- 1Department of Operating Room, Sir Run Run Shaw Hospital, Zhejiang University School of Medicine, HangZhou, China
- 2Department of Urology, Sir Run Run Shaw Hospital, Zhejiang University School of Medicine, Hangzhou, China
- 3University of New South Wales, School of Medicine, Sydney, NSW, Australia
Background: SERPINE1, a serine protease inhibitor involved in the regulation of the plasminogen activation system, was recently identified as a cancer-related gene. However, its clinical significance and potential mechanisms in pan-cancer remain obscure.
Methods: In pan-cancer multi-omics data from public datasets, including The Cancer Genome Atlas (TCGA) and Genotype-Tissue Expression (GTEx), and online web tools were used to analyze the expression of SERPINE1 in different cancers and its correlation with prognosis, genetic alteration, DNA promoter methylation, biological processes, immunoregulator expression levels, immune cell infiltration into tumor, tumor mutation burden (TMB), microsatellite instability (MSI), immunotherapy response and drug sensitivity. Further, two single-cell databases, Tumor Immune Single-cell Hub 2 (TISCH2) and CancerSEA, were used to explore the expression and potential roles of SERPINE1 at a single-cell level. The aberrant expression of SERPINE1 was further verified in clear cell renal cell carcinoma (ccRCC) through qRT-PCR of clinical patient samples, validation in independent cohorts using The Gene Expression Omnibus (GEO) database, and proteomic validation using the Clinical Proteomic Tumor Analysis Consortium (CPTAC) database.
Results: The expression of SERPINE1 was dysregulated in cancers and enriched in endothelial cells and fibroblasts. Copy number amplification and low DNA promoter methylation could be partly responsible for high SERPINE1 expression. High SERPINE1 expression was associated with poor prognosis in 21 cancers. The results of gene set enrichment analysis (GSEA) indicated SERPINE1 involvement in the immune response and tumor malignancy. SERPINE1 expression was also associated with the expression of several immunoregulators and immune cell infiltration and could play an immunosuppression role. Besides, SERPINE1 was found to be related with TMB, MSI, immunotherapy response and sensitivity to several drugs in cancers. Finally, the high expression of SERPINE1 in ccRCC was verified using qRT-PCR performed on patient samples, six independent GEO cohorts, and proteomic data from the CPTAC database.
Conclusion: The findings of the present study revealed that SERPINE1 exhibits aberrant expression in various types of cancers and is associated with cancer immunity and tumor malignancy, providing novel insights for individualized cancer treatment.
1 Introduction
As one of the leading causes of death worldwide, cancer imposes an immense burden on the human society every year (Sung et al., 2021; Siegel et al., 2023). Despite significant advances in cancer treatment and early screening over the recent years, the overall survival prognosis for patients with cancer remains unsatisfactory, especially in certain cancer types (Sung et al., 2021; Hu et al., 2022). As such, there is an urgent need to explore new targets for cancer diagnosis and treatment.
Serine protease inhibitor clade E member 1 (SERPINE1, also known as PAI-1) is a serine protease inhibitor that plays key roles in regulating the plasminogen activation system (Placencio and DeClerck, 2015). By binding to and inactivating tissue-type plasminogen activator (tPA) and urokinase-type plasminogen activator (uPA), SERPINE1 exerts antifibrinolytic effects (Declerck and Gils, 2013). The inhibition of PAI-1 leads to increased thrombolysis in artery disease (Kohler and Grant, 2000). SERPINE1 consists of 379 amino acids and is synthesized and secreted primarily by vascular endothelial cells, adipocytes, and platelets (Chen et al., 2021).
In addition to regulating the plasminogen/plasminase system, SERPINE1 has been found to be involved in a variety of other processes, such as pericellular proteolysis, tissue remodeling, cell migration, inflammation, angiogenesis, and apoptosis, implying its involvement in various diseases (Placencio and DeClerck, 2015; Sillen and Declerck, 2021). In recent years, the abnormal expression of SERPINE1 has been found in various cancer types. Specifically, SERPINE1 overexpression has been observed in breast cancer (Duffy et al., 2014; Jevrić et al., 2019), melanoma (Hanekom et al., 2002), non-small cell lung cancer (Sotiropoulos et al., 2019), bladder cancer (Becker et al., 2010), and ovarian cancer (Nakatsuka et al., 2017). The inhibition of SERPINE1 expression has been shown to impede tumor progression and angiogenesis in several cancer types (Gomes-Giacoia et al., 2013; Masuda et al., 2013; Mashiko et al., 2015; Placencio et al., 2015; Takayama et al., 2016). SERPINE1-deficient mice also exhibited delayed tumor development, cancer invasion, and vascularization (Bajou et al., 1998; Gutierrez et al., 2000). Therefore, SERPINE1 is expected to be a promising novel target for the diagnosis and treatment of cancers. However, the detailed mechanisms underlying the involvement of SERPINE1 in cancers remain unclear.
Currently, there is no comprehensive study on the role of SERPINE1 in pan-cancer. In the present study, we performed a multi-omics analysis of SERPINE1 in pan-cancer. Our results confirmed the aberrant expression of SERPINE1 in multiple cancers and the relationship of SERPINE1 expression with the tumor microenvironment and cancer immunity. Furthermore, qRT-PCR was performed to validate the abnormal expression of SERPINE1 in clear cell renal cell carcinoma samples.
2 Materials and methods
2.1 The workflow of the study
Based on several public datasets, we analyzed the expression of SERPINE1 in pan-cancer and explored the possible causes of its abnormal expression. Then we evaluated the prognostic and diagnostic value of SERPINE1 in pan-cancer to assess its clinical value. Next, we explored the biological functions associated with SERPINE1 expression and found that it was associated with tumor malignancy and cancer immunity. Co-expression analysis, immune cell infiltration analysis, and cancer immunity cycle analysis were performed as well to validate its association with cancer immunity. Then we analyzed the correlation of SERPINE1 expression with response of cancer treatments to further explore its clinical value. At last, validation analyses were performed in ccRCC. A summary of the workflow of this study is shown in Figure 1.
2.2 Tissue samples and sample size calculation
The Ethics Committee of the Sir Run Run Shaw Hospital, Zhejiang University approved this study, and written informed consent was obtained from all participating patients. The research procedures adhered to the guidelines of the Declaration of Helsinki. For assessing SERPINE1 expression, 26 tissue samples (13 tumor tissues and 13 paired adjacent normal tissues) were randomly selected from clear cell renal cell carcinoma patients. The power analysis was performed using G*Power (G*Power, version 3.1 for MAC, Dusseldorf, North Rhine-Westphalia). According to the TCGA-KIRC project, the SERPINE1 expression of tumor samples (with available paired adjacent normal tissue data) were 5.8746 ± 1.6083 (log2FPKM) and the expression of adjacent normal tissues were 3.9290 ± 1.7263 (log2FPKM), indicating a effect size of 0.8615 (Cancer Genome Atlas Research Network, 2013). With power (1-β) of 0.85 and α error of 0.05, the sample size was calculated to be 13 pairs of samples.
2.3 Gene expression analysis of SERPINE1 in pan-cancer
Transcriptional data of tumor and normal samples were collected from the UCSC Xena (https://xenabrowser.net/datapages/) dataset (Goldman et al., 2020). Expression data of cancer cell lines were downloaded from the Cancer Cell Line Encyclopedia (CCLE, https://sites.broadinstitute.org/ccle) (Barretina et al., 2012). The abbreviations of cancer names are presented in Supplementary Table S1. Wilcox rank sum test was used to compare gene expression levels. R (version 4.2.3) and R package “ggplot2” were used for statistical analysis and visualization.
2.4 Single-cell analysis of SERPINE1 expression
Expression of SERPINE1 at a single-cell level was analyzed using Tumor Immune Single-cell Hub 2 (TISCH2), based on the MAESTRO workflow (Han et al., 2022). The following parameters were used for analysis: SERPINE1 (Gene), major lineages (Cell-type annotation), and all cancers (Cancer type). The R Package “ComplexHeatmap” (version 2.14.0) was used for data visualization (Gu et al., 2016).
2.5 Genetic alteration and DNA methylation analysis
Genetic alteration and DNA methylation data from the TCGA database were download from The cBioPortal (http://cbioportal.org) and GSCA (http://bioinfo.life.hust.edu.cn/GSCA/#/) (Cerami et al., 2012; Liu et al., 2023). Spearman’s rank correlation coefficient was used to evaluate the correlations between copy number variation, the DNA methylation level, and SERPINE1 expression.
2.6 Clinical and prognostic value analysis of SERPINE1
Clinical data and different types of prognostic data were extracted from UCSC Xena (https://xenabrowser.net/datapages/) (Goldman et al., 2020). Both Cox regression and Kaplan–Meier analysis were conducted to assess the correlation between SERPINE1 expression and patient prognosis. The cutoff value of the Kaplan–Meier estimator was determined using the “surv-cutpoint” function of the R package “survminer” (version 0.4.9). Information regarding immune subtypes was obtained from a previous report (Thorsson et al., 2018). Moreover, six independent datasets from BEST (https://rookieutopia.com/) were analyzed to validate the prognostic value of SERPINE1 expression in clear cell renal cell carcinoma, including the GSE167573, GSE29609, and GSE22541 cohorts from GEO (https://www.ncbi.nlm.nih.gov/), the E-MTAB-1980 cohort from EMBL’s European Bioinformatics Institute (EMBL-EBI, https://www.ebi.ac.uk/), and Renal Cell Cancer-European Union (RECA-EU) project data from the International Cancer Genome Consortium (ICGC, https://dcc.icgc.org/). The diagnostic value of SERPINE1 was assessed by receiver operating characteristic curve (ROC) using R package “pROC” (version 1.18.4).
2.7 Functional enrichment analysis and gene set enrichment analysis of SERPINE1
The associations between SERPINE1 expression and several biological processes were examined using GSEA and ssGSEA. Gene sets were downloaded from the Molecular Signatures Database (MSigDB, https://www.gsea-msigdb.org/gsea/index.jsp). Samples were grouped according to the median SERPINE1 expression for each cancer in GSEA. The R package “GSVA” (version 1.46.0) was used for the GSEA and ssGSEA analyses.
2.8 Single cell-level analysis of SERPINE1
CancerSEA, which is based on Gene Set Variance Analysis (GSVA), was used to assess the functional status of cancer cells at the single-cell level and Spearman’s rank correlation test were used to calculate their relationship to SERPINE1 expression (Yuan et al., 2019).
2.9 Correlation analysis of SERPINE1 with immune-associated genes, immune cell infiltration, and cancer-immunity cycle
Based on TCGA in pan-cancer data, the correlation of SERPINE1 expression with several immunoregulators, infiltration scores of different cells in the tumor microenvironment, and cancer-immunity cycle were evaluated using Pearson’s correlation coefficients. A log2 (TPM+1) transformation was performed before analysis. There were four types of immune-associated genes analyzed, including immune checkpoints, chemokines, chemokine receptors, and MHC-related genes. The infiltration scores of different cells in tumor microenvironment were evaluated by seven algorithms, including ESTIMATE (Yoshihara et al., 2013), TIMER (Li et al., 2020), MCP-counter (Becht et al., 2016), CIBERSORT(Newman et al., 2015), quanTIseq (Finotello et al., 2019), xCell (Aran et al., 2017), and EPIC(Racle et al., 2017), using R package “IOBR” (version 0.99.9) (Zeng et al., 2021). The correlation between the expression of SERPINE1and marker genes of immune cells, which was obtained from a previous study, was assessed using the “Gene_Corr” module of TIMER2.0 tool (Li et al., 2020; Li et al., 2023). The activity scores of each step of cancer-immunity cycle, which reflects the stepwise events of immune systems’ response to cancer (Chen and Mellman, 2013), were calculated by Tracking Tumor Immunophenotype (TIP, http://biocc.hrbmu.edu.cn/TIP/) (Xu et al., 2018).
2.10 Analysis of the relationships of SERPINE1 expression with TMB, MSI, immunotherapy response, and drug sensitivities
The simple nucleotide variation in pan-cancer data of TCGA annotated by MuTect2 were downloaded from the TCGA GDC (https://portal.gdc.cancer.gov/), and the R package “Maftools” (version 2.8.05) was used to calculate the TMB (Beroukhim et al., 2010; Mayakonda et al., 2018). The MSI in pan-cancer data from TCGA were obtained from a previous study (Bonneville et al., 2017). Cancers with a sample size less than three were eliminated from the TMB and MSI analyses. The relationship between SERPINE1 expression and immune checkpoint blockade (ICB) therapy response was evaluated by Tumor Immune Syngeneic MOuse (TISMO, http://tismo.cistrome.org/), a syngeneic mouse tumor database for investigation of tumor immunity and immunotherapy response (Zeng et al., 2022). The accuracy of SERPINE1 in predicting ICB therapy response was further verified by comparing it with other well-known biomarkers in human cohorts based on data from Tumor Immune Dysfunction and Exclusion (TIDE, http://tide.dfci.harvard.edu/) (Fu et al., 2020). Drug sensitivity and mRNA expression data of the NCI-60 cell lines were downloaded from CellMiner (http://discover.nci.nih.gov/cellminer/) (Luna et al., 2021). The relationships of SERPINE1 expression with TMB, MSI, and drug sensitivities were evaluated using Pearson’s correlation test and the differences between groups are statistically evaluated by Wald test using DESeq2 (Love et al., 2014).
2.11 Validating the expression of SREPINE1 in tissue samples using qRT-PCR
Total RNA was isolated using the TRIzol reagent (Invitrogen). This was followed by its reverse transcription into cDNA using the HiFiScript cDNA Synthesis Kit (CWBio). Quantitative PCR was then conducted using the SYBR Green method in the LightCycler® 480 System (Roche). The relative expression levels of genes were calculated using the 2−ΔΔCT method, with β-actin as the internal reference gene. The forward and reverse primer sequences for SERPINE1 were 5′-CTCATCAGCCACTGGAAAGGCA-3′ and 5′-GACTCGTGAAGTCAGCCTGAAAC-3′, respectively.
2.12 Independent cohorts and proteomic level validation of SERPINE1 abnormal expression in ccRCC
Six ccRCC cohorts were downloaded from the GEO database to verify the abnormal mRNA expression of SERPINE1 in ccRCC: GSE14994, GSE17895, GSE53000, GSE53757, GSE68417, and GSE71963. Proteomic data and corresponding clinical data from the CPTAC ccRCC discovery Study (PDC000127) were downloaded from CPTAC (https://pdc.cancer.gov/pdc/) to further explore its expression at the protein level (Clark et al., 2019).
