- 1Department of Laboratory Medicine, The First Affiliated Hospital of Nanjing Medical University, Nanjing, China
- 2Branch of National Clinical Research Center for Laboratory Medicine, Nanjing, China
Introduction: Non-small cell lung cancer (NSCLC) exhibits heterogeneity with diverse immune cell infiltration patterns that can influence tumor cell behavior and immunotherapy. A comprehensive characterization of the tumor microenvironment can guide precision medicine.
Methods: Here, we generated a single-cell atlas of 398170 cells from 52 NSCLC patients, and investigated the imprinted genes and cellular crosstalk for macrophages. Subsequently, we evaluated the effect of tumor cells on macrophages and verified the expression of marker genes using co-culture experiments, flow cytometry and RT-qPCR assays.
Results: Remarkable macrophage adaptability to NSCLC environment was observed, which contributed to generating tumor-associated macrophages (TAMs). We identified 5 distinct functional TAM subtypes, of which the majority were SELENOP-positive macrophages, with high levels of SLC40A1 and CCL13. The TAMs were also involved in mediating CD8+ T cell activity and form intercellular interaction with cancer cells, as indicated by receptor-ligand binding. Indirect coculture of tumor cells SPC-A1 and THP-1 monocytes, produced M2-like TAMs that highly expressed several markers of SELENOP-positive macrophages. The abundance of this type TAMs seemed to be associated with poorer overall survival rates [hazard ratio (HR) = 1.34, 95% confidence interval (CI) = 0.98-1.83, p = 0.068] based on deconvolution of TCGA-LUAD dataset.
Discussion: In summary, we provided a high-resolution molecular resource of TAMs, and displayed the acquired properties in the tumor microenvironment. Dynamic crosstalk between TAMs and tumor cells via multiple ligand-receptor pairs were revealed, emphasizing its role in sustaining the pro-tumoral microenvironment and its implications for cancer therapy.
Introduction
Lung cancer is one of the most common cancers worldwide, and it remains the leading cause of cancer-related deaths (Sung et al., 2021). Although immune checkpoint inhibitors have shown remarkable efficacy in treating non-small cell lung cancer (NSCLC), only a fraction of patients respond to these therapies (Zhang Y. et al., 2023; Lin et al., 2023). Identifying the factors that contribute to the development and progression of lung cancer is critical for improving patient outcomes. In recent years, single-cell sequencing (scRNA-seq) technology has been utilized to depict the immune microenvironment of NSCLC, which offered insight into the complex interactions between tumor cells and immune cells, primarily mediated through cytokines and chemokines (Wang et al., 2022; Pai et al., 2023). This technology allows for a granular analysis, uncovering diverse cell populations within the tumor ecosystem and their molecular features, shedding light on potential therapeutic targets.
Tumor-associated macrophages (TAMs) are an essential component of the tumor microenvironment (TME) surrounding cancer cells. TAMs exhibit a heterogeneous population of macrophages that are derived from tissue-resident macrophages, monocytes, and bone marrow-derived progenitor cells. They accumulate progressively during disease progression, with high densities of TAMs being associated with poor prognosis in lung cancer patients (Garrido-Martin et al., 2020; Yoshida et al., 2021). The polarization of TAMs has been studied extensively, and two main phenotypes have been described: M1 and M2. M1 macrophages produce pro-inflammatory cytokines, involved in tumor cell killing, whereas M2 macrophages release anti-inflammatory cytokines and promote tumor growth and angiogenesis (Zhang H. et al., 2021). The function of airway macrophages can be epigenetically regulated and resulting transcriptomic and phenotypical changes (Hey et al., 2021). The majority of scRNA-seq studies have reported that the phenotype of TAMs is plastic and can be reprogrammed, contributing to exert a mixture of phenotypic characteristics (Kim et al., 2020). Targeting these modified macrophages could potentially offer therapeutic avenues for patients with lung diseases.
Recent advances in tumor biology have revealed that the differentiation and intricate communication of TAMs can be leveraged as a promising target for the development of effective therapies aimed at disrupting critical interactions within the tumor microenvironment. Ruella et al. reported that immunosuppressive M2-type TAM expressed high levels of CD123 in Hodgkin lymphoma TME, and anti-CD123 chimeric antigen receptor (CAR) T cells could recognize and kill TAMs thereby overcoming immunosuppression, representing a promising new therapeutic approach (Ruella et al., 2017). Sánchez-Paulete and colleagues developed CAR-T cells targeting macrophages to achieve significant antitumor efficacy and reprogram the immunosuppressive TME in mouse models of lung, ovarian, and pancreatic cancer (Sánchez-Paulete et al., 2022). Liang et al. engineered a macrophage-mediated cellular phagocytosis-boosting hydrogel that reshaped the TME, leading to the acceleration of TAMs’ polarization into the anti-tumoral M1-like phenotype and the initiation of tumor-specific CD8+ T cell responses (Liang et al., 2023).
In the present study, we conducted an integrative inference of previously published scRNA-seq data to profile the TME of NSCLC, characterizing the interconvertibility and interaction among different types of macrophages, with a particular focus on the cellular crosstalk of TAMs. We reported the comprehensive single-cell transcriptome profiling of NSCLC covering 52 patients, and unveiled cellular dynamics and molecular features associated with the tumor progression for TAMs, thus extending our understanding of adaptive immune system. Deciphering the foundational cellular mechanisms and orchestrating these interactions holds the potential approach for indirectly impeding the interplay among cancer cells. This novel avenue could significantly advance the creation of effective and secure therapeutic strategies in the battle against cancer. The workflow for this study is depicted in Figure 1.
Methods
Single-cell RNA-seq datasets collected in this study
The scRNA-seq data of NSCLC used in this study were downloaded from website (http://lungcancer.chenlulab.com(Lambrechts et al., 2018; Zhang et al., 2022), https://doi.org/10.24433/CO.0121060.v1(Bischoff et al., 2021). To ensure the stability and comparability of integration, scRNA-seq datasets based on the 10x Genomics Chromium platform were included. Cell annotation tables along with quality control metrics were obtained from the original publications. The quality-passed cells were used for downstream analysis.
Normalization and integration of scRNA-seq data
Cells with fewer than 200 genes detected or > 40% mitochondrial counts or > 50% ribosomal counts were removed for following analysis; genes detected in > 3 cells and with unique molecular identified (UMI) count > 1,000 were kept. We ran the Scrublet algorithm to eliminate any potential doublets, setting the expected doublet rate to 0.05 (Wolock et al., 2019). Subsequently, individual datasets were merged into a single AnnData object, and then were integrated using the scVI algorithm. This algorithm has demonstrated its capability as one of the top-performing methods for integrating atlas-level data and scaling to over one million cells (Lopez et al., 2018). Two-dimension Uniform Manifold Approximation and Projection (UMAP) embeddings and unsupervised Leiden-clustering with scanpy were computed based on a cell-cell neighborhood graph derived from scVI latent space (Becht et al., 2018). Cell clusters in the resulting two-dimensional representation were annotated to known biological cell types using canonical marker genes.
