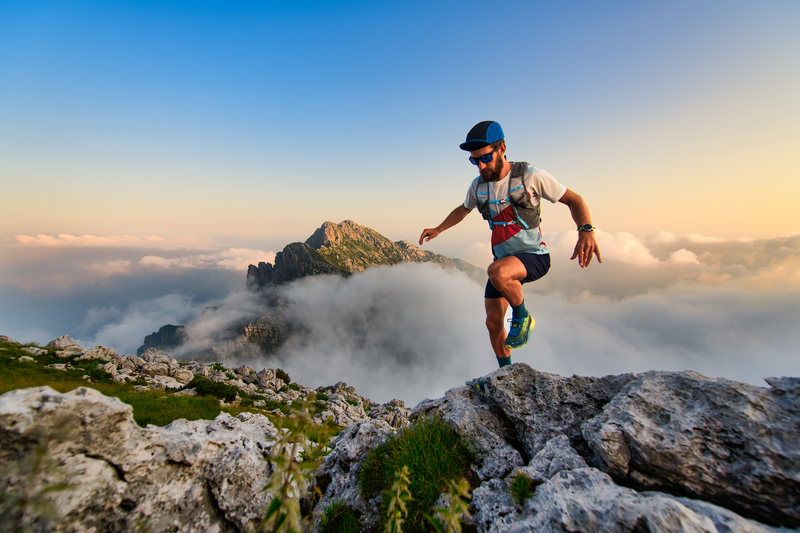
94% of researchers rate our articles as excellent or good
Learn more about the work of our research integrity team to safeguard the quality of each article we publish.
Find out more
ORIGINAL RESEARCH article
Front. Pharmacol. , 16 January 2024
Sec. Pharmacoepidemiology
Volume 14 - 2023 | https://doi.org/10.3389/fphar.2023.1255609
This article is part of the Research Topic Application of Data Mining in Pharmaceutical Research View all 12 articles
Objective: Pulmonary infection (PI), a severe complication of immunosuppressive therapy, affects patients’ prognosis. As part of this study, we aimed to construct a pulmonary infection prediction (PIP) model and validate it in patients receiving immunosuppressive drugs (ISDs).
Methods: Totally, 7,977 patients being treated with ISDs were randomised 7:3 to the developing (n = 5,583) versus validation datasets (n = 2,394). Our predictive nomogram was established using the least absolute shrinkage and selection operator (LASSO) and multivariate COX regression analyses. With the use of the concordance index (C-index) and calibration curve, the prediction performance of the final model was evaluated.
Results: Among the patients taking immunosuppressive medication, PI was observed in 548 (6.9%). The median time of PI occurrence after immunosuppressive therapy was 123.0 (interquartile range: 63.0, 436.0) days. Thirteen statistically significant independent predictors (sex, age, hypertension, DM, malignant tumour, use of biologics, use of CNIs, use of methylprednisolone at 500 mg, use of methylprednisolone at 40 mg, use of methylprednisolone at 40 mg total dose, use of oral glucocorticoids, albumin level, and haemoglobin level) were screened using the LASSO algorithm and multivariate COX regression analysis. The PIP model built on these features performed reasonably well, with the developing C-index of 0.87 (sensitivity: 85.4%; specificity: 81.0%) and validation C-indices of 0.837, 0.829, 0.832 and 0.830 for predicting 90-, 180-, 270- and 360-day PI probability, respectively. The decision curve analysis (DCA) and calibration curves displayed excellent clinical utility and calibration performance of the nomogram.
Conclusion: The PIP model presented herein could aid in the prediction of PI risk in individual patients who receive immunosuppressive treatment and help personalise clinical decision-making.
