- 1First Clinical Medical College, Nanjing University of Chinese Medicine, Nanjing, China
- 2Taicang TCM Hospital Affiliated to Nanjing University of Chinese Medicine, Taicang, China
- 3Taicang Hospital of Traditional Chinese Medicine, Taicang, China
- 4Department of Respiratory and Critical Care Medicine, Jiangsu Province Hospital of Chinese Medicine, Affiliated Hospital of Nanjing University of Chinese Medicine, Nanjing, China
- 5School of Chinese Medicine School of Integrated Chinese and Western Medicine, Nanjing University of Chinese Medicine, Nanjing, China
Aim: Chaihu-jia-Longgu-Muli-tang (CLM) is derived from “Shang Han Lun” and is traditionally prescribed for treating depression. However, there is still a lack of evidence for its antidepressant effects, and the underlying mechanism is also unclear. This study aimed to assess clinical evidence on the efficacy of CLM in patients with depression using a meta-analysis and to explore its underlying antidepressant molecular mechanisms via network pharmacology.
Methods: Eight open databases were searched for randomized controlled trials (RCTs) comparing the effects of CLM alone or combined with serotonin-norepinephrine reuptake inhibitors (SNRIs) and selective serotonin reuptake inhibitors (SSRIs) in patients with depression, evaluating the total effective rate of the treatment group (CLM alone or combined with SSRIs/SNRIs) and the control group (SNRIs or SSRIs), and comparing changes in depression scale, anxiety scale, sleep scale, inflammation indicators and adverse effects. Subsequently, the active ingredients and target genes of CLM were screened through six databases. Then Gene Ontology (GO) and Kyoto Encyclopedia of Genes and Genomes (KEGG) analysis and protein-protein interaction (PPI) network and topology analysis were performed. Finally, Molecular docking was applied to evaluate the binding affinity between components and predicted targets.
Results: Twenty-four RCTs with a total of 2,382 patients were included. For the efficacy of antidepression and adverse effects, whether CLM alone or in combination with SSRIs/SNRIs, the treatment group has no inferior to that of the control group. Additionally, the intervention of CLM + SSRI significantly improved the symptoms of anxiety and insomnia, and reduced serum IL-6 and TNF-α levels. For network pharmacology, a total of 129 compounds and 416 intersection targets in CLM were retrieved. The interaction pathway between CLM and depression is mainly enriched in PI3K-Akt, JAK-STAT, and NF-κB signaling pathway, PIK3R1, MAPK3, and AKT1 may be the potential targets of Stigmasterol, β-stiosterol, coumestrol.
Conclusion: Compared to SSRIs/SNRIs alone, CLM is more effective and safe in treating depression. It not only significantly alleviates depressive mood, but improves symptoms such as anxiety and insomnia, with fewer side effects, especially in combination with SSRI. Its antidepressant mechanism may be correlated with the regulation of the PI3K/Akt signaling pathway and inhibiting inflammatory response.
1 Introduction
Depression is a debilitating neuropsychological disorder that impairs daily functioning and is characterized by a constant sense of melancholy, loss of interest, and intellectual disability. Globally, major depression disorder (MDD) and dysthymia are responsible for approximately 46.9 million disabilities annually, with over 800,000 suicidal deaths mostly accompanied by neurological and psychiatric disorders such as MDD (Li et al., 2022a; Moitra et al., 2022). The number of patients with depression reached 542 million in 2015, with an 18.4% global increase between 2005 and 2015 (Disease and Prevalence, 2016). Due to the effects of coronavirus disease 2019, the number of patients with depression worldwide increased by 27.6% and depression is estimated to become the leading cause of death by 2030 (COVID-19 Mental Disorders Collaborators, 2021). Therefore, discovering efficient and safe treatment modalities is currently highly needed. There are three main interventions for treating depression that are currently used: 1) pharmaceutical antidepressants, such as SNRIs and SSRIs, among others; 2) research-based psychotherapy, such as cognitive behavioral therapy and interpersonal psychotherapy; and 3) physical therapy, such as vagus nerve stimulation or repeated transcranial magnetic stimulation. However, about one-third of patients still do not achieve remission after four consecutive antidepressant trials, and at least 50%–80% of patients still exist recurrence, with a progressive increase in severity and frequency, this mainly attributed to increased resistance in depression (Borbely et al., 2022). Furthermore, patients’ resistance to antidepressants is often accompanied by high levels of serum inflammatory factors such as interleukin-6 (IL-6) and tumor necrosis factor-α (TNF-α) (Beurel et al., 2020). Heightened inflammation can also exacerbate mood disorders such as anxiety and anhedonia, as well as somatic symptoms such as insomnia and gastrointestinal disorders (Kiecolt-Glaser et al., 2015). Thus, it is still necessary to find or develop antidepressant treatments with high-efficiency and fewer side effects.
Traditional Chinese Medicine (TCM) operates on the understanding that the human body is an organic whole, and the viscera coordinate with each other in function and influence each other in pathology (Xiang-yu et al., 2021). The pathogenesis of depression as viewed in TCM is a disorder of the Shao Yang pivot and dysfunction of Yin and Yang. Shao Yang is the pivot for the rise and fall of the Qi movement; Shao Yang dysfunction leads to depression and insomnia. When the Shao Yang channel restrains the Qi, the body will feel cold and physically heavy and patients will show appetite loss (Hanying et al., 2022). CLM is a TCM prescription derived from “Shang Han Lun” that is prescribed to treat mental diseases caused by the imbalance of the pivot of Shao Yang and the loss of Yin and Yang. It has the effects of mediating the Shao Yang pivot and tranquilizing the mind (Wan et al., 2021). Numerous studies have shown that CLM contributes to improvements in dementia, insomnia, anxiety, and depression (Yuanwen et al., 2022). Although the efficacy and safety of CLM for the treatment of depression have been evaluated in many studies, determining a reliable basis for evidence-based medicine is difficult due to deviations in the included studies, literature updates, and inconsistencies in patient grouping and comorbidities (Li et al., 2018; Wan et al., 2021). The potential mechanism behind CLM’s antidepressant effect may be related to the inhibition of N-methyl-D-aspartate (NMDA) receptors to increase hippocampal synaptic plasticity, positively regulation of neuronal apoptosis, and inhibition of neuroinflammation (Liu et al., 2010; Wang et al., 2018; Lizhi et al., 2021). However, owing to the intricacy of the compound constituents, particularly the molecular target mechanisms of its active substances, the mechanisms associated with its antidepressant effect remain unclear. As a novel discipline, network pharmacology can effectively and systematically explore correlations between traditional Chinese medicine substances, targets, and diseases by constructing a variety of network models comprehensively using multiple platforms and technologies to help explore potential mechanisms (Chen et al., 2018).
Based on the above background, CLM has a wide range of antidepressant efficacy, but with still a lack of clinical evidence, and its antidepressant mechanism is still unclear due to the complexity of TCM compound ingredients. Therefore, this study aimed to assess clinical evidence on the efficacy of CLM in patients with depression using a meta-analysis and to explore its underlying antidepressant molecular mechanisms via network pharmacology.
2 Materials and methods
2.1 Meta-analysis
2.1.1 Data sources and searches
Clinical trials were searched in the PubMed, Web of Science, MEDLINE, Cochrane Library, China National Knowledge Internet, VIP, Wanfang, and SinoMed databases from the inception of each database until December 2022. This meta-analysis complied with the Preferred Reporting Items for Systematic Reviews and Meta-Analyses (Page et al., 2021) (protocol shown in Figure 1). The search strategy is shown in Supplementary Table S1.
2.1.2 Inclusion and exclusion criteria
Due to the limited exact treatment methods for depression at present, physical therapy has the disadvantage of large side effects and poor compliance (e.g., repeated transcranial magnetic stimulation), and uneven efficacy (e.g., Acupuncture, massage, music, etc.). Although mainstream antidepressants (e.g., SSRIs and SNRIs) have exact therapeutic effects and are widely accepted, there are still some limitations we mentioned above, so we have made the following provisions in the inclusion and exclusion criteria.
The inclusion criteria were as follows: 1) randomized controlled trials retrieval was limited to published in Chinese and English; 2) studies including participants clearly diagnosed with major depression disorder according to the Chinese Classification of Mental Disorders Third Edition (CCMD-3) or the Diagnostic and Statistical Manual of Mental Disorders (DSM-IV) (Regier et al., 2013); 3) Studies including at least one depression related outcome indicator such as Hamilton Anxiety Rating Scale (HAMD) or Self-rating Depression Scale (SDS); 4)the treatment group received CLM or a combination of CLM and SSRIs or SNRIs, the control group treated SSRIs/SNRIs alone. In addition, the intervention of CLM was added or subtracted according to syndrome differentiation, and the botanical drugs mainly including Radix Bupleuri [Chai Hu], Fossilia Ossia Mastodi [Longgu], Radix Scutellariae [Huang Qin], Zingiber rhizoma [Shengjiang], Ginseng radix [Renshen], Cinnamomi cortex [Guizhi], Hoelen [Fuling], Pinelliae Tuber [Banxia], Radix et Rhizoma Rhei [Dahuang], Ostreae Testa [Muli], and Zizyphi fructus [Dazao]. The composition of CLM is shown in Supplementary Table S2.
The exclusion criteria were as follows: 1) incomplete data; 2) inclusion of drug-induced secondary depression, somatic or psychiatric disease-induced secondary depression, postpartum depression, or menopausal depression; 3) other comorbid conditions, 4) interventions combining other traditional Chinese medicine formulas or physical therapy (e.g., acupuncture, massage, music, etc.).
2.1.3 Data extraction and quality assessment
The search terms and their common synonyms in each database were as follows: “Depression OR Depressive” AND “chaihu jia longgu muli decoction OR CLM.” All retrieved studies were imported into Endnote20 software for screening and eliminating duplicates according to abstracts. Two researchers (YS. and YY.) extracted the information trial characteristics (study, sample size, gender, average age, interventions, duration, average course, and outcomes (Total Effective Rate, HAMD, Hamilton Anxiety Rating Scale (HAMA), Self-rating Anxiety Scale (SAS), SDS, Pittsburgh Sleep Quality Index (PSQI), Adverse effects and serum inflammatory factors: TNF-α and IL-6). Two assessors (JW and DZ.) independently evaluated study quality and risk of bias and extracted data using the Cochrane Collaboration Assessment Tool. Discrepancies between the assessors were resolved by a third assessor.
2.1.4 Data analysis
STATA software (v16.0) to analyze this meta-analysis. For continuous outcomes (change in HAMD, SDS, HAMA, SAS, TNF-α, IL-6) and dichotomous outcomes (Total Effective Rate and Adverse Effects), the standardized mean difference (SMD) and risk ratios (RR) were represented, respectively. The 95% confidence interval (CI) was used to analyze the effects of secondary variables. When heterogeneity was high (I2 > 50% or p < 0.05), a random effects model was used; otherwise, a fixed effects model was used. The subgroup analysis was based on the type of treatment group which included CLM + SSRI (CLM + SSRI vs. SSRI), CLM + SNRI (CLM + SNRI vs SNRI), and CLM (CLM vs. SSRI). Egger’s test and funnel plots were used to assess publication bias.