Identification of differentially expressed genes
We applied the Wilcoxon Rank-Sum test in FindAllMarkers function to identify differentially expressed genes for each cell clusters, with the following parameters: only considering positive markers, fraction of expressing cells inside the cluster to be ≥ 0.25, log fold change between cells inside and outside the cluster to be ≥ 0.25. Based on the subpopulation-stratified scRNA-Seq data, pseudo-bulk samples were created for differential expression assessment, and samples with fewer than 10 cells were removed.
Scoring samples for M1/M2 polarization signatures
To comprehend the characteristics of distinct macrophage subsets, scores for M1/M2 polarization were acquired utilizing the AddModuleScore function within the “Seurat” package. M1 and M2 gene profiles were obtained from the study conducted by Azizi et al (Azizi et al., 2018).
Receptor–ligand communication between cell types
We used the cellphonedb (CPDB) database to investigate differences in cell-to-cell communication (Efremova et al., 2020), and identified differentially expressed signaling molecules through this analysis (Salcher et al., 2022). Furthermore, using NicheNet, we additionally identified receptor-ligand interactions that are likely to have an impact on particular gene-expression changes in a target cell lineage with the prior knowledge on signaling and gene regulatory networks (Browaeys et al., 2020).
Estimating cell-type proportions in the lung adenocarcinoma bulk RNA-seq from LUAD TCGA
The level 3 RNA-sequencing data (HTseq counts) along with metadata were downloaded from the TCGA (The Cancer Genome Atlas), specifically by selecting “lung adenocarcinoma (LUAD)” in the GDC data portal (portal.gdc.cancer.gov). Bulk RNA-seq counts were converted to transcripts per million (TPM) and matrices were deconvoluted with CIBERSORTx using scRNA-seq annotations (Newman et al., 2019). The reference matrix was created by randomly downsampling each cell-type to a maximum of 500 cells, and then utilized to generate a signature matrix file.
The TCGA-LUAD dataset comprised 530 valid cases, with a median overall survival time of 50.0 months, and out of these, 188 patients died. The prognostic significance of macrophage marker expression and percentage of macrophage subpopulation for overall survival was assessed using Kaplan-Meier curves. Univariate Cox regression analysis was applied to calculate hazard ratios (HRs) and their corresponding 95% confidence intervals (CIs).
Cell culture and polarization of THP-1
The human lung cancer cell line SPC-A1 and human monocyte-like cells (THP-1) were purchased from the Chinese Academy of Sciences, China. Cells were cultured in RPMI 1640 medium (Gibco, USA), and supplemented with 10% fetal bovine serum (FBS) and 1% penicillin/streptomycin. After THP-1 cells were treated with phorbol 12-myristate 13-acetate (PMA, 180 ng/ml) for 24 h, the cells differentiated into macrophages, and then co-cultured with SPC-A1. At termination, tumors were excised, and single-cell suspensions were prepared, and then stained with anti-CD68-APC and anti-CD163-PE (BioLegend) and analyzed by flow cytometry.
Reverse transcription quantitative polymerase chain reaction (RT-qPCR)
Total RNA was extracted from cells using TRIzol reagent (Invitrogen, USA; 15596026), and Primescript RT Reagent Kit (TaKaRa, Japan; RR036A) was used for reverse transcription. A 7500 Real-Time PCR System (Applied Biosystems, USA) was applied for RT-qPCR using SYBR Premix Ex Taq Kit (TaKaRa, Japan; RR091A). The primers used for amplification were listed in Supplementary Table S1.
Quantification and statistical analysis
Utilizing the Wilcoxon Rank-Sum test or the two-sided Student’s t-test, differential analysis was conducted between the two groups. The Kruskal-Wallis test was used to analyze differences among multiple pairwise comparisons. p-values under 0.05 were regarded as significant.
Results
Transcriptomic characterization of the TME in NSCLC by comprehensively integrating scRNA-seq data
In order to depict the TME of NSCLC, we integrated and analyzed scRNA-seq data from three studies comprising 137 samples and 52 patients (Supplementary Table S2). The scVI tool was used to remove the batch effect, which yielded a total of 398170 cells. After quality control, normalization, identification of most variable genes, and dimensionality reduction, UMAP analyses created 26 unsupervised clusters (Figure 2A). Based on the expression levels of cell-type-specific markers, we identified 18 major cell populations, including alveolar type I cells (AT1, marked with AGER, CLIC5 and PDPN), alveolar type II cells (AT2, marked with LPCAT1, NAPSA, PGC and SLC34A2), basal cells (Basal, marked with KRT17, KRT5 and KRT6A), ciliated cells (Cilia, marked with AKAP14, ALDH3B1, ANKRD66, C11orf88, C11orf97 and DNAI1), club cells (Club, marked with PIGR, SCGB1A1 and SCGB3A1), endothelial cells (EC, marked with CDH5, CLDN5 and RAMP2), fibroblasts (Fib, marked with C1R, COL1A2 and DCN), macrophages (Mφ, marked with CD68, CD86, FCGR1A, ITGAX and CD163), Monocytes (marked with FCN1, CXCL8, EREG, S100A12), dendritic cells (DC, marked with C1orf54, LGALS2 and MZB1), mast cells (Mast, marked with KIT, MS4A2, PTGS1 and RGS13), B cells and plasma cells (marked with CD19, CD79A and MS4A1), natural killer cells (NK, marked with GNLY and NKG7), regular T cells (Tregs, marked with FOXP3, IL2RA and TNFRSF4), CD4+ T cells (T_CD4, marked with CD3D, CD3E, CD3G and CD4) and CD8+ T cells (T_CD8, marked with CD8A, CD8B and GZMK, Figures 2B, C). Based on the tissue of origin, epithelial cell compartments were classified as normal or malignant cell clusters (Figure 2D), which was mainly consistent with the copy-number status of cells (Supplementary Figure S1). Besides, the dominant malignant cells in NSCLC were AT2 and basal cells.
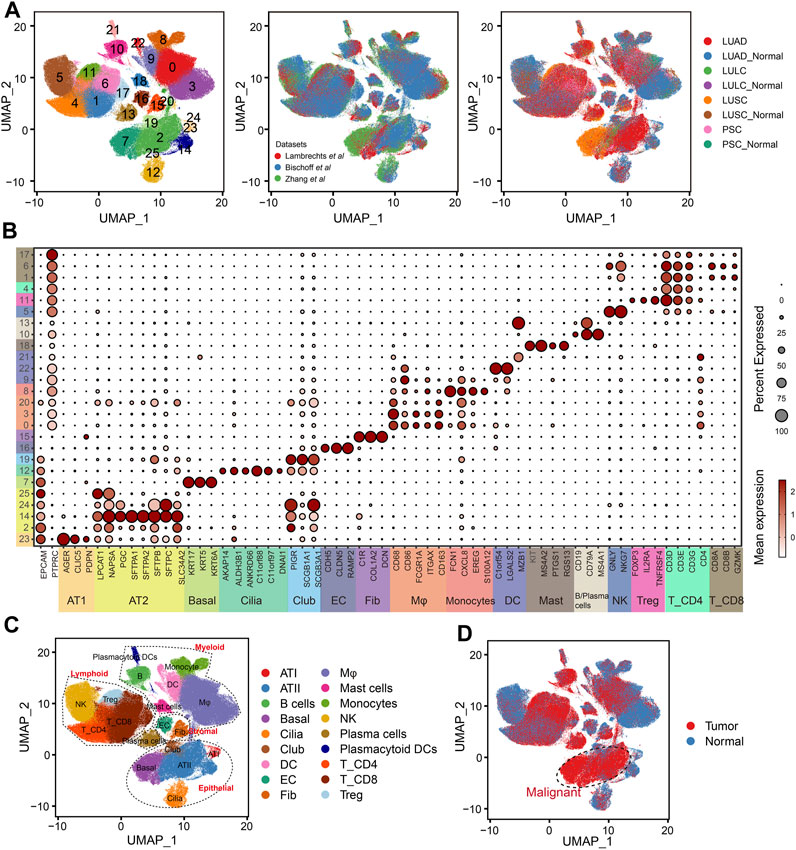
FIGURE 2. Integration of NSCLC from three scRNA-seq datasets. (A) UMAP plots showing the integration of three scRNA-seq datasets by scVI tool. (B) Dot plot displaying average and percent expression of marker genes for clusters in Figure 2A. UMAP plots showing the overview of NSCLC atlas colored by (C) major cell types, and (D) tissue type.