Immunosuppressive drugs (ISDs) are a class of drugs that exert immunosuppressive effects through various mechanisms and are primarily used to clinically modulate the immune response of patients (Suthanthiran et al., 1996; Barshes et al., 2004; Allison, 2005). Since the establishment of their immunosuppressive action, ISDs have been widely recommended as first-line therapeutics in organ transplantation cases and the treatment of autoimmune disorders (Kasiske et al., 2010; Radhakrishnan and Cattran, 2012; Fanouriakis et al., 2019). With the widespread application of glucocorticoids and other immunosuppressants, an increasing number of latent adverse effects is linked with this class of agents in recent years, and this limits their use (Penfornis and Kury-Paulin, 2006; Htwe and Khardori, 2017; Chotiyarnwong and McCloskey, 2020). Pulmonary infection (PI) is a common comorbidity in immunosuppressed patients and contributes to an exceptionally high mortality rate (Godbole and Gant, 2013; Ahuja and Kanne, 2014), which it has various potential reasons. One reason could be that immunosuppressants inhibit the immune function of patients, which significantly increases their susceptibility to a variety of microbial pathogens and the incidence of infection, especially PI, directly threatening the lives of many patients (Stahn and Buttgereit, 2008). Moreover, the symptoms of infection in patients could be overshadowed by the long-term course of immunosuppressants, which makes it difficult for early diagnosis and treatment, thus rendering rapid progression and a poor prognosis (Poowuttikul et al., 2019). The results of a 10-year cohort study from China revealed that patients with pneumonia who previously received active immunosuppressant therapy had a greater risk of mortality when hospitalised (Yin et al., 2021). Therefore, it is crucial to understand the risk factors and identify patients at a high risk of PI when initiating immunosuppressant therapy. However, there is a lack of visual prediction models that can be applied in broad and large populations.
The nomogram model, as a multi-factor calibrated visualisation tool, has been extensively used to predict various outcomes in clinical practice, and it can provide the rationale for clinicians to develop more effective and individualised therapy regimens (Iasonos et al., 2008). Accordingly, this research was devised to establish a simple and effective nomogram prediction model for PI and validate it in patients receiving immunosuppressive therapy.
The diagnostic criteria for PI in the current research encompassed the following elements: 1) Clinical manifestations comprised the onset of a new cough or expectoration, or the exacerbation of existing respiratory tract symptoms, accompanied by or without purulent sputum, chest pain, dyspnea, hemoptysis, fever, and rales detected during lung auscultation; 2) Leukocyte count exceeding 10 × 109/L or falling below 4 × 109/L (Kalil et al., 2016); 3) Imaging characteristics encompassed the emergence of infiltration, consolidation, ground-glass opacity, or effusion observed in chest plain films or computed tomography scans (Wunderink et al., 1992; Koenig and Truwit, 2006). Patients who satisfied the third criterion, in conjunction with either the first or second criterion, were subjected to a clinical diagnosis of PI. Diagnosis of PI was reviewed and reconfirmed by an experienced specialist in infectious diseases (JZ).
Immunosuppressive agents used in this study were calcineurin inhibitors (CNIs) (tacrolimus and cyclosporine A), cyclophosphamide (CTX), mycophenolate mofetil (MMF), biologics (rituximab, infliximab, etanercept, adalimumab, tocilizumab, abatacept and bortezomib), azathioprine, methotrexate, leflunomide, Tripterygium wilfordii, hydroxychloroquine, and glucocorticoids.
The inclusion criteria were as follows: 1) patients using ISDs according to electronic medical records; 2) a follow-up period of longer than 14 days after initiating immunosuppressive therapy.
The exclusion criteria were as follows: 1) age less than 18 years old; 2) PI occurred within 14 days of immunosuppressive therapy; 3) patients with pre-existing PI who need to receive ISDs treatment, such as acute exacerbations of chronic obstructive pulmonary disease (AECOPD) and COVID-19.
Demographic characteristics and laboratory data of, and physician’s orders for these patients including, but not limited to, sex, age, hypertension, diabetes mellitus (DM), malignant tumour, use of immunosuppressive agents, albumin level, haemoglobin level and lymphocyte level were excerpted from the hospital’s electronic patient management system. The study design flowchart is depicted in Figure 1.
FIGURE 1. Flow diagram of the study design. CNIs, calcineurin inhibitors; DM, diabetes mellitus; ISDs, immunosuppressive drugs; LASSO, least absolute shrinkage and selection operator; PI, pulmonary infection.
Keeping in conformity with the Helsinki Declaration (as revised in 2013), our study received ethical permission from the Zhejiang Provincial People’s Hospital Ethics Committee (No. 2021QT345) (World Medical Association, 2013).
Informed consent from the patients was waived as the study was retrospective in nature.
The baseline characteristics of the two cohorts are summarised as frequency count (percentage) for count variables and median (interquartile range [IQR]) for metric variables. To measure between-group differences, count variables were subjected to chi-square or Fisher’s exact test, and metric variables were subjected to t-test or Wilcoxon test, depending on the distribution.
Imputation was conducted if missing values were <20%. The LASSO method was adopted to screen significant covariates and prevent the overfitting of the model. In this regression model, the absolute size of the coefficients of a regression model is penalised according to the value of λ, which is a LASSO regularisation parameter. In the presence of larger penalties, the estimates of weaker factors shrink toward zero, resulting in only the strongest factors remaining in the model. The most predictive covariates were chosen based on the minimum of λ (λmin). Thereafter, we incorporated the variables identified via LASSO regression analysis into COX regression models, and the variables that were consistently statistically significant were chosen to construct the nomogram.