3 Results
3.1 Aberrant expression of SERPINE1 in pan-cancer
To explore the expression of SERPINE1 among cancers, mRNA expression data from the TCGA, GTEx, and CCLE databases were analyzed. Based on the TCGA database alone, the mRNA expression of SERPINE1 was significantly increased in BRCA, COAD, ESCA, GBM, HNSC, KIRC, READ, STAD, and THCA, but significantly decreased in KICH, KIRP, LIHC, and UCEC (Figure 2A). The paired analysis results were consistent with those of unpaired analysis, except that the result of UCEC was no longer significant (Figure 2B). When analyzed in conjunction with data from the GTEx database, SERPINE1 expression was also increased in DLBC, PAAD, TGCT, and THYM and decreased in LAML, LUAD, LUSC, OV, PRAD, SKCM, and THYM (Figure 1C). Analysis of data from the CCLE database also indicated that the expression of SERPINE1 was increased in various cancer types, including BLCA, MESO, GBM, and KIRC, similar to analysis of TCGA data (Figures 2A, D).
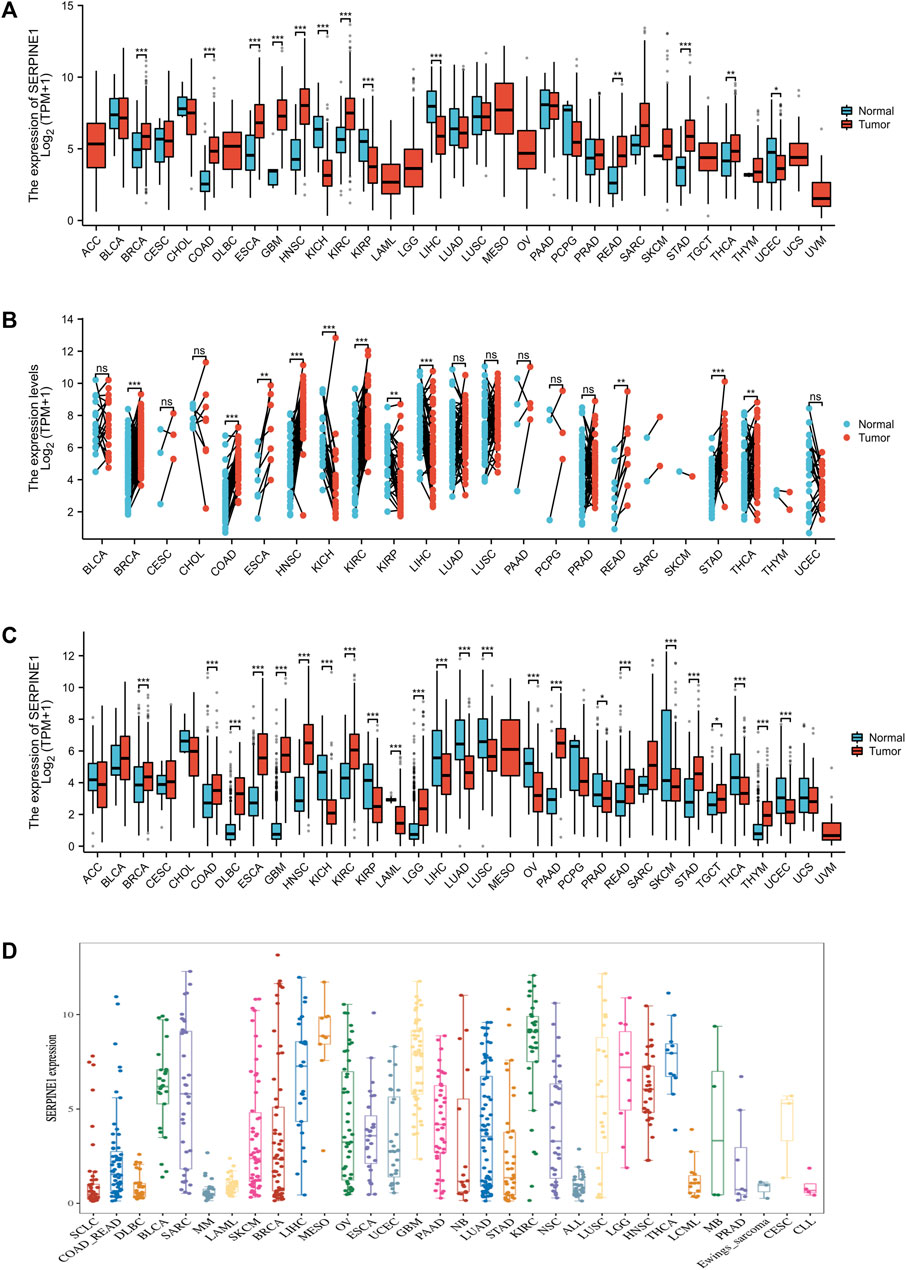
FIGURE 2. SERPINE1 expression in pan-cancer. (A) The expression of SERPINE1 in human cancers and normal tissues based on TCGA database. (B) The paired analysis of SERPINE1 expression in human cancers with adjacent normal tissues. (C) The differential expression of SERPINE1 between tumor and normal tissues among cancers based on the integrated data from TCGA and GTEx datasets. (D) The expression levels of different cancer cell lines according to CCLE database. *p < 0.05; **p < 0.01; ***p < 0.001.
3.2 SERPINE1 is enriched in endothelial cells and fibroblasts
Considering the boundedness of bulk RNA-seq, we further investigated the expression of SERPINE1 at a single-cell level using TISCH2. The results indicated that the expression of SERPINE1 was enriched in endothelial cells and fibroblasts in most cancer types (Figure 3A). The results for GSE11360 and GSE172301 are shown as examples (Figures 3B–E).
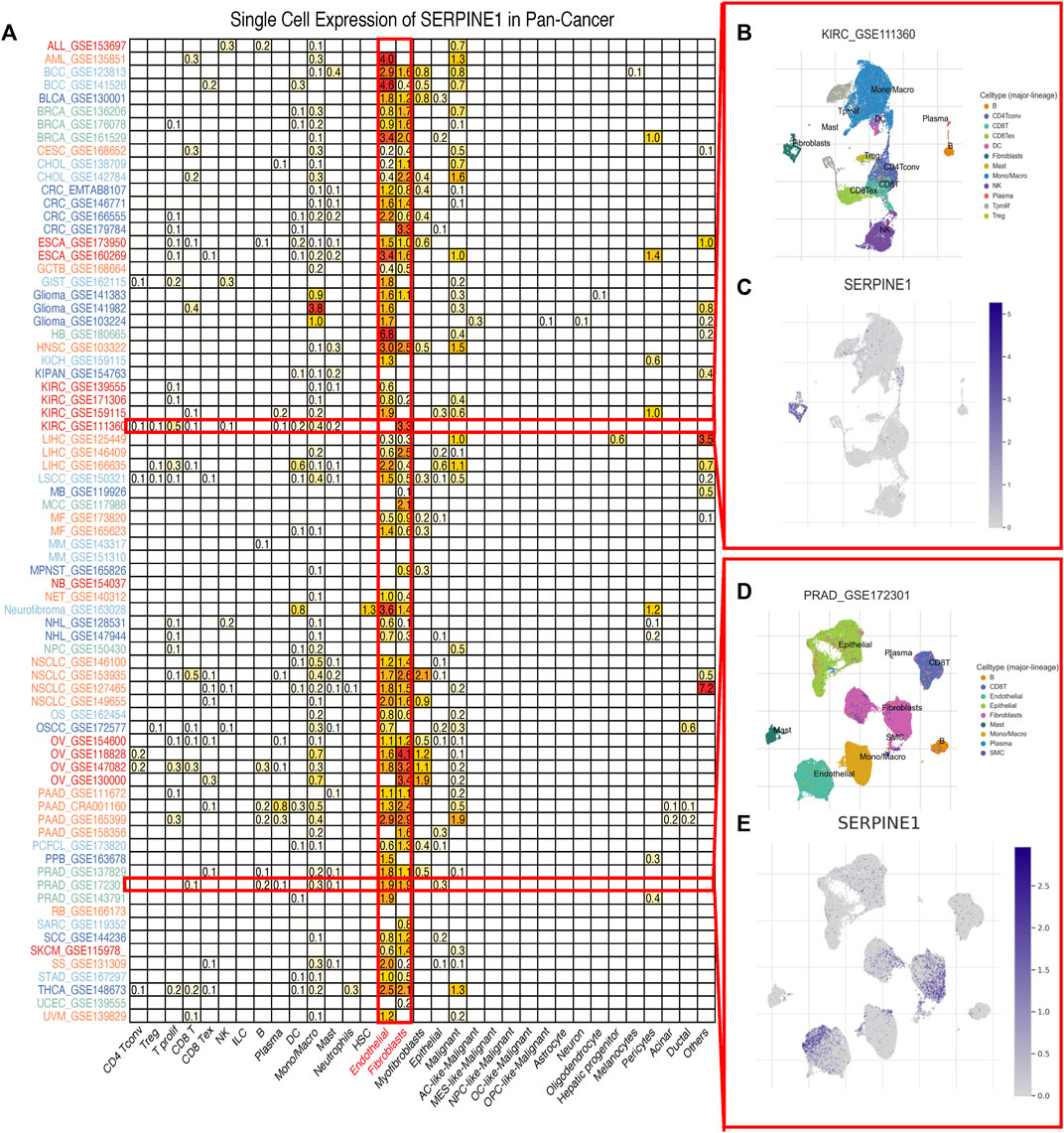
FIGURE 3. Single cell expression of SERPINE1 in pan-cancer. (A) The expression of SERPINE1 in different cell types across cancers. (B) The distribution of cell types in KIRC GSE111360 cohort. (C) The single cell expression of SERPINE1 in GSE111360 cohort. (D) The distribution of cell types in PRAD GSE172301 cohort. (E) The single cell expression of SERPINE1 in GSE 172301 cohort.
3.3 Genetic alteration and DNA methylation analysis of SERPINE1 in pan-cancer
Considering that the mRNA expression level of a gene can be influenced by epigenetics, we investigated the genetic alteration and DNA methylation level of SERPINE1 using cBioPortal and GSCA datasets. Amplification is the most common type of genetic alteration in most cancers, dominated by heterozygous amplification, followed by mutation, dominated by missense mutation (Figures 4A–C). Correlation analysis showed that the expression of SERPINE1 was negatively correlated with the DNA methylation level and positively correlated with the DNA copy number in most cancer types (Figure 4D).
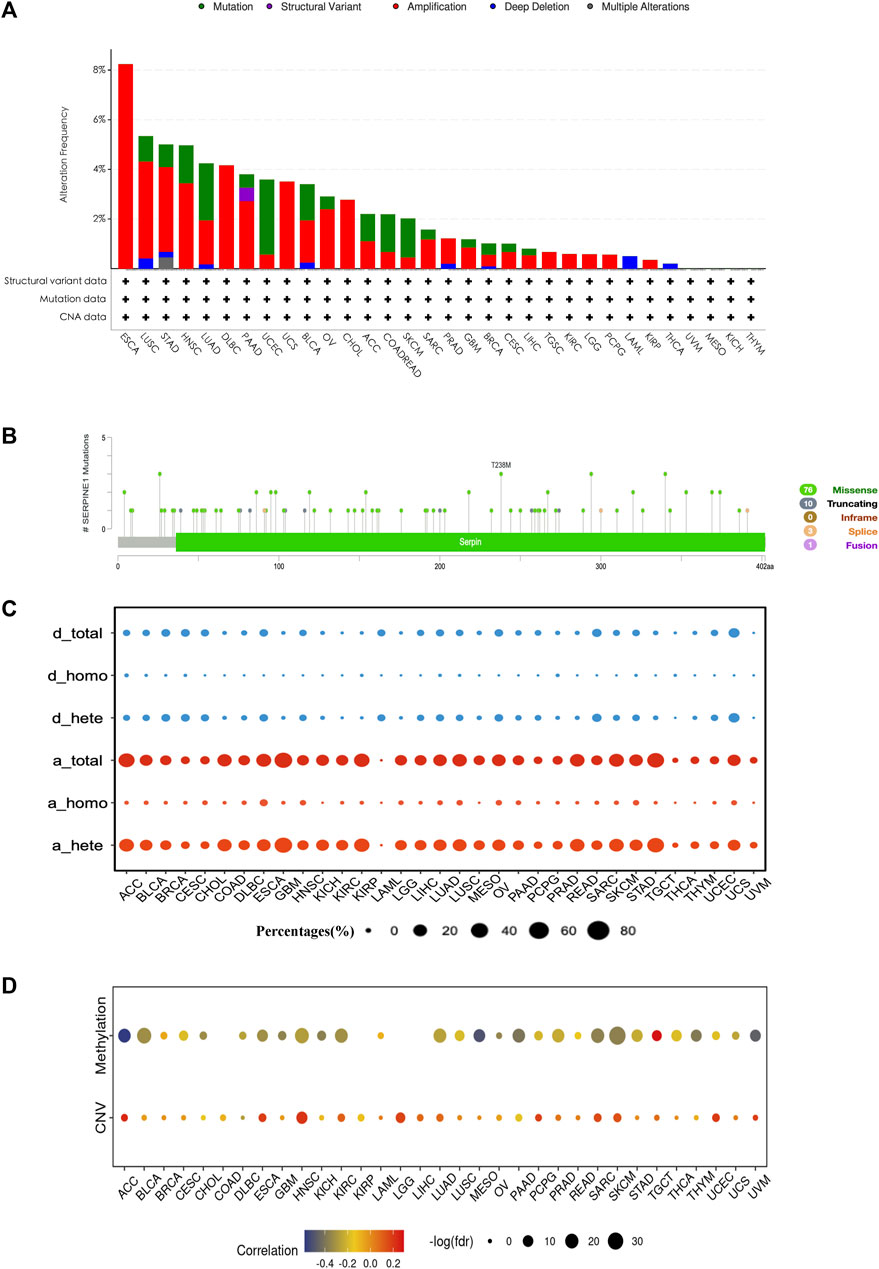
FIGURE 4. The genetic alteration and DNA methylation profile of SERPINE1 in pan-cancer. (A) The genetic alteration condition of SERPINE1 among cancers based on cBioPortal database. (B) The composition of SERPINE1 mutation in pan-cancer according to cBioPortal database. (C) The composition of SERPINE1 copy number variation according to GSCA database. d_total, total copy number deletion percentage; d_homo, homozygous copy number deletion percentage; d_hete, heterozygous copy number deletion percentage; a_total, total copy number amplification percentages percentage; a_homo, homozygous copy number amplification percentage; a_hete, heterozygous copy number amplification percentage. (D) Spearman’s rank correlations of SERPINE1 expression with the DNA methylation level and copy number variance.