Characterization of the NSCLC immune cell atlas
Single-cell immune profiling could accurately reveal the contribution of the TME, making it possible to detect diverse immune cell compositions within different cancer types. The proportion of eleven immune cell lineage varied between LUAD and LUSC (Figure 3A), revealing a heterogeneous cellular status. In addition, we found that the frequency of macrophages was significant higher in LUAD than LUSC (p = 0.016, Figure 3B). Salcher et al. have revealed four distinct tumor immune phenotypes in NSCLC, (i) immune-desert (ID); (ii) B cell dominance (B); (iii) myeloid dominance (M); and (iv) T cell dominance (T) (Salcher et al., 2022). As shown in Figure 3C, correlating immune cell population frequencies in NSCLC identified an intriguing phenomenon that patients with LUAD had high infiltration of the macrophages (the subtype of tumors with myeloid dominance, M).
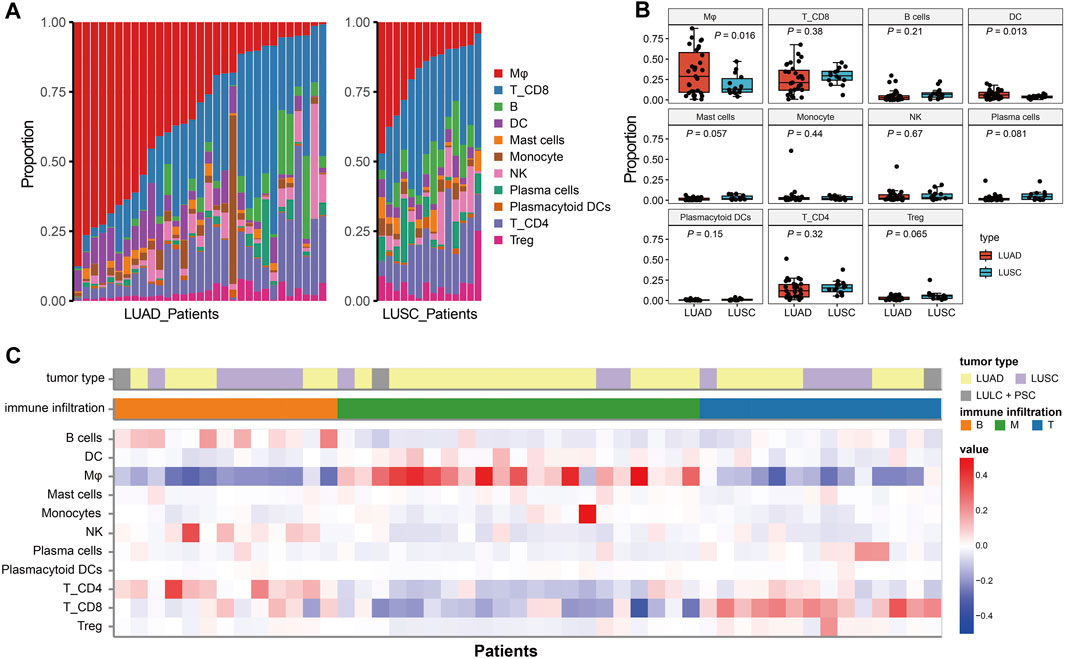
FIGURE 3. The immune infiltration phenotypes in NSCLC based on scRNA-seq. (A): Proportion of each immune cell type in LUAD and LUSC patients. (B) Bar plots represent the distribution of eleven immune cell types between LUAD and LUSC. (C) Characteristics of the NSCLC patients and classification of the tumor immune phenotypes.
Diversity within macrophage subsets in NSCLC
TAMs have diverse functions in cancer with both anti- and pro-tumorigenic properties (Ma et al., 2022). Analysis of the single-cell transcriptomic profile of 91260 macrophage cells, revealed 8 distinct clusters, including 3 normal macrophage clusters and 5 TAM clusters (Figure 4A). Compared to normal macrophage, TAMs highly expressed EBI3 which is subunit of the composite cytokines IL-27 and IL-35 and monocyte differentiation markers, such as F13A1, CCR2 and CSF1R (Figure 4B). As shown in Figure 4C, TAM_c1 cluster was characterized by a high expression of SELENOP and CCL13, that have been related to M2 polarization (Solinas et al., 2010; Sathe et al., 2020). Meanwhile, SLC40A1 is highly expressed on this subset where it mediates iron efflux from the breakdown of haem. TAM_c2 cluster displayed high expression of SPP1, which was reported to mediate macrophage polarization and associated with an immunosuppressive TME (Zhang et al., 2017). TIMP1 was significantly elevated in this subcluster, that play a crucial role in extracellular matrix regulation (Ishihara et al., 2023). There is an upregulation of M1 polarized markers (proinflammatory cytokines CXCL10 and IL1B) in the TAM_c3 subcluster. TAM_c4 showed high expression of mitochondrial genes reflecting enhanced ATP metabolism. Finally, we observed TAM_c5 subpopulation displaying high expression of LUAD markers such as SCGB3A1, SCGB3A2 and SFTPB, that seemed to have anti-inflammatory effects and anti-fibrotic activity in lung (Cai et al., 2014). The transcriptional profiles of the TAM subsets indicated their heterogeneity and plasticity. Gene set variation analysis (GSVA) of hallmark pathways revealed there were increased activities of inflammatory response and glycolysis in TAMs (Supplementary Figure S2). We further assessed the M1, and M2 signatures of different TAM subsets. The classical M1/M2 model could not completely explain the polarization of macrophages, and both M1 and M2 associated genes frequently expressed in the same subsets. TAM_c1, c2 and c4 subsets showed high anti-inflammatory M2 scores, and TAM_c3 subset had high pro-inflammatory M1 score (Figure 4D). We further calculated the fractions of all TAM clusters in 31 LUAD and 14 Lung squamous cell carcinoma (LUSC) patients. The distribution of TAM c1-c4 clusters was not significant between LUAD and LUSC, whereas a higher fraction of TAM_c5 were observed in LUAD (Figure 4E).