A nomogram was constructed based on the final model. To evaluate the predictive performance in terms of discrimination and calibration, validation of the derived nomogram was carried out. An assessment of discrimination was performed using the receiver operating characteristic (ROC) curve and concordance index (C-index). A comparison of the observed PI rates with predictions from the final model was used to evaluate calibration. R software, version 4.1.0 (2021-05-18; R Foundation for Statistical Computing, Vienna, Austria) was employed for the statistical analyses presented. R packages including mice, VIM, missForest, survival, survminer, rms, glmnet, regplot, survivalROC, and survcomp were utilised in the current study. A two-tailed P-value of <0.05 was set to denote statistical significance for all tests.
In total, 8,625 patients using ISDs between January 2010 and October 2021 were consecutively selected for this study. Among these patients, 610 were excluded because of PI before immunosuppressive treatment or the occurrence of PI within 14 days of immunosuppressive therapy, and 38 patients were excluded as they were under 18 years of age. Ultimately, 7,977 patients were selected for the current analysis. All participants were randomly assigned to either the developing dataset (n = 5,583) or validation dataset (n = 2,394) at a 7:3 ratio. The detailed study flow was given in Figure 1. There were no statistically significant differences in the demographic factors between the sets (Table 1). The prevalence of PI was 6.9% (384/5,583) in the developing set and 6.9% (164/2,394) in the validation set. The median time of PI after immunosuppressive therapy was 123.0 (IQR: 63.0, 436.0) days.
A total of 53 general variables measured at admission to the hospital were included in the LASSO regression analysis. Participants’ characteristics were shown in Supplementary Table S1. Based on the LASSO regression analysis results, 15 features were chosen to be potential predictors of PI, including sex, age, hypertension, DM, malignant tumour, CTX total dose, use of biologics, use of MMF, use of CNIs, use of methylprednisolone at 500 mg, use of methylprednisolone at 40 mg, total cumulative dose of methylprednisolone at 40 mg, use of oral glucocorticoids, albumin level, and haemoglobin level. The screening for the LASSO analysis is shown in Figure 2. The significant features from LASSO were subsequently chosen for further multivariate COX regression analysis. Thirteen variables were finally screened as statistically significant independent predictors of PI. The results of the multivariate COX regression analysis are presented in Figure 3.
FIGURE 2. Variable selection using the least absolute shrinkage and selection operator (LASSO) regression model: (A) LASSO coefficient profiles of the 58 baseline features; (B) Tuning parameter (λ) selection in the LASSO model used 10-fold cross-testing via minimum criteria.
FIGURE 3. Multivariate COX regression analysis of the developing cohort. Alb, albumin; CNIs, calcineurin inhibitors; CTX, cyclophosphamide; DM, diabetes mellitus; Hb, haemoglobin; MMF, mycophenolate mofetil; pred, methylprednisolone.
Thirteen of the clinical parameters (sex, age, hypertension, DM, malignant tumour, use of biologics, use of CNIs, use of methylprednisolone at 500 mg, use of methylprednisolone at 40 mg, use of methylprednisolone at 40 mg total dose, use of oral glucocorticoids, albumin level, and haemoglobin level) were integrated to construct a pulmonary infection prediction (PIP) model in patients receiving ISDs (Figure 4).
FIGURE 4. The nomogram of the pulmonary infection prediction (PIP) model. In the nomogram, each patient’s value is displayed on an axis for each variable, and a line is drawn upward to determine the number of points each variable receives. On the Total Points axis is the sum of these numbers, and a line is drawn downward to the survival axes to determine 90-, 180- and 360-day probability of PI. Alb, albumin; CNIs, calcineurin inhibitors; DM, diabetes mellitus; Hb, haemoglobin; PI, pulmonary infection; pred, methylprednisolone.
Notably, patients with the following features presented a greater probability of developing PI: male, old age, hypertension, DM, malignant tumour, use of biologics, use of CNIs, use of methylprednisolone at 500 mg, use of methylprednisolone at 40 mg, high-dose methylprednisolone, use of oral glucocorticoids, and low albumin and haemoglobin levels.
Moreover, the longer the length of the line, the greater the effect of these factors on the risk of developing PI. As found from the nomogram, the use of methylprednisolone at 40 mg total dose had the greatest effect on the occurrence of PI, whereas the presence of hypertension was observed to have the least effect. The top line of the nomogram corresponded to the score for each factor. Scores for each of these parameters were pooled, with higher scores indicating a higher risk of developing PI.
