- 1Gonçalo Moniz Institute, Oswaldo Cruz Foundation (IGM-FIOCRUZ/BA), Salvador, Brazil
- 2Department of Pathology and Forensic Medicine of the School of Medicine, Federal University of Bahia, Salvador, Brazil
- 3Department of Social and Pediatric Dentistry of the School of Dentistry, Federal University of Bahia, Salvador, Brazil
- 4D’Or Institute for Research and Education (IDOR), Salvador, Brazil
- 5Center of Data and Knowledge Integration for Health (CIDACS), Salvador, Brazil
- 6Department of Propaedeutics, School of Dentistry of the Federal University of Bahia, Salvador, Brazil
Introduction: Cancer refers to a group of diseases characterized by the uncontrolled growth and spread of abnormal cells in the body. Due to its complexity, it has been hard to find an ideal medicine to treat all cancer types, although there is an urgent need for it. However, the cost of developing a new drug is high and time-consuming. In this sense, drug repurposing (DR) can hasten drug discovery by giving existing drugs new disease indications. Many computational methods have been applied to achieve DR, but just a few have succeeded. Therefore, this review aims to show in silico DR approaches and the gap between these strategies and their ultimate application in oncology.
Methods: The scoping review was conducted according to the Arksey and O’Malley framework and the Joanna Briggs Institute recommendations. Relevant studies were identified through electronic searching of PubMed/MEDLINE, Embase, Scopus, and Web of Science databases, as well as the grey literature. We included peer-reviewed research articles involving in silico strategies applied to drug repurposing in oncology, published between 1 January 2003, and 31 December 2021.
Results: We identified 238 studies for inclusion in the review. Most studies revealed that the United States, India, China, South Korea, and Italy are top publishers. Regarding cancer types, breast cancer, lymphomas and leukemias, lung, colorectal, and prostate cancer are the top investigated. Additionally, most studies solely used computational methods, and just a few assessed more complex scientific models. Lastly, molecular modeling, which includes molecular docking and molecular dynamics simulations, was the most frequently used method, followed by signature-, Machine Learning-, and network-based strategies.
Discussion: DR is a trending opportunity but still demands extensive testing to ensure its safety and efficacy for the new indications. Finally, implementing DR can be challenging due to various factors, including lack of quality data, patient populations, cost, intellectual property issues, market considerations, and regulatory requirements. Despite all the hurdles, DR remains an exciting strategy for identifying new treatments for numerous diseases, including cancer types, and giving patients faster access to new medications.
1 Introduction
Cancer is a term that refers to a group of diseases that occur when cells divide uncoordinatedly with other tissues and do not respond appropriately to the signals that control cellular behavior (Sarkar et al., 2013). This feature happens as a result of multifactorial molecular events that involve interactions between the genes and the environment of an organism through a process called carcinogenesis (Karidio and Sanlier, 2021). As the disease progresses, those aberrant cells may invade and ultimately colonize other tissues and neighboring normal organs in a process called metastasis, which is a cancer hallmark that accounts for the most significant number of cancer-related deaths (Fares et al., 2020).
Given the diverse complexity of cancer phenotypes and genotypes, researchers have faced significant challenges in identifying optimal chemical entities as therapeutic agents for each type of cancer, aiming to halt disease progression at both molecular and physiological levels. However, the scarcity of approved drugs remains burdensome and requires discovering new therapeutic molecules.
Developing a new drug is expensive and time-consuming. Indeed, bringing a single medicine from scratch to pharmacy shelves ranges from US$ 944 million to US$ 2.8 billion (adjusted to 2019 prices) (Simoens and Huys, 2021) and takes approximately 10–15 years to be commercially available, with a success rate of only 2.01% (Yeu et al., 2015; Xue et al., 2018). Not to mention that nearly 90% of all clinical drug development fails due to the absence of clinical efficacy, unmanageable toxicity, poor drug-like properties, and lack of commercial demand (Sun et al., 2022). Alternatively, drug repurposing (DR, also drug repositioning, redirecting, reprofiling, and re-tasking), which consists of using existing drugs for new disease indications, can hasten the drug discovery process through the use of validated, toxicologically safe, and already regulated drugs (Martínez-García and Hernandez-Lemus, 2021), making DR a hot topic, currently.
Many methodologies can be used to achieve DR and overcome the difficulties and lengthy processes inherent to new drug discovery. An efficient DR workflow for both Academia and pharmaceutical companies requires the combination of availability and access to molecular data, analytical expertise provided by a cross-disciplinary team, experimental set-up for validation, and clinical development process (Cha et al., 2018). In this regard, repurposing methods can be subtly divided into those based on experimental screening settings and in silico approaches that analyze existing data to identify potential new drug-disease associations.
Although we may have witnessed much progress in the field, the gap between what can be found through computational methodologies and drug repurposing itself is yet to be fully understood. As a matter of fact, repurposing is successful only in certain cases. It denotes that these computational approaches have been misleading, primarily because of the questionable chosen input data, poor data quality, and debatable analysis methods. In addition, patent issues, regulatory considerations, and organizational hurdles also make drug repurposing hard to be implemented (Pushpakom et al., 2018). Thus, this review aims to give an overview of the general approaches to computational drug repurposing, showcasing the gap between the in silico strategies and their practical application in drug repurposing in oncology.
2 Material and methods
2.1 Study protocol and registration
This scoping review followed the methodological framework proposed by Arksey and O’Malley (2005) and complies with the recommendations of the Joanna Briggs Institute for elaborating scoping reviews (Peters et al., 2015). The protocol has been previously published (Cavalcante et al., 2022) and registered on the Open Science Framework (https://osf.io/yx7kp). Additionally, this review is reported following the PRISMA extension for scoping reviews (Supplementary File S1) (Moher et al., 2015; Page et al., 2021).
2.2 Population, concept, and context
We used the population-concept-context (PCC) mnemonic to guide the research questions, eligibility criteria, and literature search. The articles in this scoping review should focus on in silico approaches for drug repurposing (concept) within the Oncology research field (context). We did not specify a population since we were interested in computational methods used in Oncology research in general, including different study designs and populations (Table 1).
2.3 Research question
The following research question guided this scoping review: How can in silico strategies be implemented in DR in oncology? Based on this question, the three main objectives were: 1) to identify the most used in silico strategies, 2) to identify the gap between what can be found through computational strategies and the lacking knowledge concerning DR in oncology and 3) to identify the regulatory barriers to DR when using in silico strategies in cancer research.
2.4 Eligibility criteria
The eligibility criteria were determined through the PCC mnemonic. We included peer-reviewed, English-language research articles published between January 2003 and December 2021 that have implemented in silico strategies for DR in oncology. We excluded narrative or systematic reviews, book chapters, author’s opinions/comments, editorials, erratum, meeting abstracts, conference abstracts, and study protocols. Also, we excluded studies that used in silico strategies for other objectives rather than DR, where no abstract was available or full-text articles could not be obtained, and studies on DR in oncology intended for animal use.
2.5 Information sources and search
At first, a preliminary search was conducted on Pubmed (Medline) to map relevant articles on DR in oncology. The titles and abstracts of these articles and their keywords were used to create a comprehensive search strategy. Our search strategy included Medical Subject Headings (MeSH) terms, their corresponding synonyms, and the Boolean operators “AND” and “OR”. The search strategy was organized into three concept clusters: 1) in silico approach, 2) drug repurposing, and 3) oncology. The search strategy initially created for Pubmed (Medline) (Table 2) was modified for the Embase, Scopus, and Web of Science databases. We also explored the grey literature through Open Grey, and the reference lists of all the included sources of evidence were examined to identify any additional studies (Supplementary File S2). The electronic search strategies were conducted on 22 March 2022. Details regarding the search strategies employed in each database were previously published (Cavalcante et al., 2022).
2.6 Selection of sources of evidence
Following the search, all identified citations were exported in Comma-Separated Values (CSV) format to Microsoft Excel (Version 2019), and duplicates were removed. Two independent reviewers (BRRC and LOSR) screened all titles and abstracts for assessment against the inclusion criteria. The full text of selected articles was assessed against the exclusion criteria by the same reviewers, independently. Any disagreements between the reviewers were resolved through discussion. The inter-rater agreement was assessed through Cohen’s κ before the abstract review stage using the Jamovi software (version 1.2).
2.7 Data charting and data items
Data was extracted from the included articles using a Microsoft Excel spreadsheet (Version 2019). Following the review questions, two independent reviewers (BRRC and GVR) developed and tested the data charting tool with ten random studies. Data extracted comprised the following items: 1) title, 2) publication date, 3) authors, 4) country, 5) study aim, 6) study design, 7) type of cancer, 8) in silico method used, 9) study outcome, 10) mention of regulatory aspects, and 11) occurrence of drug rescue or repurposing.
2.8 Synthesis of results
We present the results narratively and use tables containing the topics detailed in the previous section. The key findings are described according to the review questions, with maps to portray the geographical location of publications and graphs for better data visualization. Also, this review was divided into sections that include the main results of individual sources of evidence followed by the most used methodologies in the included studies and challenges in DR in oncology.
The world map was constructed using the rnaturalearth and ggplot2 packages in the R programming environment (version 4.3.1). These packages provided the geographical data of the globe, where we built a data frame of the publication count with the selected countries from the previous charting of the studied articles. Publications that involved the collaboration of different countries, each country was counted as one. Next, we merged the map with the data frame and generated the plot using the geom_sf function to plot the selected countries. We also used the scale_fill_gradient function to create a color scale for the assigned values.
3 Results
3.1 Selection of sources of evidence
We identified 2,701 articles through our search, 907 of which were duplicates. After de-duplicating the sample, we screened 1,794 articles for relevance. The full list of records screened for eligibility are available at OSF (https://osf.io/yx7kp/files). We found 1,515 records eligible for full-text review, 279 of which were included in our complete analysis. After thorough analysis, 41 articles were excluded for not meeting inclusion criteria, leaving 238 articles eligible for full-text scrutiny (Figure 1). A table with the summary of all included studies is available in Supplementary File S3 and at OSF (See “data charting” at https://osf.io/yx7kp/files/).
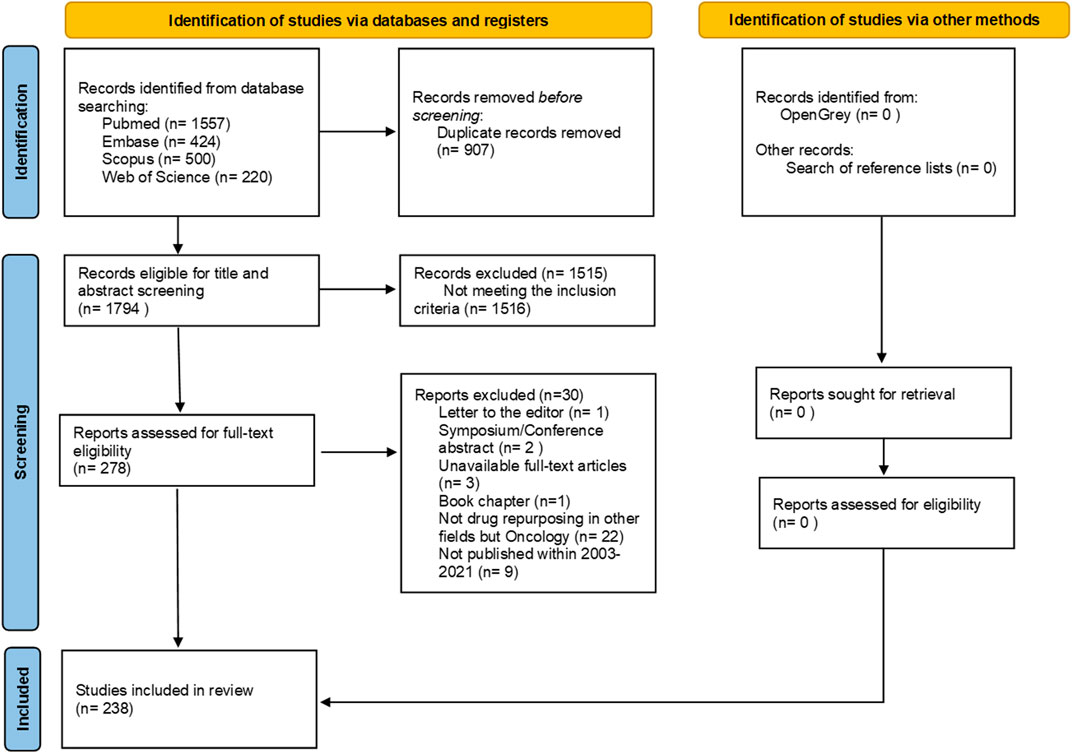
Figure 1. Flow diagram of literature search and selection criteria adapted from PRISMA (Page et al., 2021).
3.2 Characteristics of sources of evidence
The 238 studies that met our inclusion criteria were developed in 44 countries (Figures 2A, B). Most studies identified were from the United States, India, China, South Korea, and Italy, which stand out as the top publishers. Other countries and regions, such as the United Kingdom, Germany, and Spain, have also contributed to the progress of employing computational approaches in oncology. The remaining countries had fewer than ten published studies across all the continents. The central-eastern portions of Europe, many regions of South America and Africa, and northern parts of the Asian continent are regions that did not appear in the data charting of the selected articles.
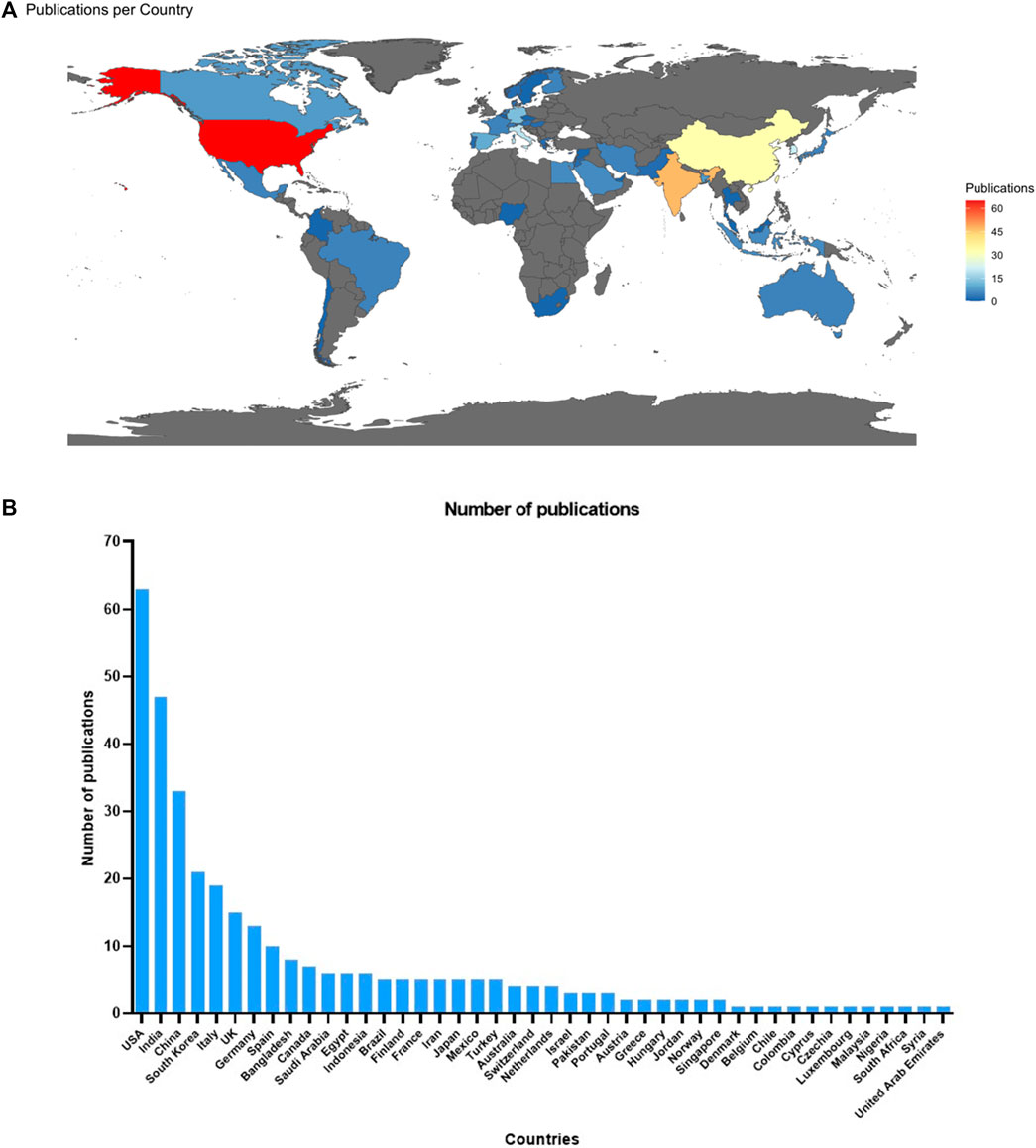
Figure 2. (A) Worldwide distribution of selected studies about drug repurposing in oncology (n = 238). (B) Distribution of publications per country.