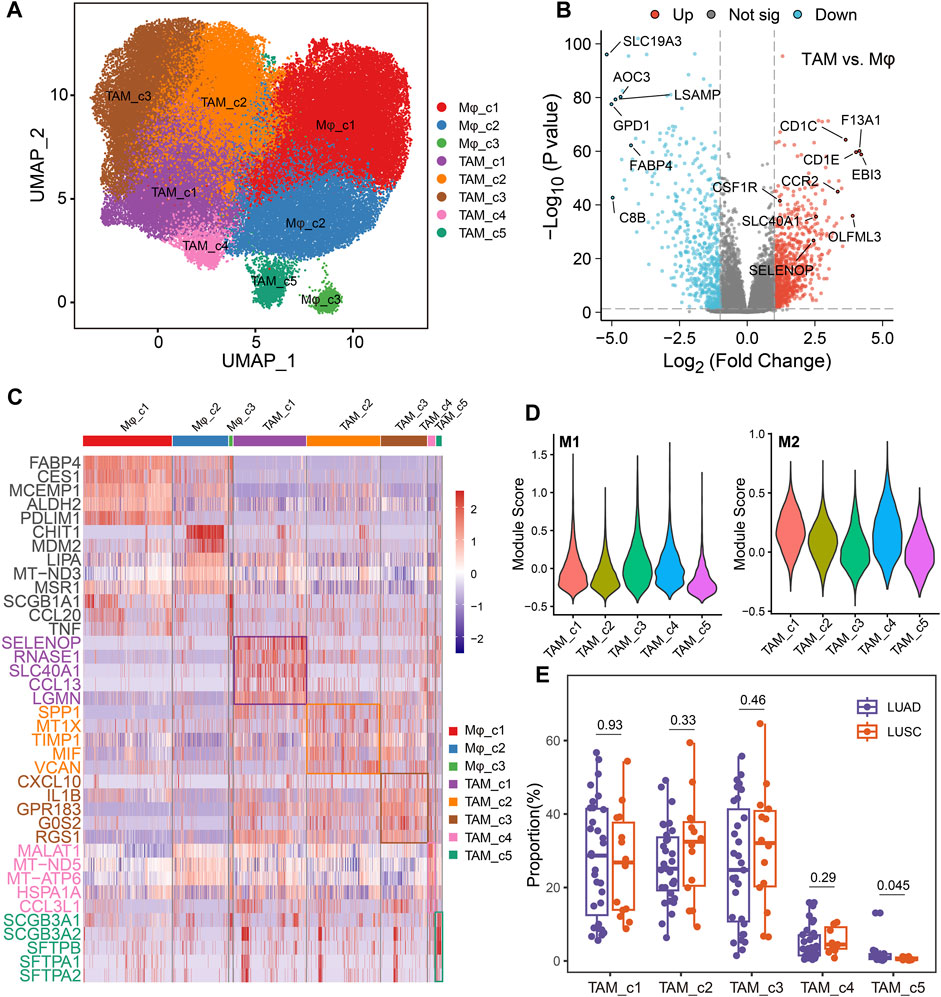
FIGURE 4. Dynamic restructuring of macrophages in NSCLC. (A) UMAP plots showing the macrophages in NSCLC, classified into tumor-associated macrophages (TAMs) and normal-adjacent associated macrophages (Mφ). (B) Volcano plot of differentially expressed genes between TAMs and Mφs. (C) Heatmap showing the differentially expressed genes (rows) across different macrophage clusters (columns). (D) Module scores of gene signatures related to M1/M2 polarization across different TAM clusters. (E) Boxplot showing cellular fractions of TAM clusters in 31 LUAD and 14 LUSC patients. One-sided unpaired Wilcoxon test was used.
Cellular crosstalk in the TME
To explore the contribution of TAM subsets in NSCLC, we analyzed the cellular interactions between TAMs with cancer cells and CD8+ T cells, based on the differentially expressed TAM ligands [False Discovery Rate (FDR) < 0.01, absolute log2FC > 1]. As shown in Figure 5A, we discovered VEGFA signaling toward cancer cells in all TAM subsets, highlighting its significant proangiogenic significance. Enhancement of the SPP1 signaling was observed in the TAM_c1, c2 and c4 subsets and suppressed T cell activation, which partially explained their high M2 scores. Expression of CXCL12 is highest in TAM_c1 subset, supporting revascularization of ischemic tissue and tumor growth (Teicher and Fricker, 2010). We further used the NicheNet algorithm to identify intercellular communication of tumor cells that might influence the transcriptional phenotype of TAMs. Interestingly, we found PTHLH derived from tumor cells can target macrophages and regulate the expression of CCL13, PLAU and ICAM1, resulting the phenotype of TAM_c1. Ligand APP from tumor cells and fibroblasts might increase the expression of SELENOP in macrophages (Figure 5B).
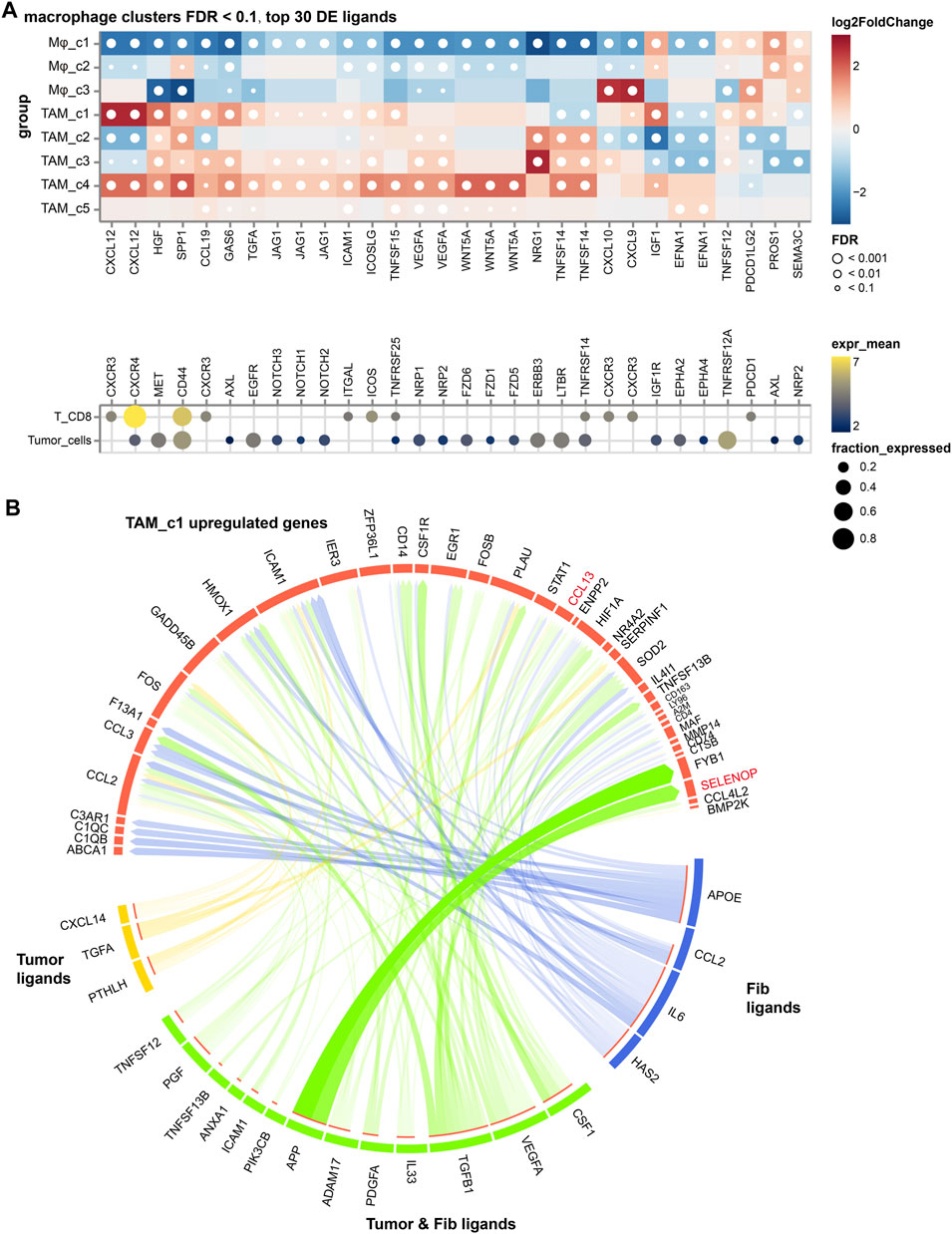
FIGURE 5. The cellular crosstalk of macrophages in NSCLC. (A) Outgoing interactions of different macrophage subclusters with cancer cells and CD8+ T cells. (B) Predicted ligands in tumor cells and fibroblasts that regulate respective target genes in TAM_c1 cluster.