2.2 Network pharmacology
2.2.1 Screening for compounds and corresponding targets of CLM
According to the 11 Chinese herbs included in CLM as recorded in the “Shang Han Lun”, the compounds and corresponding targets of each drug were screened from three databases TCMSP (https://tcmspw.com/tcmsp.php) (Zeng et al., 2022), the HERB database (http://herb.ac.cn/) (Fang et al., 2021), and the BATMAN-TCM database (http://bionet.ncpsb.org/batman-tcm/; score ≥20, p-value ≤0.05) (Gao et al., 2021). Subsequently, all screened ingredients were imported into the TCMSP for normalization according to ADME (absorption, distribution, metabolism, excretion) criteria. In addition, oral bioavailability ≥30% and drug-likeness ≥0.18 as the most commonly used pharmacokinetic parameters to measure drug properties.
2.2.2 Target prediction for depression
The keyword “depression” was searched to obtain potential targets related to depression from the GeneCards (Stelzer et al., 2016) and DisGeNET (Pinero et al., 2017) databases. To forecast CLM’s therapeutic targets for treating depression, the common targets of compounds and depression were sorted out, and a Venn diagram was obtained using the Venn 2.1.0 platform (Heberle et al., 2015). The intersection targets were entered into Cytoscape software (v 3.8.2) to construct Herb-Compound-Target (H-C-T) networks.
2.2.3 Gene ontology and kyoto encyclopedia of genes and genomes enrichment analyses
For KEGG and GO enrichment analyses, we uploaded intersection targets into the Metascape platform (http://metascape.org/Homo sapiens, p < 0.05, and p-values were corrected by the Benjamini–Hochberg procedure) (Zhou et al., 2019).
2.2.4 Construction of protein-protein interaction network and topological analysis
To further evaluate the core of regulatory targets in the treatment of depression and discover potential connections, intersection targets were uploaded to the STRING database (H. sapiens, minimum required interaction score: 0.9) to construct a PPI network (Szklarczyk et al., 2019). Subsequently, to evaluate the topological characteristics of the nodes, the three parameters of “betweenness centrality” (BC), “closeness centrality” (CC), and “degree centrality” (DC) were computed.
2.2.5 Molecular docking verification
To assess the binding affinity of components with predicted targets, the active components of CLM were docked with core targets. The selection of active ingredients and core targets were as follows: 1) the degree values of H-C-T and PPI; 2) the correlation of enriched signal pathways according to GO and KEGG analysis. First, the core targets’ three-dimensional structure “PDB” files were retrieved from the PDB database (O'Boyle et al., 2011). Autodock Tools (v1.5.7) was used to remove the water molecules, original ligands, add hydrogens, calculate Gasteiger partial charges, and set atom types. The “SDF” format of the CLM bioactive component was obtained from the PubChem database (https://www.rcsb.org/), and the torsion angles in the ligand were identified, the solvent model was added, and the Kollman atomic charges were assigned to the protein using Autogrid4 and Autodock4. The “Local Search Parameters” algorithm was used for the docking operation. Finally, we constructed the docking interaction pattern diagram and displayed the docking findings via PyMoL (v2.4.0).
3 Results of meta-analysis
3.1 Study screening
A total of 1280 relevant original studies were retrieved from eight databases including PubMed (14 studies), MEDLINE (17), Web of Science (15), Cochrane Library (6), China National Knowledge Internet (319), VIP (185), Wan Fang (406), and Sino Med (318). There were 589 duplicates that were eliminated. Primary screening was then performed on the remaining 691 articles, and 661 trials were removed based on the abstracts and titles. Out of the remaining 30 articles, 24 items were included in this study. (Figure 2; Table 1).
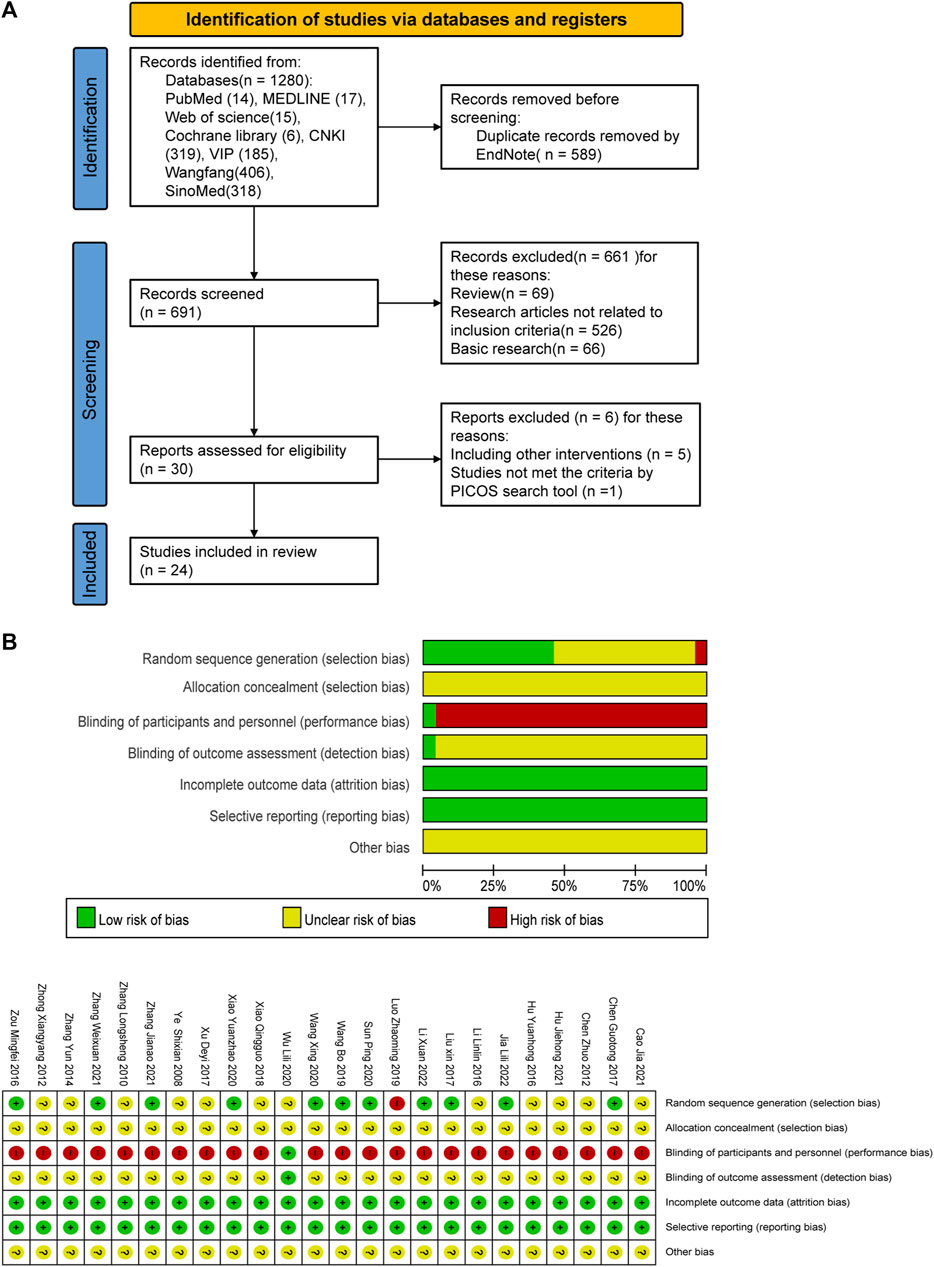
FIGURE 2. PRISMA flow diagram and risk-of-bias assessment; (A) literature screening process; (B) risk-of-bias summary.
3.2 Characteristics and quality of study
Twenty-four RCTs with a total of 2,382 patients with depression were included (1195 cases in the treatment group, 1187 cases in the control group), involving five interventions, mainly including (SSRI, SNRI, CLM, CLM + SSRI, CLM + SNRI). According to the intervention in the treatment group, 13 trials on CLM + SSRI included 1337 patients, 3 trials on CLM + SNRI included 280 patients, and 8 trials on CLM alone included 765 patients. The treatment duration varies from 2 to 12 weeks.
A total of 21 trials discussed the total effective rate, of which 8 trials were for CLM alone, 10 trials were for CLM + SSRI, and 3 trials were for CLM + SNRI; 19 trials reported HAMD scores, among which 7 trials were for CLM alone, 10 trials were for CLM + SSRI, and 2 trials were for CLM + SNRI; 11 trials reported SDS scores, of which 6 trials were for CLM alone, 4 trials were for CLM + SSRI, and 1 trial was for CLM + SNRI; 2 trials reported HAMA scores, of which was for CLM and CLM + SNRI respectively; 5 trials reported SAS scores, among which 1 trial was for CLM alone, 3 trials were for CLM + SSRI, and 1 trial was for CLM + SNRI; 3 trials reported PSQI scores, all of included were for CLM + SSRI; 2 trials detected the serum levels of IL-6 and TNF-α for CLM + SSRI; and 12 trials assessed adverse effects after treatment, among which 2 trials were for CLM alone, 9 trials were for CLM + SSRI, and 1 trial were for CLM + SNRI. Additionally, Table 1 and Figure 2 show the basic characteristics of the studies and results of the risk-of-bias assessment.
3.3 Total effective rate
21 studies discussed the effective rate of CLM for treating depression. According to heterogeneity testing (p = 0.062, I2 = 34.5%), the fixed effects model was applied. The results indicated that the effective rate of the treatment group was significantly higher than that of the control group (RR = 1.17%, 95% CI [1.13, 1.22]; Figure 3A). For the subgroup analysis, the anti-depressive effect on CLM + SSRI, CLM, and CLM + SNRI is significantly better than that of SSRI or SNRI alone (RR = 1.23%, 95% CI [1.16, 1.30], p = 0.339, I2 = 11.3%; RR = 1.10%, 95% CI [1.04, 1.17], p = 0.241, I2 = 23.6%; RR = 1.17%, 95% CI [1.06, 1.29], p = 0.470, I2 = 0.0%; Figure 3A). Moreover, the funnel plot symmetry indicated no publication bias (Egger’s test: p = 0.280, Figure 3B).
