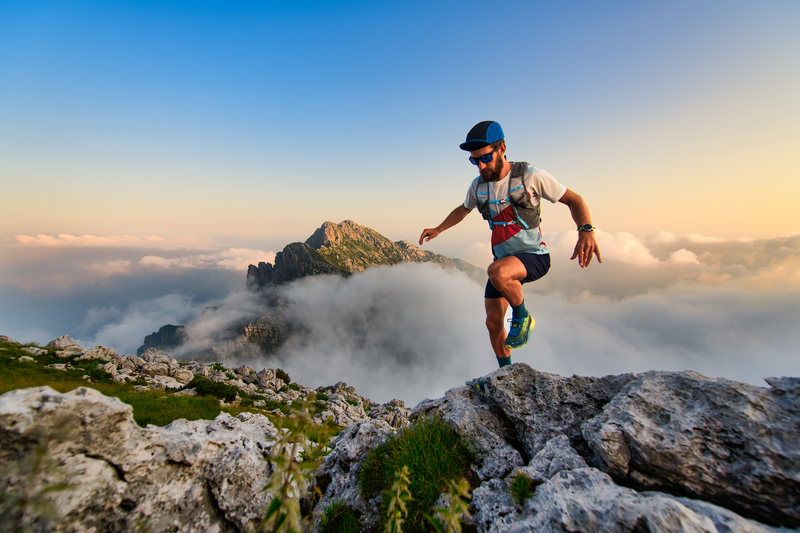
95% of researchers rate our articles as excellent or good
Learn more about the work of our research integrity team to safeguard the quality of each article we publish.
Find out more
ORIGINAL RESEARCH article
Front. Pharmacol. , 10 April 2023
Sec. Experimental Pharmacology and Drug Discovery
Volume 14 - 2023 | https://doi.org/10.3389/fphar.2023.1143437
This article is part of the Research Topic Experimental and computational aspects of bioactive proteins from animal venoms: An insight into pharmacological properties and drug discovery View all 10 articles
Introduction: Most elapid snakes produce venoms that contain alpha-neurotoxins (α-NTXs), which are proteins that cause post-synaptic blockade and paralysis in snakebite envenoming. However, existing elapid antivenoms are known for their low potency in neutralizing the neurotoxic activity of α-NTXs, while the immunological basis has not been elucidated.
Methods: In this study, a structure-based major histocompatibility complex II (MHCII) epitope predictor of horse (Equus caballus), complemented with DM-editing determinant screening algorithm was adopted to assess the immunogenicity of α-NTXs in the venoms of major Asiatic elapids (Naja kaouthia, Ophiophagus hannah, Laticauda colubrina, Hydrophis schistosus, Hydrophis curtus).
Results: The scoring metric M2R, representing the relative immunogenic performance of respective α-NTXs, showed all α-NTXs have an overall low M2R of <0.3, and most of the predicted binders feature non-optimal P1 anchor residues. The M2R scores correlate strongly (R2 = 0.82) with the potency scores (p-score) generated based on the relative abundances of α-NTXs and the neutralization potency of commercial antivenoms.
Discussion: The immunoinformatic analysis indicates that the inferior antigenicity of α-NTXs is not only due to their small molecular size but also the subpar immunogenicity affected by their amino acid composition. Structural modification with conjugation and synthetic epitope as immunogen may potentially enhance the immunogenicity for improved antivenom potency against α-NTXs of elapid snakes.
Alpha-neurotoxins (α-NTXs) belong to the three-finger toxins (3FTx) family and are commonly found in the venoms of most Asiatic elapid snakes, e.g., cobras (Naja spp.), sea snakes (Hydrophis spp.), King Cobra (Ophiphagus hannah) and kraits (Bungarus spp.) (Tan et al., 2015a; Tan et al., 2015b; Tan et al., 2015c; Leong et al., 2015; Oh et al., 2021). Depending on the amino acid composition, the α-NTXs are further classified into short neurotoxins (SαNTX, 6–7 kDa) and long neurotoxins (LαNTX, 7–9 kDa) (Barber et al., 2013). As antagonists of post-synaptic nicotinic acetylcholine receptors (nAChR) of neuromuscular junctions, these non-enzymatic neurotoxins account for the neurotoxicity in most elapid envenomation, resulting in a fast onset of flaccid systemic paralysis, with death ensuing rapidly in the absence of proper treatment (Barber et al., 2013; Ranawaka et al., 2013).