3.4 SERPINE1 is a prognostic and diagnostic factor for various cancers
To evaluate the prognostic capacity of SERPINE1 in cancers, both univariate Cox regression and Kaplan–Meier analysis were performed. SERPINE1 was found to significantly reduce overall survival in ACC, BLCA, BRCA, CESC, COAD, GBM, HNSC, KIRC, KIRP, LGG, LIHC, LUAD, LUSC, MESO, OV, PAAD, SARC, STAD, THCA, UCEC, and UVM, while it was found to play a protective role for overall survival in PCPG and SKCM (Figure 5A). Besides, it is noteworthy that SERPINE1 was associated with poor prognosis for all four types of prognostic outcomes in LUAD and PAAD (Figure 5A). Univariate Cox regression results for the OS of all cancers are shown in Figure 5B. These findings suggested the general prognostic value of SERPINE1 expression in various cancers. The diagnostic value of SERPINE1 was examined using ROC curve as well. As shown in Supplementary Figure S1, SERPINE1 showed high diagnostic value in 11 kinds of cancers (AUC > 0.7), including CHOL, COAD, ESCA, GBM, HNSC, KICH, KIRC, KIRP, LIHC, READ, and STAD, indicating its crucial role in cancer diagnosis.
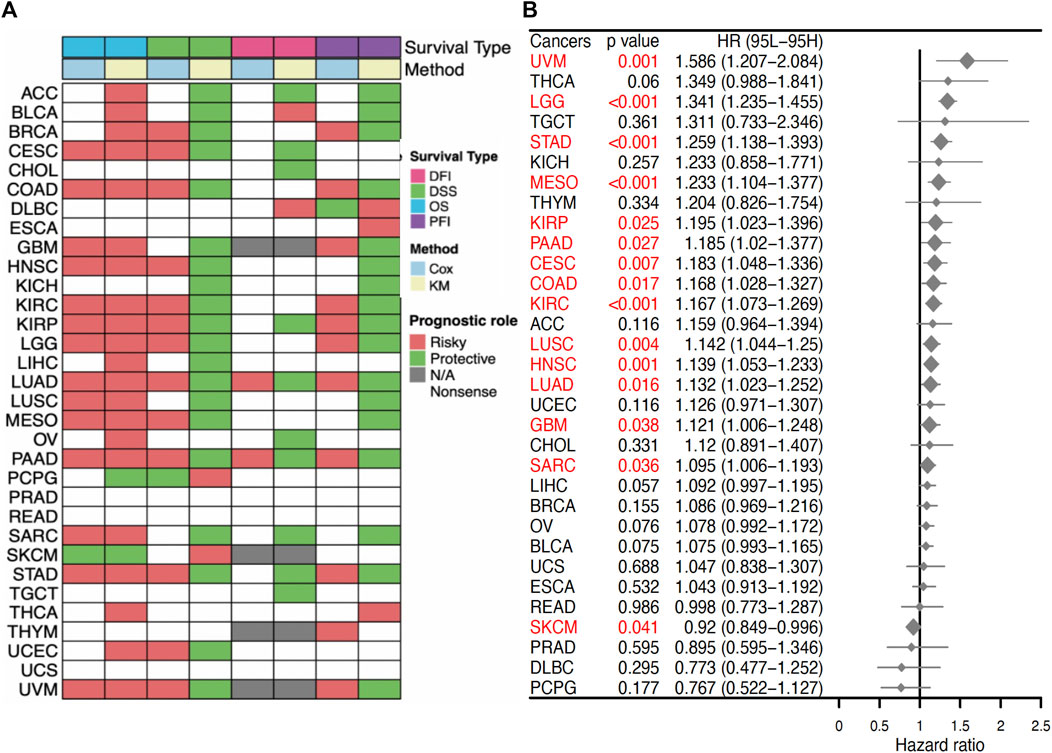
FIGURE 5. Prognostic value of SERPINE1 in pan-cancer. (A) Correlations of SERPINE1 expression with overall survival (OS), disease-specific survival (DSS), disease-free interval (DFI) and progression-free interval (PFI) based on univariate Cox regression and Kaplan-Meier method. Red indicates a risky role of SERPINE1 for prognosis, while green represents a protective role. (B) The prognostic role of SERPINE1 expression to patients’ overall survival (OS) in cancers by univariate Cox regression. Significant results (p < 0.05) are highlighted in red.
3.5 SERPINE1 is associated with the immune response and tumor malignancy in pan-cancer
To better understand the biological roles of SERPINE1, we evaluated the enrichment of pathways associated with SERPINE1 expression using GSEA at the bulk-RNA level and CancerSEA at the single-cell level. The GSEA results revealed that a number of immune-related pathways were enriched in samples with high SERPINE1 expression in most cancers, including TNF-α signaling via NF-κB, IFN-γ response, IFN-β response, inflammation response, IL-6-JAK-STAT3 signaling, IL-2-STATA5 signaling, and complement and allograft rejection, indicating a potential relationship between SERPINE1 and cancer immunity (Figure 6A). The results of ssGSEA for selected gene sets were consistent with those of GSEA (Figure 6B). The results from CancerSEA demonstrated that SERPINE1 was positively related with angiogenesis, hypoxia, inflammation, and metastasis in most cancers and negatively related with DNA damage, DNA repair, and stemness in some cancers (Figures 7A, B), which were consistent with the abovementioned results. Based on these results, we speculated that SERPINE1 may regulate tumor progression by influencing immune-related processes within tumors.
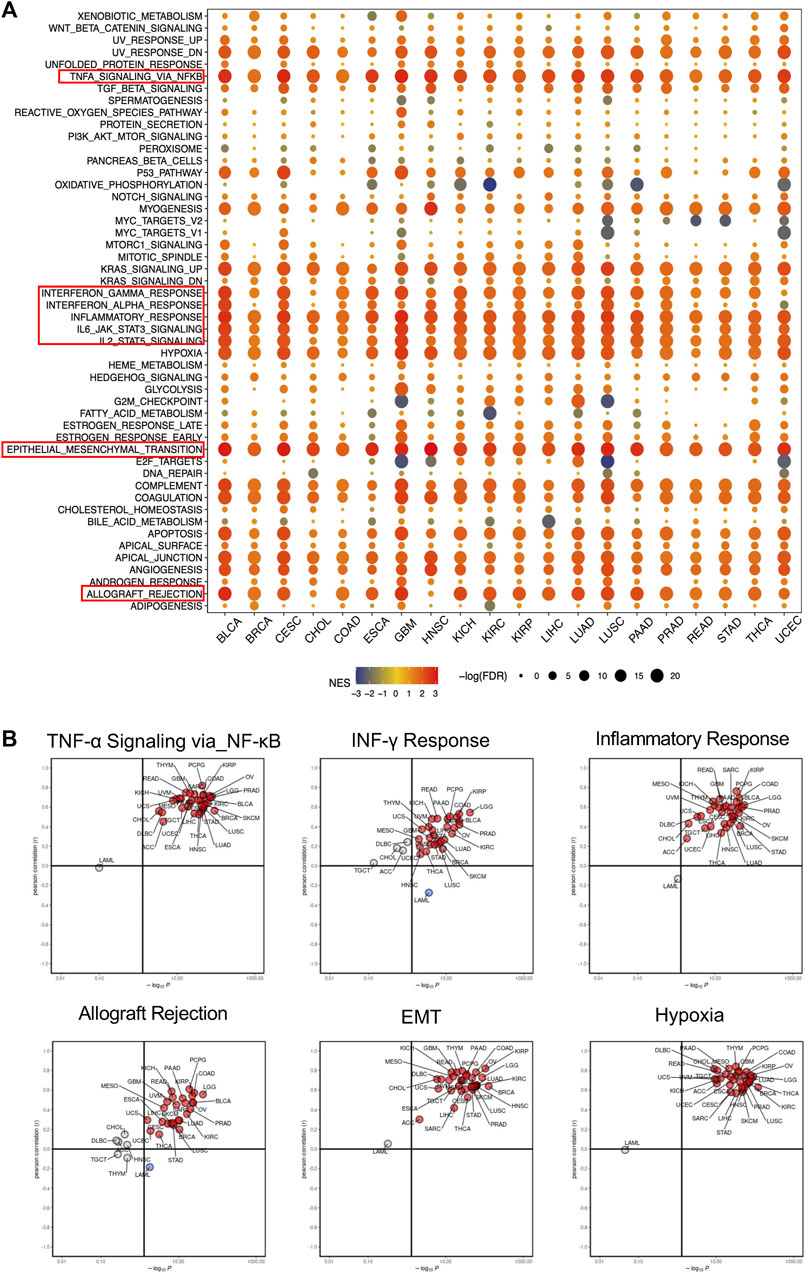
FIGURE 6. Gene function analysis of SERPINE1 in pan-cancer based on GSEA and ssGSEA. (A) The hallmarks gene set enrichment analysis (GSEA) of SERPINE1 in pan-cancer. The size of circle represents the FDR value of enrich term in each cancer, and the color indicates the normalized enrichment score (NES) of each term. (B) The single sample gene set enrichment analysis (ssGSEA) of SERPINE1 in pan-cancer. Cancer types with significant positive correlations (p < 0.05) with ssGSEA scores are highlighted in red, while those with significant negative correlations (p < 0.05) are highlighted in blue.
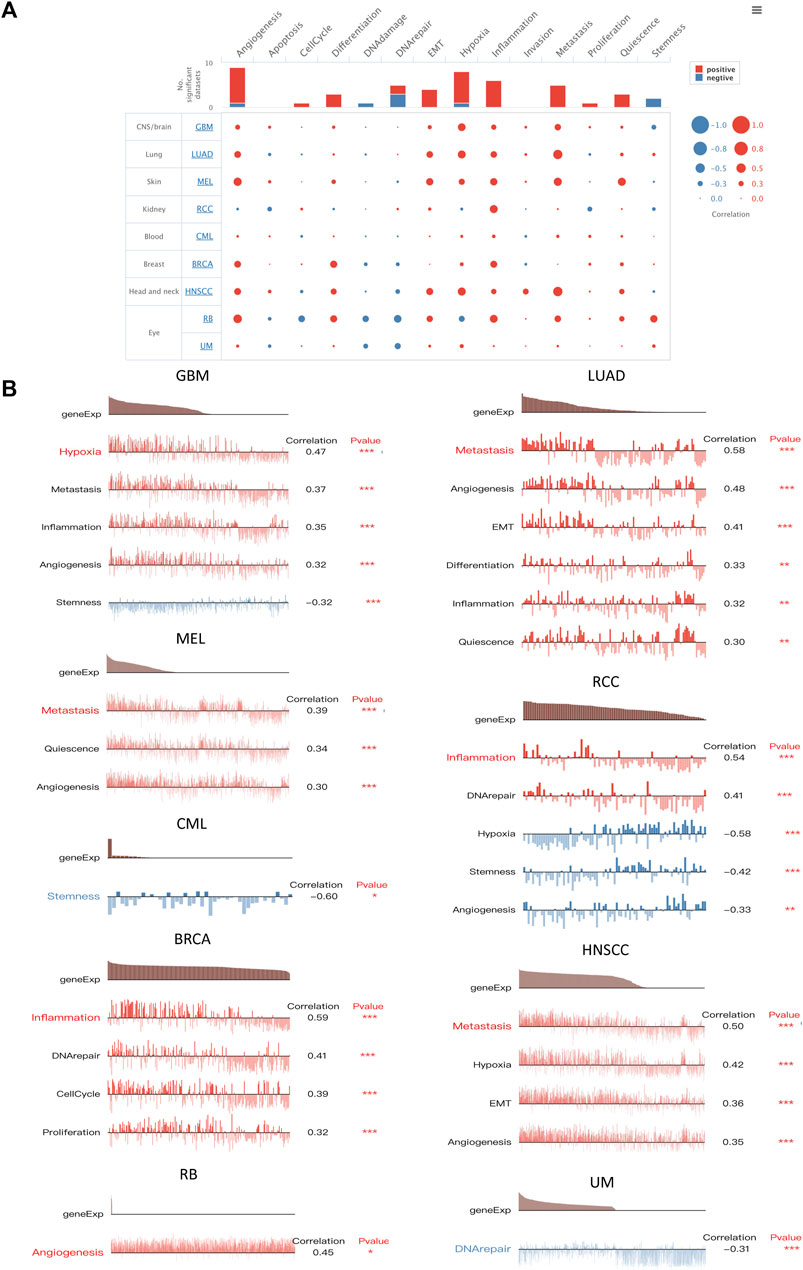
FIGURE 7. Functional relevance analysis of SERPINE1 in single-cell resolution by CancerSEA. (A) The association between SERPINE1 expression and single-cell states in cancers. (B) Single-cell states that significantly associated with each cancer. *p < 0.05; **p < 0.01; ***p < 0.001.
3.6 SERPINE1 expression is correlated with the expression of various immunoregulators in pan-cancer
Given the well-known roles of immunoregulators in cancer immunity, the relationships between SERPINE1 and immune checkpoints, chemokines, chemokine receptors, and MHC-related genes were analyzed. The results demonstrated that SERPINE1 expression was positively correlated with the expression of most inhibitory immune checkpoints, including CD274 (PD-L1), PDCD1 (PD-1), CTLA4, and HAVCR2 (TIM-3) in most cancers, especially GBM-LGG, KIPAN, UVM, PAAD, and COAD-READ (Figure 8A). Moreover, expression of SERPINE1 was found to be significantly associated with expressions of various immunostimulators, chemokines, chemokine receptors and MHC-related genes in pan cancer as well (Figures 8B–E).