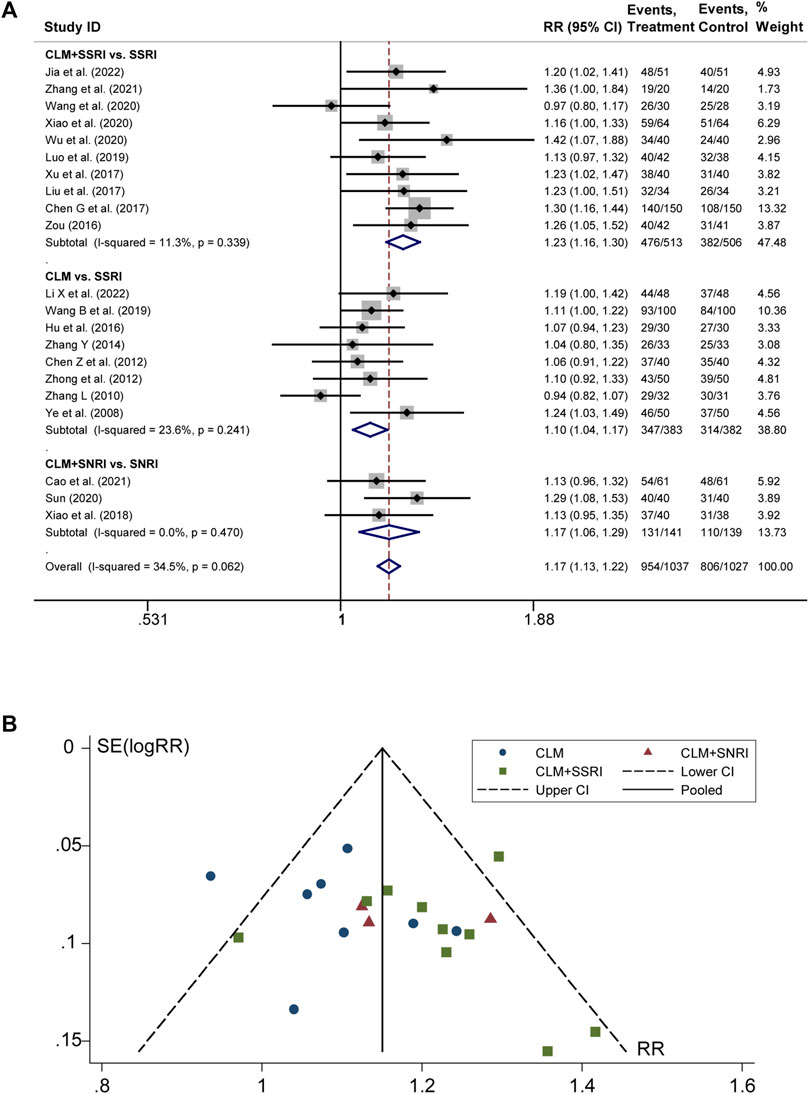
FIGURE 3. Comparative forest plots of total effective rate; (A) forest plot showing the total effective rate of CLM alone or anti-depressant drug therapy on depression; Treatment: CLM alone or combined with pharmaceutical anti-depressants; Control: pharmaceutical anti-depressants; (B) funnel plot of total effective rates.
3.4 HAMD and self-rating depression scale scores
19 trials reported the post-treatment HAMD scores. According to heterogeneity testing (p = 0.000, I2 = 94.7%), the random effects model was applied. Results indicated that the CLM treatment group showed more reduction in HAMD scores than the control group (SMD = −1.40%, 95% CI [−1.88, −0.92], Figure 4A). For the subgroup analysis, the CLM + SSRI, CLM, and CLM + SNRI showed significant reduction in HAMD scores comparing SSRI or SNRI alone (RR = −1.94%, 95% CI [−2.68, −1.19], p = 0.000, I2 = 95.0%; RR = −0.54%, 95% CI [−0.101, −0.08], p = 0.000, I2 = 88.1%; RR = −1.93%, 95% CI [−4.71, 0.86], p = 0.000, I2 = 97.8%; Figure 4A).
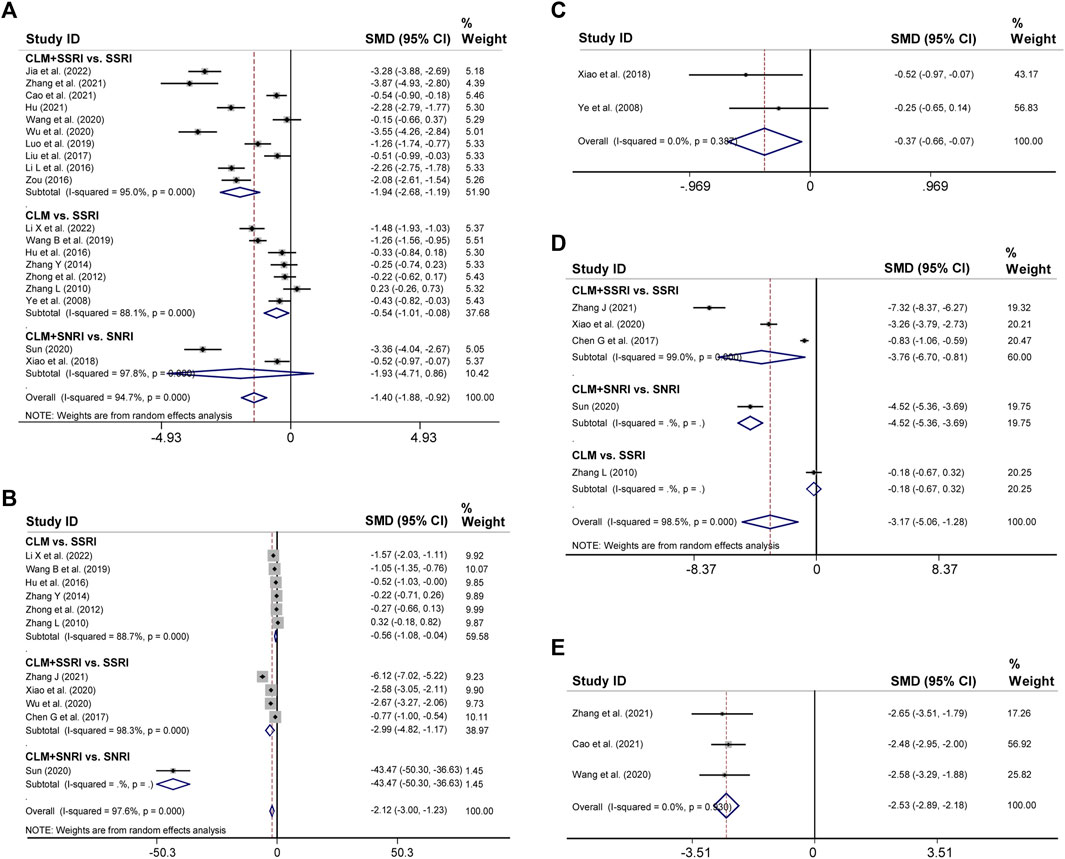
FIGURE 4. Comparative forest plots of HAMD, SDS, HAMA, SAS, and PSQI scores; (A) HAMD scores; (B) SDS scores; (C) HAMA scores; (D) SAS scores; (E) PSQI scores.
Regarding SDS, 11 trials had high heterogeneity (p = 0.000, I2 = 97.6%), and the random effects model was adopted. The results showed that the treatment group significantly improved negative emotions compared to the control group (SMD = −2.12%, 95% CI [−3, −1.23], Figure 4B). For the subgroup analysis, the CLM, CLM + SSRI, and CLM + SNRI showed significant reduction in SDS scores comparing SSRI or SNRI alone (RR = −0.56%, 95% CI [−1.08, −0.04], p = 0.000, I2 = 88.7%; RR = −2.99%, 95% CI [−4.82, −1.17], p = 0.000, I2 = 98.3%; RR = −2.12, 95% CI [−3.00, −1.23], p = Not applicable, I2 = Not applicable; Figure 4B)
3.5 HAMA, self-rating anxiety scale, pittsburgh sleep quality index scores
Two trials reported HAMA scores for CLM and CLM + SNRI respectively. According to heterogeneity testing (p = 0.387, I2 = 0.0%), the fixed effects model was applied. The HAMA scores of the group of patients receiving CLM or combined with antidepressant drugs were significantly lower than those of the control group (SMD = −0.37%, 95% CI [−0.66, −0.07], Figure 4C).
11 trials reported SAS scores. According to heterogeneity testing (p = 0.000, I2 = 98.5%), the random effects model was performed. The SAS scores of the treatment group were significantly lower than those of the control group (SMD = −3.17%, 95% CI [−5.06, −1.28], Figure 4D). For the subgroup analysis, the CLM + SSRI, CLM + SNRI, and CLM showed marked reduction in SAS scores comparing SSRI or SNRI alone (RR = −3.76%, 95% CI [−6.70, −0.81], p = 0.000, I2 = 99.0%; RR = −4.52%, 95% CI [−5.36, −3.69], p = Not applicable, I2 = Not applicable; RR = −0.18%, 95% CI [−0.67, −0.32], p = Not applicable, I2 = Not applicable; Figure 4B)
Three trials reported PSQI scores, all of included were for CLM + SSRI. According to heterogeneity testing (p = 0.930, I2 = 0.0%), the fixed effects model was applied. The PSQI scores of the treatment group were significantly lower than those of the control group (SMD = −2.53, 95% CI [−2.89, −2.18], Figure 4E).
3.6 Inflammation index
There were 2 studies both for CLM + SSRI that assessed serum levels of TNF-α and IL-6. For TNF-α, according to heterogeneity testing (p = 0.002, I2 = 89.6%), the random effects model was applied, which in the treatment group were significantly lower than those in the control group (SMD = −1.82%, 95% CI [−3.03, −0.61], Figure 5A). For IL-6, according to heterogeneity testing (p = 0.601, I2 = 0.0%), the fixed effects model was applied. Compared with the control group, the treatment group showed significantly lower levels (SMD = −1.19%, 95% CI [−1.54, −0.84], Figure 5B).
3.7 Adverse effects
There were 12 trials that assessed adverse occurrences in the 24 included studies, covering neurological symptoms such as dizziness, headache, sleep disorders, hyperactivity, blurred vision, and fatigue, as well as gastrointestinal symptoms such as dry mouth, diarrhea, anorexia, nausea, vomiting, and constipation. The most common adverse reactions were dizziness, blurred vision, dry mouth, anorexia, and vomiting. According to heterogeneity testing (p = 0.250, I2 = 13.2%), the fixed effects model was applied. The treatment group showed better safety in treating depression than the control group (RR = 0.36%, 95% CI [0.23, 0.54], Figure 6). For the subgroup analysis, CLM + SSRI and CLM alone showed fewer adverse effects compared the SSRI alone (RR = 0.36%, 95% CI [0.21, 0.59], Figure 6), however, there was no statistical significance between CLM + SNRI and SNRI alone.
