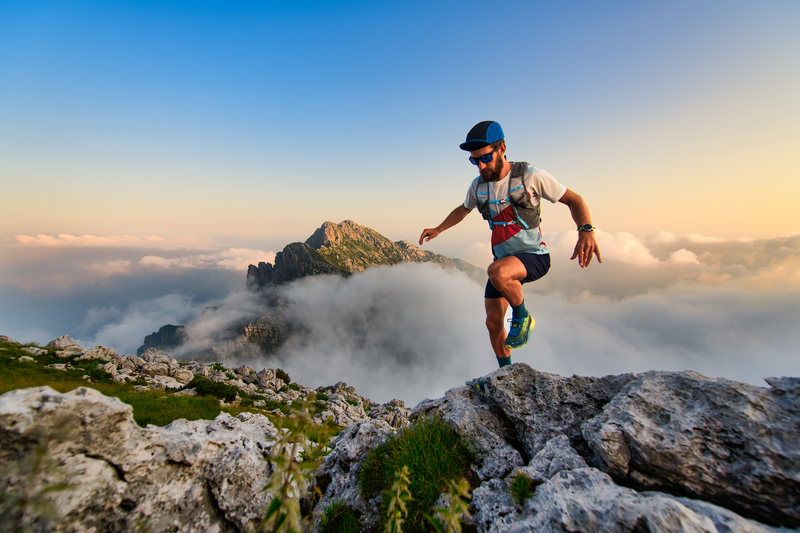
94% of researchers rate our articles as excellent or good
Learn more about the work of our research integrity team to safeguard the quality of each article we publish.
Find out more
ORIGINAL RESEARCH article
Front. Pharmacol. , 07 December 2023
Sec. Pharmacology of Anti-Cancer Drugs
Volume 14 - 2023 | https://doi.org/10.3389/fphar.2023.1298245
G2/M cell cycle checkpoint protein WEE1 kinase is a promising target for inhibiting tumor growth. Although various WEE1 inhibitors have entered clinical investigations, their therapeutic efficacy and safety profile remain unsatisfactory. In this study, we employed a comprehensive virtual screening workflow, which included Schrödinger-Glide molecular docking at different precision levels, as well as the utilization of tools such as MM/GBSA and Deepdock to predict the binding affinity between targets and ligands, in order to identify potential WEE1 inhibitors. Out of ten molecules screened, 50% of these molecules exhibited strong inhibitory activity against WEE1. Among them, compounds 4 and 5 showed excellent inhibitory activity with IC50 values of 1.069 and 3.77 nM respectively, which was comparable to AZD1775. Further investigations revealed that compound 4 displayed significant anti-proliferative effects in A549, PC9, and HuH-7 cells and could also induce apoptosis and G1 phase arrest in PC9 cells. Additionally, molecular dynamics simulations unveiled the binding details of compound 4 with WEE1, notably the crucial hydrogen bond interactions formed with Cys379. In summary, this comprehensive virtual screening workflow, combined with in vitro testing and computational modeling, holds significant importance in the development of promising WEE1 inhibitors.
Various external factors such as ultraviolet, ionizing radiations and cytotoxic drugs can cause DNA damage (Ou and Schumacher, 2018). Normally, tumor cells halt cell cycle to provide time for DNA repair. WEE1 is a serine/threonine kinase and serves as a crucial regulatory protein for the G2/M checkpoint (Do et al., 2013; Matheson et al., 2016; Geenen and Schellens, 2017). Specifically, in response to DNA damage, WEE1 induces G2/M arrest by inhibitory phosphorylation of CDK at Tyr15 (Li et al., 2020). Previous research has demonstrated that over 50% of tumor cells exhibit p53 mutations that disable G1/S checkpoint, thereby rendering cells more reliant on the G2/M checkpoint (Dillon et al., 2014). Inhibition of WEE1 results in the elimination of G2/M arrest, allowing tumor cells to enter the division phase with incompletely-repaired DNA, finally leading to mitotic catastrophe and cell death (Vakili-Samiani et al., 2022). Moreover, WEE1 is associated with cancer progression and highly expressed in breast cancer (Ha et al., 2020), lung cancer (Liu et al., 2019), head and neck cancer (Diab et al., 2019), and ovarian cancer (Zhang et al., 2017). Consequently, WEE1 emerges as a promising target for therapeutic interventions directed at malignancies. WEE1 inhibitors can be utilized as single agents or in combination with DNA-damaging agents to enhance their therapeutic efficacy.
Although several WEE1 inhibitors such as Adavosertib (AZD1775), ZN-c3, IMP7-68, IMP7068, SY-4835, and Debio-0123 (Fu et al., 2018; Huang et al., 2021; Lin et al., 2022; Papadopoulos et al., 2022) have entered clinical research (Figure 1), none of them have been approved for marketing. Among these candidates, AZD1775 and ZN-c3 have achieved notable progress and are currently undergoing phase II clinical trials. Most WEE1 inhibitors have terminated clinical research due to inadequate efficacy and safety issues. Notably, AZD1775, especially in combination regimens, was associated with a heightened incidence of adverse events such as anemia, diarrhea and vomiting (Leijen et al., 2016). In contrast, ZN-c3 has exhibited superior kinase selectivity and favorable in vivo oral exposure and is presently in phase II clinical investigation. Therefore, the development of highly effective small molecule inhibitors possesses promising clinical prospects.
