- 1Department of Neurology, The First Affiliated Hospital of Zhengzhou University, Zhengzhou, China
- 2Department of Pharmacy, Wuhan Children’s Hospital, Tongji Medical College, Huazhong University of Science and Technology, Wuhan, Hubei, China
- 3Department of Pharmacy, Huashan Hospital, Fudan University, Shanghai, China
- 4Department of Pediatrics, The First Affiliated Hospital of Zhengzhou University, Zhengzhou, China
- 5Department of Pharmacy, The First Affiliated Hospital of Zhengzhou University, Zhengzhou, China
Background: Several studies have investigated the population pharmacokinetics (popPK) of valproic acid (VPA) in children with epilepsy. However, the predictive performance of these models in the extrapolation to other clinical environments has not been studied. Hence, this study evaluated the predictive abilities of pediatric popPK models of VPA and identified the potential effects of protein binding modeling strategies.
Methods: A dataset of 255 trough concentrations in 202 children with epilepsy was analyzed to assess the predictive performance of qualified models, following literature review. The evaluation of external predictive ability was conducted by prediction- and simulation-based diagnostics as well as Bayesian forecasting. Furthermore, five popPK models with different protein binding modeling strategies were developed to investigate the discrepancy among the one-binding site model, Langmuir equation, dose-dependent maximum effect model, linear non-saturable binding equation and the simple exponent model on model predictive ability.
Results: Ten popPK models were identified in the literature. Co-medication, body weight, daily dose, and age were the four most commonly involved covariates influencing VPA clearance. The model proposed by Serrano et al. showed the best performance with a median prediction error (MDPE) of 1.40%, median absolute prediction error (MAPE) of 17.38%, and percentages of PE within 20% (F20, 55.69%) and 30% (F30, 76.47%). However, all models performed inadequately in terms of the simulation-based normalized prediction distribution error, indicating unsatisfactory normality. Bayesian forecasting enhanced predictive performance, as prior observations were available. More prior observations are needed for model predictability to reach a stable state. The linear non-saturable binding equation had a higher predictive value than other protein binding models.
Conclusion: The predictive abilities of most popPK models of VPA in children with epilepsy were unsatisfactory. The linear non-saturable binding equation is more suitable for modeling non-linearity. Moreover, Bayesian forecasting with prior observations improved model fitness.
1 Introduction
Epilepsy is a common chronic neurological disorder in pediatrics that burdens patients with huge biological, psychological, and social hardship and requires long-term antiseizure medications (ASMs) therapy (Moshe et al., 2015; Patsalos et al., 2018). As a first-line ASM for both focal and generalized epilepsy syndromes and seizures in male children, the advantages of valproic acid (VPA) include a wide spectrum of antiepileptic properties and an acceptable tolerability profile (Johannessen Landmark and Svein, 2016; Romoli et al., 2019).
The oral bioavailability of VPA is almost complete in all commonly prescribed formulations (Romoli et al., 2019). VPA exhibits high (90%–95%), concentration-dependent, and saturable plasma protein-binding (Kodama et al., 1992a; Johannessen and Johannessen, 2003), resulting in non-linear pharmacokinetics (PK) (Zaccara et al., 1988). VPA is mainly metabolized through glucuronidation via uridine diphosphate glucuronosyltransferase (UGT), β-oxidation in the mitochondria, and cytochrome P450 (CYP)-catalyzed oxidation in the liver. Ultimately, the metabolites are mainly excreted in the urine (Silva et al., 2008; Romoli et al., 2019).
In clinical practice, the clearance (CL/F) and plasma concentrations of VPA vary greatly among individuals, which can be primarily ascribed to demographic and clinical characteristics, concomitant medications, and genetic variants (Ghodke-Puranik et al., 2013; Zhu et al., 2017). Owing to the narrow therapeutic range (50–100 mg/L) and considerable interpatient PK variability of VPA, close therapeutic drug monitoring (TDM) and individualized medication are indispensable (Patsalos et al., 2008; Johannessen Landmark et al., 2020).
Individual PK parameters required for optimizing dosage regimens can be obtained using population pharmacokinetic (popPK) modeling combined with Bayesian forecasting (Sheiner et al., 1979). Unlike traditional PK approaches, popPK analysis is superior in estimating intra- and inter-subject variabilities and predicting plasma concentrations by quantifying the effects of relevant covariates on PK parameters (Sun et al., 1999; Ette and Williams, 2004). This method allows the use of sparse TDM data and is suitable for studies in children due to logistical and ethical constraints with respect to performing intensive sampling strategies in this vulnerable population.
Several popPK models have been established to quantitatively explore the PK characteristics of VPA (Methaneethorn, 2018; Zang et al., 2022). Body weight, sex, age, VPA daily dose, and concomitant medications have been most frequently reported as covariates that influence VPA CL/F. Approximately one-third of the studies have assessed the predictive performances of the final models. The predictive abilities of VPA popPK models for patients with bipolar disorder in China (Zang et al., 2022) and patients with mania in Thailand (Methaneethorn and Leelakanok, 2021) have been externally evaluated. However, popPK models and the influence of incorporating non-linear properties on the model’s predictive ability for children with epilepsy have not been evaluated. The modeling strategy and functional forms of the covariates involved may affect the model’s predictive ability (Mao et al., 2020).
VPA concentrations are disproportionately higher when protein binding is saturated, particularly as the concentrations of VPA exceed 50 mg/L (Winter, 2010). To investigate the non-linear relationship of VPA concentrations between the total and free serum, a one-binding site model (Dutta et al., 2007), Langmuir equation (Ueshima et al., 2008), dose-dependent maximum effect model (Ding et al., 2015), linear non-saturable binding equation (Gu et al., 2021), and the VPA daily dose incorporated as a covariate have been used in modeling development. However, most published models have been developed using empirical covariate selection and have not considered non-linear properties. Theoretical covariate selection, which is based on the understanding of PK mechanisms rather than solely on the data, combined with the relationships between covariates and parameters, may help improve model prediction (Danhof et al., 2008; Mao et al., 2020).
To fully exploit the benefits of model-informed precision dosing, the most suitable popPK model that can accurately describe the PK process in the target population should be obtained (Kluwe et al., 2020). This study aimed to systematically assess the predictive performance of published popPK models for VPA in children with epilepsy as well as explore the potential effects of protein binding modeling strategies on model transferability.
