- 1Key Laboratory of Environmental Medicine Engineering, Ministry of Education, School of Public Health, Southeast University, Nanjing, China
- 2Shenzhen Institute of Pharmacovigilance and Risk Management, Shenzhen, China
Purpose: This study aimed to characterize the safety profiles of rivaroxaban-associated suspected adverse events by mining the Food and Drug Administration Adverse Event Reporting System (FAERS).
Methods: A disproportionality analysis of spontaneously reported suspected adverse drug reactions (ADRs) was conducted. The reports in FAERS from 2014 to 2024 were compiled. Frequentist and Bayesian statistics were both applied to calculate drug-AE combinations in system organ classes and preferred-term levels. Reporting odds ratio (ROR), proportional reporting ratio (PRR), the Medicines and Healthcare products Regulatory Agency (MHRA), Bayesian confidence propagation neural network (BCPNN), and multi-item gamma Poisson shrinker (MGPS) methods were analyzed and used to compare the suspected AEs.
Results: Of 77,384 ADR reports, 66,705 (86.20%) were serious rivaroxaban AE reports. The most common age group was above 65 years. The suspected adverse effects of rivaroxaban emerging for system organ classes (SOCs) primarily included “Gastrointestinal disorders”; “Injury, poisoning, and procedural complications”, “Nervous system disorders” and “Vascular disorders”. Ranked by EBGM, the top signal strength of suspected AE signals of rivaroxaban under ROR algorithm at the preferred-term (PT) level were “Haemorrhagic arteriovenous malformation” (N = 571, ROR = 756.520, PRR = 754.029, Information Component (IC) = 7.197, Empirical Bayesian Geometric Mean (EBGM) = 146.725), “Gastrointestinal vascular malformation haemorrhagic” (N = 197, ROR = 211.138, PRR = 210.950, IC = 6.614, EBGM = 97.923), and “Diverticulum intestinal haemorrhagic” (N = 722, ROR = 169.898, PRR = 169.210, IC = 6.458, EBGM = 97.920). Moreover, uncommon but significantly suspected AE signals, such as “Coagulation factor X level increased”, “Basal ganglia haematoma”, and “Proctitis haemorrhagic” were observed. Notably, “Gastrointestinal haemorrhage” (N = 13,436, ROR = 80.477, PRR = 74.460, IC = 5.729, EBGM = 53.042), “Upper gastrointestinal haemorrhage”(N = 2,872, ROR = 73.978, PRR = 72.797, IC = 5.706, EBGM = 52.198) and “Internal haemorrhage” (N = 2,368, ROR = 91.979, PRR = 80.899, IC = 5.813, EBGM = 56.212) exhibited relatively high occurrence rates and signal strengths. From 2014 to 2024, the IC values of rivaroxaban-associated suspected AEs for “Surgical and medical procedures” and “Cardiac disorders” showed an annual increasing trend in the time-span analysis. Based on the various visulization plots, a key discovery is that “Gastrointestinal hemorrhage” emerged as the most significant suspected AE across five algorithms. The exciting finding was that the MGPS algorithm revealed a higher risk of suspected AEs under the “Investigations” category. However, the results of the analyses of the other algorithms at the SOC level were not akin to this. Moreover, the results of signal mining for the three main types of indication populations with adverse drug reactions (ADRs), including Atrial fibrillation, Cerebrovascular accident prophylaxis, and Deep vein thrombosis were shown that “Gastrointestinal haemorrhage”, “Epistaxis”, “Haematuria”, “Rectal haemorrhage”, and “Upper gastrointestinal haemorrhage” were detected as the most common and significant signals of suspected adverse events.
Conclusion: Rivaroxaban has risks of various suspected adverse reactions while providing therapeutic effects and being used widely. Our pharmacovigilance study may provide valuable hints that practitioners should closely monitor occurrences of “Gastrointestinal disorders”, “Injury, poisoning, and procedural complications” and “Nervous system disorders”, and other events in clinical applications. Consequently, it remains to persist in monitoring rivaroxaban, assessing the associated risks in the future.
Introduction
Rivaroxaban is a direct inhibitor of Factor Xa (FXa), a drug produced by Bayer Pharma AG based in Germany (Perzborn et al., 2010). The drug has an increased selectivity and comprises a small molecule, which is nearly insoluble in water and has a molecular weight of 436 g/mol. It has high binding for plasma proteins (92%–95%) (albumin as the dominant binding substance) (AG. BP, 2016). In 2008, rivaroxaban was the first new oral direct FXa inhibitor to receive authorization for clinical use. This drug can be used for treating individuals with the prophylaxis of venous thromboembolism (VTE) after elective knee or hip replacement surgical intervention (Mueck et al., 2008). Additionally, it is indicated for the prevention of VTE and pulmonary embolism in cases of ischemic stroke and nonvalvular atrial fibrillation (Maximiliano et al., 2023). This drug is administered orally, making it widely used and accepted compared with subcutaneous injections of anticoagulants such as warfarin.
However, many reports claim adverse effects of rivaroxaban in clinical settings. In 2018, a 79-year-old woman who used rivaroxaban for a stroke developed a rash as an adverse reaction (Sasson et al., 2018). At the same time, Licata et al. summarized the published studies and case reports of rivaroxaban-induced hepatotoxicity (Licata et al., 2018). In 2019, rivaroxaban was reported to induce acute interstitial nephritis in a 70-year-old man (Zafar et al., 2019). In 2020, an 82-year-old patient was hospitalized after being prescribed rivaroxaban for atrial fibrillation. The patient developed a drug-induced hypersensitivity syndrome characterized by low-grade fever, a petechial rash on the legs, and acute renal failure (Marcelino et al., 2020). Simultaneously, a case report showed that a patient developed acute thrombocytopenia [an extremely rare adverse drug reaction (ADR)] after using rivaroxaban (He and Bai, 2020). Studies reported that using rivaroxaban could increase the risk of intraocular hemorrhage (Talany et al., 2017), and rivaroxaban combined with other drugs might increase the risk of bleeding (Gruenebaum et al., 2014). A retrospective cohort study including 581,451 patients aged 65 years and older with atrial fibrillation showed that treatment with rivaroxaban was associated with a significantly increased risk of severe ischemia or bleeding time compared with treatment with apixaban (Ray et al., 2021). Therefore, the adverse effects of rivaroxaban are numerous, necessitating a systematic study to provide a reference for the rational use of this drug.
ADRs are hazardous reactions resulting from the use of medical products, placing a burden on people who use these drugs. At present, the healthcare systems of many developed countries take actions to reduce this impact. These actions include educating clinicians and patients, establishing and managing spontaneous adverse event reporting systems and platforms, providing guidelines for ADR management, and manufacturing safer drugs and antidotes (McDonnell and Jacobs, 2002; Curry et al., 2005; Davies et al., 2007; Plumpton et al., 2016; Khalil and Huang, 2020). In particular, a system of spontaneous reporting of adverse events has emerged as a significant basis for the post-marketing surveillance of medicines. The World Health Organization, the United States Food and Drug Administration, and the European Medicines Agency have successively established databases for monitoring ADRs as an effective method of monitoring the safety of new drugs and regularly supervising the effects of old drugs (Doua and Van Geertruyden, 2014; Guan et al., 2022). In line with this, data mining based on the spontaneous reporting of ADR databases has received extensive attention. Data mining is an application of classical epidemiological and statistical methods to describe and analyse the distribution of suspected drug use and effects (occurrence of adverse reactions) within a certain period, and then explore possible associations between the two. It is significant that mining ADR databases to identify risk signals, intervene the possibe drug risk, guarantee the safety and improve the quality of medical treatment. ADR signal detection is thus the most important technical work in ADR monitoring (Arnaud et al., 2017).
Faced with so many complicated adverse reaction reports of rivaroxaban, it is essential to caary out a data mining study based on of the Food and Drug Administration Adverse Event Reporting System (FAERS) to summarize these yearly reports. And even, it is better to update and visualize the adverse event signals associated with rivaroxaban using some new technique. If possible it is be hoped that this study can provide a basis for the safe clinical use and mangement of the drug.