Antivenoms, which contain animal immunoglobulins or derivatives raised against snake venoms, remain the definitive treatment for snakebite envenoming (World Health Organization, 2016). However, earlier studies consistently showed elapid antivenoms have low efficacy, with neutralization potency (P, expressed in the amount of venom neutralized completely per milliliter of antivenom) well below 1 mg/ml (Leong et al., 2012; Leong et al., 2015). In contrast, viperid antivenoms appear to have higher efficacy, with Potency (P) values up to 10 mg/ml (Leong et al., 2014). The lower efficacy of elapid antivenoms would inevitably lead to the need for a higher dose of antivenom treatment, resulting in higher medical expenses, curtail of antivenom stockpile, and at the same time exposing patients to a greater risk of hypersensitive reactions that can be fatal (World Health Organization, 2016; Patikorn et al., 2022). The elapid antivenom efficacy is particularly low in neutralizing venom lethality driven by α-NTXs (Ratanabanangkoon et al., 2016; Wong et al., 2016; Tan et al., 2017), suggesting inadequate immunogenic capacity of the α-NTX for the production of neurotoxin-specific antibodies. In this regard, factors such as the size, dose and foreignness of a molecule are known to affect its immunogenicity as an antigen during immunization (Clementi et al., 1991; Irais et al., 2020). The small size of α-NTX indicates a lower number of epitopes in eliciting an immune response, and the immunogenicity may be further compromised by other larger snake venom toxins present in an immunogen mixture. To overcome the current predicament, the concept of “diverse toxin repertoire” has been proposed and trialled whereby the pool of α-NTXs of various elapid species was used to enrich the venom immunogen mixture containing minimum high molecular weight (MW) components for hyperimmunization in horses (Ratanabanangkoon, 2021). The resultant antisera showed an exceptionally broad para-specificity of neutralization against diverse elapid venoms, while the strategy did not translate into a major improvement of neutralizing potency per individual snake species (Ratanabanangkoon et al., 2016; Ratanabanangkoon et al., 2020). Furthermore, even antivenoms raised against elapid venoms that contain abundant α-NTX (>40% of total venom proteins) showed generally low neutralizing potency, questioning if the “dose” of α-NTX is indeed the key determinant of eliciting good immunization response (Ratanabanangkoon et al., 2016; Palasuberniam et al., 2021; Tan et al., 2021). Hypothetically, there are other factors at play that intrinsically modulate the makeup of epitope(s) in the α-NTX, and this results in variable immunorecognition by the B-cell receptor (BCR) and/or T-cell receptor (TCR) for antibody production.
Conventionally, antivenom production exploits the adaptive immune system of host animals such as horses, in which venom proteins are used as specific antigens to strategically mount a humoral immune response. This entails the activation and maturation of naïve B-cells into antibody-producing plasma B-cells, under the regulation of helper T cells (also called CD4+ cells). To produce specific venom-targeting antibodies, the venom antigens must first be recognized, bound and internalized by professional antigen-presenting cells (APC) which include the naïve B-cells (via the BCR), and other phagocytes, e.g., macrophages and dendritic cells. The endocytosed venom proteins are degraded in the endo-lysosomal compartments, yielding peptide fragments as antigens that will be loaded onto major histocompatibility complex II (MHCII) and displayed on the external cell membrane. The MCHII-loaded peptide antigens displayed on phagocytes, especially dendritic cells, will be recognized by naïve T helper cells (Th cells) via the TCR, activating them into effector and memory Th cells. The effector Th cells recognize the particular antigen peptide displayed by the MCHII complex of B-cells, fully activating them to produce toxin-specific antibodies (immunoglobulin G) with longer half-life and higher affinity, while the same Th cells also release cytokines that mediate proliferation of the activated B-cells (Hoogeboom and Tolar, 2016).
All in all, initiating the venom/toxin-specific humoral immunity requires proper loading of the toxin peptides onto MHCII membrane-bound proteins for subsequent antigen presentation to the Th cells. The MHCII protein is composed of two subunits (α and β) chains, whose α1 and β1 domains of the extra-cellular segments form the antigen-binding groove with multiple pockets destined to bind antigenic peptides (of the exogenous toxins) in the endosomal compartment. From the endoplasmic reticulum where MHCII proteins are assembled, throughout their intracellular transport to the Golgi apparatus and finally to endosomes, the MHCII binding groove is blocked from endogenous peptide binding by the invariant (Ii) chain. In the endosomes, the drastic change in pH partially degrades the MHCII-bound Ii chain while leaving behind a short peptide named CLIP (class II MHC associated Ii peptide) that continues to shield the MHCII binding site. Here, the equine version of human leukocyte antigen-DM (HLA-DM) molecule plays a critical role for peptide exchange, where it accelerates the removal of CLIP and other peptides that lack appropriate anchor residues, thereby “editing” the repertoire of internalized peptides to make way for proper MHCII-peptide loading and subsequent antigen presentation on APCs (Morris et al., 1994; Denzin and Cresswell, 1995; Fung-Leung et al., 1996; Zhou et al., 2009; Schulze et al., 2013). The susceptibility to DM-mediated peptide editing is modulated by the dynamic conformation of MHCII bound with peptide. Previous studies highlighted the importance of the hydrogen bond network formed between peptide backbone and conserved residues in MHCII, especially those at the P1 and P2 pockets which are key sites of peptide binding (Stratikos et al., 2004; Anders et al., 2011; Yin et al., 2014). Accordingly, only strong-binding peptides with appropriate anchor residues for the pockets will succeed in the DM-mediated MHCII peptide exchange, demonstrating immunodominance of epitopes over others that favors their recognition by Th cells in mounting a good humoral immune response. In this context, the understanding of the DM-editing susceptibility of various peptides display of α-NTXs to proper MHCII-peptide loading is important to elucidate the immunogenicity of these venom proteins, which has deep implication in antivenom production. Therefore, the present study aimed to assess immunogenic capacity of α-NTXs by using the modified structure-based predictor in complement with the screening algorithm of DM-editing determinants. In addition, a scoring metric was configured to determine the relative immunogenicity of α-NTXs. It is hoped that this study will provide a deeper understanding about the poor efficacy of elapid antivenoms.