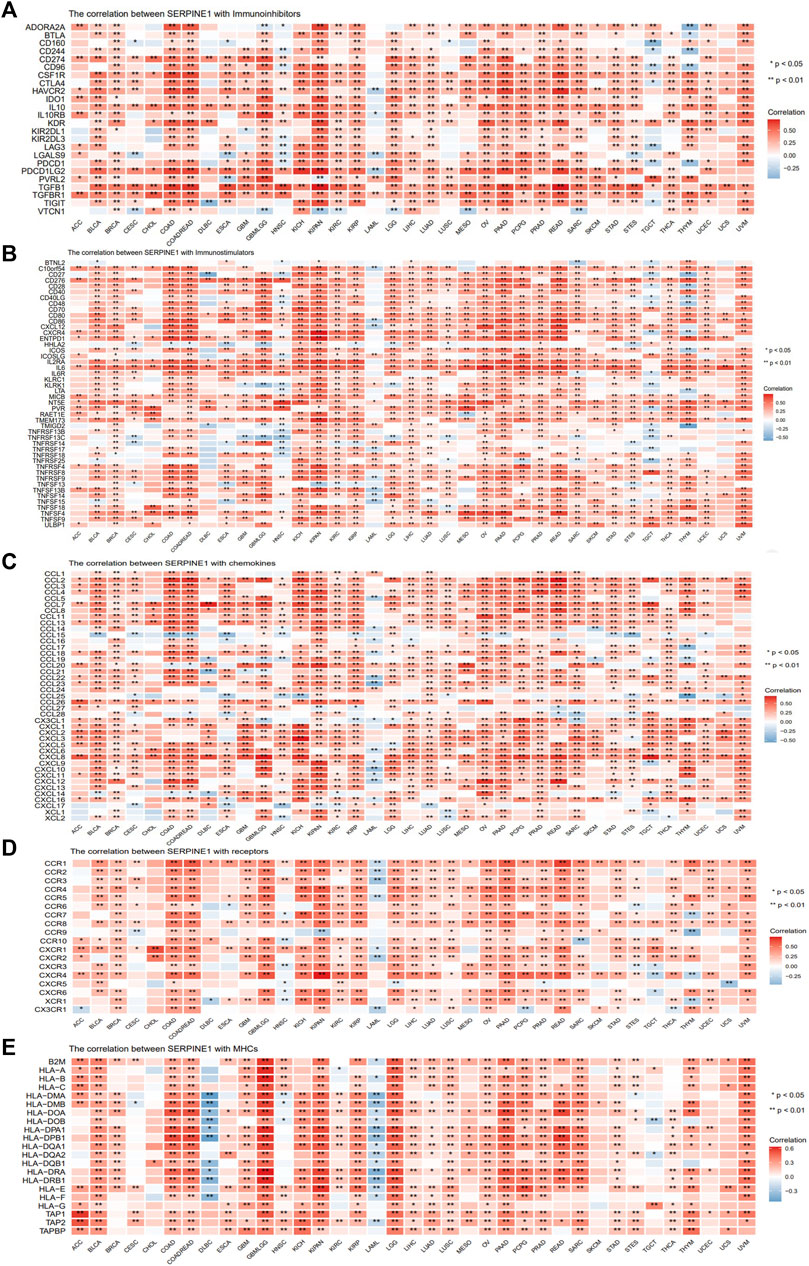
FIGURE 8. Co-expression analysis of SERPINE1 with immunoregulators in pan-cancer. (A) The Pearson’s correlation of SERPINE1 with inhibitory immune-checkpoints genes in cancers. (B) The Pearson’s correlation of SERPINE1 with stimulatory immune-checkpoints genes in cancers. (C) The Pearson’s correlation of SERPINE1 with chemokines. (D) The Pearson’s correlation of SERPINE1 with chemokine receptors (E) The Pearson’s correlation of SERPINE1 with MHC-related genes in cancers. *p < 0.05; **p < 0.01; ***p < 0.001.
3.7 SERPINE1 expression is correlated with immune cell infiltration and cancer-immunity cycle in pan-cancer
To gain further insight into the relationship between SERPINE1 and cancer immunity, we conducted immune cell infiltration analysis using different algorithms across a range of cancers. All algorithms showed that SERPINE1 expression was significantly correlated with the infiltration scores of multiple immune cells, although there was some variation in the results for specific cell types. The results from ESTIMATE indicated that SERPINE1 expression was positively correlated with both the immune score and stromal score in most cancers, especially KIPAN, GBM-LGG, and COAD-READ. Notably, no significantly negative relationship was found between SERPINE1 expression and stromal score in all cancers (Supplementary Figure S2). According to the CIBERSORT algorithm, SERPINE1 expression was negatively correlated with the infiltration of plasma cells, memory B cells, naïve CD4+ T cells, CD8+ T cells, follicular helper T cells, regulatory T cells, activated NK cells, and resting mast cells and positively correlated with the infiltration of activated memory CD4+ T cells, macrophages, activated mast cells, and neutrophils in most cancers (Figure 9A). As for the xCell algorithm, its results indicated that SERPINE1 expression was negatively correlated with the infiltration of CD4+ Tcm cells, NKT cells, plasma cells, and Th1 cells and positively correlated with the infiltration of fibroblasts, macrophages, neutrophils and regulatory T cells (Figure 9B). The results from TIMER algorithm revealed that SERPINE1 expression was positively correlated with the infiltration of all cell types, except B cells which did not reach significance in most cancers (Figure 9C). The results of MCP-counter algorithm were similar with that of TIMER algorithm, except that the infiltration of T cells showed significant negative correlation with SERPINE1 expression in 10 types of cancers (Supplementary Figure S3A). The results of the quanTIseq algorithm indicated that SERPINE1 expression was positively correlated with M1 macrophages, M2 macrophages, neutrophils and regulatory T cells (Supplementary Figure S3B). The results of the EPIC algorithm showed that SERPINE1 expression was positively correlated with the infiltration of cancer associated fibroblasts (CAFs), endothelial cells, and macrophages, and negatively correlated with the infiltration of CD8+ T cells (Supplementary Figure S3C). We also analyzed the correlation between the expression of SERPINE1 and immune cells’ marker genes. The results showed the expression of SERPINE1 was positively correlated with that of most immune cells’ marker genes in most cancer, especially the maker genes of neutrophils, monocyte, tumor-associated macrophages (TAMs), M2 macrophages, and regulatory T cells (Supplementary Figure S4). These results suggested that SERPINE1 may regulate the immune response of cancers.
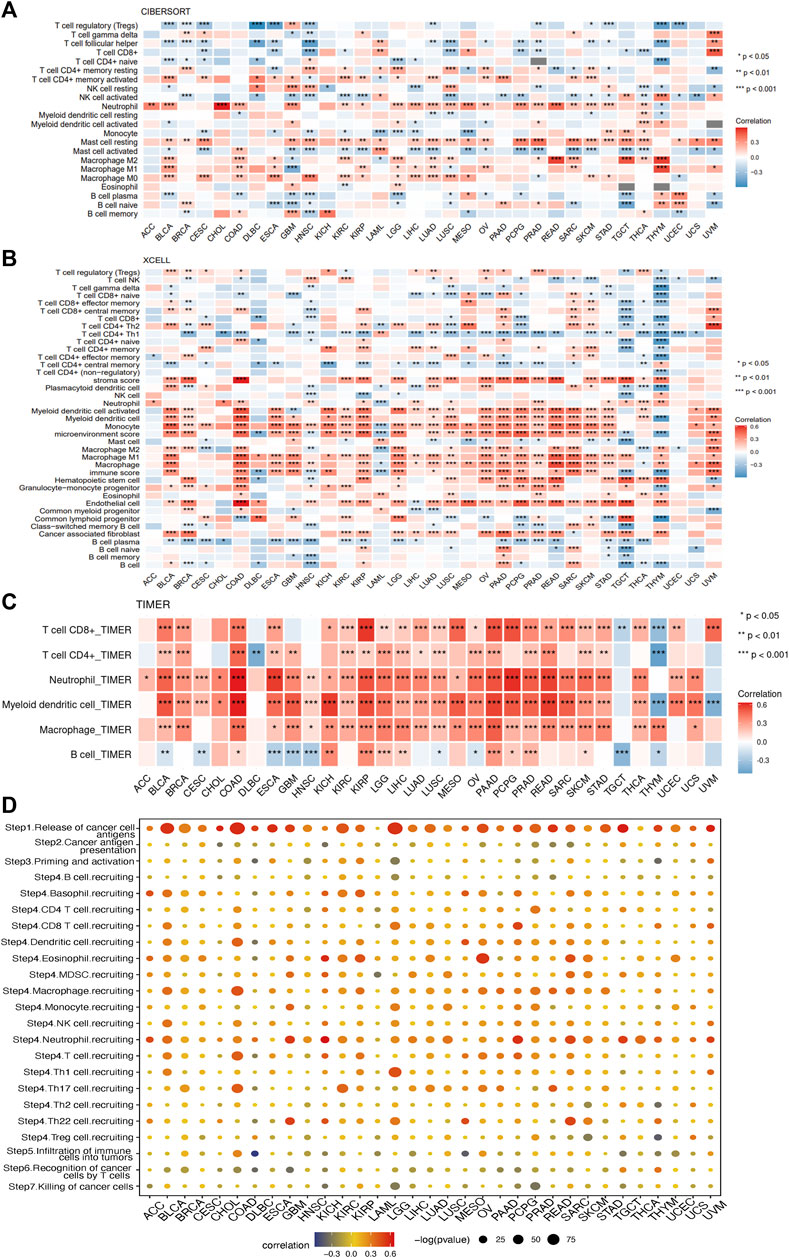
FIGURE 9. Correlations of SERPINE1 expression with immune cells infiltrations in pan-cancer. (A) The correlations of SERPINE1 expression with infiltration of 22 types of immune cells in cancers based on CIBERSORT algorithm. (B) The correlations of SERPINE1 expression with infiltration of 64 types of immune and stromal cell in cancers based on xCell algorithm. (C) The correlations of SERPINE1 expression with infiltration of six types of immune cell in cancers based on TIMER algorithm. (D) Correlation between SERPINE1 expression and cancer-immunity cycle. The size of circle represents the p-value of correlation test, and the color indicates the Pearson’s correlation coefficient. *p < 0.05; **p < 0.01; ***p < 0.001.
The relationship between SERPINE1 expression and the cancer-immunity cycle, which can reflect the stepwise events in anticancer immune response, was analyzed as well. As shown in Figure 8D, high expression of SERPINE1 was positively correlated with the activities of step 1 and step 4, which represents the release of cancer cell antigen and the recruiting of immune cells respectively, but negatively correlated with the activities of step 5, 6 and 7, which represents the infiltration of immune cells into tumors, the recognition of cancer cells by T cells, and the killing of cancer cells, in lots of cancer types. These results provide further evidence that SERPINE1 may be involved in the regulation of the immune response to cancer.
3.8 SERPINE1 is associated with TMB, MSI, immunotherapy response, and drug sensitivity in pan-cancer
Due to the predictive value of TMB and MSI for immunotherapy response, the relationships between TMB, MSI, and SERPINE1 expression were evaluated. SERPINE1 expression was found to be positively associated with TMB in THYM, COAD, COADREAD, SARC, and OV and negatively correlated with STES, STAD, and HNSC (Figure 10A). In addition, SERPINE1 expression levels in seven cancers were also significantly correlated with MSI in GBM-LGG, KIPAN, STES, HNSC, STAD, CHOL, SARC and THYM (Figure 10B). Then, we directly analyzed the relationship between SERPINE1 expression and immunotherapy response. As shown in Figure 10C, SERPINE1 expression could significantly predict the immunotherapy response in nine murine cohorts, with responders showing elevated SERPINE1 expression levels in six cohorts and decreased SERPINE1 expression levels in three cohorts. We also compared the predictive power of SERPINE1 to ICB response with other biomarkers in human cohorts. The area under curve (AUC) of SERPINE1 was above 0.5 in 12 cohorts and above 0.7 in two cohorts, which is similar to T clonality but lower than other classical biomarkers such as MSI, TMB, and CD274 (Figure 10D). These results indicated that SERPINE1 could predict the response of cancer patients to immunotherapy to some extent, though its predictive power might be lower than that of some classical markers. Furthermore, the correlations between SERPINE1 expression and drug sensitivities were explored using CellMiner. We found that SERPINE1 expression was positively correlated with sensitivities towards 13 drugs, including simvastatin, staurosporine, and pazopanib, and negatively correlated with sensitivities towards 30 drugs, including tamoxifen, tanespimycin, and nilotinib (Figure 10E).
