- 1Queen Mary College, Medical School of Nanchang University, Nanchang, China
- 2Department of Neurosurgery, First Affiliated Hospital of Anhui Medical University, Hefei, China
- 3School of Stomatology, Southwest Medical University, Luzhou, China
- 4Department of Breast Surgery, The First Affiliated Hospital of Nanjing Medical University, Nanjing, China
- 5First Teaching Hospital of Tianjin University of Traditional Chinese Medicine, Tianjin, China
- 6Heilongjiang University of Chinese Medicine, Harbin, China
- 7Clinical Medical College, Southwest Medical University, Luzhou, China
- 8Department of Specialty Medicine, Ohio University, Athens, OH, United States
Background: Uveal melanoma (UVM) is a primary intraocular malignancy that poses a significant threat to patients’ visual function and life. The basement membrane (BM) is critical for establishing and maintaining cell polarity, adult function, embryonic and organ morphogenesis, and many other biological processes. Some basement membrane protein genes have been proven to be prognostic biomarkers for various cancers. This research aimed to develop a novel risk assessment system based on BMRGs that would serve as a theoretical foundation for tailored and accurate treatment.
Methods: We used gene expression profiles and clinical data from the TCGA-UVM cohort of 80 UVM patients as a training set. 56 UVM patients from the combined cohort of GSE84976 and GSE22138 were employed as an external validation dataset. Prognostic characteristics of basement membrane protein-related genes (BMRGs) were characterized by Lasso, stepwise multifactorial Cox. Multivariate analysis revealed BMRGs to be independent predictors of UVM. The TISCH database probes the crosstalk of BMEGs in the tumor microenvironment at the single-cell level. Finally, we investigated the function of ITGA5 in UVM using multiple experimental techniques, including CCK8, transwell, wound healing assay, and colony formation assay.
Results: There are three genes in the prognostic risk model (ADAMTS10, ADAMTS14, and ITGA5). After validation, we determined that the model is quite reliable and accurately forecasts the prognosis of UVM patients. Immunotherapy is more likely to be beneficial for UVM patients in the high-risk group, whereas the survival advantage may be greater for UVM patients in the low-risk group. Knockdown of ITGA5 expression was shown to inhibit the proliferation, migration, and invasive ability of UVM cells in vitro experiments.
Conclusion: The 3-BMRGs feature model we constructed has excellent predictive performance which plays a key role in the prognosis, informing the individualized treatment of UVM patients. It also provides a new perspective for assessing pre-immune efficacy.
1 Introduction
Uveal melanoma (UVM) is a rare yet aggressive primary intraocular malignancy arising from ocular melanocytes, constituting a small fraction of all melanomas (Singh et al., 2005; Singh et al., 2011; Chattopadhyay et al., 2016; Chi et al., 2022). It imposes significant threats to patients’ visual function and overall survival, with a high mortality rate of up to 50% attributed to its severe clinical presentation, malignancy, and limited treatment options (Andreoli et al., 2015). Notably, UVM exhibits a propensity for metastasis, with approximately half of the patients developing distant organ metastases, most commonly involving the liver, lung, and bone (Rusňák et al., 2020). Unfortunately, current therapeutic modalities for UVM have shown limited efficacy in managing metastatic disease (Augsburger et al., 2009; Damato, 2018). As a result, recent research endeavors have focused on the development of targeted therapeutics and immunotherapeutic strategies, including immune checkpoint inhibitors, vaccines, and adoptive cell therapy, to address the unmet medical needs in UVM (Curran et al., 2010; Larkin et al., 2015; Bol et al., 2016). However, the underlying etiology and molecular mechanisms driving UVM remain largely elusive (Smit et al., 2020; Derrien et al., 2021; Katopodis et al., 2021). Consequently, there is a critical need to identify novel prognostic biomarkers and molecular targets that can accurately predict patient outcomes and facilitate personalized treatment approaches, ultimately improving the quality of life for individuals affected by UVM.
The basement membrane (BM) is a specialized extracellular matrix located at the basal aspect of epithelial tissues, primarily composed of collagen IV, laminin, heparan sulfate proteoglycans, BM-40, and nidogen (Timpl, 1989). Its crucial role in establishing and maintaining cellular polarity and providing mechanical support to tissues is well-recognized (Banerjee et al., 2022). Moreover, BMs play critical roles in various physiological processes, including embryonic development, organ morphogenesis, and adult tissue homeostasis (Li et al., 2003). Perturbations in BM protein expression and turnover have been implicated in tumorigenesis, and dysregulation of BM integrity has been associated with tumor metastasis (Valastyan and Weinberg, 2011; Naba et al., 2014). While BM-related genes have shown prognostic significance in several cancers, their role, and prognostic implications in uveal melanoma (UVM) remain poorly understood. To elucidate the immunological status of UVM patients and accurately predict prognosis, this study aimed to develop a novel risk-scoring system based on BM-related genes. The objective was to establish a theoretical foundation for personalized therapeutic interventions tailored to individual patients. By comprehensively characterizing the expression and functional relevance of BMRGs, this risk-scoring system would enable precise prognostic stratification and facilitate tailored treatment strategies in UVM.
Following the rapid advancement of bioinformatics (Song et al., 2022a; Zhao et al., 2022a; Jin et al., 2022), a considerable amount of research has been conducted to establish models for predicting the prognosis of UVM through machine learning. For example, Zheng et al. established an autophagy-related gene (ARG) risk model and validated it with TCGA and four external independent UVM cohorts, revealing that UVM patients with higher risk scores exhibited higher levels of immune cell infiltration and enrichment of tumor markers (Zheng et al., 2021); Lv et al. constructed a UVM prognostic model based on the Epithelial-mesenchymal transition (EMT) signature, which found that patients with high EMT scores potentially had higher response rates to immunotherapy (Lv et al., 2022); Yang et al. utilized immune markers systematically to develop a prognostic six-immune-gene signature via RNA sequencing data from TCGA and GEO databases for predicting the overall survival outcome of UVM patients (Yang et al., 2023). Meanwhile, several studies have reported that BMRG signatures could predict the prognosis of tumor survivors and provide a potential target for immunotherapy (Cai et al., 2022; Shen et al., 2023). However, BMRG-related models have not yet been established and validated for prognostic prediction in UVM patients (Song et al., 2022a).
In this study, we developed a prognostic model for UVM using the TCGA-UVM cohort. We carefully selected three reliable basement membrane-related genes (BMRGs) through a rigorous screening process and employed two machine learning techniques to construct the model. By integrating genetic information from UVM patients, we aimed to explore the prognostic value of these three BMRGs and develop novel tools to enhance therapeutic strategies. Our analysis involved assessing the interaction between BMRGs and the immune microenvironment, as well as evaluating the impact of BMRGs on immunotherapy and chemotherapy sensitivity. We eventually verified the functional role of the ITGA5, the gene with the highest absolute HR value, in UVM cells by an in vitro experiment. By leveraging advanced computational methods and integrating multi-dimensional data, we sought to gain insights into the role of BMRGs in determining the prognosis of UVM, identify potential avenues for improving treatment regimens, and offer possibilities for developing personalized therapeutic approaches. These findings have the potential to enhance patient outcomes and pave the way for further advancements in UVM research and clinical practice.
2 Materials and methods
2.1 Patient data sources
We utilized the TCGA-UVM cohort, obtained from the publicly available TCGA database, as our training set, consisting of gene expression profiles and clinical data from 80 tumor patients. To ensure accurate analysis, we performed preprocessing steps on the data. Initially, we converted the level 3 HTSeq-fragments per kilobase (FPKM) data into transcripts per million reads (TPM) to account for gene length and sequencing depth variations across samples. This conversion was done using a formula that normalized the TPM values. Subsequently, we applied a logarithmic transformation to the TPM values to normalize the data and enhance comparability between samples. It is important to note that due to significant variation in sample sizes among UVM patients at stages M and N, these stages were excluded from our analysis to ensure robustness and reliability. Furthermore, we incorporated two external validation datasets, GSE84976 and GSE22138, from the GEO database. It is worth noting that datasets GSE84976 and GSE22138 were merged together to act as a validation set, and in order to mitigate the effects of batch differences between the microarray expression data, we utilized the ComBat function in the R package “sva” to achieve correction for batch effects. For comprehensive details on these datasets, see Supplementary Material. These datasets included genetic profiles and clinical data from 56 UVM patients, and their inclusion aimed to enhance the validity and generalizability of our analyses. In the training cohort, we transcribed and analyzed tissue samples from eye cancer patients for comparative analyses to obtain genes that were aberrantly expressed in eye cancer patients. While in the external validation set, we only included samples from eye cancer patients analyzed. In addition, we required complete patient follow-up and clinical information in the cohort and complete micro-matrix data in the cohort to ensure data quality for subsequent bioinformatics analysis. By employing these rigorous preprocessing steps and integrating multiple datasets, we aimed to improve the accuracy and reliability of our findings, providing valuable insights into the molecular characteristics and clinical implications of UVM.
2.2 Consensus clustering analysis
To gain deeper insights into the mechanistic implications of BMRGs in UVM, we employed advanced analytical methodologies. The “Consensus Cluster Plus” R package (Zhao et al., 2022b; Wang et al., 2022) was leveraged to classify UVM patient samples into distinct clusters based on the expression patterns of BMRGs, thereby unveiling unique gene expression profiles associated with specific subtypes. Differential expression patterns of BMRGs across clusters, along with clinicopathological parameters, were visualized using the “pheatmap” R package (Bhattacherjee et al., 2019; Song et al., 2022b). To elucidate the distinct biological pathways and processes underlying these clusters, we retrieved the “c2. cp.kegg.v7.4. symbols.gmt” file from the MSigDB database (Liberzon et al., 2015) for genomic variation analysis via GSVA. Employing the “GSVA” R package (Hänzelmann et al., 2013), we systematically analyzed pathway differences between clusters, revealing noteworthy disparities in key pathways among diverse UVM subtypes. Furthermore, the Single Sample Genome Enrichment Analysis (ssGSEA) algorithm (Zhuang et al., 2021; Huang et al., 2023) was applied to assess the infiltration levels of immune cells and expression levels of immune checkpoints within the identified clusters. This integrative approach shed light on potential variations in the immune microenvironment across UVM subtypes, offering crucial insights into the prospective efficacy of immune checkpoint-based therapies in specific patient cohorts.
2.3 Model construction and validation
The dataset of basement membrane (BM) genes was obtained from the Basement Membrane BASE database (https://bmbase.manchester.ac.uk), comprising a comprehensive collection of 224 genes associated with the basement membrane protein. To explore the potential prognostic relevance of these genes, univariate Cox regression analysis was performed, resulting in the identification of 81 genes significantly associated with survival outcomes. To further refine the gene set and mitigate the risk of overfitting, we employed the LASSO (Least Absolute Shrinkage and Selection Operator) method, a powerful machine learning approach (Chi et al., 2023a; Chi et al., 2023b). The “glmnet” R package (Engebretsen and Bohlin, 2019; Ren et al., 2023) was utilized to implement LASSO, which involves adding a penalty term to the regression model. This penalty encourages the coefficients of less influential predictors to shrink toward zero, effectively selecting the most informative subset of predictors. By applying LASSO, we successfully narrowed down the candidate genes to eight. Subsequently, a stepwise multi-factor Cox regression model was employed to identify and estimate the coefficients of the core genes from the selected set. Through this iterative procedure, we ultimately derived a risk profile consisting of four BMRGs. For each patient, the risk score was calculated by combining the expression levels of these genes with their corresponding coefficients: Risk score = ExpressionmRNA1 × CoefmRNA1 + ExpressionmRNA2 × CoefmRNA2 + ExpressionmRNAn × CoefmRNAn. By leveraging these analytical approaches, we aimed to establish a robust and concise set of BMRGs with prognostic implications in order to facilitate risk stratification and inform personalized treatment strategies for patients.
2.4 Correlation between clinicopathological features and risk scores
Investigating the relationship between risk scores and relevant clinical features in patients with uveal melanoma (UVM) can provide valuable insights for clinical prognostic assessment. To visualize the associations between clinical features and the modeled genes, we employed the “pheatmap” R package (Lu et al., 2021) to generate heat maps displaying multiple groups of clinical features. To gain a deeper understanding of the differences in risk scores among various patient subgroups, we performed clinical analyses on the entire sample cohort. The patients were stratified based on different clinical characteristics, including age (≤65 and >65 years), sex (male and female), pathological stage (II and III-IV), and T-stage (T2 and T3-4). Between-group differences were assessed using the “ggpubr” package (Whitehead et al., 2019). By examining the relationships between risk scores and clinical subgroups, we aimed to identify potential variations in risk profiles based on different demographic and pathological factors. These analyses would contribute to a more comprehensive understanding of the prognostic implications of risk scores in UVM patients and their clinical relevance.