3.3 Results of individual sources of evidence and data synthesis
Regarding study design, we sought to investigate if the publications fit into a broad categorization of the in silico strategies. We found that most studies solely used computational methods without further testing their findings in other scientific settings (46.64%) (Shah et al., 2012; Abazeed et al., 2013; Ain et al., 2013; Eichhorn et al., 2013; Emig et al., 2013; Leung et al., 2013; Liu et al., 2013, 2020, 2021; Menden et al., 2013; Naresh et al., 2013; Omotuyi et al., 2013; Singh et al., 2013; Chen, 2014; Dean et al., 2014; Xu and Wang, 2014; Chuang et al., 2015; Dunna et al., 2015; Gustafson et al., 2015; Hammad and Azam, 2015; Kaur et al., 2015; Paula et al., 2015; Rubio-Perez et al., 2015; Sarvagalla et al., 2015; Zhang et al., 2015; Brown et al., 2016; Gayvert et al., 2016; Lee et al., 2016; Shafique et al., 2016; Tan, 2016; Verma et al., 2016; Spiliotopoulos et al., 2017a, 2017b; Jadhav and Karuppayil, 2017; Kabir et al., 2017, 2018, 2019; Khanam et al., 2017; Salentin et al., 2017; Sohraby et al., 2017; Ulfa et al., 2017; Arora and Singh, 2018; Barua et al., 2018; Fröhlich et al., 2018; Jubie et al., 2018; Juritz et al., 2018; Karube et al., 2018; Konidala et al., 2018; Lagarde et al., 2018; Mady et al., 2018; Montes-Grajales et al., 2018; Shi et al., 2018; Shin et al., 2018; Wang et al., 2018; Xu et al., 2018; Yu et al., 2018; Amusengeri and Bishop, 2019; Catalano et al., 2019; Cheng et al., 2019; Floresta et al., 2019; Kuthuru et al., 2019; Natarajan et al., 2019; Patidar et al., 2019; Ramos et al., 2019; Sahrawat and Kaur, 2019; Sweta et al., 2019; Turanli et al., 2019; Yadav et al., 2019; Yang et al., 2019, 2021; Çınaroğlu and Timuçin, 2019; Wu et al., 2020a; Choi et al., 2020; Jiang et al., 2020; Karthik and Vijayakumar, 2020; Kumar et al., 2020; Liñares-Blanco et al., 2020; Prakash and Nath Dwivedi, 2020; Shehadi et al., 2020; Siam et al., 2020; Aier et al., 2021a; Alisha and Tripti, 2021; Aier et al., 2021b; Biswas et al., 2021; Bourdakou et al., 2021; Cadow et al., 2021; Chandel et al., 2021; Florio et al., 2021; Kadioglu et al., 2021; Nasiri et al., 2021; Parveen, 2021; Radaeva et al., 2021; Saranyadevi, 2021; Song et al., 2021; Zagidullin et al., 2021; Deokar and Shaikh, 2022; Gao et al., 2022; Madhukar and Subbarao, 2022; Cell et al., 2024). Surprisingly, several studies combined in silico and in vitro approaches to validate their results (42.02%) (Jordheim et al., 2013; Lafleur et al., 2013; Schonbrunn et al., 2013; Tabchy et al., 2013; Brewer et al., 2014; Kanematsu et al., 2014; Stachnik et al., 2014; Takeuchi et al., 2014; Calvete et al., 2015; De La Cruz-Hernandez et al., 2015; Fako et al., 2015; Istyastono et al., 2015; Liao et al., 2015; McNeil et al., 2015; Rameseder et al., 2015; Riddick et al., 2015; Segura-Cabrera et al., 2015; Zalloum et al., 2015; Zeino et al., 2015; Zhong et al., 2015; Chong et al., 2016; Hengel et al., 2016; Lara-Castillo et al., 2016; Montes-Grajales et al., 2016; Radi et al., 2016; Rocca et al., 2016; Wilson et al., 2016; Chen et al., 2017; Di Lello et al., 2017; Digiacomo et al., 2017; Jaeger et al., 2017; Jernigan et al., 2017; Klingenberg et al., 2017; Kolosenko et al., 2017; Mould et al., 2017; Ozsvari et al., 2017; Platonova et al., 2017; Prabhu et al., 2017; Salim et al., 2017; Vilaboa et al., 2017; Yang et al., 2017; Amaral et al., 2018; Chang et al., 2018; He et al., 2018; Holt et al., 2018; Hyter et al., 2018; Krishna et al., 2018; Manohar et al., 2018; Ayoub et al., 2018; Sakr et al., 2018; Tian et al., 2018; Amrutha et al., 2019; Fischer et al., 2019; Li et al., 2019; Li et al., 2021; Metz et al., 2019; Mishra et al., 2019; Poli et al., 2019; Qi et al., 2019; Song et al., 2019; Ben-Hamo et al., 2019; Frejno et al., 2020; Gibbons et al., 2020; Anderson et al., 2020; Ariey-Bonnet et al., 2020; Naeem et al., 2020; Aydin et al., 2020; Strätker et al., 2020; Faridi et al., 2020; Velázquez-Quesada et al., 2020; Wakai et al., 2020; Benavides-Serrato et al., 2020; Wu et al., 2020b; Zhang J. et al., 2020; Bonnet et al., 2020; Al-Shar’i et al., 2021; Correia et al., 2021; Elkamhawy et al., 2021; Enríquez-Flores et al., 2021; Giubilaro et al., 2021; Nagamalla and Kumar, 2021; Parcha et al., 2021; Rashidieh et al., 2021; Renna et al., 2021; Rimpelová et al., 2021; Suphavilai et al., 2021; Traylor et al., 2021). Only a few publications combined in silico, in vitro, and in vivo methods (10.92%) (Chan et al., 2013; Shi et al., 2013; Van Kesteren et al., 2013; Choi D. S. et al., 2014, Choi et al., 2014 B. H.; Gazzard et al., 2014; Nakamura et al., 2014; Parks et al., 2014; Liu et al., 2015; Druzhyna et al., 2016; Jiang et al., 2017; Ke et al., 2017; Mirza et al., 2017; Pessetto et al., 2017; Kang et al., 2018; Lodagekar et al., 2019; Mottini et al., 2019; Zou et al., 2019; Zhang M. et al., 2020; Kwon et al., 2020; Chequin et al., 2021; Rabben et al., 2021; Sulsenti et al., 2021; Zhao et al., 2021), and a single study added ex vivo models in their approach (0.42%) (Jahchan et al., 2013) (Figure 3A).
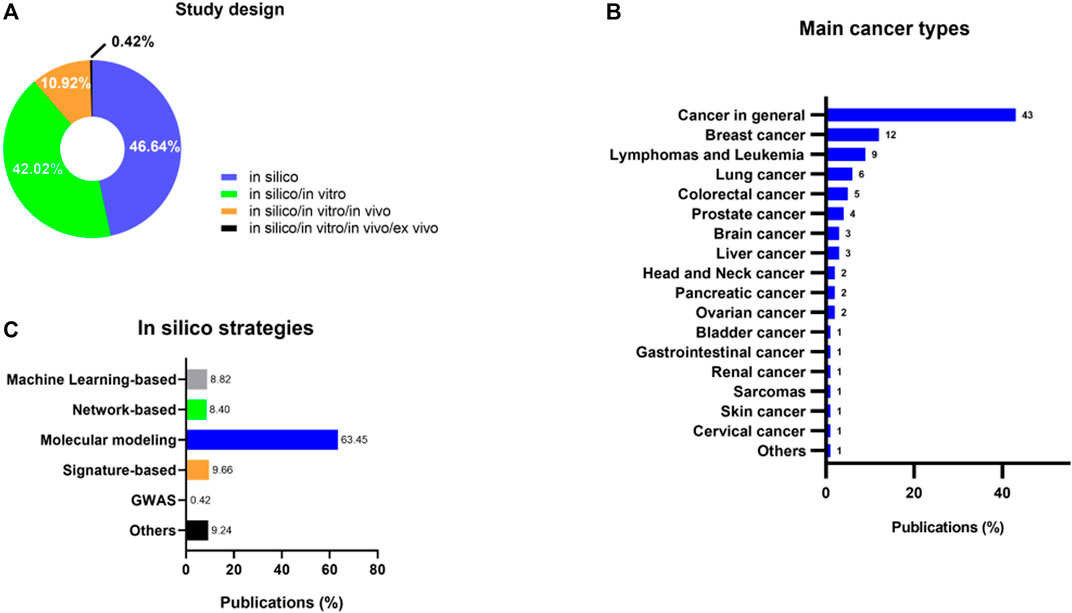
Figure 3. (A) Study design of publications broadly categorized as in silico, in silico/in vitro, in silico/in vitro/in vivo, and in silico/in vitro/in vivo/ex vivo models. (B) Prevalence of the most investigated cancer types under in silico DR approaches. (C) Prevalence of the most used methodologies in the included studies.
Many cancer types can benefit from DR. While the majority of publications (43%) utilized computational techniques to target cancer broadly, including identifying inhibitors (Brewer et al., 2014; Zhong et al., 2015; Shafique et al., 2016; Ulfa et al., 2017; Çınaroğlu and Timuçin, 2019; Siam et al., 2020), agonists (Kaur et al., 2015; Wang et al., 2018), Drug-Target interaction predictions (Emig et al., 2013; Kuthuru et al., 2019), and virtual screening (Rocca et al., 2016; Spiliotopoulos et al., 2017b; Lagarde et al., 2018), a portion of the literature concentrated on specific cancer types. Among these, breast cancer (12%) (Emig et al., 2013; Jiang et al., 2017; Montes-Grajales et al., 2018; Velázquez-Quesada et al., 2020; Chequin et al., 2021), lymphomas and leukemias (9%) (Lara-Castillo et al., 2016; Sohraby et al., 2017; Karube et al., 2018; Sahrawat and Kaur, 2019; Boulos et al., 2021; Parcha et al., 2021), lung (6%) (Kwon et al., 2020; Saranyadevi, 2021), colorectal (5%) (Liñares-Blanco et al., 2020; Biswas et al., 2021; Deokar and Shaikh, 2022; Leung et al., 2022), and prostate (4%) (Kang et al., 2018; Ariey-Bonnet et al., 2020; Benavides-Serrato et al., 2020; Liu et al., 2021; Lin et al., 2022) cancers were the most frequently investigated (Figure 3B).
Several promising computational techniques have been developed to aid in repurposing. They can be broadly categorized as 1) signature-based, 2) molecular modeling, 3) network-based, 4) genome-wide association studies (GWAS) (Altay et al., 2020), and Machine Learning (ML)-based. Most of these methodologies require precise and curated data inputs that demand many datasets and databases. As shown in Figure 3C, molecular modeling (MM), which includes molecular docking (MD) and molecular dynamics simulations (MDS), has emerged as one of the most frequently used methods (63.45% of publications), followed by signature-based (9.66%). Next, some authors have employed ML- (8.82%) and network-based strategies (8.40%). Lastly, a single publication (0.42%) used GWAS to achieve DR. Supplementary File S4 summarizes the main in silico methods used for drug repurposing and their respective softwares.
3.4 Molecular modeling (molecular docking and molecular dynamics simulations)
In molecular modeling, MD has become an increasingly important tool for DR. It can be used to model the interaction between a small molecule and a protein at the atomic level (Meng et al., 2011). Then, some calculations can be performed to investigate the binding affinity of the ligands within the protein active site (i.e., groove or pocket formed by the folding pattern of the protein), elucidating fundamental biochemical processes. Complementarily, MDS can be used to obtain more accurate results since the dynamic behavior of proteins and other biomolecules can be monitored in full atomic detail and at different timescales since MDS allow the understanding of several critical biomolecular processes, such as conformational change, ligand binding, and protein folding, revealing the positions of all the atoms at femtosecond (Hollingsworth and Dror, 2018).
Some publications have used molecular modeling to identify new inhibitors (Mady et al., 2018; Radaeva et al., 2021) or propose new pipelines (Liu et al., 2020; Deokar and Shaikh, 2022), considering only in silico approaches. Nevertheless, some authors used MD as a kick-off start of a more extensive approach to check biological activity in vitro and in vivo for breast cancer and bladder carcinoma. Chequin et al. (2021) showed a repurposing strategy through molecular docking studies of the antitumoral activity of liraglutide, a well-known diabetes type II drug, by modulating epigenetic modifications in breast cancer cell lines in vitro and in vivo using Ehrlich mice tumors models. Ke et al. (2017) used MD to identify potential inhibitors of FGFR3 from 3,167 worldwide approved small-molecule drugs through a repositioning approach. After in vitro testing, the acaricide drug fluazuron exhibited the highest anti-proliferative effect in human bladder carcinoma cell lines RT112 and RT4 and anticancer effect in vivo in BALB/C nude mice subcutaneously xenografted with RT112, suggesting that fluazuron is a potential inhibitor of FGFR3 and a candidate anticancer drug for the treatment of this carcinoma.
Some authors have taken advantage of molecular studies to elucidate whether some drugs display relevant binding properties. Kang et al. (2018) used Molecular modeling simulation to explain the capability of the synthesized analogs to increase the intracellular Ca2+ levels to understand variations in the ability of synthesized analogs of the antipsychotic drug trifluoperazine for the treatment of glioblastoma. They identified fourteen compounds that were biologically active in vitro and in vivo (assessed in brain xenograft mouse model of glioblastoma), presenting the compound 3dc as a new tool for the adjuvant chemotherapy of glioblastoma. Druzhyna et al. (2016) used docking simulations to elucidate how benserazide, a DOPA decarboxylase inhibitor, is related to the inhibition of cystathionine-β-synthase activity and suppresses colon cancer cell proliferation and bioenergetics in vitro and tumor growth in vivo in nude mice bearing human colon cancer cell.
Although applications of combined workflows, including MD and MDS, have been explored to assist different tasks of DR, it is noteworthy to mention that each computational method has its limitations, especially when analyzing less-characterized molecular targets (Pinzi and Rastelli, 2019).
3.5 Signature-based strategies
The exponential growth of omics data across multiple biological levels, encompassing genomics, transcriptomics, and metabolomics, has presented significant opportunities for identifying previously undisclosed targets and comprehending underlying mechanisms in various cancer types. The accessibility of such data in publicly accessible repositories has played an indispensable role in driving substantial progress within the realm of DR research, particularly in exploring genetic signatures associated with dysfunctional signaling pathways in cancer. Signature-based methods are frequently employed to compare gene expression profiles between non-disease and disease states to identify specific gene signatures, which can serve as targets for repurposing existing drugs or guiding the development of new therapeutic compounds. This approach holds the potential for more targeted and effective therapeutic strategies in treating diseases.
Choi D. S. et al. (2014) identified Chloroquine as a potential cancer stem cell (CSC) inhibitor through in silico gene expression signature analysis of the CD44(+)/CD24(-/low) CSC population in Triple-negative breast cancer (TNBC). They reported that Chloroquine could sensitize TNBC cells to paclitaxel through inhibition of autophagy and reduced the CD44(+)/CD24(-/low) CSC population in both preclinical and clinical settings. Similarly, to identify new therapeutic options for Ewing sarcoma (EWS), Pessetto et al. (2017) employed an integrated bioinformatics approach based on disease signature alongside an in vitro screen of FDA-approved drugs to predict drug activity. They showed that two drugs, Auranofin (a thioredoxin reductase inhibitor) and Ganetespib (an HSP90 inhibitor), displayed anticancer activities in silico and in vitro. Still taking advantage of disease signature patterns, Jahchan et al. (2013) employed a DR strategy to identify FDA-approved candidate drugs to treat non-small cell lung cancer (SCLC). They identified tricyclic antidepressants and related G protein–coupled receptor inhibitors as potent inducers of cell death in SCLC cells and other neuroendocrine tumors.