2 Materials and methods
2.1 Review of studies on popPK analyses of VPA in children with epilepsy
A systematic review of studies on the popPK of VPA in children with epilepsy was conducted by retrieving literature from PubMed, Web of Science, and Embase from inception up to 30 June 2022. The criteria for inclusion in published models were as follows: (1) a study using a non-linear mixed effect modeling approach to analyze VPA PK parameters in children with epilepsy, and (2) publications written in English. Studies were excluded if (1) they were methodological papers or reviews, (2) the required information was insufficient for external evaluation, and (3) the data or cohorts overlapped. In addition, popPK studies, including genetic polymorphisms as covariates, were excluded as genotyping is not routinely performed in TDM of VPA. Furthermore, citations in the identified publications were screened.
2.2 Data for external evaluation
2.2.1 Participants
Overall, 202 Chinese children with epilepsy (139 males and 63 females) undergoing VPA treatment and who had undergone routine monitoring of plasma concentrations at Wuhan Children’s Hospital between January 2016 and November 2018 were eligible. The clinical and demographic characteristics of each participant, including sex, age, body weight (BW), dosage regimen, concomitant drugs, and seizure control, were obtained at each TDM visit for the current evaluation. Patients with missing information on the required covariates, hepatic or renal impairment, abnormal albumin levels, or poor medication adherence were excluded. The Ethics Committee of Wuhan Children’s Hospital approved the protocol (Serial Number: 2015015), and patients’ direct relatives were well informed and signed informed consent declarations voluntarily.
2.2.2 VPA concentration determination
VPA total plasma concentrations were measured by gas chromatography (GC). The calibration range of the hydrogen flame ionization detector was 12.5–150 mg/L. The detection limit was 1 mg/L, and the coefficient of variation was below 10%, as reported in an earlier study (Liu et al., 2020).
2.3 External predictive ability evaluation
The assessment of predictive ability was conducted with NONMEM® 7.4 (ICON Development Solutions, Ellicott City, MD, United States) and assisted by Intel Fortran XE 2011 Update 13 (Intel Corp, Santa Clara, CA, United States). We reconstructed published popPK models by incorporating reported parameters and subsequently assessed the predictive performance of the eligible models using prediction- and simulation-based diagnostics, as well as Bayesian forecasting (Zhao et al., 2016a; Mao et al., 2018), with an external dataset. To analyze the output of NONMEM, R software (version 4.2.1, http://www.r-project.org/) was used.
2.3.1 Prediction-based diagnostics
For each participant, we calculated the prediction error (PE%) using population predictions (PREDs) and corresponding observations (OBS) according to Eq. 1. To evaluate the predictive accuracy and precision, median prediction error (MDPE) and median absolute prediction error (MAPE) were used (Sheiner and Beal, 1981).
Subsequently, we calculated the PE% within ±20% (F20) and ±30% (F30) to serve as an indicator of the combined performance of model accuracy and precision. The candidate model’s predictive ability was considered to be satisfactory if the following criteria were met: MDPE ≤ ± 15%, MAPE ≤30%, F20 > 35%, and F30 > 50% (Mao et al., 2018). The PE% was visualized using boxplots and cumulative distribution curve plots.
2.3.2 Simulation-based diagnostics
The evaluation and simulated data were statistically compared via a prediction-corrected visual predictive check (pcVPC) (Bergstrand et al., 2011) and normalized prediction distribution error (NPDE) (Comets et al., 2008) to assess each candidate model’s predictive ability for VPA via simulation. Based on the reported final model parameters, 2,000 times simulations of the dataset were carried out.
The graphical visualizations and calculations for pcVPC were conducted using PsN. The comparisons between the simulations and the observations at different time points were performed in pcVPCs using automatic binning. The NPDE was determined using an R package (NPDE, version 2.0, www.npde.biostat.fr) (Comets et al., 2008). To test the normal distribution property of NPDE data, diagnostic graphs were generated. Statistical tests were conducted with the null hypothesis, and the derived model satisfactorily accounted for the evaluation data. The hypothesis was examined with the Wilcoxon signed-rank test, Fisher’s exact variance test, and Shapiro-Wilk test, as appropriate (Brendel et al., 2010).
2.3.3 Bayesian forecasting
To assess the impact of priors on model predictive performance, maximum a posteriori Bayesian (MAPB) forecasting using data from individuals with observed concentrations was performed. For each patient, one prior measurement was used to predict the individual prediction (IPRED) of the last observation, and the individual PE% (IPE%) was calculated using Eq. 2 to assess the model’s accuracy. Further details are shown in Supplementary Text S1.
The median IPE% (MDIPE), median absolute IPE% (MAIPE), and IF20 and IF30 (representing F20 and F30 of IPE%, respectively) were calculated to assess the model’s predictive performance with increasing prior information.
2.4 The impact of protein binding modeling strategy
VPA is known to follow non-linear PK based on concentration-dependent protein binding (Zaccara et al., 1988). Based on the review of literature and data characteristics, a one-compartment model with first-order absorption was used as base model to describe VPA PK. Subsequently, five protein-binding modeling strategies (Eqs 3–7) were applied to compare the potential effect of functional forms and the non-linearity of covariates on the model’s predictive performance.
Model I: One-binding site model (Eq. 3)
where Cb and Cu represent the bound and unbound plasma concentrations of VPA, respectively, and ALB is the albumin level. The number of binding sites (N) per unit of single-site binding material was set as 1.98 while the binding site association constant (K) was set to 15.5 mM−1 as reported (Dutta et al., 2007).
Model II: Langmuir equation (Eq. 4)
The dissociation constant of VPA (Kd) and maximum binding site concentration (Bm) were set to 7.8 and 130 mg/L, respectively (Ueshima et al., 2008).
Model III: Dose-dependent maximum effect model (Eq. 5)
where CLp is the apparent plasma clearance, Emax is the maximum effect of VPA, DD50 is the daily dose (mg kg−1 day−1) when Emax is increased by 50%, and the sigmoid decline slope is specified by the Hill coefficient (γ). Emax and γ were set to 2.8 and 1.68, respectively, as reported (Ding et al., 2015).
Model IV: Linear non-saturable binding equation (Eq. 6)
where NS is the slope of non-saturable protein-binding. Kd, Bm, and NS were set to 2.12, 67.3 mg/L, and 2.25, respectively (Gu et al., 2021).
Model V: The simple exponent model (Eq. 7)
where k is the exponent of the daily dose, which was estimated using our dataset.
The evaluation approaches comprise the aforementioned diagnostic methods based on prediction and simulation as well as Bayesian forecasting methods. The tendency plots based on established models Ⅲ and Ⅴ were used to assess the relation of the daily dose to CL/F within these two modeling strategies.