2.5 Independent prognostic analysis and nomogram construction
To evaluate the independent prognostic value of the risk score in predicting uveal melanoma (UVM) outcomes, we conducted both univariate and multivariate Cox regression analyses. These analyses aimed to assess whether the risk score could serve as a reliable prognostic factor, independent of conventional clinicopathological characteristics. The “rms” R package (Zhang et al., 2022) was employed to construct a nomogram incorporating the risk score and clinicopathological features. This nomogram provided a visual tool for predicting the survival of patients in the TCGA-UVM cohort, enabling clinicians to estimate individual patient prognoses more accurately. To assess the predictive performance of the nomogram, we utilized the “ggDCA” R package (Mao et al., 2021) to develop decision curve analysis (DCA) and calibration curves. The DCA allowed us to evaluate the clinical benefits of using the nomogram compared to other predictive models or strategies. Calibration curves were generated to assess the calibration accuracy of the nomogram in predicting patient survival. These comprehensive analyses aimed to validate the prognostic value of the risk score and provide clinicians with a practical tool for prognostic assessment in UVM patients. By integrating the risk score with clinicopathological characteristics, the nomogram offered improved prognostic accuracy, ultimately enhancing patient management and treatment decision-making.
2.6 Establishing the equations for signatures
After scoring all UVM patients based on the risk model equation, we determined the median risk score using the ‘survminer’ R package. Subsequently, we categorized the patients into a low-risk group and a high-risk group. Survival curves were plotted for both groups to visually compare their survival outcomes. To evaluate the predictive performance of the risk model, we calculated the C-index using the ‘pec’ R package. The C-index provides a measure of concordance between predicted risk scores and actual survival outcomes. To further assess the predictive power of the genetic traits, we conducted an analysis of receiver operating characteristic (ROC) curves using the ‘time-ROC’ R package. ROC curves allow us to evaluate the sensitivity and specificity of the genetic traits in predicting survival outcomes. Additionally, decision curve analysis (DCA) was performed for the multi-factor Cox regression model using the ‘ggDCA’ R package. DCA provides insights into the clinical utility of the predictive model by assessing the net benefits of different strategies or models across a range of threshold probabilities. Through these analyses, we aimed to assess the predictive accuracy and clinical usefulness of the risk model in UVM patients. The survival curves, C-index, ROC curves, and DCA plots provide valuable information for understanding the prognostic value and potential application of genetic traits in UVM patient management and treatment decision-making.
2.7 Enrichment analysis
To analyze the Gene Ontology (GO) pathway, we utilized the “ClusterProfiler” R package (Song et al., 2023; Zhang et al., 2023). In the generated graphs, a p-value of less than 0.05 indicated a statistically significant difference, highlighting the enriched pathways and functional categories associated with the genes of interest. For further enrichment analysis, we conducted GSVA using the “GSVA” R package. The data from “c2. cp.kegg.v7.5.1. symbols.gmt” in the MSigDB database were utilized to explore the functional annotation and enrichment pathways. To visualize the results, heatmaps were generated using the ‘heatmap’ R package. Adjusted p-values of less than 0.05, obtained through the ‘limma’ R package, indicated the statistical significance of subgroup differences in the heatmap. Through functional enrichment analysis, we aimed to gain insights into the biological functions, pathways, and processes associated with differentially expressed genes related to BMRGs in UVM. These analyses contribute to a better understanding of the molecular mechanisms underlying UVM and provide valuable information on functional annotations and enriched pathways associated with BMRGs in the context of UVM.
2.8 Immuno-infiltration analysis
Multiple methods have been developed to quantify immune infiltration scores, including XCELL, TIMER, QUANTISEQ, MCPCOUNT, EPIC, CIBERSORT, and CIBERSORT-ABS. These methods offer diverse approaches for evaluating the presence and abundance of immune cells within the tumor microenvironment. To investigate the association between immune cells and risk scores, Spearman correlation analysis was employed, allowing for a comprehensive understanding of the immune landscape in UVM. Utilizing the immune cell profiles of UVM patients, we applied the ssGSEA method to stratify patients into distinct low- and high-risk groups based on their immune signatures. Furthermore, we examined the differential expression of 20 suppressive immune checkpoints between the identified high-risk and low-risk groups, shedding light on the potential influence of immune checkpoint blockade therapies. To assess and visualize the impact of immunotherapy in UVM patients, we utilized the widely adopted ‘limma’ and ‘ggpubr’ R packages. To expand our understanding of the genetic underpinnings related to cancer and immunity, we referred to the curated collection of genes provided by Xu et al., available on their website (Xu et al., 2018). Employing the R package “ggcor,” we explored the correlation between risk scores and these two genetic traits, unraveling potential associations between genetic alterations and disease prognosis in UVM. Additionally, to predict immune infiltration estimates and immunotherapy response data, we leveraged the computational tool ImmuCellAI (Miao et al., 2020). This powerful resource enables comprehensive analyses of the immune landscape and aids in guiding immunotherapeutic strategies for UVM patients.
2.9 TISCH analysis
The Tumor Immunological Single Cell Centre (TISCH) hosts a comprehensive single-cell RNA sequencing database that focuses on investigating the intricate tumor microenvironment (TME). This valuable resource facilitates detailed annotation of various single-cell types, enabling in-depth analysis of gene expression within distinct cellular populations. By examining gene expression patterns across different cell types, we can unravel the intricate variations within the tumor microenvironment of individual UVM patients, thus shedding light on the underlying heterogeneity of UVM. This comprehensive characterization of the TME aids in elucidating the complex dynamics and functional implications of different cell types within the UVM context.
2.10 Cell culture
The human uveal melanoma cells (MuM-2B, OCM-1) utilized in this investigation were generously provided by the Cell Resource Center at Shanghai Life Sciences Institute. These cells were cultivated under controlled conditions in Dulbecco’s Modified Eagle’s Medium (DMEM) (Gibco, United States), supplemented with 1% penicillin/streptomycin and 10% fetal bovine serum (FBS) (Gibco, United States), within a humidified incubator set at 37°C with a 5% CO2 atmosphere.
2.11 CCK-8 assay
To assess the impact of ITGA5 on the proliferative capacity of uveal melanoma (UVM) cells, the Cell Counting Kit-8 (CCK-8) assay was employed. UVM cells were cultured in 96-well microplates in triplicate, with each well initially seeded with 5,000 cells. Subsequent to transfection, the cells were subjected to treatment at 37°C for a duration of 2 h, utilizing 10 μL of CCK-8 solution (A311-01, vazyme, Nanjing, China) mixed with 90 μL of complete media in each well at specific time points (0, 24, 48, 72, or 96 h). Following the respective incubation periods, the absorbance of each well was quantified at 450 nm using a microplate reader.
2.12 Wound-healing assay
The wound healing assay was employed to evaluate the migratory behavior of MuM-2B and OCM-1 cells, providing valuable insights into their migratory patterns. The transfected cells were cultured in a six-well plate and incubated at 37°C until they reached approximately 80% confluence. To create a standardized wound, a sterile 200 μL pipette tip was carefully used to generate a linear scrape across the cell monolayers. Following this, the medium was replaced with serum-free medium after two washes with phosphate-buffered saline (PBS) to eliminate any cellular debris. The movement of cells into the wound area was monitored at 0 h and 48 h using an inverted microscope (Olympus, Japan), enabling the quantification of the distance traveled by the cells into the wound surface.
2.13 Transwell assay
Cell migration was assessed using the Transwell migration assay, which involved a 24-well Transwell plate equipped with 8 μm-pore membrane filters. Briefly, the bottom chamber of the Transwell plate was supplemented with media containing 10% fetal bovine serum (FBS), while the top chamber was coated with 2 × 10^5 cells suspended in serum-free medium. Following a 48-h incubation period, the cells that had migrated to the bottom chamber were fixed in 4% methanol for 10 min and subsequently stained with 0.1% crystal violet (Solarbio, Beijing, China) for 15 min.
2.14 Statistical analysis
The statistical analysis was conducted using R software version 4.1.3. To compare the overall survival (OS) between the high-risk and low-risk groups, Kaplan-Meier (KM) survival curves and log-rank tests were employed. In addition, Lasso regression analyses were performed to assess the potential relevance of BMRGs. A stepwise multivariate Cox regression analysis was then employed to construct a BMRG signature. The predictive performance of the model was evaluated using a time-dependent ROC curve. The relationship between the risk score and immune cell infiltration was assessed using Spearman correlation analysis. To compare the ratios of tumor immune infiltrating cells (TIIC), immunological checkpoints, and immune function between the two groups, the Wilcox test was applied. Statistical significance was determined by p-values <0.05, and a false discovery rate (FDR) < 0.05 was considered statistically significant. The CCK-8 data analysis was conducted using GraphPad Prism Software version 8.3.0. The mean values ±standard deviation (SD) were determined based on data obtained from three independent experiments. Statistical significance was assessed using analysis of variance (ANOVA), with a significance level set at p < 0.05.
3 Results
3.1 Consensus clustering determined the molecular subtypes of BMRGs
The primary study design is presented in Figure 1, illustrating the overall flow of the investigation. The cumulative distribution function (CDF) values demonstrated an increasing trend in relation to the consensus index, indicating successful classification. To assess cluster composition and quantity, the consensus matrix serves as an excellent visual tool. We generated a color-coded heat map based on the consensus matrix, which revealed higher intra-cluster correlations and lower inter-cluster correlations when considering k = 2. These findings strongly support the acceptance of two subtypes (Cluster A and Cluster B) for categorizing UVM patients. Based on the CDF curves and the Delta area, k = 2 represents the optimal point to achieve maximal inter-cluster differences as the clustering index “k” increases from 2 to 9. Consequently, we divided the UVM patients into two subgroups (Figures 2A–D).
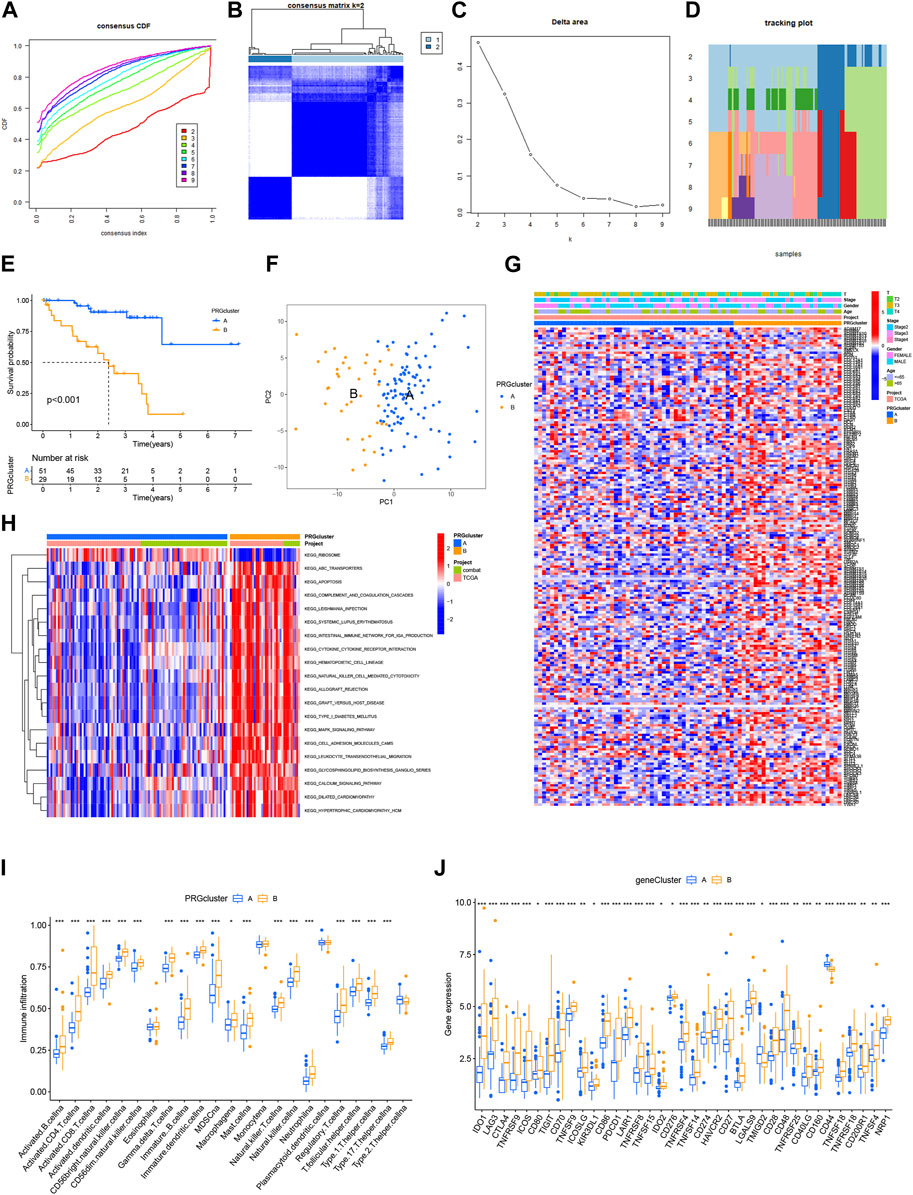
FIGURE 2. Consensus clustering determined the molecular subtypes of BMRGs. (A) Consensus clustering CDF with K = 2 to 9. (B) Consensus matrix heatmap for K = 2 clusters. (C) CDF plot illustrating the consensus clustering results for K = 2 to 9. (D) Tracking plot displaying the sample classification across K = 2 to 9 clusters. (E) Kaplan-Meier survival curves comparing the survival outcomes between Cluster A and Cluster B. (F) Principal component analysis (PCA) plot visualizing the distribution of samples. (G) Correlation analysis depicting the relationship between BMRGs expression and clinicopathological parameters. (H) Enrichment analysis of KEGG pathways in Cluster A and Cluster B. (I) Comparison of immune cell infiltration levels between clusters. (J) Differential expression of immune checkpoints between Cluster A and Cluster B. Statistical significance: *p < 0.05, **p < 0.01, ***p < 0.001, ns > 0.05.