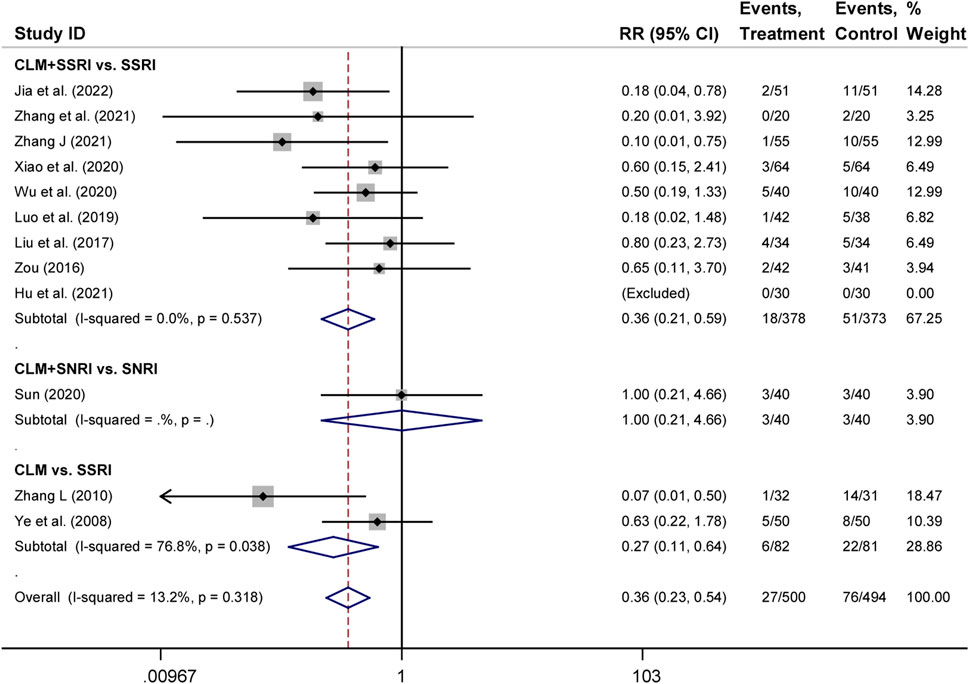
FIGURE 6. Comparative forest plots of adverse effects; Treament: CLM alone or combined with pharmaceutical anti-depressants; Control: pharmaceutical anti-depressants.
4 Results of network pharmacology analysis
4.1 Active compounds and treatment targets of CLM
We obtained 129 active compounds from the four databases, including Radix Bupleuri (16 compounds), Radix Scutellariae (29 compounds), Pinelliae Tuber (10 compounds), Ginseng radix (35 compounds), Hoelen (12 compounds), Zingiber rhizoma (5 compounds), Zizyphi fructus (25 compounds), Radix et Rhizoma Rhei (7 compounds), and Cinnamomi cortex (7 compounds). The primary active components of CLM are listed in Supplementary Table S3.
There were 2356 targets screened from the 129 active compounds CLM using the Swiss, TCMSP, HERB, and BATMAN-TCM databases. In addition, 1490 depression-related targets were identified using the DisGeNET and GeneCards databases. The intersection of drug targets and depression targets resulted in a total of 416 CLM treatment depression targets, as shown in the Venn diagram (Figure 7A).
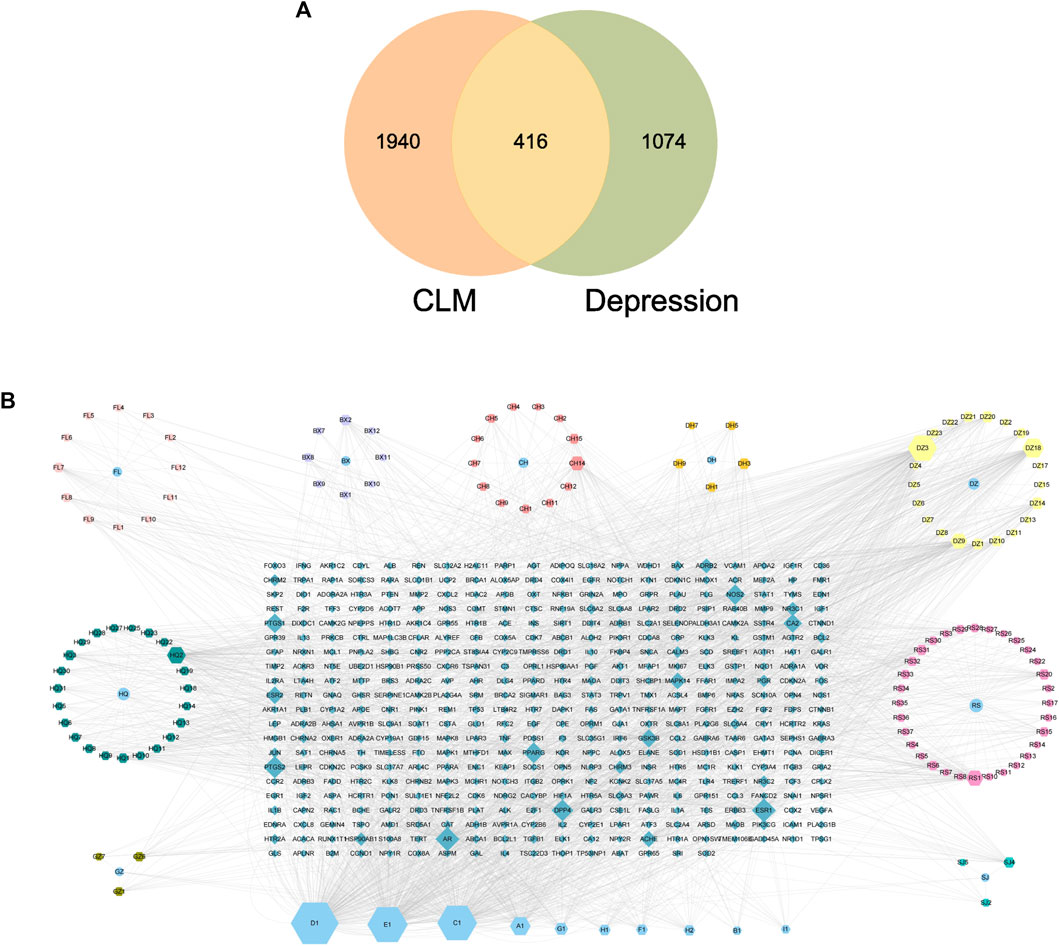
FIGURE 7. Venn diagram and H-C-T network; (A) Venn diagram of the active ingredients of CLM and potential treatment targets; 2356 predicted targets of CLM, 1490 therapeutic targets for depression, and 416 targets intersected; (B) H-C-T network of CLM; BX: Ban Xia, CH: Chai Hu, DZ: Da Zao, FL: Fu Ling, SJ: Sheng Jiang, GZ: Gui Zhi, DH: Da Huang, HQ: Sheng Jiang, RS: Ren Shen; A–H: Common components of different drugs.
4.2 The construction of the H-C-T network
The H-C-T network included 555 nodes and 2480 edges and contained nine herbs, 130 compounds, and 416 genes (Figure 7B). Larger nodes have higher statistical significance. The top five compounds, as determined by degree analysis, were MOL000449 (stigmasterol), MOL000098 (quercetin), MOL000358 (β-stigmasterol), MOL012976 (coumestrol), and MOL000627 (stepholidine), with respective degrees of 245°, 199°, 196°, 130°, and 85°. More details are given in Table 2.
4.3 Gene ontology and kyoto encyclopedia of genes and genomes enrichment analyze
For GO enrichment analysis, a total of 3516 items were selected, and the top 20 significant terms in the BP, CC, and MF categories are shown in Figure 8A. The intersected target proteins in the BP category mainly included responses to hormones, behavior, positive regulation of cell death, inflammatory response, and synaptic signaling. The CC category mainly included dendrite, cell body, membrane raft, perinuclear region of cytoplasm, and side of membrane. The MF category mainly included kinase binding, transcription factor binding, protein domain-specific binding, signaling receptor regulator activity, and neurotransmitter receptor activity.
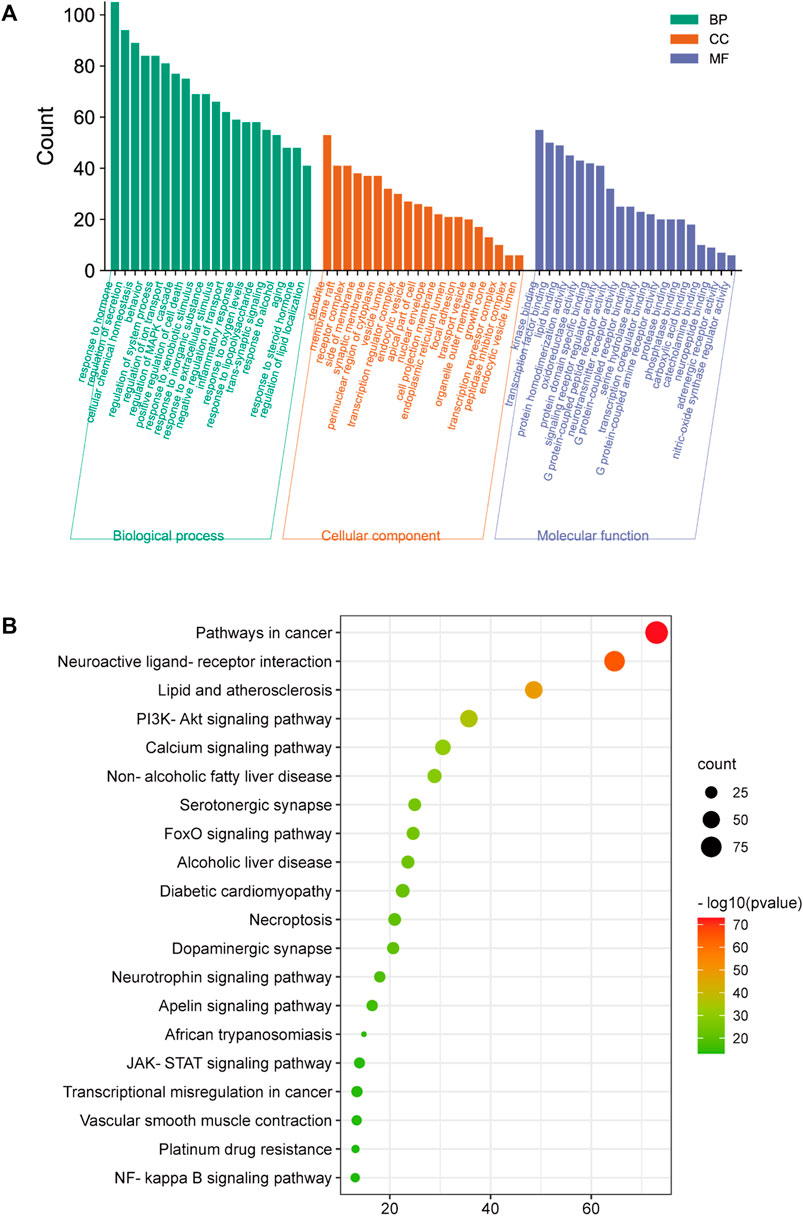
FIGURE 8. GO and KEGG analysis; (A) BP, MF, and CC categories in GO analysis of 416 intersection targets; (B) top 20 signaling pathways in KEGG enrichment analysis of 416 intersected targets.