While five tumor-associated macrophage (TAM) subtypes are present in both LUAD and LUSC, macrophages are notably more abundant in adenocarcinoma. Furthermore, we used in vitro transwell co-culture experiment to verify the certain cytokine secreted by tumors could induce THP-1 monocyte differentiated into the state in the LUAD TME (Figure 6A). When THP-1 monocytes co-cultured with SPC-A1 cells, about 33.7% THP-1 could be successfully polarized into M2 macrophages (THP1-M2, Figure 6B). Importantly, the expression of SELENOP, SLC40A1, CCL13 and SPP1 in the macrophages were significantly upregulated in THP1-M2, while FABP4, CES1 and MCEMP1 displayed slight variation during the induction (Figure 6C).
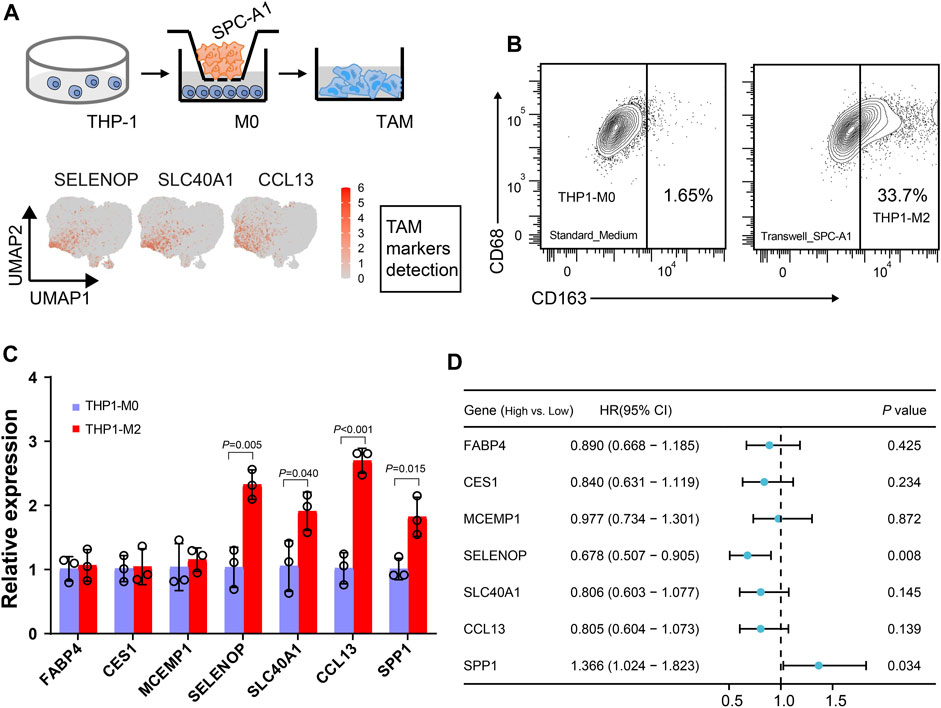
FIGURE 6. Dissection of the interaction between macrophages and tumor cells. (A) Workflow depictingTHP-1 monocyte-derived macrophages that were co-cultured with SPC-A1. (B) Flow cytometry analysis of M2-like macrophages. (C) Boxplot showing the mean expression of the marker genes of Mφ_c1 and TAM_c1 clusters for M2-like macrophages. (D) Univariate Cox regression analysis of macrophage markers expression and TAM_c1 abundance associated with the survival in LUAD patients. LUAD patients were stratified into high and low groups based on the median TPM (transcripts per million) values of the analyzed genes, as well as the median TAM_c1 abundance.
Using clinical data collected from TCGA, we next evaluated the impact of these TAM marker genes on prognosis. Forest plot from univariate regression analysis demonstrated that LUAD patients with high levels of SPP1 were related to poor prognosis (Figure 6D, Supplementary Figure S3A), whereas high SELENOP expression indicated favor prognosis (Figure 6D, Supplementary Figure S3B). Focusing on the clinical relevance of TAM_c1 in LUAD, we utilized CIBERSORTx to estimate the proportion in TCGA dataset based on the cell-type markers in scRNA-seq. Estimates of the TAM_c1 showed high intra-group correlation within other TAM groups (TAM_c2-4, Supplementary Figure S4A), and CCL13 was included in the signature matrix for TAM_c1 deconvolution (Supplementary Figure S4B). Importantly, TAM_c1 abundance seemed to be associated with poorer overall survival rates (HR = 1.34, 95% CI = 0.98-1.83), but the difference was not statistically significant (p = 0.068, Figure 6D, Supplementary Figure S3C).
Discussion
Some clinical studies have demonstrated that decreasing the number of TAMs might be an effective tumor treatment. Hu et al. reported that tissue-resident macrophages were expanded after neoadjuvant immunotherapy in NSCLC, and M2-TAMs were more likely remodeled into a neutral (M0) instead of an anti-tumor phenotype (M1) (Hu et al., 2023). Francisco-Cruz et al. found that a higher level of infiltration of PD-L1+ macrophages (CD68+PD-L1+) that were in closer proximity to malignant cells in the NSCLC was associated with poor overall survival (Francisco-Cruz et al., 2023). Backman et al. investigated the spatial immunophenotyping of TME for NSCLC using multiplex immunofluorescence staining. They found that the high densities of M1 and CD163 macrophages exhibited a positive prognostic influence, whereas short M2–M1 distances were prognostically unfavourable (Backman et al., 2023). Therefore, identification of targets that can prevent M2-like TAM transition is a crucial task for future research.