Furthermore, we investigated the differential survival prognosis across clusters using the Cluster Survival R package. The results indicated that patients in cluster A exhibited significantly better survival prognoses than those in cluster B (p < 0.001) (Figure 2E).
Principal component analysis (PCA) was performed to visualize risk distribution among different patient groups. The PCA plot (Figure 2F) demonstrated distinct differences between Cluster A and Cluster B patients. Additionally, we conducted further analysis to explore metabolic variations in BMRGs between clusters A and B. The heat map revealed notable expression differences and clinical traits associated with BMRGs in cluster B (Figure 2G).
To investigate potential biological pathways, we performed an enrichment analysis using the Kyoto Encyclopedia of Genes and Genomes (KEGG) pathway database on the clustered samples. We explored correlations among various cancer-related pathways, such as apoptosis, transporters, and the MAPK signaling pathway (Figure 2H). Moreover, we employed the ssGSEA algorithm to assess the distribution and correlation of 23 tumor-infiltrating immune cells (TIICs) to guide immunotherapy. Notably, cluster B exhibited higher levels of immune cell infiltration compared to cluster A (Figure 2I). Considering the critical role of immune checkpoints in tumor immunotherapy effectiveness and their prominence within the tumor microenvironment (TME), we evaluated immune checkpoint expression between the two patient clusters. The analysis revealed significantly upregulated immune checkpoint expression in Cluster B patients, except for TMIGD2 and CD44. Based on these findings, we conclude that Cluster B demonstrates a more favorable response and effectiveness toward immunotherapy (Figure 2J).
3.2 Development and validation of the BMRGs signature
We developed a risk score model based on BMRGs to identify prognostic biomarkers in UVM patients. Differentially expressed BMRGs with prognostic value were selected using LASSO regression analysis, and the resulting LASSO regression curves and cross-validation plots are shown in Figures 3A,B, respectively. To address batch effects between GSE22138 and GSE84976 datasets, we employed the R package “Combat” for batch effect removal (Figures 3C,D). The prognostic index (PI) was calculated using the formula (−0.974 * ADAMTS10 exp.) + (1.015 * ADAMTS14 exp.) + (0.026 * CSF2 exp.) + (2.973 * ITGA5 exp.), and the risk score for each UVM patient was determined based on the median score using the equation. The optimal number of genes for cross-validation plots was 3, and the selected genes were ADAMTS10, ADAMTS14, and ITGA5.
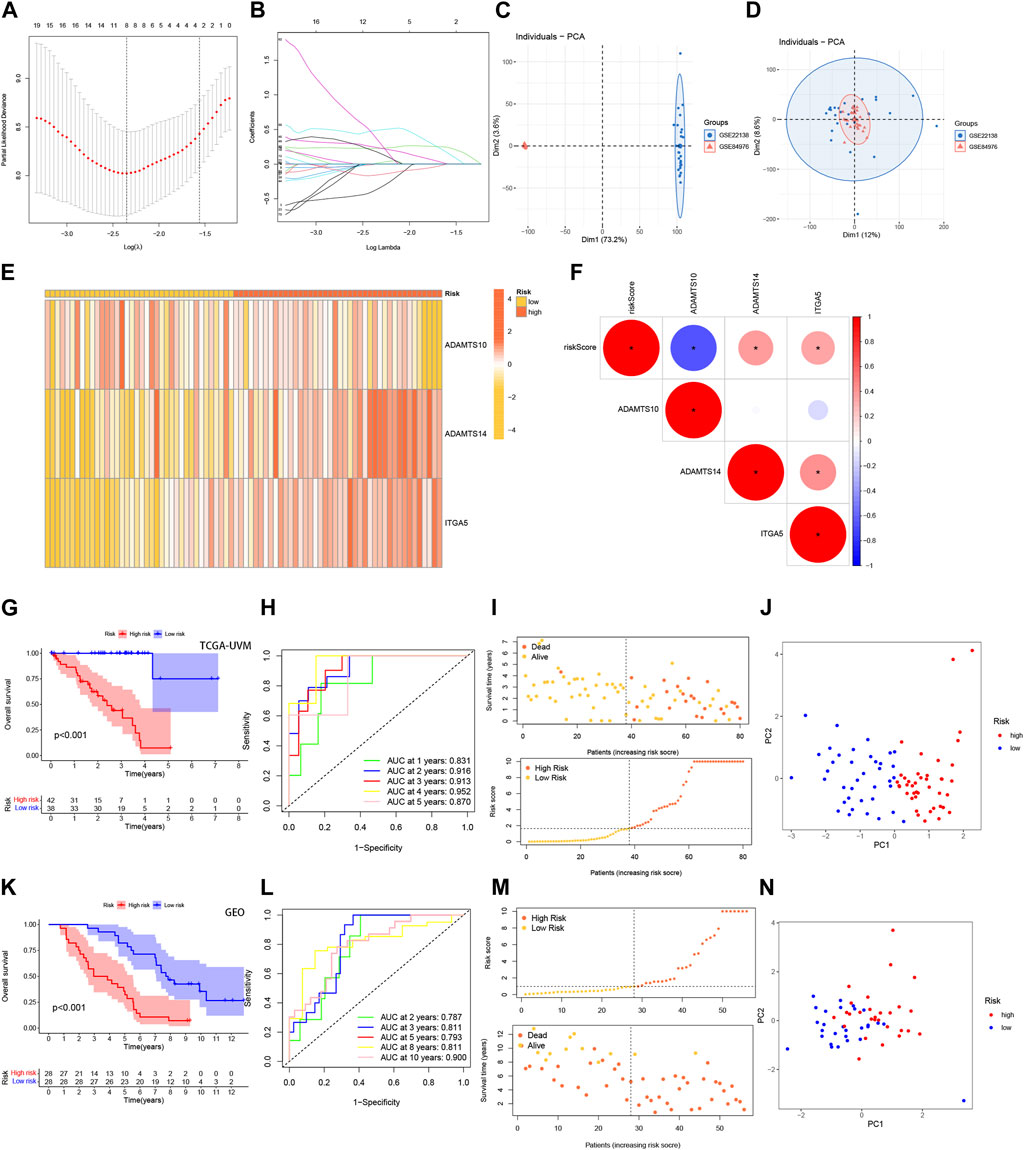
FIGURE 3. Development and validation of the BMRGs signature. (A) Ten-fold cross-validation for parameter selection using the LASSO model. (B) Profiles of LASSO coefficients. (C) Principal component analysis (PCA) plot of GSE22138. (D) PCA plot of GSE84976 after removing batch effects using Combat. (E) Heatmap illustrating the risk factors in high- and low-risk patients. (F) Correlation between three BMRGs and the risk score. (G, K) Kaplan-Meier curves comparing overall survival between low- and high-risk groups in the TCGA-UVM cohort and the GSE84976 cohort. (H, L) Time-dependent receiver operating characteristic (ROC) curves analysis of the TCGA-UVM cohort and the GSE84976 cohort. (I, M) Distribution of risk scores and survival status of UVM patients in the low- and high-risk groups in the TCGA-UVM cohort and the GSE84976 cohort. (J, N) PCA plot of the TCGA-UVM cohort and the GSE84976 cohort.
Further analysis revealed a strong correlation between the expression of the investigated BMRGs and the risk score. The risk score correlation heatmap (Figure 3E) and dot plot (Figure 3F) indicated that the expression levels of ADAMTS10 and ITGA5 were positively correlated with the risk score, while ADAMTS14 was negatively correlated. The TCGA-UVM cohort was used as the training set, and the de-batched GSE84976 dataset was used for validation. In the TCGA-UVM cohort, the low-risk group demonstrated significantly better prognostic outcomes (p < 0.001) (Figure 3G). The predictive model showed excellent performance as evidenced by the ROC curves, with high sensitivity and specificity reflected by the AUC values at 1, 2, 3, 4, and 5 years (0.831, 0.916, 0.913, 0.952, and 0.870) (Figure 3H). Moreover, there was an observed increase in mortality and a decrease in survival with higher risk scores (Figure 3I). Principal component analysis (PCA) clearly distinguished low-risk and high-risk patients from each other (Figure 3J). The results obtained in the GSE41613 cohort replicated those of the TCGA-UVM cohort (Figure 3K-N), indicating the reliability and consistency of our predictive model. In conclusion, our prediction model demonstrates high accuracy and reliability, providing valuable guidance for clinical management.
3.3 Construction of nomograms based on 3-BMRGs signatures with clinical features
Given the strong correlation between our constructed risk model and poor prognosis, we conducted univariate and multivariate Cox analyses to determine whether the prognostic characteristics based on the 3-BMRGs could serve as independent predictors of prognosis in UVM patients. In the univariate analysis, age (p = 0.011), T-stage (p = 0.033), and risk scores (p < 0.001) showed significant correlations with prognosis (Figure 4A). The subsequent multivariate analysis confirmed that age (p = 0.009), T-stage (p = 0.011), and risk scores (p < 0.001) remained accurate and independent predictors in this patient cohort (Figure 4B).
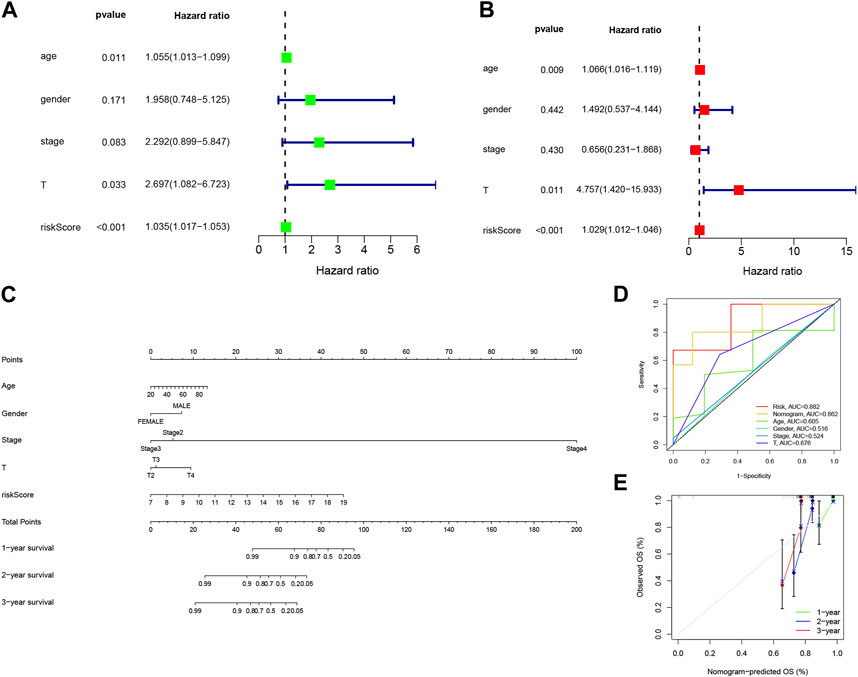
FIGURE 4. Building nomograms based on clinical characteristics. (A) Univariate Cox regression analysis of the signature and various clinical profiles. (B) Multivariate Cox regression analysis incorporating the signature and clinical characteristics. (C) Nomogram depicting age, gender, stage, T-stage, and risk score. (D) Calibration curves of the nomogram for 1-, 3-, and 5-year survival. (E) Time-dependent ROC curve.
To enhance the clinical applicability and usability of the risk model, we developed Nomogram plots that incorporated age, gender, clinical stage, T-stage, and risk scores as predictors of survival probability at 1, 2, and 3 years for UVM patients. The risk score exhibited a substantial impact on predicting overall survival (OS), as demonstrated by the model analysis, indicating that the BMRGs-based risk model could provide more accurate prognostic predictions for UVM patients (Figure 4C). Additionally, we found that the risk score (AUC = 0.882) and Nomogram (AUC = 0.862) outperformed single independent clinical indicators in terms of predictive performance and discriminatory power (Figure 4D). Furthermore, the calibration analysis showed relatively consistent results between the predicted and observed 1-year, 3-year, and 5-year OS rates, as indicated by the calibration line closely aligning with the ideal 45-degree line (Figure 4E).