The model showed a high degree of discrimination, with the developing C-index of 0.87 and the validation C-indices of 0.837, 0.829, 0.832 and 0.830 for predicting 90-, 180-, 270- and 360-day PI probability, respectively (Figure 5). The dynamic alterations of the C-indices for the PIP model in the validation cohort are shown in Figure 6. The calibration plot also displayed excellent concordance between the predicted probability of PI and observations, which indicated good calibration of the model in the validation dataset (Figure 7). The decision curve analysis (DCA) of the developing and validation datasets proved the potential clinical value of the model (Figure 8).
FIGURE 5. The receiver operating characteristic (ROC) curves based on the nomogram in the validation dataset: (A) ROC curve predicting 90-day probability of pulmonary infection (PI); (B) ROC curve predicting 180-day probability of PI; (C) ROC curve predicting 270-day probability of PI; (D) ROC curve predicting 360-day probability of PI.
FIGURE 6. The dynamic alterations of concordance index (C-index) for the model in the validation dataset.
FIGURE 8. The decision curve analysis (DCA) of the model: (A) DCA in the developing dataset; (B) DCA in the validation dataset.
We built a simplified prediction model based on 13 easily accessible variables selected using LASSO and multivariate regression analyses to facilitate the personalised estimation of the likelihood of PI and validated it in patients who received immunosuppressant medications. The LASSO regression analysis allows the shrinkage of the coefficients of the less contributive variables to be exactly zero, which effectively deals with the multicollinearity problem in the model and the enormous number of clinical factors (Hepp et al., 2016). The nomogram provides a simple pictorial representation of sophisticated mathematical calculations, and it has been recognised as a reliable and valuable predictive tool for clinical use (Xie et al., 2015). However, it must be clarified that the relatively large number of parameters involved in this model did not affect its usefulness and feasibility. This is because the combination of two or three ISDs is the most commonly used therapy, and it is seldom that all of the listed ISDs are simultaneously prescribed in clinical practice. Further, all patients receiving immunosuppressant therapy were included in our study, regardless of hospital departments. As a result, our predictive model seemed to have a better generalizability to some extent. In 2019, Wang et al. (2019), utilising clinical data of 333 patients, proposed using the AIM-7C score for the early evaluation of the risk of developing PI after the initiation of a cyclosporine regimen. Nevertheless, this score was only applicable to patients with primary membranous nephropathy, and not to all patients receiving immunosuppressive therapy. To our understanding, this is the inaugural instance of a visual nomogram model designed explicitly for the early detection of PI in all patients treated with ISDs.
In this study, we found that the prevalence of PI was 6.9% (548/7,977), illustrating that patients receiving immunosuppressive therapy are a high-risk population for PI. Furthermore, the results showed that the incidence rate of PI among males was 11.9% (302/2,546), whereas that among females was 4.2% (246/5,431), demonstrating that male patients were more vulnerable to PI than females. The reason for this phenomenon could not be elucidated. One possible reason is the different effects of sex hormones on the immune response. Previous studies have reported that testosterone has an immunosuppressive effect, whereas oestrogens tend to reinforce immunological hyper-response (Beery, 2003). Additionally, the majority of males have a history of smoking and alcohol consumption (Fujii et al., 2021; Shrestha et al., 2021). Smoking and alcohol consumption can aggravate underlying diseases and reduce cardiopulmonary function, which is another possible reason for this observation. Another independent risk factor that we found was the age of the patients. There is increasing evidence that the lung immune system declines with age, increasing the risk of developing infections and chronic inflammatory diseases, as well as the mortality rate (Larbi et al., 2008). Moreover, we found low albumin and haemoglobin levels to be independent risk factors for PI development, and this was consistent with the findings of previous research (Levy et al., 2005; Wang et al., 2019). As a well-established marker of malnutritional status, a low serum albumin level strongly suggests poor dietary choices in patients. Long-term immunosuppressive therapy can further affect nutrient balance, which in turn reduces the body’s immunity to pathogens. Patients receiving immunosuppressive therapy who have anaemia may experience weakened immune systems, further increasing their risk of infection. Furthermore, we found that malignant tumours, and the use of biologics and CNIs, were significantly associated with the occurrence of PI. It is generally known that patients with malignant tumours often experience complications with infection owing to their immunocompromised status (Azevedo et al., 2020). Infection is frequently cited as an adverse effect in the administration of biologics and CNIs (Thaunat et al., 2004; Evens et al., 2011; Kelesidis et al., 2011; Sułkowska et al., 2012; High and Olivry, 2020). The pathogenic microorganisms identified in patients with infection included viruses, bacteria, fungi, and virus-bacteria co-infection. Besides the above clinical parameters, we found that the use of methylprednisolone at 500 mg, use of methylprednisolone at 40 mg, total cumulative dose of methylprednisolone at 40 mg, and use of oral glucocorticoids were independent predictors of PI development. Long-term use of glucocorticoids can inhibit the antigen-antibody reaction in the human body, thereby leading to the development of infection (McEwen, 2008). A previous animal study confirmed the relationship of glucocorticoid use with the development of PI (Reis e Sousa, 2001). As such, rational clinical use of glucocorticoids appears to be particularly valuable. In addition, DM and hypertension were correlated with an elevated risk of developing PI. Indeed, DM has been considered a significant risk factor for lower respiratory tract infections in susceptible patients (Klekotka et al., 2015). Chronic hypertension damages blood vessels, resulting in pulmonary oedema, pulmonary congestion, systemic hypoxia, and even PI (Ponte et al., 2013).