Although widely employed for DR, signature-based approaches face significant challenges. There are genetic signatures that do not provide comprehensive coverage of the transcriptome, that is, all possible gene expressions in an organism. Also, interpreting these signatures often requires a deep understanding of the biological context and the intricate interactions between genes and proteins, which may hinder the identification of causal relationships between signatures and observed effects.
3.6 Machine learning-based approaches
ML is a branch of artificial intelligence (AI) and refers to acquiring predictive information or identifying informative clusters within data. In recent years, ML has been in the spotlight, being applied to several purposes, including the field of drug design, such as the prediction of drug–target interaction and drug discovery. In fact, ML has been used in many other approaches revised in this work, including structure-based approximations (ML-based scoring functions), network-based approaches, etc. Overall, machine learning offers powerful tools and methodologies for accelerating drug repurposing efforts, enabling researchers to leverage existing knowledge and data to identify new therapeutic opportunities, improve drug safety and efficacy, and advance personalized medicine approaches.
Since DR depends on extensive data from existing drugs and diseases, ML methods have been widely used to supply the application of data science to signaling disease, medicine, therapeutics, and identifying potential targets (Yang et al., 2022). Song et al. (2021) developed an ML approach to infer unknown DTIs for breast cancer, called PsePDC-DTIs. The model achieved good prediction results and provided ten potential DTIs. Further, Salim et al. (2017) identified the molecule COTI-2 through a computational platform and checked its role in in vitro and in vivo settings. They showed that COTI-2 was effective against a diverse group of human cancer cell lines regardless of their tissue of origin or genetic makeup. Anderson et al. (2020) used two strategies to enable DR, one by taking advantage of ML models of chordoma inhibition to further screen compounds of interest in vitro and the other by testing combinations of approved kinase inhibitors already being evaluated for chordoma.
If, on one hand, ML has brought more understanding and has provided advances in DR, on the other hand, some limitations may pose some challenges for its implementation, which involve (a) the lack or quality of good data, (b) bias and discrimination (the data might not represent overall population), (c) overfitting and underfitting problems (when ML models fail to provide a suitable output), and (d) lack of reproducibility (inability to replicate or reproduce the results of a ML experiment or study).
3.7 Network-based approaches
Network-based approaches have emerged as promising methods for DR, specifically for treating diverse types of cancer. These techniques require the integration of multiple data sources, such as publicly available datasets and high-throughput data, to model the molecular interactions within complex biological systems using graph theory. In this system, the nodes can be represented by drugs, proteins, diseases, or genes, and edges represent their relationships. The networks modeling drug-drug, drug-target, drug-disease, disease-gene, and protein-protein interactions can provide insights into non-obvious associations related to drug mechanisms of action (MOA).
Since many drug targets act like transcription factors in gene regulation, transcriptome data are extensively used to identify potential drug targets through gene regulatory networks. Network measures such as neighborhood scoring, interconnectivity, network propagation, and random walk enable the discovery of new gene-target associations, prioritizing new candidate targets, and revealing new diseases associated with the selected target.
Protein-protein interaction (PPI) networks are strategies to find drug targets that interact directly or indirectly with other proteins. This method aims to predict drug-target interactions (DTI), considering that proteins targeted by similar drugs are neighbors and functionally related within the interaction network. Many drugs are non-specific and may modulate additional targets beyond their primary targets. Therefore, in addition to protein-protein similarity, network analysis is employed to elucidate drug-drug similarity. When a drug has a known interaction target, models can be predicted based on protein similarity; however, if the drug lacks a known target, its potential target is determined based on the similarity of its molecular structure. Moreover, proteins exhibiting high sequence similarity may interact with analogous drugs.
Some authors used network analysis to provide insightful strategies to promote DR. Emig et al. (2013), for instance, employed a network-based approach for the prediction of drug targets for different types of cancer with their underlying biological processes by using cancer gene expression signature and a high-quality interaction network as inputs and having a prioritized list of drug targets as outputs. Jaeger et al. (2017) used a computational network biology approach to discover new synergistic drug combinations for breast cancer treatment and many cancer types. They found that the combination of raloxifene with the c-Met/VEGFR2 kinase inhibitor cabozantinib potentiated the drugs’ individual antitumor effects in a mouse model of breast cancer. Another exciting approach proposed by Segura-Cabrera et al. (2015) integrated random walk-based network framework to identify known and novel drug indications against different subsets of breast cancers through contextual prioritization based on genome-wide gene expression, shRNA, and drug screen and clinical survival data, conceiving a platform, NetWalker (http://netwalkersuite.org), for contextual prioritization of drugs, genes, and pathways. Similarly, Cheng et al. (2019) proposed an integrated, network-based methodology for cancer type-specific disease module identification and in silico drug repurposing. They developed a Genome-Wide Positioning System Network (GPSnet) algorithm for DR using whole-exome sequencing and transcriptome profiles from ∼5,000 patients across 15 cancer types from The Cancer Genome Atlas. They found that ouabain, an approved cardiac arrhythmia and heart failure drug, displays potential antitumor activities in lung adenocarcinoma.
The drug repositioning strategies based on applying molecular interactomes have significant limitations. There needs to be a complete understanding of the molecular interactions at different biological levels: the interactions among the various molecules within a cell or tissue can encompass interactions between different levels, from the interaction of two proteins to the interaction of multiple metabolic pathways. This complexity requires the consideration of many variables that affect molecular interactions, which can lead to inconsistent or difficult-to-interpret results.
3.8 Genome-wide association studies
Current scientific advances have allowed new visions and therapeutic strategies to be included in medical approaches, making room for an increasingly personalized and inclusive medicine regarding human variability. In this context, one relevant approach is the GWAS.
GWAS comprise a genomic approach that uses the association of genotypes with the phenotypes by testing for differences in the allele frequency of genetic variants between individuals who are ancestrally similar but differ phenotypically (Uffelmann et al., 2021). In this approach, DNA strand loci are used for further analysis of the allele frequency of this region. GWAS aims to identify genetic variants, or single nucleotide polymorphisms (SNPs), that are associated with a particular trait or disease. These SNPs are then used to create a genomic risk score that can be used to predict an individual’s likelihood of developing the disease or trait.
Over the past decades, GWAS have uncovered many genetic variants that may provide targets for DR. However, only one publication used GWAS to promote DR. Zhang et al. (2015) developed an in silico pipeline based on GWAS to prioritize the candidate genes at the colorectal cancer risk loci, targeting them with approved therapies for colorectal cancer, such as crizotinib, arsenic trioxide, vrinostat, dasatinib, estramustine, and tamibarotene.
The valuable information GWAS provide has outstanding potential to guide drug discovery or repurposing. However, there are several limitations underlying these studies, which include: (a) the top genes identified from GWAS may not be easily druggable, (b) the focus on the effect of the top SNPs may miss biologically relevant target genes with small effect sizes, (c) the use of a single candidate gene may miss multi-target drugs, and (d) the inadequate annotation procedures due to the complexity of the human genome (Lau and So, 2020).
3.9 Why is it so challenging to use computational strategies to promote de facto drug repurposing in oncology?
Cancer is a complex disease encompassing a range of different tumor types in terms of genetic and molecular characteristics (Shlyakhtina et al., 2021; Hanahan, 2022) and demands new therapeutic agents. Although DR through computational approaches might be beneficial, it has been quite challenging to implement for numerous reasons.
Several public databases, such as The Cancer Genome Atlas Program (TCGA), broadly provided omics (e.g., genomic, transcriptomics, and proteomic) and clinical data, enabling comprehensive in silico studies in DR in oncology (Mottini et al., 2021). However, high-quality data is not always readily accessible or standardized across studies and databases (Grannis et al., 2019). For the results to be reliable, the datasets must be accurate, comprehensive, reproducible, and representative. Data quality is key for any scientific and clinical research, and it starts with mitigating data imprecisions that lead to inaccurate or misleading analytics results. Some problems include unstructured dataset metadata and inconsistent data processing and quality control, which demand crucial extensive curation and data reprocessing (Lim et al., 2021). Due to the data’s heterogeneity, combining different data types, such as transcriptomic data, chemical structure data, and clinical literature data, poses another computational challenge for effective DR (Ko, 2020). Additionally, relevant and public patient data is limited in low- and mid-income countries (Hung et al., 2020; Abdul-Rahman et al., 2023). Other issues involve reproducibility and replicability in science. Although there are journals with an open data and code policy that require that, as a rule, code and data be released at the latest upon publication (Laurinavichyute et al., 2022), several published studies do not supply raw data and codes, leading to a high burden for those conducting the reproductions (Seibold et al., 2021). Underrepresented data in genomics and multi-omics studies, especially regarding racial and ethnic groups, has hindered advances in human genetics, with the vast majority of research conducted, developed, and validated in individuals of European descent (Landry et al., 2018).
Translating computational predictions into experimental preclinical validation and clinical studies requires time, resources, and collaboration among researchers, clinicians, and regulatory agencies (Seyhan, 2019). Regarding cancer, capturing the complexity and dynamics of the microenvironment (Baghban et al., 2020) through computational strategies, cell lines, and animal models struggles to predict drug responses accurately. Thus, identifying existing drug candidates for repurposing requires a deep understanding of underlying biological mechanisms and pathways involved in diverse cancer types. The accurate prediction of potential drug candidates and their efficacy is related to the knowledge of drug mechanisms, potential side effects, and safety concerns (Berlin et al., 2008). However, there are numerous drugs whose mechanisms and targets still need to be fully understood, and some drugs may have multiple targets or display off-target effects (Palve et al., 2021), further complicating the repurposing process.
The gap between in silico approaches and DR arises because computational strategies and experimental studies may sometimes disagree. The simulations’ results must be validated through experimental and clinical studies before they can be used to support drug repurposing efforts. In vitro and in vivo models are commonly used to validate the predicted potential drugs. Nevertheless, these settings diverge from physiological conditions, requiring new robust experimental designs that resemble in vivo tissue and disease pathology (Ko, 2020), which can be costly. Since drug response differs among patients, more clinical studies are needed to provide a new appropriate dose for the candidate drug since the original dose might no longer be the same. Indeed, pharmaceutical companies still struggle with rising clinical trial costs yearly due to more complex clinical development programs, which involve increased regulatory scrutiny and the growing need to demonstrate the safety and efficacy of new drugs and their value (Martin et al., 2017). A recent paper that assessed the clinical trials cost, gathering data from 726 studies conducted in patients from 2010 to 2015, showed that the median cost of conducting a study from protocol approval to final clinical trial report was US$3.4 million for phase I trials involving patients, $8.6 million for phase II trials and $21.4 million for phase III trials (Martin et al., 2017). Although repurposing a drug can be a cost-effective alternative to developing a new drug, the cost of clinical trials and regulatory submissions can still be substantial.
3.10 Regulatory barriers to drug repurposing when using in silico strategies in cancer research
DR can face several difficulties in the regulatory agencies’ approval process. Overcoming the legal and regulatory barriers is critical for succeeding, as the potential drug candidates must comply with regulatory agencies’ demands. In an ideal scenario, those drugs that show relevant clinical endpoints must be available quickly. In this regard, the US Food and Drug Administration (FDA) has an accelerated approval pathway for cases in which there is an improvement in overall survival or patients willing to tolerate uncertainty about such benefits in exchange for early access to promising cancer drugs (Gyawali et al., 2021). However, the same is not true regarding how other agencies worldwide deal with this issue. Considering that different countries may have different requirements for drug approval, it is difficult to obtain global approval for a repurposed drug. Overall, the approval process for DR can be more challenging than for new drugs. Still, with the increasing recognition of the potential benefits of drug repurposing, regulatory agencies are expected to become more willing to consider repurposed drugs for approval.
Intellectual property issues must also be considered concerning DR. Pharmaceutical companies may only be willing to invest in developing repurposed drugs if they can obtain new patents for the new indications (Krishnamurthy et al., 2022). Another problem is that pharmaceutical companies were described as patenting many compounds, even if they will be abandoned later, thus preventing others from developing these compounds without a license (Tartaglia, 2006). Overall, the financial support for DR has been insufficient, along with shorter patent durations and low returns on investments.
4 Discussion
Computational methods contribute to the process of drug repurposing by streamlining target discovery and drug screening at a preclinical level. This scoping review has highlighted significant advancements and challenges in the field of DR in oncology, led by a variety of in silico strategies. We have shown the dominance of certain countries, such as the United States, India, China, South Korea, and Italy, in computational approaches for drug repurposing for cancer treatment. Overall, these countries often have well-established research infrastructures, including academic institutions, research centers and collaborative networks, which enable the establishment of a thriving environment, filled with necessary resources and expertise for conducting computational research in drug repurposing. Additionally, these countries are often leaders in technological innovation, including advancements in artificial intelligence, machine learning, and bioinformatics. Finally, countries with large populations, such as India and China, may have access to diverse patient populations and clinical datasets, which are invaluable for training computational models and identifying potential drug candidates for repurposing.
Molecular modeling is crucial in drug repurposing efforts in oncology by providing insights into the interactions between drugs and their molecular targets. This can be achieved by predicting existing drugs’ binding affinity and mode of action against specific molecular targets implicated in cancer. Molecular modeling provides detailed structural insights into drug-target interactions and enables rational drug design, whereas signature-, machine learning-, and network-based strategies offer complementary approaches for identifying potential drug candidates and understanding drug-disease associations. These approaches lack mechanistic interpretability or correlations without explicit consideration of molecular structure and function. The incorporation of molecular modeling, particularly MD and MDS, has identified novel inhibitors and repurposing strategies for existing drugs, as evidenced in studies targeting breast cancer and bladder carcinoma (Ke et al., 2017; Chequin et al., 2021). However, applying these methodologies is limited, especially when exploring less-characterized molecular targets (Pinzi and Rastelli, 2019).
Signature-based strategies have capitalized on the rapid expansion of omics data, revealing new targets and disease mechanisms. These methods hold great promise in the quest for potential cancer treatments, as exemplified by the identification of Chloroquine for Triple-negative breast cancer (TNBC) and Auranofin and Ganetespib for Ewing sarcoma (EWS)) (Choi D. S. et al., 2014; Pessetto et al., 2017). However, the challenges associated with these strategies, such as the incomplete coverage of the transcriptome and the complexity of interpreting gene expression signatures within their biological context, remain (Jahchan et al., 2013).
ML-based approaches have introduced a new perspective to DR, enabling the prediction of drug-target interactions and the discovery of new drugs. Notable examples include creating the PsePDC-DTIs model for breast cancer and identifying COTI-2 as an effective agent against various cancer cell lines (Salim et al., 2017; Song et al., 2021). However, the effectiveness of ML models is dependent on the quality and representativeness of the data, and challenges such as bias, overfitting, and lack of reproducibility continue to exist (Yang et al., 2022).
Network-based approaches offer a holistic view of the molecular interactions in cancer, facilitating the discovery of new drug targets and drug-drug similarities. These methods have been successfully employed in predicting drug targets and synergistic drug combinations for various cancers (Emig et al., 2013; Jaeger et al., 2017). Nevertheless, the complexity of molecular interactions and the need for comprehensive data integration present significant hurdles (Segura-Cabrera et al., 2015).
GWAS have also contributed to DR, identifying genetic variants linked to specific diseases. Zhang et al.'s use of GWAS for colorectal cancer illustrates this approach’s potential (Zhang et al., 2015). Nevertheless, the challenges associated with GWAS, including the difficulty in identifying druggable genes and the limitations of focusing on single-gene effects, must be considered (Lau and So, 2020).
The overarching challenge in applying computational strategies for DR in oncology lies in the disease’s complexity and the discrepancies between in silico predictions and experimental validations (Shlyakhtina et al., 2021; Hanahan, 2022), since translating computational findings into clinical applications demands considerable resources and collaboration, with the additional complexity of capturing tumor microenvironments and predicting drug responses (Berlin et al., 2008; Baghban et al., 2020). However, our study identified many works that have employed solely computational approaches. Without further validations and tests, these computational findings become merely suggestive or predictive, hindering the chances of a potentially repurposed drug reaching clinical practice. Furthermore, repurposing drugs also implies that other scientific methodologies (i.e., in vitro validations, in vivo or ex vivo models, accurate toxicology studies, efficacy and effectiveness trials) must be undertaken to reduce the risks to the patient and increase the success rate of repurposed drugs in the product development process.
Lastly, regulatory barriers and intellectual property issues pose significant drug approval and commercialization challenges. The varied requirements of different regulatory agencies and the financial aspects associated with DR, including patenting and investment returns, are critical considerations in this field (Tartaglia, 2006; Gyawali et al., 2021; Krishnamurthy et al., 2022). Additionally, intellectual property issues and market considerations significantly impact the uptake and commercialization of repurposed drugs for cancer treatment. While patent protection and market exclusivity incentivize investment in repurposing efforts, they can also lead to pricing pressures and access barriers. Balancing the need for innovation and profitability with patient access and affordability is essential to ensure that repurposed drugs effectively address unmet medical needs in cancer treatment.