For KEGG enrichment analysis, the top 20 items were selected for visualization according to the criteria above (Figure 8B). The results mainly involved neuroactive ligand-receptor interactions, the PI3K-Akt signaling pathway, serotonergic synapses, the JAK-STAT signaling pathway, and the NF-κB signaling pathway.
4.4 Protein-protein interaction network analysis
In the PPI network analysis, 416 predicted targets were submitted to the STRING platform, and interactions with high confidence (>0.9) were chosen. Isolated nodes were removed, and the remaining targets were visualized in Cytoscape 3.8.2. The PPI relationship network, which consisted of 312 nodes and 1321 edges, is shown in Figure 9A. In addition, to further screen for key targets for CLM in the treatment of depression, we conducted topology analysis based on BC, CC, and DC. Ultimately, 28 core targets were obtained and ranked by degree values (Figures 9B–D; Table 3). The top seven core targets were MAPK3, JUN, TP53, MAPK1, AKT1, STAT3, and PIK3R1.
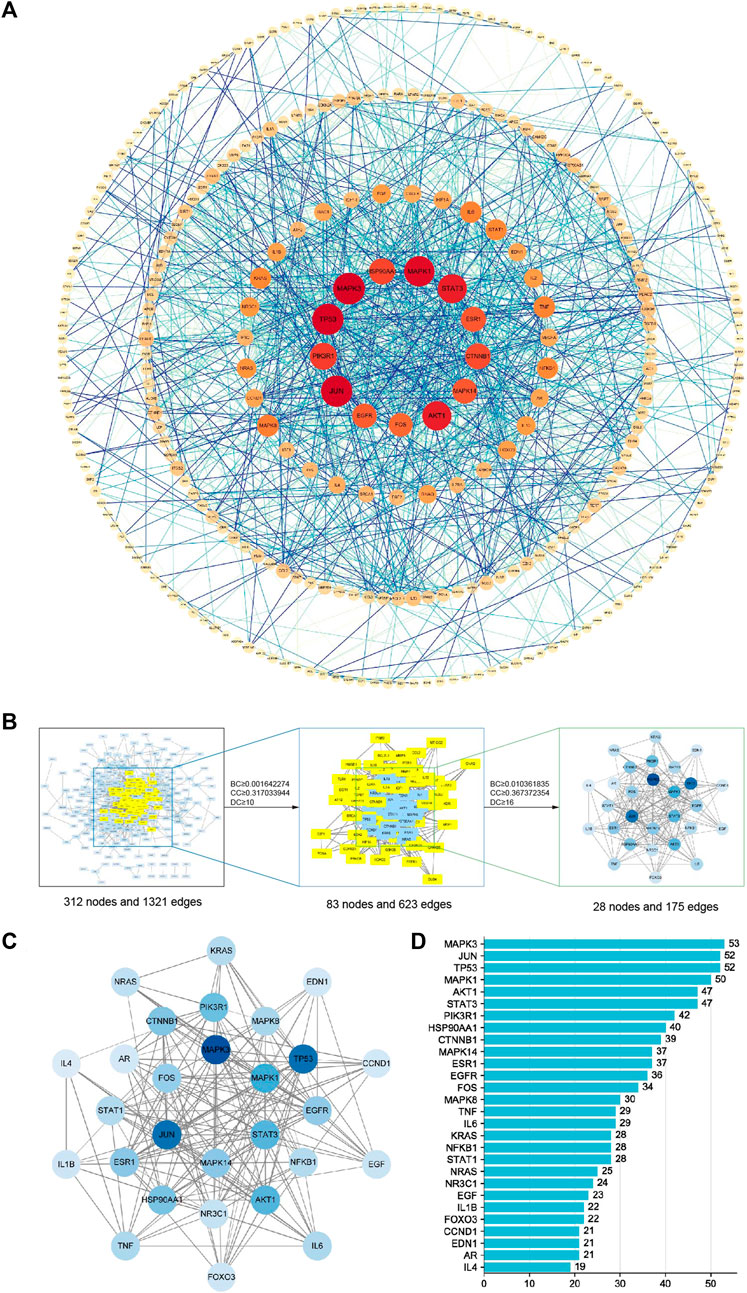
FIGURE 9. Interaction network diagram of 416 predicted targets and topology analysis; (A) PPI network of 416 predicted targets; (B) topology analysis; BC: betweenness centrality; CC: closeness centrality; DC: degree centrality; (C) network of the top 28 core proteins; (D) top 28 targets ranked by degree values.
4.5 Molecular docking
Based on the above results in H-C-T, GO, and PPI network analysis, molecular docking analysis was performed between the top five compounds of CLM and the top seven key targets. The docking visualizations and scores are shown in Figures 10A,B. The stronger the binding force between the compound and the protein, the lower the docking score (the higher the negative number). When the docking score is ≤ −5.0 kJ/mol, the exertion of a strong binding effect is suggested. The results showed that 35 pairs of active compounds and ligands were relatively stable. PIK3R1 (PDB: 2IUG), MAPK3 (PDB: 4QTB), and AKT1 (PDB: 1UNP) might be potential therapeutic targets for depression using CLM, among which stigmasterol (MOL000449) had the highest binding energy with PIK3R1.
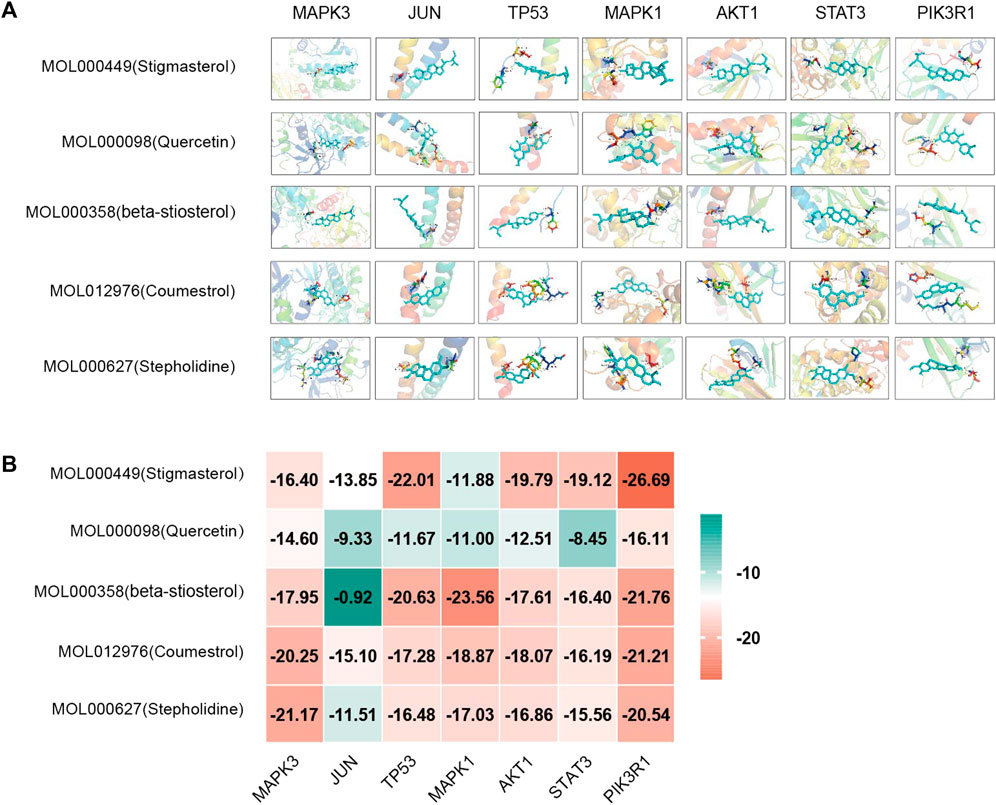
FIGURE 10. Molecular docking analysis of main compounds binding to key targets; (A) molecular docking models of main chemical components; (B) heat map showing values of docking affinity.
5 Discussion
At present, the pathological mechanisms of depression mainly involve the monoamine hypothesis, hypothalamus–pituitary–adrenal axis hyperactivity, neuroplasticity and neurogenesis, structural and functional brain changes, and inflammation (Malhi and Mann, 2018). However, due to the unclear pathophysiological mechanisms of depression, one-third of patients face treatment resistance or adverse reactions to common treatments, and the treatment of depression still faces challenges (Dodd et al., 2021). CLM is derived from “Shang Han Lun” and has a significant effect on depression. However, due to the complexity of its components, the mechanism of action of this prescription has not been fully clarified. The results of present study indicated that the antidepressant efficacy of CLM, whether administered alone or in combination with antidepressants, was significantly higher than that of antidepressants alone. In addition, whether in CLM alone or CLM + SSRI/SNRI, the symptoms of depression, anxiety, and insomnia in patients with depression were significantly improved comparing antidepressants alone. CLM and CLM + SSRI also showed fewer adverse reactions than SSRI alone. Five compounds in CLM and seven of its key targets were shown to be related to antidepressant activity in the present analysis.
CLM has extensive neural activities in the treatment of depression, anxiety, insomnia, and neurocognitive disorders (Niitsu et al., 2013). A meta-analysis of CLM’s efficiency and safety in treating post-stroke depression revealed that, regardless of the length of the treatment period, CLM showed better efficacy than an antidepressant group. Additionally, in the group receiving CLM combined with pharmaceutical antidepressants, the incidence of adverse reactions such as abnormal blood or urine routine tests, abnormal liver function, sleeplessness, and digestive tract pain was dramatically decreased (Wan et al., 2021). In addition, a correlation has been shown between depression, anxiety, and insomnia; the association between insomnia and feelings of self-disgust was fully mediated by anxiety and depression (Ypsilanti et al., 2018). This means that a good treatment strategy for patients with depression seems to be relieving anxiety and insomnia while adjusting mood. In the present findings, compared with antidepressants, both CLM alone and combined with SSRI/SNRI markedly reduced the scores of HAMD and SDS. For anxiety and insomnia, we conducted a meta-analysis of HAMA, SAS, and PSQI scores, results indicated that the synergistic effect of CLM and SSRI was significantly better than that of SSRI alone. In addition, due to the limited literature on CLM alone and CLM + SNRI, the synergistic effect of CLM alone or with SNRI may need further exploration. However, for overall efficacy in anxiety, CLM alone or in combination is not inferior to antidepressants alone. In terms of adverse reactions, whether CLM alone or in combination with SSRI, the incidence of adverse reactions is significantly fewer than that of SSRI alone. It is worth noting that the incidence of adverse reactions in CLM combined with SNRI is the same as that of SNRI alone, which may be the reason for only including one trial. Therefore, CLM could not only improve the symptoms of depression, anxiety, and insomnia, but can also reduce the incidence of adverse responses caused by commonly used antidepressants, especially in combination with SSRI.