The full amino acid sequence of respective α-NTXs was acquired from UniProt and submitted to the input panel for B-cell epitope prediction with Kolaskar and Tongaonkar antigenicity scale (http://tools.iedb.org/bcell/) (Kolaskar and Tongaonkar, 1990). Selected α-NTXs for this study are: (i) LαNTXs, which include (a) α-cobratoxin (Nk_LαNTX-α-Cbtx, UniProt ID: P01391, Naja kaouthia); (b) long neurotoxin OH55 (Oh_LαNTX-OH55, UniProt ID: Q53B58, O. hannah); and (c) long neurotoxin 1 (Hc_LαNTX-1, UniProt ID: Q8UW29, Hydrophis curtus), and (ii) SαNTXs: (a) cobrotoxin-c (Nk_SαNTX-Cbtx-c, UniProt ID: P59276, N. kaouthia); (b) short neurotoxin 1 (Hs_SαNTX-1, UniProt ID: P68415, Hydrophis schistosus); and (c) short neurotoxin 2 (Lc_SαNTX-2, UniProt ID: P10457, Laticauda colubrina). Zinc metalloproteinase-disintegrin-like atragin (Na_SVMP, Uniprot ID: D3TTC2, Naja atra) was selected as a standard of high MW toxin.
The homology structures of horse (Equus caballus) major histocompatibility complex II (Eqca-DR) were modeled after human HLA-DR1 crystal structure [PDB_ID: 1HXY] obtained from Protein Data Bank (https://www.rcsb.org). With HLA-DR1 selected as a template, molecular modeling was carried out for the two allelic variants of Eqca-DR (Eqca-DRA*00103:DRB2*00201 and Eqca-DRA*00201:DRB2*00201; sequence obtained from EMBL’s European Bioinformatics Institute (https://www.ebi.ac.uk) and modeled via webserver Swiss-Model (https://www.swissmodel.expasy.org). The 3D protein models were generated after transferring conserved atom coordinates as identified by the target-template alignment. To acquire a full-atom protein model, the construction of non-conserved amino acid side chains was performed with OpenStructure computational structural biology framework and ProMod3 modeling engine. Both models with the highest Global Model Quality Estimation (GMQE) and optimal QMEAN scores were submitted to Structural Analysis and Verification Server of UCLA (https://saves.mbi.ucla.edu/), and passed the Verify3D (at least 80% of the amino acids have scored ≥0.2 in the 3D/1D profile) and PROCHECK (Ramachandran plot: 92.4% residues in most favored regions, 6.9% residues in additional allowed regions, 0.3% residues in generously allowed regions and 0.3% residues in disallowed regions) assessments (Laskowski et al., 1993; Eisenberg et al., 1997).
15-mer peptide sequences were generated automatically with an in-house Bash script that extracts 15 continuous amino acid residues from respective α-NTXs. Following that, the 3-dimensional structures of 15-mer were constructed with Pymol (Schrödinger and DeLano, 2020). Open Babel software was used to minimize the energy of the resulting 15-mer peptide structures with a conjugated algorithm for 100 cycles (O'Boyle et al., 2011).
The series of docking analyses were performed via the guru interface of the HADDOCK 2.2 webserver (https://alcazar.science.uu.nl/services/HADDOCK2.2/). The HADDOCK protocol encompasses three docking stages: (i) rigid body minimization (it0), (ii) semi-flexible annealing (it1), and (iii) explicit solvent refinement (water) (van Zundert et al., 2016). The decoys generated were clustered with Fraction of Common Contacts algorithm (FCC: 0.6) (Rodrigues et al., 2012; Takemura and Kitao, 2019). Cluster with the lowest HADDOCK scores of the top 4 models was ranked on top. By default, the HADDOCK scoring function settings are for protein-protein complexes that entail weighted sum of following terms:
where Evdw is the van der Waals energy, Eelec is the electrostatic energy, Edesol is the desolvation energy, Eair restraints (i.e., distance) violation energies, BSA is the buried surface area. More comprehensive HADDOCK protocol and different docking parameter settings were reported in de Vries et al. (2010).
To optimize input parameters of HADDOCK, hemagglutinin (HA) [PDB_ID: 1HXY] crystal structure was selected as the standard ligand (used in all quantitative analysis); endogenous peptide A2 (A2) [PDB_ID: 1AQD] crystal structure was selected as reference ligand (used to establish arbitrary binding threshold for binders classification). Both ligands were docked to both allelic variants of Eqca-DR due to their experimental proven MHCII binding (Stern et al., 1994; Murthy and Stern, 1997). The intermolecular interactions of the resulting Eqca-DR:HA and Eqca-DR:A2 complexes were assessed for hydrogen bonding interactions, via BIOVIA Discovery Studio Visualizer, between the main-chain peptide and conserved HLA-DR1 residues as reported earlier (Stern et al., 1994; Murthy and Stern, 1997). The sampling size across rigid body minimization (It0), semi-flexible annealing (It1) and explicit solvent refinement (water) stages was optimized at 5000:300:300; random exclusion of ambiguous interaction restraints (AIRs) was disabled. The conserved residues of membrane-distal alpha-helices and whole peptides were selected as input for active residues; passive residues of receptor and ligand were defined automatically by HADDOCK. The computed linear combination of various energies and buried surface area of the Eqca-DR:HA and Eqca-DR:A2 complexes in the form of arbitrary HADDOCK scores were recorded.
The energy-minimized 15-mer peptide fragments derived from α-NTXs were respectively docked onto Eqca-DRA*00103:DRB2*00201 and Eqca-DRA*00201:DRB2*00201 as per Section 2.4.1. The resulting Fraction of Common Contacts algorithm (FCC)-clustered decoys were screened and selected for susceptibility to DM-catalyzed peptide editing based on the defined algorithms in the present study:
1) The backbone atoms of P-1, P1, P2 anchor residues of the examined peptides must be hydrogen-bonded to Serα53-Asnβ82 of MHCII molecules.
2) The examined peptide N-terminus must extrude from the P1 pocket.
3) Bidentate hydrogen bonds must be formed between Asn82β and P2 anchor residue of the examined peptides.