Computational methods for drug repurposing in cancer treatment have limitations that restrain their use to a preclinical level. Molecular models that predict compound-target interaction and treatment response may result in an oversimplification of the pharmacological complexity behind oncological chemotherapy, as it does not consider tumor heterogeneity, spatial and structural complexity, and potential treatment resistance development. Additionally, using biomedical databases harbors a potential human error, as information may be incomplete or misleading, resulting in output bias. Therefore, the application of in silico approaches in drug repurposing does not excuse the validation of results through qualified in vitro or in vivo methods. Finally, most datasets consist of transcriptomes from Caucasian populations, reflecting the lack of representation of minorities in clinical studies. In this sense, it is hard to extrapolate results and adapt promising treatments in Caucasian populations to a racially diverse population, especially black and Hispanic ethnic-racial identities.
Expediting drug discovery through repurposing existing medications for cancer treatment has ethical implications. This requires careful consideration of patient safety, transparency in communication, equitable access to treatments, robust regulatory oversight, management of conflicts of interest, and responsible stewardship of resources. Collaboration among stakeholders, including patients, healthcare providers, researchers, regulatory agencies, and pharmaceutical companies, is essential to navigate these ethical challenges and ensure that the benefits of expediting drug discovery through repurposing existing medications outweigh the potential risks.
As a scoping review, a notable limitation of this study design is the absence of a critical quality assessment of the included studies. However, it is crucial to emphasize that the objective of this review was to map the available evidence, rather than to conduct a methodological quality appraisal of the studies. For such detailed evaluations, more focused systematic reviews are advisable. Another limitation observed in this scoping review is that the studies generally failed to provide scripts or databases necessary for reproducibility. Furthermore, it is significant to point out the lack of established guidelines for a robust methodology or best practices in implementing in silico approaches, which should be explored in further research within this field.
5 Conclusion
Cancer is a generic term for a large group of diseases in which some are more likely to have a more suitable treatment and eventually be cured than others. Because each cancer needs to be treated differently, new approaches, including chemical entities, must be taken to improve oncological patients’ outcomes. In this scenario, repurposing drugs might be an advantageous alternative for faster clinical translation.
DR is an exciting opportunity to give the existing marketed drug new therapeutic indications. However, identifying potential drugs to be repurposed is not trivial, and implementing DR can be challenging due to various factors, including lack of quality data, patient populations, cost, intellectual property issues, market considerations, and regulatory requirements.
It is important to note that the trend of DR continues to grow, propelled mainly by employing advanced AI techniques. These techniques offer fresh perspectives on disease drug targets and enhance the likelihood of successful drug repurposing. In conclusion, despite the inherent challenges, DR is a promising strategy for discovering new treatments for a range of diseases, including various types of cancer, facilitating quicker patient access to novel medications and treatments.
Data availability statement
The original contributions presented in the study are included in the article/Supplementary Material, further inquiries can be directed to the corresponding author.
Author contributions
BC: Conceptualization, Data curation, Formal Analysis, Investigation, Methodology, Software, Validation, Visualization, Writing–original draft, Writing–review and editing. RF: Conceptualization, Data curation, Formal Analysis, Investigation, Methodology, Supervision, Validation, Visualization, Writing–original draft, Writing–review and editing. LdO: Conceptualization, Writing–original draft, Writing–review and editing. RS: Writing–original draft, Writing–review and editing. BS: Conceptualization, Investigation, Writing–original draft, Writing–review and editing. PR: Writing–original draft, Writing–review and editing. GR: Writing–original draft, Writing–review and editing. CG: Conceptualization, Funding acquisition, Investigation, Methodology, Project administration, Resources, Supervision, Writing–original draft, Writing–review and editing.
Funding
The authors declare that financial support was received for the research, authorship, and/or publication of this article. This work was financially supported by the following institutions: National Support Program for Oncology Care (PRONON) [#25000.192875/2019-78], Conselho Nacional de Desenvolvimento Científico e Tecnológico (CNPq) [#308276/2019-1], INOVA Fiocruz [PP1192029]. BRRC is supported by the Gonçalo Moniz Institute, Oswaldo Cruz Foundation. LOSR is supported by CNPq [#9196892774005300] and the Coordination for the Improvement of Higher Education Personnel (CAPES) [#88887.803546/2023-00]. RDF was supported by INOVA Fiocruz. GVR was funded by Maria Emília Foundation (Salvador, Bahia, Brazil). CAGR is granted for research productivity with a scholarship award by CNPq. The funders played no role in study design, data collection, analysis and interpretation of data, or the writing of this manuscript.
Conflict of interest
The authors declare that the research was conducted in the absence of any commercial or financial relationships that could be construed as a potential conflict of interest.
Publisher’s note
All claims expressed in this article are solely those of the authors and do not necessarily represent those of their affiliated organizations, or those of the publisher, the editors and the reviewers. Any product that may be evaluated in this article, or claim that may be made by its manufacturer, is not guaranteed or endorsed by the publisher.
Supplementary material
The Supplementary Material for this article can be found online at: https://www.frontiersin.org/articles/10.3389/fphar.2024.1400029/full#supplementary-material
References
Abazeed, M. E., Adams, D. J., Hurov, K. E., Tamayo, P., Creighton, C. J., Sonkin, D., et al. (2013). Integrative radiogenomic profiling of squamous cell lung Cancer. Cancer Res. 73, 6289–6298. doi:10.1158/0008-5472.CAN-13-1616
Abdul-Rahman, T., Ghosh, S., Lukman, L., Bamigbade, G. B., Oladipo, O. V., Amarachi, O. R., et al. (2023). Inaccessibility and low maintenance of medical data archive in low-middle income countries: mystery behind public health statistics and measures. J. Infect. Public Health 16, 1556–1561. doi:10.1016/j.jiph.2023.07.001
Aier, I., Semwal, R., Raj, U., and Varadwaj, P. K. (2021a). Comparative modeling and structure based drug repurposing of PAX2 transcription factor for targeting acquired chemoresistance in pancreatic ductal adenocarcinoma. J. Biomol. Struct. Dyn. 39, 2071–2078. doi:10.1080/07391102.2020.1742793
Aier, I., Semwal, R., Sharma, A., and Varadwaj, P. K. (2021b). In silico identification of therapeutic compounds against microRNA targets in drug-resistant pancreatic ductal adenocarcinoma. J. Biomol. Struct. Dyn. 39, 4893–4901. doi:10.1080/07391102.2020.1782262
Ain, Q. U., Seemab, U., Rashid, S., Nawaz, M. S., and Kamal, M. A. (2013). Prediction of structure of human WNT-CRD (FZD) complex for computational drug repurposing. PLoS One 8, e54630–e54639. doi:10.1371/journal.pone.0054630
Alisha, K., and Tripti, S. (2021). Repurposing statins as a potential ligand for estrogen receptor alpha via molecular docking. Res. J. Pharm. Technol. 14, 3757–3762. doi:10.52711/0974-360X.2021.00650
Al-Shar’i, N. A., Al-Balas, Q. A., Hassan, M. A., El-Elimat, T. M., Aljabal, G. A., and Almaaytah, A. M. (2021). Ellagic acid: a potent glyoxalase-I inhibitor with a unique scaffold. Acta Pharm. 71, 115–130. doi:10.2478/acph-2021-0005
Altay, O., Mohammadi, E., Lam, S., Turkez, H., Boren, J., Nielsen, J., et al. (2020). Current status of COVID-19 therapies and drug repositioning applications. iScience 23, 101303. doi:10.1016/j.isci.2020.101303
Amaral, M. E. A., Nery, L. R., Leite, C. E., de Azevedo Junior, W. F., and Campos, M. M. (2018). Pre-clinical effects of metformin and aspirin on the cell lines of different breast cancer subtypes. Invest. New Drugs 36, 782–796. doi:10.1007/s10637-018-0568-y
Amrutha, N. A., Archana, P. R., Mohan, S. G., Anto, R. J., and Sadasivan, C. (2019). Pyridine derivatives as anticancer lead compounds with Fatty Acid Synthase as the target: an in silico-guided in vitro study. J. Cell. Biochem. 120, 16643–16657. doi:10.1002/jcb.28923
Amusengeri, A., and Bishop, Ö. T. (2019). Discorhabdin N, a South African natural compound, for Hsp72 and Hsc70 allosteric modulation: combined study of molecular modeling and dynamic residue network analysis. Molecules 24, 188. doi:10.3390/molecules24010188
Anderson, E., Havener, T. M., Zorn, K. M., Foil, D. H., Lane, T. R., Capuzzi, S. J., et al. (2020). Synergistic drug combinations and machine learning for drug repurposing in chordoma. Sci. Rep. 10, 12982–13010. doi:10.1038/s41598-020-70026-w
Ariey-Bonnet, J., Carrasco, K., Le Grand, M., Hoffer, L., Betzi, S., Feracci, M., et al. (2020). In silico molecular target prediction unveils mebendazole as a potent MAPK14 inhibitor. Mol. Oncol. 14, 3083–3099. doi:10.1002/1878-0261.12810
Arksey, H., and O’Malley, L. (2005). Scoping studies: towards a methodological framework. Int. J. Soc. Res. Methodol. Theory Pract. 8, 19–32. doi:10.1080/1364557032000119616
Arora, D., and Singh, A. (2018). Systems biology approach deciphering the biochemical signaling pathway and pharmacokinetic study of PI3K/mTOR/p53-Mdm2 module involved in neoplastic transformation. Netw. Model. Anal. Heal. Inf. Bioinforma. 7, 2–11. doi:10.1007/s13721-017-0162-9
Aydin, G., Paksoy, M. N., Orhan, M. D., Avsar, T., Yurtsever, M., and Durdagi, S. (2020). Proposing novel MDM2 inhibitors: combined physics-driven high-throughput virtual screening and in vitro studies. Chem. Biol. Drug Des. 96, 684–700. doi:10.1111/cbdd.13694
Ayoub, B. M., Attia, Y. M., and Ahmed, M. S. (2018). Structural re-positioning, in silico molecular modelling, oxidative degradation, and biological screening of linagliptin as adenosine 3 receptor (ADORA3) modulators targeting hepatocellular carcinoma. J. Enzyme Inhib. Med. Chem. 33, 858–866. doi:10.1080/14756366.2018.1462801
Baghban, R., Roshangar, L., Jahanban-Esfahlan, R., Seidi, K., Ebrahimi-Kalan, A., Jaymand, M., et al. (2020). Tumor microenvironment complexity and therapeutic implications at a glance. Cell Commun. Signal. 18, 59–19. doi:10.1186/s12964-020-0530-4
Barua, A., Kesavan, K., and Jayanthi, S. (2018). Molecular docking studies of plant compounds to identify efficient inhibitors for ovarian cancer. Res. J. Pharm. Technol. 11, 3811–3815. doi:10.5958/0974-360X.2018.00698.4
Benavides-Serrato, A., Saunders, J. T., Holmes, B., Nishimura, R. N., Lichtenstein, A., and Gera, J. (2020). Repurposing potential of riluzole as an ITAF inhibitor in mTOR therapy resistant glioblastoma. Int. J. Mol. Sci. 21, 344–416. doi:10.3390/ijms21010344
Ben-Hamo, R., Zilberberg, A., Cohen, H., Bahar-Shany, K., Wachtel, C., Korach, J., et al. (2019). Resistance to paclitaxel is associated with a variant of the gene BCL2 in multiple tumor types. npj Precis. Oncol. 3, 12. doi:10.1038/s41698-019-0084-3
Berlin, J. A., Glasser, S. C., and Ellenberg, S. S. (2008). Adverse event detection in drug development: recommendations and obligations beyond phase 3. Am. J. Public Health 98, 1366–1371. doi:10.2105/AJPH.2007.124537
Biswas, P., Dey, D., Rahman, A., Islam, M. A., Susmi, T. F., Kaium, M. A., et al. (2021). Analysis of SYK gene as a prognostic biomarker and suggested potential bioactive phytochemicals as an alternative therapeutic option for colorectal cancer: an in-silico pharmaco-informatics investigation. J. Pers. Med. 11, 888. doi:10.3390/jpm11090888
Bonnet, R., Nebout, M., Brousse, C., Reinier, F., Imbert, V., Rohrlich, P. S., et al. (2020). New drug repositioning candidates for T-ALL identified via human/murine gene signature comparison. Front. Oncol. 10, 557643–557711. doi:10.3389/fonc.2020.557643
Boulos, J. C., Saeed, M. E. M., Chatterjee, M., Bülbül, Y., Crudo, F., Marko, D., et al. (2021). Repurposing of the alk inhibitor crizotinib for acute leukemia and multiple myeloma cells. Pharmaceuticals 14, 1126. doi:10.3390/ph14111126
Bourdakou, M. M., Spyrou, G. M., and Kolios, G. (2021). Colon cancer progression is reflected to monotonic differentiation in gene expression and pathway deregulation facilitating stage–specific drug repurposing. Cancer Genomics Proteomics 18, 757–769. doi:10.21873/cgp.20295
Brewer, F. K., Follit, C. A., Vogel, P. D., and Wise, J. G. (2014). In silico screening for inhibitors of P-glycoprotein that target the nucleotide binding domains. Mol. Pharmacol. 86, 716–726. doi:10.1124/mol.114.095414
Brown, A. S., Kong, S. W., Kohane, I. S., and Patel, C. J. (2016). ksRepo: a generalized platform for computational drug repositioning. BMC Bioinforma. 17, 78–85. doi:10.1186/s12859-016-0931-y
Cadow, J., Born, J., Manica, M., Oskooei, A., and Martínez, M. R. (2021). PaccMann: a web service for interpretable anticancer compound sensitivity prediction. Nucleic Acids Res. 48, W502–W508. doi:10.1093/NAR/GKAA327
Calvete, O., Martinez, P., Garcia-Pavia, P., Benitez-Buelga, C., Paumard-Hernández, B., Fernandez, V., et al. (2015). A mutation in the POT1 gene is responsible for cardiac angiosarcoma in TP53-negative Li-Fraumeni-like families. Nat. Commun. 6, 8383. doi:10.1038/ncomms9383
Catalano, R., Rocca, R., Juli, G., Costa, G., Maruca, A., Artese, A., et al. (2019). A drug repurposing screening reveals a novel epigenetic activity of hydroxychloroquine. Eur. J. Med. Chem. 183, 111715. doi:10.1016/j.ejmech.2019.111715
Cavalcante, B. R. R., Freitas, R. D., de Oliveira Siquara da Rocha, L., Rocha, G. V., de Carvalho Pachêco, T. C., Pereira Ramos, P. I., et al. (2022). In silico approaches for drug repurposing in oncology: protocol for a scoping review of existing evidence. PLoS One 17, 1–6. doi:10.1371/journal.pone.0271002
Cell, S., Serrano, D., Gimeno, M., Planes, F. J., and Pio, R. (2024) DrugSniper, a tool to exploit loss-of-function screens, identifies CREBBP as a predictive, 1–18.