3 Results
3.1 Review of published popPK analyses on VPA in children with epilepsy
After literature retrieval (Figure 1; Supplementary Text S2), 10 published popPK studies on VPA in children with epilepsy were identified for external evaluation (Serrano et al., 1999; Desoky et al., 2004; Jiang et al., 2007; Correa et al., 2008; Williams et al., 2012; Ogusu et al., 2014; Ding et al., 2015; Rodrigues et al., 2018; Gu et al., 2021; Teixeira-da-Silva et al., 2022). Four studies were conducted in East Asian countries [three in China (Jiang et al., 2007; Ding et al., 2015; Gu et al., 2021) and one in Japan (Ogusu et al., 2014)], three in Europe [two in Spain (Serrano et al., 1999; Teixeira-da-Silva et al., 2022) and one in France (Rodrigues et al., 2018)], two in North America [one each in Mexico (Correa et al., 2008) and United States (Williams et al., 2012)], and one in Africa (Desoky et al., 2004). Among these studies, four were multi-center studies (Jiang et al., 2007; Williams et al., 2012; Ding et al., 2015; Rodrigues et al., 2018) and the others were single-center studies. Additionally, three studies used sample size of ≤100 participants (Desoky et al., 2004; Williams et al., 2012; Rodrigues et al., 2018).
Four bioassays were used in specific publications. Specifically, fluorescence polarization immunoassays (FPIA) were used in seven studies (Serrano et al., 1999; Desoky et al., 2004; Jiang et al., 2007; Williams et al., 2012; Ding et al., 2015; Rodrigues et al., 2018; Teixeira-da-Silva et al., 2022), enzyme multiplied immunoassay technique (EMIT), cloned enzyme donor immunoassays (CEDIAs), and GC were used in the remaining three studies (Correa et al., 2008; Ogusu et al., 2014; Gu et al., 2021) (Table 1). The demographic and pharmacokinetic characteristics of the models are listed in Supplementary Table S1.
Most studies described VPA PK using a one-compartment (1CMT) model with first-order absorption (Serrano et al., 1999; Desoky et al., 2004; Jiang et al., 2007; Correa et al., 2008; Ogusu et al., 2014; Ding et al., 2015; Rodrigues et al., 2018; Gu et al., 2021; Teixeira-da-Silva et al., 2022), while only one study reported a two-compartment (2CMT) model (Williams et al., 2012). Three models were established with data from both pediatric and adult patients (Desoky et al., 2004; Ogusu et al., 2014; Teixeira-da-Silva et al., 2022). Six studies considered the effects of the formulation on the absorption rate (Desoky et al., 2004; Jiang et al., 2007; Williams et al., 2012; Ding et al., 2015; Gu et al., 2021; Teixeira-da-Silva et al., 2022), of which most used a fixed absorption rate constant (ka) (Desoky et al., 2004; Williams et al., 2012; Ding et al., 2015; Gu et al., 2021; Teixeira-da-Silva et al., 2022). The four covariates that most frequently influenced VPA CL/F were concomitant medication intake, BW, daily dose, and age, accounting for 80.0%, 70.0%, 50.0%, and 50.0% of the studies, respectively. The most commonly reported co-medications were carbamazepine, phenobarbital, phenytoin, and lamotrigine.
3.2 External predictive ability evaluation
3.2.1 Participants
A total of 202 volunteers who met the inclusion and exclusion criteria were included, providing 255 VPA concentrations for analysis. Table 2 shows the demographic and clinical characteristics of all participants. Age, BW, VPA daily dose, and serum concentrations were 4.92 (0.17–15.00) years, 19.00 (4.00–70.00) kg, 23.40 (8.70–49.20) mg/kg/day, and 50.40 (22.60–118.50) µg/mL, respectively. A sustained-release formulation or a syrup was administered orally one, two, or three times per day. Trough concentrations were calculated under steady-state conditions. The three most commonly prescribed co-medications in our cohort were levetiracetam, oxcarbazepine, and topiramate.
3.2.2 Prediction-based diagnostics
The prediction-based diagnostic results are listed in Table 3. The models proposed by Serrano et al. (1999), Desoky et al. (2004), Correa et al. (2008), Ogusu et al. (2014), Gu et al. (2021), and Teixeira-da-Silva et al. (2022) met the criteria mentioned above (MDPE ≤ ± 15%, MAPE ≤30%, F20 > 35%, and F30 > 50%). The model proposed by Serrano et al. (1999) (MDPE, 1.40%; MAPE, 17.38%; F20, 55.69%; F30, 76.47%) performed the best. The predictive abilities of these models are displayed in box plots (Figure 2) and cumulative distribution curve plots (Figure 3).
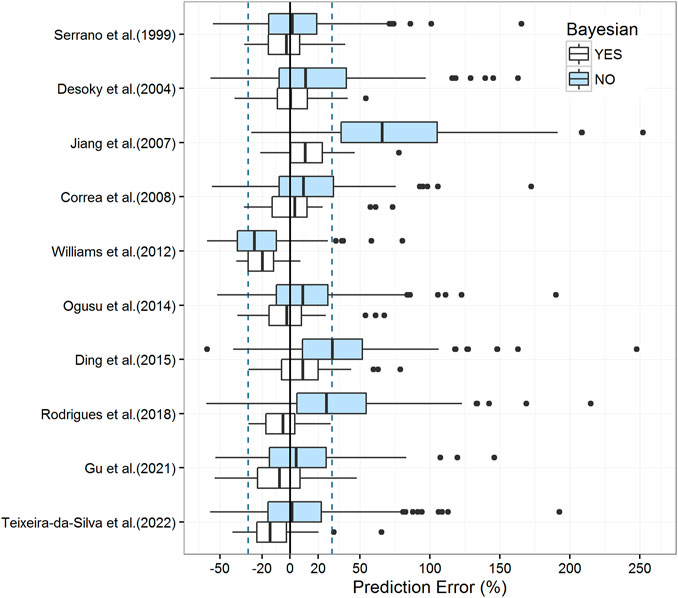
FIGURE 2. Box plots of the prediction error with or without Bayesian forecasting for published population pharmacokinetic models. The blue box represents predictions without prior information, while the white box represents predictions with one prior observation. Black solid lines and blue dotted lines are reference lines indicating PE% of 0% and ±30%, respectively.
3.2.3 Simulation-based diagnostics
The results of pcVPC indicated a significant deviation between observations and simulations among the most involved models (Supplementary Figure S1). A clear pattern of over- or under-prediction of true variability among subjects was observed, except in the relatively superior model of prediction-based diagnostics developed by Serrano et al. (1999). However, the NPDE results of the model proposed by Serrano et al. (1999) were not as accurate as those of pcVPC, which failed to obey a normal distribution, especially for the description of variance. For the global test (Supplementary Table S2), all models were statistically rejected (p-values >0.05). The NPDE results are shown in Supplementary Figure S2 and Supplementary Table S2.