3.4 Clinical correlation and survival analysis of BMRGs in patients with UVM
A heat map was generated to visualize the correlation between the prognostic risk model identified using the 3-BMRGs and the clinical characteristics, risk scores, and expression levels of the 3-BMRGs in all UVM patients from the TCGA dataset (Figure 5A). Additionally, we compared the distribution of patients with different clinicopathological features between the high-risk and low-risk groups (Figure 5B). To further examine the association between risk scores and clinicopathological characteristics, box plots were constructed for different subgroups based on gender (male and female), age (>65 and ≤65 years), clinical stage (II and III-IV), and T-stage (T2, T3, T4). Notably, the analysis revealed that patients with stage T4 had significantly higher risk scores compared to those with stage T3 (p = 0.045) (Figures 5C–F).
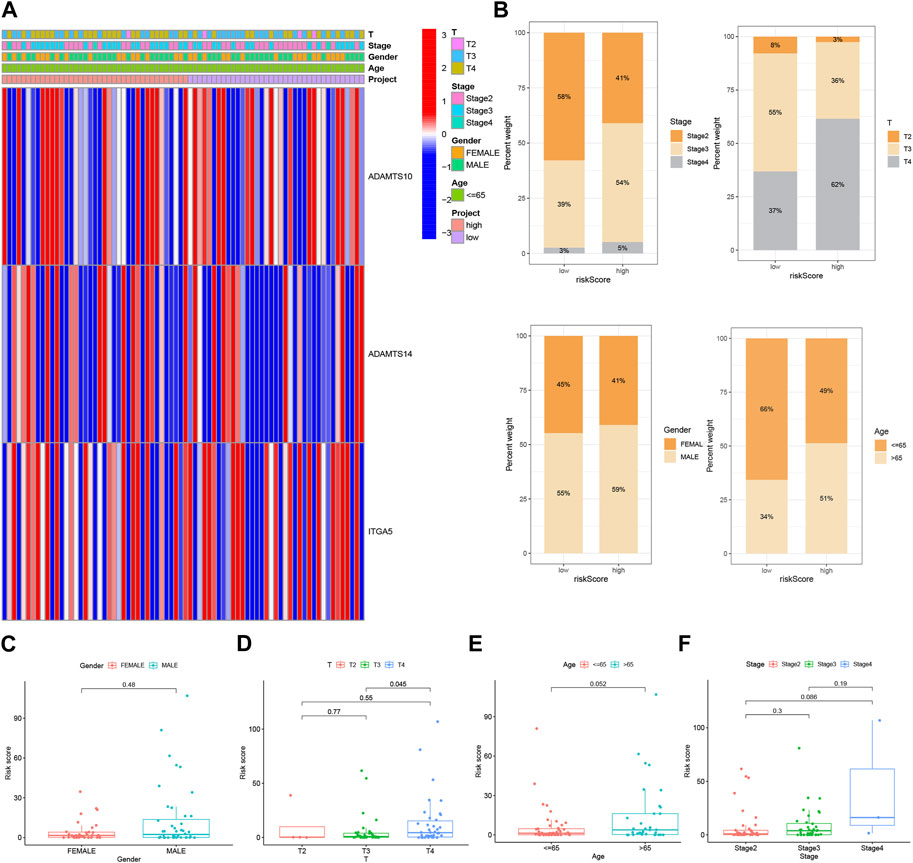
FIGURE 5. Clinical correlation and survival analysis of BMRGs in UVM patients. (A) Heatmap showing the relationship between clinical features and high-risk and low-risk scores in UVM patients. (B) Histogram presenting the distribution of clinical characteristics, including stage, T-stage, gender, and age percentages for each category. BMRGs can identify high-risk patients across different subgroups based on various clinicopathological traits. (C) Gender, (D) T-stage, (E) age, and (F) stage were analyzed for clinical correlation and survival in UVM patients.
Considering the significant differences in individual clinical characteristics between the high-risk and low-risk groups for overall survival (OS), we further divided UVM patients into subgroups based on age (≤65 years, >65 years), gender (male and female), pathological stage (II and III-IV), and T-stage (T2 and T3-4). Remarkably, except for patients in stage T2, the low-risk subgroup exhibited a significant survival advantage with longer survival times compared to the high-risk subgroup (Figure 6). Based on these analyses, the 3-BMRGs risk model demonstrated its reliability as a clinical prediction tool for UVM patients.
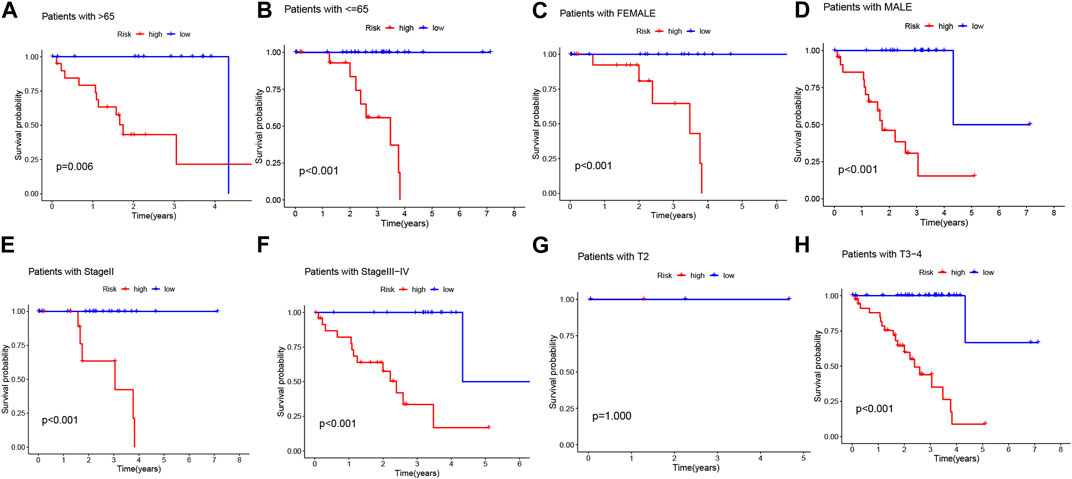
FIGURE 6. Clinical correlation and survival analysis of 3-BMRGs in UVM patients. (A, B) Age, (C, D) gender, (E, F) tumor grade, and (G, H) T-stage evaluated for clinical correlation and survival in UVM patients.
3.5 3-BMRGs signatures exhibit superior performance compared to others in prognostic prediction
In order to assess the predictive performance of our BMRGs signature in UVM patients, we compared it with five previously published prognostic signatures, namely, the Xia signature, Xie signature, Zhang signature, Shi signature, and Hu signature. Using the same method, we calculated risk scores for each UVM sample in the entire TCGA cohort and found that our signature exhibited the highest correlation with survival outcomes (Figure 7AB, IJ). Despite successfully stratifying UVM patients into two subgroups with significantly different prognoses, the AUC values of the five compared signatures at 1-, 3-, and 5-year survival were lower than those of our model (Figures 7E,F,K,L). Additionally, the C-index analysis demonstrated that our signature outperformed the other signatures (Figure 7M). Overall, our study indicates that our constructed BMRGs signature possesses excellent predictive ability in prognosticating UVM patients.
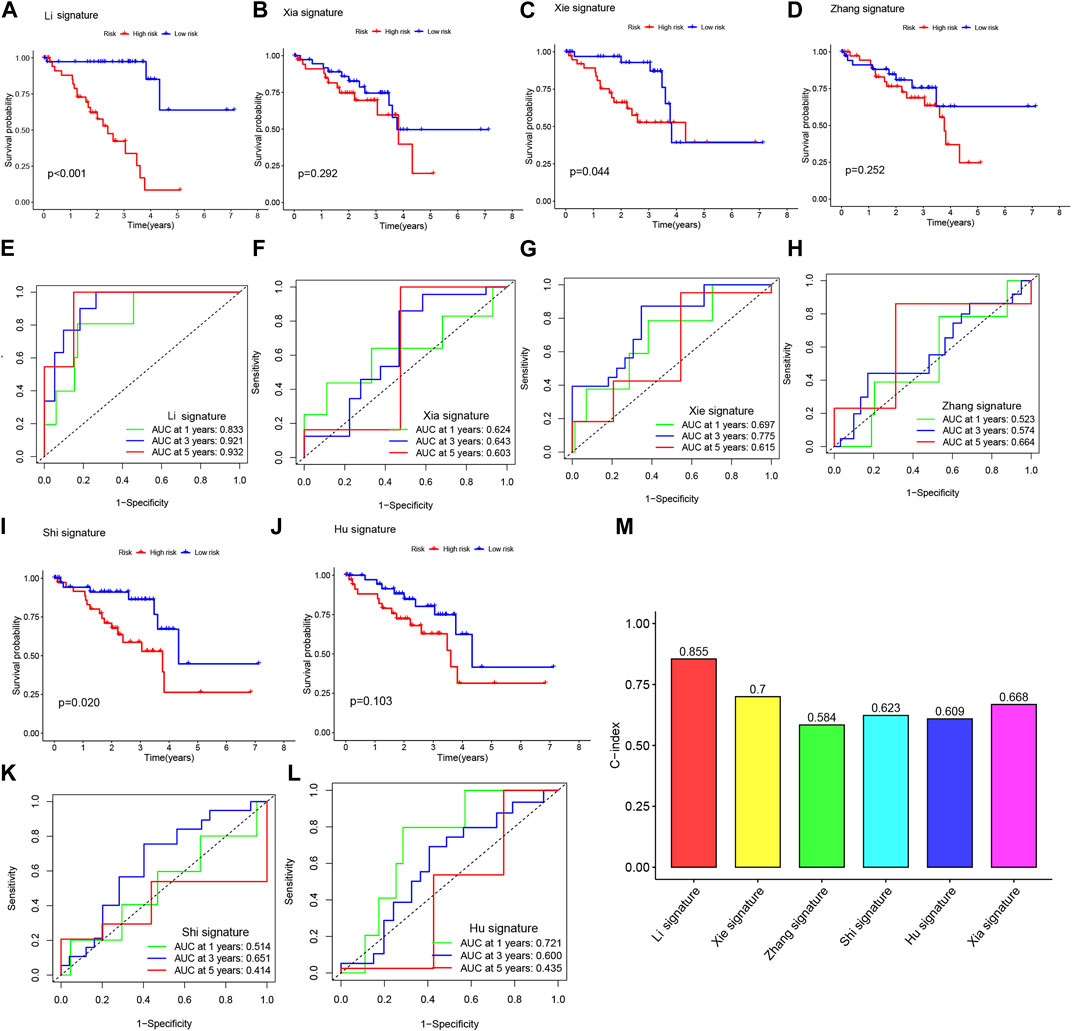
FIGURE 7. The BMRGs signature demonstrated superior prognostic prediction performance compared to other signatures. (A, E) Kaplan-Meier (KM) and receiver operating characteristic (ROC) curves of the BMRGs signature. (B, F) KM and ROC curves of the Xia signature. (C, G) KM and ROC curves of the Xie signature. (D, H) KM and ROC curves of the Zhang signature. (I, K) KM and ROC curves of the Shi signature. (J, L) KM and ROC curves of the Hu signature. (M) C-indexes of the six risk models.
3.6 Functional enrichment analysis of DEGs in TCGA-UVM
In order to gain insights into the potential bioactivities and signaling pathways involved in UVM, and to understand the molecular mechanisms underlying UVM progression, we conducted Kyoto Encyclopedia of Genes and Genomes (KEGG) enrichment analysis and gene ontology (GO) functional analysis. We applied stringent thresholds of FDR<0.05 and p < 0.05 to select significantly enriched items, as depicted in Figure 8A and Supplementary Table S1. The biological process (BP) analysis revealed enrichment in various processes such as rhythmic process, regulation of hormone levels, and circadian rhythm. Cellular component (CC) analysis highlighted correlations with neuronal cell body, presynaptic active zone, and terminal bouton, among others. Molecular function (MF) analysis indicated associations with functions such as G protein-coupled receptor binding, receptor-ligand activity, and signaling receptor activator. Furthermore, the KEGG enrichment analysis unveiled disease pathways including Circadian entrainment, Allograft rejection, and the Chemokine signaling pathway (Figure 8B). Moreover, the Gene Set Variation Analysis (GSVA) identified 50 significantly enriched pathways (Figure 8C). In-depth analysis revealed that in the low-risk population, pathways related to the Regulation of autophagy and RNA degradation were enriched. Conversely, in the high-risk population, pathway enrichment primarily involved immune and substance metabolism pathways such as leukocyte transendothelial migration and antigen processing and presentation. These findings contribute to our understanding of the molecular mechanisms underlying UVM and may provide valuable insights for the development of effective therapeutic strategies for UVM patients.