Depression and inflammation are intertwined; heightened inflammation exacerbates not only physical symptoms such as pain sensitivity, fatigue, and anhedonia but also psychological symptoms such as negative mood, loss of appetite, and feelings of inferiority (Kiecolt-Glaser et al., 2015). Levels of proinflammatory cytokines in peripheral blood were shown to be significantly increased in patients with major depressive disorder, such as IL-6, TNF-α and IL-1β (Howren et al., 2009; Dowlati et al., 2010; Liu et al., 2012). Moreover, nonsteroidal anti-inflammatory drugs could significantly reduce depressive symptoms compared to placebo treatment (Kohler et al., 2014). Therefore, anti-inflammatory treatments may be effective for depression. The present results indicated that CLM dramatically decreased the levels of TNF-α and IL-6 in the peripheral blood of patients with depression compared to antidepressant treatment groups.
In order to further investigate the aforementioned putative mechanisms of CLM’s anti-depressant effects, network pharmacology was conducted. We intersected 416 therapeutic targets from 1490 depression targets and 2356 targets of CLM compound components. According to the H-C-T network, the top five active ingredients were stigmasterol, quercetin, β-stiosterol, coumestrol, and stepholidine. Recent research has shown that oral and intraperitoneal treatment of stigmasterol and β-stiosterol significantly reduced immobility time in tail suspension and forced swimming tests in rat models of depression, and its antidepressant activity might be mediated by the glutamatergic systems (Zhao et al., 2016; Ghosh et al., 2022). Quercetin has been reported to inhibit inflammation and attenuate depression-like behavior by regulating PI3K/AKT/NF-κB and promoting mitophagy in a lipopolysaccharide-induced mouse model of depression (Han et al., 2021; Sun et al., 2021). Monoamine oxidase A inhibitors, as third-line antidepressants, are widely used in clinics, and coumestrol has been proven to be a selective and competitive monoamine oxidase A inhibitor (Seong et al., 2022). As a specific dopamine receptor D1 agonist, stepholidine could activate the PKA/mTOR pathway to upregulate the expression of synaptogenesis-related proteins and exert antidepressant effects (Zhang et al., 2017). The above research provides a pharmacological basis for the clinical efficacy of CLM in depression, although the effect of CLM on depression has been revealed directly or indirectly through the above ingredients, due to the complexity of the ingredients, the specific mechanisms have not been clarified. Therefore, we assessed the relevant pathways for CLM in the treatment of depression using KEGG and GO enrichment analyses.
Research has confirmed that inflammation induced by glial cells is an important mechanism leading to depression. Neuroglial cells can regulate neuron electrical activity and even death by adjusting synaptic plasticity and integrating and transmitting synaptic information (Perez-Catalan et al., 2021). This may explain the anti-inflammatory and antidepressant mechanism of CLM, where GO analysis showed that the antidepressant effect of CLM mainly plays a protective role by regulating the inflammatory response. In addition, at the level of CC and MF, CLM mainly plays a role in synaptic spines, synapses, membrane rafts, and nuclear envelope by combining with enzymes and neurotransmitters. The present KEGG analysis of CLM also pointed to neuroinflammation, which involves the PI3K/Akt, JAK-STAT, and NF-κB signaling pathways. The PI3K/Akt signaling pathway is involved in synaptic plasticity, learning and memory, and inflammation, which are important in the pathogenesis of depression (Matsuda et al., 2019). Enzymatic activity of PI3K and Akt was shown to be decreased in patients with depression, which may lead to neuron loss, decreased neuroplasticity, and dysregulation of neurotrophic factors (Karege et al., 2011). Evidence has also shown that activation of Akt signaling can alleviate stress-induced depressive behaviors in mice by inhibiting neuroinflammation and increasing neurotrophic factors (Xian et al., 2019). Accordingly, by activating Akt signaling, CLM was shown to reverse the abnormal expression of AMPA and NMDA receptors in the prefrontal cortex of depressed mice (Wang et al., 2018). The JAK-STAT and NF-κB signaling pathways are downstream cascade signals of the PI3K/Akt pathway and both play key roles in cell proliferation, differentiation, migration, and apoptosis. The involvement of the JAK/STAT pathway in synaptic plasticity has been confirmed to depend on the activation of NMDA receptors in the hippocampal CA1 region (Nicolas et al., 2012). Therefore, we speculate that the antidepressant effect of CLM may be related to the activation of PI3K/Akt and its downstream signaling pathways, inhibition of inflammatory storms, and protection of neurons.
Notably, the therapeutic efficacy of ketamine, an NMDA receptor antagonist, has highlighted the emerging data linking the regulation of NMDA to the etiology of depression. More significantly, increases in proinflammatory cytokines (such as IL-1, IL-6, and TNF-α) have been directly associated with JAK-STAT activation. Antidepressants may work by decreasing proinflammatory cytokines via modulation of the JAK/STAT pathway, and they may also improve somatic symptoms, anhedonia, and low energy levels (Malemud and Miller, 2008). The NF-κB signaling pathway contributes to the pathogenesis of neurodegenerative diseases, with the PI3K/Akt pathway as its major upstream component (Dong et al., 2016). Activation of the PI3K/Akt signaling pathway has been reported to significantly reduce the nuclear translocation of NF-κB and the activation of microglia, thus inhibiting neuronal apoptosis and inflammation (Zhu et al., 2018). Although CLM likely exerts anti-depressant effects via the PI3K/Akt, JAK/STAT, NF-κB, or apoptosis signaling pathways, it remains urgently necessary to clarify the therapeutic targets of CLM, which may contribute to the development of novel therapeutic strategies.
Next, according to the PPI network and molecular docking analyses, MAPK3, TP53, MAPK1, STAT3, and PI3KR1 were potential antidepressant targets of CLM. Similar to the above results from the network pharmacology analysis, stigmasterol showed high binding energy with PI3KR1, suggesting that CLM alleviates depression via modulation of the PI3K/Akt signaling pathway. In summary, the above evidence suggests that CLM is a safe and effective antidepressant prescription with multiple pathways and targets. Its mechanism is likely related to the regulation of neuronal synaptic plasticity, inflammation, and apoptosis.
6 Limitations
This study still exist limitations. The treatment time was not explored on anti-depression efficacy. Secondly, it is still shallow that the evidence for selecting serum IL-6 and TNF-α as inflammation indicators. On the one hand, it is limited by available studies, on the other hand, other indicators such as serum IL-1β and CRP also affect the resistance to antidepressants. Meanwhile, the increase in these indicators also indicates more severe disease course outcomes and more extensive somatization symptoms (Kiecolt-Glaser et al., 2015). Furthermore, the number of trials included in this study was insufficient to clearly state the specific efficacy of some subgroup analyses such as HAMA and SAS, so part results have to be roughly concluded by assessing the comprehensive efficacy of CLM alone or in combination with antidepressants. Then, part of literature included by allocation concealment and blinding is not yet clear. TCM has the characteristics of personalized treatment, which is limited to the unique odor and color, making it difficult to implement blind methods. In addition, screening the active ingredients based on OB and DL may exist inconsistency with the precise ingredients, and the predicted targets are likely to be limited by the hot spots of current research. Finally, the specific mechanism in this study still needs to be verified by further vivo and vitro experiments.
7 Conclusion
This study analyzed the effectiveness and mechanism of CLM in the treatment of depression. Our findings revealed that compared to antidepressants, CLM had better clinical efficacy and fewer side effects, especially in combination with SSRI. Its mechanism in treating depression may be related to regulating neuronal synaptic plasticity, inhibiting neuroinflammation, and improving neuronal damage. Stigmasterol, β-stiosterol, and coumestrol, three components of CLM. may play antidepressant roles via the targets of PIK3R1, MAPK3, and AKT1. The PI3K/Akt signaling pathway may be one of the main pathways for CLM to achieve its antidepressant effect.
Data availability statement
The original contributions presented in the study are included in the article/Supplementary Materials, further inquiries can be directed to the corresponding authors.
Author contributions
YZ: Formal Analysis, Writing–original draft, Writing–review and editing. DX: Funding acquisition, Resources, Writing–review and editing. JW: Data curation, Investigation, Writing–review and editing. DZ: Data curation, Methodology, Software, Writing–review and editing. AL: Data curation, Formal Analysis, Software, Writing–review and editing. YS: Data curation, Formal Analysis, Software, Writing–review and editing. YY: Formal Analysis, Software, Writing–review and editing. JL: Supervision, Writing–review and editing. WG: Supervision, Writing–review and editing.
Funding
DX was supported by the Project of Taicang Science and Technology Bureau of Jiangsu Province (TC2021JCYL07).
Conflict of interest
The authors declare that the research was conducted in the absence of any commercial or financial relationships that could be construed as a potential conflict of interest.
Publisher’s note
All claims expressed in this article are solely those of the authors and do not necessarily represent those of their affiliated organizations, or those of the publisher, the editors and the reviewers. Any product that may be evaluated in this article, or claim that may be made by its manufacturer, is not guaranteed or endorsed by the publisher.
Supplementary material
The Supplementary Material for this article can be found online at: https://www.frontiersin.org/articles/10.3389/fphar.2023.1257617/full#supplementary-material
References
Beurel, E., Toups, M., and Nemeroff, C. B. (2020). The bidirectional relationship of depression and inflammation: Double trouble. Neuron 107 (2), 234–256. doi:10.1016/j.neuron.2020.06.002
Borbely, E., Simon, M., Fuchs, E., Wiborg, O., Czeh, B., and Helyes, Z. (2022). Novel drug developmental strategies for treatment-resistant depression. Br. J. Pharmacol. 179 (6), 1146–1186. doi:10.1111/bph.15753
Cao, J., and Guo, Y. (2021). Clinical observation on the treatment of depressive insomnia with modified Chaihu longgu oyster soup and mirtazapine. World Latest Med. Inf. 21 (88), 227–229. doi:10.3969/j.issn.1671-3141.2021.88.070
Chen, G., He, Y., and Wang, B. (2017). Curative effect and mechanism analysis of modified Chaihu longgu muli decoction combined with anti-anxiety medication for somatization, depression and anxiety state. Chin. Archives Traditional Chin. Med. 35 (3), 758–760. doi:10.13193/j.issn.1673-7717.2017.03.070
Chen, L., Cao, Y., Zhang, H., Lv, D., Zhao, Y., Liu, Y., et al. (2018). Network pharmacology-based strategy for predicting active ingredients and potential targets of Yangxinshi tablet for treating heart failure. J. Ethnopharmacol. 219, 359–368. doi:10.1016/j.jep.2017.12.011
Chen, Z., and Ding, L. (2012). Clinical observation on treating 40 cases of depression with the Chaihu plus Longgu Muli decoction and lily anemarrhena decoction. Clin. J. Chin. Med. 4 (3), 38–39.
