver Cancer; CI, confidence interval; C-index, concordance index; DCA, decision curve analysisu; ECOG, Eastern Cooperative oncology Group; HCC, unresectable hepatocellular carcinoma; HAIC, hepatic arterial infusion chemotherapy; LASSO, least absolute shrinkage and selection operator; OS, overall survival; TAE, transcatheter arterial embolization intervention; TNM, tumor-node-metastasis; HR, hazard ratio.
References
An, J., Kim, D., Oh, B., Oh, Y. J., Song, J., Park, N., et al. (2022). Comprehensive characterization of viral integrations and genomic aberrations in HBV-infected intrahepatic cholangiocarcinomas. Hepatology 75 (4), 997–1011. doi:10.1002/hep.32135
Anwanwan, D., Singh, S. K., Singh, S., Saikam, V., and Singh, R. (2020). Challenges in liver cancer and possible treatment approaches. Biochim. Biophys. Acta Rev. Cancer 1873 (1), 188314. doi:10.1016/j.bbcan.2019.188314
Banales, J. M., Marin, J. J. G., Lamarca, A., Rodrigues, P. M., Khan, S. A., Roberts, L. R., et al. (2020). Cholangiocarcinoma 2020: the next horizon in mechanisms and management. Nat. Rev. Gastroenterol. Hepatol. 17 (9), 557–588. doi:10.1038/s41575-020-0310-z
Burkhart, R. A., and Pawlik, T. M. (2017). Staging and prognostic models for hepatocellular carcinoma and intrahepatic cholangiocarcinoma. Cancer control. 24 (3), 1073274817729235. doi:10.1177/1073274817729235
Chen, C. T., Liu, T. H., Shao, Y. Y., Liu, K. L., Liang, P. C., and Lin, Z. Z. (2021). Revisiting hepatic artery infusion chemotherapy in the treatment of advanced hepatocellular carcinoma. Int. J. Mol. Sci. 22 (23), 12880. doi:10.3390/ijms222312880
Chun, Y. S., Pawlik, T. M., and Vauthey, J. N. (2018). 8th edition of the AJCC cancer staging manual: pancreas and hepatobiliary cancers. Ann. Surg. Oncol. 25 (4), 845–847. doi:10.1245/s10434-017-6025-x
Collins, G. S., Reitsma, J. B., Altman, D. G., and Moons, K. G. (2015). Transparent reporting of a multivariable prediction model for individual prognosis or diagnosis (TRIPOD): the TRIPOD statement. BMJ 350, g7594. doi:10.1136/bmj.g7594
Esparza-Baquer, A., Labiano, I., Sharif, O., Agirre-Lizaso, A., Oakley, F., Rodrigues, P. M., et al. (2021). TREM-2 defends the liver against hepatocellular carcinoma through multifactorial protective mechanisms. Gut 70 (7), 1345–1361. doi:10.1136/gutjnl-2019-319227
Forner, A., Reig, M., and Bruix, J. (2018). Hepatocellular carcinoma. Lancet 391 (10127), 1301–1314. doi:10.1016/S0140-6736(18)30010-2
Galle, P. R., Tovoli, F., Foerster, F., Worns, M. A., Cucchetti, A., and Bolondi, L. (2017). The treatment of intermediate stage tumours beyond TACE: from surgery to systemic therapy. J. Hepatol. 67 (1), 173–183. doi:10.1016/j.jhep.2017.03.007
Groot Koerkamp, B., Wiggers, J. K., Gonen, M., Doussot, A., Allen, P. J., Besselink, M. G. H., et al. (2015). Survival after resection of perihilar cholangiocarcinoma-development and external validation of a prognostic nomogram. Ann. Oncol. 26 (9), 1930–1935. doi:10.1093/annonc/mdv279
Lyu, N., Wang, X., Li, J. B., Lai, J. F., Chen, Q. F., Li, S. L., et al. (2022). Arterial chemotherapy of oxaliplatin plus fluorouracil versus sorafenib in advanced hepatocellular carcinoma: a biomolecular exploratory, randomized, phase III trial (FOHAIC-1). J. Clin. Oncol. 40 (5), 468–480. doi:10.1200/JCO.21.01963