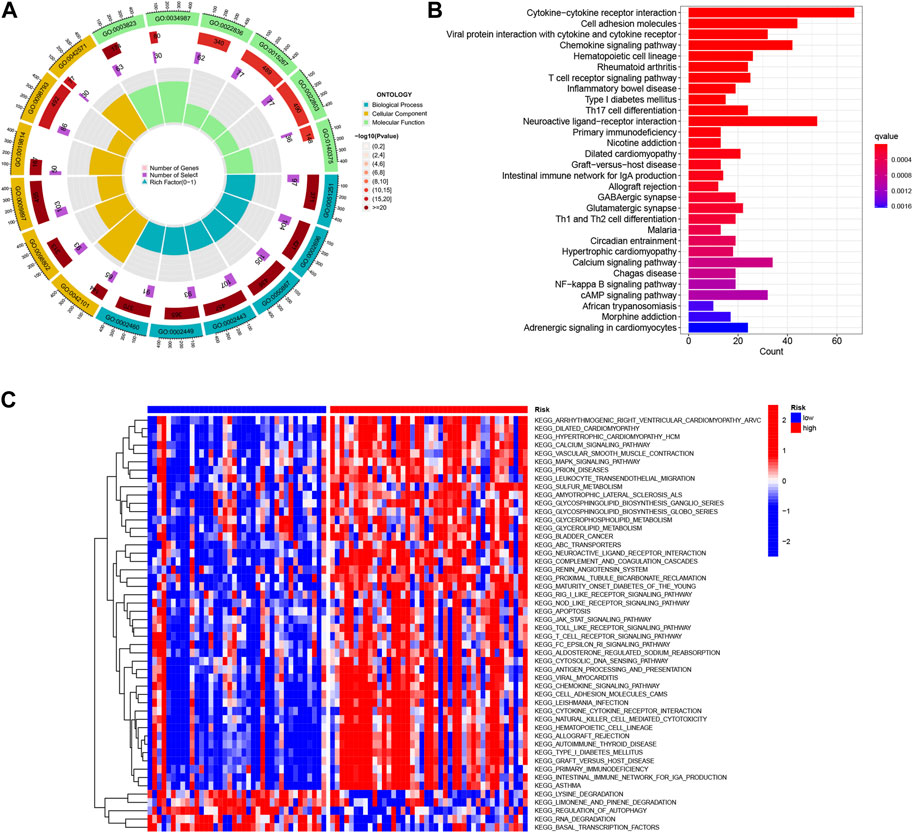
FIGURE 8. Functional enrichment analysis of Differentially Expressed Genes (DEGs) in TCGA-UVM was performed. (A) Gene Ontology (GO) enrichment analysis was conducted to investigate the differences in basement membrane x genes between UVM and normal samples. The analysis included biological processes (BP), cellular components (CC), and molecular functions (MF). (B) KEGG enrichment analysis was performed to identify enriched pathways associated with the DEGs. (C) Gene Set Variation Analysis (GSVA) was utilized to compare the enrichment scores between high-risk and low-risk cohorts, providing insights into the functional differences between these groups.
3.7 Risk score predicts TME and immune cell infiltration
Interactions between cancer cells and the tumor microenvironment (TME) play a crucial role in tumorigenesis, progression, and treatment outcomes. Tumor-infiltrating immune cells (TIICs) are integral components of the TME, and their distribution and alterations are closely associated with tumor progression. In this study, we investigated the relationship between risk scores and immune cell infiltration in the context of 3 BMRGs using seven algorithms: XCELL, TIMER, QUANTISEQ, MCPCOUNTER, CIBERSORT, CIBERSORT-ABS, and EPIC. Our results revealed a positive correlation between risk scores and the presence of T cell CD8+ cells across multiple algorithms (Figure 9A). Furthermore, we analyzed the proportions of 22 immune cell infiltrates between the high-risk and low-risk groups of TCGA-UVM patients using the CIBERSORT algorithm. The results were visualized using stacked plots, demonstrating differences in immune cell composition between the two risk groups (Figure 9B). We also utilized the immune AI portal to assess immunotherapy response in UVM patients. Our analysis revealed that patients with higher risk scores were more likely to benefit from immunotherapy (Figures 9C,D), while those with lower risk scores exhibited a survival advantage. The ROC curves demonstrated the excellent performance of the 3-BMRGs biomarkers in predicting treatment outcomes for patients (Figure 9F). To further explore the immune profile of the tumor microenvironment, we plotted a correlation butterfly diagram to examine the relationship between risk scores and various steps of the tumor immune cycle. The analysis revealed a positive correlation between risk scores and most immune cycle steps, suggesting potential implications for immune modulation in UVM (Figure 9G).
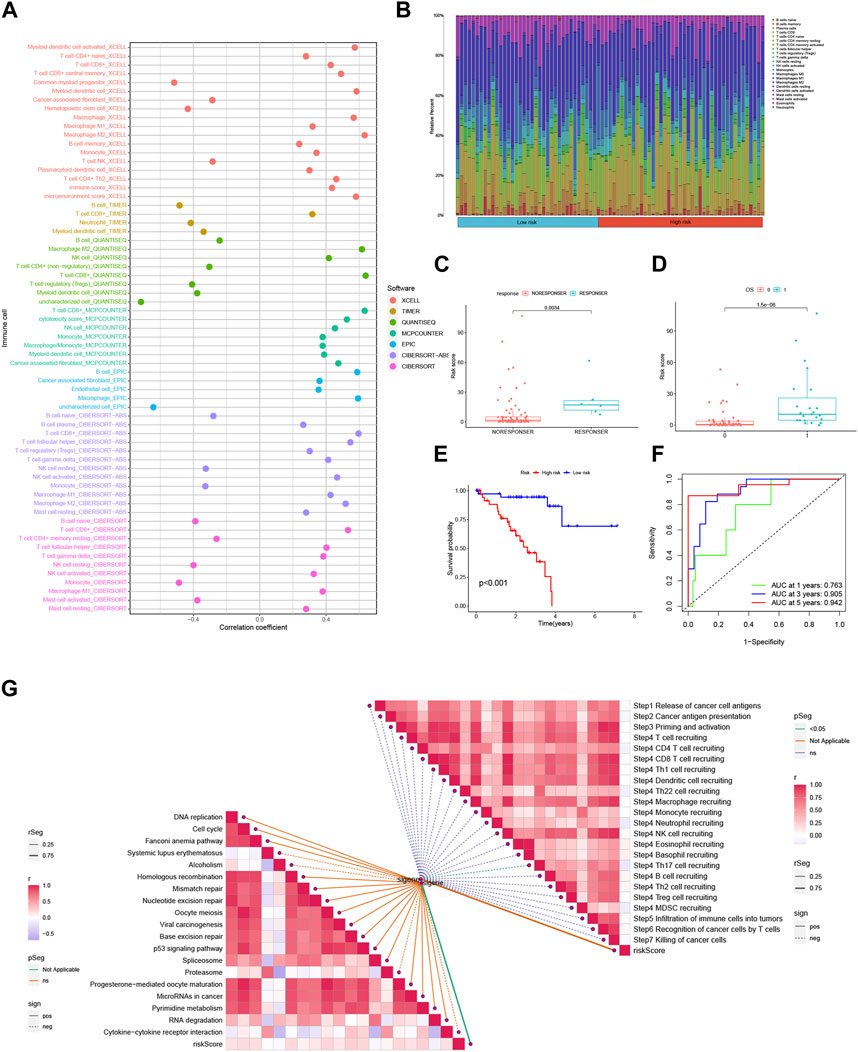
FIGURE 9. The risk score is predictive of the tumor microenvironment (TME) and immune cell infiltration. (A) An immune cell bubble plot was generated to visualize the composition of immune cell types. (B) A stacked plot illustrates the differences in immune cell infiltration between the high-risk and low-risk groups. (C, D) The expression levels of the 3-BMRGs were utilized to predict the response of patients to immune therapy. (E) Kaplan-Meier (KM) curves compare the survival outcomes between the high-risk and low-risk groups after receiving immunotherapy. (F) The receiver operating characteristic (ROC) curve analysis demonstrates the robust predictive performance of the marker model. (H) The correlation between risk scores and immune checkpoint blockade (ICB) response characteristics was examined. (G) The correlation between risk scores and each step of the tumor immunization cycle was investigated.
3.8 Relationships between 3-BMRGs signatures and tumor microenvironment
In order to investigate the expression patterns of the 3-BMRGs in the tumor microenvironment, we utilized the cellular dataset UVM_GSE139829 obtained from the TISCH database. The distribution and numbers of 31 cell populations and 8 immune cell types in the UVM_GSE139829 dataset were analyzed and displayed (Figures 10A–D). Furthermore, we examined the expression of the 3-BMRGs in different immune cell populations. The expression of BMRGs was found to be lower in the ADAMTS14 immune microenvironment (Figure 10E). On the other hand, ADAMTS10 and ITGA5 were expressed in various immune cell populations, as demonstrated in Figures 10F,G, respectively. Notably, ITGA5 showed predominant expression in CD8 Tex, Mono/Macro, and CD8T immune cell populations. These findings provide insights into the expression patterns of the 3-BMRGs within the immune cell landscape of UVM.
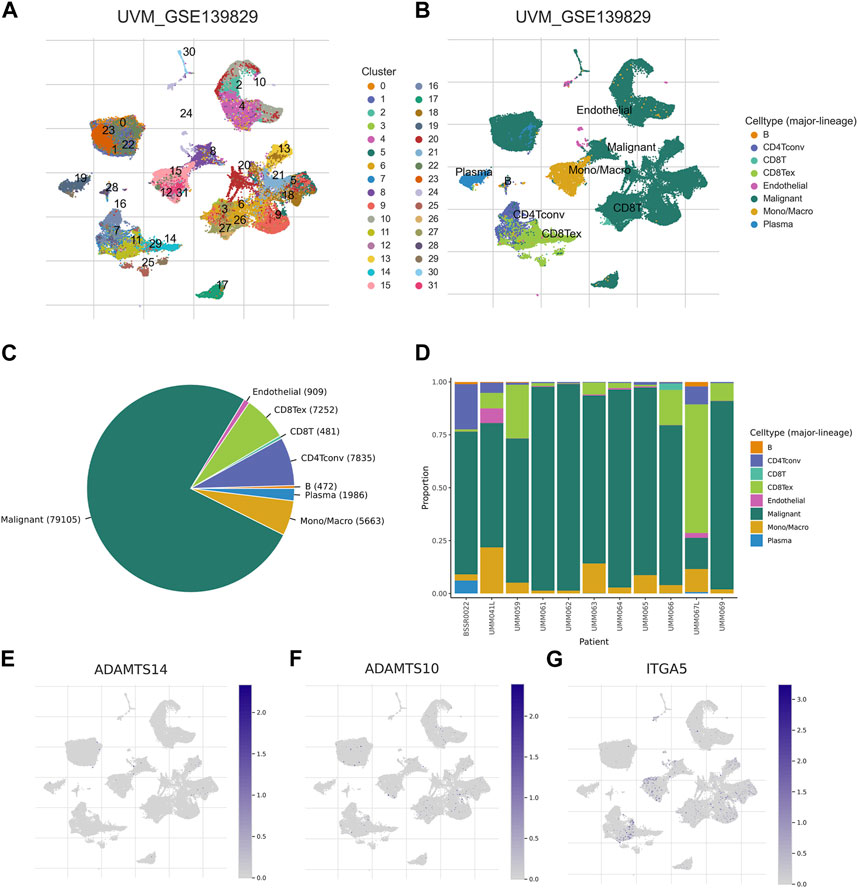
FIGURE 10. Association of BMRGs with the tumor microenvironment. Annotation of (A) 31 cell clusters and (B) 8 cell types in UVM_GSE139829. (C, D) The distribution and proportion of each cell type, including CD8T cells, endothelial cells, B cells, etc. Expression and percentage of (E) ADAMTS14, (F) ADAMTS10, (G) ITGA5.
3.9 ITGA5 facilitates the proliferation, migration, and invasion of uveal melanoma (UVM) cells
Considering the identification of ITGA5 as a high-risk gene exhibiting a maximum absolute hazard ratio (HR) in uveal melanoma (UVM) patients, we conducted additional in vitro experiments to elucidate the specific role of ITGA5 in UVM. Knockdown systems targeting ITGA5 were established in OCM-1 and MUM-2C cell lines. The CCK-8 assay, and colony formation assay demonstrated a significant reduction in the proliferation rate of UVM cells following ITGA5 silencing (Figures 11A,B). Moreover, both the Transwell assay and the wound healing assay revealed diminished migration and invasiveness of UVM cells after ITGA5 knockdown, in comparison to cells transfected with si-NC (Figures 11C,D). Collectively, these findings provide evidence that ITGA5 functions as an oncogene, promoting the malignant characteristics of UVM cells, including proliferation, invasion, and migration.