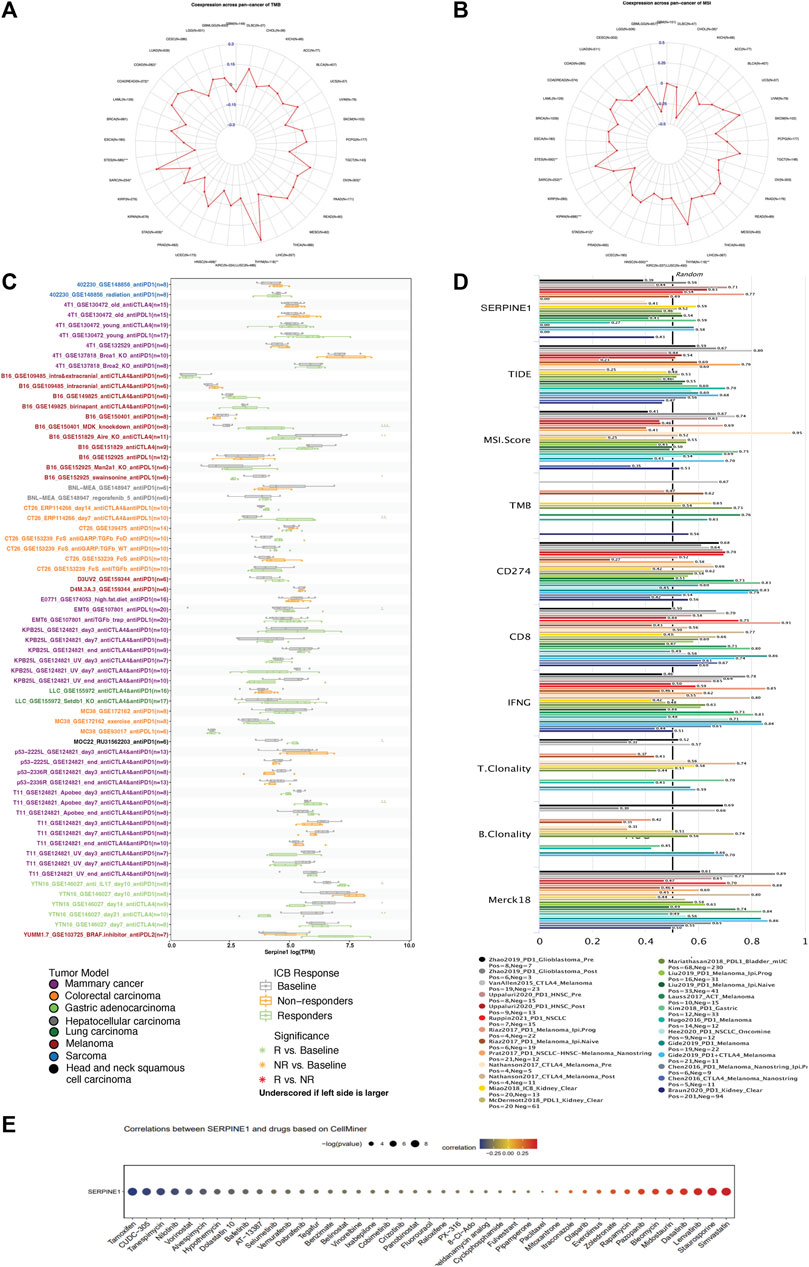
FIGURE 10. Relevance analysis of SERPINE1 expression with tumor mutation burden (TMB), microsatellite instability (MSI), immunotherapy response, and drug sensitivity. (A) The correlation of SERPINE1 with TMB in pan-cancer. (B) The correlation of SERPINE1 with MSI in pan-cancer. (C) Expression of SERPINE1 in mice with different responses to immune checkpoint blockade (ICB) therapy based on data from TISMO database. (D) The area under the receiver operating characteristic curve (AUC) of SERPINE1 and other biomarkers in predicting ICB therapy response. (E) The association of SERPINE1 expression with drug sensitivity based on CellMiner database. *p < 0.05; **p < 0.01; ***p < 0.001.
3.9 Elevated expression of SERPINE1 is associated with unfavorable prognosis in patients with ccRCC
Given the limited availability of reports on the role of SERPINE1 in ccRCC, we investigated the role of SERPINE1 in ccRCC. Our findings revealed that SERPINE1 expression is associated with male sex, lymph node metastasis, higher T stage, higher histological grade, higher pathological stage, and different immune subtypes (Figures 11A–H). Cox regression analyses revealed upregulated SERPINE1 expression as a risk factor for OS, DSS, and PFS using data from the TCGA database and as a risk factor for OS using GSE167573 cohort data from the GEO database (Figure 11I). However, the result of Cox regression using RECA-EU project data from ICGC database indicated upregulated SERPINE1 expression as a protective factor for OS (Figure 11I). The multivariate Cox regression was performed based on TCGA database, and the results verified the independence of SERPINE1’s prognostic value for patient with ccRCC (Supplementary Figure S5).
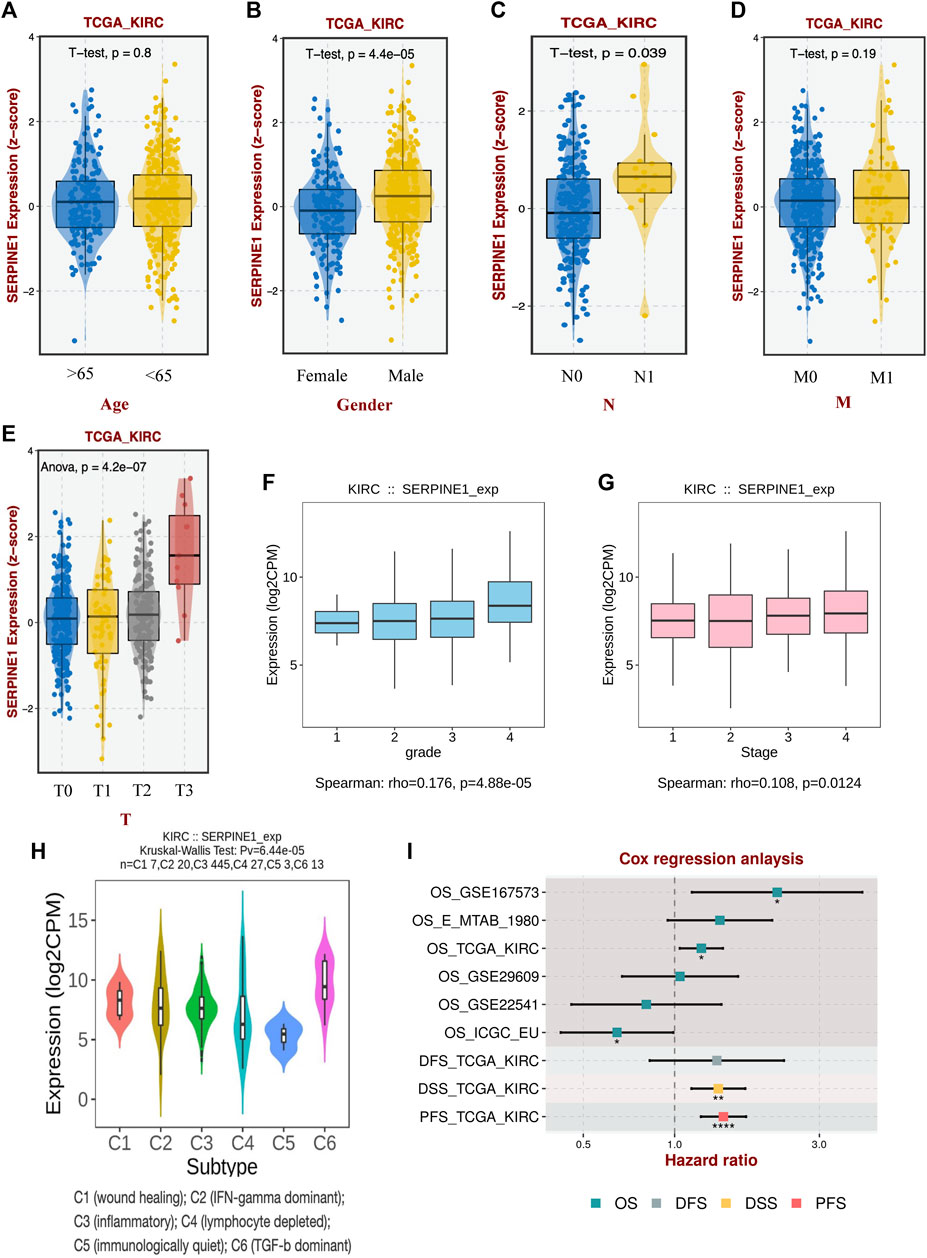
FIGURE 11. Association between SERPINE1 and clinicopathological characteristics of clear cell renal cell carcinoma. (A) The expression of SERPINE1 in patients of different ages. (B) The expression of SERPINE1 in patients of different genders. (C) The expression of SERPINE1 in patients of different lymph node metastasis statuses. (D) The expression of SERPINE1 in patients of different remote metastasis statuses. (E) The expression of SERPINE1 in patients of different primary tumor stages. (F) The expression of SERPINE1 in different histological grades. (G) The expression of SERPINE1 in different cancer stages. (H) The expression of SERPINE1 in different immune subtypes. (I) The correlation of SERPINE1 with prognosis based on different datasets. OS, overall survival; DFS, disease-free survival; DSS, disease-specific survival; PFS, progression-free survival; *p < 0.05; **p < 0.01; ***p < 0.001.
3.10 SERPINE1 expression is associated with the tumor microenvironment in clear cell renal cell carcinoma
To further investigate the role of SERPINE1 in ccRCC, gene set enrichment analysis was performed. The results revealed that SERPINE1 expression was significantly related with various biological processes. Further, GO analysis showed that SERPINE1 expression was positively correlated with collagen fibril organization, collagen biosynthetic process, and the regulation of T helper 1 type immune response, while it was negatively correlated with metabolism-related processes, such as tricarboxylic acid cycle, fatty acid beta oxidation, and the alpha amino acid catabolic process (Figure 12A). In the KEGG pathway analysis, several malignancy-related pathways, such as the P53 signaling pathway, cell cycle and DNA replication pathways, as well as immunity-related pathways, such as natural killer cell mediated cytotoxicity and T cell receptor signaling pathways, were found to be significantly positively correlated with SERPINE1 expression (Figure 12B). Similar results were obtained through the hallmark analysis (Figures 12C, D). These findings revealed that SERPINE1 may be involved in the formation of the tumor microenvironment in ccRCC and were consistent with the results in pan-cancer.
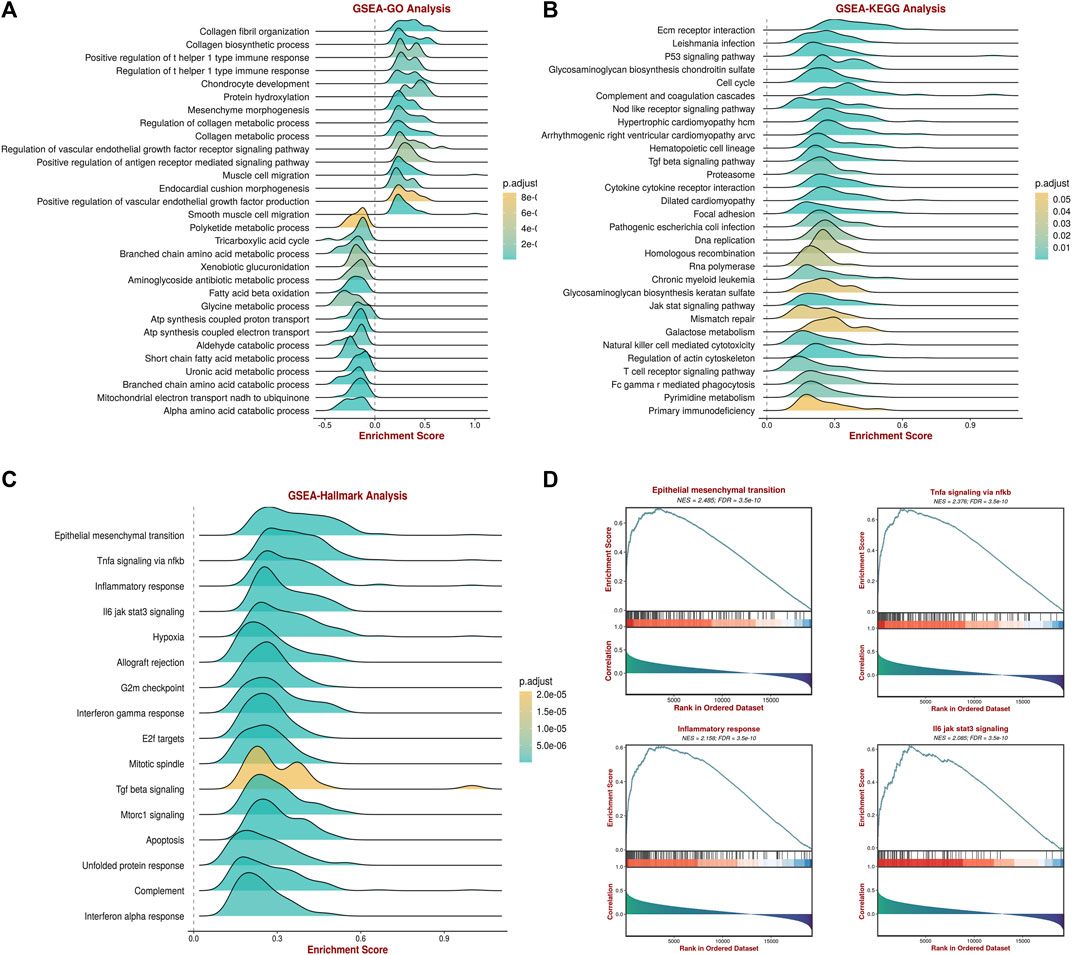
FIGURE 12. Gene set enrichment analysis (GSEA) of SERPINE1 in clear cell renal cell carcinoma (ccRCC). (A) The associations of SERPINE1 expression with several Gene Ontology (GO) terms. (B) The associations of SERPINE1 expression with several Kyoto Encyclopedia of Genes and Genomes (KEGG) pathways. (C) The associations of SERPINE1 expression with several Hallmark terms. (D) The GSEA results of certain Hallmark terms.
3.11 Validation of SERPINE1 expression in tumor tissues using qRT-PCR, independent cohorts, and proteomic data
Finally, we confirmed the aberrant expression of SERPINE1 in ccRCC samples. Our ccRCC patient samples and six independent GEO cohorts exhibited significantly increased levels of SERPINE1 expression, as expected (Figures 13A, B). Additionally, analysis of CPTAC data revealed that SERPINE1 expression was also elevated at the protein level in ccRCC samples (Figures 13C–F), which further enhanced the credibility of our study findings.