4) The combined count of hydrogen bonds and salt bridges formed between membrane distal alpha-helices of MHCII molecules and the examined peptides must be ≥14. This is to enrich the pool of epitopes featuring binding stability and appropriate poses.
The lowest HADDOCK score of respective MHCII-peptide complexes was computed and inverted bars were plotted. The HADDOCK score of binders filtered via the algorithm outlined above was highlighted.
Considering the immune response is elicited based on the binding strength and successful intermolecular interactions of a complex, the immunodominant regions of α-NTXs were estimated by HADDOCK score along with the scoring of amino acid residues of 15-mer α-NTXs peptides from immunogenic binders. The M2R scoring metric, which represents the degree of computed immune response elicited by respective 15-mer peptides in the context of MHCII, was quantified as described in the formulation:
Where “S” is the score of individual residue constituting α-NTXs, “I’” is the total number of amino acid residues constituting α-NTXs, “r” is the frequency of individual amino acid residue that constitutes the filtered 15-mer peptides, “R’” represents the maximum frequency attainable for each residue, “i” is the total number of MHCII-bound 15-mer peptides, “I” is the total number of 15-mer peptides constructed from respective α-NTXs, “h” is the HADDOCK score ratio of respective algorithm-filtered decoys and standard ligand (HA), and “H’” is the maximum attainable HADDOCK score ratio.
The cumulative score of each residue was quantified, accumulated and plotted to quantify M2R which represents the immunogenic spectrum of individual α-NTX.
In the present study, six different lethal elapid α-NTXs (Figure 1) belonging to short-chain and long-chain groups, and a snake venom metalloproteinase [SVMP, a higher molecular weight (MW) toxin] were analyzed for their antigenicity using the B-cell epitope prediction tool (Kolaskar and Tongaonkar, 1990). As shown in Figure 2, the sheer number of predicted antigenic sites of SVMP (MW∼47 kDa, 15 antigenic sites identified) far exceeded that of the six α-NTXs (MW∼7–8 kDa, 1–3 antigenic sites identified), consistent with previous findings of low MW toxins possessing less antigenic peptides (Tan et al., 2019b; Tan and Tan, 2021). The higher number of antigenic peptides in high MW proteins may provide greater exposures to BCRs, thus eliciting a higher antibody titer specific to these antigens, in comparison to the low MW proteins where α-NTXs are classified (MW∼7–8 kDa). Moreover, the presence of high MW proteins in an immunogen mixture containing proteins with varying MW may dampen the antigenicity of low MW α-NTXs (<10 kDa) (Clementi et al., 1991; Irais et al., 2020) in eliciting immune responses amid the antigenic competition for BCRs (Hunt et al., 1995), consequently, the resulting neutralizing antibodies may be skewed toward high MW toxins. This is well exemplified by previous immunoprofiling studies applying microarrays (Engmark et al., 2016) and enzyme-linked immunosorbent assay (Lingam et al., 2020), as well as in vivo neutralization studies that showed viperid antivenoms are significantly more potent than elapid antivenoms (Calvete et al., 2016; Ratanabanangkoon et al., 2016; Bailon Calderon et al., 2020; Faisal et al., 2021). This is likely due to most viperid venoms are predominated with high MW toxins (MW > 25 kDa) (e.g., SVMP and snake venom serine protease, SVSP) (Offor et al., 2022), which are capable of eliciting stronger immune response for the production of neutralizing antibodies. However, given that venoms constituting neurotoxins of more than ∼40% failed to raise antivenoms with major improvement in neutralization efficacy (Tan et al., 2015b; Tan et al., 2016a; Tan et al., 2019a; Palasuberniam et al., 2021), it is therefore suggested that the low neutralizing efficacy of elapid antivenom may be also attributed to other factors, such as amino acid composition, that intrinsically modulate the epitope configuration of α-NTXs to be recognized by T-cell receptor (TCR) to elicit a strong humoral response.
FIGURE 1. Multiple sequence alignment of selected elapids long-chain and short-chain alpha-neurotoxins. Sequences were acquired from the UniProt database. Abbreviations: NAJKA, Naja kaouthia; OPHHA, Ophiophagus hannah; LATCO, Laticauda colubrina; HYDSC, Hydrophis schistosus; HYDHA, Hydrophis curtus. Asterisk symbols indicate the location of disulfide bonds.
FIGURE 2. Prediction of antigenic determinants (B-cell epitope) of α-NTXs with Kolaskar and Tongaonkar antigenicity scale (Kolaskar and Tongaonkar, 1990). Abbreviations: Nk_LαNTX-α-Cbtx, α-cobratoxin (UniProt ID: P01391, N. kaouthia); Oh_LαNTX-OH55, long neurotoxin OH55 (UniProt ID: Q53B58, O. hannah); Hc_LαNTX-1, long neurotoxin 1 (UniProt ID: Q8UW29, H. curtus); Nk_SαNTX-Cbtx-c, cobrotoxin-c (UniProt: P59276, N. kaouthia); Hs_SαNTX-1, short neurotoxin 1 (UniProt ID: P68415, H. schistosus); Lc_SαNTX-2, short neurotoxin 2 (UniProt ID: P10457, L. colubrina); Na_SVMP, snake venom zinc metalloproteinase (Uniprot ID: D3TTC2, Naja atra) (high MW toxin). Yellow areas represent predicted B-cell epitopes with antigenicity scores above the dynamic threshold (∼1.0).