Based on 13 independent risk factors (male, age, hypertension, DM, malignant tumour, use of biologics, use of CNIs, use of methylprednisolone at 500 mg, use of methylprednisolone at 40 mg, total cumulative dose of methylprednisolone at 40 mg, use of oral glucocorticoids, and low albumin and haemoglobin levels) established from a large cohort with sufficient sample size, we constructed the first practical nomogram model and demonstrated that it was advantageous for the individualised risk stratification of PI in patients taking immunosuppressants. Our nomogram model demonstrated satisfactory prediction ability, with C-indices of 0.837, 0.829, 0.832, and 0.830 for the 90-, 180-, 270- and 360-day probability of PI in the validation set, respectively, which demonstrated the model’s favourable discriminative ability to differentiate patients who were at a risk of developing PI from those who were not. Furthermore, the calibration plot demonstrated a strong agreement between the calibration and standard curves in the validation cohort, suggesting that the predicted occurrence of PI was close to the observed data. In addition to acknowledging uncontrolled factors, such as sex, age, and malignant tumours, medical staff should strengthen the management of controllable factors. In this prediction model, we consider a value greater than 0.5 as high risk and a value less than 0.5 as low risk. If the value is greater than 0.5, we will not only increase the frequency and content of follow-up, but also communicate with the patient about the risk of infection and adjust the treatment plan if necessary. Once high-risk cases are detected, aggressive preventive interventions should also be undertaken at the earliest opportunity to reduce the morbidity rate of PI. For instance, it is imperative to strongly advocate smoking cessation for all patients receiving immunosuppressants who are still smoking. And nursing staff should assume the responsibility of instructing patients in respiratory function exercises to enhance lung function. Moreover, regular nutritional screening and management should be reinforced as a fundamental element of preventive measures, ensuring efficient support in maintaining optimal patient health.
A number of limitations were identified in the current study. In the first place, due to its retrospective nature, its generalizability was limited by the fact that it was restricted to a single site. Yet, our study enrolled all patients treated with ISDs in a single center irrespective of disciplines, which might somewhat compensate for the aforementioned drawback. Second, our nomogram has not yet undergone external validation, and thus, the extrapolation of our nomogram to other cohorts remains unknown. Further independent external validation using additional large datasets to verify these findings has been planned. Third, some patients were administered more than one ISD during the study, which unavoidably introduced bias into the study. Consequently, further studies should take into account the potential interactions among multiple drugs. Last but not least, our study did not grade the risk of developing PI in patients with immunosuppressive therapy. Accordingly, we plan to explore this issue in our future research.
To summarize, sex, age, hypertension, DM, malignant tumour, use of biologics, use of CNIs, use of methylprednisolone at 500 mg, use of methylprednisolone at 40 mg, total cumulative dose of methylprednisolone at 40 mg, use of oral glucocorticoids, and albumin and haemoglobin levels were independent predictors of the occurrence of PI in patients who were receiving immunosuppressant therapy. The nomogram model established in this study has the potential to assist in the development of an optimal therapeutic intervention for this condition, thereby effectively reducing the occurrence of PI. This suggests that our nomogram model holds promise for widespread implementation in clinical practice.
The raw data supporting the conclusion of this article will be made available by the authors, without undue reservation.