3.2.4 Bayesian forecasting
In Bayesian forecasting, the prediction accuracy was substantially enhanced by one prior observation in most models (Figure 2; Table 3), which highlighted the usefulness of popPK modeling combined with Bayesian estimation in VPA dosage adjustments. Due to the limitation of the sample size, the Bayesian forecasting results of two or three prior observations were not available in this study. As model predictability reaches a stable state after two or three prior observations (Zhao et al., 2016a; Mao et al., 2018), this limitation might have caused fluctuation in model accuracy, such as those observed for models proposed by Gu et al. (2021) and Teixeira-da-Silva et al. (2022).
3.3 The impact of protein binding modeling strategy
The estimated parameters of the five protein-binding modeling strategies based on our evaluation dataset are shown in Supplementary Table S3. The objective function value decreased dramatically as non-linearity was involved in modeling, except in the dose-dependent maximum effect model. Moreover, the aforementioned assessments were employed to evaluate the predictive ability of these models. Results for these metrics are presented in Table 3 and Figure 4.
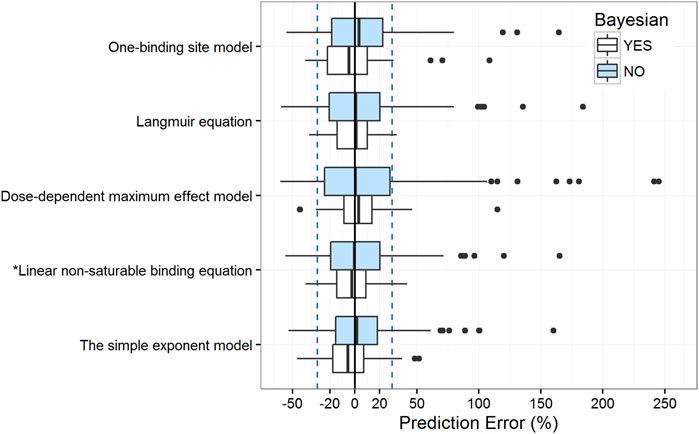
FIGURE 4. Box plots of the prediction error with or without Bayesian forecasting for five protein binding models. The blue box represents predictions without prior information, while the white box represents predictions with one prior observation. Black solid lines and blue dotted lines are reference lines indicating PE% of 0% and ±30%, respectively. The model with an asterisk (*) performed the best.
The results of the prediction-based diagnostics showed that the linear non-saturable binding equation and the simple exponent model performed better than the other models. After Bayesian forecasting, the prediction accuracy improved substantially with one prior observation. The simulation-based diagnostics (Supplementary Table S2, Supplementary Figures S3, S4) indicated that more covariates should be identified to quantify the variabilities.
Although the simple exponent model had a satisfactory predictive ability, the equation did not describe the non-linear properties of the VPA PK process (Supplementary Figure S5). According to the tendency plot results, the dose-dependent maximum effect model describes the non-linear property of the VPA PK process. However, regarding the empirical-estimated parameters and center-related variability, the dose-dependent maximum effect model did not had a satisfactory simulation-based predictive ability. Therefore, the linear non-saturable binding equation is more suitable for modeling the non-linear kinetics of VPA according to the saturation of protein-binding.
4 Discussion
Although several studies have reported popPK characteristics in children with epilepsy, the predictive ability of these models have not been fully assessed. In this study, we systematically evaluated 10 published popPK models in children with epilepsy. Although six models met the criteria (MDPE ≤ ± 15%, MAPE ≤30%, F20 > 35%, and F30 > 50%) in prediction-based diagnostics, large variabilities existed in simulation-based diagnostics, indicating the discrepancies across centers, especially for variance. With prior observations available, the performance of popPK models significantly improves, indicating that Bayesian forecasting substantially improves the prediction accuracy of the popPK model (Zhao et al., 2016a; Mao et al., 2018; Cai et al., 2020).
Given the similarity of participants’ race, prescription regimen, dietary habits, and genetics, models established in populations with similar evaluation datasets might have superior predictive ability. However, models developed for East Asians (Jiang et al., 2007; Ding et al., 2015) did not show any advantages in the evaluation. The typical CL/F values in those studies were 0.18 and 0.24 L/h, respectively, which are lower than that of our base model (0.31 L/h), resulting in an overestimation of concentration. Moreover, the best method for prediction-based diagnostics and simulation-based pcVPC was performed in Spain, with 255 patients and 770 samples (Serrano et al., 1999). Indeed, no obvious relationship between superior predictive performance and sample size or ethnicity was observed in our study.
VPA is highly bind to plasma proteins, and the binding sites can be saturated as total VPA concentration increases, thus following non-linear PK. To capture this phenomenon, five candidate studies included dose as a covariate in the models to characterize the non-linear relationship between VPA dose and CL/F (Serrano et al., 1999; Desoky et al., 2004; Correa et al., 2008; Ogusu et al., 2014; Ding et al., 2015). Most of them chose a simple exponent model (Serrano et al., 1999; Desoky et al., 2004; Correa et al., 2008; Ogusu et al., 2014), while only a study by Ding et al. (2015) proposed a dose-dependent maximum effect (DDE) model.
Moreover, the incorporation of the VPA daily dose is controversial with regard to its influence on CL/F. In patients with a higher clearance rate, lower drug concentrations may be present. Therefore, they require higher TDM-guided doses. Regarding the complicated TDM effect, the simple exponential model may not be suitable for describing non-linear PK profiles (Ahn et al., 2005). In addition, the simple exponent model was insufficient in describing the non-linear correlation between the daily dose and CL/F as indicated by the tendency plot results. Therefore, although model predictive ability may be improved when considering the effect of the daily dose, it is not a suitable strategy for modeling PK non-linearity by adding daily doses to CL/F (Vucicevic et al., 2009; Teixeira-da-Silva et al., 2022). In fact, as the VPA daily dose was the goal of prediction, it should not be used as a covariate for prediction.
Regardless of whether the DDE model or the simple exponent model were data-driven empirical models, the transferability of these models may be influenced by center-related factors, which might be partly due to the differences in study design (such as age and dosage regimen) and retained covariates between different clinical sites. Adding covariates based on the mechanisms of PK processes may assist in improvement of a model’s predictive ability (Danhof et al., 2008). In addition, only free VPA can access the central nervous system, where its pharmacological action occurs. Therefore, the estimation of the free VPA concentration based on the understanding of serum protein binding is essential (Kodama et al., 1992b). However, as a low extraction ratio drug, the effective concentration of VPA does not only depend on protein binding, but also on the intrinsic ability of the eliminating organ. Saturation or competition would result in dose-normalized reduction of the total concentration whereas the free concentration may remain unchanged (Benet and Hoener, 2002).