COVID-19 Mental Disorders Collaborators (2021). Global prevalence and burden of depressive and anxiety disorders in 204 countries and territories in 2020 due to the COVID-19 pandemic. Lancet 398 (10312), 1700–1712. doi:10.1016/S0140-6736(21)02143-7
Dodd, S., Bauer, M., Carvalho, A. F., Eyre, H., Fava, M., Kasper, S., et al. (2021). A clinical approach to treatment resistance in depressed patients: What to do when the usual treatments don't work well enough? World J. Biol. Psychiatry 22 (7), 483–494. doi:10.1080/15622975.2020.1851052
Dong, L., Li, Y. Z., An, H. T., Wang, Y. L., Chen, S. H., Qian, Y. J., et al. (2016). The E3 ubiquitin ligase c-cbl inhibits microglia-mediated CNS inflammation by regulating PI3K/akt/NF-κB pathway. CNS Neurosci. Ther. 22 (8), 661–669. doi:10.1111/cns.12557
Dowlati, Y., Herrmann, N., Swardfager, W., Liu, H., Sham, L., Reim, E. K., et al. (2010). A meta-analysis of cytokines in major depression. Biol. Psychiatry 67 (5), 446–457. doi:10.1016/j.biopsych.2009.09.033
Fang, S., Dong, L., Liu, L., Guo, J., Zhao, L., Zhang, J., et al. (2021). Herb: A high-throughput experiment- and reference-guided database of traditional Chinese medicine. Nucleic Acids Res. 49 (D1), D1197–D1206. doi:10.1093/nar/gkaa1063
Gao, Q., Han, Z. Y., Tian, D. F., Liu, G. L., Wang, Z. Y., Lin, J. F., et al. (2021). Xinglou Chengqi Decoction improves neurological function in experimental stroke mice as evidenced by gut microbiota analysis and network pharmacology. Chin. J. Nat. Med. 19 (12), 881–899. doi:10.1016/S1875-5364(21)60079-1
GBD 2015 Disease and Injury Incidence and Prevalence Collaborators (2016). Global, regional, and national incidence, prevalence, and years lived with disability for 310 diseases and injuries, 1990-2015: A systematic analysis for the global burden of disease study 2015. Lancet 388 (10053), 1545–1602. doi:10.1016/S0140-6736(16)31678-6
Ghosh, S., Kumar, A., Sachan, N., and Chandra, P. (2022). Evaluation of the antidepressant-like effect of total sterols fraction and Stigmasterol isolated from leaves of aegle marmelos and possible mechanism(s) of action involved. Curr. Drug Discov. Technol. 19 (2), e290721195144. doi:10.2174/1570163818666210729165310
Han, X., Xu, T., Fang, Q., Zhang, H., Yue, L., Hu, G., et al. (2021). Quercetin hinders microglial activation to alleviate neurotoxicity via the interplay between NLRP3 inflammasome and mitophagy. Redox Biol. 44, 102010. doi:10.1016/j.redox.2021.102010
Hanying, F., Xinxia, G., Yaqi, L., Siqi, L., and Weifeng, G. (2022). Thoughts and methods of treating emotional diseases from mental disorders. J. Hubei Univ. Chin. Med. 24 (5), 61–64. doi:10.3969/j.issn.1008987x.2022.05.14
Heberle, H., Meirelles, G. V., da Silva, F. R., Telles, G. P., and Minghim, R. (2015). InteractiVenn: A web-based tool for the analysis of sets through venn diagrams. BMC Bioinforma. 16 (1), 169. doi:10.1186/s12859-015-0611-3
Howren, M. B., Lamkin, D. M., and Suls, J. (2009). Associations of depression with C-reactive protein, IL-1, and IL-6: A meta-analysis. Psychosom. Med. 71 (2), 171–186. doi:10.1097/PSY.0b013e3181907c1b
Hu, J. (2021). Clinical observation on the combination of Chinese and western medicines in the treatment of depression. J. Pract. Traditional Chin. Med. 37 (8), 1366–1367.
Hu, Y., Yang, Y., and Wang, X. (2016). Clinical observation and theoretical discussion of Chaihu plus Longgu Oyster soup in the treatment of depression. World Latest Med. Inf. 16 (8), 204–205. doi:10.3969/j.issn.1671-3141.2016.08.129
Jia, L., and Li, X. (2022). Analysis of the efficacy and safety of modified Chaihu plus Longgu Muli Decoction in the treatment of depression. China Pract. Med. 17 (10), 30–33. doi:10.14163/j.cnki.11-5547/r.2022.10.009
Karege, F., Perroud, N., Burkhardt, S., Fernandez, R., Ballmann, E., La Harpe, R., et al. (2011). Alterations in phosphatidylinositol 3-kinase activity and PTEN phosphatase in the prefrontal cortex of depressed suicide victims. Neuropsychobiology 63 (4), 224–231. doi:10.1159/000322145
Kiecolt-Glaser, J. K., Derry, H. M., and Fagundes, C. P. (2015). Inflammation: Depression fans the flames and feasts on the heat. Am. J. Psychiatry 172 (11), 1075–1091. doi:10.1176/appi.ajp.2015.15020152
Kohler, O., Benros, M. E., Nordentoft, M., Farkouh, M. E., Iyengar, R. L., Mors, O., et al. (2014). Effect of anti-inflammatory treatment on depression, depressive symptoms, and adverse effects: A systematic review and meta-analysis of randomized clinical trials. JAMA Psychiatry 71 (12), 1381–1391. doi:10.1001/jamapsychiatry.2014.1611
Li, G., Chunhua, J., Cuilan, M., Hui-wen, H., Zhang, X., Yang, Z., et al. (2018). Mouse macrophages capture and kill Giardia lamblia by means of releasing extracellular trap. Henan Tradit. Chin. Med. 38 (2), 206–212. doi:10.1016/j.dci.2018.07.024
Li, L., Liu, X., and Xu, B. (2016). Fifty-five cases with depression treated with supplemented longgu muli decoction combined with paroxetine. Henan Tradit. Chin. Med. 36 (5), 770–772. doi:10.16367/j.issn.1003-5028.2016.05.0329
Li, Q. F., Lu, W. T., Zhang, Q., Zhao, Y. D., Wu, C. Y., and Zhou, H. F. (2022a). Proprietary medicines containing bupleurum chinense DC. (Chaihu) for depression: Network meta-analysis and network pharmacology prediction. Front. Pharmacol. 13, 773537. doi:10.3389/fphar.2022.773537
Li, X., Jia, L., Lu, Y., Wang, J., and Yao, W. (2022b). Genetic analysis of platelet-related genes in hepatocellular carcinoma reveals a novel prognostic signature and determines PRKCD as the potential molecular bridge. China Prac. Med. 17 (12), 22–25. doi:10.1186/s12575-022-00185-9
Liu, X., Kong, Y., Feng, Y., and Yang, W. (2017). Effects of traditional Chinese medicine and sertraline on clinical efficacy and serum cytokines levels in first-episode depression patients. J. Int. psychiatry 44 (6), 1018–1020. doi:10.13479/j.cnki.jip.2017.06.017
Liu, Y., Ho, R. C., and Mak, A. (2012). Interleukin (IL)-6, tumour necrosis factor alpha (TNF-alpha) and soluble interleukin-2 receptors (sIL-2R) are elevated in patients with major depressive disorder: A meta-analysis and meta-regression. J. Affect Disord. 139 (3), 230–239. doi:10.1016/j.jad.2011.08.003
Liu, Y., Ma, S., and Qu, R. (2010). SCLM, total saponins extracted from Chaihu-jia-longgu-muli-tang, reduces chronic mild stress-induced apoptosis in the hippocampus in mice. Pharm. Biol. 48 (8), 840–848. doi:10.3109/13880200903296154
Lizhi, S., Mengdi, M., Erping, X., Mingyuan, Z., Chunyu, Z., Guoqiang, W., et al. (2021). Immunoregulatory effect of Chaihu jia longgu mulitang modified with bupleuri radix on hippocampal NLRP3 pathway in depressed rats. Chin. J. Exp. Traditional Med. Formulae 27 (24), 33–39. doi:10.13422/j.cnki.syfjx.20202303
Luo, Z., Lv, X., and Xie, W. (2019). Clinical study on 42 cases of depressive disorder treated with chaihu-jia-longgu-muli-tang combined with western medicine. Chin. J. Ethnomedicine Ethnopharmacy 28 (3), 104–105.