In our study, Mφ_c1 cells are tissue-resident alveolar macrophages with the expression of the canonical markers (FABP4 and MCEMP1). FABP4 is a member of the fatty acid-binding protein family and plays important functions in inflammation and metabolism (Xu et al., 2015). We also found that this cluster showed activity related to fatty acid metabolism, which is an accessory characteristic of alveolar macrophages. Moreover, macro_FABP4 alveolar macrophages were reported to be significantly elevated in post-treatment NSCLC patients who received neoadjuvant PD-1 blockade combined with chemotherapy (Hu et al., 2023). This phenomenon indicated that alveolar macrophages might work together with AT2 cells, being involved in alveolar epithelial regeneration. TAM_c1 showed high expression of SELENOP, which was previously reported to have an anti-inflammation role (Barrett et al., 2015). This cluster also highly expressed LGMN and HLA-DP, DQ, DR, indicating their intimate correlation with antigen processing and presentation. The JAK-STAT signaling shows dynamic activity in TAM_c1 and c3 clusters that is essential for a wide range of cytokines such as CCL13, CXCL10 and IL1B, leading to critical cellular events, such as hematopoiesis. Besides, the TAM_c2 cluster highly expressed SPP1 and demonstrated maximum activity in glycolysis, which produces adenosine triphosphate (ATP) and carbon intermediates to facilitate TAM reprogramming (Zhang Q. et al., 2021). Enhanced glycolysis in TAMs supported several metabolic pathways and regulated cell signaling to promote tumor development (Zhang X. et al., 2023). Matsubara et al. found that SPP1 was highly expressed in immunologically “hot” areas such as CD163-positive TAMs, which predicted a poor prognosis in LUAD, but this association did not hold for LUSC. Additionally, they found that macrophage-derived SPP1 suppresses the apoptosis of cancer cells when exposed to anticancer drugs (PTX or PEM) (Matsubara et al., 2022).
We have also demonstrated molecular interactions between the tumor cells and immune compartments. Several significant interactions (such as SPP1-CD44, CXCL12-CXCR4 and PDCD1LG2-PDCD1) were inferred among TAMs, tumor cells and CD8+ T cells, which involved the activation of ERK, TGF-β and NF-κB signaling pathways in tumor cells and the negative regulation of activated T cell proliferation. Besides, we quantified fully polarized THP-1-derived M2-type macrophages using flow cytometry based on CD68 and CD163, and validated the expression of SELENOP, SLC40A1, CCL13 and SPP1. It is particularly noteworthy that cell-based cancer immunotherapies hinge on the capacity of natural or engineered receptors present on immune cells to interact with specific antigens on cancer cells, resulting in the induction of tumor cell destruction. The combination of CXCR4 therapeutic agent blockade and PDCD1 resulted in the reduction of suppressive leukocytes and promoted the transition of M2-to-M1 macrophage polarization within the tumor (Pei et al., 2023). Martinez-Usatorre et al. reported that both TAMs derived from monocytes, reliant on CSF1R, and alveolar-origin TAMs, sensitive to cisplatin, played a pivotal role in shaping TME enriched in TGF-β, which facilitated the presence of PD-1+ Tregs. The concurrent targeting of TAMs through a combination of a CSF1R inhibitor and cisplatin diminished Tregs, redirected the function of PD-1 antibodies toward CD8+ T cells, and bolstered the efficacy of antiangiogenic immunotherapy, resulting in significant tumor regression (Martinez-Usatorre et al., 2021). Hence, exploring cellular dynamic crosstalk can serve as a novel strategy to indirectly disrupt the interplay of cancer cells, which contributing to the development of efficient and safe therapeutic strategies for combating cancer.
By integrating bulk and single-cell RNA sequencing, high levels of TAM_c1 subset seemed to be correlated with poor survival for lung cancer patients. Deconvolution typically relies on a set of known marker genes, that are expressed at different levels in different cell types (Erdmann-Pham et al., 2021). While certain marker genes demonstrated discernible prognostic potential, the process of deconvoluting bulk transcriptome data to estimate the relative proportions of various cell types remained unaffected. This disparity may lead to incongruities in prognostic implications between marker genes and TAM subgroups. Besides, we have observed a certain degree of correlation among different TAM subtypes based on single-cell technology, which could introduce some error when inferring the expression of individual TAM subgroups. Therefore, a more comprehensive understanding of the regulatory mechanisms governing different macrophage subsets is imperative for future research.
Conclusion
In summary, our study generates an intricate high-resolution portrait of TME in NSCLC with 18 major cell types. Notably, we elucidate distinct cell-type composition patterns within LUAD and LUSC, providing more precise functional transcriptomic classification of macrophages in both histotypes. The biology of TAMs presented in this study could provide the theoretical basis for developing immune-checkpoint therapies for patients with NSCLC.
Data availability statement
The original contributions presented in the study are included in the article/Supplementary Material, further inquiries can be directed to the corresponding author.
Author contributions
LW: Conceptualization, Data curation, Methodology, Writing–original draft. WX: Data curation, Investigation, Methodology, Writing–review and editing. YH: Data curation, Formal Analysis, Writing–review and editing. KF: Data curation, Investigation, Writing–review and editing. YL: Data curation, Formal Analysis, Writing–review and editing. MW: Formal Analysis, Writing–review and editing. YJ: Investigation, Methodology, Writing–review and editing. WZ: Conceptualization, Data curation, Methodology, Resources, Writing–review and editing. SP: Writing–review and editing.
Funding
The author(s) declare financial support was received for the research, authorship, and/or publication of this article. This research was funded by the National Natural Science Foundation of China (Grant No. 82272401).
Conflict of interest
The authors declare that the research was conducted in the absence of any commercial or financial relationships that could be construed as a potential conflict of interest.
Publisher’s note
All claims expressed in this article are solely those of the authors and do not necessarily represent those of their affiliated organizations, or those of the publisher, the editors and the reviewers. Any product that may be evaluated in this article, or claim that may be made by its manufacturer, is not guaranteed or endorsed by the publisher.
Supplementary material
The Supplementary Material for this article can be found online at: https://www.frontiersin.org/articles/10.3389/fphar.2023.1295442/full#supplementary-material
SUPPLEMENTARY TABLE S1 | Table showing the list of primer sequences for RT-qPCR.