In this study, a comprehensive virtual screening workflow has designed to discover new WEE1 inhibitors with novel skeletons (Figure 2). The workflow included several processes such as Schrödinger-Glide molecular docking at different precisions, MM/GBSA, and Deepdock for high-precision evaluation. After multiple iterations, molecules with potential were screened for further evaluation. Ten molecules were screened in this study, and five of them exhibited good inhibition rates against WEE1 kinases. Compound 4 (GSK3182571) and compound 5 (Milciclib) showed comparable inhibitory activity to the positive molecules AZD1775. To explore the therapeutic potential of two compounds on tumor cells, we conducted anti-proliferation inhibitory assays in human lung cancer cells A549 and PC9, and human liver cancer cells Bel-7402 and HuH-7. Compound 4 demonstrated significant inhibitory effects on Bel-7402, HuH-7, and PC9 cells. Furthermore, we performed an analysis of apoptosis and cell cycle in PC9 cells after treated with compound 4, revealing its ability to induce apoptosis and arrest cell cycle in G1 phase. These results indicate that compound 4 has the potential to serve as a lead compound targeting WEE1, with prospects for further optimization. To gain a better understanding of the molecular interactions between compounds and their targets, we conducted molecular dynamics simulations. The investigation into the key contributions of residues around the binding pocket of compound 4 in its interaction with WEE1 could serve as a valuable theoretical reference for future structural modifications.
In the present study, we investigated the protein structures with the aim to discern strategies that could enrich high-affinity compounds specific to WEE1. High-definition structural data for these proteins were sourced from the RCSB Protein Data Bank. Specifically, the human-originated WEE1 protein structure, labeled 5VD8, was assessed, boasting a resolution of less than 2Å.
This protein was subjected to further preparation via the Schrödinger’s Protein Preparation Wizard module. Subsequent evaluations of the prepared proteins were conducted using the Glide_SP to ascertain the efficacy of these techniques and protein chains in differentiating between inhibitors and decoys. A substantial dataset, consisting of 389 validated WEE1 inhibitors obtained from ChEMBL database (Stein et al., 2021) and 139,320 random decoys acquired through DUDE-Z method (Mendez et al., 2019), was created, and these ligands were docked to the protein pocket. Throughout this docking procedure, scores were documented, facilitating the calculation of p-values. The achieved area under the curve (AUC) was 0.969 with a p-value falling below 0.001 (Figure 3A). This remarkable statistical significance underscores the robustness of our method in distinguishing between inhibitors and decoys. A frequency histogram evaluation illuminated that 5VD8 exhibits exemplary discriminatory capabilities and was thus selected for further screening processes (Figure 3B).
FIGURE 3. (A) Receiver operating characteristic (ROC) curve for evaluating virtual screening methods; (B) Docking score distribution of WEE1 inhibitors and decoys.
Our “in-house” database currently contains 160,0000 unique molecules sourced from the Maybridge compound database and MCE drug-like compound database. To ensure data accuracy, we utilized RDKit to preprocess and eliminate any redundant molecular structures. The resulting molecules were then transformed into 3D structures using Schrödingers LigPrep module.
The protein structure with PDB ID 5VD8 was retrieved from the RCSB protein database (https://www.rcsb.org/) for further preparation. The Protein Preparation Wizard in Schrödinger 2021–2 suite was used for protein preparation, which involved filling in missing hydrogens, partial charges, side chains, and loops, optimizing hydrogen bond assignments, and minimizing energy.
A multi-stage process was designed, which consisted of several key components. The initial screening was performed by Schrödinger-Glide molecular docking, using varying levels of precision to identify promising candidates. The selected molecules were then further evaluated and refined by MM/GBSA and Deepdock. This iterative and comprehensive screening approach ensures efficient exploration of potential WEE1 inhibitors. In this study, the Glide docking tool in the Schrödinger package was utilized for screening molecules in the internal database. The screening process consisted of two rounds, with the first round using standard precision (SP) to select the top 25,000 molecules with a score lower than or equal to −6.5 kcal/mol. The second round used ultra-precision (XP) with stricter restrictions on ligand-receptor shape complementarity, resulting in the selection of 2000 molecules with a score lower than or equal to −8 kcal/mol (Vass et al., 2012). MM/GBSA calculation and the geometric deep learning framework algorithm Deepdock were then used to predict the molecular and target affinity of the selected molecules (Sándor et al., 2010). Compared to traditional docking scoring functions, MM/GBSA has higher accuracy in predicting binding affinity by taking into account both molecular mechanical energy and solvation energy. The study followed standard MM/GBSA procedures as outlined in Schrödinger, including the preparation of the protein-ligand complex and energy minimization using the OPLS4 force field (Jorgensen et al., 1996; Halgren et al., 2004; Friesner et al., 2006). The use of the Deepdock model further improved the understanding of the interaction between small molecules and WEE1 protein by predicting the binding mode between ligand and target protein.