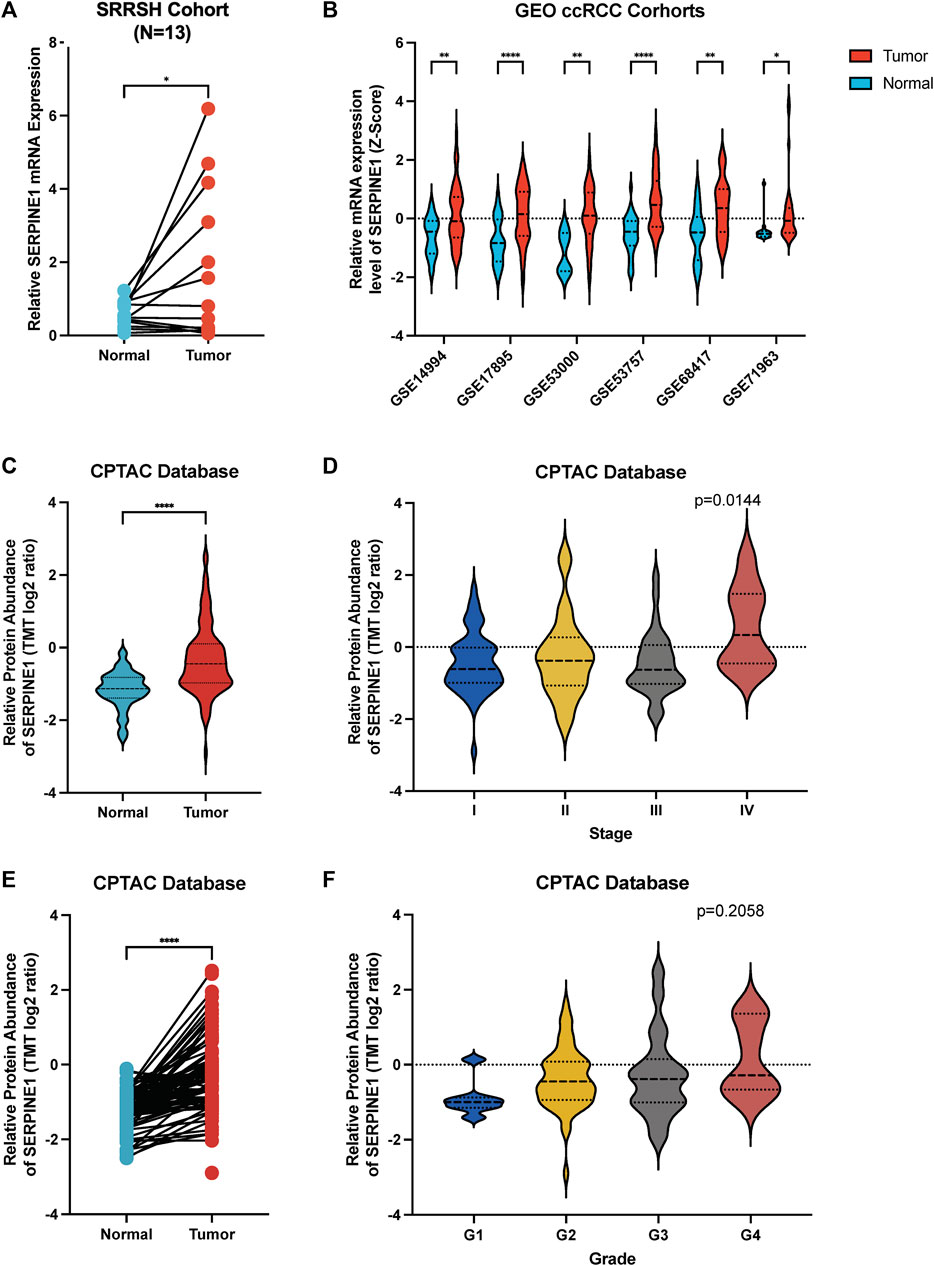
FIGURE 13. Validation of aberrant expression of SERPINE1 in ccRCC by qRT-PCR, independent cohorts and proteome. (A) qRT-PCR analysis of SERPINE1 expression in clear cell renal cell carcinoma and paired adjacent normal tissues based on patient samples from Sir Run Run Shaw Hospital. (B) Analysis of 6 GEO cohorts regarding SERPINE1 expression in ccRCC and normal samples. (C) Unpaired analysis of SERPINE1 protein abundance in ccRCC and adjacent normal tissue based on CPTAC database. (D) The SERPINE1 protein abundance in patients with different stages of ccRCC. (E) Paired analysis of SERPINE1 protein abundance in ccRCC and adjacent normal tissue based on CPTAC database. (F) The SERPINE1 protein abundance in patients with different histological grades of ccRCC *p < 0.05; **p < 0.01; ***p < 0.001.
4 Discussion
SERPINE1, a regulator of the fibrinolytic system, was found to be associated with tumor progression and metastasis in several cancers; however, its detailed mechanisms of action in various cancers remain obscure (Hanekom et al., 2002; Becker et al., 2010; Duffy et al., 2014; Mashiko et al., 2015; Jevrić et al., 2019; Sotiropoulos et al., 2019). In the present research, we performed a multi-omics integrated analysis to explore the expression, prognostic value, and possible underlying mechanisms of action of SERPINE1 in pan-cancer.
Expression analysis based on the TCGA and GTEx databases indicated the aberrant expression of SERPINE1 in several cancers. Overexpression of SERPINE1 and its correlation with poor prognosis has been reported in several cancers (Hanekom et al., 2002; Becker et al., 2010; Duffy et al., 2014; Nakatsuka et al., 2017; Jevrić et al., 2019; Sotiropoulos et al., 2019). The results of the present study confirmed the prevalence of aberrant SERPINE1 expression among cancers. Given the bulk RNA sequencing data is the average expression of different cells, which will lead to the loss of information about cells heterogeneity, we analyzed the expression of SERPINE1 at single-cell level as well, and the results showed that SERPINE1 expression was enriched in the endothelial cells and fibroblasts, consistent with the findings of a previous study, indicating the functions of SERPINE1 might be related with these two cell types, such as angiogenesis and regulating TME (Placencio and DeClerck, 2015; Clark et al., 2019; Chen et al., 2021b; de Visser and Joyce, 2023). Subsequently, we analyzed possible reasons for the aberrant expression of SERPINE1 in cancers. DNA methylation level and DNA copy number variation are well-known ways to influence the gene expression, thus their correlation with SERPINE1 was analyzed (Morgan et al., 2018; Pös et al., 2021). SERPINE1 expression showed a positive correlation with copy number variance and a negative correlation with the DNA promoter methylation level in most cancers, suggesting its potential role in CNV and the DNA methylation level. Some studies have indicated that some microRNAs and long noncoding RNAs are involved in the regulation of SERPINE1 expression (Tan et al., 2021; Teng et al., 2021; Zhao and Liu, 2021). Moreover, transforming growth factor β (TGF-β) has been implicated in the regulation of SERPINE1 expression (Kutz et al., 2001; Ma et al., 2002), and a positive correlation of TGF-β expression with SERPINE1 expression was observed through the GSEA analysis conducted in the present study.
Next, we explored the relationship between SERPINE1 expression and the prognosis of cancer patients. High SERPINE1 expression was found to be a risk factor for overall survival in several cancers, consistent with the findings of previous studies (Hanekom et al., 2002; Becker et al., 2010; Duffy et al., 2014; Nakatsuka et al., 2017; Jevrić et al., 2019; Sotiropoulos et al., 2019). Hence, we speculated that SERPINE1 may be a prognostic biomarker for various cancers.
To further explore the mechanisms underlying the role of SERPINE1 in cancers, we performed GSEA on pan-cancer data from TCGA. Several immune-related pathways were found to be significantly associated with high SERPINE1 expression in most cancers, including TNF-α signaling via NF-κβ, INF-γ response, and inflammation response. Similar results were also found in the single cell-level analysis performed by us using CancerSEA. Therefore, we believe that SERPINE1 is involved in cancer immunity and tumor malignancy (Iwaki et al., 2012; Declerck and Gils, 2013; Placencio and DeClerck, 2015; Chen et al., 2021; Sillen and Declerck, 2021).
Since immunoregulators are known to be important for the immune response, we next performed a co-expression analysis of SERPINE1 to further understand its roles in cancer immunity. The results demonstrated that SERPINE1 expression was positively and significantly correlated with the expression of immune-checkpoints, chemokines, chemokine receptors, and MHC-related genes in most cancers, indicating the remarkable effect of SERPINE1 on the immune system and consistent with previous studies about the pro-inflammatory and pro-angiogenesis roles of SERPINE1 (Iwaki et al., 2012; Declerck and Gils, 2013; Placencio and DeClerck, 2015). Traditionally, CD8+ T cells, memory B cells, plasma cells, follicular helper T cells, activated NK cells, NKT cells, and M1 macrophages are thought to be anti-cancer cells in the tumor microenvironment (TME), while regulatory T cells, M2 macrophages, and cancer associated fibroblasts are considered to be pro-cancer cells (Fridman et al., 2012; Sica and Mantovani, 2012; Bae et al., 2019; Chen and Song, 2019; Togashi et al., 2019; St Paul and Ohashi, 2020; Laskowski et al., 2022; Laumont et al., 2022; Li H. et al., 2023; Cai et al., 2023; Gutiérrez-Melo and Baumjohann, 2023). Therefore, we speculated that high SERPINE1 expression may play an immunosuppressive role in the tumor microenvironment due to its inverse correlation with several anti-cancer cells and positive correlation with several pro-cancer cells. The result of cancer-immunity cycle analysis further validated our speculation. Although SERPINE1 expression was positive correlated with the recruiting of most immune cells, the infiltration of immune cells into tumors, recognition of cancer cells by T cells, and killing of cancer cells were found to be negatively correlated with SERPINE1 expression in lots of cancers. However, given that our bioinformatic analysis based on bulk-RNA sequencing data has several limitations, further investigations are warranted.
Next, we validated the abnormal expression of SERPINE1 and its potential biological functions in clear cell renal cell carcinoma. Upregulated SERPINE1 expression was found to be associated with several clinical features of ccRCC, such as lymph node metastasis, high T stage, high histological grade, and high pathological stage. As for the gene function analysis performed using GSEA, collagen-associated processes, immune-associated processes, and malignancy-related pathways were found to be positively correlated with SERPINE1 expression in ccRCC. These findings are consistent with our speculation that SERPINE1 expression is involved in the regulation of the tumor microenvironment. Next, we further explored SERPINE1 expression in ccRCC in clinical patient samples and independent datasets.
Given the role of abnormal SERPINE1 expression in cancer development, it is important to consider how to interfere its expression to benefit cancer patients. As PAI-1 is a well-known regulator of the plasminogen activation system, many efforts have been devoted to the development of selective PAI-1 inhibitors (Fortenberry, 2013; Placencio et al., 2015; Sillen and Declerck, 2020; 2021). Some marketed drugs, including insulin-sensitizing agents, angiotensin-converting enzyme inhibitors (ACEI), and statins, have shown the ability to attenuate the synthesis or secretion of SERPINE1 (Brown et al., 2002b; Ersoy et al., 2008; Baluta and Vintila, 2015). Specifically, insulin resistance has been found to be associated with elevated plasma PAI-1 levels (Juhan-Vague and Alessi, 1997), and both proinsulin and insulin can stimulate PAI-1 expression (Sakamoto et al., 1999; Nordt et al., 2001), thus insulin-sensitizing agents such as metformin may have independent effects in decreasing PAI-1 levels in patients with type 2 diabetic (Ersoy et al., 2008). Besides, activation of renin-angiotensin-aldosterone system (RASS) has also been found to be involved in the regulation of PAI-1 levels, and ACEI, such as quinapril, ramipril, and perindopril, have shown the ability to reduce PAI-1 level in both healthy people and hypertensive patients (Brown et al., 1998; 2002a; 2002b; Erdem et al., 1999). Statins can inhibit the production of PAI-1 by regulating a variety of signaling pathways as well (Ma et al., 2002; Laumen et al., 2008; Dunoyer-Geindre et al., 2011; Ni et al., 2013). However, the role of these drugs in cancer remains unclear. Apart from these traditional drugs, there are many more novel drugs under development. Due to the crucial role of reactive center loop (RCL) in inhibitory mechanism of PAI-1, several synthetic peptides that mimic various parts of the RCL of PAI-1 were developed (Eitzman et al., 1995; Kvassman et al., 1995; Xue et al., 1998; D’Amico et al., 2012). In general, peptides mimicking the C-terminal part of the loop can accelerate the irreversible transition of PAI-1 to its latent form, while peptides mimicking the N-terminal part can induce it to be cleaved (D’Amico et al., 2012). In addition, several RNA aptamers designed to block the interaction of pai-1 with its partner have been developed as well, and have shown the ability to reduce cancer migration, invasion, and angiogenesis (Blake et al., 2009; Damare et al., 2014; Fortenberry et al., 2016). Another class of PAI-1 inhibitors is small molecules. These compounds work by binding a common binding pocket within the flexible joint region of PAI-1 or by interfering structural elements within that region through interactions at the surface of PAI-1, thereby inducing the substrate behavior of PAI-1 and its conversion to an inert form (Egelund et al., 2001; Fjellström et al., 2013; Lin et al., 2013; Sillen et al., 2021). There are also lots of antibody based PAI-1 inhibitors, including antibodies and antibody derivatives. Their target sits and mechanisms are more extensive and complex than drugs mentioned above (Sillen and Declerck, 2020). However, although these different types of novel PAI-1inhibitors have been shown to be efficient in vivo or in vitro, their role and safety in cancers remain unclear and require more research and clinical trials to understand them, but they still promise a bright future for cancer therapies based on SERPINE1
Admittedly, there are several limitations to our study. First, there are some contradictory findings in our study. For example, in the Cox regression analysis, the result based on RECA-EU project data from ICGC database indicated a protective role of SERPINE1 in renal cell carcinoma, which is contrary to the results of other datasets. We speculate that this may be related to the heterogeneity of samples from different datasets, and the sample size of this dataset (n = 91) is smaller than that of TCGA database, but further studies and follow-up are still needed to verify it. In addition, the results of different cancers are not always consistent in pan-cancer analysis. Therefore, further research focused on the differences in SERPINE1 roles among cancers is needed as well. Second, although we have identified the prognostic value and possible action mechanisms of SERPINE1 in cancers through correlation analysis in the present study, direct evidence supporting these conclusions are required. Finally, our research is mainly based on public databases, which may have inevitably introduced systemic bias; further experimental verification is therefore needed.
5 Conclusion
In the present study, we conducted a comprehensive multi-omics analysis of SERPINE1 in pan-cancer, revealing its prognostic value and potential action mechanisms in cancers. The aberrant expression of SERPINE1 is common in cancers and is associated with patient prognosis, cancer immunity, immunotherapy response and drug sensitivities. SERPINE1 may thus be a promising new target for cancer diagnosis and treatment.
Data availability statement
The datasets presented in this study can be found in online repositories. The names of the repository/repositories and accession number(s) can be found in the article/Supplementary Material.
Ethics statement
The studies involving humans were approved by The Ethics Committee of the Sir Run Run Shaw Hospital, Zhejiang University. The studies were conducted in accordance with the local legislation and institutional requirements. The participants provided their written informed consent to participate in this study.
Author contributions
FL, LqL, LyL, and MW performed bioinformatic analysis. ZX, HH, and YL performed and analyzed experiments. SY and LG were involved in data analysis and interpretation. FL, LG, and MW designed the experiment, interpreted the data, and wrote the manuscript. All authors contributed to the article and approved the submitted version.