The one-binding site model (Dutta et al., 2007), Langmuir equation (Ueshima et al., 2008), and linear non-saturable binding equation (Gu et al., 2021) have been built based on the understanding of the non-linear relationship between the total and free serum concentrations of VPA. Age, ultrafiltration temperature, albumin level, and the existence of various metabolites affect VPA binding in a population of patients (Kodama et al., 1992b). Age is positively correlated with the VPA free fraction (Zaccara et al., 1988). Therefore, a one-binding site model built on adult patients may not be suitable for the pediatric population. The linear non-saturable binding equation incorporating the slope of non-saturable protein binding may be superior to the Langmuir equation, which has been confirmed in a previous study (Gu et al., 2021).
BW is a body size indicator associated with the functionality of the liver, which is responsible for VPA metabolism. It is one of the most common covariates considered in final models, accounting for nearly 62.5% of candidate studies (Xu et al., 2018). Although the 3/4 allometric exponent model has been universally applied to scale clearance (Anderson et al., 2007; Holford et al., 2013), the value of 0.75 in this approach might not be reliable for estimating clearance in pediatric patients (Mahmood, 2006; Peeters et al., 2010). Moreover, age is an important maturation marker, and some studies have shown that VPA CL/F varies with age in children (Chiba et al., 1985; Cloyd et al., 1993). However, age was not included in half of the selected models, including the superior model proposed by Serrano et al. (1999).
Both age and BW reportedly determine maturation in children <2 years old, whereas BW is the most important factor influencing CL/F in children ≥2 years old (Ding et al., 2015; Gu et al., 2021). The age-dependence of PK in young children may be partially due to the maturation of UGT enzymes that mediate VPA elimination. The activities of UGT1A9 and UGT1A6 reach adult levels at 2 years and 14 months of age, respectively (Choonara et al., 1989; Miyagi and Collier, 2011). The ability of another UGT enzyme involved in VPA elimination, UGT2B7, reaches adult levels between two and 6 months of age (Ebner and Burchell, 1993). The median age in each of the studies analyzed here was above 2 years. That explains why BW was the most common covariate in the final models.
In addition, several other center-based factors could have resulted in inter-study variability and affected the model’s predictive performance. The VPA bioassay is one of the most frequently mentioned factors. Three immunoassay methods were used in the candidate publications, including FPIA, EMIT, and CEDIA, whereas a GC technique was used for our dataset. The analytical performance of these immunoassays was reported to be practically equivalent to that of chromatographic methods (Johannessen and Johannessen, 2003). Correlation coefficients for ultraperformance liquid chromatography mass spectrometry versus FPIA, and high-performance liquid chromatography has been reported as 0.989, and 0.987, respectively; Bland-Altman analysis has also shown these methods to be comparable (Zhao et al., 2016b), indicating their agreement. Although EMIT overestimates VPA levels compared with chromatographic methods (Xia et al., 2021), no clear correlation between model predictive ability and bioassay method was observed (Zang et al., 2022).
Pharmacogenetics may also contribute to drug PK variability. VPA metabolism is related to several metabolic enzymes and transporters, including UGTs, CYPs, ATP-binding cassette (ABC) transporters, and monocarboxylate transporters (MCTs). Genetic polymorphisms of these enzymes and transporters may influence the PKs and VPA concentrations (Hung et al., 2011; Mei et al., 2018). However, few researchers have regarded polymorphisms as covariates of the popPK characteristics of VPA (Jiang et al., 2009; Mei et al., 2018; Xu et al., 2018), and the findings remain controversial in pediatric patients. In our previous study, 11 single-nucleotide polymorphisms in UGT2B7, UGT1A6, and CYP2C9 were investigated, revealing no significant influence of any of them on VPA responsiveness (Liu et al., 2020). As pharmacogenomic considerations have not been verified in clinical practice, genotyping is not routinely performed in VPA therapy, and genetic polymorphisms were not available in a part of our external evaluation dataset. The role of pharmacogenetics in model predictive ability was not explored in this work, and needs further clarification in future research, specifically in children.
This study has several limitations. First, the external dataset consisted of a fraction of participants (202 children) from a single center, which could limit statistical power. In addition, the collected data were mostly at trough concentrations; therefore, parameters for the absorption and distribution stages could not be obtained precisely. Furthermore, among the candidate studies, five reported traditional ASMs, such as phenobarbital, phenytoin, and carbamazepine, have enzymatic induction effects that can enhance VPA metabolism in children. However, novel ASMs (levetiracetam, oxcarbazepine, and topiramate) had been commonly prescribed for polytherapy in our population. Owing to the low proportion of patients treated concomitantly with classical enzyme inducers, drug-drug interactions were applied as a predictive factor, which may have led to misspecification to some extent. Furthermore, the inconsistency of bioassays may result in systematic biases.
5 Conclusion
The predictive performance of most selected popPK models of VPA in children with epilepsy was unsatisfactory and diverse, and the direct extrapolation of these models to the clinical application should be performed with caution. Describing the non-linear kinetics of VPA based on the mechanisms of PK processes may enhance model predictive ability. Importantly, the linear non-saturable binding equation is more suitable for modeling the non-linearity in terms of protein-binding saturation. Moreover, Bayesian forecasting with prior observations led to an improvement in model fitness.
Principal investigator statement
The authors confirm that the Principal Investigator for this paper is Maochang Liu and that he had direct clinical responsibility for patients.
Data availability statement
The raw data supporting the conclusion of this article will be made available by the authors, without undue reservation.
Ethics statement
The studies involving humans were approved by the Ethics Committee of Wuhan Children’s Hospital. The studies were conducted in accordance with the local legislation and institutional requirements. Written informed consent for participation in this study was provided by the participants’ legal guardians/next of kin.
Author contributions
Conceptualization, methodology, and investigation, JM and ML; software, JM; validation, LZ, JM, and ML; formal analysis, LZ, WQ, JM, and ML; resources, ZL, JM, and ML; data curation and writing-original draft preparation, LZ, JM, and ML; writing-review and editing, LZ and JM; visualization, JM and DS; supervision, ZL and JM; project administration, ZL and JM; funding acquisition, ML. All authors contributed to the article and approved the submitted version.
Funding
This work was jointly funded by a research project of the Wuhan Municipal Health Commission (Grant Number. WX16B18) and a construction project of the Hubei Provincial Clinical Medical Research Center for Neurodevelopmental Disabilities in Children (Grant Number. HST 2020-19).
Acknowledgments
We would like to thank Editage (www.editage.cn) for English language editing.
Conflict of interest
The authors declare that the research was conducted in the absence of any commercial or financial relationships that could be construed as a potential conflict of interest.