The present study generated homolog models of Eqca-DR due to the absence of equine MHCII alleles binding data in the currently available databases. The immunogenicity of α-NTXs derived from the venoms of selected Asiatic elapids was examined by docking 15-mer peptides of α-NTXs generated to Eqca-DR, to assess for MHCII epitopes. The use of a structure-based approach in the present study is mainly due to the limitations of the existing range of bioinformatics tools (i.e., NetMHC-3.4, PSSM, SMM, TEPITOPE) in the provision of an accurate estimate on MHCII epitopes. Despite the availability of data-driven avenue, most of the data-driven machine-learning mediated predictors of MHC epitopes were trained with MHC-peptidome composed of synthetic sequences (Sidney et al., 2013) that sidestep proteasome cleavage and DM-editing selection. Although this method could narrow down the potential candidate of MHC epitopes, but it may not provide accurate prediction (Robbins et al., 2013; Yadav et al., 2014). Furthermore, although recent development entails the adoption of naturally processed and presented mass spectrometry (MS) derived MHC peptides to hone the accuracy of the existing predictors, the current MS-derived peptidome datasets still do not correlate well with predicted binding affinity (Zhao and Sher, 2018). Factors such as the indirect linear relationship between the kinetic stability of MHCII-peptide complexes and alignment issues due to peptide length varieties may have bugged the algorithm from performing at full capacity in predicting the MHCII binder (Belmares et al., 2002; Nielsen et al., 2010; ten Broeke et al., 2013). Thus, these limitations raise the need for an alternative avenue for immunogenicity study.
Contrary to the data-driven predictor, the structure-based approach does not rely on binding data. Adding to that, it provides visualization of atomic-resolution intermolecular interaction between the MHCII-peptide complex. Peptides are bound to MHCII via hydrogen bonding network between conserved MHCII side chains and peptide backbone and through interactions between MHCII pockets and peptide side-chain anchors. The complementing screening algorithm was customized based on the identification of multiple critical hydrogen bonds between conserved MHCII side-chains and peptide backbone (Figure 3) as a gauge to the susceptibility of DM-catalyzed peptide editing (McFarland et al., 2001; Stratikos et al., 2004; Zhou et al., 2009; Ferrante and Gorski, 2010; Anders et al., 2011; Painter et al., 2011). Following the same principle, MHC-loading enhancers (MLE) target P1 pocket as canonical MLE site to enhance Th cells response, by maintaining the peptide-receptive conformation of MHCII which in turn expedited antigen-loading and ligand exchange on the cell surface (Gupta et al., 2008; Dickhaut et al., 2009). Validation of the MHCII homologs and optimization of docking simulation parameters were conducted by redocking standard (HA) and reference ligands (A2) with two allelic variants of Eqca-DR models (Figure 4). Two homologs examined feature critical intermolecular hydrogen bonding formed between MHCII residues with HA and A2 peptides (with side chains occupying major pockets, hence designated as P-1, P1 and P2 anchor residues), which verified the compatibility of the generated Eqca-DR models and the optimized docking simulation parameters for this study.
FIGURE 3. An atomic resolution of HLA-DR1:HA complex derived from PDB crystal structure (PDB:1HXY) with annotated critical intermolecular interactions between peptide ligand and the side chains of MHCII. Peptide backbone that formed conserved hydrogen bonds with MHCII residues (α53S and β82N) were denoted as P-1, P1 and P2 anchor residues and highlighted in yellow.
FIGURE 4. Molecular interactive illustration showing the interaction of redocked standard ligands with homology models of the two allelic Eqca-DR. (A) hemagglutinin (HA) and (B) endogenous peptide A2 (A2). 2D chemical structure represents the ligand surrounded by interactive MHCII binding groove residues. P-1, P1 and P2 anchor residues of HA and A2 and their hydrogen bonding interaction with residues of the binding grooves were circled in red. Two allelic Eqca-DR models examined were Eqca-DRA*00103:DRB2*00101 and Eqca-DRA*00201:DRB2*00101.
Herein, structure-based MHCII epitopes predictor augmented with DM-editing determinant algorithm, mimicry of humoral response, was adopted to assess the immunogenicity of α-NTXs derived from venoms of selected terrestrial, submarine and marine Asiatic elapids (Figure 1). The use of α-NTXs selected from cross-genus elapids was to validate the flexibility of the structural approach in determining the immunogenicity of α-NTXs, as well as for comparison to the previously reported data from neutralization studies (Tan et al., 2016b; Tan et al., 2018).
The arbitrary binding affinity threshold is commonly used as a gauge for the prediction of MHCII epitope ligands (Sette et al., 1994; Southwood et al., 1998). Table 1 showed the HADDOCK score of decoys of two allelic Eqca-DR models (Eqca-DRA*00103:DRB2*00201 and Eqca-DRA*00201:DRB2*00201) redocked with HA and A2 ligands that provide inference for a strong binder (Stern et al., 1994; Murthy and Stern, 1997). Applying the same approach, the inverted stacked HADDOCK scores of decoys of 15-mer peptides with respective allelic variants of Eqca-DR were shown in Figure 5. As delineated by Table 2, the 15-mer peptides were further benchmarked arbitrarily as strong (≤−230), moderate (−230 to −180) and weak binders (≥−180) based upon the HADDOCK score of HA and A2 ligand bindings (Table 1).
TABLE 1. HADDOCK scores of redocking of two allelic variants of Eqca-DR MHCII models with standard and reference ligand.