The studies involving humans were approved by the Zhejiang Provincial People’s Hospital Ethics Committee. The studies were conducted in accordance with the local legislation and institutional requirements. The ethics committee/institutional review board waived the requirement of written informed consent for participation from the participants or the participants’ legal guardians/next of kin because the study was retrospective in nature.
CL: Writing–original draft. YZ: Writing–original draft. JZ: Writing–original draft. CJ: Writing–original draft. XY: Writing–original draft. YR: Writing–original draft. HS: Writing–original draft. MC: Writing–original draft. YL: Writing–original draft. QH: Writing–review and editing. GX: Writing–review and editing. LS: Writing–review and editing.
The authors declare financial support was received for the research, authorship, and/or publication of this article. This work was supported by the Medical and Health Technology Program of Zhejiang Province (Grant Nos 2021KY468 and 2023KY523), and the National Natural Science Foundation of China (Grant No. 82202042).
We would like to thank our colleagues at Zhejiang Provincial People’s Hospital for their valuable contributions to this work and Dr. Zenglei He for their support with the statistical analysis. We are particularly grateful for the technical support and data platform that Yidu Cloud Technology Company Ltd. provided to make this project a reality. Lastly, we would like to thank Editage (www.editage.cn) for English language editing.
The authors declare that the research was conducted in the absence of any commercial or financial relationships that could be construed as a potential conflict of interest.
All claims expressed in this article are solely those of the authors and do not necessarily represent those of their affiliated organizations, or those of the publisher, the editors and the reviewers. Any product that may be evaluated in this article, or claim that may be made by its manufacturer, is not guaranteed or endorsed by the publisher.
The Supplementary Material for this article can be found online at: https://www.frontiersin.org/articles/10.3389/fphar.2023.1255609/full#supplementary-material
C-index, concordance index; CNIs, calcineurin inhibitors; CTX, cyclophosphamide; DCA, decision curve analysis; DM, diabetes mellitus; IQR, interquartile range; ISDs, immunosuppressive drugs; λmin, minimum of λ; LASSO, least absolute shrinkage and selection operator; MMF, mycophenolate mofetil; PI, pulmonary infection; PIP, pulmonary infection prediction; ROC; receiver operating characteristic.
Ahuja, J., and Kanne, J. P. (2014). Thoracic infections in immunocompromised patients. Radiol. Clin. North. Am. 52 (1), 121–136. doi:10.1016/j.rcl.2013.08.010
Allison, A. C. (2005). Mechanisms of action of mycophenolate mofetil. Lupus 14 (Suppl. 1), s2–s8. doi:10.1191/0961203305lu2109oa
Azevedo, M. M., Pina-Vaz, C., and Baltazar, F. (2020). Microbes and cancer: friends or faux? Int. J. Mol. Sci. 21 (9), 3115. doi:10.3390/ijms21093115
Barshes, N. R., Goodpastor, S. E., and Goss, J. A. (2004). Pharmacologic immunosuppression. Front. Biosci. 9, 411–420. doi:10.2741/1249
Beery, T. A. (2003). Sex differences in infection and sepsis. Crit. Care. Nurs. Clin. North. Am. 15 (1), 55–62. doi:10.1016/s0899-5885(02)00028-x
Chotiyarnwong, P., and McCloskey, E. V. (2020). Pathogenesis of glucocorticoid-induced osteoporosis and options for treatment. Nat. Rev. Endocrinol. 16 (8), 437–447. doi:10.1038/s41574-020-0341-0
Evens, A. M., Jovanovic, B. D., Su, Y. C., Raisch, D. W., Ganger, D., Belknap, S. M., et al. (2011). Rituximab-associated hepatitis B virus (HBV) reactivation in lymphoproliferative diseases: meta-analysis and examination of FDA safety reports. Ann. Oncol. 22 (5), 1170–1180. doi:10.1093/annonc/mdq583
Fanouriakis, A., Kostopoulou, M., Alunno, A., Aringer, M., Bajema, I., Boletis, J. N., et al. (2019). 2019 update of the EULAR recommendations for the management of systemic lupus erythematosus. Ann. Rheum. Dis. 78 (6), 736–745. doi:10.1136/annrheumdis-2019-215089
Fujii, M., Kuwabara, Y., Kinjo, A., Imamoto, A., Jike, M., Otsuka, Y., et al. (2021). Trends in the co-use of alcohol and tobacco among Japanese adolescents: periodical nationwide cross-sectional surveys 1996-2017. BMJ Open 11 (8), e045063. doi:10.1136/bmjopen-2020-045063
Godbole, G., and Gant, V. (2013). Respiratory tract infections in the immunocompromised. Curr. Opin. Pulm. Med. 19 (3), 244–250. doi:10.1097/MCP.0b013e32835f82a9
Hepp, T., Schmid, M., Gefeller, O., Waldmann, E., and Mayr, A. (2016). Approaches to regularized regression - a comparison between gradient boosting and the lasso. Methods. Inf. Med. 55 (5), 422–430. doi:10.3414/ME16-01-0033
High, E. J., and Olivry, T. (2020). The prevalence of bacterial infections during cyclosporine therapy in dogs: a critically appraised topic. Can. Vet. J. 61 (12), 1283–1289.