From the remaining molecules, a manual selection process was used to identify 10 compounds (Figure 4; Table 1). This selection method took into account the interaction pattern scores and molecular combinations from different software, while also excluding molecules with incorrect binding patterns. We then conducted empirical assessments by evaluating the binding scores, binding modes (with a focus on critical amino acid interactions), inherent properties of the molecules (molecular weight less than 600, hydrogen bond donor number less than 5, hydrogen bond acceptor less than 10, topologically polarized surface (TPSA) less than 120, rotational bond number (RotB) less than 10, calculated value of lipid water partition coefficient (clogP) less than 5, etc.), and molecular synthesizability. This determination aimed to identify molecules with higher binding affinities, while excluding those lacking potential for drug development.
The kinase activity of these ten compounds obtained by virtual screening were evaluated. Compounds 2, 3, 4, 5, and 8 exhibited a strong inhibitory effect on WEE1 protein, with an inhibition rate of almost 100% at a concentration of 25 μM (Figure 5; Table 2). Consequently, these five molecules were selected for further testing to determine their half maximal inhibitory concentration (IC50). The results indicated that five compounds displayed good inhibitory activity, with IC50 values less than 1 μM. Compound 4 and Compound 5 showed strongest inhibitory effect, displaying IC50 values of 1.069 and 3.77 nM, respectively. These IC50 values were comparable to the activity result of the positive control AZD1775, with an IC50 value of 0.786 nM. Therefore, the anti-proliferative activity of these two compounds was investigated in further studies.
FIGURE 5. (A) The inhibition rate (%) assay of ten selected compounds (25 μM) against WEE1; (B) The IC50 value of compound 4, compound 5 and AZD1775 against WEE1 kinase.
As shown in Figure 6, The anti-proliferation activity of compound 4 and compound 5 against lung cancer (A549 and PC9) and liver cancer (HuH-7) cells were studied. The results indicated that compound 4 exhibited better anti-proliferation activity than compound 5 in 3 cells. Moreover, PC9 cells demonstrated higher sensitivity to compound 4, with an IC50 value of 0.44 nM. Meanwhile, compound 4 displayed anti-proliferation activity against A549 and HuH-7 cells with the IC50 values 5.171 and 0.875 μM, respectively. Additionally, compound 4 was assessed for its anti-proliferation activity on liver cancer cell Bel-7402, and it exhibited promising activity with an IC50 of 0.147 μM. Thus, the kinase and cell antiproliferative activity evaluation suggested that compound 4 could be a potential WEE1 inhibitor, and therefore, it may be selected as the lead compound for structural optimization.
FIGURE 6. (A) The IC50 value of compound 4 and compound 5 against HuH-7 cell; (B) The IC50 value of compound 4 and compound 5 against A549 cells; (C) The IC50 value of compound 4 and compound 5 against PC9 cells; (D) The IC50 value of compound 4 against Bel-7402 cells.
To validate the inhibitory effects of WEE1 inhibitors in PC9 and A549 cells, Annexin V/PI was utilized to stain apoptotic cells (early apoptosis + late apoptosis). After PC9 cells were treated with 500 nM compound 4 or AZD1775 for 72 h, the percentage of apoptotic cells reached to 13.24%, 27.20%, and 84.75% in blank control group, AZD1775 group and compound 4 group, respectively. A549 cells were treated with 2 μM compound 4 or AZD1775 for 72 h, the percentage of apoptotic cells reached to 9.86%, 16.62%, and 17.73% in blank control group, AZD1775 group and compound 4 group, respectively. These results suggest that compound 4 is more effective than AZD1775 in inducing apoptosis in PC9 cells. Furthermore, compared to A549 cells (p53 wild type), compound 4 or AZD1775 can induce more apoptosis in PC9 cells (p53 mutant) (Figure 7).
FIGURE 7. Cell apoptosis analysis of compound 4. (A) PC9 cells were treated with AZD1775 or compound 4 (500 nM) for 72 h; (B) A549 cells were treated with AZD1775 or compound 4 (2 μM) for 72 h. Apoptosis rate = Q2% (late apoptosis rate) + Q3% (early apoptosis rate), (ANOVA multiple comparison tests).
As shown in Figure 8, the impact of compound 4 on cell cycle distribution of PC9 and A549 cells were studied by flow cytometry using propidium iodine (PI) staining. Results showed that when PC9 cells were treated with 500 nM compound 4 for 24 h, the proportion of cells in the G1 phase was significantly increased compared to the blank group, while the proportion of cells in the G2 phase did not change significantly. However, after A549 cells were treated with 2 μM compound 4 or AZD1775 for 24 h, there was no significant change on cell cycle distribution. These findings suggested that WEE1 inhibitor abolished G2/M phase arrest and increased the proportion of G1 phase cells, likely due to compound 4 also appeared to exert significant effects on the G1/S checkpoint. Moreover, in comparison to cells with wild-type p53, WEE1 inhibitors exert a more pronounced impact on the cell cycle of cells with p53 mutations.
FIGURE 8. Cell cycle analysis of compound 4. (A) PC9 cells were treated with AZD1775 or compound 4 (500 nM) for 24 h; (B) A549 cells were treated with AZD1775 or compound 4 (2 μM) for 24 h.