FIGURE 5. Accumulative inverted HADDOCK scores of 15-mer peptides binding decoys (Eqca-DR:15-mer) derived from docking of Eqca-DRA MHCII models to 15-mer peptides generated from α-neurotoxins. (A) Long-chain alpha-neurotoxins (LαNTX), and (B) Short-chain alpha-neurotoxins (SαNTX). Blue bars indicate Eqca-DRA*00103:DRB2*00201; and orange bars indicate Eqca-DRA*00201:DRB2*00201. Bars with dark blue/dark brown indicate the decoys (Eqca:15-mer) that fulfilled the defined algorithm in the present study. Abbreviations: Nk_LαNTX-α-Cbtx, α-cobratoxin (UniProt ID: P01391, N. kaouthia); Oh_LαNTX-OH55, long neurotoxin OH55 (UniProt ID: Q53B58, O. hannah); Hc_LαNTX-1, long neurotoxin 1 (UniProt ID: Q8UW29, H. curtus); Nk_SαNTX-Cbtx-c, cobrotoxin-c (UniProt: P59276, N. kaouthia); Hs_SαNTX-1, short neurotoxin 1 (UniProt ID: P68415, H. schistosus); and Lc_SαNTX-2, short neurotoxin 2 (UniProt ID: P10457, L. colubrina).
The present study showed none of these 15-mer peptides generated from all α-NTXs were considered as “strong binder.” Out of the 57–58 15-mer peptides generated from the three LαNTXs, there were only 3–12 that were considered as “moderate binders” while the remaining were “weak binders.” Similarly, the SαNTXs that are shorter in sequence length also showed no “strong binders” among the 46–48 15-mer peptides. At this point, the pool of low affinity 15-mer binding MHCII decoys already foreshadows weak immunogenicity, because it is suggested that the peptide that binds with a long half-life has the propensity to dominate the Th cell response (Pos et al., 2013). Although previous studies suggested that MHCII binders with higher affinity may not be translated to MHCII binding in vivo (Zhao and Sher, 2018), this may be a result of excluding the importance of peptide-editing role of DM molecules in the prediction or study performed (Belmares et al., 2002).
With the DM-editing determinant screening algorithm used in this study, the filtered 15-mer peptides were selected as predicted MHCII epitopes (Table 2). In comparison, LαNTXs generally feature a higher percentage of predicted MHCII binders (24%–38%) than SαNTXs (12%–34%) (Table 2). Of the LαNTXs derived 15-mer peptides, only 14–22 were predicted as MHCII epitopes, with the lowest binder proportion shown in Nk_LαNTX-α-Cbtx (24%–26%), followed by Oh_LαNTX-OH55 (∼34–36%), and Hc_LαNTX-1 (31%–38%). For SαNTXs, the MHCII binder proportion was ranging from 12% to 34%, with the lowest shown in Lc_SαNTX-2 (∼12%), followed by Hs_SαNTX-1 (26%–28%), and Nk_SαNTX-Cbtx-c (30%–34%). Unsurprisingly, most predicted MHCII binders were on the “weak binder” spectrum, with only a few classified as “moderate binders.”
Binding affinity relative to algorithm-identified binders is suggested as a vital indicator that represents the degree of posing fit which indirectly reflects the importance of amino acid composition, and consequently dictates the dynamic conformation of MHCII-peptide that is least susceptible to DM-editing mechanism (Pos et al., 2013; Yin et al., 2014). Also, it relays information on the possible disposition of P9 leucine-binder in sidestepping the hydrogen bonding and pocket interaction at P1 (Yin et al., 2014), which may be a perk of strong MHCII binder (i.e., endogenous peptide A2). Ergo, it is suggested that the low amount of MHCII epitopes and their relatively weak affinity may compromise the chances of α-NTXs to be processed for MHCII display by APCs that non-specifically uptake and process antigens for the activation of Th cell in the context of humoral immunity (Hilhorst et al., 2014).
Compositional analysis of α-NTXs was performed by profiling the amino acid residues of predicted MHCII binders derived from respective α-NTXs occupying P-1, P1 and P2 binding pockets of Eqca-MHCII. The known major anchor sites are P1, P4, P6 and P9 as evidenced by their substantial buried side chain solvent-exposed surface area (Murthy and Stern, 1997). However, we specifically look into the P1 pocket as the residue preference in this position plays a major role in dictating DM-catalyzed peptide editing susceptibility relative to other major pockets (Painter et al., 2011).
An earlier study showed tryptophan, tyrosine, phenylalanine and leucine that feature hydrophobic side chains are optimal P1 anchor residues (Yin et al., 2014). As depicted in Figure 6, only 16 out of 177 (<10%) identified predicted MHCII binders feature optimal P1 anchor amino acid residues mentioned above. These include Nk_SαNTX-Cbtx-c (8 binders), Hs_SαNTX-1 (5 binders), and Hc_LαNTX-1 (3 binders), and worth noting that all 16 binders consistently possess leucine as anchor residue. The lack of optimal P1 anchor residues may have culminated in binders with weak affinity to Eqca-DR which coincides with the predominant of weak MHCII binders as displayed in Table 2. Thus, this finding further validates that the amino acid composition of α-NTXs could be one of the major limiting factors in eliciting a strong humoral response, during the immunization process of antivenom production.
FIGURE 6. Anchor residue preferences of P1 pocket. Violet box: Positive side chain; Orange box: Negative side chain; Yellow box: Polar side chain; Blue box: Special cases; Green box: Non-polar side chain; Red square: Optimal P1 anchor residues. Abbreviations: Nk_LαNTX-α-Cbtx, α-cobratoxin (UniProt ID: P01391, N. kaouthia); Oh_LαNTX-OH55, long neurotoxin OH55 (UniProt ID: Q53B58, O. hannah); Hc_LαNTX-1, long neurotoxin 1 (UniProt ID: Q8UW29, H. curtus); Nk_SαNTX-Cbtx-c, cobrotoxin-c (UniProt: P59276, N. kaouthia); Hs_SαNTX-1, short neurotoxin 1 (UniProt ID: P68415, H. schistosus); and Lc_SαNTX-2, short neurotoxin 2 (UniProt ID: P10457, L. colubrina).