Cha, Y., Erez, T., Reynolds, I. J., Kumar, D., Ross, J., Koytiger, G., et al. (2018). Drug repurposing from the perspective of pharmaceutical companies. Br. J. Pharmacol. 175, 168–180. doi:10.1111/bph.13798
Chan, C. H., Morrow, J. K., Li, C. F., Gao, Y., Jin, G., Moten, A., et al. (2013). Pharmacological inactivation of Skp2 SCF ubiquitin ligase restricts cancer stem cell traits and cancer progression. Cell 154, 556–568. doi:10.1016/j.cell.2013.06.048
Chandel, V., Sharma, P. P., Nayar, S. A., Jha, N. K., Jha, S. K., Rathi, B., et al. (2021). In silico identification of potential inhibitor for TP53-induced glycolysis and apoptosis regulator in head and neck squamous cell carcinoma. 3 Biotech. 11, 117–216. doi:10.1007/s13205-021-02665-3
Chang, Y., Park, H., Yang, H. J., Lee, S., Lee, K. Y., Kim, T. S., et al. (2018). Cancer drug response profile scan (CDRscan): a deep learning model that predicts drug effectiveness from cancer genomic signature. Sci. Rep. 8, 8857–8911. doi:10.1038/s41598-018-27214-6
Chen, B., Wang, H., Wu, Z., Duan, B., Bai, P., Zhang, K., et al. (2017). Conformational stabilization of FOX–DNA complex architecture to sensitize prostate cancer chemotherapy. Amino Acids 49, 1247–1254. doi:10.1007/s00726-017-2426-1
Chen, S. J. (2014). A potential target of tanshinone IIA for acute promyelocytic leukemia revealed by inverse docking and drug repurposing. Asian Pac. J. Cancer Prev. 15, 4301–4305. doi:10.7314/APJCP.2014.15.10.4301
Cheng, F., Lu, W., Liu, C., Fang, J., Hou, Y., Handy, D. E., et al. (2019). A genome-wide positioning systems network algorithm for in silico drug repurposing. Nat. Commun. 10, 3476. doi:10.1038/s41467-019-10744-6
Chequin, A., Costa, L. E., de Campos, F. F., Moncada, A. D. B., de Lima, L. T. F., Sledz, L. R., et al. (2021). Antitumoral activity of liraglutide, a new DNMT inhibitor in breast cancer cells in vitro and in vivo. Chem. Biol. Interact. 349, 109641. doi:10.1016/j.cbi.2021.109641
Choi, B. H., Chattopadhaya, S., Thanh, L. N., Feng, L., Nguyen, Q. T., Lim, C. B., et al. (2014a). Suprafenacine, an indazole-hydrazide agent, targets cancer cells through microtubule destabilization. PLoS One 9, e110955. doi:10.1371/journal.pone.0110955
Choi, D. S., Blanco, E., Kim, Y. S., Rodriguez, A. A., Zhao, H., Huang, T. H. M., et al. (2014b). Chloroquine eliminates cancer stem cells through deregulation of Jak2 and DNMT1. Stem Cells 32, 2309–2323. doi:10.1002/stem.1746
Choi, J., Park, S., and Ahn, J. (2020). RefDNN: a reference drug based neural network for more accurate prediction of anticancer drug resistance. Sci. Rep. 10, 1861–1911. doi:10.1038/s41598-020-58821-x
Chong, C. M., Kou, M. T., Pan, P., Zhou, H., Ai, N., Li, C., et al. (2016). Discovery of a novel ROCK2 inhibitor with anti-migration effects: via docking and high-content drug screening. Mol. Biosyst. 12, 2713–2721. doi:10.1039/c6mb00343e
Chuang, C. H., Cheng, T. C., Leu, Y. L., Chuang, K. H., Tzou, S. C., and Chen, C. S. (2015). Discovery of akt kinase inhibitors through structure-based virtual screening and their evaluation as potential anticancer agents. Int. J. Mol. Sci. 16, 3202–3212. doi:10.3390/ijms16023202
Çınaroğlu, S. S., and Timuçin, E. (2019). In silico identification of inhibitors targeting N-Terminal domain of human Replication Protein A. J. Mol. Graph. Model. 86, 149–159. doi:10.1016/j.jmgm.2018.10.011
Correia, C., Ferreira, A., Santos, J., Lapa, R., Yliperttula, M., Urtti, A., et al. (2021). New in vitro-in silico approach for the prediction of in vivo performance of drug combinations. Molecules 26, 4257–4324. doi:10.3390/molecules26144257
Dean, R. A., Fam, H. K., An, J., Choi, K., Shimizu, Y., Jones, S. J. M., et al. (2014). Identification of a putative tdp1 inhibitor (CD00509) by in vitro and cell-based assays. J. Biomol. Screen. 19, 1372–1382. doi:10.1177/1087057114546551
De La Cruz-Hernandez, E., Medina-Franco, J. L., Trujillo, J., Chavez-Blanco, A., Dominguez-Gomez, G., Perez-Cardenas, E., et al. (2015). Ribavirin as a tri-targeted antitumor repositioned drug. Oncol. Rep. 33, 2384–2392. doi:10.3892/or.2015.3816
Deokar, S. S., and Shaikh, K. S. (2022). Exploring cytotoxic potential of ciclopirox on colorectal cancer cells by in-silico methodology. Biointerface Res. Appl. Chem. 12, 7287–7310. doi:10.33263/BRIAC126.72877310
Digiacomo, V., De Opakua, A. I., Papakonstantinou, M. P., Nguyen, L. T., Merino, N., Blanco-Canosa, J. B., et al. (2017). The Gαi-GIV binding interface is a druggable protein-protein interaction. Sci. Rep. 7, 8575–8617. doi:10.1038/s41598-017-08829-7
Di Lello, P., Pastor, R., Murray, J. M., Blake, R. A., Cohen, F., Crawford, T. D., et al. (2017). Discovery of small-molecule inhibitors of ubiquitin specific protease 7 (USP7) using integrated NMR and in silico techniques. J. Med. Chem. 60, 10056–10070. doi:10.1021/acs.jmedchem.7b01293
Druzhyna, N., Szczesny, B., Olah, G., Módis, K., Asimakopoulou, A., Pavlidou, A., et al. (2016). Screening of a composite library of clinically used drugs and well-characterized pharmacological compounds for cystathionine β-synthase inhibition identifies benserazide as a drug potentially suitable for repurposing for the experimental therapy of colon cancer. Pharmacol. Res. 113, 18–37. doi:10.1016/j.phrs.2016.08.016
Dunna, N., Bandaru, S., Akare, U., Rajadhyax, S., Gutlapalli, V., Yadav, M., et al. (2015). Multiclass comparative virtual screening to identify novel Hsp90 inhibitors: a therapeutic breast cancer drug target. Curr. Top. Med. Chem. 15, 57–64. doi:10.2174/1568026615666150112113627
Eichhorn, T., Hiller, C., Hirschfelder, K., Frank, M., Krauth-Siege, R. L., Müller, R., et al. (2013). Identification by high-throughput in silico screening of radio-protecting compounds targeting the DNA-binding domain of the tumor suppressor p53. Cancer Genomics Proteomics 10, 35–45.
Elkamhawy, A., Ammar, U. M., Paik, S., Abdellattif, M. H., Elsherbeny, M. H., Lee, K., et al. (2021). Scaffold repurposing of in-house small molecule candidates leads to discovery of first-in-class cdk-1/her-2 dual inhibitors: in vitro and in silico screening. Molecules 26, 5324–5412. doi:10.3390/molecules26175324
Emig, D., Ivliev, A., Pustovalova, O., Lancashire, L., Bureeva, S., Nikolsky, Y., et al. (2013). Drug target prediction and repositioning using an integrated network-based approach. PLoS One 8, e60618. doi:10.1371/journal.pone.0060618
Enríquez-Flores, S., López, L. A. F., Mora-De la Mora, I. D. L., García-Torres, I., Gracia-Mora, I., Gutiérrez-Castrellón, P., et al. (2021). Deamidated TPI is an efficacious target for cell-selective therapy in triple-negative breast cancer. bioRxiv. doi:10.1101/2021.11.09.467888
Fako, V. E., Wu, X., Pflug, B., Liu, J. Y., and Zhang, J. T. (2015). Repositioning proton pump inhibitors as anticancer drugs by targeting the thioesterase domain of human fatty acid synthase. J. Med. Chem. 58, 778–784. doi:10.1021/jm501543u
Fares, J., Fares, M. Y., Khachfe, H. H., Salhab, H. A., and Fares, Y. (2020). Molecular principles of metastasis: a hallmark of cancer revisited. Signal Transduct. Target. Ther. 5, 28. doi:10.1038/s41392-020-0134-x
Faridi, U., Al-Mutairi, F., Parveen, H., and Khateeb, S. (2020). An in-vitro and in silico anticancer study of FDA approved antidiabetic drugs glimepiride and empagliflozin. Int. J. Life Sci. Pharma Res. 10, 52–57. doi:10.22376/ijpbs/lpr.2020.10.2.l52-57
Fischer, C. L., Bates, A. M., Lanzel, E. A., Guthmiller, J. M., Johnson, G. K., Singh, N. K., et al. (2019). Computational models accurately predict multi-cell biomarker profiles in inflammation and cancer. Sci. Rep. 9, 10877–10913. doi:10.1038/s41598-019-47381-4
Floresta, G., Amata, E., Gentile, D., Romeo, G., Marrazzo, A., Pittalà, V., et al. (2019). Fourfold filtered statistical/computational approach for the identification of imidazole compounds as HO-1 inhibitors from natural products. Mar. Drugs 17, 113. doi:10.3390/md17020113
Florio, R., Carradori, S., Veschi, S., Brocco, D., Genni, T. D., Cirilli, R., et al. (2021). Screening of benzimidazole-based anthelmintics and their enantiomers as repurposed drug candidates in cancer therapy. Pharmaceuticals 14, 372–416. doi:10.3390/ph14040372
Frejno, M., Meng, C., Ruprecht, B., Oellerich, T., Scheich, S., Kleigrewe, K., et al. (2020). Proteome activity landscapes of tumor cell lines determine drug responses. Nat. Commun. 11, 3639–3712. doi:10.1038/s41467-020-17336-9
Fröhlich, F., Kessler, T., Weindl, D., Shadrin, A., Schmiester, L., Hache, H., et al. (2018). Efficient parameter estimation enables the prediction of drug response using a mechanistic pan-cancer pathway model. Cell Syst. 7, 567–579. doi:10.1016/j.cels.2018.10.013
Gao, Q., Yao, P., Wang, Y., Yao, Q., and Zhang, J. (2022). Discovery of potent HDAC2 inhibitors based on virtual screening in combination with drug repurposing. J. Mol. Struct. 1247, 131399. doi:10.1016/j.molstruc.2021.131399
Gayvert, K. M., Dardenne, E., Cheung, C., Boland, M. R., Lorberbaum, T., Wanjala, J., et al. (2016). A computational drug repositioning approach for targeting oncogenic transcription factors. Cell Rep. 15, 2348–2356. doi:10.1016/j.celrep.2016.05.037
Gazzard, L., Appleton, B., Chapman, K., Chen, H., Clark, K., Drobnick, J., et al. (2014). Discovery of the 1,7-diazacarbazole class of inhibitors of checkpoint kinase 1. Bioorg. Med. Chem. Lett. 24, 5704–5709. doi:10.1016/j.bmcl.2014.10.063
Gibbons, G. S., Chakraborty, A., Grigsby, S. M., Umeano, A. C., Liao, C., Moukha-Chafiq, O., et al. (2020). Identification of DOT1L inhibitors by structure-based virtual screening adapted from a nucleoside-focused library. Eur. J. Med. Chem. 189, 112023–112119. doi:10.1016/j.ejmech.2019.112023
Giubilaro, J., Schuetz, D. A., Stepniewski, T. M., Namkung, Y., Khoury, E., Lara-Márquez, M., et al. (2021). Discovery of a dual Ras and ARF6 inhibitor from a GPCR endocytosis screen. Nat. Commun. 12, 4688. doi:10.1038/s41467-021-24968-y
Grannis, S. J., Xu, H., Vest, J. R., Kasthurirathne, S., Bo, N., Moscovitch, B., et al. (2019). Evaluating the effect of data standardization and validation on patient matching accuracy. J. Am. Med. Inf. Assoc. 26, 447–456. doi:10.1093/jamia/ocy191
Gustafson, D. L., Fowles, J. S., Brown, K. C., and Theodorescu, D. (2015). Drug selection in the genomic age: application of the coexpression extrapolation principle for drug repositioning in cancer therapy. Assay. Drug Dev. Technol. 13, 623–627. doi:10.1089/adt.2015.29012.dlgdrrr
Gyawali, B., Rome, B. N., and Kesselheim, A. S. (2021). Regulatory and clinical consequences of negative confirmatory trials of accelerated approval cancer drugs: retrospective observational study. BMJ 374, 19599–n1968. doi:10.1136/bmj.n1959
Hammad, M. A., and Azam, S. S. (2015). Structural dynamics and inhibitor searching for Wnt-4 protein using comparative computational studies. Drug Des. devel. Ther. 9, 2449–2461. doi:10.2147/DDDT.S79784
Hanahan, D. (2022). Hallmarks of cancer: new dimensions. Cancer Discov. 12, 31–46. doi:10.1158/2159-8290.CD-21-1059
He, L., Tang, J., Andersson, E. I., Timonen, S., Koschmieder, S., Wennerberg, K., et al. (2018). Patient-customized drug combination prediction and testing for t-cell prolymphocytic leukemia patients. Cancer Res. 78, 2407–2418. doi:10.1158/0008-5472.CAN-17-3644
Hengel, S. R., Malacaria, E., Da Silva Constantino, L. F., Bain, F. E., Diaz, A., Koch, B. G., et al. (2016). Small-molecule inhibitors identify the RAD52-ssDNA interaction as critical for recovery from replication stress and for survival of BRCA2 deficient cells. Elife 5, 1–30. doi:10.7554/eLife.14740
Hollingsworth, S. A., and Dror, R. O. (2018). Molecular dynamics simulation for all. Neuron 99, 1129–1143. doi:10.1016/j.neuron.2018.08.011
Holt, M. C., Assar, Z., Beheshti Zavareh, R., Lin, L., Anglin, J., Mashadova, O., et al. (2018). Biochemical characterization and structure-based mutational analysis provide insight into the binding and mechanism of action of novel aspartate aminotransferase inhibitors. Biochemistry 57, 6604–6614. doi:10.1021/acs.biochem.8b00914
Hung, Y. W., Hoxha, K., Irwin, B. R., Law, M. R., and Grépin, K. A. (2020). Using routine health information data for research in low- and middle-income countries: a systematic review. BMC Health Serv. Res. 20, 790–815. doi:10.1186/s12913-020-05660-1
Hyter, S., Hirst, J., Pathak, H., Pessetto, Z. Y., Koestler, D. C., Raghavan, R., et al. (2018). Developing a genetic signature to predict drug response in ovarian cancer. Oncotarget 9, 14828–14848. doi:10.18632/oncotarget.23663
Istyastono, E. P., Riswanto, F. D. O., and Yuliani, S. H. (2015). Computer-aided drug repurposing: a cyclooxygenase-2 inhibitor celecoxib as a ligand for estrogen receptor alpha. Indones. J. Chem. 15, 274–280. doi:10.22146/ijc.21196
Jadhav, A. K., and Karuppayil, S. M. (2017). Molecular docking studies on thirteen fluoroquinolines with human topoisomerase II a and b. Silico Pharmacol. 5, 4–12. doi:10.1007/s40203-017-0024-2
Jaeger, S., Igea, A., Arroyo, R., Alcalde, V., Canovas, B., Orozco, M., et al. (2017). Quantification of pathway cross-talk reveals novel synergistic drug combinations for breast cancer. Cancer Res. 77, 459–469. doi:10.1158/0008-5472.CAN-16-0097
Jahchan, N. S., Dudley, J. T., Mazur, P. K., Flores, N., Yang, D., Palmerton, A., et al. (2013). A drug repositioning approach identifies tricyclic antidepressants as inhibitors of small cell lung cancer and other neuroendocrine tumors. Cancer Discov. 3, 1364–1377. doi:10.1158/2159-8290.CD-13-0183
Jernigan, F. E., Hanai, J. ichi, Sukhatme, V. P., and Sun, L. (2017). Discovery of furan carboxylate derivatives as novel inhibitors of ATP-citrate lyase via virtual high-throughput screening. Bioorg. Med. Chem. Lett. 27, 929–935. doi:10.1016/j.bmcl.2017.01.001
Jiang, P., Huang, S., Fu, Z., Sun, Z., Lakowski, T. M., and Hu, P. (2020). Deep graph embedding for prioritizing synergistic anticancer drug combinations. Comput. Struct. Biotechnol. J. 18, 427–438. doi:10.1016/j.csbj.2020.02.006
Jiang, W., Li, X., Li, T., Wang, H., Shi, W., Qi, P., et al. (2017). Repositioning of amprenavir as a novel extracellular signal-regulated kinase-2 inhibitor and apoptosis inducer in MCF-7 human breast cancer. Int. J. Oncol. 50, 823–834. doi:10.3892/ijo.2017.3860
Jordheim, L. P., Marton, Z., Rhimi, M., Cros-Perrial, E., Lionne, C., Peyrottes, S., et al. (2013). Identification and characterization of inhibitors of cytoplasmic 5′-nucleotidase cN-II issued from virtual screening. Biochem. Pharmacol. 85, 497–506. doi:10.1016/j.bcp.2012.11.024
Jubie, S., Selvaraj, A., Neethu, Y., Parvesh, B., Puru, K., Shreyan, S., et al. (2018). Early stage repurposing of benzimidazole scaffolds towards breast cancer through in-silico tools. J. Pharm. Sci. Res. 10, 2419–2423.