Materials and methods
Data source and data processing
The data for this study were obtained from the FAERS database, which is the largest database globally. It has collected billions of spontaneous adverse event (AE) reports from various regions and districts since 2004. The database is updated quarterly and has been freely available to the public. In this study, all American Standard Code for Information Interchange (ASCII) packet data from 41 quarters spanning from 2014 Q1 to 2024 Q1 were extracted and imported into Statistics Analysis System (SAS) 9.4 software for data cleaning and analysis. According to the recommendations of the US FDA and the data description document, the reports were cleaned, de-duplicated and organized. And ADE reports with rivaroxaban as the primary suspected drug (PS) were obtained by entering “rivaroxaban” in the “DRUGNAME” field and “PROD_AI” field. The original report is characterized by a high degree of data structuring and regularity, and a large amount of available information (Carnovale et al., 2018). In the FAERS, AEs are coded at the preferred-term (PT) level of the Medical Dictionary for Regulatory Activities (MedDRA) classification.
Detection method of signal mining
Disproportionality analysis is one of the most frequently used methods of safety signal detection and consists of two categories: frequentist statistics and Bayesian statistics. On the one hand, Frequentist statistics includes methods such as reporting odds ratio (ROR) and proportional reporting ratio (PRR). Moreover, the Europe Medicines Agency has proposed a method named the Medicines and Healthcare Products Regulatory Agency (MHRA) method to identify signals. On the other hand, Bayesian statistics includes Bayesian confidence propagation neural network (BCPNN) and multi-item gamma Poisson shrinker (MGPS). The frequentist method has its characteristics: the sensitivity of the frequentist method is high, but it easily produces false-positive signals when the number of reports is small. The specificity of the Bayesian method is good; however, signal detection is relatively delayed. Specially, ROR and PRR could identify abnormally higher than expected proportions of AE reporting, thus highlighting the risks associated with the targeted drug, but PRR is its higher specificity compared to ROR. BCPNN excels in integrating multi-source data and performing cross-validation, which can be capable of capturing potential drug-AE associations. The MGPS algorithm is more comprehensive, quantifying AE signals based on considering the number of reports and background risk, which can detect signals from rare events (Jiang et al., 2024; Zhu et al., 2024). Five methods, such as ROR, PRR, BCPNN, MHRA, and MGPS, were used for signal detection in this study to minimize the result bias caused by using a single algorithm alone. When each algorithm was positive according to its own criteria, it was judged indicating suspicious signals. The 2 × 2 cell table used in the disproportionality analysis method is shown in Table 1. In the table, “a” means the number of reports of a specific AE caused by the target drug; “b” means the total number of all other AEs related to the target drug; “c” means the number of reports of the target AEs caused by all other drugs; and “d” means the total number of all other AEs related to all other drugs.
Risk signal criteria
The formulas of each algorithm and standard of signal detection are shown in Supplementary Table S1, (Guan et al., 2022; Shu et al., 2022). Regarding ROR, a ≥3 and the lower limit of two-sided 95% confidence interval (CI) > 1 were the signal criteria. For PRR, the screening criteria were the same as that for ROR. For MHRA, the criteria for an AE defined as a significant signal were PRR >2, χ2 > 4, and a >3. Regarding BCPNN, the screening criteria for a significant signal were the lower limit of the CI (IC-2SD) > 0. According to the signal strength level of BCPNN, if IC-2SD ≤ 0, one drug-AE combination was acknowledged as no signal (−); if 0 < IC-2SD ≤ 1.5, a drug-AE combination was recognized as a weak signal (+); if 1.5 < IC-2SD ≤ 3, one drug-AE combination was defined as a moderate signal (++); if IC-2SD > 3, the drug-AE combination was defined as a strong signal (+++). Regarding MGPS, EBGM05 > 2 was denoted as a significant signal.
Statistical analysis
Descriptive statistics were used to analyze patient and reporter demographics. Means and standard deviations were reported for continuous variables, whereas frequencies and percentages were used for categorical variables. Data management, processing, and analysis were performed using SAS (version 9.4). Figures were illustrated using GraphPad Prism (version 8.2) or R (version 4.2.2).
Results
Descriptive analysis
Information on demographic characteristics of all reports associated with rivaroxaban is shown in Table 2. A total of 77,384 rivaroxaban case reports were included in the study between 2014 and 2024. Among these, the proportion of male patients was more than that of female patients (47.74% vs. 46.24%). Further, 42,613 (55.07%) patients were aged ≥65 years. The median age was 72 years. The year with the highest number of reports was 2015 (19.06%). Further, more than half of all reports were shared by consumers (53.84%). The most reported country was the United States (74.38%), and the most common route of administration was oral (98.69%). Additionally, 86.20% of the reports were classified as serious reports. Hospitalization was the most common outcome of adverse effects for patients (60.24%). The indications of the top 60 most frequently reported rivaroxaban-associated are presented in Table 3. Of these, the most common rivaroxaban-associated indication was “Atrial fibrillation” (n = 25,886), followed by “Cerebrovascular accident prophylaxis” (n = 13,259), “Deep vein thrombosis” (n = 12,346), “Pulmonary embolism” (n = 6,248) and “Thrombosis prophylaxis” (n = 5,834). As the above three indications accounted for more than 60% of the reports, we conducted separate analyses of adverse reaction reports for each of the three indications. The demographic characteristics of three indications’ reports were also shown in Table 2.
The proportion of suspected adverse events under SOC level with rivaroxaban
The proportion of suspected adverse events reports under SOC level was drawn (Figure 1), which was equal to the number of suspected adverse event reports under organ system classification (SOC)/total number of suspected adverse event reports of target drugs. “Gastrointestinal disorders” were associated with the highest number of suspected adverse event reports (20.90%), followed by “Injury, poisoning, and procedural complications” (12.13%), “Nervous system disorders” (11.95%), “Vascular disorders” (8.07%), and “General disorders and administration site conditions” (7.00%). The detailed information was provided in Supplementary Table S2.
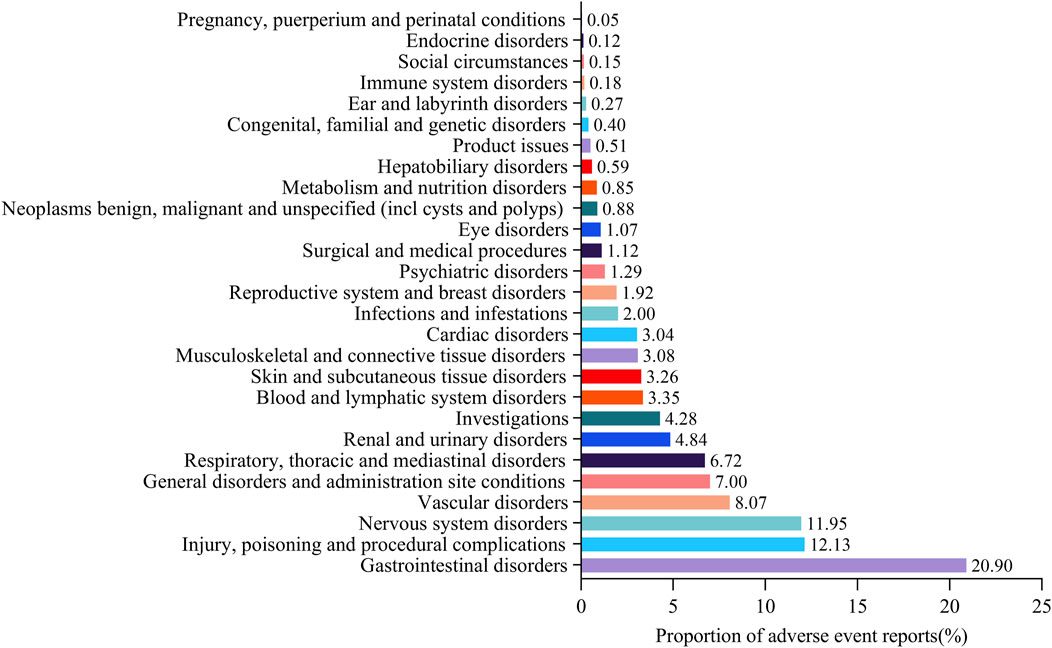
Figure 1. Proportion of suspected adverse event reports under system organ class (SOC) for rivaroxaban.* Proportion of suspected adverse event reports under organ system classification (SOC) = the number of suspected adverse event reports under organ system classification (SOC)/total number of suspected adverse event reports of target drugs.
Time trend of different SOCs for rivaroxaban-associated suspected AEs based on their IC and their 95% CIs
This study generated time series analyses of safety signals of different SOCs for investigating the changes in each signal over time. When a graph showed an upward trend and the CI gradually narrowed, it indicated that the signal was stable and strongly correlated with the use of rivaroxaban. The horizontal axis represented the year of the report, whereas the vertical axis represented the information component (IC) value and its 95% CI.