According to the results of biological evaluation, compound 4 was selected for MD simulation. The MD simulation was performed for 500 ns, and equilibrium was reached at 100 ns (Figure 9A). Figure 9B shows the RMSF plot for WEE1, indicating that the fluctuation of the amino acid residue index ranges from 0.5 Å to 4.5 Å. As demonstrated in Figure 9C, the data indicate that Cys379 interacted with compound 4 at nearly all time points. To explain these observations, we selected the last conformation for further analysis and plotted a schematic illustrating the detailed ligand atom interactions with specific protein residues. We observed that the hydrogen bond interactions with Cys379 mainly involved the nitrogen and amino groups on the pyrimidine of compound 4, as indicated in Figures 9D,E. In addition, compound 4 also interacts with Glu390 of WEE1 protein through hydrogen bonding interactions, which is different from that of AZD1775. Compound 4 interacts with Lys328 through water bridge interactions. The amino group on the benzene ring creates an intramolecular hydrogen bond with the carbonyl group, serving to stabilize the compound’s conformation. Importantly, this interaction of Cys379 is a well-established model and has also been observed in other WEE1 inhibitors.
FIGURE 9. (A) RMSD value of compound 4 (red) and WEE1 (blue); (B) The RMSF profile of WEE1 (PDB ID 5VD8) which represents local changes along the protein chain throughout simulation trajectory; (C) Timeline representation of WEE1-compound 4 interactions; (D) The 2D diagram of protein-ligand interaction. The purple arrows represented H-bond interactions and water bridges; (E) The major contributions of individual amino acid residues to the compound 4-WEE1 complex.
This research has utilized a robust virtual screening workflow to identify potential WEE1 inhibitors. Compounds 4 and 5 have been identified as potent inhibitors of WEE1 kinases. Particularly, compound 4 has demonstrated significant therapeutic potential against various cancer types, exhibiting inhibitory and anti-proliferation characteristics that are comparable to, if not better than, existing WEE1 inhibitors. The investigation of compound 4′s ability to induce apoptosis and its impact on the cell cycle further highlights its potential application in cancer treatment. These results confirm that compound 4 is a novel WEE1 inhibitor, which can be used as a potential lead compound for the further development of highly active molecules targeting the WEE1 signaling pathway. However, our study still has some limitations. For instance, the selectivity of compound 4 towards other kinases, as well as the pharmacokinetic properties and safety of compound 4, necessitate further experimental validation. In summary, these findings contribute significantly to the scientific understanding of WEE1 inhibitors and provide a foundation for future refinement of molecular structures and laboratory optimization.
Compound 1 (Denfivontinib, HY-12333), Compound 2 (AEE788, HY-10045), Compound 3 (ALK inhibitor 2, HY-15358), Compound 4 (GSK3182571, HY-12400), Compound 5 (Milciclib, HY-10424), Compound 6 (CX-6258 hydrochloride, HY-18095B), Compound 7 (GNE-317, HY-12763), Compound 8 (TCS7010, HY-70061), Compound 9 (LY2874455, HY-13304) and Compound 10 (LDN-214117, HY-16712) were purchased from MedChemExpress (Junction, NJ, United States).
The X-ray co-crystallized protein structure (PDB ID: 5VD8) was obtained from the RCSB Protein Database for further protein preparation (Zhu et al., 2017; Burley et al., 2023). Among the total of WEE1 protein structures recorded in the RCSB database, PDB ID: 5VD8 was selected as our target for virtual screening after filtering based on species and non-covalent ligand. The protein preparation process, including preprocessing, hydrogen bond assignment, and restrained minimization, was carried out using the Protein Preparation Wizard module from the Schrödinger 2021–2 suite (Sastry et al., 2013; Schrödinger, 2021a). During the preprocessing step, any issues with the protein were identified and resolved using default parameters, such as filling in missing side chains and loops. The hydrogen bond assignments were then optimized by sampling water orientations and using PROPKA at a pH of 7.4 (Rostkowski et al., 2011). Finally, a restrained energy minimization was performed, using the OPLS4 force field to converge the heavy atoms to an RMSD of 0.30 Å (Lu et al., 2021).
The in-house database used for screening was comprised of two components, the commercial Maybridge compound database and MCE’s drug-like molecule library. The preparation of all ligands was carried out using the default parameters of the LigPrep module from the Schrödinger 2021–2 suite (Schrödinger, 2021b). The Epik (version 5.6) program was utilized to perform hydrogenation, salt removal, tautomer generation, and calculation of ionization states, all under the OPLS4 force field and at a pH of 7.0 ± 2.0 (Shelley et al., 2007; Greenwood et al., 2010; Schrödinger, 2021c). Additionally, up to 32 stereoisomers can be generated for each ligand while preserving the specific chirality under the computational conditions.
To prepare for the subsequent docking stage, a file was generated containing the center coordinates of the protein pockets. The central coordinates of the original ligand were selected as a reference for the docking process. Subsequently, the original ligands were deleted, resulting in the creation of the grid file. These steps were performed using the receptor mesh generation module from the Schrödinger 2021–2 suite.
The virtual screening workflow described in this paper consists of a series of filtering steps, each increasing in precision. The process began by selecting the top 250,000 molecules from the in-house database through the use of the SP precision in Glide docking. These molecules were then screened through the XP precision of Glide docking, where 10 docking conformations were established for each compound. In the third step, 20,000 molecules obtained from the previous round of Glide docking were filtered through MM/GBSA to identify the molecules with the highest scores, which were determined using a combination of DeepDock and manual selection. Finally, 10 molecules were chosen for further analysis of their bioactivity.