Htwe, T. H., and Khardori, N. M. (2017). Legionnaire's disease and immunosuppressive drugs. Infect. Dis. Clin. North. Am. 31 (1), 29–42. doi:10.1016/j.idc.2016.10.003
Iasonos, A., Schrag, D., Raj, G. V., and Panageas, K. S. (2008). How to build and interpret a nomogram for cancer prognosis. J. Clin. Oncol. 26 (8), 1364–1370. doi:10.1200/JCO.2007.12.9791
Kalil, A. C., Metersky, M. L., Klompas, M., Muscedere, J., Sweeney, D. A., Palmer, L. B., et al. (2016). Management of adults with hospital-acquired and ventilator-associated pneumonia: 2016 clinical practice guidelines by the infectious diseases society of America and the American thoracic society. Clin. Infect. Dis. 63 (5), e61–e111. doi:10.1093/cid/ciw353
Kasiske, B. L., Zeier, M. G., Chapman, J. R., Craig, J. C., Ekberg, H., Garvey, C. A., et al. (2010). KDIGO clinical practice guideline for the care of kidney transplant recipients: a summary. Kidney. Int. 77 (4), 299–311. doi:10.1038/ki.2009.377
Kelesidis, T., Daikos, G., Boumpas, D., and Tsiodras, S. (2011). Does rituximab increase the incidence of infectious complications? A narrative review. Int. J. Infect. Dis. 15 (1), e2–e16. doi:10.1016/j.ijid.2010.03.025
Klekotka, R. B., Mizgała, E., and Król, W. (2015). The etiology of lower respiratory tract infections in people with diabetes. Pneumonol. Alergol. Pol. 83 (5), 401–408. doi:10.5603/PiAP.2015.0065
Koenig, S. M., and Truwit, J. D. (2006). Ventilator-associated pneumonia: diagnosis, treatment, and prevention. Clin. Microbiol. Rev. 19 (4), 637–657. doi:10.1128/CMR.00051-05
Larbi, A., Franceschi, C., Mazzatti, D., Solana, R., Wikby, A., and Pawelec, G. (2008). Aging of the immune system as a prognostic factor for human longevity. Physiol. (Bethesda) 23, 64–74. doi:10.1152/physiol.00040.2007
Levy, A., Fraser, D., Rosen, S. D., Dagan, R., Deckelbaum, R. J., Coles, C., et al. (2005). Anemia as a risk factor for infectious diseases in infants and toddlers: results from a prospective study. Eur. J. Epidemiol. 20 (3), 277–284. doi:10.1007/s10654-004-6515-6
McEwen, B. S. (2008). Central effects of stress hormones in health and disease: understanding the protective and damaging effects of stress and stress mediators. Eur. J. Pharmacol. 583 (2-3), 174–185. doi:10.1016/j.ejphar.2007.11.071
Penfornis, A., and Kury-Paulin, S. (2006). Immunosuppressive drug-induced diabetes. Diabetes. Metab. 32 (5 Pt 2), 539–546. doi:10.1016/s1262-3636(06)72809-9
Ponte, D. F., Moraes, R., Hizume, D. C., and Alencar, A. M. (2013). Characterization of crackles from patients with fibrosis, heart failure and pneumonia. Med. Eng. Phys. 35 (4), 448–456. doi:10.1016/j.medengphy.2012.06.009
Poowuttikul, P., Taki, M., Daher, R., and Secord, E. (2019). T cell profile after systemic steroid burst in inner-city asthmatic children with recurrent infections. Pediatr. Allergy. Immunol. Pulmonol. 32 (2), 56–62. doi:10.1089/ped.2018.0988
Radhakrishnan, J., and Cattran, D. C. (2012). The KDIGO practice guideline on glomerulonephritis: reading between the (guide)lines--application to the individual patient. Kidney. Int. 82 (8), 840–856. doi:10.1038/ki.2012.280
Reis e Sousa, C. (2001). Dendritic cells as sensors of infection. Immunity 14 (5), 495–498. doi:10.1016/s1074-7613(01)00136-4
Shrestha, G., Phuyal, P., Gautam, R., Mulmi, R., and Pradhan, P. M. S. (2021). Burden of tobacco in Nepal: a systematic analysis from the global burden of disease study 1990-2017. BMJ Open 11 (8), e047847. doi:10.1136/bmjopen-2020-047847