Conflict of interest
The authors declare that the research was conducted in the absence of any commercial or financial relationships that could be construed as a potential conflict of interest.
Publisher’s note
All claims expressed in this article are solely those of the authors and do not necessarily represent those of their affiliated organizations, or those of the publisher, the editors and the reviewers. Any product that may be evaluated in this article, or claim that may be made by its manufacturer, is not guaranteed or endorsed by the publisher.
Supplementary material
The Supplementary Material for this article can be found online at: https://www.frontiersin.org/articles/10.3389/fphar.2023.1213891/full#supplementary-material
References
Aran, D., Hu, Z., and Butte, A. J. (2017). xCell: digitally portraying the tissue cellular heterogeneity landscape. Genome Biol. 18, 220. doi:10.1186/s13059-017-1349-1
Bae, E. A., Seo, H., Kim, I. K., Jeon, I., and Kang, C. Y. (2019). Roles of NKT cells in cancer immunotherapy. Arch. Pharm. Res. 42, 543–548. doi:10.1007/s12272-019-01139-8
Bajou, K., Noël, A., Gerard, R. D., Masson, V., Brunner, N., Holst-Hansen, C., et al. (1998). Absence of host plasminogen activator inhibitor 1 prevents cancer invasion and vascularization. Nat. Med. 4, 923–928. doi:10.1038/nm0898-923
Baluta, M. M., and Vintila, M. M. (2015). PAI-1 inhibition - another therapeutic option for cardiovascular protection. Maedica (Bucur) 10, 147–152.
Barretina, J., Caponigro, G., Stransky, N., Venkatesan, K., Margolin, A. A., Kim, S., et al. (2012). The Cancer Cell Line Encyclopedia enables predictive modelling of anticancer drug sensitivity. Nature 483, 603–607. doi:10.1038/nature11003
Becht, E., Giraldo, N. A., Lacroix, L., Buttard, B., Elarouci, N., Petitprez, F., et al. (2016). Estimating the population abundance of tissue-infiltrating immune and stromal cell populations using gene expression. Genome Biol. 17, 218. doi:10.1186/s13059-016-1070-5
Becker, M., Szarvas, T., Wittschier, M., vom Dorp, F., Tötsch, M., Schmid, K. W., et al. (2010). Prognostic impact of plasminogen activator inhibitor type 1 expression in bladder cancer. Cancer 116, 4502–4512. doi:10.1002/cncr.25326
Beroukhim, R., Mermel, C. H., Porter, D., Wei, G., Raychaudhuri, S., Donovan, J., et al. (2010). The landscape of somatic copy-number alteration across human cancers. Nature 463, 899–905. doi:10.1038/nature08822
Blake, C. M., Sullenger, B. A., Lawrence, D. A., and Fortenberry, Y. M. (2009). Antimetastatic potential of PAI-1-specific RNA aptamers. Oligonucleotides 19, 117–128. doi:10.1089/oli.2008.0177
Bonneville, R., Krook, M. A., Kautto, E. A., Miya, J., Wing, M. R., Chen, H. Z., et al. (2017). Landscape of microsatellite instability across 39 cancer types. JCO Precis. Oncol. 1, 1–15. doi:10.1200/PO.17.00073
Brown, N. J., Abbas, A., Byrne, D., Schoenhard, J. A., and Vaughan, D. E. (2002a). Comparative effects of estrogen and angiotensin-converting enzyme inhibition on plasminogen activator inhibitor-1 in healthy postmenopausal women. Circulation 105, 304–309. doi:10.1161/hc0302.102570
Brown, N. J., Agirbasli, M. A., Williams, G. H., Litchfield, W. R., and Vaughan, D. E. (1998). Effect of activation and inhibition of the renin-angiotensin system on plasma PAI-1. Hypertension 32, 965–971. doi:10.1161/01.hyp.32.6.965
Brown, N. J., Kumar, S., Painter, C. A., and Vaughan, D. E. (2002b). ACE inhibition versus angiotensin type 1 receptor antagonism: differential effects on PAI-1 over time. Hypertension 40, 859–865. doi:10.1161/01.hyp.0000040264.15961.48
Cai, Z., Chen, J., Yu, Z., Li, H., Liu, Z., Deng, D., et al. (2023). BCAT2 shapes a noninflamed tumor microenvironment and induces resistance to anti-PD-1/PD-L1 immunotherapy by negatively regulating proinflammatory chemokines and anticancer immunity. Adv. Sci. (Weinh) 10, e2207155. doi:10.1002/advs.202207155
Cancer Genome Atlas Research Network (2013). Comprehensive molecular characterization of clear cell renal cell carcinoma. Nature 499, 43–49. doi:10.1038/nature12222
Cerami, E., Gao, J., Dogrusoz, U., Gross, B. E., Sumer, S. O., Aksoy, B. A., et al. (2012). The cBio cancer genomics portal: an open platform for exploring multidimensional cancer genomics data. Cancer Discov. 2, 401–404. doi:10.1158/2159-8290.CD-12-0095
Chen, D. S., and Mellman, I. (2013). Oncology meets immunology: the cancer-immunity cycle. Immunity 39, 1–10. doi:10.1016/j.immuni.2013.07.012
Chen, T. Y., Zhou, M., Lin, M. Q., Liang, S. T., Yan, Y., Wang, S. M., et al. (2021a). Research progress on the SERPINE1 protein and chronic inflammatory diseases of the upper respiratory tract: a literature review. Int. Arch. Allergy Immunol. 182, 1097–1102. doi:10.1159/000516195
Chen, X., and Song, E. (2019). Turning foes to friends: targeting cancer-associated fibroblasts. Nat. Rev. Drug Discov. 18, 99–115. doi:10.1038/s41573-018-0004-1
Chen, Y., McAndrews, K. M., and Kalluri, R. (2021b). Clinical and therapeutic relevance of cancer-associated fibroblasts. Nat. Rev. Clin. Oncol. 18, 792–804. doi:10.1038/s41571-021-00546-5
Clark, D. J., Dhanasekaran, S. M., Petralia, F., Pan, J., Song, X., Hu, Y., et al. (2019). Integrated proteogenomic characterization of clear cell renal cell carcinoma. Cell. 179, 964–983.e31. doi:10.1016/j.cell.2019.10.007
Damare, J., Brandal, S., and Fortenberry, Y. M. (2014). Inhibition of PAI-1 antiproteolytic activity against tPA by RNA aptamers. Nucleic Acid. Ther. 24, 239–249. doi:10.1089/nat.2013.0475
D’Amico, S., Martial, J. A., and Struman, I. (2012). A peptide mimicking the C-terminal part of the reactive center loop induces the transition to the latent form of plasminogen activator inhibitor type-1. FEBS Lett. 586, 686–692. doi:10.1016/j.febslet.2012.02.013
de Visser, K. E., and Joyce, J. A. (2023). The evolving tumor microenvironment: from cancer initiation to metastatic outgrowth. Cancer Cell. 41, 374–403. doi:10.1016/j.ccell.2023.02.016
Declerck, P. J., and Gils, A. (2013). Three decades of research on plasminogen activator inhibitor-1: a multifaceted serpin. Semin. Thromb. Hemost. 39, 356–364. doi:10.1055/s-0033-1334487
Duffy, M. J., McGowan, P. M., Harbeck, N., Thomssen, C., and Schmitt, M. (2014). uPA and PAI-1 as biomarkers in breast cancer: validated for clinical use in level-of-evidence-1 studies. Breast Cancer Res. 16, 428. doi:10.1186/s13058-014-0428-4
Dunoyer-Geindre, S., Fish, R. J., and Kruithof, E. K. O. (2011). Regulation of the endothelial plasminogen activator system by fluvastatin. Role of Rho family proteins, actin polymerisation and p38 MAP kinase. Thromb. Haemost. 105, 461–472. doi:10.1160/TH10-07-0444
Egelund, R., Einholm, A. P., Pedersen, K. E., Nielsen, R. W., Christensen, A., Deinum, J., et al. (2001). A regulatory hydrophobic area in the flexible joint region of plasminogen activator inhibitor-1, defined with fluorescent activity-neutralizing ligands. Ligand-induced serpin polymerization. J. Biol. Chem. 276, 13077–13086. doi:10.1074/jbc.M009024200
Eitzman, D. T., Fay, W. P., Lawrence, D. A., Francis-Chmura, A. M., Shore, J. D., Olson, S. T., et al. (1995). Peptide-mediated inactivation of recombinant and platelet plasminogen activator inhibitor-1 in vitro. J. Clin. Invest. 95, 2416–2420. doi:10.1172/JCI117937
Erdem, Y., Usalan, C., Haznedaroğlu, I. C., Altun, B., Arici, M., Yasavul, U., et al. (1999). Effects of angiotensin converting enzyme and angiotensin II receptor inhibition on impaired fibrinolysis in systemic hypertension. Am. J. Hypertens. 12, 1071–1076. doi:10.1016/s0895-7061(99)00145-4
Ersoy, C., Kiyici, S., Budak, F., Oral, B., Guclu, M., Duran, C., et al. (2008). The effect of metformin treatment on VEGF and PAI-1 levels in obese type 2 diabetic patients. Diabetes Res. Clin. Pract. 81, 56–60. doi:10.1016/j.diabres.2008.02.006
Finotello, F., Mayer, C., Plattner, C., Laschober, G., Rieder, D., Hackl, H., et al. (2019). Molecular and pharmacological modulators of the tumor immune contexture revealed by deconvolution of RNA-seq data. Genome Med. 11, 34. doi:10.1186/s13073-019-0638-6
Fjellström, O., Deinum, J., Sjögren, T., Johansson, C., Geschwindner, S., Nerme, V., et al. (2013). Characterization of a small molecule inhibitor of plasminogen activator inhibitor type 1 that accelerates the transition into the latent conformation. J. Biol. Chem. 288, 873–885. doi:10.1074/jbc.M112.371732
Fortenberry, Y. M., Brandal, S. M., Carpentier, G., Hemani, M., and Pathak, A. P. (2016). Intracellular expression of PAI-1 specific aptamers alters breast cancer cell migration, invasion and angiogenesis. PLoS One 11, e0164288. doi:10.1371/journal.pone.0164288
Fortenberry, Y. M. (2013). Plasminogen activator inhibitor-1 inhibitors: a patent review (2006-present). Expert Opin. Ther. Pat. 23, 801–815. doi:10.1517/13543776.2013.782393
Fridman, W. H., Pagès, F., Sautès-Fridman, C., and Galon, J. (2012). The immune contexture in human tumours: impact on clinical outcome. Nat. Rev. Cancer 12, 298–306. doi:10.1038/nrc3245
Fu, J., Li, K., Zhang, W., Wan, C., Zhang, J., Jiang, P., et al. (2020). Large-scale public data reuse to model immunotherapy response and resistance. Genome Med. 12, 21. doi:10.1186/s13073-020-0721-z
Goldman, M. J., Craft, B., Hastie, M., Repečka, K., McDade, F., Kamath, A., et al. (2020). Visualizing and interpreting cancer genomics data via the Xena platform. Nat. Biotechnol. 38, 675–678. doi:10.1038/s41587-020-0546-8
Gomes-Giacoia, E., Miyake, M., Goodison, S., and Rosser, C. J. (2013). Targeting plasminogen activator inhibitor-1 inhibits angiogenesis and tumor growth in a human cancer xenograft model. Mol. Cancer Ther. 12, 2697–2708. doi:10.1158/1535-7163.MCT-13-0500
Gu, Z., Eils, R., and Schlesner, M. (2016). Complex heatmaps reveal patterns and correlations in multidimensional genomic data. Bioinformatics 32, 2847–2849. doi:10.1093/bioinformatics/btw313
Gutierrez, L. S., Schulman, A., Brito-Robinson, T., Noria, F., Ploplis, V. A., and Castellino, F. J. (2000). Tumor development is retarded in mice lacking the gene for urokinase-type plasminogen activator or its inhibitor, plasminogen activator inhibitor-1. Cancer Res. 60, 5839–5847.