Publisher’s note
All claims expressed in this article are solely those of the authors and do not necessarily represent those of their affiliated organizations, or those of the publisher, the editors and the reviewers. Any product that may be evaluated in this article, or claim that may be made by its manufacturer, is not guaranteed or endorsed by the publisher.
Supplementary material
The Supplementary Material for this article can be found online at: https://www.frontiersin.org/articles/10.3389/fphar.2023.1228641/full#supplementary-material
References
Ahn, J. E., Birnbaum, A. K., and Brundage, R. C. (2005). Inherent correlation between dose and clearance in therapeutic drug monitoring settings: Possible misinterpretation in population pharmacokinetic analyses. J. Pharmacokinet. Pharmacodyn. 32 (5-6), 703–718. doi:10.1007/s10928-005-0083-6
Anderson, B. J., Allegaert, K., Van den Anker, J. N., Cossey, V., and Holford, N. H. (2007). Vancomycin pharmacokinetics in preterm neonates and the prediction of adult clearance. Br. J. Clin. Pharmacol. 63 (1), 75–84. doi:10.1111/j.1365-2125.2006.02725.x
Benet, L. Z., and Hoener, B. A. (2002). Changes in plasma protein binding have little clinical relevance. Clin. Pharmacol. Ther. 71 (3), 115–121. doi:10.1067/mcp.2002.121829
Bergstrand, M., Hooker, A. C., Wallin, J. E., and Karlsson, M. O. (2011). Prediction-corrected visual predictive checks for diagnosing nonlinear mixed-effects models. AAPS J. 13 (2), 143–151. doi:10.1208/s12248-011-9255-z
Brendel, K., Comets, E., Laffont, C., and Mentre, F. (2010). Evaluation of different tests based on observations for external model evaluation of population analyses. J. Pharmacokinet. Pharmacodyn. 37 (1), 49–65. doi:10.1007/s10928-009-9143-7
Cai, X., Li, R., Sheng, C., Tao, Y., Zhang, Q., Zhang, X., et al. (2020). Systematic external evaluation of published population pharmacokinetic models for tacrolimus in adult liver transplant recipients. Eur. J. Pharm. Sci. 105237, 105237. doi:10.1016/j.ejps.2020.105237
Chiba, K., Suganuma, T., Ishizaki, T., Iriki, T., Shirai, Y., Naitoh, H., et al. (1985). Comparison of steady-state pharmacokinetics of valproic acid in children between monotherapy and multiple antiepileptic drug treatment. J. Pediatr. 106 (4), 653–658. doi:10.1016/s0022-3476(85)80097-4
Choonara, I. A., McKay, P., Hain, R., and Rane, A. (1989). Morphine metabolism in children. Br. J. Clin. Pharmacol. 28 (5), 599–604. doi:10.1111/j.1365-2125.1989.tb03548.x
Cloyd, J. C., Fischer, J. H., Kriel, R. L., and Kraus, D. M. (1993). Valproic acid pharmacokinetics in children. IV. Effects of age and antiepileptic drugs on protein binding and intrinsic clearance. Clin. Pharmacol. Ther. 53 (1), 22–29. doi:10.1038/clpt.1993.5
Comets, E., Brendel, K., and Mentre, F. (2008). Computing normalised prediction distribution errors to evaluate nonlinear mixed-effect models: The npde add-on package for R. Comput. Methods Programs Biomed. 90 (2), 154–166. doi:10.1016/j.cmpb.2007.12.002
Correa, T., Rodriguez, I., and Romano, S. (2008). Population pharmacokinetics of valproate in Mexican children with epilepsy. Biopharm. Drug Dispos. 29 (9), 511–520. doi:10.1002/bdd.636
Danhof, M., de Lange, E. C., Della Pasqua, O. E., Ploeger, B. A., and Voskuyl, R. A. (2008). Mechanism-based pharmacokinetic-pharmacodynamic (PK-PD) modeling in translational drug research. Trends Pharmacol. Sci. 29 (4), 186–191. doi:10.1016/j.tips.2008.01.007
Desoky, E. S. E., Fuseau, E., Amry, S. E. D., and Cosson, V. (2004). Pharmacokinetic modelling of valproic acid from routine clinical data in Egyptian epileptic patients. Eur. J. Clin. Pharmacol. 59 (11), 783–790. doi:10.1007/s00228-003-0699-7
Ding, J., Wang, Y., Lin, W., Wang, C., Zhao, L., Li, X., et al. (2015). A population pharmacokinetic model of valproic acid in pediatric patients with epilepsy: A non-linear pharmacokinetic model based on protein-binding saturation. Clin. Pharmacokinet. 54 (3), 305–317. doi:10.1007/s40262-014-0212-8
Dutta, S., Faught, E., and Limdi, N. A. (2007). Valproate protein binding following rapid intravenous administration of high doses of valproic acid in patients with epilepsy. J. Clin. Pharm. Ther. 32 (4), 365–371. doi:10.1111/j.1365-2710.2007.00831.x
Ebner, T., and Burchell, B. (1993). Substrate specificities of two stably expressed human liver UDP-glucuronosyltransferases of the UGT1 gene family. Drug Metab. Dispos. 21 (1), 50–55.