As shown in Figures 2G, H, the IC values of rivaroxaban-induced suspected AEs for “Surgical and medical procedures” and “Cardiac disorders” had an augmented trend with the increase in the number of years. Because the 2024 data for this study only included the first quarter, the picture shows a wide range of ICs and their 95% confidence intervals. So, the IC values across “Surgical and medical procedures” and “Cardiac disorders” accumulated gradually, and the range of CI continued to narrow or hold the line from 2014 to 2023. However, the other SOCs did not display this upward trend. Specially, the IC values of other SOCs, such as “Gastrointestinal disorders”, “Vascular disorders”, “Respiratory, thoracic, and mediastinal disorders”, “Nervous system disorders”, “Blood and lymphatic system disorders”, “Renal and urinary disorders” and “Injury, poisoning and procedural complications” were all above 0, suggesting that these SOCs had some suspect signals (Figures 2A–F, I). However, no such obvious hints were found in additional SOCs presented in Supplementary Figure S1 and Supplementary Figure S2. Their IC values and its 95%CI were all under 0, which means as far as BCPNN algorithm was concerned, these SOCs might not have suspect signals from statistical meaning.
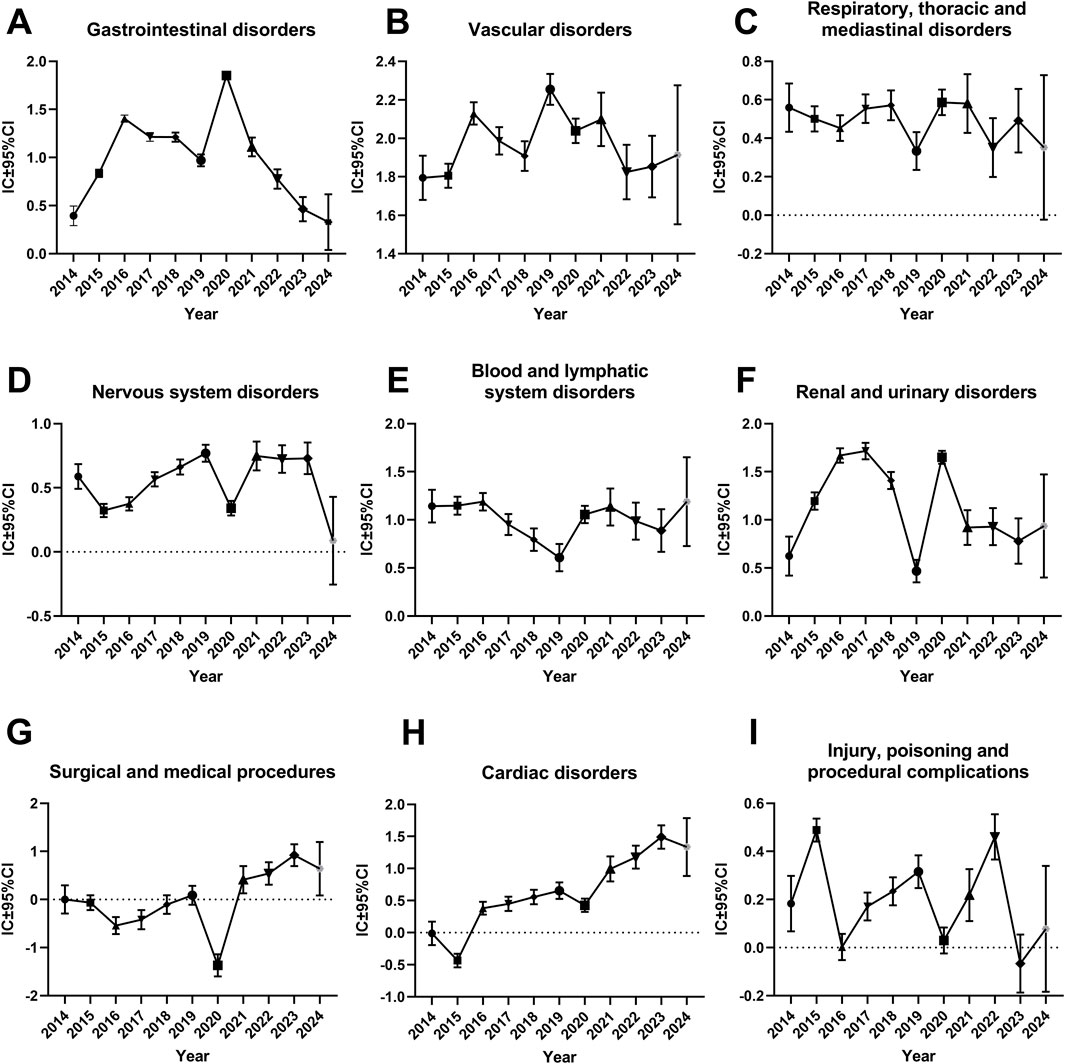
Figure 2. Information component and its 95% confidence interval over time for different system organ classes of rivaroxaban-associated adverse events. (A) Gastrointestinal disorders (B) Vascular disorders (C) Respiratory, thoracic and mediastinal disorders (D) Nervous system disorders (E) Blood and lymphatic system disorders (F) Renal and urinary disorders (G) Surgical and medical procedures (H) cardiac disorders (I) Injury, poisoning and procedural complications. Abbreviations: CI, confidence interval; IC, information component.
Suspected AE signals associated with rivaroxaban
This study used five algorithms, including ROR, PRR, MHRA, MGPS, and BCPNN, to detect the suspected AE signals for rivaroxaban at the PT level. There were 177,465 combinations of rivaroxaban-associated AEs reported in the FAERS databas. One report (patient) may report several adverse events, so the data was generally be greater than the number of reports. The ROR and PRR algorithms recognized 780 signals of rivaroxaban-associated AEs. A total of 718 signals of rivaroxaban-associated AEs were identified by the MHRA algorithm. EBGM picked out 707 signals, whereas BCPNN distinguished 704 signals. The top 50 signal strength of suspected adverse events with rivaroxaban under the ROR algorithm are sorted by EBGM at the PT level in FAERS are exhibited in Table 4. The European Medicines Agency has drawn up a list of criteria to differentiate the important medical events (IMEs). Based on the latest version of MedDRA 26.1, IMEs integrated 1,627 PTs. The UpSet diagram provided the number of common and unique elements between five or more groups. Accordingly, we added the IME classification to the five algorithms to draw an UpSet diagram to show the detected signals of suspected adverse events, as shown in Figure 3. Furthermore, 305 PTs were confirmed for important medical events and 278PTs represent non-important medical events by the five methods detected. However, 1,175 were IMEs but they were not detected as suspect signals by five algorithms. Regardless of whether it was a significant medical event or not, it represented the signal that all five algorithms collectively detected, which was 305 + 243 = 548; this number was consistent with the result of the Venn diagrams (Supplementary Figure S3).