Juritz, E. I., Bascur, J. P., Almonacid, D. E., and González-Nilo, F. D. (2018). Novel insights for inhibiting mutant heterodimer IDH1wt-r132H in cancer: an in-silico approach. Mol. Diagn. Ther. 22, 369–380. doi:10.1007/s40291-018-0331-2
Kabir, E. R., Mustafa, N., Kawsar, M., Siam, S., and Kabir, S. M. (2018). Molecular docking reveals pitavastatin and related molecules antagonize 1DHF and its pseudogene DHFR2 in cancer treatment. ACM Int. Conf. Proceeding Ser. doi:10.1145/3291757.3291763
Kabir, E. R., Siam, M. K. S., and Mustafa, N. (2019). “Scaffold of N -(2-(2-(tosylcarbamoyl)hydrazinyl)ethyl)isonicotinamidereveals anticancer effects through selective inhibition of FAP,” in Proceedings of the tenth international conference on computational systems-biology and bioinformatics (New York, NY, USA: ACM), 1–11. doi:10.1145/3365953.3365963
Kabir, E. R., Siami, M. K. S., Kabir, S. M., Khan, A., and Rajib, S. A. (2017). Drug repurposing: targeting mTOR inhibitors for anticancer activity. ACM Int. Conf. Proceeding Ser., 68–75. doi:10.1145/3156346.3156359
Kadioglu, O., Elbadawi, M., Fleischer, E., and Efferth, T. (2021). Identification of novel anthracycline resistance genes and their inhibitors. Pharmaceuticals 14, 1051–1113. doi:10.3390/ph14101051
Kanematsu, S., Tanimoto, K., Suzuki, Y., and Sugano, S. (2014). Screening for possible miRNA-mRNA associations in a colon cancer cell line. Gene 533, 520–531. doi:10.1016/j.gene.2013.08.005
Kang, S., Lee, J. M., Jeon, B., Elkamhawy, A., Paik, S., Hong, J., et al. (2018). Repositioning of the antipsychotic trifluoperazine: synthesis, biological evaluation and in silico study of trifluoperazine analogs as anti-glioblastoma agents. Eur. J. Med. Chem. 151, 186–198. doi:10.1016/j.ejmech.2018.03.055
Karthik, L., and Vijayakumar, B. (2020). Screening of anti-cancer properties of beta- sitosterol and its derivatives against microtubules: molecular modeling approach. Int. J. Pharm. Phytopharm. Res. 10, 8–21.
Karube, K., Enjuanes, A., Dlouhy, I., Jares, P., Martin-Garcia, D., Nadeu, F., et al. (2018). Integrating genomic alterations in diffuse large B-cell lymphoma identifies new relevant pathways and potential therapeutic targets. Leukemia 32, 675–684. doi:10.1038/leu.2017.251
Kaur, H., Ahmad, N., and Chauhan, R. (2015). In-silico study of computational modelling and GLP-1 receptor inverse agonist compounds on a cancer cell line inhibitory bioassay dataset. Int. J. Comput. Biol. Drug Des. 8, 293–309. doi:10.1504/IJCBDD.2015.072116
Ke, K., Li, H., Yao, H., Shi, X. N., Dong, C., Zhu, Y., et al. (2017). In silico prediction and in vitro and in vivo validation of acaricide fluazuron as a potential inhibitor of FGFR3 and a candidate anticancer drug for bladder carcinoma. Chem. Biol. Drug Des. 89, 505–513. doi:10.1111/cbdd.12872
Khanam, U., Malik, B. K., and Rathi, B. (2017). Identification of human camp dependent protein kinase catalytic subunit inhibitors. IJPSR 8, 3452–3457. doi:10.13040/IJPSR.0975-8232.8(8).3452-57
Klingenberg, M., Groß, M., Goyal, A., Polycarpou-Schwarz, M., Miersch, T., and Ernst, A.-S. (2017). The lncRNA CASC9 and RNA binding protein HNRNPL form a complex and co-regulate genes linked to AKT signaling. Hepatology 777, 1–36. doi:10.1002/hep
Ko, Y. (2020). Computational drug repositioning: current progress and challenges. Appl. Sci. 10, 5076. doi:10.3390/app10155076
Kolosenko, I., Yu, Y., Busker, S., Dyczynski, M., Liu, J., Haraldsson, M., et al. (2017). Identification of novel small molecules that inhibit STAT3-dependent transcription and function. PLoS One 12, 01788444–e178921. doi:10.1371/journal.pone.0178844
Konidala, K. K., Bommu, U. D., Yeguvapalli, S., and Pabbaraju, N. (2018). In silico insights into prediction and analysis of potential novel pyrrolopyridine analogs against human MAPKAPK-2: a new SAR-based hierarchical clustering approach. 3 Biotech. 8, 385. doi:10.1007/s13205-018-1405-x
Krishna, S., Kumar, S., Singh, D. K., Lakra, A. D., Banerjee, D., and Siddiqi, M. I. (2018). Multiple machine learning based-chemoinformatics models for identification of histone acetyl transferase inhibitors. Mol. Inf. 37, 17001500–e1700216. doi:10.1002/minf.201700150
Krishnamurthy, N., Grimshaw, A. A., Axson, S. A., Choe, S. H., and Miller, J. E. (2022). Drug repurposing: a systematic review on root causes, barriers and facilitators. BMC Health Serv. Res. 22, 970. doi:10.1186/s12913-022-08272-z
Kumar, A., Rathi, E., and Kini, S. G. (2020). Drug repurposing approach for the identification and designing of potential E6 inhibitors against cervical cancer: an in silico investigation. Struct. Chem. 31, 141–153. doi:10.1007/s11224-019-01378-x
Kuthuru, S., Szafran, A. T., Stossi, F., Mancini, M. A., and Rao, A. (2019). Leveraging image-derived phenotypic measurements for drug-target interaction predictions. Cancer Inf. 18, 1176935119856595. doi:10.1177/1176935119856595
Kwon, O. S., Lee, H., Kong, H. J., Kwon, E. J., Park, J. E., Lee, W., et al. (2020). Connectivity map-based drug repositioning of bortezomib to reverse the metastatic effect of GALNT14 in lung cancer. Oncogene 39, 4567–4580. doi:10.1038/s41388-020-1316-2
Lafleur, K., Dong, J., Huang, D., Caflisch, A., and Nevado, C. (2013). Optimization of inhibitors of the tyrosine kinase EphB4. 2. Cellular potency improvement and binding mode validation by X-ray crystallography. J. Med. Chem. 56, 84–96. doi:10.1021/jm301187e
Lagarde, N., Rey, J., Gyulkhandanyan, A., Tufféry, P., Miteva, M. A., and Villoutreix, B. O. (2018). Online structure-based screening of purchasable approved drugs and natural compounds: retrospective examples of drug repositioning on cancer targets. Oncotarget 9, 32346–32361. doi:10.18632/oncotarget.25966
Landry, L. G., Ali, N., Williams, D. R., Rehm, H. L., and Bonham, V. L. (2018). Lack of diversity in genomic databases is a barrier to translating precision medicine research into practice. Health Aff. 37, 780–785. doi:10.1377/hlthaff.2017.1595
Lara-Castillo, M. C., Cornet-Masana, J. M., Etxabe, A., Banús-Mulet, A., Torrente, M. Á., Nomdedeu, M., et al. (2016). Repositioning of bromocriptine for treatment of acute myeloid leukemia. J. Transl. Med. 14, 261–310. doi:10.1186/s12967-016-1007-5
Lau, A., and So, H. C. (2020). Turning genome-wide association study findings into opportunities for drug repositioning. Comput. Struct. Biotechnol. J. 18, 1639–1650. doi:10.1016/j.csbj.2020.06.015
Laurinavichyute, A., Yadav, H., and Vasishth, S. (2022). Share the code, not just the data: a case study of the reproducibility of articles published in the Journal of Memory and Language under the open data policy. J. Mem. Lang. 125, 104332. doi:10.1016/j.jml.2022.104332
Lee, H., Kang, S., and Kim, W. (2016). Drug repositioning for cancer therapy based on large-scale drug-induced transcriptional signatures. PLoS One 11, 01504600–e150517. doi:10.1371/journal.pone.0150460
Leung, C. H., Chan, D. S. H., Li, Y. W., Fong, W. F., and Ma, D. L. (2013). Hit identification of IKKβ natural product inhibitor. BMC Pharmacol. Toxicol. 14, 3–7. doi:10.1186/2050-6511-14-3
Leung, W. H., Shih, J. W., Chen, J. S., Mokgautsi, N., Wei, P. L., and Huang, Y. J. (2022). Preclinical identification of sulfasalazine’s therapeutic potential for suppressing colorectal cancer stemness and metastasis through targeting KRAS/MMP7/CD44 signaling. Biomedicines 10, 377. doi:10.3390/biomedicines10020377
Li, H., Wang, Z., Liang, S., Liu, Q., Wang, P., Cai, L., et al. (2021). Radiation induces epithelial to mesenchymal transition via upregulation of PD-L1 in nasopharyngeal carcinoma cell. Transl. Cancer Res. 10, 372–381. doi:10.21037/tcr-20-1899
Li, X., Payne, D. T., Ampolu, B., Bland, N., Brown, J. T., Dutton, M. J., et al. (2019). Derivatisation of parthenolide to address chemoresistant chronic lymphocytic leukaemia. Medchemcomm 10, 1379–1390. doi:10.1039/c9md00297a
Liao, C., Wang, Y., Tan, X., Sun, L., and Liu, S. (2015). Discovery of novel inhibitors of human S-adenosylmethionine decarboxylase based on in silico high-throughput screening and a non-radioactive enzymatic assay. Sci. Rep. 5, 10754–10811. doi:10.1038/srep10754
Lim, N., Tesar, S., Belmadani, M., Poirier-Morency, G., Mancarci, B. O., Sicherman, J., et al. (2021). Curation of over 10 000 transcriptomic studies to enable data reuse. Database 2021, baab006–23. doi:10.1093/database/baab006
Lin, W. Z., Liu, Y. C., Lee, M. C., Tang, C. T., Wu, G. J., Chang, Y. T., et al. (2022). From GWAS to drug screening: repurposing antipsychotics for glioblastoma. J. Transl. Med. 20, 70–13. doi:10.1186/s12967-021-03209-2
Liñares-Blanco, J., Munteanu, C. R., Pazos, A., and Fernandez-Lozano, C. (2020). Molecular docking and machine learning analysis of Abemaciclib in colon cancer. BMC Mol. Cell Biol. 21, 52–18. doi:10.1186/s12860-020-00295-w
Liu, J., Huang, J., and Ma, S. (2013). Incorporating network structure in integrative analysis of cancer prognosis data. Genet. Epidemiol. 37, 173–183. doi:10.1002/gepi.21697
Liu, J., Zhu, Y., He, Y., Zhu, H., Gao, Y., Li, Z., et al. (2020). Combined pharmacophore modeling, 3D-QSAR and docking studies to identify novel HDAC inhibitors using drug repurposing. J. Biomol. Struct. Dyn. 38, 533–547. doi:10.1080/07391102.2019.1590241
Liu, X., Yang, X., Chen, X., Zhang, Y., Pan, X., Wang, G. P., et al. (2015). Expression profiling identifies bezafibrate as potential therapeutic drug for lung adenocarcinoma. J. Cancer 6, 1214–1221. doi:10.7150/jca.12191
Liu, Z., Chen, X., Roberts, R., Huang, R., Mikailov, M., and Tong, W. (2021). Unraveling gene fusions for drug repositioning in high-risk neuroblastoma. Front. Pharmacol. 12, 608778–608818. doi:10.3389/fphar.2021.608778
Lodagekar, A., Borkar, R. M., Thatikonda, S., Chavan, R. B., Naidu, V. G. M., Shastri, N. R., et al. (2019). Formulation and evaluation of cyclodextrin complexes for improved anticancer activity of repurposed drug: niclosamide. Carbohydr. Polym. 212, 252–259. doi:10.1016/j.carbpol.2019.02.041
Madhukar, G., and Subbarao, N. (2022). In-silico prediction of potential inhibitors against phosphatidylinositol 3-kinase catalytic subunit alpha involved in head and neck squamous cell carcinomas. J. Biomol. Struct. Dyn. 40, 4697–4712. doi:10.1080/07391102.2020.1861980
Mady, A. S. A., Liao, C., Bajwa, N., Kump, K. J., Abulwerdi, F. A., Lev, K. L., et al. (2018). Discovery of Mcl-1 inhibitors from integrated high throughput and virtual screening. Sci. Rep. 8, 10210–10215. doi:10.1038/s41598-018-27899-9
Manohar, C. S., Manikandan, A., Sridhar, P., Sivakumar, A., Siva Kumar, B., and Reddy, S. R. (2018). Drug repurposing of novel quinoline acetohydrazide derivatives as potent COX-2 inhibitors and anti-cancer agents. J. Mol. Struct. 1154, 437–444. doi:10.1016/j.molstruc.2017.10.075
Martin, L., Hutchens, M., Hawkins, C., and Radnov, A. (2017). How much do clinical trials cost? Nat. Rev. Drug Discov. 16, 381–382. doi:10.1038/nrd.2017.70
Martínez-García, M., and Hernandez-Lemus, E. (2021). Pathway-based drug-repurposing schemes in cancer: the role of translational bioinformatics. Front. Oncol. 10, 605680–605715. doi:10.3389/fonc.2020.605680
McNeil, E. M., Astell, K. R., Ritchie, A. M., Shave, S., Houston, D. R., Bakrania, P., et al. (2015). Inhibition of the ERCC1-XPF structure-specific endonuclease to overcome cancer chemoresistance. DNA Repair (Amst) 31, 19–28. doi:10.1016/j.dnarep.2015.04.002
Menden, M. P., Iorio, F., Garnett, M., McDermott, U., Benes, C. H., Ballester, P. J., et al. (2013). Machine learning prediction of cancer cell sensitivity to drugs based on genomic and chemical properties. PLoS One 8, e61318. doi:10.1371/journal.pone.0061318
Meng, X.-Y., Zhang, H.-X., Mezei, M., and Cui, M. (2011). Molecular docking: a powerful approach for structure-based drug discovery. Curr. Comput. Aided-Drug Des. 7, 146–157. doi:10.2174/157340911795677602
Metz, P., Tjan, M. J. H., Wu, S., Pervaiz, M., Hermans, S., Shettigar, A., et al. (2019). Drug discovery and repurposing inhibits a major gut pathogen-derived oncogenic toxin. Front. Cell. Infect. Microbiol. 9, 364–369. doi:10.3389/fcimb.2019.00364
Mirza, A. N., Fry, M. A., Urman, N. M., Atwood, S. X., Roffey, J., Ott, G. R., et al. (2017). Combined inhibition of atypical PKC and histone deacetylase 1 is cooperative in basal cell carcinoma treatment. JCI Insight 2, 970711–e97112. doi:10.1172/jci.insight.97071
Mishra, R. K., Clutter, M. R., Blyth, G. T., Kosciuczuk, E. M., Blackburn, A. Z., Beauchamp, E. M., et al. (2019). Discovery of novel Mnk inhibitors using mutation-based induced-fit virtual high-throughput screening. Chem. Biol. Drug Des. 94, 1813–1823. doi:10.1111/cbdd.13585
Moher, D., Shamseer, L., Clarke, M., Ghersi, D., Liberati, A., Petticrew, M., et al. (2015). Preferred reporting items for systematic review and meta-analysis protocols (PRISMA-P) 2015 statement. Syst. Rev. 4, 1. doi:10.1186/2046-4053-4-1
Montes-Grajales, D., Bernardes, G. J. L., and Olivero-Verbel, J. (2016). Urban endocrine disruptors targeting breast cancer proteins. Chem. Res. Toxicol. 29, 150–161. doi:10.1021/acs.chemrestox.5b00342
Montes-Grajales, D., Martínez-Romero, E., and Olivero-Verbel, J. (2018). Phytoestrogens and mycoestrogens interacting with breast cancer proteins. Steroids 134, 9–15. doi:10.1016/j.steroids.2018.03.010
Mottini, C., Napolitano, F., Li, Z., Gao, X., and Cardone, L. (2021). Computer-aided drug repurposing for cancer therapy: approaches and opportunities to challenge anticancer targets. Semin. Cancer Biol. 68, 59–74. doi:10.1016/j.semcancer.2019.09.023
Mottini, C., Tomihara, H., Carrella, D., Lamolinara, A., Iezzi, M., Huang, J. K., et al. (2019). Predictive signatures inform the effective repurposing of decitabine to treat KRAS-dependent pancreatic ductal adenocarcinoma. Cancer Res. 79, 5612–5625. doi:10.1158/0008-5472.CAN-19-0187
Mould, D. P., Alli, C., Bremberg, U., Cartic, S., Jordan, A. M., Geitmann, M., et al. (2017). Development of (4-cyanophenyl)glycine derivatives as reversible inhibitors of lysine specific demethylase 1. J. Med. Chem. 60, 7984–7999. doi:10.1021/acs.jmedchem.7b00462
Naeem, A., Dakshanamurthy, S., Walthieu, H., Parasido, E., Avantaggiati, M., Tricoli, L., et al. (2020). Predicting new drug indications for prostate cancer: the integration of an in silico proteochemometric network pharmacology platform with patient-derived primary prostate cells. Prostate 80, 1233–1243. doi:10.1002/pros.24050
Nagamalla, L., and Kumar, J. V. S. (2021). In silico screening of FDA approved drugs on AXL kinase and validation for breast cancer cell line. J. Biomol. Struct. Dyn. 39, 2056–2070. doi:10.1080/07391102.2020.1742791
Nakamura, Y., Suganami, A., Fukuda, M., Hasan, M. K. amru., Yokochi, T., Takatori, A., et al. (2014). Identification of novel candidate compounds targeting TrkB to induce apoptosis in neuroblastoma. Cancer Med. 3, 25–35. doi:10.1002/cam4.175
Naresh, R., Nazeer, Y., and Palani, S. (2013). In silico evaluation of modes of action of anticancer compounds on molecular targets for cancer. Med. Chem. Res. 22, 1938–1947. doi:10.1007/s00044-012-0198-4
Nasiri, F., Atanaki, F. F., Behrouzi, S., Kavousi, K., and Bagheri, M. (2021). CpACpP: in silico cell-penetrating anticancer peptide prediction using a novel bioinformatics framework. ACS Omega 6, 19846–19859. doi:10.1021/acsomega.1c02569
Natarajan, A., Thangarajan, R., and Kesavan, S. (2019). Repurposing drugs by in silico methods to target BCR kinase domain in chronic myeloid leukemia. Asian Pac. J. Cancer Prev. 20, 3399–3406. doi:10.31557/APJCP.2019.20.11.3399
Omotuyi, I. O., Elekofehinti, O. O., Ejelonu, O. C., and Kamdem, J. P. (2013). Combined peptidomimetic and high throughput virtual screening identify novel inhibitors of fascin-catalyzed actin-bundling. Int. J. Pharm. Pharm. Sci. 5, 211–220.