The Glide molecular docking module of Schrödinger’s suite is a widely-used tool in the field of computational drug discovery, allowing for the prediction of the 3-dimensional structure of a small molecule when in complex with a protein target (Friesner et al., 2004). These interactions, determined by physical and chemical properties such as shape complementarity, hydrogen bonding, and electrostatic interactions, are crucial for understanding the efficacy and specificity of a drug candidate.
In this study, both the Glide SP and XP precision were utilized. The receptor grid file of the WEE1 target and ligand file were loaded into the system and the OPLS4 force field was selected. The desired precision level was set to determine the accuracy of the simulation, and the maximum number of output structures and number of poses per ligand were set to 5. The docking simulation was initiated by running the program.
The MM/GBSA method, which stands for Molecular Mechanics Generalized Born Surface Area, is a widely used computational technique for predicting the binding affinity of a small molecule to a protein target in computational drug discovery (Rastelli et al., 2010; Genheden and Ryde, 2015). It integrates the benefits of molecular mechanics and generalized Born methods to provide a precise and efficient estimation of binding free energy. In this study, the binding free energy was calculated using the MM/GBSA calculation method. The WEE1-ligand complexes obtained from docking were optimized using the local optimization feature in Prime wizard of Maestro (Schrödinger Release 2021–2) (Jacobson et al., 2002; Jacobson et al., 2004; Schrödinger, 2021d). The force field employed in this calculation was OPLS4, and the binding energy was determined based on a set of receptor and ligand. The equation used to calculate the binding free energy was as follows:
ΔG (bind) = ΔG (solv) + ΔE (MM) + ΔG (SA). The binding free energy was calculated using the MM/GBSA method, which combines molecular mechanics and generalized Born methods. The minimized energy of the protein-ligand complexes, represented as ΔE (MM), was determined using the OPLS4 force field. The solvation energy variance between the protein-ligand complexes and the sum of the solvation energies for the protein and ligand, represented as ΔG (Solv), was also considered. Furthermore, the difference in surface area energies for the complexes, represented as ΔG (SA), was included in the calculation. The minimization of the docked complexes was conducted using the local optimization feature of Prime in Maestro (Schrödinger Release 2021–2).
The DeepDock program on GitHub is a deep learning framework designed for protein-ligand docking, a computational approach that predicts the binding affinity between a protein and a small molecule (Liao et al., 2019). This program uses a convolutional neural network (CNN) to learn the correlation between the three-dimensional structures of the protein and the ligand and their binding affinity. The process started with the application of the masif algorithm to determine the interfacial characteristics of WEE1 when bound to a molecule.
The program inputs 2D molecular and protein pocket graphs and generates continuous representations. Based on these representations, it calculates a statistical potential based on the likelihood of distances between the protein and the molecule. Finally, it employs an optimization technique to produce the binding configuration of the molecule.
The tested compounds were dissolved in DMSO to make a 10 mM stock solution, which was further diluted to a drug solution of 25 µM. Initially, 2× ATP and substrate solution and 2× kinase and metal solution were prepared using assay buffer (Hepes 50 mM, MgCl2 2 mM, Brij35 0.01%, EGTA 1 mM, DTT 2 mM and ddH2O.). Transfer 20 nL compound to 384 assay plate by Echo 655. Then, 2 μL of 2× kinase and metal solution was mixed and incubated in a 384 assay plate for 10 min at 25°C. 2 μL of 2× substrate & ATP solution were added to the well, and incubated at 25°C for 60 min 4 μL of ADP-Glo reagent was added to the well, and incubated for 40 min at 25°C. 8 μL of kinase detection reagent was added to the well, and incubated for 40 min at 25°C. Read the RLU (relative light-emitting unit) signal using the BMG multifunction microplate reader, and the signal intensity is used to characterize the degree of kinase activity. We used Staurosporine (10 µM) as a positive control compound to calculate the relative inhibition rate. Staurosporine is a prototypical ATP-competitive kinase inhibitor in that it binds to many kinases with high affinity. The inhibition rates were calculated using the equations (X: log of inhibitor concentration; Y: % Inhibition). Assays were performed on three independent experiments.
For apoptosis detection, Cells were performed with Pharmingen FITC Annexin V Detection KitⅠ(BD Bioscience, Oxford, UK). Briefly, Cells were harvested and washed once with 1×binding buffer and then incubated with Annexin V and Propidium Iodide (PI) for 15 min in the dark at 37°C.
For cell cycle detection, Cells were harvested and gently washed with PBS, and fixed with 70% cold ethanol −20°C overnight. After centrifugation, Cells were retained for precipitation and incubated with PI/RNase Staining Buffer (BD Bioscience, Oxford, United Kingdom) for 15 min in the dark at 37°C.
All samples were acquired by flow cytometry (FACS CantoⅡ, BD Bioscience) and analysed with Flowjo software version 10.4.
Anti-proliferation activity assay was detected by Cell Counting Kit-8 detection kit (CCK-8, #K1018) provided by Apexbio (United States). In brief, cells were seeded in 96-wells plates at a density of 3 × 103/well for 24 h. Then, cells were treated with indicated concentrations of compounds for 72 h. Supernatant was totally removed, and 100 μL of CCK-8 solution was added to each well and cultured for another 1 h at 37°C. The absorbance of plates was measured by SpectraMax M2 (Molecular Devices, San Jose, CA, United States) at 450 nm. The inhibition rates were calculated for each wells as (1-(OD450 treated cells/OD450 control cells)) × 100%. Assays were performed on three independent experiments.