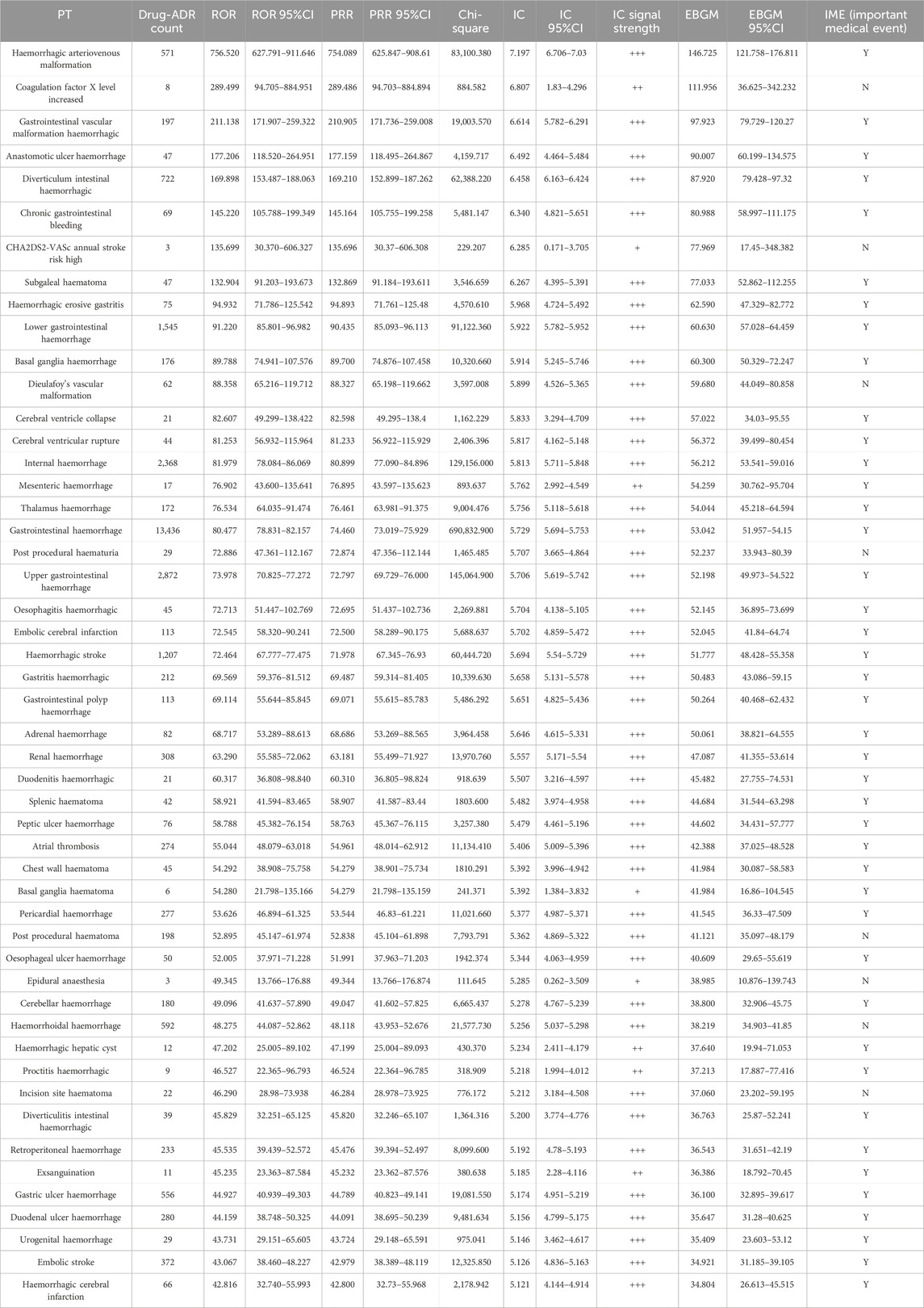
Table 4. The top 50 signal strength of adverse events with rivaroxaban under the ROR algorithm are sorted by EBGM at the PT level in FAERS.
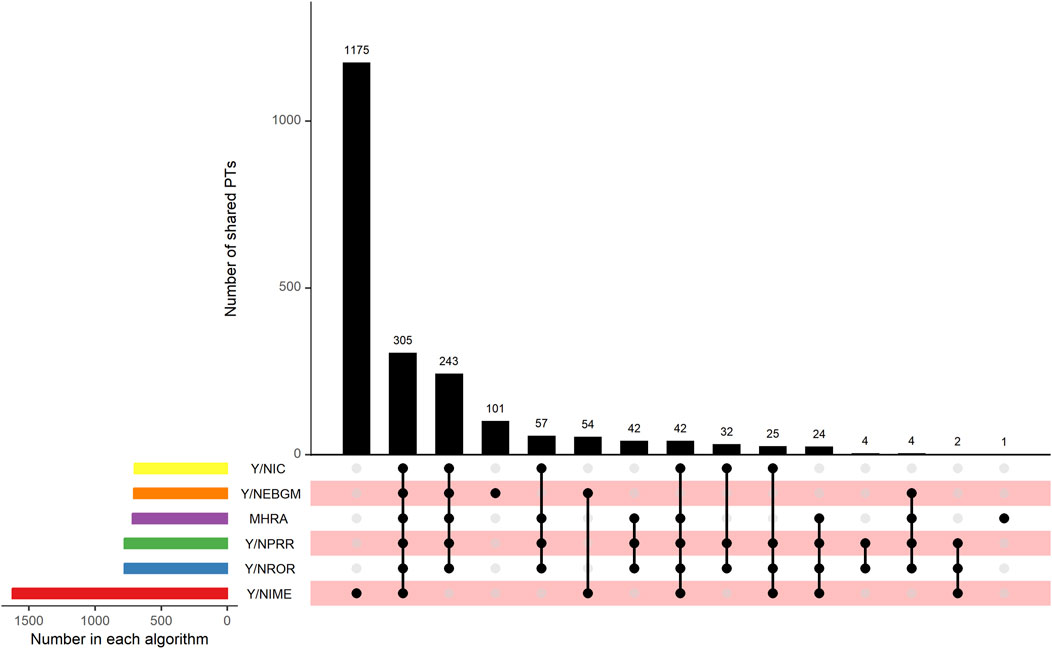
Figure 3. UpSet plot shows the number of PTs in each algorithm. Abbreviations: IC, a value named information component in BCPNN algorithm; BCPNN, Bayesian confidence propagation neural network; EBGM, a value named empirical Bayesian geometric mean in MGPS algorithm; MGPS, multi-item gamma Poisson shrinker; MHRA, a ≥3 a, PRR ≥2, and χ2 ≥ 4; PRR, proportional reporting ratio; ROR, reporting odds ratio; IME, important medical event.
The heat map plot was drawn to reflect the signals of suspected AEs detected by ROR, BCPNN, MHRA, and MGPS algorithms at the SOC level. The horizontal axis denotes different algorithms, the vertical axis denotes different SOC classifications, and the color shades denote the signal numbers of PTs. A darker color indicates more signal numbers of PTs. It was obvious that “Gastrointestinal disorders” had the maximum signal numbers of PTs regardless of the algorithms (Figure 4). All five methods found that the risk of AEs was the highest for “Gastrointestinal disorders”, followed by “Nervous system disorders” and “Injury, poisoning and procedural complications”. However, the risk of ADR was low for “Pregnancy, puerperium and perinatal conditions”, “Immune system disorders”, and “Psychiatric disorders”. Interestingly, the MGPS algorithm found a higher risk of AEs under “Investigations” category, but the results of the analyses of the other methods were not consistent with this. The “Investigations” included “Heamatology investigations (including blood groups)”, “Cardiac and vascular investigations (excluding enzyme tests)”, “Renal and urinary tract investigations and urinalyses”, and so on. The Venn diagrams of rivaroxaban were designed to find similar numbers of suspected AE signals. A total of 548 signals were collectively identified using five algorithms, as shown in Supplementary Figure S3. Of these, the ROR, PRR, and IC methods detected the highest overlap of signals and were therefore considered to be more conservative and reliable. The EBGM method additionally detected the maximum number of signals (n = 155) and might be more sensitive. Supplementary Figure S4 shows the percentage of every high-level group term under the “Investigations” category. A volcano plot was developed to investigate the relationship between the ROR, PRR, IC, and EBGM and significant differences to identify rivaroxaban associated with AEs (Supplementary Figure S5). The x-axis denotes the logarithm of the reporting ROR or PRR or IC lower limit or EBGM lower limit, and the y-axis denotes the negative logarithm of the P value calculated using the chi-square test (-ln(P value)). Positive values in the direction of the y-axis represent significant differences. The colors of the points represent the difference in the logarithm of the number of each ADR. In this scatterplot, the point in the upper right corner indicates a stronger signal. The blue-to-red colors represent the number of times of reported adverse effects. Each label represents a specific PT. Based on the plots, “Gastrointestinal Hemorrhage”, “Upper gastrointestinal haemorrhage”, “Rectal haemorrhage”, “Internal haemorrhage” and “haematuria” were identified as the most significant signals across four algorithms.