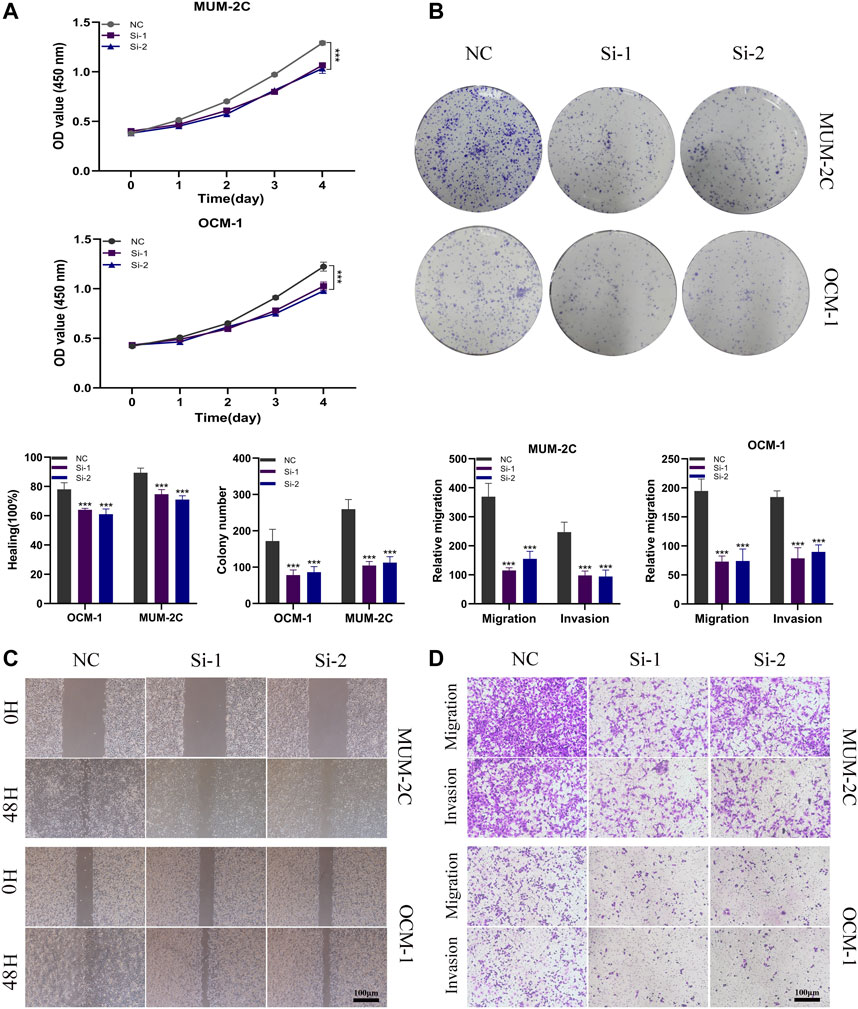
FIGURE 11. ITGA5 facilitates the proliferation, migration, and invasion of UVM cells. (A) CCK-8 assay showed that the proliferative capacity of UVM cells was significantly reduced after silencing of ITGA5. (B) Colony formation assays showed that the ability of UVM cells to form colonies was significantly reduced after ITGA5 silencing. (C, D) In wound healing and transwell assays, silencing of ITGA5 significantly reduced the migratory and invasive capacity of MuM-2B and OCM-1 cell lines.
4 Discussion
Although the prevalence of UVM is not extremely high, it accounts for 85% of all ocular melanomas, with up to 50% of patients of primary UVM developing distant metastases, 90% with liver damage, and a median survival of 4–5 months (Baggetto et al., 2005; Tabernero, 2007; Carvajal et al., 2017; Brouwer et al., 2019). Additionally, the metastatic rates for UVM throughout the course of 5 and 10 years are roughly 25% and 34%, respectively, and the mortality rate for UVM 1 year after metastasis is 80%. After diagnosis, the majority of patients with metastatic UVM have a survival span of 6–12 months for which metastatic UVM is virtually always challenging to treat. Due to the poor prognosis of UVM, few individuals can receive possibly curative surgery (Singh et al., 2005; Straatsma et al., 2018). Meanwhile, 5-year survival rates have remained essentially unchanged over the past 3 decades despite the development of efficient local therapy. There are currently no effective adjuvant systemic medications that have been proven to lower the risk of metastasis as well as actually extend survival, according to a recent review study (Triozzi and Singh, 2014).
Actually, early diagnosis and therapy are crucial to improving prognosis, while UVM diagnosis and prognosis prediction are currently reliant on clinical presentation and histological examination, which are insufficient to identify tumor heterogeneity and developmental patterns. Therefore, using the TCGA-UVM dataset, this study constructed a multigene prognostic model of genes related to basement membrane proteins from a molecular perspective in order to better predict the diagnosis and prognosis of UVM. It opens up new avenues for the investigation of individualized treatment strategies and prognosis prediction.
Defects in BM protein expression and turnover play a major role in the development of cancer, fibrosis, and diabetes (Tsilibary, 2003; Naba et al., 2014; Foster, 2017). Specifically, the overexpression of laminin, a component of BM protein, is closely associated with the overproliferation of certain tumor cells, such as those found in colon and breast cancer (Jayadev and Sherwood, 2017). Moreover, BM is significantly involved in the progression of tumors. During the early development of breast cancer, cancer cells invade through the BM foramen, which is a crucial step in metastasis (Sikic et al., 2022). In addition, the level of netrin-4 in BM is highly correlated with the prognosis of breast cancer, renal cancer, and uveal melanoma (Reuten et al., 2021). Several recent studies have already attempted to mine public databases to identify prognostic gene signatures related to BM proteins in tumors. For example, Cai et al. identified a 7-gene signature associated with basement membrane proteins that predicted the prognostic status of breast cancer patients and provided insights for immunotherapy (Cai et al., 2022); Zhou et al. developed a risk model using 8 BMRGs, which revealed that clear-cell renal cell carcinoma patients in the low-risk group had a better response to immunotherapy (Zhou et al., 2022); Lin et al. established a 7-BMRG signature and identified five small compounds that could potentially be used for the treatment of pancreatic cancer patients, providing new perspectives for a deeper understanding of this disease (Lin et al., 2023). Overall, these studies highlight the significance of basement membrane proteins in the precise treatment of tumors.
In our study, we selected three basement membrane related-genes (ADAMTS10, ADAMTS14, and ITGA5) to create the novel prognostic model by utilizing Lasso regression analysis, SVM-RFE, and stepwise multiple COX regression analysis. Numerous studies have proven that the ADAMTS (a disintegrin and metalloproteinase with thrombospondin motif) family of proteins contributes to the development of malignant tumors, cell proliferation, apoptosis, migration, invasion, and angiogenesis (Held-Feindt et al., 2006; Rocks et al., 2006; Filou et al., 2015; Sun et al., 2015). ADAMTSs have a negative impact on the prognosis of patients with 24 tumors, in particular the patients with Adrenocortical carcinoma, Uveal Melanoma, Kidney renal clear cell carcinoma, Colon adenocarcinoma, Thyroid carcinoma, etc. (Wu et al., 2021). Although the precise mechanism by which ADAMTS play a role in tumor progression and metastasis remains uncertain, several research has explored these protein hydrolases and confirmed their relevance in various tumor types. ADAMTS10 expression is significantly downregulated in tumors (Sun et al., 2015). Furthermore, a variety of ADAMTSs, including ADAMTS20, ADAMTS10, and ADAMTS3, exhibit significant levels of methylation in a range of tumors. Analysis of the relationship between methylation and gene expression levels reveals a negative relationship between the two, suggesting that the main function of ADAMTS methylation is to silence the ADAMTS gene, leading to a decrease in its expression (Wu et al., 2021). Besides, ADAMTS10 is frequently mutated in metastatic colorectal cancer, and mutated ADAMTS10 transcripts are actively expressed in the corresponding tumors implicating a possible role for ADAMTS10 in tumor metastasis (Oga et al., 2019). According to numerous research, the ADAMTS14 gene has been associated with an elevated likelihood of developing tumors. The expression of ADAMTS14 was identified to be considerably higher in human breast cancer tissues, according to Porter et al. (2004). As reported by Sheu et al., ADAMTS14 gene polymorphisms serve a part in the progression of hepatocellular carcinoma (Sheu et al., 2017). The expression of ADAMTS14 in oral squamous carcinoma (OSCC) is low. In OSCC patients, the downregulation of ADAMTS14 may be an effective independent prognostic marker for predicting overall survival because it is predictive of unfavorable clinicopathological characteristics (Lin et al., 2020). Furthermore, there is mounting evidence that Integrin A5 (ITGA5), which plays a major role in the adhesion, migration, and invasion of cancer cells, is highly expressed in several malignancies and contributes to tumor progression (Ohyagi-Hara et al., 2013). One of the markers of invasiveness in head and neck squamous cell carcinoma has been identified as ITGA5 (Yu et al., 2008). Additionally, a study demonstrated that pancreatic ductal malignant adenomas upregulate the ITGA5 gene. Silencing of ITGA5 inhibits the differentiation of human pancreatic stellate cells and reduces connective tissue formation (Kuninty et al., 2019). Furthermore, ITGA5 promotes the development, migration, and invasion of cells that undergo an epithelial-mesenchymal transition in oral cancer (Deng et al., 2019).
The 3-BMRGs we constructed proved to be an independent prognostic factor for UVM. Based on median risk ratings, patients with UVM were separated into high-risk and low-risk groups; there were notable prognostic differences between the two groups. The 3-BMRGs that we created turned out to be a reliable indicator of UVM’s future. Based on median risk ratings, individuals with UVM were separated into high-risk and low-risk groups; there were notable prognostic differences between the two groups. Additionally, evaluations of the ROC and calibration curves revealed that the 3-BMRGs signature had excellent predictive power. To extend the predictive ability of the 3-BMRGs signature and to demonstrate its utility in the prognostic evaluation of UVM patients, we plotted a line graph based on clinical factors and risk scores. Meanwhile, we discovered that the 3-BMRGs signature has better predictive power than clinicopathological features, which could offer clinicians a basis for decision-making.
The tumor microenvironment (TME) is crucial to the metastasis and progression of cancer (Zhao et al., 2022c; Gong et al., 2022; Xiong et al., 2023). The TME comprises cancer cells, surrounding stromal cells, and tumor-infiltrating immune cells, with immune cells playing a dominant role in the TME (Hinshaw and Shevde, 2019; Shen et al., 2022). Through the strengthening of a weakened immune response to tumor cells and the resultant production of an immunological-mediated anti-tumor impact, immunotherapy has made significant strides in the treatment of cancers in recent years (Zhang et al., 2021). As a result, we assessed immune checkpoint expression and discovered that, with the exception of TMIGD2 and CD44, it was highly elevated in the high-risk group of UVM patients. Immune infiltration is closely related to immunotherapy’s efficacy (Nishida and Kudo, 2020). The high-risk group exhibited higher levels of immune cell infiltration, which suggested that they responded more favorably to immunotherapy, according to the ssGSEA enrichment score. Meanwhile, we performed GO and KEGG enrichment analysis to provide more light on the biological pathways and putative molecular mechanisms associated with the BMRG signature. We noted that in the high-risk population, pathway enrichment mainly involved immune and substance metabolism pathways, such as leukocyte transendothelial migration, antigen processing, and presentation. In contrast, pathways related to the regulation of autophagy and RNA degradation were enriched in the low-risk population.
Immune checkpoint blockade has shown significant benefits in the treatment of malignant tumors. Nevertheless, its non-response rate and side effects have posed challenges in clinical practice (Hodi et al., 2010; Kennedy and Salama, 2020; Su et al., 2022). Thus, it is critical to identify individuals who are responsive to different immune checkpoint medicines based on the expression of immune checkpoint genes. Our model has demonstrated excellent results in this regard. In our study, we found that UVM patients with higher risk scores were more likely to benefit from immunotherapy, while patients with lower risk scores could have a higher survival advantage. Furthermore, the TME is closely correlated to the risk model that we built based on the BMRGs.
Our study suffers from the following limitations. Firstly, our study is retrospective, and based on data analysis in public databases with limited inclusion of UVM patients which still demands more clinical data and prospective studies to validate the model and improve the credibility of risk scores; Meanwhile, the extrapolation of our findings is limited due to the possible inherent bias and limitations of the TCGA-UVM and GEO cohorts themselves; In addition, the mechanisms by which BMRGs affect the prognosis of UVM patients are required to be further explored in more in vivo experiments.
5 Conclusion
To sum up, we have developed a model of the BMRG prognostic signatures including ADAMTS10, ADAMTS14, and ITGA5. Two external validation cohorts were employed to verify the reliability and applicability of the BMRGs scores. This constructed model exhibited robust predictive ability which could act as an independent prognostic factor for UVM, assisting clinicians to identify specific subgroups of patients who may benefit from immunotherapy and chemotherapy, and providing a novel strategy for individualized treatment of UVM patients.
Data availability statement
The datasets presented in this study can be found in online repositories. The names of the repository/repositories and accession number(s) can be found in the article/Supplementary Material.