The compound 4 selected for complex formation with WEE1 underwent molecular dynamics simulations. The initial structure of WEE1-compound 4 used for MD simulations was obtained by extra-precision (XP) docking (Schrödinger, 2021e). It was first processed through the Protein Preparation Wizard module. The input system was built using the System Builder module from the Schrödinger 2021–2 Suite. With default parameters, the complex molecule was placed at the center of a box filled with SPC water molecules, and the ensemble class for molecular dynamics simulations was set to NPT. The temperature was kept at 300K, and the boundaries of the box were established 10 Å away from the farthest radius of the protein. 0.15 M NaCl, was added to balance the system charge, with energy minimized to 100 ps? The prepared model contained 34,412 atoms was loaded into the Molecular Dynamics module for further simulation. The simulation duration was set at 500 ns, with a recording interval of 1 ns for each recording. The dynamics simulations were performed under OPLS4 force field at the temperature of 300 K and the pressure of 1.01325 bar. The results of the simulations were analyzed using Simulation Interaction Analysis Diagram.
The results were presented as mean ± SD of three independent experiments. We analyzed data with GraphPad Prism 8.0 software (San Diego, CA, United States). The significance of differences between two groups were determined by unpaired Student’s t tests and ANOVA multiple comparison tests. Statistically significant p values were labelled as: *p < 0.05, **p < 0.01, ***p < 0.001, ****p < 0.0001.
The raw data supporting the conclusion of this article will be made available by the authors, without undue reservation.
Ethical approval was not required for the studies on animals in accordance with the local legislation and institutional requirements because only commercially available established cell lines were used.
TJ: Writing–original draft. WX: Writing–review and editing. RC: Formal Analysis, Writing–original draft. LS: Data curation, Writing–original draft. JG: Formal Analysis, Writing–original draft. LX: Methodology, Writing–original draft. XC: Methodology, Writing–original draft. NL: Supervision, Writing–review and editing. LZ: Supervision, Writing–review and editing. ZS: Conceptualization, Project administration, Writing–review and editing. BZ: Supervision, Writing–review and editing.
The author(s) declare financial support was received for the research, authorship, and/or publication of this article. This work was supported by National Natural Science Foundation (82073297), Department of Science and Technology of Zhejiang Province Key R&D Projects (2022C03155 and 2023C03112), Zhejiang Provincial Program for the Cultivation of High-level Leading Health Talents (2020-18), Clinical Pharmacy of Hangzhou Medical Key Discipline (2021-21-16).
The authors declare that the research was conducted in the absence of any commercial or financial relationships that could be construed as a potential conflict of interest.
All claims expressed in this article are solely those of the authors and do not necessarily represent those of their affiliated organizations, or those of the publisher, the editors and the reviewers. Any product that may be evaluated in this article, or claim that may be made by its manufacturer, is not guaranteed or endorsed by the publisher.
The Supplementary Material for this article can be found online at: https://www.frontiersin.org/articles/10.3389/fphar.2023.1298245/full#supplementary-material
Burley, S. K., Bhikadiya, C., Bi, C., Bittrich, S., Chao, H., Chen, L., et al. (2023). RCSB Protein Data Bank: powerful new tools for exploring 3D structures of biological macromolecules for basic and applied research and education in fundamental biology, biomedicine, biotechnology, bioengineering and energy sciences. Nucleic Acids Res. 51, D437–D451. doi:10.1093/nar/gkaa1038
Diab, A., Kao, M., Kehrli, K., Kim, H. Y., Sidorova, J., and Mendez, E. (2019). Multiple defects sensitize p53-deficient head and neck cancer cells to the WEE1 kinase inhibition. Mol. Cancer Res. 17, 1115–1128. doi:10.1158/1541-7786.MCR-18-0860
Dillon, M. T., Good, J. S., and Harrington, K. J. (2014). Selective targeting of the G2/M cell cycle checkpoint to improve the therapeutic index of radiotherapy. Clin. Oncol. R. Coll. Radiol.) 26, 257–265. doi:10.1016/j.clon.2014.01.009
Do, K., Doroshow, J. H., and Kummar, S. (2013). WEE1 kinase as a target for cancer therapy. Cell cycleGeorget. Tex.) 12, 3159–3164. doi:10.4161/cc.26062