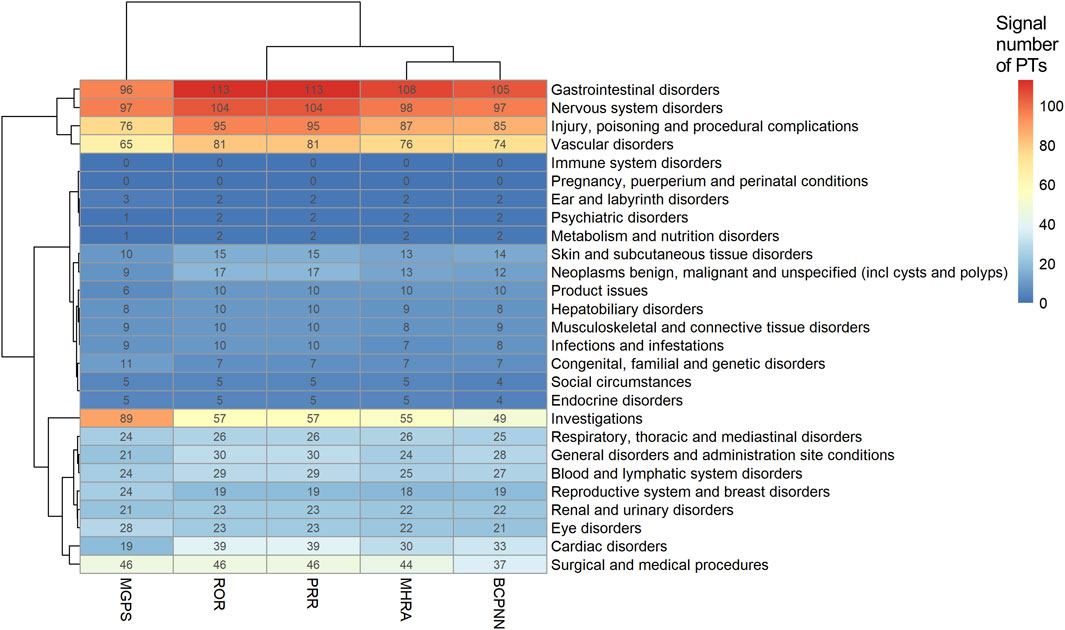
Figure 4. Signals of adverse events detected using ROR, BCPNN, MHRA, and MGPS algorithms at the SOC level. Abbreviations: MGPS, multi-item gamma Poisson shrinker; ROR, reporting odds ratio; PRR, proportional reporting ratio; MHRA, a ≥3, PRR ≥2, and χ2 ≥ 4; BCPNN, Bayesian confidence propagation neural network.
Signals of suspected adverse events associated with atrial fibrillation, cerebrovascular accident prophylaxis and deep vein thrombosis
The top 10 signals sorted by the number of drug–adverse event combinations associated with three indications at the PT level in FAERS are exhibited in Table 5. Notably, regardless of indication, “Gastrointestinal haemorrhage”, “Epistaxis”, “Haematuria”, “Rectal haemorrhage”, and “Upper gastrointestinal haemorrhage” (Underline fonts) were detected as the most common and significant signals of suspected adverse events. Among Atrial fibrillation, Cerebrovascular accident prophylaxis and Deep vein thrombosis and the total reports of rivaroxaban, 9 signals were collectively identified using BCPNN algorithm, as shown in Figure 5. Volcano plots were used to visualise information intuitively on suspected adverse reactions by MGPS algorithm under the three indications and the total reports of rivaroxaban. The x-axis denotes the logarithm of the reporting EBGM lower limit, and the y-axis denotes the negative logarithm of the P value calculated using the chi-square test (-ln (P value)). Positive values in the direction of the y-axis represent significant differences. The colors of the points represent the difference in the logarithm of the number of each ADR. In this scatterplot, the point in the upper right corner indicates a stronger signal. The blue-to-red colors represent the number of times of reported adverse effects. Each label represents a specific PT. Based on the plots, “Gastrointestinal Hemorrhage” were identified jointly as the most significant signal (Figure 6).
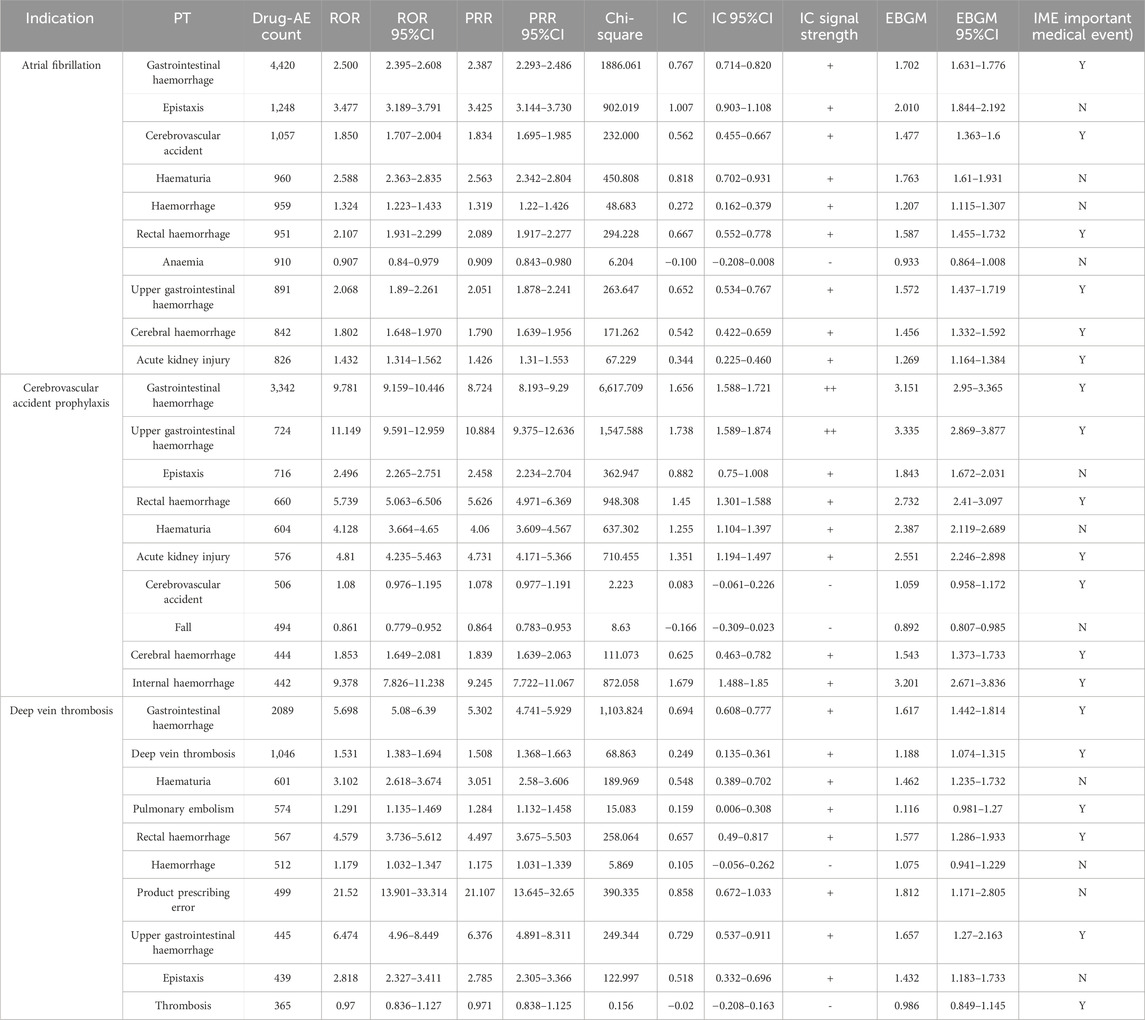
Table 5. The top 10 signals sorted by the number of drug–adverse event combinations associated with three indications at the PT level.
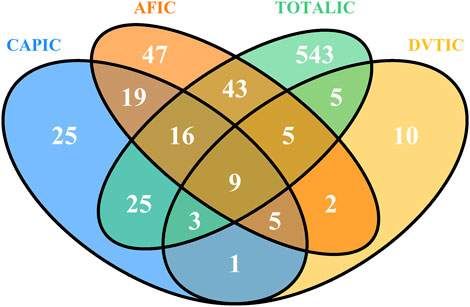
Figure 5. Venn diagram of the suspected AE signals associated with rivaroxaban detected using BCPNN algorithms at the PT level. Abbreviations: CAP: Cerebrovascular accident prophylaxis; AF: Atrial fibrillation; DVT: Deep vein thrombosis; TOTAL: All indications of rivaroxaban; IC, a value named information component in BCPNN algorithm.