Stahn, C., and Buttgereit, F. (2008). Genomic and nongenomic effects of glucocorticoids. Nat. Clin. Pract. Rheumatol. 4 (10), 525–533. doi:10.1038/ncprheum0898
Sułkowska, K., Palczewski, P., Miszewska-Szyszkowska, D., Durlik, M., Gołębiowski, M., and Małkowski, P. (2012). Early everolimus-induced pneumonitis in a renal transplant recipient: a case report. Ann. Transpl. 17 (4), 144–148. doi:10.12659/aot.883706
Suthanthiran, M., Morris, R. E., and Strom, T. B. (1996). Immunosuppressants: cellular and molecular mechanisms of action. Am. J. Kidney. Dis. 28 (2), 159–172. doi:10.1016/s0272-6386(96)90297-8
Thaunat, O., Morelon, E., Stern, M., Buffet, P., Offredo, C., Mamzer-Bruneel, M. F., et al. (2004). Mycobacterium xenopi pulmonary infection in two renal transplant recipients under sirolimus therapy. Transpl. Infect. Dis. 6 (4), 179–182. doi:10.1111/j.1399-3062.2004.00071.x
Wang, T., Zhang, Y., Ping, F., Zhao, H., Yan, L., Lin, Q., et al. (2019). Predicting risk of pulmonary infection in patients with primary membranous nephropathy on immunosuppressive therapy: the AIM-7C score. Nephrol. Carlt. 24 (10), 1009–1016. doi:10.1111/nep.13544
World Medical Association (2013). World Medical Association Declaration of Helsinki: ethical principles for medical research involving human subjects. JAMA 310 (20), 2191–2194. doi:10.1001/jama.2013.281053
Wunderink, R. G., Woldenberg, L. S., Zeiss, J., Day, C. M., Ciemins, J., and Lacher, D. A. (1992). The radiologic diagnosis of autopsy-proven ventilator-associated pneumonia. Chest 101 (2), 458–463. doi:10.1378/chest.101.2.458
Xie, D., Marks, R., Zhang, M., Jiang, G., Jatoi, A., Garces, Y. I., et al. (2015). Nomograms predict overall survival for patients with small-cell lung cancer incorporating pretreatment peripheral blood markers. J. Thorac. Oncol. 10 (8), 1213–1220. doi:10.1097/JTO.0000000000000585
Keywords: immunosuppressive drugs, LASSO, nomogram, pulmonary infection, predictive model
Citation: Luo C, Zhang Y, Zhang J, Jin C, Ye X, Ren Y, Shen H, Chen M, Li Y, He Q, Xu G and Shao L (2024) Development and validation of a nomogram for predicting pulmonary infection in patients receiving immunosuppressive drugs. Front. Pharmacol. 14:1255609. doi: 10.3389/fphar.2023.1255609
Received: 09 July 2023; Accepted: 31 December 2023;
Published: 16 January 2024.
Edited by:
Zhenyu Pan, Xi’an Children’s Hospital, ChinaReviewed by:
Xiao Li, Shandong Provincial Qianfoshan Hospital, ChinaCopyright © 2024 Luo, Zhang, Zhang, Jin, Ye, Ren, Shen, Chen, Li, He, Xu and Shao. This is an open-access article distributed under the terms of the Creative Commons Attribution License (CC BY). The use, distribution or reproduction in other forums is permitted, provided the original author(s) and the copyright owner(s) are credited and that the original publication in this journal is cited, in accordance with accepted academic practice. No use, distribution or reproduction is permitted which does not comply with these terms.
*Correspondence: Lina Shao, c2hhb2xpbmFAaG1jLmVkdS5jbg==; Guangbiao Xu, eGdiMDExMEAxMjYuY29t; Qiang He, cWlhbmdoZTE5NzNAMTI2LmNvbQ==
Disclaimer: All claims expressed in this article are solely those of the authors and do not necessarily represent those of their affiliated organizations, or those of the publisher, the editors and the reviewers. Any product that may be evaluated in this article or claim that may be made by its manufacturer is not guaranteed or endorsed by the publisher.
Research integrity at Frontiers
Learn more about the work of our research integrity team to safeguard the quality of each article we publish.