Gutiérrez-Melo, N., and Baumjohann, D. (2023). T follicular helper cells in cancer. Trends Cancer 9, 309–325. doi:10.1016/j.trecan.2022.12.007
Han, Y., Wang, Y., Dong, X., Sun, D., Liu, Z., Yue, J., et al. (2022). TISCH2: expanded datasets and new tools for single-cell transcriptome analyses of the tumor microenvironment. Nucleic Acids Res. 51, D1425–D1431. doi:10.1093/nar/gkac959
Hanekom, G. S., Stubbings, H. M., and Kidson, S. H. (2002). The active fraction of plasmatic plasminogen activator inhibitor type 1 as a possible indicator of increased risk for metastatic melanoma. Cancer Detect Prev. 26, 50–59. doi:10.1016/s0361-090x(02)00002-8
Hu, J., Chen, J., Ou, Z., Chen, H., Liu, Z., Chen, M., et al. (2022). Neoadjuvant immunotherapy, chemotherapy, and combination therapy in muscle-invasive bladder cancer: a multi-center real-world retrospective study. Cell. Rep. Med. 3, 100785. doi:10.1016/j.xcrm.2022.100785
Iwaki, T., Urano, T., and Umemura, K. (2012). PAI-1, progress in understanding the clinical problem and its aetiology. Br. J. Haematol. 157, 291–298. doi:10.1111/j.1365-2141.2012.09074.x
Jevrić, M., Matić, I. Z., Krivokuća, A., Đorđić Crnogorac, M., Besu, I., Damjanović, A., et al. (2019). Association of uPA and PAI-1 tumor levels and 4G/5G variants of PAI-1 gene with disease outcome in luminal HER2-negative node-negative breast cancer patients treated with adjuvant endocrine therapy. BMC Cancer 19, 71. doi:10.1186/s12885-018-5255-z
Juhan-Vague, I., and Alessi, M. C. (1997). PAI-1, obesity, insulin resistance and risk of cardiovascular events. Thromb. Haemost. 78, 656–660. doi:10.1055/s-0038-1657607
Kohler, H. P., and Grant, P. J. (2000). Plasminogen-activator inhibitor type 1 and coronary artery disease. N. Engl. J. Med. 342, 1792–1801. doi:10.1056/NEJM200006153422406
Kutz, S. M., Hordines, J., McKeown-Longo, P. J., and Higgins, P. J. (2001). TGF-beta1-induced PAI-1 gene expression requires MEK activity and cell-to-substrate adhesion. J. Cell. Sci. 114, 3905–3914. doi:10.1242/jcs.114.21.3905
Kvassman, J. O., Lawrence, D. A., and Shore, J. D. (1995). The acid stabilization of plasminogen activator inhibitor-1 depends on protonation of a single group that affects loop insertion into beta-sheet A. J. Biol. Chem. 270, 27942–27947. doi:10.1074/jbc.270.46.27942
Laskowski, T. J., Biederstädt, A., and Rezvani, K. (2022). Natural killer cells in antitumour adoptive cell immunotherapy. Nat. Rev. Cancer 22, 557–575. doi:10.1038/s41568-022-00491-0
Laumen, H., Skurk, T., and Hauner, H. (2008). The HMG-CoA reductase inhibitor rosuvastatin inhibits plasminogen activator inhibitor-1 expression and secretion in human adipocytes. Atherosclerosis 196, 565–573. doi:10.1016/j.atherosclerosis.2007.06.005
Laumont, C. M., Banville, A. C., Gilardi, M., Hollern, D. P., and Nelson, B. H. (2022). Tumour-infiltrating B cells: immunological mechanisms, clinical impact and therapeutic opportunities. Nat. Rev. Cancer 22, 414–430. doi:10.1038/s41568-022-00466-1
Li, H., Chen, J., Li, Z., Chen, M., Ou, Z., Mo, M., et al. (2023a). S100A5 attenuates efficiency of anti-PD-L1/PD-1 immunotherapy by inhibiting CD8+ T cell-mediated anti-cancer immunity in bladder carcinoma. Adv. Sci. (Weinh) 2023, e2300110. doi:10.1002/advs.202300110
Li, T., Fu, J., Zeng, Z., Cohen, D., Li, J., Chen, Q., et al. (2020). TIMER2.0 for analysis of tumor-infiltrating immune cells. Nucleic Acids Res. 48, W509–W514. doi:10.1093/nar/gkaa407
Li, Y. Z., Xie, J., Wang, R. Q., Gao, X. Q., Liu, P. J., and Liu, J. (2023b). KLF2 is a clinical diagnostic and treatment biomarker of breast cancer. Front. Cell. Dev. Biol. 11, 1182123. doi:10.3389/fcell.2023.1182123
Lin, Z., Jensen, J. K., Hong, Z., Shi, X., Hu, L., Andreasen, P. A., et al. (2013). Structural insight into inactivation of plasminogen activator inhibitor-1 by a small-molecule antagonist. Chem. Biol. 20, 253–261. doi:10.1016/j.chembiol.2013.01.002
Liu, C. J., Hu, F. F., Xie, G. Y., Miao, Y. R., Li, X. W., Zeng, Y., et al. (2023). Gsca: an integrated platform for gene set cancer analysis at genomic, pharmacogenomic and immunogenomic levels. Brief. Bioinform 24, bbac558. doi:10.1093/bib/bbac558
Love, M. I., Huber, W., and Anders, S. (2014). Moderated estimation of fold change and dispersion for RNA-seq data with DESeq2. Genome Biol. 15, 550. doi:10.1186/s13059-014-0550-8
Luna, A., Elloumi, F., Varma, S., Wang, Y., Rajapakse, V. N., Aladjem, M. I., et al. (2021). CellMiner Cross-Database (CellMinerCDB) version 1.2: exploration of patient-derived cancer cell line pharmacogenomics. Nucleic Acids Res. 49, D1083–D1093. doi:10.1093/nar/gkaa968
Ma, Y., Ryu, J. S., Dulay, A., Segal, M., and Guller, S. (2002). Regulation of plasminogen activator inhibitor (PAI)-1 expression in a human trophoblast cell line by glucocorticoid (GC) and transforming growth factor (TGF)-beta. Placenta 23, 727–734. doi:10.1016/s0143-4004(02)90863-5
Mashiko, S., Kitatani, K., Toyoshima, M., Ichimura, A., Dan, T., Usui, T., et al. (2015). Inhibition of plasminogen activator inhibitor-1 is a potential therapeutic strategy in ovarian cancer. Cancer Biol. Ther. 16, 253–260. doi:10.1080/15384047.2014.1001271
Masuda, T., Hattori, N., Senoo, T., Akita, S., Ishikawa, N., Fujitaka, K., et al. (2013). SK-216, an inhibitor of plasminogen activator inhibitor-1, limits tumor progression and angiogenesis. Mol. Cancer Ther. 12, 2378–2388. doi:10.1158/1535-7163.MCT-13-0041
Mayakonda, A., Lin, D. C., Assenov, Y., Plass, C., and Koeffler, H. P. (2018). Maftools: efficient and comprehensive analysis of somatic variants in cancer. Genome Res. 28, 1747–1756. doi:10.1101/gr.239244.118
Morgan, A. E., Davies, T. J., and Mc Auley, M. T. (2018). The role of DNA methylation in ageing and cancer. Proc. Nutr. Soc. 77, 412–422. doi:10.1017/S0029665118000150
Nakatsuka, E., Sawada, K., Nakamura, K., Yoshimura, A., Kinose, Y., Kodama, M., et al. (2017). Plasminogen activator inhibitor-1 is an independent prognostic factor of ovarian cancer and IMD-4482, a novel plasminogen activator inhibitor-1 inhibitor, inhibits ovarian cancer peritoneal dissemination. Oncotarget 8, 89887–89902. doi:10.18632/oncotarget.20834
Newman, A. M., Liu, C. L., Green, M. R., Gentles, A. J., Feng, W., Xu, Y., et al. (2015). Robust enumeration of cell subsets from tissue expression profiles. Nat. Methods 12, 453–457. doi:10.1038/nmeth.3337
Ni, X. Q., Zhu, J. H., Yao, N. H., Qian, J., and Yang, X. J. (2013). Statins suppress glucose-induced plasminogen activator inhibitor-1 expression by regulating RhoA and nuclear factor-κB activities in cardiac microvascular endothelial cells. Exp. Biol. Med. (Maywood) 238, 37–46. doi:10.1258/ebm.2012.012127
Nordt, T. K., Bode, C., and Sobel, B. E. (2001). Stimulation in vivo of expression of intra-abdominal adipose tissue plasminogen activator inhibitor Type I by proinsulin. Diabetologia 44, 1121–1124. doi:10.1007/s001250100618
Placencio, V. R., and DeClerck, Y. A. (2015). Plasminogen activator inhibitor-1 in cancer: rationale and insight for future therapeutic testing. Cancer Res. 75, 2969–2974. doi:10.1158/0008-5472.CAN-15-0876
Placencio, V. R., Ichimura, A., Miyata, T., and DeClerck, Y. A. (2015). Small molecule inhibitors of plasminogen activator inhibitor-1 elicit anti-tumorigenic and anti-angiogenic activity. PLoS One 10, e0133786. doi:10.1371/journal.pone.0133786
Pös, O., Radvanszky, J., Buglyó, G., Pös, Z., Rusnakova, D., Nagy, B., et al. (2021). DNA copy number variation: main characteristics, evolutionary significance, and pathological aspects. Biomed. J. 44, 548–559. doi:10.1016/j.bj.2021.02.003
Racle, J., de Jonge, K., Baumgaertner, P., Speiser, D. E., and Gfeller, D. (2017). Simultaneous enumeration of cancer and immune cell types from bulk tumor gene expression data. Elife 6, e26476. doi:10.7554/eLife.26476
Sakamoto, T., Woodcock-Mitchell, J., Marutsuka, K., Mitchell, J. J., Sobel, B. E., and Fujii, S. (1999). TNF-alpha and insulin, alone and synergistically, induce plasminogen activator inhibitor-1 expression in adipocytes. Am. J. Physiol. 276, C1391–C1397. doi:10.1152/ajpcell.1999.276.6.C1391
Sica, A., and Mantovani, A. (2012). Macrophage plasticity and polarization: in vivo veritas. J. Clin. Invest. 122, 787–795. doi:10.1172/JCI59643
Siegel, R. L., Miller, K. D., Wagle, N. S., and Jemal, A. (2023). Cancer statistics, 2023. CA Cancer J. Clin. 73, 17–48. doi:10.3322/caac.21763
Sillen, M., and Declerck, P. J. (2021). A narrative review on plasminogen activator inhibitor-1 and its (Patho)Physiological role: to target or not to target? Int. J. Mol. Sci. 22, 2721. doi:10.3390/ijms22052721
Sillen, M., and Declerck, P. J. (2020). Targeting PAI-1 in cardiovascular disease: structural insights into PAI-1 functionality and inhibition. Front. Cardiovasc Med. 7, 622473. doi:10.3389/fcvm.2020.622473
Sillen, M., Miyata, T., Vaughan, D. E., Strelkov, S. V., and Declerck, P. J. (2021). Structural insight into the two-step mechanism of PAI-1 inhibition by small molecule TM5484. Int. J. Mol. Sci. 22, 1482. doi:10.3390/ijms22031482
Sotiropoulos, G., Kotopouli, M., Karampela, I., Christodoulatos, G. S., Antonakos, G., Marinou, I., et al. (2019). Circulating plasminogen activator inhibitor-1 activity: a biomarker for resectable non-small cell lung cancer? J. BUON 24, 943–954.
St Paul, M., and Ohashi, P. S. (2020). The roles of CD8+ T cell subsets in antitumor immunity. Trends Cell. Biol. 30, 695–704. doi:10.1016/j.tcb.2020.06.003
Sung, H., Ferlay, J., Siegel, R. L., Laversanne, M., Soerjomataram, I., Jemal, A., et al. (2021). Global cancer statistics 2020: GLOBOCAN estimates of incidence and mortality worldwide for 36 cancers in 185 countries. CA Cancer J. Clin. 71, 209–249. doi:10.3322/caac.21660
Takayama, Y., Hattori, N., Hamada, H., Masuda, T., Omori, K., Akita, S., et al. (2016). Inhibition of PAI-1 limits tumor angiogenesis regardless of angiogenic stimuli in malignant pleural mesothelioma. Cancer Res. 76, 3285–3294. doi:10.1158/0008-5472.CAN-15-1796
Tan, P., Chen, H., Huang, Z., Huang, M., Du, Y., Li, T., et al. (2021). MMP25-AS1/hsa-miR-10a-5p/SERPINE1 axis as a novel prognostic biomarker associated with immune cell infiltration in KIRC. Mol. Ther. Oncolytics 22, 307–325. doi:10.1016/j.omto.2021.07.008
Teng, F., Zhang, J., Chen, Y., Shen, X., Su, C., Guo, Y., et al. (2021). LncRNA NKX2-1-AS1 promotes tumor progression and angiogenesis via upregulation of SERPINE1 expression and activation of the VEGFR-2 signaling pathway in gastric cancer. Mol. Oncol. 15, 1234–1255. doi:10.1002/1878-0261.12911
Thorsson, V., Gibbs, D. L., Brown, S. D., Wolf, D., Bortone, D. S., Ou Yang, T. H., et al. (2018). The immune landscape of cancer. Immunity 48, 812–830. doi:10.1016/j.immuni.2018.03.023
Togashi, Y., Shitara, K., and Nishikawa, H. (2019). Regulatory T cells in cancer immunosuppression - implications for anticancer therapy. Nat. Rev. Clin. Oncol. 16, 356–371. doi:10.1038/s41571-019-0175-7
Xu, L., Deng, C., Pang, B., Zhang, X., Liu, W., Liao, G., et al. (2018). Tip: a web server for resolving tumor Immunophenotype profiling. Cancer Res. 78, 6575–6580. doi:10.1158/0008-5472.CAN-18-0689
Xue, Y., Björquist, P., Inghardt, T., Linschoten, M., Musil, D., Sjölin, L., et al. (1998). Interfering with the inhibitory mechanism of serpins: crystal structure of a complex formed between cleaved plasminogen activator inhibitor type 1 and a reactive-centre loop peptide. Structure 6, 627–636. doi:10.1016/s0969-2126(98)00064-1
Yoshihara, K., Shahmoradgoli, M., Martínez, E., Vegesna, R., Kim, H., Torres-Garcia, W., et al. (2013). Inferring tumour purity and stromal and immune cell admixture from expression data. Nat. Commun. 4, 2612. doi:10.1038/ncomms3612
Yuan, H., Yan, M., Zhang, G., Liu, W., Deng, C., Liao, G., et al. (2019). CancerSEA: a cancer single-cell state atlas. Nucleic Acids Res. 47, D900–D908. doi:10.1093/nar/gky939
Zeng, D., Ye, Z., Shen, R., Yu, G., Wu, J., Xiong, Y., et al. (2021). Iobr: multi-omics immuno-oncology biological research to decode tumor microenvironment and Signatures. Front. Immunol. 12, 687975. doi:10.3389/fimmu.2021.687975
Zeng, Z., Wong, C. J., Yang, L., Ouardaoui, N., Li, D., Zhang, W., et al. (2022). Tismo: syngeneic mouse tumor database to model tumor immunity and immunotherapy response. Nucleic Acids Res. 50, D1391–D1397. doi:10.1093/nar/gkab804
Keywords: SERPINE1, pan-cancer, multi-omics, clear cell renal cell carcinoma, cancer immunity
Citation: Li L, Li F, Xu Z, Li L, Hu H, Li Y, Yu S, Wang M and Gao L (2023) Identification and validation of SERPINE1 as a prognostic and immunological biomarker in pan-cancer and in ccRCC. Front. Pharmacol. 14:1213891. doi: 10.3389/fphar.2023.1213891
Received: 28 April 2023; Accepted: 07 August 2023;
Published: 23 August 2023.
Edited by:
Linhui Wang, Second Military Medical University, ChinaReviewed by:
Min Tang, Nanjing Medical University, ChinaYing Luo, East China Normal University, China
Jiao Hu, Central South University, China
Copyright © 2023 Li, Li, Xu, Li, Hu, Li, Yu, Wang and Gao. This is an open-access article distributed under the terms of the Creative Commons Attribution License (CC BY). The use, distribution or reproduction in other forums is permitted, provided the original author(s) and the copyright owner(s) are credited and that the original publication in this journal is cited, in accordance with accepted academic practice. No use, distribution or reproduction is permitted which does not comply with these terms.
*Correspondence: Mingchao Wang, 3316019@zju.edu.cn; Lei Gao, drgaolei0417@zju.edu.cn
†These authors have contributed equally to this work and share first authorship