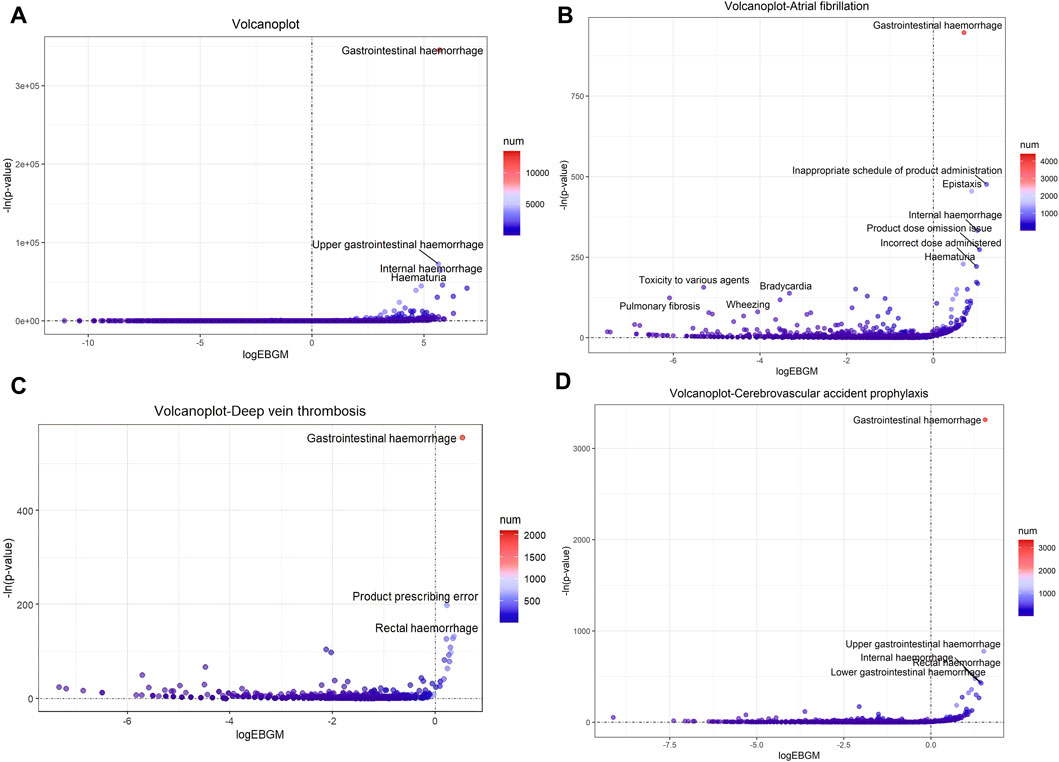
Figure 6. Signals of suspected AEs associated with rivaroxaban using MGPS algorithm at the PT level. (A) Volcano plot of detected AEs related to all indications (B) Volcano plot of detected AEs related to Atrial fibrillation (C) Volcano plot of detected AE related to Deep vein thrombosis (D) Volcano plot of detected AE related to Cerebrovascular accident prophylaxis. The x-axis denotes the logarithm of the reporting ROR (log2 (ROR)) or PRR (log2 (ROR)) or IC lower limit (log2(IC)) or EBGM lower limit (log2 (EBGM)). The y-axis is the negative logarithm of the P value calculated using the chi-square test (−ln (P value)). Positive values in the direction of the y-axis represent significant differences. The colors of the points represent the difference in the logarithm of the number of each ADR. In this scatterplot, the point in the upper right corner has a greater signal. The blue-to-red colors represent the number of times an adverse effect was reported. Abbreviations: EBGM, a value named empirical Bayesian geometric mean in MGPS algorithm; MGPS, multi-item gamma Poisson shrinker.
Discussion
Our study found that suspected adverse events related to rivaroxaban were most frequently reported among individuals aged 65 years and older, accounting for 55% of the adverse reaction reports, totaling 42,613 case. This was roughly similar to the age of the seven patients with adverse reaction reports associated with rivaroxaban covered in the preamble. It is worth mentioning that we found 60 commonly reported indications for rivaroxaban to be “Atrial fibrillation”, “Cerebrovascular accident prophylaxis”, “Deep vein thrombosis”, “Pulmonary embolism”and “Thrombosis prophylaxis”, which is similar to the therapeutic indications of rivaroxaban according to the European medicines agency’s production information. According to the baseline characteristics, we also found that people over 65 years old had the largest number of adverse reaction reports, which is consistent with epidemiological data that older adults are more likely to develop deep vein thrombosis and venous thromboembolism (Bulger et al., 2004; Wollenman et al., 2024).
Rivaroxaban is used for the prevention and treatment of deep vein thrombosis and pulmonary embolism formation in adults, as well as for the prevention of stroke and non- Central Nervous System embolism in patients with atrial fibrillation and in patients with non-valvular atrial fibrillation, and also for the treatment of hepatitis C. Based on this, the data mining results also showed that the suspected AE signals of “Surgical and medical surgery” and “Heart disease” were robust, because rivaroxaban is widely used in hip and knee replacement surgery and anticoagulation therapy for patients with nonvalvular atrial fibrillation. All these further indicate that our results are credible. And combined with the results of signal mining of overall rivaroxaban adverse drug reaction reports, the three most used indications for rivaroxaban were analysed individually, it was found that “Gastrointestinal haemorrhage”, and “Upper gastrointestinal haemorrhage” were the most common and signal significant suspected adverse reactions (Specific information is detailed in bold in Tables 4, 5).
The year 2015 saw the highest number of adverse reaction reports for rivaroxaban, with 14,751 cases accounting for 19% of the total. This surge in reports might be closely related to the drug’s approval for use in the United States since 2011. The number of AE reports decreased each year. We speculated that this might be due to the emergence of an increasing number of oral anticoagulants gradually replacing rivaroxaban. Using signal mining, we also found that rivaroxaban was significantly associated with an increased risk of gastrointestinal adverse events, especially gastrointestinal hemorrhage. This result was consistent with the findings of other studies. Fernandez et al. summarized the drug–drug interactions of rivaroxaban with other drugs in a systematic review including 31 studies and 28 case reports. They found that the drug-drug interactions of rivaroxaban with other drugs resulted in an increased risk of hemorrhage or thromboembolic events (Fernandez et al., 2021). In a prospective study, Njuguna et al. reported that the use of rivaroxaban was associated with a higher frequency of bleeding. This association was observed by evaluating patients who were newly initiated on rivaroxaban or switched from warfarin to rivaroxaban for treating venous thromboembolism at the National Referral Hospital in Western Kenya (Njuguna et al., 2023). A pharmacovigilance analysis by Sun et al. of suspected hemorrhagic events following antithrombotic drug use, based on the FAERS database, indicated that rivaroxaban was more strongly associated with hemorrhage than were other antithrombotic drug monotherapies. The combination of rivaroxaban with a drug such as clopidogrel was associated with an earlier onset of hemorrhagic events (Sun et al., 2022). These researches manifested that rivaroxaban use was a prominent risk factor for gastrointestinal bleeding.
Our study provided a comprehensive summary of the suspected AEs associated with rivaroxaban by five algorithms based on two statistical principles, and used the latest data and visualization methods innovatively to show the results. This study may provide a basis and supplement for the rational and safe use and regulation and policing of the drug, which can fill up a little study gap on adverse reaction reports of rivaroxaban alone. However, the use of databases in this study had certain shortcomings: First, the spontaneous adverse event reporting system suffered from reporting bias (Zhu et al., 2024) and shortcomings such as omissions absence, misreporting, the lack of denominator and missing data (Kong et al., 2023). Key patient information such as gender, age, and medication initiation time were missing in some proportions, which could impact the results. The information in these reports reflects only the observations of the reporters. Second, it was probably unable to estimate true risk or assess incidence. For one thing, the data lacked meaningful denominators to exclude prevalent cases. For another thing, these reports originate from actual patients who have several concomitant conditions and are being treated with different medicines, isolating from the complex patient reality could cause confusion and produce inaccurate information. Confounding factors such as potential drug-drug interactions; preexisting diseases; comorbidities; acute versus chronic duration of treatment; synergistic, antagonistic, additive effects with other pharmacologic agents; and genetic predisposition to therapy response29 30 could not be fully controlled. Third, most of the dosage information was missing, and hence we did not consider the effect of dose, which may be an essential factor in the AEs of rivaroxaban (Sun et al., 2022). Moreover, the analyzed reports were filtered by “primary suspect drug”, and did not include concomitant medicines, which could miss some crucial drug interaction signals and became one of the limitations of our study. Fourth, the FAERS is open and free, consumers and healthcare professionals are encouraged to report adverse events, 53.84% of the reporters were consumers. This high percentage of non-medical staff reporting probably undermines the credibility of the results. The documentation of one or more outcomes in a report does not necessarily mean that the suspect product mentioned in the report was the cause of those outcomes. Although we used various data mining algorithms for our study, the submission of a report to the FAERS database does not imply that the information contained in the report has been medically confirmed, nor does it imply that the reporter acknowledges that the drug caused or contributed to the event. Additionally, the disproportionality analysis neither quantified risk nor existed causality, but only provided an estimation of the signal strength from statistically significant only, so the results of the data mining methods should be used with caution when extrapolating conclusions and a causal relationship (Shu et al., 2022). More clinical data are still needed to support this as evidence.