Ozsvari, B., Sotgia, F., Simmons, K., Trowbridge, R., Foster, R., and Lisanti, M. P. (2017). Mitoketoscins: novel mitochondrial inhibitors for targeting ketone metabolism in cancer stem cells (CSCs). Oncotarget 8, 78340–78350. doi:10.18632/oncotarget.21259
Page, M. J., McKenzie, J. E., Bossuyt, P. M., Boutron, I., Hoffmann, T. C., Mulrow, C. D., et al. (2021). The PRISMA 2020 statement: an updated guideline for reporting systematic reviews. BMJ 372, n71. doi:10.1136/bmj.n71
Palve, V., Liao, Y., Remsing Rix, L. L., and Rix, U. (2021). Turning liabilities into opportunities: off-target based drug repurposing in cancer. Semin. Cancer Biol. 68, 209–229. doi:10.1016/j.semcancer.2020.02.003
Parcha, P. K., Sarvagalla, S., Ashok, C., Sudharshan, S. J., Dyavaiah, M., Coumar, M. S., et al. (2021). Repositioning antispasmodic drug Papaverine for the treatment of chronic myeloid leukemia. Pharmacol. Rep. 73, 615–628. doi:10.1007/s43440-020-00196-x
Parks, A. J., Pollastri, M. P., Hahn, M. E., Stanford, E. A., Novikov, O., Franks, D. G., et al. (2014). In silico identification of an aryl hydrocarbon receptor antagonist with biological activity in vitro and in vivo. Mol. Pharmacol. 86, 593–608. doi:10.1124/mol.114.093369
Parveen, S. (2021). In silico drug repurposing of fda-approved artemisinins as potent chemotherapeutics targeting BCL-2, CDK-6 and VEGFR-2: density functional exploration and molecular docking study. Biointerface Res. Appl. Chem. 11, 9604–9618. doi:10.33263/BRIAC112.96049618
Patidar, K., Panwar, U., Vuree, S., Sweta, J., Sandhu, M. K., Nayarisseri, A., et al. (2019). An in silico approach to identify high affinity small molecule targeting m-TOR inhibitors for the clinical treatment of breast cancer. Asian Pac. J. Cancer Prev. 20, 1229–1241. doi:10.31557/APJCP.2019.20.4.1229
Paula, S., Hofmann, E., Burden, J., and Stanton, D. T. (2015). Novel phenolic inhibitors of the sarco/endoplasmic reticulum calcium ATPase: identification and characterization by quantitative structure-activity relationship modeling and virtual screening. J. Enzyme Inhib. Med. Chem. 30, 1–8. doi:10.3109/14756366.2013.866659
Pessetto, Z. Y., Chen, B., Alturkmani, H., Hyter, S., Flynn, C. A., Baltezor, M., et al. (2017). In silico and in vitro drug screening identifies new therapeutic approaches for Ewing sarcoma. Oncotarget 8, 4079–4095. doi:10.18632/oncotarget.13385
Peters, M. D. J., Godfrey, C. M., Khalil, H., McInerney, P., Parker, D., and Soares, C. B. (2015). Guidance for conducting systematic scoping reviews. Int. J. Evid. Based. Healthc. 13, 141–146. doi:10.1097/XEB.0000000000000050
Pinzi, L., and Rastelli, G. (2019). Molecular docking: shifting paradigms in drug discovery. Int. J. Mol. Sci. 20, 4331. doi:10.3390/ijms20184331
Platonova, N., Parravicini, C., Sensi, C., Paoli, A., Colombo, M., Neri, A., et al. (2017). Identification of small molecules uncoupling the Notch::Jagged interaction through an integrated high-throughput screening. PLoS One 12, 01826400–e182718. doi:10.1371/journal.pone.0182640
Poli, G., Lapillo, M., Jha, V., Mouawad, N., Caligiuri, I., Macchia, M., et al. (2019). Computationally driven discovery of phenyl(piperazin-1-yl)methanone derivatives as reversible monoacylglycerol lipase (MAGL) inhibitors. J. Enzyme Inhib. Med. Chem. 34, 589–596. doi:10.1080/14756366.2019.1571271
Prabhu, L., Wei, H., Chen, L., Demir, Ö., Sandusky, G., Sun, E., et al. (2017). Adapting AlphaLISA high throughput screen to discover a novel small-molecule inhibitor targeting protein arginine methyltransferase 5 in pancreatic and colorectal cancers. Oncotarget 8, 39963–39977. doi:10.18632/oncotarget.18102
Prakash, O., and Nath Dwivedi, U. (2020). Identification of repurposed protein kinase B binders from FDA-approved drug library: a hybrid–structure activity relationship and systems modeling based approach. J. Biomol. Struct. Dyn. 38, 660–672. doi:10.1080/07391102.2019.1585293
Pushpakom, S., Iorio, F., Eyers, P. A., Escott, K. J., Hopper, S., Wells, A., et al. (2018). Drug repurposing: progress, challenges and recommendations. Nat. Publ. Gr. 18, 41–58. doi:10.1038/nrd.2018.168
Qi, B., Zhong, L., He, J., Zhang, H., Li, F., Wang, T., et al. (2019). Discovery of inhibitors of aurora/PLK targets as anticancer agents. J. Med. Chem. 62, 7697–7707. doi:10.1021/acs.jmedchem.9b00353
Rabben, H. L., Andersen, G. T., Ianevski, A., Olsen, M. K., Kainov, D., Grønbech, J. E., et al. (2021). Computational drug repositioning and experimental validation of ivermectin in treatment of gastric cancer. Front. Pharmacol. 12, 625991–626014. doi:10.3389/fphar.2021.625991
Radaeva, M., Ban, F., Zhang, F., Leblanc, E., Lallous, N., Rennie, P. S., et al. (2021). Development of novel inhibitors targeting the d-box of the dna binding domain of androgen receptor. Int. J. Mol. Sci. 22, 2493–2510. doi:10.3390/ijms22052493
Radi, M., Schneider, R., Fallacara, A. L., Botta, L., Crespan, E., Tintori, C., et al. (2016). A cascade screening approach for the identification of Bcr-Abl myristate pocket binders active against wild type and T315I mutant. Bioorg. Med. Chem. Lett. 26, 3436–3440. doi:10.1016/j.bmcl.2016.06.051
Rameseder, J., Krismer, K., Dayma, Y., Ehrenberger, T., Hwang, M. K., Airoldi, E. M., et al. (2015). A multivariate computational method to analyze high-content RNAi screening data. J. Biomol. Screen. 20, 985–997. doi:10.1177/1087057115583037
Ramos, J., Muthukumaran, J., Freire, F., Paquete-Ferreira, J., Otrelo-Cardoso, A. R., Svergun, D., et al. (2019). Shedding light on the interaction of human anti-apoptotic Bcl-2 protein with ligands through biophysical and in silico studies. Int. J. Mol. Sci. 20, 860. doi:10.3390/ijms20040860
Rashidieh, B., Molakarimi, M., Mohseni, A., Tria, S. M., Truong, H., Srihari, S., et al. (2021). Targeting brf2 in cancer using repurposed drugs. Cancers (Basel) 13, 3778. doi:10.3390/cancers13153778
Renna, R. K., Rodrigues, C. T., Jean, J. C., Paradela, L. S., Dias, M. M., Novaes Da Silva, B., et al. (2021). High-throughput screening reveals new glutaminase inhibitor molecules. ACS Pharmacol. Transl. Sci. 4, 1849–1866. doi:10.1021/acsptsci.1c00226
Riddick, G., Song, H., Holbeck, S. L., Kopp, W., Walling, J., Ahn, S., et al. (2015). An in silico screen links gene expression signatures to drug response in glioblastoma stem cells. Pharmacogenomics J. 15, 347–353. doi:10.1038/tpj.2014.61
Rimpelová, S., Zimmermann, T., Drašar, P. B., Dolenský, B., Bejček, J., Kmoníčková, E., et al. (2021). Steroid glycosides hyrcanoside and deglucohyrcanoside: on isolation, structural identification, and anticancer activity. Foods 10, 136. doi:10.3390/foods10010136
Rocca, R., Costa, G., Artese, A., Parrotta, L., Ortuso, F., Maccioni, E., et al. (2016). Hit identification of a novel dual binder for h-telo/c-myc G-quadruplex by a combination of pharmacophore structure-based virtual screening and docking refinement. ChemMedChem 11, 1721–1733. doi:10.1002/cmdc.201600053
Rubio-Perez, C., Tamborero, D., Schroeder, M. P., Antolín, A. A., Deu-Pons, J., Perez-Llamas, C., et al. (2015). In silico prescription of anticancer drugs to cohorts of 28 tumor types reveals targeting opportunities. Cancer Cell 27, 382–396. doi:10.1016/j.ccell.2015.02.007
Sahrawat, T. R., and Kaur, P. (2019). Polypharmacological study of Ceritinib using a structure based in silico approach. Bionatura 4, 836–840. doi:10.21931/RB/2019.04.02.3
Sakr, T. M., Khedr, M. A., Rashed, H. M., and Mohamed, M. E. (2018). In silico-based repositioning of phosphinothricin as a novel technetium-99m imaging probe with potential anti-cancer activity. Molecules 23, 496–516. doi:10.3390/molecules23020496
Salentin, S., Adasme, M. F., Heinrich, J. C., Haupt, V. J., Daminelli, S., Zhang, Y., et al. (2017). From malaria to cancer: computational drug repositioning of amodiaquine using PLIP interaction patterns. Sci. Rep. 7, 11401–11413. doi:10.1038/s41598-017-11924-4
Salim, K. Y., Vareki, S. M., Danter, W. R., San-Marina, S., and Koropatnick, J. (2017). COTI-2, a novel small molecule that is active against multiple human cancer cell lines in vitro and in vivo. Oncotarget 8, 60724. doi:10.18632/oncotarget.20600
Saranyadevi, S. (2021). Repurposing natural compounds as potent inhibitors of β-catenin: an integrative in-silico approaches. Netw. Model. Anal. Heal. Inf. Bioinforma. 10, 38. doi:10.1007/s13721-021-00320-8
Sarkar, S., Horn, G., Moulton, K., Oza, A., Byler, S., Kokolus, S., et al. (2013). Cancer development, progression, and therapy: an epigenetic overview. Int. J. Mol. Sci. 14, 21087–21113. doi:10.3390/ijms141021087
Sarvagalla, S., Singh, V. K., Ke, Y. Y., Shiao, H. Y., Lin, W. H., Hsieh, H. P., et al. (2015). Identification of ligand efficient, fragment-like hits from an HTS library: structure-based virtual screening and docking investigations of 2H- and 3H-pyrazolo tautomers for Aurora kinase A selectivity. J. Comput. Aided. Mol. Des. 29, 89–100. doi:10.1007/s10822-014-9807-2
Schonbrunn, E., Betzi, S., Alam, R., Martin, M. P., Becker, A., Han, H., et al. (2013). Development of highly potent and selective diaminothiazole inhibitors of cyclin-dependent kinases. J. Med. Chem. 56, 3768–3782. doi:10.1021/jm301234k
Segura-Cabrera, A., Singh, N., and Komurov, K. (2015). An integrated network platform for contextual prioritization of drugs and pathways. Mol. Biosyst. 11, 2850–2859. doi:10.1039/c5mb00444f
Seibold, H., Czerny, S., Decke, S., Dieterle, R., Eder, T., Fohr, S., et al. (2021). A computational reproducibility study of PLOS ONE articles featuring longitudinal data analyses. PLoS One 16, 02511944–e251215. doi:10.1371/journal.pone.0251194
Seyhan, A. A. (2019). Lost in translation: the valley of death across preclinical and clinical divide – identification of problems and overcoming obstacles. Transl. Med. Commun. 4, 18–19. doi:10.1186/s41231-019-0050-7
Shafique, S., Bibi, N., and Rashid, S. (2016). In silico identification of putative bifunctional Plk1 inhibitors by integrative virtual screening and structural dynamics approach. J. Theor. Biol. 388, 72–84. doi:10.1016/j.jtbi.2015.10.006
Shah, S. P., Roth, A., Goya, R., Oloumi, A., Ha, G., Zhao, Y., et al. (2012). The clonal and mutational evolution spectrum of primary triple-negative breast cancers. Nature 486, 395–399. doi:10.1038/nature10933
Shehadi, I. A., Rashdan, H. R. M., and Abdelmonsef, A. H. (2020). Homology modeling and virtual screening studies of antigen MLAA-42Protein: identification of noveldrugcandidates against leukemia-an in silico approach. Comput. Math. Methods Med. 2020, 8196147. doi:10.1155/2020/8196147
Shi, Z., Chen, J., Guo, X., Cheng, L., Guo, X., and Yu, T. (2018). In silico identification of potent small molecule inhibitors targeting epidermal growth factor receptor 1. J. Cancer Res. Ther. 14, 18–23. doi:10.4103/jcrt.JCRT_365_17
Shi, Z., Zhang, J., Qian, X., Han, L., Zhang, K., Chen, L., et al. (2013). AC1MMYR2, an inhibitor of dicer-mediated biogenesis of oncomir miR-21, reverses epithelial–mesenchymal transition and suppresses tumor growth and progression. Cancer Res. 73, 5519–5531. doi:10.1158/0008-5472.CAN-13-0280
Shin, M., Jang, D., Nam, H., Lee, K. H., and Lee, D. (2018). Predicting the absorption potential of chemical compounds through a deep learning approach. IEEE/ACM Trans. Comput. Biol. Bioinforma. 15, 432–440. doi:10.1109/TCBB.2016.2535233
Shlyakhtina, Y., Moran, K. L., and Portal, M. M. (2021). Genetic and non-genetic mechanisms underlying cancer evolution. Cancers (Basel) 13, 1380–1442. doi:10.3390/cancers13061380
Siam, M. K. S., Karim, A., and Shohan, M. U. S. (2020). In-silico study for potential inhibitors of both HSP72 and HSC70 proteins in the treatment of cancer. ACM Int. Conf. Proceeding Ser., 61–67. doi:10.1145/3429210.3429226
Simoens, S., and Huys, I. (2021). R&D costs of new medicines: a landscape analysis. Front. Med. 8, 1–6. doi:10.3389/fmed.2021.760762
Singh, S., Das, S., Pandey, A., Sharma, S., and Paliwal, S. (2013). Pharmacophore-based in silico high-throughput screening to identify novel topoisomerase-I inhibitors. Med. Chem. Res. 22, 5356–5380. doi:10.1007/s00044-013-0526-3
Sohraby, F., Bagheri, M., Aliyar, M., and Aryapour, H. (2017). In silico drug repurposing of FDA-approved drugs to predict new inhibitors for drug resistant T315I mutant and wild-type BCR-ABL1: a virtual screening and molecular dynamics study. J. Mol. Graph. Model. 74, 234–240. doi:10.1016/j.jmgm.2017.04.005
Song, J., Xu, Z., Cao, L., Wang, M., Hou, Y., and Li, K. (2021). The discovery of new drug-target interactions for breast cancer treatment. Molecules 26, 7474. doi:10.3390/molecules26247474