Malemud, C. J., and Miller, A. H. (2008). Pro-inflammatory cytokine-induced SAPK/MAPK and JAK/STAT in rheumatoid arthritis and the new anti-depression drugs. Expert Opin. Ther. Targets 12 (2), 171–183. doi:10.1517/14728222.12.2.171
Malhi, G. S., and Mann, J. J. (2018). Depression. Lancet 392 (10161), 2299–2312. doi:10.1016/S0140-6736(18)31948-2
Matsuda, S., Ikeda, Y., Murakami, M., Nakagawa, Y., Tsuji, A., and Kitagishi, Y. (2019). Roles of PI3K/AKT/GSK3 pathway involved in psychiatric illnesses. Diseases 7 (1), 22. doi:10.3390/diseases7010022
Moitra, M., Santomauro, D., Collins, P. Y., Vos, T., Whiteford, H., Saxena, S., et al. (2022). The global gap in treatment coverage for major depressive disorder in 84 countries from 2000-2019: A systematic review and bayesian meta-regression analysis. PLoS Med. 19 (2), e1003901. doi:10.1371/journal.pmed.1003901
Nicolas, C. S., Peineau, S., Amici, M., Csaba, Z., Fafouri, A., Javalet, C., et al. (2012). The Jak/STAT pathway is involved in synaptic plasticity. Neuron 73 (2), 374–390. doi:10.1016/j.neuron.2011.11.024
Niitsu, T., Okamoto, H., and Iyo, M. (2013). Behavioural and psychological symptoms of dementia in an alzheimer's disease case successfully treated with natural medicine: Association with gonadotropins. Psychogeriatrics 13 (2), 124–127. doi:10.1111/psyg.12010
O'Boyle, N. M., Banck, M., James, C. A., Morley, C., Vandermeersch, T., and Hutchison, G. R. (2011). Open Babel: An open chemical toolbox. J. Cheminform 3, 33. doi:10.1186/1758-2946-3-33
Page, M. J., McKenzie, J. E., Bossuyt, P. M., Boutron, I., Hoffmann, T. C., Mulrow, C. D., et al. (2021). The PRISMA 2020 statement: An updated guideline for reporting systematic reviews. BMJ 372, n71. doi:10.1136/bmj.n71
Perez-Catalan, N. A., Doe, C. Q., and Ackerman, S. D. (2021). The role of astrocyte-mediated plasticity in neural circuit development and function. Neural Dev. 16 (1), 1. doi:10.1186/s13064-020-00151-9
Pinero, J., Bravo, A., Queralt-Rosinach, N., Gutierrez-Sacristan, A., Deu-Pons, J., Centeno, E., et al. (2017). DisGeNET: A comprehensive platform integrating information on human disease-associated genes and variants. Nucleic Acids Res. 45 (D1), D833–D839. doi:10.1093/nar/gkw943
Regier, D. A., Kuhl, E. A., and Kupfer, D. J. (2013). The DSM-5: Classification and criteria changes. World Psychiatry 12 (2), 92–98. doi:10.1002/wps.20050
Seong, S. H., Kim, B. R., Cho, M. L., Kim, T. S., Im, S., Han, S., et al. (2022). Phytoestrogen coumestrol selectively inhibits monoamine oxidase-A and amyloid beta self-aggregation. Nutrients 14 (18), 3822. doi:10.3390/nu14183822
Stelzer, G., Rosen, N., Plaschkes, I., Zimmerman, S., Twik, M., Fishilevich, S., et al. (2016). The GeneCards suite: From gene data mining to disease genome sequence analyses. Curr. Protoc. Bioinforma. 54, 1.30.1–1.30.33. doi:10.1002/cpbi.5
Sun, P. (2020). Evaluation of the effect of chaihulonggumuli decoction in the auxiliary treatment of depression. World J. Complex Med. 6 (8), 136–138. doi:10.11966/j.issn.2095-994X.2020.06.08.46
Sun, Y., Zhang, H., Wu, Z., Yu, X., Yin, Y., Qian, S., et al. (2021). Quercitrin rapidly alleviated depression-like behaviors in lipopolysaccharide-treated mice: The involvement of PI3K/AKT/NF-κB signaling suppression and CREB/BDNF signaling restoration in the Hippocampus. ACS Chem. Neurosci. 12 (18), 3387–3396. doi:10.1021/acschemneuro.1c00371
Szklarczyk, D., Gable, A. L., Lyon, D., Junge, A., Wyder, S., Huerta-Cepas, J., et al. (2019). STRING v11: Protein-protein association networks with increased coverage, supporting functional discovery in genome-wide experimental datasets. Nucleic Acids Res. 47 (D1), D607–D613. doi:10.1093/nar/gky1131
Wan, R., Song, R., Fan, Y., Li, L., Zhang, J., Zhang, B., et al. (2021). Efficacy and safety of Chaihu jia longgu muli decoction in the treatment of poststroke depression: A systematic review and meta-analysis. Evid. Based Complement. Altern. Med. 2021, 7604537. doi:10.1155/2021/7604537
Wang, B., Xia, Y., Li, R., Fu, W., and Dai, H. (2019). Clinical observation of Chaihu plus Longgu Oyster soup in the treatment of depression. Chin. Archives Traditional Chin. Med. 59 (20), 62–64. doi:10.3969/j.issn.1002-266X.2019.20.017
Wang, X., Chen, J., Yang, N., and Qiao, H. (2020). Clinical study on 30 cases of major depressive disorder with insomnia treated by chaihu-jia-longgu-muli-tang combined with western medicine. Jiangsu J. Traditional Chin. Med. 52 (10), 27–29. doi:10.19844/j.cnki.1672-397X.2020.10.009
Wang, X., Zou, Z., Shen, Q., Huang, Z., Chen, J., Tang, J., et al. (2018). Involvement of NMDA-AKT-mTOR signaling in rapid antidepressant-like activity of chaihu-jia-longgu-muli-tang on olfactory bulbectomized mice. Front. Pharmacol. 9, 1537. doi:10.3389/fphar.2018.01537
Wu, L., Pan, K., and Wu, A. (2020). Clinical study of Chaihu longgu oyster soup combined with paroxetine in the treatment of depression. Chin. J. Integr. Med. Cardio-Cerebrovascular Dis. 18 (12), 1994–1996. doi:10.12102/j.issn.1672-1349.2020.12.041
Xian, Y. F., Ip, S. P., Li, H. Q., Qu, C., Su, Z. R., Chen, J. N., et al. (2019). Isorhynchophylline exerts antidepressant-like effects in mice via modulating neuroinflammation and neurotrophins: Involvement of the PI3K/Akt/GSK-3β signaling pathway. FASEB J. 33 (9), 10393–10408. doi:10.1096/fj.201802743RR
Xiang-yu, M., Peng, S., Ling, X., Ying-hui, G., Dong-mei, G., Jie-qiong, W., et al. (2021). Discussion on the research progress of proteomics in traditional Chinese medicine based on holistic concept. China J. Traditional Chin. Med. Pharm. 36 (11), 6585–6588.
Xiao, Q., Li, S., Wang, L., and Guo, N. (2018). Clinical observation of Chaihu-jia-Longgu-Muli-tang plus duloxetine hydrochloride in the treatment of anxiety depression. Chin. J. Clin. Ration. Drug Use 11 (6B), 35–36. doi:10.15887/j.cnki.13-1389/r.2018.17.021
Xiao, Y., and Han, G. (2020). Clinical effect of Chaihu-jia-Longgu-Muli-tang combined with droperithiazide and melitracen tablets in the treatment of anxiety and depression patients. Henan Med. Res. 29 (7), 1286–1288. doi:10.3969/j.issn.1004-437X.2020.07.070
Xu, D., and Zhao, J. (2017). The efficacy of Jiayichaihu Longgu Oyster soup combined with paroxetine in the treatment of depression and its influence on serum inflammatory factors and quality of life of patients. Mod. J. Integr. Traditional Chin. West. Med. 26 (12), 1303–1305. doi:10.3969/j.issn.1008-8849.2017.12.016
Ye, S., and Luo, B. (2008). A clinical observation on treating 50 case of depression syndrome with TCM medicine. Chin. J. Pract. Chin. Mod. Med. 21 (3), 239–240.
Ypsilanti, A., Lazuras, L., Robson, A., and Akram, U. (2018). Anxiety and depression mediate the relationship between self-disgust and insomnia disorder. Sleep. Health 4 (4), 349–351. doi:10.1016/j.sleh.2018.06.001
Yuanwen, Z., Tianhui, Y., Jianlue, P., Pangning, H., and Rongfa, H. (2022). Meta-analysis of Chaihu plus longgu muli decoction in the treatment of coronary heart disease complicated with anxiety and depression. Traditional Chin. Drug Res. Clin. Pharmacol. 33 (10), 1435–1444. doi:10.19378/j.issn.1003-9783.2022.10.019
Zeng, P., Su, H. F., Ye, C. Y., Qiu, S. W., Shi, A., Wang, J. Z., et al. (2022). A tau pathogenesis-based network pharmacology approach for exploring the protections of chuanxiong rhizoma in alzheimer's disease. Front. Pharmacol. 13, 877806. doi:10.3389/fphar.2022.877806
Zhang, B., Guo, F., Ma, Y., Song, Y., Lin, R., Shen, F. Y., et al. (2017). Activation of D1R/PKA/mTOR signaling cascade in medial prefrontal cortex underlying the antidepressant effects of l-SPD. Sci. Rep. 7 (1), 3809. doi:10.1038/s41598-017-03680-2
Zhang, J. (2021). Effect of Chaihu longgu oyster soup on negative emotion of patients with depression. Mod. Med. Health Res. Electron. J. 5 (13), 91–93.
Zhang, L. (2010). Clinical observation of Chaihu longgu oyster soup on depression. Hebei Med. J. 32 (22), 3185–3186.
Zhang, W., and Lin, Y. (2021). Clinical effectiveness of Chaihu plus Longgu Oyster soup in the treatment of depression. Word Latest Med. Inf. 21 (92), 149–151. doi:10.3969/j.issn.1671-3141.2021.92.050
Zhang, Y. (2014). Analysis on the effect of Chaihu plus Longgu Oyster soup in the treatment of depression. Contemp. Med. Symp. 12 (15), 21.
Zhao, D., Zheng, L., Qi, L., Wang, S., Guan, L., Xia, Y., et al. (2016). Structural features and potent antidepressant effects of total sterols and beta-sitosterol extracted from sargassum horneri. Mar. Drugs 14 (7), 123. doi:10.3390/md14070123
Zhong, X., Li, X., and Miao, X. (2012). Chaihu-jia-Longgu-Muli-tang in the treatment of 50 cases of depression. China Health Care & Nutr. 22 (20), 4746–4747. doi:10.3969/j.issn.1004-7484X.2012.11.629
Zhou, Y., Zhou, B., Pache, L., Chang, M., Khodabakhshi, A. H., Tanaseichuk, O., et al. (2019). Metascape provides a biologist-oriented resource for the analysis of systems-level datasets. Nat. Commun. 10 (1), 1523. doi:10.1038/s41467-019-09234-6
Zhu, Q., Enkhjargal, B., Huang, L., Zhang, T., Sun, C., Xie, Z., et al. (2018). Aggf1 attenuates neuroinflammation and BBB disruption via PI3K/Akt/NF-κB pathway after subarachnoid hemorrhage in rats. J. Neuroinflammation 15 (1), 178. doi:10.1186/s12974-018-1211-8
Keywords: depression, chaihu-jia-longgu-muli-tang, meta-analysis, network pharmacology, inflammation
Citation: Zhao Y, Xu D, Wang J, Zhou D, Liu A, Sun Y, Yuan Y, Li J and Guo W (2023) The pharmacological mechanism of chaihu-jia-longgu-muli-tang for treating depression: integrated meta-analysis and network pharmacology analysis. Front. Pharmacol. 14:1257617. doi: 10.3389/fphar.2023.1257617
Received: 12 July 2023; Accepted: 05 September 2023;
Published: 21 September 2023.
Edited by:
Song Zhang, Shanghai Jiao Tong University, ChinaReviewed by:
Dalinda Isabel Sánchez-Vidaña, Hong Kong Polytechnic University, Hong Kong SAR, ChinaOpeyemi Iwaloye, Federal University of Technology, Nigeria
Copyright © 2023 Zhao, Xu, Wang, Zhou, Liu, Sun, Yuan, Li and Guo. This is an open-access article distributed under the terms of the Creative Commons Attribution License (CC BY). The use, distribution or reproduction in other forums is permitted, provided the original author(s) and the copyright owner(s) are credited and that the original publication in this journal is cited, in accordance with accepted academic practice. No use, distribution or reproduction is permitted which does not comply with these terms.
*Correspondence: Jianxiang Li, bGp4MDI5NTA0QG5qdWNtLmVkdS5jbg==; Weifeng Guo, Z3dmd2ZnMjAwM0BuanVjbS5lZHUuY24=
†These authors have contributed equally to this work and shared the first authorship