Friesner, R. A., Banks, J. L., Murphy, R. B., Halgren, T. A., Klicic, J. J., Mainz, D. T., et al. (2004). Glide: a new approach for rapid, accurate docking and scoring. 1. Method and assessment of docking accuracy. J. Med. Chem. 47, 1739–1749. doi:10.1021/jm0306430
Friesner, R. A., Murphy, R. B., Repasky, M. P., Frye, L. L., Greenwood, J. R., Halgren, T. A., et al. (2006). Extra precision glide: docking and scoring incorporating a model of hydrophobic enclosure for protein-ligand complexes. J. Med. Chem. 49, 6177–6196. doi:10.1021/jm051256o
Fu, S., Wang, Y., Keyomarsi, K., Meric-Bernstam, F., and Meric-Bernstein, F. (2018). Strategic development of AZD1775, a WEE1 kinase inhibitor, for cancer therapy. Expert Opin. Investig. Drugs 27, 741–751. doi:10.1080/13543784.2018.1511700
Geenen, J. J. J., and Schellens, J. H. M. (2017). Molecular pathways: targeting the protein kinase WEE1 in cancer. Clin. Cancer Res. 23, 4540–4544. doi:10.1158/1078-0432.CCR-17-0520
Genheden, S., and Ryde, U. (2015). The MM/PBSA and MM/GBSA methods to estimate ligand-binding affinities. Expert Opin. Drug Discov. 10, 449–461. doi:10.1517/17460441.2015.1032936
Greenwood, J. R., Calkins, D., Sullivan, A. P., and Shelley, J. C. (2010). Towards the comprehensive, rapid, and accurate prediction of the favorable tautomeric states of drug-like molecules in aqueous solution. J. Comput. Aided Mol. Des. 24, 591–604. doi:10.1007/s10822-010-9349-1
Ha, D.-H., Min, A., Kim, S., Jang, H., Kim, S. H., Kim, H.-J., et al. (2020). Antitumor effect of a WEE1 inhibitor and potentiation of olaparib sensitivity by DNA damage response modulation in triple-negative breast cancer. Sci. Rep. 10, 9930. doi:10.1038/s41598-020-66018-5
Halgren, T. A., Murphy, R. B., Friesner, R. A., Beard, H. S., Frye, L. L., Pollard, W. T., et al. (2004). Glide: a new approach for rapid, accurate docking and scoring. 2. Enrichment factors in database screening. J. Med. Chem. 47, 1750–1759. doi:10.1021/jm030644s
Huang, P. Q., Boren, B. C., Hegde, S. G., Liu, H., Unni, A. K., Abraham, S., et al. (2021). Discovery of ZN-c3, a highly potent and selective WEE1 inhibitor undergoing evaluation in clinical trials for the treatment of cancer. J. Med. Chem. 64, 13004–13024. doi:10.1021/acs.jmedchem.1c01121
Jacobson, M. P., Friesner, R. A., Xiang, Z., and Honig, B. (2002). On the role of the crystal environment in determining protein side-chain conformations. J. Mol. Biol. 320, 597–608. doi:10.1016/s0022-2836(02)00470-9
Jacobson, M. P., Pincus, D. L., Rapp, C. S., Day, T. J., Honig, B., Shaw, D. E., et al. (2004). A hierarchical approach to all-atom protein loop prediction. Proteins 55, 351–367. doi:10.1002/prot.10613
Jorgensen, W. L., Maxwell, D. S., and Tirado-Rives, J. (1996). Development and testing of the OPLS all-atom force field on conformational energetics and properties of organic liquids. J. Am. Chem. Soc. 118, 11225–11236. doi:10.1021/ja9621760
Leijen, S., van Geel, R. M., Pavlick, A. C., Tibes, R., Rosen, L., Razak, A. R., et al. (2016). Phase I study evaluating WEE1 inhibitor AZD1775 as monotherapy and in combination with gemcitabine, cisplatin, or carboplatin in patients with advanced solid tumors. J. Clin. Oncol. 34, 4371–4380. doi:10.1200/JCO.2016.67.5991
Li, Z., Pinch, B. J., Olson, C. M., Donovan, K. A., Nowak, R. P., Mills, C. E., et al. (2020). Development and characterization of a WEE1 kinase degrader. Cell Chem. Biol. 27, 57–65. doi:10.1016/j.chembiol.2019.10.013
Liao, Z., You, R., Huang, X., Yao, X., Huang, T., and Zhu, S. (2019). “DeepDock: enhancing ligand-protein interaction prediction by a combination of ligand and structure information,” in 2019 IEEE International Conference on Bioinformatics and Biomedicine (BIBM), San Diego, CA, USA, November, 2019, 311–317.
Lin, C.-C., Grewal, J. S., Sommerhalder, D., Strauss, J. F., Bai, L.-Y., Shen, L., et al. (2022). A phase 1 dose-escalation and -expansion study of IMP7068, a WEE1 inhibitor, in patients with advanced solid tumors. J. Clin. Oncol. 40, e15052. doi:10.1200/jco.2022.40.16_suppl.e15052
Liu, D., Cao, Z., Xu, W., Lin, G., Zhou, X., Ding, X., et al. (2019). Enhancement of chemosensitivity by WEE1 inhibition in EGFR-TKIs resistant non-small cell lung cancer. Biomed. Pharmacother. 117, 109185. doi:10.1016/j.biopha.2019.109185
Lu, C., Wu, C., Ghoreishi, D., Chen, W., Wang, L., Damm, W., et al. (2021). OPLS4: improving force field accuracy on challenging regimes of chemical space. J. Chem. Theory Comput. 17, 4291–4300. doi:10.1021/acs.jctc.1c00302
Matheson, C. J., Backos, D. S., and Reigan, P. (2016). Targeting WEE1 kinase in cancer. Trends Pharmacol. Sci. 37, 872–881. doi:10.1016/j.tips.2016.06.006