Song, P. L., Wang, G., Su, Y., Wang, H. X., Wang, J., Li, F., et al. (2019). Strategy and validation of a structure-based method for the discovery of selective inhibitors of PAK isoforms and the evaluation of their anti-cancer activity. Bioorg. Chem. 91, 103168. doi:10.1016/j.bioorg.2019.103168
Spiliotopoulos, D., Wamhoff, E. C., Lolli, G., Rademacher, C., and Caflisch, A. (2017a). Discovery of BAZ2A bromodomain ligands. Eur. J. Med. Chem. 139, 564–572. doi:10.1016/j.ejmech.2017.08.028
Spiliotopoulos, D., Zhu, J., Wamhoff, E. C., Deerain, N., Marchand, J. R., Aretz, J., et al. (2017b). Virtual screen to NMR (VS2NMR): discovery of fragment hits for the CBP bromodomain. Bioorg. Med. Chem. Lett. 27, 2472–2478. doi:10.1016/j.bmcl.2017.04.001
Stachnik, A., Yuen, T., Iqbal, J., Sgobba, M., Gupta, Y., Lu, P., et al. (2014). Repurposing of bisphosphonates for the prevention and therapy of nonsmall cell lung and breast cancer. Proc. Natl. Acad. Sci. U. S. A. 111, 17995–18000. doi:10.1073/pnas.1421422111
Strätker, K., Haidar, S., Amesty, Á., El-Awaad, E., Götz, C., Estévez-Braun, A., et al. (2020). Development of an in vitro screening assay for PIP5K1α lipid kinase and identification of potent inhibitors. FEBS J. 287, 3042–3064. doi:10.1111/febs.15194
Sulsenti, R., Frossi, B., Bongiovanni, L., Cancila, V., Ostano, P., Fischetti, I., et al. (2021). Repurposing of the antiepileptic drug levetiracetam to restrain neuroendocrine prostate cancer and inhibit mast cell support to adenocarcinoma. Front. Immunol. 12, 622001–622015. doi:10.3389/fimmu.2021.622001
Sun, D., Gao, W., Hu, H., and Zhou, S. (2022). Why 90% of clinical drug development fails and how to improve it? Acta Pharm. Sin. B 12, 3049–3062. doi:10.1016/j.apsb.2022.02.002
Suphavilai, C., Chia, S., Sharma, A., Tu, L., Da Silva, R. P., Mongia, A., et al. (2021). Predicting heterogeneity in clone-specific therapeutic vulnerabilities using single-cell transcriptomic signatures. Genome Med. 13, 189–214. doi:10.1186/s13073-021-01000-y
Sweta, J., Khandelwal, R., Srinitha, S., Pancholi, R., Adhikary, R., Ali, M. A., et al. (2019). Identification of high-affinity small molecule targeting IDH2 for the clinical treatment of acute myeloid leukemia. Asian Pac. J. Cancer Prev. 20, 2287–2297. doi:10.31557/APJCP.2019.20.8.2287
Tabchy, A., Eltonsy, N., Housman, D. E., and Mills, G. B. (2013). Systematic identification of combinatorial drivers and targets in cancer cell lines. PLoS One 8, e60339. doi:10.1371/journal.pone.0060339
Takeuchi, M., Niimi, T., Masumoto, M., Orita, M., Yokota, H., and Yamamoto, T. (2014). Discovery of a novel nicotinamide phosphoribosyl transferase (nampt) inhibitor via in silico screening. Biol. Pharm. Bull. 37, 31–36. doi:10.1248/bpb.b13-00495
Tan, M. (2016). Prediction of anti-cancer drug response by kernelized multi-task learning. Artif. Intell. Med. 73, 70–77. doi:10.1016/j.artmed.2016.09.004
Tartaglia, L. A. (2006). Complementary new approaches enable repositioning of failed drug candidates. Expert Opin. Investig. Drugs 15, 1295–1298. doi:10.1517/13543784.15.11.1295
Tian, K., Bakker, E., Hussain, M., Guazzelli, A., Alhebshi, H., Meysami, P., et al. (2018). P53 modeling as a route to mesothelioma patients stratification and novel therapeutic identification. J. Transl. Med. 16, 282–315. doi:10.1186/s12967-018-1650-0
Traylor, J. I., Sheppard, H. E., Ravikumar, V., Breshears, J., Raza, S. M., Lin, C. Y., et al. (2021). Computational drug repositioning identifies potentially active therapies for chordoma. Neurosurgery 88, 428–436. doi:10.1093/neuros/nyaa398
Turanli, B., Zhang, C., Kim, W., Benfeitas, R., Uhlen, M., Arga, K. Y., et al. (2019). Discovery of therapeutic agents for prostate cancer using genome-scale metabolic modeling and drug repositioning. EBioMedicine 42, 386–396. doi:10.1016/j.ebiom.2019.03.009
Uffelmann, E., Huang, Q. Q., Munung, N. S., de Vries, J., Okada, Y., Martin, A. R., et al. (2021). Genome-wide association studies. Nat. Rev. Methods Prim. 1, 59. doi:10.1038/s43586-021-00056-9
Ulfa, D. M., Arsianti, A., and Radji, M. (2017). In silico docking studies of gallic acid structural analogs as Bcl-Xl inhibitor in cancer. Asian J. Pharm. Clin. Res. 10, 119–122. doi:10.22159/ajpcr.2017.v10i4.16269
Van Kesteren, P. C. E., Zwart, P. E., Schaap, M. M., Pronk, T. E., Van Herwijnen, M. H. M., Kleinjans, J. C. S., et al. (2013). Benzo[a]pyrene-induced transcriptomic responses in primary hepatocytes and in vivo liver: toxicokinetics is essential for in vivo-in vitro comparisons. Arch. Toxicol. 87, 505–515. doi:10.1007/s00204-012-0949-5
Velázquez-Quesada, I., Ruiz-Moreno, A. J., Casique-Aguirre, D., Aguirre-Alvarado, C., Cortés-Mendoza, F., de la Fuente-Granada, M., et al. (2020). Pranlukast antagonizes cd49f and reduces stemness in triple-negative breast cancer cells. Drug Des. devel. Ther. 14, 1799–1811. doi:10.2147/DDDT.S247730
Verma, N., Rai, A. K., Kaushik, V., Brünnert, D., Chahar, K. R., Pandey, J., et al. (2016). Identification of gefitinib off-targets using a structure-based systems biology approach; Their validation with reverse docking and retrospective data mining. Sci. Rep. 6, 33949–34012. doi:10.1038/srep33949
Vilaboa, N., Boré, A., Martin-Saavedra, F., Bayford, M., Winfield, N., Firth-Clark, S., et al. (2017). New inhibitor targeting human transcription factor HSF1: effects on the heat shock response and tumor cell survival. Nucleic Acids Res. 45, 5797–5817. doi:10.1093/nar/gkx194
Wakai, E., Suzumura, Y., Ikemura, K., Mizuno, T., Watanabe, M., Takeuchi, K., et al. (2020). An integrated in silico and in vivo approach to identify protective effects of palonosetron in cisplatin-induced nephrotoxicity. Pharmaceuticals 13, 480–513. doi:10.3390/ph13120480
Wang, X., Lu, K., Luo, H., Liang, D., Long, X., Yuan, Y., et al. (2018). In silico identification of small molecules as novel LXR agonists for the treatment of cardiovascular disease and cancer. J. Mol. Model. 24, 57. doi:10.1007/s00894-018-3578-y
Wilson, J. L., Dalin, S., Gosline, S., Hemann, M., Fraenkel, E., and Lauffenburger, D. A. (2016). Pathway-based network modeling finds hidden genes in shRNA screen for regulators of acute lymphoblastic leukemia. Integr. Biol. (United Kingdom) 8, 761–774. doi:10.1039/c6ib00040a
Wu, C. P., Hung, T. H., Hsiao, S. H., Huang, Y. H., Hung, L. C., Yu, Y. J., et al. (2020a). Erdafitinib resensitizes ABCB1-overexpressing multidrug-resistant cancer cells to cytotoxic anticancer drugs. Cancers (Basel) 12, 1366–1418. doi:10.3390/cancers12061366
Wu, C. P., Lusvarghi, S., Wang, J. C., Hsiao, S. H., Huang, Y. H., Hung, T. H., et al. (2020b). The selective class iia histone deacetylase inhibitor TMP195 resensitizes ABCB1-and ABCG2-overexpressing multidrug-resistant cancer cells to cytotoxic anticancer drugs. Int. J. Mol. Sci. 21, 238. doi:10.3390/ijms21010238
Xu, C., Ai, D., Suo, S., Chen, X., Yan, Y., Cao, Y., et al. (2018). Accurate drug repositioning through non-tissue-specific core signatures from cancer transcriptomes. Cell Rep. 25, 523–535.e5. doi:10.1016/j.celrep.2018.09.031
Xu, R., and Wang, Q. Q. (2014). Toward creation of a cancer drug toxicity knowledge base: automatically extracting cancer drug-side effect relationships from the literature. J. Am. Med. Inf. Assoc. 21, 90–96. doi:10.1136/amiajnl-2012-001584
Xue, H., Li, J., Xie, H., and Wang, Y. (2018). Review of drug repositioning approaches and resources. Int. J. Biol. Sci. 14, 1232–1244. doi:10.7150/ijbs.24612
Yadav, M., Khandelwal, R., Mudgal, U., Srinitha, S., Khandekar, N., Nayarisseri, A., et al. (2019). Identification of potent VEGF inhibitors for the clinical treatment of glioblastoma, a virtual screening approach. Asian Pac. J. Cancer Prev. 20, 2681–2692. doi:10.31557/APJCP.2019.20.9.2681
Yang, C., Chen, J., Li, Y., Huang, X., Liu, Z., Wang, J., et al. (2021). Exploring subclass-specific therapeutic agents for hepatocellular carcinoma by informatics-guided drug screen. Brief. Bioinform. 22, bbaa295–11. doi:10.1093/bib/bbaa295
Yang, F., Zhang, Q., Ji, X., Zhang, Y., Li, W., Peng, S., et al. (2022). Machine learning applications in drug repurposing. Interdiscip. Sci. – Comput. Life Sci. 14, 15–21. doi:10.1007/s12539-021-00487-8
Yang, P. M., Chou, C. J., Tseng, S. H., and Hung, C. F. (2017). Bioinformatics and in vitro experimental analyses identify the selective therapeutic potential of interferon gamma and apigenin against cervical squamous cell carcinoma and adenocarcinoma. Oncotarget 8, 46145–46162. doi:10.18632/oncotarget.17574
Yang, X., Huang, W. T., Wu, H. Y., He, R. Q., Ma, J., Liu, A. G., et al. (2019). Novel drug candidate for the treatment of several soft-tissue sarcoma histologic subtypes: a computational method using survival-associated gene signatures for drug repurposing. Oncol. Rep. 41, 2241–2253. doi:10.3892/or.2019.7033
Yeu, Y., Yoon, Y., and Park, S. (2015). Protein localization vector propagation: a method for improving the accuracy of drug repositioning. Mol. Biosyst. 11, 2096–2102. doi:10.1039/c5mb00306g
Yu, L., Zhao, J., and Gao, L. (2018). Predicting potential drugs for breast cancer based on miRNA and tissue specificity. Int. J. Biol. Sci. 14, 971–982. doi:10.7150/ijbs.23350
Zagidullin, B., Wang, Z., Guan, Y., Pitkänen, E., and Tang, J. (2021). Comparative analysis of molecular fingerprints in prediction of drug combination effects. Brief. Bioinform. 22, bbab291–15. doi:10.1093/bib/bbab291
Zalloum, H., Tayyem, R., Abu-Irmaileh, B., Bustanji, Y., Zihlif, M., Mohammad, M., et al. (2015). Discovery of new human epidermal growth factor receptor-2 (HER2) inhibitors for potential use as anticancer agents via ligand-based pharmacophore modeling. J. Mol. Graph. Model. 61, 61–84. doi:10.1016/j.jmgm.2015.06.008
Zeino, M., Paulsen, M. S., Zehl, M., Urban, E., Kopp, B., and Efferth, T. (2015). Identification of new P-glycoprotein inhibitors derived from cardiotonic steroids. Biochem. Pharmacol. 93, 11–24. doi:10.1016/j.bcp.2014.10.009
Zhang, J., Jiang, K., Lv, L., Wang, H., Shen, Z., Gao, Z., et al. (2015). Use of genome-wide association studies for cancer research and drug repositioning. PLoS One 10, 01164777–e116513. doi:10.1371/journal.pone.0116477
Zhang, J., Sun, Y., Zhong, L. Y., Yu, N. N., Ouyang, L., Fang, R. D., et al. (2020a). Structure-based discovery of neoandrographolide as a novel inhibitor of Rab5 to suppress cancer growth. Comput. Struct. Biotechnol. J. 18, 3936–3946. doi:10.1016/j.csbj.2020.11.033
Zhang, M., Tian, J., Wang, R., Song, M., Zhao, R., Chen, H., et al. (2020b). Dasatinib inhibits lung cancer cell growth and patient derived tumor growth in mice by targeting LIMK1. Front. Cell Dev. Biol. 8, 556532–556612. doi:10.3389/fcell.2020.556532
Zhao, R., Fu, L., Yuan, Z., Liu, Y., Zhang, K., Chen, Y., et al. (2021). Discovery of a novel small-molecule inhibitor of Fam20C that induces apoptosis and inhibits migration in triple negative breast cancer. Eur. J. Med. Chem. 210, 113088. doi:10.1016/j.ejmech.2020.113088
Zhong, H. J., Lin, S., Tam, I. L., Lu, L., Chan, D. S. H., Ma, D. L., et al. (2015). In silico identification of natural product inhibitors of JAK2. Methods 71, 21–25. doi:10.1016/j.ymeth.2014.07.003
Keywords: drug repurposing, drug repositioning, cancer, in silico methods, scoping review
Citation: Cavalcante BRR, Freitas RD, Siquara da Rocha L dO, Santos R dSB D, Souza BSdF, Ramos PIP, Rocha GV and Gurgel Rocha CA (2024) In silico approaches for drug repurposing in oncology: a scoping review. Front. Pharmacol. 15:1400029. doi: 10.3389/fphar.2024.1400029
Received: 12 March 2024; Accepted: 14 May 2024;
Published: 11 June 2024.
Edited by:
Vinícius Gonçalves Maltarollo, Federal University of Minas Gerais, BrazilReviewed by:
Alan Talevi, National University of La Plata, ArgentinaSheila Cruz Araujo, Federal University of Minas Gerais, Brazil
Glaucio Monteiro Ferreira, University of São Paulo, Brazil
Copyright © 2024 Cavalcante, Freitas, Siquara da Rocha, Santos, Souza, Ramos, Rocha and Gurgel Rocha. This is an open-access article distributed under the terms of the Creative Commons Attribution License (CC BY). The use, distribution or reproduction in other forums is permitted, provided the original author(s) and the copyright owner(s) are credited and that the original publication in this journal is cited, in accordance with accepted academic practice. No use, distribution or reproduction is permitted which does not comply with these terms.
*Correspondence: Clarissa Araújo Gurgel Rocha, Y2xhcmlzc2EuZ3VyZ2VsQGZpb2NydXouYnI=, Z3VyZ2VsLmNsYXJpc3NhQGdtYWlsLmNvbQ==