Ette, E. I., and Williams, P. J. (2004). Population pharmacokinetics I: Background, concepts, and models. Ann. Pharmacother. 38 (10), 1702–1706. doi:10.1345/aph.1D374
Ghodke-Puranik, Y., Thorn, C. F., Lamba, J. K., Leeder, J. S., Song, W., Birnbaum, A. K., et al. (2013). Valproic acid pathway: Pharmacokinetics and pharmacodynamics. Pharmacogenet Genomics 23 (4), 236–241. doi:10.1097/FPC.0b013e32835ea0b2
Gu, X., Zhu, M., Sheng, C., Yu, S., Peng, Q., Ma, M., et al. (2021). Population pharmacokinetics of unbound valproic acid in pediatric epilepsy patients in China: A protein binding model. Eur. J. Clin. Pharmacol. 77 (7), 999–1009. doi:10.1007/s00228-020-03080-y
Holford, N., Heo, Y. A., and Anderson, B. (2013). A pharmacokinetic standard for babies and adults. J. Pharm. Sci. 102 (9), 2941–2952. doi:10.1002/jps.23574
Hung, C. C., Ho, J. L., Chang, W. L., Tai, J. J., Hsieh, T. J., Hsieh, Y. W., et al. (2011). Association of genetic variants in six candidate genes with valproic acid therapy optimization. Pharmacogenomics 12 (8), 1107–1117. doi:10.2217/pgs.11.64
Jiang, D., Bai, X., Zhang, Q., Lu, W., Wang, Y., Li, L., et al. (2009). Effects of CYP2C19 and CYP2C9 genotypes on pharmacokinetic variability of valproic acid in Chinese epileptic patients: Nonlinear mixed-effect modeling. Eur. J. Clin. Pharmacol. 65 (12), 1187–1193. doi:10.1007/s00228-009-0712-x
Jiang, D. C., Wang, L., Wang, Y. Q., Li, L., Lu, W., and Bai, X. R. (2007). Population pharmacokinetics of valproate in Chinese children with epilepsy. Acta Pharmacol. Sin. 28 (10), 1677–1684. doi:10.1111/j.1745-7254.2007.00704.x
Johannessen, C. U., and Johannessen, S. I. (2003). Valproate: Past, present, and future. CNS Drug Rev. 9 (2), 199–216. doi:10.1111/j.1527-3458.2003.tb00249.x
Johannessen Landmark, C., Heger, K., Lund, C., Burns, M. L., Bjornvold, M., Saetre, E., et al. (2020). Pharmacokinetic variability during long-term therapeutic drug monitoring of valproate, clobazam, and levetiracetam in patients with dravet syndrome. Ther. Drug Monit. 42 (5), 744–753. doi:10.1097/FTD.0000000000000781
Johannessen Landmark, C., and Svein, I. J. (2016). Pharmacotherapy in epilepsy - does gender affect safety? Expert Opin. Drug Saf. 15 (1), 1–4. doi:10.1517/14740338.2016.1117606
Kluwe, F., Michelet, R., Mueller-Schoell, A., Maier, C., Klopp-Schulze, L., van Dyk, M., et al. (2020). Perspectives on model-informed precision dosing in the digital Health era: Challenges, opportunities, and recommendations. Clin. Pharmacol. Ther. 109, 29–36. doi:10.1002/cpt.2049
Kodama, Y., Koike, Y., Kimoto, H., Yasunaga, F., Takeyama, M., Teraoka, I., et al. (1992a). Binding parameters of valproic acid to serum protein in healthy adults at steady state. Ther. Drug Monit. 14 (1), 55–60. doi:10.1097/00007691-199202000-00009
Kodama, Y., Kuranari, M., Tsutsumi, K., Kimoto, H., Fujii, I., and Takeyama, M. (1992b). Prediction of unbound serum valproic acid concentration by using in vivo binding parameters. Ther. Drug Monit. 14 (5), 349–353. doi:10.1097/00007691-199210000-00001
Liu, M., Mao, J., Xu, H., Wang, J., Zhao, P., Xu, Q., et al. (2020). Effects of SCN1A and SCN2A polymorphisms on responsiveness to valproic acid monotherapy in epileptic children. Epilepsy Res. 168, 106485. doi:10.1016/j.eplepsyres.2020.106485
Mahmood, I. (2006). Prediction of drug clearance in children from adults: A comparison of several allometric methods. Br. J. Clin. Pharmacol. 61 (5), 545–557. doi:10.1111/j.1365-2125.2006.02622.x
Mao, J., Jiao, Z., Qiu, X., Zhang, M., and Zhong, M. (2020). Incorporating nonlinear kinetics to improve predictive performance of population pharmacokinetic models for ciclosporin in adult renal transplant recipients: A comparison of modelling strategies. Eur. J. Pharm. Sci. 153, 105471. doi:10.1016/j.ejps.2020.105471
Mao, J. J., Jiao, Z., Yun, H. Y., Zhao, C. Y., Chen, H. C., Qiu, X. Y., et al. (2018). External evaluation of population pharmacokinetic models for ciclosporin in adult renal transplant recipients. Br. J. Clin. Pharmacol. 84 (1), 153–171. doi:10.1111/bcp.13431
Mei, S., Feng, W., Zhu, L., Li, X., Yu, Y., Yang, W., et al. (2018). Effect of CYP2C19, UGT1A8, and UGT2B7 on valproic acid clearance in children with epilepsy: A population pharmacokinetic model. Eur. J. Clin. Pharmacol. 74 (8), 1029–1036. doi:10.1007/s00228-018-2440-6
Methaneethorn, J. (2018). A systematic review of population pharmacokinetics of valproic acid. Br. J. Clin. Pharmacol. 84 (5), 816–834. doi:10.1111/bcp.13510
Methaneethorn, J., and Leelakanok, N. (2021). Predictive ability of published population pharmacokinetic models of valproic acid in Thai manic patients. J. Clin. Pharm. Ther. 46 (1), 198–207. doi:10.1111/jcpt.13280
Miyagi, S. J., and Collier, A. C. (2011). The development of UDP-glucuronosyltransferases 1A1 and 1A6 in the pediatric liver. Drug Metab. Dispos. 39 (5), 912–919. doi:10.1124/dmd.110.037192
Moshe, S. L., Perucca, E., Ryvlin, P., and Tomson, T. (2015). Epilepsy: New advances. Lancet 385 (9971), 884–898. doi:10.1016/S0140-6736(14)60456-6
Ogusu, N., Saruwatari, J., Nakashima, H., Noai, M., Nishimura, M., Deguchi, M., et al. (2014). Impact of the superoxide dismutase 2 Val16Ala polymorphism on the relationship between valproic acid exposure and elevation of gamma-glutamyltransferase in patients with epilepsy: A population pharmacokinetic-pharmacodynamic analysis. PLoS One 9 (11), e111066. doi:10.1371/journal.pone.0111066
Patsalos, P. N., Berry, D. J., Bourgeois, B. F., Cloyd, J. C., Glauser, T. A., Johannessen, S. I., et al. (2008). Antiepileptic drugs-best practice guidelines for therapeutic drug monitoring: A position paper by the subcommission on therapeutic drug monitoring, ILAE commission on therapeutic strategies. Epilepsia 49 (7), 1239–1276. doi:10.1111/j.1528-1167.2008.01561.x
Patsalos, P. N., Spencer, E. P., and Berry, D. J. (2018). Therapeutic drug monitoring of antiepileptic drugs in epilepsy: A 2018 update. Ther. Drug Monit. 40 (5), 526–548. doi:10.1097/FTD.0000000000000546