Author contributions
YL: Writing–original draft. HC: Validation, Writing–original draft. JY: Data curation, Visualization, Writing–original draft. XX: Data curation, Visualization, Writing–original draft. SP: Validation, Writing–original draft. YW: Writing–original draft. JZ: Writing–original draft. GS: Writing–original draft. JZ: Writing–original draft. QZ: Writing–review and editing. HC: Conceptualization, Data curation, Visualization, Writing–original draft, Writing–review and editing, Validation. GY: Conceptualization, Writing–review and editing.
Funding
The author(s) declare that no financial support was received for the research, authorship, and/or publication of this article.
Acknowledgments
All authors acknowledge the contributions from the TCGA and GEO project.
Conflict of interest
The authors declare that the research was conducted in the absence of any commercial or financial relationships that could be construed as a potential conflict of interest.
Publisher’s note
All claims expressed in this article are solely those of the authors and do not necessarily represent those of their affiliated organizations, or those of the publisher, the editors and the reviewers. Any product that may be evaluated in this article, or claim that may be made by its manufacturer, is not guaranteed or endorsed by the publisher.
Supplementary material
The Supplementary Material for this article can be found online at: https://www.frontiersin.org/articles/10.3389/fphar.2023.1264345/full#supplementary-material
References
Andreoli, M. T., Mieler, W. F., and Leiderman, Y. I. (2015). Epidemiological trends in uveal melanoma. Br. J. Ophthalmol. 99 (11), 1550–1553. doi:10.1136/bjophthalmol-2015-306810
Augsburger, J. J., Corrêa, Z. M., and Shaikh, A. H. (2009). Effectiveness of treatments for metastatic uveal melanoma. Am. J. Ophthalmol. 148 (1), 119–127. doi:10.1016/j.ajo.2009.01.023
Baggetto, L. G., Gambrelle, J., Dayan, G., Labialle, S., Barakat, S., Michaud, M., et al. (2005). Major cytogenetic aberrations and typical multidrug resistance phenotype of uveal melanoma: Current views and new therapeutic prospects. Cancer Treat. Rev. 31 (5), 361–379. doi:10.1016/j.ctrv.2005.05.001
Banerjee, S., Lo, W. C., Majumder, P., Roy, D., Ghorai, M., Shaikh, N. K., et al. (2022). Multiple roles for basement membrane proteins in cancer progression and EMT. Eur. J. Cell. Biol. 101 (2), 151220. doi:10.1016/j.ejcb.2022.151220
Bhattacherjee, A., Djekidel, M. N., Chen, R., Chen, W., Tuesta, L. M., and Zhang, Y. (2019). Cell type-specific transcriptional programs in mouse prefrontal cortex during adolescence and addiction. Nat. Commun. 10 (1), 4169. doi:10.1038/s41467-019-12054-3
Bol, K. F., van den Bosch, T., Schreibelt, G., Mensink, H. W., Keunen, J. E., Kiliç, E., et al. (2016). Adjuvant dendritic cell vaccination in high-risk uveal melanoma. Ophthalmology 123 (10), 2265–2267. doi:10.1016/j.ophtha.2016.06.027
Brouwer, N. J., Gezgin, G., Wierenga, A. P. A., Bronkhorst, I. H. G., Marinkovic, M., Luyten, G. P. M., et al. (2019). Tumour angiogenesis in uveal melanoma is related to genetic evolution. Cancers (Basel) 11 (7), 979. doi:10.3390/cancers11070979
Cai, J., Zhang, X., Xie, W., Li, Z., Liu, W., and Liu, A. (2022). Identification of a basement membrane-related gene signature for predicting prognosis and estimating the tumor immune microenvironment in breast cancer. Front. Endocrinol. (Lausanne) 13, 1065530. doi:10.3389/fendo.2022.1065530
Carvajal, R. D., Schwartz, G. K., Tezel, T., Marr, B., Francis, J. H., and Nathan, P. D. (2017). Metastatic disease from uveal melanoma: Treatment options and future prospects. Br. J. Ophthalmol. 101 (1), 38–44. doi:10.1136/bjophthalmol-2016-309034
Chattopadhyay, C., Kim, D. W., Gombos, D. S., Oba, J., Qin, Y., Williams, M. D., et al. (2016). Uveal melanoma: From diagnosis to treatment and the science in between. Cancer 122 (15), 2299–2312. doi:10.1002/cncr.29727
Chi, H., Peng, G., Yang, J., Zhang, J., Song, G., Xie, X., et al. (2022). Machine learning to construct sphingolipid metabolism genes signature to characterize the immune landscape and prognosis of patients with uveal melanoma. Front. Endocrinol. (Lausanne). 13, 1056310. doi:10.3389/fendo.2022.1056310
Chi, H., Gao, X., Xia, Z., Yu, W., Yin, X., Pan, Y., et al. (2023a). FAM family gene prediction model reveals heterogeneity, stemness and immune microenvironment of UCEC. Front. Mol. Biosci. 10, 1200335. doi:10.3389/fmolb.2023.1200335
Chi, H., Chen, H., Wang, R., Zhang, J., Jiang, L., Zhang, S., et al. (2023b). Proposing new early detection indicators for pancreatic cancer: Combining machine learning and neural networks for serum miRNA-based diagnostic model. Front. Oncol. 13, 1244578. doi:10.3389/fonc.2023.1244578
Curran, M. A., Montalvo, W., Yagita, H., and Allison, J. P. (2010). PD-1 and CTLA-4 combination blockade expands infiltrating T cells and reduces regulatory T and myeloid cells within B16 melanoma tumors. Proc. Natl. Acad. Sci. U. S. A. 107 (9), 4275–4280. doi:10.1073/pnas.0915174107
Damato, B. (2018). Ocular treatment of choroidal melanoma in relation to the prevention of metastatic death - a personal view. Prog. Retin Eye Res. 66, 187–199. doi:10.1016/j.preteyeres.2018.03.004
Deng, Y., Wan, Q., and Yan, W. (2019). Integrin α5/ITGA5 promotes the proliferation, migration, invasion and progression of oral squamous carcinoma by epithelial-mesenchymal transition. Cancer Manag. Res. 11, 9609–9620. doi:10.2147/CMAR.S223201
Derrien, A. C., Rodrigues, M., Eeckhoutte, A., Dayot, S., Houy, A., Mobuchon, L., et al. (2021). Germline MBD4 mutations and predisposition to uveal melanoma. J. Natl. Cancer Inst. 113 (1), 80–87. doi:10.1093/jnci/djaa047
Engebretsen, S., and Bohlin, J. (2019). Statistical predictions with glmnet. Clin. Epigenetics 11 (1), 123. doi:10.1186/s13148-019-0730-1
Filou, S., Korpetinou, A., Kyriakopoulou, D., Bounias, D., Stavropoulos, M., Ravazoula, P., et al. (2015). ADAMTS expression in colorectal cancer. PLoS One 10 (3), e0121209. doi:10.1371/journal.pone.0121209
Foster, M. H. (2017). Basement membranes and autoimmune diseases. Matrix Biol. 57-58, 149–168. doi:10.1016/j.matbio.2016.07.008
Gong, X., Chi, H., Strohmer, D. F., Teichmann, A. T., Xia, Z., and Wang, Q. (2022). Exosomes: A potential tool for immunotherapy of ovarian cancer. Front. Immunol. 13, 1089410. doi:10.3389/fimmu.2022.1089410
Hänzelmann, S., Castelo, R., and Guinney, J. (2013). Gsva: Gene set variation analysis for microarray and RNA-seq data. BMC Bioinforma. 14, 7. doi:10.1186/1471-2105-14-7
Held-Feindt, J., Paredes, E. B., Blömer, U., Seidenbecher, C., Stark, A. M., Mehdorn, H. M., et al. (2006). Matrix-degrading proteases ADAMTS4 and ADAMTS5 (disintegrins and metalloproteinases with thrombospondin motifs 4 and 5) are expressed in human glioblastomas. Int. J. Cancer 118 (1), 55–61. doi:10.1002/ijc.21258
Hinshaw, D. C., and Shevde, L. A. (2019). The tumor microenvironment innately modulates cancer progression. Cancer Res. 79 (18), 4557–4566. doi:10.1158/0008-5472.CAN-18-3962
Hodi, F. S., O'Day, S. J., McDermott, D. F., Weber, R. W., Sosman, J. A., Haanen, J. B., et al. (2010). Improved survival with ipilimumab in patients with metastatic melanoma. N. Engl. J. Med. 363 (8), 711–723. doi:10.1056/NEJMoa1003466
Huang, X., Chi, H., Gou, S., Guo, X., Li, L., Peng, G., et al. (2023). An aggrephagy-related LncRNA signature for the prognosis of pancreatic adenocarcinoma. Genes. (Basel) 14 (1), 124. doi:10.3390/genes14010124
Jayadev, R., and Sherwood, D. R. (2017). Basement membranes. Curr. Biol. 27 (6), R207–R211. doi:10.1016/j.cub.2017.02.006
Jin, W., Yang, Q., Chi, H., Wei, K., Zhang, P., Zhao, G., et al. (2022). Ensemble deep learning enhanced with self-attention for predicting immunotherapeutic responses to cancers. Front. Immunol. 13, 1025330. doi:10.3389/fimmu.2022.1025330
Katopodis, P., Khalifa, M. S., and Anikin, V. (2021). Molecular characteristics of uveal melanoma and intraocular tumors. Oncol. Lett. 21 (1), 9. doi:10.3892/ol.2020.12270
Kennedy, L. B., and Salama, A. K. S. (2020). A review of cancer immunotherapy toxicity. CA Cancer J. Clin. 70 (2), 86–104. doi:10.3322/caac.21596
Kuninty, P. R., Bansal, R., De Geus, S. W. L., Mardhian, D. F., Schnittert, J., van Baarlen, J., et al. (2019). ITGA5 inhibition in pancreatic stellate cells attenuates desmoplasia and potentiates efficacy of chemotherapy in pancreatic cancer. Sci. Adv. 5 (9), eaax2770. doi:10.1126/sciadv.aax2770
Larkin, J., Chiarion-Sileni, V., Gonzalez, R., Grob, J. J., Cowey, C. L., Lao, C. D., et al. (2015). Combined nivolumab and ipilimumab or monotherapy in untreated melanoma. N. Engl. J. Med. 373 (1), 23–34. doi:10.1056/NEJMoa1504030
Li, S., Edgar, D., Fässler, R., Wadsworth, W., and Yurchenco, P. D. (2003). The role of laminin in embryonic cell polarization and tissue organization. Dev. Cell. 4 (5), 613–624. doi:10.1016/s1534-5807(03)00128-x
Liberzon, A., Birger, C., Thorvaldsdóttir, H., Ghandi, M., Mesirov, J. P., and Tamayo, P. (2015). The Molecular Signatures Database (MSigDB) hallmark gene set collection. Cell. Syst. 1 (6), 417–425. doi:10.1016/j.cels.2015.12.004
Lin, Y. M., Lin, C. W., Lu, J. W., Yeh, K. T., Lin, S. H., and Yang, S. F. (2020). Decreased cytoplasmic expression of ADAMTS14 is correlated with reduced survival rates in oral squamous cell carcinoma patients. Diagn. (Basel). 10 (2), 122. doi:10.3390/diagnostics10020122
Lin, K., Xu, D., Wang, X., Shi, J., and Gao, W. (2023). Development of a basement membrane gene signature and identification of the potential candidate therapeutic targets for pancreatic cancer. Gland. Surg. 12 (2), 263–281. doi:10.21037/gs-23-24
Lu, Y., Liu, L., Pan, J., Luo, B., Zeng, H., Shao, Y., et al. (2021). MFG-E8 regulated by miR-99b-5p protects against osteoarthritis by targeting chondrocyte senescence and macrophage reprogramming via the NF-κB pathway. Cell. Death Dis. 12 (6), 533. doi:10.1038/s41419-021-03800-x
Lv, Y., He, L., Jin, M., Sun, W., Tan, G., and Liu, Z. (2022). EMT-related gene signature predicts the prognosis in uveal melanoma patients. J. Oncol. 2022, 5436988. doi:10.1155/2022/5436988
Mao, S., Yu, X., Shan, Y., Fan, R., Wu, S., and Lu, C. (2021). Albumin-bilirubin (ALBI) and monocyte to lymphocyte ratio (MLR)-Based nomogram model to predict tumor recurrence of AFP-negative hepatocellular carcinoma. J. Hepatocell. Carcinoma 8, 1355–1365. doi:10.2147/JHC.S339707
Miao, Y. R., Zhang, Q., Lei, Q., Luo, M., Xie, G. Y., Wang, H., et al. (2020). ImmuCellAI: A unique method for comprehensive T-cell subsets abundance prediction and its application in cancer immunotherapy. Adv. Sci. (Weinh) 7 (7), 1902880. doi:10.1002/advs.201902880
Naba, A., Clauser, K. R., Whittaker, C. A., Carr, S. A., Tanabe, K. K., and Hynes, R. O. (2014). Extracellular matrix signatures of human primary metastatic colon cancers and their metastases to liver. BMC Cancer 14, 518. doi:10.1186/1471-2407-14-518