For ease of interpretation, scoring metric that reflects both binding affinity and proportion of predicted MHCII binders are vital for multilayered analysis. Based on the predicted MHCII epitopes, each amino acid composing α-NTXs was scored and cumulatively quantified as M2R to enable elucidation of immunodominance, in the context of Th cell activation, across the entire sequence of α-NTXs (Figure 7; Supplementary File S1).
FIGURE 7. The immunodominant coverage across the amino acid sequences of α-neurotoxins. (A) Long-chain alpha-neurotoxins (LαNTX), and (B) Short-chain alpha-neurotoxins (SαNTX). Dashed squares in the figure represent the different loops and C-terminus tail of α-NTXs. The brown-dash dotted line indicates the maximum score of respective amino acid residues. Abbreviations: Nk_LαNTX-α-Cbtx, α-cobratoxin (UniProt ID: P01391, N. kaouthia); Oh_LαNTX-OH55, long neurotoxin OH55 (UniProt ID: Q53B58, O. hannah); Hc_LαNTX-1, long neurotoxin 1 (UniProt ID: Q8UW29, H. curtus); Nk_SαNTX-Cbtx-c, cobrotoxin-c (UniProt: P59276, N. kaouthia); Hs_SαNTX-1, short neurotoxin 1 (UniProt ID: P68415, H. schistosus); and Lc_SαNTX-2, short neurotoxin 2 (UniProt ID: P10457, L. colubrina). The details analyses were provided in Supplementary File S1.
In general, the immunodominant amino acid sequences were noted to be skewed differently for all three LαNTXs from different species, where Nk_LαNTX-α-Cbtx and Oh_LαNTX-OH55 were shown to be more immunogenic at loop II, and to a lesser extent for Hc_LαNTX-1 that is more loop III dominant. All three α-NTXs feature minute responsiveness at loop I as indicated by their extremely low M2R score. On the contrary, Nk_SαNTX-Cbtx-c, Hs_SαNTX-1 and Lc_SαNTX-2 are responsive across the three loops, except that Lc_SαNTX-2 featured a negligible response for loop I. Overall, all α-NTXs were predicted to be relatively responsive on loop II, which corroborates with a previous study that employed a data-driven MHCII epitope predictor utilizing human and mouse MHCII alleles binding data in predicting the immunogenicity of snake 3FTXs (Pruksaphon et al., 2022).
The general trend of M2R scores for respective α-NTXs is shown to be less than 0.3 (Figure 8) while all predicted epitopes feature multiplier HADDOCK score ratio (h) ≤1 (Maximum M2R = 1), suggesting α-NTXs are generally poor immunogens. The result is paralleled with the algorithm clustering of MHCII binders that showed the predomination of weak MHCII binders (Table 2). The accumulated overall M2R scores for each α-NTX were also estimated and shown descending from Oh_LαNTX-OH55(0.28) > Hc_LαNTX-1(0.27) > Nk_SαNTX-Cbtx-c(0.25) > Hs_SαNTX-1(0.22) > Nk_LαNTX-α-Cbtx(0.2) > Lc_SαNTX-2(0.11) (Figure 8).
FIGURE 8. M2R scoring of α-neurotoxins. Abbreviations: Nk_LαNTX-α-Cbtx, α-cobratoxin (UniProt ID: P01391, N. kaouthia); Oh_LαNTX-OH55, long neurotoxin OH55 (UniProt ID: Q53B58, O. hannah); Hc_LαNTX-1, long neurotoxin 1 (UniProt ID: Q8UW29, H. curtus); Nk_SαNTX-Cbtx-c, cobrotoxin-c (UniProt: P59276, N. kaouthia); Hs_SαNTX-1, short neurotoxin 1 (UniProt ID: P68415, H. schistosus); and Lc_SαNTX-2, short neurotoxin 2 (UniProt ID: P10457, L. colubrina). The details analyses were provided in Supplementary File S1.
For comparative purposes, Table 3 illustrates the correlation study of reported commercial antivenoms on five selected α-NTXs examined in the present study. The p-score, defined as the relative potency of commercial antivenom for respective α-NTXs, is highly correlated (coefficient of determination, R2 = 0.82) with M2R scoring in the present study (Figure 9). The p-score trend showed as: Oh_LαNTX-OH55(0.62) > Hc_LαNTX-1(0.53) > Nk_SαNTX-Cbtx-c(0.29) > Nk_LαNTX-α-Cbtx(0.16) > Hs_SαNTX-1(0.03). Except for the slight swapping order between Nk_LαNTX-α-Cbtx and Hs_SαNTX-1, the p-score generated is consistent with the M2R score in showing Hc_LαNTX-1 and Nk_SαNTX-Cbtx-c are more immunogenic compared to their homologous Hs_SαNTX-1 and Nk_LαNTX-α-Cbtx, respectively. The finding is however inconsistent with the earlier postulation where antigenicity and immunogenicity of LαNTXs are generally greater than SαNTXs, attributed to the slightly greater molecular size (Tan et al., 2016b; Wong et al., 2016; Tan et al., 2017). The present study showed a mixed observation that both LαNTXs and SαNTXs are generally low in antigenicity and immunogenicity, without clear distinction.
TABLE 3. Correlation of neurotoxin abundance and neutralization efficacy of its homologous antivenom.