In recent years, direct oral anticoagulants have been essential in treating and preventing thromboembolic incidents (Bozic et al., 2023). Two clinical trials of rivaroxaban for the treatment and post-surgical prophylaxis of thrombosis in pediatric populations reported that rivaroxaban was safe and effective in the treatment and secondary prevention of venous thromboembolism in children (McCrindle et al., 2021; Bosch and Albisetti, 2022). However, a study including 236 cases of direct oral anticoagulant use in pregnancy showed that rivaroxaban was the most reported direct oral anticoagulant in pregnancy and that rivaroxaban resulted in a higher rate of miscarriage and a 4% rate of malformations (Lameijer et al., 2018). Future research should expand the exploration of patient populations using rivaroxaban, focusing not only on the elderly population but also on the child–adolescent population and women during pregnancy. In addition, if rivaroxaban is to be used safely and appropriately, clinicians and pharmacists must weigh the pros and cons, considering the patient’s age, sex, medical condition, physical condition, drug dose, combination of drugs, and many other factors (Mardi et al., 2023; Moldenhauer et al., 2023). Moreover, researchers also can be encouraged to better design their studies on databases, so that providing more information on studying concomitant drugs with this drug would be useful for the readers and for future researches. Some studies have shown that genes were also associated with the adverse effects of rivaroxaban, providing a reference and basis for individualized treatment (Kim et al., 2023; Li et al., 2023). With a large number of pharmacovigilance (PV) studies are currently being published vigorously, it is necessary that large PV database analyses must be conducted taking into consideration some basic aspects to start giving signals.
In conclusion, our study employs a combined application of ROR, PRR, MHRA, BCPNN, and MGPS algorithms with the aim of leveraging the strengths of each to broaden the detection scope, verify results from multiple perspectives, and make rational use of the distinctive features of different algorithms to detect more comprehensive and reliable suspected signals. “Gastrointestinal haemorrhage”, “Upper gastrointestinal haemorrhage” should be the focus of attention to prevent adverse events. And it is worth noting that uncommon but significantly ADE signals, such as “Coagulation factor X level increased”, “Basal ganglia haematoma”, and “Proctitis haemorrhagic” also should be concentrated on.
Conclusions
This study provided an objective reference for pharmacovigilance by mining the safety signals of rivaroxaban. “Gastrointestinal disorders”, “Injury, poisoning, and procedural complications”, “Nervous system disorders” and “Vascular disorders” were the significant system organ classes with adverse events. Focus also should be placed on suspected AEs with strong signals not common reported, such as “Coagulation factor X level increased”, “Basal ganglia haematoma”, and “Proctitis haemorrhagic”. In addition, both the risk and benefit should be appropriately weighed when prescribing rivaroxaban for patients. Close attention should be paid to disease progression, and timely intervention measures should be taken against AEs to reduce the risk of poor outcomes.
Data availability statement
Publicly available datasets were analyzed in this study. These data can be found at: https://www.fda.gov/drugs/drug-approvals-and-databases/fda-adverse-event-reporting-system-faers. Further inquiries can be directed to the corresponding author.
Author contributions
JnW: Conceptualization, Data curation, Formal Analysis, Methodology, Software, Visualization, Writing–original draft, Writing–review and editing. JaW: Conceptualization, Data curation, Formal Analysis, Visualization, Writing–original draft, Writing–review and editing. BT: Conceptualization, Funding acquisition, Investigation, Methodology, Writing–review and editing. XW: Writing–review and editing. FW: Resources, Writing–review and editing. YZ: Writing–review and editing. LL: Software, Writing–original draft. HL: Writing–review and editing. BW: Funding acquisition, Project administration, Writing–review and editing. WW: Funding acquisition, Project administration, Writing–review and editing. XH: Funding acquisition, Project administration, Supervision, Writing–review and editing.
Funding
The author(s) declare that financial support was received for the research, authorship, and/or publication of this article. This work was supported by Guangdong Basic and Applied Basic Research Foundation (2023A1515011495); Guangdong Medical Products Administration Scientific and Technological Innovation Project (2021ZDB01); National Natural Science Foundation of China (No. 82204057), Natural Science Foundation of Jiangsu Province, China (No. BK20220827).
Acknowledgments
The authors are grateful for the free access to the open-source data. They also thank the experts who provided valuable suggestions for the successful completion of this study. We thank International Science Editing (http://www.internationalscienceediting.com) for editing this manuscript.
Conflict of interest
The authors declare that the research was conducted in the absence of any commercial or financial relationships that could be construed as a potential conflict of interest.
Publisher’s note
All claims expressed in this article are solely those of the authors and do not necessarily represent those of their affiliated organizations, or those of the publisher, the editors and the reviewers. Any product that may be evaluated in this article, or claim that may be made by its manufacturer, is not guaranteed or endorsed by the publisher.
Supplementary material
The Supplementary Material for this article can be found online at: https://www.frontiersin.org/articles/10.3389/fphar.2024.1399172/full#supplementary-material
References
AG. BP (2016). Xarelto1 summary of product characteristics. Available at: https://www.ema.europa.eu/en/documents/product-information/xarelto-epar-product-information_en.pdf.
Arnaud, M., Bégaud, B., Thurin, N., Moore, N., Pariente, A., and Salvo, F. (2017). Methods for safety signal detection in healthcare databases: a literature review. Expert Opin. Drug Saf. 16 (6), 721–732. doi:10.1080/14740338.2017.1325463
Bosch, A., and Albisetti, M. (2022). Adverse events of DOACs in children. Front. Pediatr. 10, 932085. doi:10.3389/fped.2022.932085
Bozic, D., Alicic, D., Martinovic, D., Zaja, I., Bilandzic-Ivisic, J., Sodan, R., et al. (2023). Plasma drug values of DOACs in patients presenting with gastrointestinal bleeding: a prospective observational study. Med. Kaunas. 59 (8), 1466. doi:10.3390/medicina59081466
Bulger, C. M., Jacobs, C., and Patel, N. H. (2004). Epidemiology of acute deep vein thrombosis. Tech. Vasc. Interv. Radiol. 7 (2), 50–54. doi:10.1053/j.tvir.2004.02.001
Carnovale, C., Mazhar, F., Pozzi, M., Gentili, M., Clementi, E., and Radice, S. (2018). A characterization and disproportionality analysis of medication error related adverse events reported to the FAERS database. Expert Opin. Drug Saf. 17 (12), 1161–1169. doi:10.1080/14740338.2018.1550069
Curry, L. C., Walker, C., Hogstel, M. O., and Burns, P. (2005). Teaching older adults to self-manage medications: preventing adverse drug reactions. J. Gerontol. Nurs. 31 (4), 32–42. doi:10.3928/0098-9134-20050401-09
Davies, E. C., Green, C. F., Mottram, D. R., and Pirmohamed, M. (2007). Adverse drug reactions in hospitals: a narrative review. Curr. Drug Saf. 2 (1), 79–87. doi:10.2174/157488607779315507
Doua, J. Y., and Van Geertruyden, J. P. (2014). Registering medicines for low-income countries: how suitable are the stringent review procedures of the World Health Organisation, the US Food and Drug Administration and the European Medicines Agency? Trop. Med. Int. Health 19 (1), 23–36. doi:10.1111/tmi.12201