References
Azizi, E., Carr, A. J., Plitas, G., Cornish, A. E., Konopacki, C., Prabhakaran, S., et al. (2018). Single-cell map of diverse immune phenotypes in the breast tumor microenvironment. Cell 174 (5), 1293–1308. doi:10.1016/j.cell.2018.05.060
Backman, M., Strell, C., Lindberg, A., Mattsson, J. S. M., Elfving, H., Brunnström, H., et al. (2023). Spatial immunophenotyping of the tumour microenvironment in non-small cell lung cancer. Eur. J. Cancer 185, 40–52. doi:10.1016/j.ejca.2023.02.012
Barrett, C. W., Reddy, V. K., Short, S. P., Motley, A. K., Lintel, M. K., Bradley, A. M., et al. (2015). Selenoprotein P influences colitis-induced tumorigenesis by mediating stemness and oxidative damage. J. Clin. Invest. 125 (7), 2646–2660. doi:10.1172/jci76099
Becht, E., McInnes, L., Healy, J., Dutertre, C. A., Kwok, I. W. H., Ng, L. G., et al. (2018). Dimensionality reduction for visualizing single-cell data using UMAP. Nat. Biotechnol. 37, 38–44. doi:10.1038/nbt.4314
Bischoff, P., Trinks, A., Obermayer, B., Pett, J. P., Wiederspahn, J., Uhlitz, F., et al. (2021). Single-cell RNA sequencing reveals distinct tumor microenvironmental patterns in lung adenocarcinoma. Oncogene 40 (50), 6748–6758. doi:10.1038/s41388-021-02054-3
Browaeys, R., Saelens, W., and Saeys, Y. (2020). NicheNet: modeling intercellular communication by linking ligands to target genes. Nat. Methods 17 (2), 159–162. doi:10.1038/s41592-019-0667-5
Cai, Y., Winn, M. E., Zehmer, J. K., Gillette, W. K., Lubkowski, J. T., Pilon, A. L., et al. (2014). Preclinical evaluation of human secretoglobin 3A2 in mouse models of lung development and fibrosis. Am. J. Physiol. Lung Cell Mol. Physiol. 306 (1), L10–L22. doi:10.1152/ajplung.00037.2013
Efremova, M., Vento-Tormo, M., Teichmann, S. A., and Vento-Tormo, R. (2020). CellPhoneDB: inferring cell-cell communication from combined expression of multi-subunit ligand-receptor complexes. Nat. Protoc. 15 (4), 1484–1506. doi:10.1038/s41596-020-0292-x
Erdmann-Pham, D. D., Fischer, J., Hong, J., and Song, Y. S. (2021). Likelihood-based deconvolution of bulk gene expression data using single-cell references. Genome Res. 31 (10), 1794–1806. doi:10.1101/gr.272344.120
Francisco-Cruz, A., Rocha, P., Reuben, A., Krishnan, S. N., Das, P., Chen, R., et al. (2023). Analysis of immune intratumor heterogeneity highlights immunoregulatory and coinhibitory lymphocytes as hallmarks of recurrence in stage I non-small cell lung cancer. Mod. Pathol. 36 (1), 100028. doi:10.1016/j.modpat.2022.100028
Garrido-Martin, E. M., Mellows, T. W. P., Clarke, J., Ganesan, A. P., Wood, O., Cazaly, A., et al. (2020). M1(hot) tumor-associated macrophages boost tissue-resident memory T cells infiltration and survival in human lung cancer. J. Immunother. Cancer 8 (2), e000778. doi:10.1136/jitc-2020-000778
Hey, J., Paulsen, M., Toth, R., Weichenhan, D., Butz, S., Schatterny, J., et al. (2021). Epigenetic reprogramming of airway macrophages promotes polarization and inflammation in muco-obstructive lung disease. Nat. Commun. 12 (1), 6520. doi:10.1038/s41467-021-26777-9
Hu, J., Zhang, L., Xia, H., Yan, Y., Zhu, X., Sun, F., et al. (2023). Tumor microenvironment remodeling after neoadjuvant immunotherapy in non-small cell lung cancer revealed by single-cell RNA sequencing. Genome Med. 15 (1), 14. doi:10.1186/s13073-023-01164-9
Ishihara, R., Oda, T., Murakami, Y., Matsumura, I., Watanabe, S., Asao, Y., et al. (2023). Myeloma microenvironmental TIMP1 induces the invasive phenotype in fibroblasts to modulate disease progression. Int. J. Mol. Sci. 24 (3), 2216. doi:10.3390/ijms24032216
Kim, N., Kim, H. K., Lee, K., Hong, Y., Cho, J. H., Choi, J. W., et al. (2020). Single-cell RNA sequencing demonstrates the molecular and cellular reprogramming of metastatic lung adenocarcinoma. Nat. Commun. 11 (1), 2285. doi:10.1038/s41467-020-16164-1
Lambrechts, D., Wauters, E., Boeckx, B., Aibar, S., Nittner, D., Burton, O., et al. (2018). Phenotype molding of stromal cells in the lung tumor microenvironment. Nat. Med. 24 (8), 1277–1289. doi:10.1038/s41591-018-0096-5
Liang, J. L., Jin, X. K., Luo, G. F., Zhang, S. M., Huang, Q. X., Lin, Y. T., et al. (2023). Immunostimulant hydrogel-guided tumor microenvironment reprogramming to efficiently potentiate macrophage-mediated cellular phagocytosis for systemic cancer immunotherapy. ACS Nano 17 (17), 17217–17232. doi:10.1021/acsnano.3c05093
Lin, C. Y., Huang, K. Y., Kao, S. H., Lin, M. S., Lin, C. C., Yang, S. C., et al. (2023). Small-molecule PIK-93 modulates the tumor microenvironment to improve immune checkpoint blockade response. Sci. Adv. 9 (14), eade9944. doi:10.1126/sciadv.ade9944
Lopez, R., Regier, J., Cole, M. B., Jordan, M. I., and Yosef, N. (2018). Deep generative modeling for single-cell transcriptomics. Nat. Methods 15 (12), 1053–1058. doi:10.1038/s41592-018-0229-2
Ma, R. Y., Black, A., and Qian, B. Z. (2022). Macrophage diversity in cancer revisited in the era of single-cell omics. Trends Immunol. 43 (7), 546–563. doi:10.1016/j.it.2022.04.008
Martinez-Usatorre, A., Kadioglu, E., Boivin, G., Cianciaruso, C., Guichard, A., Torchia, B., et al. (2021). Overcoming microenvironmental resistance to PD-1 blockade in genetically engineered lung cancer models. Sci. Transl. Med. 13 (606), eabd1616. doi:10.1126/scitranslmed.abd1616
Matsubara, E., Komohara, Y., Esumi, S., Shinchi, Y., Ishizuka, S., Mito, R., et al. (2022). SPP1 derived from macrophages is associated with a worse clinical course and chemo-resistance in lung adenocarcinoma. Cancers (Basel) 14 (18), 4374. doi:10.3390/cancers14184374
Newman, A. M., Steen, C. B., Liu, C. L., Gentles, A. J., Chaudhuri, A. A., Scherer, F., et al. (2019). Determining cell type abundance and expression from bulk tissues with digital cytometry. Nat. Biotechnol. 37 (7), 773–782. doi:10.1038/s41587-019-0114-2
Pai, J. A., Hellmann, M. D., Sauter, J. L., Mattar, M., Rizvi, H., Woo, H. J., et al. (2023). Lineage tracing reveals clonal progenitors and long-term persistence of tumor-specific T cells during immune checkpoint blockade. Cancer Cell 41, 776–790.e7. doi:10.1016/j.ccell.2023.03.009