Mendez, D., Gaulton, A., Bento, A. P., Chambers, J., De Veij, M., Felix, E., et al. (2019). ChEMBL: towards direct deposition of bioassay data. Nucleic Acids Res. 47, D930-D940–D940. doi:10.1093/nar/gky1075
Ou, H. L., and Schumacher, B. (2018). DNA damage responses and p53 in the aging process. Blood 131, 488–495. doi:10.1182/blood-2017-07-746396
Papadopoulos, K. P., Sharma, M., Dummer, R., Rodrigo Imedio, E., Yge, M.-L., Micallef, S., et al. (2022). A phase 1 dose-finding and dose-expansion study evaluating the safety, tolerability, pharmacokinetics, and efficacy of a highly selective WEE1 inhibitor (Debio 0123) in adult patients with advanced solid tumors. J. Clin. Oncol. 40, TPS2702. doi:10.1200/jco.2022.40.16_suppl.tps2702
Rastelli, G., Del Rio, A., Degliesposti, G., and Sgobba, M. (2010). Fast and accurate predictions of binding free energies using MM-PBSA and MM-GBSA. J. Comput. Chem. 31, 797–810. doi:10.1002/jcc.21372
Rostkowski, M., Olsson, M. H., Sondergaard, C. R., and Jensen, J. H. (2011). Graphical analysis of pH-dependent properties of proteins predicted using PROPKA. BMC Struct. Biol. 11, 6. doi:10.1186/1472-6807-11-6
Sándor, M., Kiss, R., and Keseru, G. M. (2010). Virtual fragment docking by Glide: a validation study on 190 protein-fragment complexes. J. Chem. Inf. Model. 50, 1165–1172. doi:10.1021/ci1000407
Sastry, G. M., Adzhigirey, M., Day, T., Annabhimoju, R., and Sherman, W. (2013). Protein and ligand preparation: parameters, protocols, and influence on virtual screening enrichments. J. Comput. Aided Mol. Des. 27, 221–234. doi:10.1007/s10822-013-9644-8
Schrödinger, (2021a). Protein preparation wizard; epik, impact, Prime. New York, NY, USA: Schrödinger, LLC.
Schrödinger, (2021e). “Desmond molecular dynamics system, D. E. Shaw research,” in Maestro-desmond interoperability tools (New York, NY, USA: Schrödinger).
Shelley, J. C., Cholleti, A., Frye, L. L., Greenwood, J. R., Timlin, M. R., and Uchimaya, M. (2007). Epik: a software program for pK(a) prediction and protonation state generation for drug-like molecules. J. Comput. Aided Mol. Des. 21, 681–691. doi:10.1007/s10822-007-9133-z
Stein, R. M., Yang, Y., Balius, T. E., O'Meara, M. J., Lyu, J., Young, J., et al. (2021). Property-unmatched decoys in docking benchmarks. J. Chem. Inf. Model 61, 699–714. doi:10.1021/acs.jcim.0c00598
Vakili-Samiani, S., Khanghah, O. J., Gholipour, E., Najafi, F., Zeinalzadeh, E., Samadi, P., et al. (2022). Cell cycle involvement in cancer therapy; WEE1 kinase, a potential target as therapeutic strategy. Mutat. Res. 824, 111776. doi:10.1016/j.mrfmmm.2022.111776
Vass, M., Tarcsay, Á., and Keserű, G. M. (2012). Multiple ligand docking by Glide: implications for virtual second-site screening. J. Comput. Aided Mol. Des. 26, 821–834. doi:10.1007/s10822-012-9578-6
Zhang, M., Dominguez, D., Chen, S., Fan, J., Qin, L., Long, A., et al. (2017). WEE1 inhibition by MK1775 as a single-agent therapy inhibits ovarian cancer viability. Oncol. Lett. 14, 3580–3586. doi:10.3892/ol.2017.6584
Keywords: virtual screening, WEE1, molecular dynamics, geometric deep learning, cell cycle
Citation: Jin T, Xu W, Chen R, Shen L, Gao J, Xu L, Chi X, Lin N, Zhou L, Shen Z and Zhang B (2023) Discovery of potential WEE1 inhibitors via hybrid virtual screening. Front. Pharmacol. 14:1298245. doi: 10.3389/fphar.2023.1298245
Received: 21 September 2023; Accepted: 28 November 2023;
Published: 07 December 2023.
Edited by:
Brian Gabrielli, The University of Queensland, AustraliaReviewed by:
Moataz Ahmed Shaldam, Kafrelsheikh University, EgyptCopyright © 2023 Jin, Xu, Chen, Shen, Gao, Xu, Chi, Lin, Zhou, Shen and Zhang. This is an open-access article distributed under the terms of the Creative Commons Attribution License (CC BY). The use, distribution or reproduction in other forums is permitted, provided the original author(s) and the copyright owner(s) are credited and that the original publication in this journal is cited, in accordance with accepted academic practice. No use, distribution or reproduction is permitted which does not comply with these terms.
*Correspondence: Nengming Lin, bG5tMTAxM0B6anUuZWR1LmNu Lixin Zhou, emhvdWx4aW44a0AxMjYuY29t; Zheyuan Shen, MTgyODAwQHpqdS5lZHUuY24= Bo Zhang, emhhbmdibzEwMDlAemp1LmVkdS5jbg==
Disclaimer: All claims expressed in this article are solely those of the authors and do not necessarily represent those of their affiliated organizations, or those of the publisher, the editors and the reviewers. Any product that may be evaluated in this article or claim that may be made by its manufacturer is not guaranteed or endorsed by the publisher.
Research integrity at Frontiers
Learn more about the work of our research integrity team to safeguard the quality of each article we publish.