Fernandez, S., Lenoir, C., Samer, C. F., and Rollason, V. (2021). Drug-drug interactions leading to adverse drug reactions with rivaroxaban: a systematic review of the literature and analysis of VigiBase. J. Pers. Med. 11 (4), 250. doi:10.3390/jpm11040250
Gruenebaum, D. D., Alsarah, A., Alsara, O., and Laird-Fick, H. (2014). Bleeding complication of triple therapy of rivaroxaban, prasugrel, and aspirin: a case report and general discussion. Case Rep. Cardiol. 2014, 293476. doi:10.1155/2014/293476
Guan, Y., Ji, L., Zheng, L., Yang, J., Qin, Y., Ding, N., et al. (2022). Development of a drug risk analysis and assessment system and its application in signal excavation and analysis of 263 cases of fluoroquinolone-induced adverse reactions. Front. Pharmacol. 13, 892503. doi:10.3389/fphar.2022.892503
He, X. Y., and Bai, Y. (2020). Acute thrombocytopenia after anticoagulation with rivaroxaban: a case report. World J. Clin. Cases 8 (5), 928–931. doi:10.12998/wjcc.v8.i5.928
Jiang, Y., Zhou, L. Y., Shen, Y., Zhou, Q., Ji, Y., and Zhu, H. (2024). Safety assessment of Brexpiprazole: real-world adverse event analysis from the FAERS database. J. Affect Disord. 346, 223–229. doi:10.1016/j.jad.2023.11.025
Khalil, H., and Huang, C. (2020). Adverse drug reactions in primary care: a scoping review. BMC Health Serv. Res. 20 (1), 5. doi:10.1186/s12913-019-4651-7
Kim, H., Song, T. J., Yee, J., Kim, D. H., Park, J., and Gwak, H. S. (2023). ABCG2 gene polymorphisms may affect the bleeding risk in patients on apixaban and rivaroxaban. Drug Des. Devel Ther. 17, 2513–2522. doi:10.2147/DDDT.S417096
Kong, W., Mao, W., Zhang, L., and Wu, Y. (2023). Disproportionality analysis of quinolone safety in children using data from the FDA adverse event reporting system (FAERS). Front. Pediatr. 10, 1069504. doi:10.3389/fped.2022.1069504
Lameijer, H., Aalberts, J. J. J., van Veldhuisen, D. J., Meijer, K., and Pieper, P. G. (2018). Efficacy and safety of direct oral anticoagulants during pregnancy; a systematic literature review. Thromb. Res. 169, 123–127. doi:10.1016/j.thromres.2018.07.022
Li, D., Yan, C., Guo, M., Zhao, Y., Zhang, Y., and Cui, X. (2023). Evidence of potential pro-haemorrhagic drug interactions between CYP3A4 inhibitors and direct oral anticoagulants: analysis of the FAERS database. Br. J. Clin. Pharmacol. 89 (8), 2423–2429. doi:10.1111/bcp.15710
Licata, A., Puccia, F., Lombardo, V., Serruto, A., Minissale, M. G., Morreale, I., et al. (2018). Rivaroxaban-induced hepatotoxicity: review of the literature and report of new cases. Eur. J. Gastroenterol. Hepatol. 30 (2), 226–232. doi:10.1097/MEG.0000000000001030
Marcelino, G., Hemett, O. M., and Descombes, E. (2020). Acute renal failure in a patient with rivaroxaban-induced hypersensitivity syndrome: a case report with a review of the literature and of pharmacovigilance registries. Case Rep. Nephrol. 2020, 6940183. doi:10.1155/2020/6940183
Mardi, P., Abbasi, B., Shafiee, A., and Afsharmoghaddam, T. (2023). Pharmacogenetic approach for the prevention of rivaroxaban's ADRs: a systematic review and meta-analysis. Genet. Res. (Camb) 2023, 6105320. doi:10.1155/2023/6105320
Maximiliano, C. L., Jaime, G. C., and Erika, M. H. (2023). Rivaroxaban plus aspirin versus acenocoumarol to manage recurrent venous thromboembolic events despite systemic anticoagulation with rivaroxaban. Thromb. Res. 222, 43–48. doi:10.1016/j.thromres.2022.12.008
McCrindle, B. W., Michelson, A. D., Van Bergen, A. H., Suzana Horowitz, E., Pablo Sandoval, J., Justino, H., et al. (2021). Thromboprophylaxis for children post-fontan procedure: insights from the UNIVERSE study. J. Am. Heart Assoc. 10 (22), e021765. doi:10.1161/JAHA.120.021765
McDonnell, P. J., and Jacobs, M. R. (2002). Hospital admissions resulting from preventable adverse drug reactions. Ann. Pharmacother. 36 (9), 1331–1336. doi:10.1345/aph.1A333
Moldenhauer, A., Hellstern, P., Hoffmann, T., and Fischer, J. C. (2023). Gender-associated variation in DOAC levels. Clin. Lab. 69 (7). doi:10.7754/Clin.Lab.2023.230101
Mueck, W., Eriksson, B. I., Bauer, K. A., Borris, L., Dahl, O. E., Fisher, W. D., et al. (2008). Population pharmacokinetics and pharmacodynamics of rivaroxaban – an oral, direct factor Xa inhibitor – in patients undergoing major orthopaedic surgery. Clin. Pharmacokinet. 47 (3), 203–216. doi:10.2165/00003088-200847030-00006
Njuguna, D., Nwaneri, F., Prichard, A. C., Manji, I., Kigen, G., Busakhala, N., et al. (2023). Risk of bleeding associated with outpatient use of rivaroxaban in VTE management at a national referral hospital in western Kenya. Clin. Appl. Thromb. Hemost. 29, 10760296231184216. doi:10.1177/10760296231184216
Perzborn, E., Roehrig, S., Straub, A., Kubitza, D., Mueck, W., and Laux, V. (2010). Rivaroxaban: a new oral factor Xa inhibitor. Arterioscler. Thromb. Vasc. Biol. 30 (3), 376–381. doi:10.1161/ATVBAHA.110.202978
Plumpton, C. O., Roberts, D., Pirmohamed, M., and Hughes, D. A. (2016). A systematic review of economic evaluations of pharmacogenetic testing for prevention of adverse drug reactions. Pharmacoeconomics 34 (8), 771–793. doi:10.1007/s40273-016-0397-9
Ray, W. A., Chung, C. P., Stein, C. M., Smalley, W., Zimmerman, E., Dupont, W. D., et al. (2021). Association of rivaroxaban vs apixaban with major ischemic or hemorrhagic events in patients with atrial fibrillation. JAMA 326 (23), 2395–2404. doi:10.1001/jama.2021.21222
Sasson, E., James, M., Russell, M., Todorov, D., and Cohen, H. (2018). Probable rivaroxaban-induced full body rash: a case report. J. Pharm. Pract. 31 (5), 503–506. doi:10.1177/0897190017722872
Shu, Y., He, X., Liu, Y., Wu, P., and Zhang, Q. (2022). A real-world disproportionality analysis of olaparib: data mining of the public version of FDA adverse event reporting system. Clin. Epidemiol. 14, 789–802. doi:10.2147/CLEP.S365513
Sun, X., Ze, B., Zhang, L.-J., BaiMa, Y. Z., Zuo, W., Zhao, B., et al. (2022). Hemorrhage risk profiles among different antithrombotic regimens: evidence from a real-world analysis of postmarketing surveillance data. Cardiovasc. Drugs Ther. 36 (1), 103–112. doi:10.1007/s10557-020-07110-w
Talany, G., Guo, M., and Etminan, M. (2017). Risk of intraocular hemorrhage with new oral anticoagulants. Eye (Lond) 31 (4), 628–631. doi:10.1038/eye.2016.265
Wollenman, C. C., Cox, C. L., Schoenecker, J. G., and Wright, R. W. (2024). Venous thromboembolism after knee arthroscopy: incidence, risk factors, prophylaxis, and management. J. Am. Acad. Orthop. Surg. 32 (17), e850–e860. doi:10.5435/JAAOS-D-24-00232
Zafar, F., Iqbal, A. M., Mubarik, A., Rojas, M., and Muddassir, S. (2019). Rivaroxaban-induced acute interstitial nephritis: a case report. Am. J. Case Rep. 20, 1719–1722. doi:10.12659/AJCR.917492
Keywords: suspected adverse event, disproportionality analysis, drug safety, pharmacovigilance, rivaroxaban
Citation: Wu J, Wu J, Tang B, Wang X, Wei F, Zhang Y, Li L, Li H, Wang B, Wu W and Hong X (2024) Suspected adverse drug reactions of rivaroxaban reported in the United States food and drug administration adverse event reporting system database: a pharmacovigilance study. Front. Pharmacol. 15:1399172. doi: 10.3389/fphar.2024.1399172
Received: 11 March 2024; Accepted: 26 August 2024;
Published: 06 September 2024.
Edited by:
Albert Figueras, Independent Researcher, Barcelona, SpainReviewed by:
Mingwei Zhang, First Affiliated Hospital of Fujian Medical University, ChinaYuan Xie, Shanghai University of Traditional Chinese Medicine, China
Copyright © 2024 Wu, Wu, Tang, Wang, Wei, Zhang, Li, Li, Wang, Wu and Hong. This is an open-access article distributed under the terms of the Creative Commons Attribution License (CC BY). The use, distribution or reproduction in other forums is permitted, provided the original author(s) and the copyright owner(s) are credited and that the original publication in this journal is cited, in accordance with accepted academic practice. No use, distribution or reproduction is permitted which does not comply with these terms.
*Correspondence: Wenyu Wu, wuwenyu000@163.com; Xiang Hong, hongxiang@seu.edu.cn
†These authors have contributed equally to this work and share first authorship