Nishida, N., and Kudo, M. (2020). Immune phenotype and immune checkpoint inhibitors for the treatment of human hepatocellular carcinoma. Cancers (Basel) 12 (5), 1274. doi:10.3390/cancers12051274
Oga, T., Yamashita, Y., Soda, M., Kojima, S., Ueno, T., Kawazu, M., et al. (2019). Genomic profiles of colorectal carcinoma with liver metastases and newly identified fusion genes. Cancer Sci. 110 (9), 2973–2981. doi:10.1111/cas.14127
Ohyagi-Hara, C., Sawada, K., Kamiura, S., Tomita, Y., Isobe, A., Hashimoto, K., et al. (2013). miR-92a inhibits peritoneal dissemination of ovarian cancer cells by inhibiting integrin α5 expression. Am. J. Pathol. 182 (5), 1876–1889. doi:10.1016/j.ajpath.2013.01.039
Porter, S., Scott, S. D., Sassoon, E. M., Williams, M. R., Jones, J. L., Girling, A. C., et al. (2004). Dysregulated expression of adamalysin-thrombospondin genes in human breast carcinoma. Clin. Cancer Res. 10 (7), 2429–2440. doi:10.1158/1078-0432.ccr-0398-3
Ren, Q., Zhang, P., Lin, H., Feng, Y., Chi, H., Zhang, X., et al. (2023). A novel signature predicts prognosis and immunotherapy in lung adenocarcinoma based on cancer-associated fibroblasts. Front. Immunol. 14, 1201573. doi:10.3389/fimmu.2023.1201573
Reuten, R., Zendehroud, S., Nicolau, M., Fleischhauer, L., Laitala, A., Kiderlen, S., et al. (2021). Basement membrane stiffness determines metastases formation. Nat. Mater 20 (6), 892–903. doi:10.1038/s41563-020-00894-0
Rocks, N., Paulissen, G., Quesada Calvo, F., Polette, M., Gueders, M., Munaut, C., et al. (2006). Expression of a disintegrin and metalloprotease (ADAM and ADAMTS) enzymes in human non-small-cell lung carcinomas (NSCLC). Br. J. Cancer 94 (5), 724–730. doi:10.1038/sj.bjc.6602990
Rusňák, Š., Hecová, L., Kasl, Z., Sobotová, M., and Hauer, L. (2020). Therapy of uveal melanoma A review. Cesk Slov. Oftalmol. 77 (1), 1–13. doi:10.31348/2020/10
Shen, Y., Chi, H., Xu, K., Li, Y., Yin, X., Chen, S., et al. (2022). A novel classification model for lower-grade glioma patients based on pyroptosis-related genes. Brain Sci. 12 (6), 700. doi:10.3390/brainsci12060700
Shen, J., Wei, Z., Lv, L., He, J., Du, S., Wang, F., et al. (2023). A model of basement membrane-associated gene signature predicts liver hepatocellular carcinoma response to immune checkpoint inhibitors. Mediat. Inflamm. 2023, 7992140. doi:10.1155/2023/7992140
Sheu, M. J., Hsieh, M. J., Chou, Y. E., Wang, P. H., Yeh, C. B., Yang, S. F., et al. (2017). Effects of ADAMTS14 genetic polymorphism and cigarette smoking on the clinicopathologic development of hepatocellular carcinoma. PLoS One 12 (2), e0172506. doi:10.1371/journal.pone.0172506
Sikic, L., Schulman, E., Kosklin, A., Saraswathibhatla, A., Chaudhuri, O., and Pokki, J. (2022). Nanoscale tracking combined with cell-scale microrheology reveals stepwise increases in force generated by cancer cell protrusions. Nano Lett. 22 (18), 7742–7750. doi:10.1021/acs.nanolett.2c01327
Singh, A. D., Bergman, L., and Seregard, S. (2005). Uveal melanoma: Epidemiologic aspects. Ophthalmol. Clin. North Am. 18 (1), 75–84. viii. doi:10.1016/j.ohc.2004.07.002
Singh, A. D., Turell, M. E., and Topham, A. K. (2011). Uveal melanoma: Trends in incidence, treatment, and survival. Ophthalmology 118 (9), 1881–1885. doi:10.1016/j.ophtha.2011.01.040
Smit, K. N., Jager, M. J., de Klein, A., and Kiliҫ, E. (2020). Uveal melanoma: Towards a molecular understanding. Prog. Retin Eye Res. 75, 100800. doi:10.1016/j.preteyeres.2019.100800
Song, B., Wu, P., Liang, Z., Wang, J., Zheng, Y., Wang, Y., et al. (2022a). A novel necroptosis-related gene signature in skin cutaneous melanoma prognosis and tumor microenvironment. Front. Genet. 13, 917007. doi:10.3389/fgene.2022.917007
Song, B., Chi, H., Peng, G., Song, Y., Cui, Z., Zhu, Y., et al. (2022b). Characterization of coagulation-related gene signature to predict prognosis and tumor immune microenvironment in skin cutaneous melanoma. Front. Oncol. 12, 975255. doi:10.3389/fonc.2022.975255
Song, G., Peng, G., Zhang, J., Song, B., Yang, J., Xie, X., et al. (2023). Uncovering the potential role of oxidative stress in the development of periodontitis and establishing a stable diagnostic model via combining single-cell and machine learning analysis. Front. Immunol. 14, 1181467. doi:10.3389/fimmu.2023.1181467
Straatsma, B. R., Diener-West, M., Caldwell, R., and Engstrom, R. E.Collaborative Ocular Melanoma Study Group* (2018). Mortality after deferral of treatment or no treatment for choroidal melanoma. Indian J. Ophthalmol. 66 (10), 1395–1400. doi:10.4103/ijo.IJO_1499_18
Su, K., Guo, L., Ma, W., Wang, J., Xie, Y., Rao, M., et al. (2022). PD-1 inhibitors plus anti-angiogenic therapy with or without intensity-modulated radiotherapy for advanced hepatocellular carcinoma: A propensity score matching study. Front. Immunol. 13, 972503. doi:10.3389/fimmu.2022.972503
Sun, Y., Huang, J., and Yang, Z. (2015). The roles of ADAMTS in angiogenesis and cancer. Tumour Biol. 36 (6), 4039–4051. doi:10.1007/s13277-015-3461-8
Tabernero, J. (2007). The role of VEGF and EGFR inhibition: Implications for combining anti-VEGF and anti-EGFR agents. Mol. Cancer Res. 5 (3), 203–220. doi:10.1158/1541-7786.MCR-06-0404
Timpl, R. (1989). Structure and biological activity of basement membrane proteins. Eur. J. Biochem. 180 (3), 487–502. doi:10.1111/j.1432-1033.1989.tb14673.x
Triozzi, P. L., and Singh, A. D. (2014). Adjuvant therapy of uveal melanoma: Current status. Ocul. Oncol. Pathol. 1 (1), 54–62. doi:10.1159/000367715
Tsilibary, E. C. (2003). Microvascular basement membranes in diabetes mellitus. J. Pathol. 200 (4), 537–546. doi:10.1002/path.1439
Valastyan, S., and Weinberg, R. A. (2011). Tumor metastasis: Molecular insights and evolving paradigms. Cell. 147 (2), 275–292. doi:10.1016/j.cell.2011.09.024
Wang, X., Ye, F., Xiong, M., Xiu, B., Chi, W., Zhang, Q., et al. (2022). Cross-talk of four types of RNA modification proteins with adenosine reveals the landscape of multivariate prognostic patterns in breast cancer. Front. Genet. 13, 943378. doi:10.3389/fgene.2022.943378
Whitehead, M. J., McCanney, G. A., Willison, H. J., and Barnett, S. C. (2019). MyelinJ: An ImageJ macro for high throughput analysis of myelinating cultures. Bioinformatics 35 (21), 4528–4530. doi:10.1093/bioinformatics/btz403
Wu, G., Li, J., Xu, Y., Che, X., Chen, F., and Wang, Q. (2021). A new survival model based on ADAMTSs for prognostic prediction in clear cell renal cell carcinoma. J. Oncol. 2021, 2606213. doi:10.1155/2021/2606213
Xiong, J., Chi, H., Yang, G., Zhao, S., Zhang, J., Tran, L. J., et al. (2023). Revolutionizing anti-tumor therapy: Unleashing the potential of B cell-derived exosomes. Front. Immunol. 14, 1188760. doi:10.3389/fimmu.2023.1188760
Xu, L., Deng, C., Pang, B., Zhang, X., Liu, W., Liao, G., et al. (2018). Tip: A web server for resolving tumor immunophenotype profiling. Cancer Res. 78 (23), 6575–6580. doi:10.1158/0008-5472.CAN-18-0689
Yang, B., Fan, Y., Liang, R., Wu, Y., and Gu, A. (2023). Identification of a prognostic six-immune-gene signature and a nomogram model for uveal melanoma. BMC Ophthalmol. 23 (1), 2. doi:10.1186/s12886-022-02723-1
Yu, Y. H., Kuo, H. K., and Chang, K. W. (2008). The evolving transcriptome of head and neck squamous cell carcinoma: A systematic review. PLoS One 3 (9), e3215. doi:10.1371/journal.pone.0003215
Zhang, Z., Hao, R., Guo, Q., Zhang, S., and Wang, X. (2021). TP53 mutation infers a poor prognosis and is correlated to immunocytes infiltration in breast cancer. Front. Cell. Dev. Biol. 9, 759154. doi:10.3389/fcell.2021.759154
Zhang, Z., Zhang, T., Zhou, L., and Guan, J. (2022). Identification of diagnostic genes and effective drugs associated with osteoporosis treatment by single-cell RNA-seq analysis and network Pharmacology. Mediat. Inflamm. 2022, 6830635. doi:10.1155/2022/6830635
Zhang, J., Peng, G., Chi, H., Yang, J., Xie, X., Song, G., et al. (2023). CD8 + T-cell marker genes reveal different immune subtypes of oral lichen planus by integrating single-cell RNA-seq and bulk RNA-sequencing. BMC Oral Health 23 (1), 464. doi:10.1186/s12903-023-03138-0
Zhao, S., Zhang, L., Ji, W., Shi, Y., Lai, G., Chi, H., et al. (2022a). Machine learning-based characterization of cuprotosis-related biomarkers and immune infiltration in Parkinson's disease. Front. Genet. 13, 1010361. doi:10.3389/fgene.2022.1010361
Zhao, S., Chi, H., Ji, W., He, Q., Lai, G., Peng, G., et al. (2022b). A bioinformatics-based analysis of an anoikis-related gene signature predicts the prognosis of patients with low-grade gliomas. Brain Sci. 12 (10), 1349. doi:10.3390/brainsci12101349
Zhao, Y., Wei, K., Chi, H., Xia, Z., and Li, X. (2022c). IL-7: A promising adjuvant ensuring effective T cell responses and memory in combination with cancer vaccines? Front. Immunol. 13, 1022808. doi:10.3389/fimmu.2022.1022808
Zheng, Z., Zhang, L., Tu, Z., Deng, Y., and Yin, X. (2021). An autophagy-related prognostic signature associated with immune microenvironment features of uveal melanoma. Biosci. Rep. 41 (3). doi:10.1042/BSR20203812
Zhou, T., Chen, W., Wu, Z., Cai, J., and Zhou, C. (2022). A newly defined basement membrane-related gene signature for the prognosis of clear-cell renal cell carcinoma. Front. Genet. 13, 994208. doi:10.3389/fgene.2022.994208
Keywords: uveal melanoma, basement membrane genes, machine learning, multi-omics, tumor heterogeneity, cancer immunotherapy
Citation: Li Y, Cai H, Yang J, Xie X, Pei S, Wu Y, Zhang J, Song G, Zhang J, Zhang Q, Chi H and Yang G (2023) Decoding tumor heterogeneity in uveal melanoma: basement membrane genes as novel biomarkers and therapeutic targets revealed by multi-omics approaches for cancer immunotherapy. Front. Pharmacol. 14:1264345. doi: 10.3389/fphar.2023.1264345
Received: 20 July 2023; Accepted: 15 September 2023;
Published: 26 September 2023.
Edited by:
Linhui Wang, Second Military Medical University, ChinaReviewed by:
Zhengrui Li, Shanghai Jiao Tong University, ChinaHaizhou Lou, Zhejiang University, China
Zhaomin Yao, Northeastern University, China
Copyright © 2023 Li, Cai, Yang, Xie, Pei, Wu, Zhang, Song, Zhang, Zhang, Chi and Yang. This is an open-access article distributed under the terms of the Creative Commons Attribution License (CC BY). The use, distribution or reproduction in other forums is permitted, provided the original author(s) and the copyright owner(s) are credited and that the original publication in this journal is cited, in accordance with accepted academic practice. No use, distribution or reproduction is permitted which does not comply with these terms.
*Correspondence: Qinhong Zhang, zhangqh0451@163.com; Hao Chi, Chihao7511@163.com; Guanhu Yang, guanhuyang@gmail.com
†These authors have contributed equally to this work