Peeters, M. Y., Allegaert, K., Blusse van Oud-Alblas, H. J., Cella, M., Tibboel, D., Danhof, M., et al. (2010). Prediction of propofol clearance in children from an allometric model developed in rats, children and adults versus a 0.75 fixed-exponent allometric model. Clin. Pharmacokinet. 49 (4), 269–275. doi:10.2165/11319350-000000000-00000
Rodrigues, C., Chhun, S., Chiron, C., Dulac, O., Rey, E., Pons, G., et al. (2018). A population pharmacokinetic model taking into account protein binding for the sustained-release granule formulation of valproic acid in children with epilepsy. Eur. J. Clin. Pharmacol. 74 (6), 793–803. doi:10.1007/s00228-018-2444-2
Romoli, M., Mazzocchetti, P., D'Alonzo, R., Siliquini, S., Rinaldi, V. E., Verrotti, A., et al. (2019). Valproic acid and epilepsy: From molecular mechanisms to clinical evidences. Curr. Neuropharmacol. 17 (10), 926–946. doi:10.2174/1570159X17666181227165722
Serrano, B. B., Garcia Sanchez, M. J., Otero, M. J., Buelga, D. S., Serrano, J., and Dominguez-Gil, A. (1999). Valproate population pharmacokinetics in children. J. Clin. Pharm. Ther. 24 (1), 73–80. doi:10.1046/j.1365-2710.1999.00202.x
Sheiner, L. B., and Beal, S. L. (1981). Some suggestions for measuring predictive performance. J. Pharmacokinet. Biopharm. 9(4), 503–512. Doi doi:10.1007/Bf01060893
Sheiner, L. B., Beal, S., Rosenberg, B., and Marathe, V. V. (1979). Forecasting individual pharmacokinetics. Clin. Pharmacol. Ther. 26 (3), 294–305. doi:10.1002/cpt1979263294
Silva, M. F., Aires, C. C., Luis, P. B., Ruiter, J. P., Duran, M., et al. (2008). Valproic acid metabolism and its effects on mitochondrial fatty acid oxidation: A review. J. Inherit. Metab. Dis. 31 (2), 205–216. doi:10.1007/s10545-008-0841-x
Sun, H., Fadiran, E. O., Jones, C. D., Lesko, L., Huang, S. M., Higgins, K., et al. (1999). Population pharmacokinetics. A regulatory perspective. Clin. Pharmacokinet. 37 (1), 41–58. doi:10.2165/00003088-199937010-00003
Teixeira-da-Silva, P., Perez-Blanco, J. S., Santos-Buelga, D., Otero, M. J., and Garcia, M. J. (2022). Population pharmacokinetics of valproic acid in pediatric and adult caucasian patients. Pharmaceutics 14 (4), 811. doi:10.3390/pharmaceutics14040811
Ueshima, S., Aiba, T., Makita, T., Nishihara, S., Kitamura, Y., Kurosaki, Y., et al. (2008). Characterization of non-linear relationship between total and unbound serum concentrations of valproic acid in epileptic children. J. Clin. Pharm. Ther. 33 (1), 31–38. doi:10.1111/j.1365-2710.2008.00885.x
Vucicevic, K., Miljkovic, B., Pokrajac, M., Prostran, M., Martinovic, Z., and Grabnar, I. (2009). The influence of drug-drug interaction and patients' characteristics on valproic acid's clearance in adults with epilepsy using nonlinear mixed effects modeling. Eur. J. Pharm. Sci. 38 (5), 512–518. doi:10.1016/j.ejps.2009.09.017
Williams, J. H., Jayaraman, B., Swoboda, K. J., and Barrett, J. S. (2012). Population pharmacokinetics of valproic acid in pediatric patients with epilepsy: Considerations for dosing spinal muscular atrophy patients. J. Clin. Pharmacol. 52 (11), 1676–1688. doi:10.1177/0091270011428138
Winter, M. (2010). Basic clinical pharmacokinetics. 5th edn. Philadelphia, PA: LippincottWilliams &Wilkins Health.
Xia, Y., Long, J. Y., Shen, M. Y., Dong, N., Guo, H. L., Hu, Y. H., et al. (2021). Switching between LC-ESI-MS/MS and EMIT methods for routine TDM of valproic acid in pediatric patients with epilepsy: What clinicians and researchers need to know. Front. Pharmacol. 12, 750744. doi:10.3389/fphar.2021.750744
Xu, S., Chen, Y., Zhao, M., Guo, Y., Wang, Z., and Zhao, L. (2018). Population pharmacokinetics of valproic acid in epileptic children: Effects of clinical and genetic factors. Eur. J. Pharm. Sci. 122, 170–178. doi:10.1016/j.ejps.2018.06.033
Zaccara, G., Messori, A., and Moroni, F. (1988). Clinical pharmacokinetics of valproic acid-1988. Clin. Pharmacokinet. 15 (6), 367–389. doi:10.2165/00003088-198815060-00002
Zang, Y. N., Guo, W., Dong, F., Li, A. N., de Leon, J., and Ruan, C. J. (2022). Published population pharmacokinetic models of valproic acid in adult patients: A systematic review and external validation in a Chinese sample of inpatients with bipolar disorder. Expert Rev. Clin. Pharmacol. 15 (5), 621–635. doi:10.1080/17512433.2022.2075849
Zhao, C. Y., Jiao, Z., Mao, J. J., and Qiu, X. Y. (2016a). External evaluation of published population pharmacokinetic models of tacrolimus in adult renal transplant recipients. Br. J. Clin. Pharmacol. 81 (5), 891–907. doi:10.1111/bcp.12830
Zhao, M., Li, G., Qiu, F., Sun, Y., Xu, Y., and Zhao, L. (2016b). Development and validation of a simple and rapid UPLC-MS assay for valproic acid and its comparison with immunoassay and HPLC methods. Ther. Drug Monit. 38 (2), 246–252. doi:10.1097/FTD.0000000000000256
Keywords: population pharmacokinetics, valproic acid, external evaluation, pediatric epilepsy, protein-binding saturation, therapeutic drug monitoring
Citation: Zhang L, Liu M, Qin W, Shi D, Mao J and Li Z (2023) Modeling the protein binding non-linearity in population pharmacokinetic model of valproic acid in children with epilepsy: a systematic evaluation study. Front. Pharmacol. 14:1228641. doi: 10.3389/fphar.2023.1228641
Received: 25 May 2023; Accepted: 19 September 2023;
Published: 06 October 2023.
Edited by:
Sara Eyal, Hebrew University of Jerusalem, IsraelReviewed by:
Shenghui Mei, Capital Medical University, ChinaHong-Li Guo, Children’s Hospital of Nanjing Medical University, China
Copyright © 2023 Zhang, Liu, Qin, Shi, Mao and Li. This is an open-access article distributed under the terms of the Creative Commons Attribution License (CC BY). The use, distribution or reproduction in other forums is permitted, provided the original author(s) and the copyright owner(s) are credited and that the original publication in this journal is cited, in accordance with accepted academic practice. No use, distribution or reproduction is permitted which does not comply with these terms.
*Correspondence: Zeyun Li, zeyunli@zzu.edu.cn; Junjun Mao, jmao12@fudan.edu.cn
†These authors have contributed equally to this work and share first authorship