Perugorria, M. J., Olaizola, P., Labiano, I., Esparza-Baquer, A., Marzioni, M., Marin, J. J. G., et al. (2019). Wnt-β-catenin signalling in liver development, health and disease. Nat. Rev. Gastroenterol. Hepatol. 16 (2), 121–136. doi:10.1038/s41575-018-0075-9
Sangro, B., Inarrairaegui, M., and Bilbao, J. I. (2012). Radioembolization for hepatocellular carcinoma. J. Hepatol. 56 (2), 464–473. doi:10.1016/j.jhep.2011.07.012
Shi, A., Liu, Z., Fan, Z., Li, K., Liu, X., Tang, Y., et al. (2024). Function of mast cell and bile-cholangiocarcinoma interplay in cholangiocarcinoma microenvironment. Gut 73, 1350–1363. doi:10.1136/gutjnl-2023-331715
Shi, J., and Line, P. J. W. j.o.g. (2014). Effect of liver regeneration on malignant hepatic tumors. World J. Gastroenterol. 20 (43), 16167–16177. doi:10.3748/wjg.v20.i43.16167
Sunnapwar, A., Katre, R., Policarpio-Nicolas, M., Katabathina, V., and Erian, M. (2016). Imaging of rare primary malignant hepatic tumors in adults with histopathological correlation. J. Comput. Assist. Tomogr. 40 (3), 452–462. doi:10.1097/RCT.0000000000000382
Vickers, A. J., Cronin, A. M., Elkin, E. B., and Gonen, M. (2008). Extensions to decision curve analysis, a novel method for evaluating diagnostic tests, prediction models and molecular markers. BMC Med. Inf. Decis. Mak. 8, 53. doi:10.1186/1472-6947-8-53
Wang, Y., Zhang, Y., Wang, L., Zhang, N., Xu, W., Zhou, J., et al. (2022). Development and experimental verification of a prognosis model for cuproptosis-related subtypes in HCC. Hepatol. Int. 16 (6), 1435–1447. doi:10.1007/s12072-022-10381-0
Yang, C., Zhang, H., Zhang, L., Zhu, A. X., Bernards, R., Qin, W., et al. (2023). Evolving therapeutic landscape of advanced hepatocellular carcinoma. Nat. Rev. Gastroenterol. Hepatol. 20 (4), 203–222. doi:10.1038/s41575-022-00704-9
Keywords: unresectable hepatocellular carcinoma, HAIC, TAE, survival analysis, prognostic model
Citation: Du L-x, Sheng G-l, Shi A-d, Li K-s, Liu Z-l, Tang Y-c, Liu Y and Zhang Z-l (2024) Prognostic nomogram for patients with advanced unresectable hepatocellular carcinoma treated with TAE combined with HAIC. Front. Pharmacol. 15:1426912. doi: 10.3389/fphar.2024.1426912
Received: 02 May 2024; Accepted: 05 August 2024;
Published: 21 August 2024.
Edited by:
Jiajie Peng, Northwestern Polytechnical University, ChinaCopyright © 2024 Du, Sheng, Shi, Li, Liu, Tang, Liu and Zhang. This is an open-access article distributed under the terms of the Creative Commons Attribution License (CC BY). The use, distribution or reproduction in other forums is permitted, provided the original author(s) and the copyright owner(s) are credited and that the original publication in this journal is cited, in accordance with accepted academic practice. No use, distribution or reproduction is permitted which does not comply with these terms.
*Correspondence: Yi Liu, MjgxMDY2MjU5QHFxLmNvbQ==; Zong-li Zhang, enpsenpsMTkwMEAxNjMuY29t
†These authors have contributed equally to this work