Pei, W., Wei, K., Wu, Y., Qiu, Q., Zhu, H., Mao, L., et al. (2023). Colorectal cancer tumor cell-derived exosomal miR-203a-3p promotes CRC metastasis by targeting PTEN-induced macrophage polarization. Gene 885, 147692. doi:10.1016/j.gene.2023.147692
Ruella, M., Klichinsky, M., Kenderian, S. S., Shestova, O., Ziober, A., Kraft, D. O., et al. (2017). Overcoming the immunosuppressive tumor microenvironment of Hodgkin lymphoma using chimeric antigen receptor T cells. Cancer Discov. 7 (10), 1154–1167. doi:10.1158/2159-8290.Cd-16-0850
Salcher, S., Sturm, G., Horvath, L., Untergasser, G., Kuempers, C., Fotakis, G., et al. (2022). High-resolution single-cell atlas reveals diversity and plasticity of tissue-resident neutrophils in non-small cell lung cancer. Cancer Cell 40 (12), 1503–1520.e8. doi:10.1016/j.ccell.2022.10.008
Sánchez-Paulete, A. R., Mateus-Tique, J., Mollaoglu, G., Nielsen, S. R., Marks, A., Lakshmi, A., et al. (2022). Targeting macrophages with CAR T cells delays solid tumor progression and enhances antitumor immunity. Cancer Immunol. Res. 10 (11), 1354–1369. doi:10.1158/2326-6066.Cir-21-1075
Sathe, A., Grimes, S. M., Lau, B. T., Chen, J., Suarez, C., Huang, R. J., et al. (2020). Single-cell genomic characterization reveals the cellular reprogramming of the gastric tumor microenvironment. Clin. Cancer Res. 26 (11), 2640–2653. doi:10.1158/1078-0432.Ccr-19-3231
Solinas, G., Schiarea, S., Liguori, M., Fabbri, M., Pesce, S., Zammataro, L., et al. (2010). Tumor-conditioned macrophages secrete migration-stimulating factor: a new marker for M2-polarization, influencing tumor cell motility. J. Immunol. 185 (1), 642–652. doi:10.4049/jimmunol.1000413
Sung, H., Ferlay, J., Siegel, R. L., Laversanne, M., Soerjomataram, I., Jemal, A., et al. (2021). Global cancer statistics 2020: GLOBOCAN estimates of incidence and mortality worldwide for 36 cancers in 185 countries. CA Cancer J. Clin. 71 (3), 209–249. doi:10.3322/caac.21660
Teicher, B. A., and Fricker, S. P. (2010). CXCL12 (SDF-1)/CXCR4 pathway in cancer. Clin. Cancer Res. 16 (11), 2927–2931. doi:10.1158/1078-0432.Ccr-09-2329
Wang, C., Yu, Q., Song, T., Wang, Z., Song, L., Yang, Y., et al. (2022). The heterogeneous immune landscape between lung adenocarcinoma and squamous carcinoma revealed by single-cell RNA sequencing. Signal Transduct. Target Ther. 7 (1), 289. doi:10.1038/s41392-022-01130-8
Wolock, S. L., Lopez, R., and Klein, A. M. (2019). Scrublet: computational identification of cell doublets in single-cell transcriptomic data. Cell Syst. 8 (4), 281–291. doi:10.1016/j.cels.2018.11.005
Xu, H., Hertzel, A. V., Steen, K. A., Wang, Q., Suttles, J., and Bernlohr, D. A. (2015). Uncoupling lipid metabolism from inflammation through fatty acid binding protein-dependent expression of UCP2. Mol. Cell Biol. 35 (6), 1055–1065. doi:10.1128/mcb.01122-14
Yoshida, C., Kadota, K., Ikeda, T., Ibuki, E., Go, T., Haba, R., et al. (2021). Tumor-associated macrophage infiltration is associated with a higher rate of tumor spread through air spaces in resected lung adenocarcinomas. Lung Cancer 158, 91–96. doi:10.1016/j.lungcan.2021.06.009
Zhang, H., Wang, S. Q., Hang, L., Zhang, C. F., Wang, L., Duan, C. J., et al. (2021a). GRP78 facilitates M2 macrophage polarization and tumour progression. Cell Mol. Life Sci. 78 (23), 7709–7732. doi:10.1007/s00018-021-03997-2
Zhang, L., Zhang, Y., Wang, C., Yang, Y., Ni, Y., Wang, Z., et al. (2022). Integrated single-cell RNA sequencing analysis reveals distinct cellular and transcriptional modules associated with survival in lung cancer. Signal Transduct. Target Ther. 7 (1), 9. doi:10.1038/s41392-021-00824-9
Zhang, Q., Wang, J., Yadav, D. K., Bai, X., and Liang, T. (2021b). Glucose metabolism: the metabolic signature of tumor associated macrophage. Front. Immunol. 12, 702580. doi:10.3389/fimmu.2021.702580
Zhang, X., Ji, L., and Li, M. O. (2023a). Control of tumor-associated macrophage responses by nutrient acquisition and metabolism. Immunity 56 (1), 14–31. doi:10.1016/j.immuni.2022.12.003
Zhang, Y., Du, W., Chen, Z., and Xiang, C. (2017). Upregulation of PD-L1 by SPP1 mediates macrophage polarization and facilitates immune escape in lung adenocarcinoma. Exp. Cell Res. 359 (2), 449–457. doi:10.1016/j.yexcr.2017.08.028
Zhang, Y., Tian, Y., Zheng, L., Sun, X., Zhao, Z., Zheng, Y., et al. (2023b). Efficacy and safety of consolidation durvalumab after chemoradiation therapy for stage III non-small-cell lung cancer: a systematic review, meta-analysis, and meta-regression of real-world studies. Front. Pharmacol. 14, 1103927. doi:10.3389/fphar.2023.1103927
Glossary
Keywords: NSCLC, scRNA-seq, tumor-associated macrophages, cellular crosstalk, therapeutic implications
Citation: Wu L, Xia W, Hua Y, Fan K, Lu Y, Wang M, Jin Y, Zhang W and Pan S (2023) Cellular crosstalk of macrophages and therapeutic implications in non-small cell lung cancer revealed by integrative inference of single-cell transcriptomics. Front. Pharmacol. 14:1295442. doi: 10.3389/fphar.2023.1295442
Received: 16 September 2023; Accepted: 02 November 2023;
Published: 15 November 2023.
Edited by:
Mou Peng, Central South University, ChinaReviewed by:
Qun Liu, China Pharmaceutical University, ChinaKeming Yang, University of Pittsburgh Medical Center, United States
Copyright © 2023 Wu, Xia, Hua, Fan, Lu, Wang, Jin, Zhang and Pan. This is an open-access article distributed under the terms of the Creative Commons Attribution License (CC BY). The use, distribution or reproduction in other forums is permitted, provided the original author(s) and the copyright owner(s) are credited and that the original publication in this journal is cited, in accordance with accepted academic practice. No use, distribution or reproduction is permitted which does not comply with these terms.
*Correspondence: Shiyang Pan, c3lwYW5AbmptdS5lZHUuY24=
†These authors have contributed equally to this work