On the other hand, the p-score shown in Table 3 also highlights the disproportionate link between the relative abundance of α-NTXs and the neutralization efficacy of antivenoms. The poor neutralization potency of antivenom against α-NTXs could be multifactorial (e.g., molecular size, relative abundance, amino acid composition, and peptide binding recognition by MHCII), in particular the amino acid composition as highlighted in the present study, contributing to the inadequate immunogenic capacity of α-NTXs in eliciting a strong humoral response during the immunization in an animal host. Furthermore, the antigenicity could have been impacted by its small size when competing for B-cell receptors in the context of a heterogenous immunogen mixture (Hunt et al., 1995).
To date, a number of innovative strategies for new antivenom production have been reported, such as the use of synthetic peptide antigen (de la Rosa et al., 2018), multiepitopes string (Ramos et al., 2016) and virus-displayed epitopes (Menzies et al., 2022) as immunogens. These strategies selected the toxins mainly based on targeted snake species and showed that it is possible to produce elapid antivenoms by replacing crude venom immunogens. In the future exploration of antivenom production, the immunoinformatic approach developed in this study can be applied to further strengthen the design of synthetic epitopes, thereby increasing the neutralization efficacy of antivenoms, especially in targeting the alpha-neurotoxins of elapid species.
In the present study, antigenicity tool and structure-based MHCII epitope predictor augmented with DM-editing determinant screening algorithm, collectively allude α-NTXs as poor antigen and immunogen. This is further supported by the relative immunogenicity of α-NTXs derived from the computed prediction that correlates strongly to the empirical data of in vivo neutralization efficacy of commercial antivenoms. Adding to that, most of the predicted binders feature non-optimal P1 anchor residues that greatly impede the formation of the MHCII-peptide complex with stronger affinity and stability. Overall, the outcome of this study suggests that both molecular size and amino acid composition are the main factors limiting the epitope configuration of α-NTXs as recognized by B-cell receptor (BCR) and T-cell receptor (TCR), resulting in a weaker humoral response in the animal host during immunization that applies a whole venom immunogen. The finding of the study provides insight into the low efficacy of elapid antivenoms against most elapid venoms which contain α-NTX as the principal toxins. Furthermore, DM-editing determinants could be incorporated as feature descriptors to the existing machine-learning-based predictors to enhance their accuracy in predicting MHCII epitopes while enabling the elucidation of immunodominant coverage of α-NTXs. This application has potential utility in the design and production of synthetic epitope immunogens for developing a better antivenom by applying various innovation strategies (Ramos et al., 2016; de la Rosa et al., 2018; Menzies et al., 2022).
The original contributions presented in the study are included in the article/Supplementary Material, further inquiries can be directed to the corresponding author.
Conceptualization, KYT and CHT; methodology, KYT, CHH, and CHT; validation, KYT, CHH, and CHT; formal analysis, YWC; investigation, YWC; resources, KYT and CHT; data curation, KYT, CHH, and CHT; writing—original draft preparation, YWC; writing—review and editing, YWC, KYT, CHH, and CHT; visualization, YWC, KYT, CHH, and CHT; supervision, KYT, CHH, and CHT; funding acquisition, KYT. All authors have read and agreed to the published version of the manuscript.
This work was supported by the Fundamental Research Grant (FRGS/1/2019/SKK08/UM/02/2) from the Ministry of Higher Education, Malaysia.
The authors declare that the research was conducted in the absence of any commercial or financial relationships that could be construed as a potential conflict of interest.
All claims expressed in this article are solely those of the authors and do not necessarily represent those of their affiliated organizations, or those of the publisher, the editors and the reviewers. Any product that may be evaluated in this article, or claim that may be made by its manufacturer, is not guaranteed or endorsed by the publisher.
The Supplementary Material for this article can be found online at: https://www.frontiersin.org/articles/10.3389/fphar.2023.1143437/full#supplementary-material
Anders, A. K., Call, M. J., Schulze, M. S., Fowler, K. D., Schubert, D. A., Seth, N. P., et al. (2011). HLA-DM captures partially empty HLA-DR molecules for catalyzed removal of peptide. Nat. Immunol. 12, 54–61. doi:10.1038/ni.1967
Bailon Calderon, H., Yaniro Coronel, V. O., Caceres Rey, O. A., Colque Alave, E. G., Leiva Duran, W. J., Padilla Rojas, C., et al. (2020). Development of nanobodies against hemorrhagic and myotoxic components of Bothrops atrox snake venom. Front. Immunol. 11, 655. doi:10.3389/fimmu.2020.00655
Barber, C. M., Isbister, G. K., and Hodgson, W. C. (2013). Alpha neurotoxins. Toxicon 66, 47–58. doi:10.1016/j.toxicon.2013.01.019
Belmares, M. P., Busch, R., Wucherpfennig, K. W., Mcconnell, H. M., and Mellins, E. D. (2002). Structural factors contributing to DM susceptibility of MHC class II/peptide complexes. J. Immunol. 169, 5109–5117. doi:10.4049/jimmunol.169.9.5109
Calvete, J. J., Arias, A. S., Rodriguez, Y., Quesada-Bernat, S., Sanchez, L. V., Chippaux, J. P., et al. (2016). Preclinical evaluation of three polyspecific antivenoms against the venom of Echis ocellatus: Neutralization of toxic activities and antivenomics. Toxicon 119, 280–288. doi:10.1016/j.toxicon.2016.06.022
Clementi, M., Marini, S., Condo, S., and Giardina, B. (1991). Antibodies against small molecules. Ann. dell'Istituto Super. sanità 27, 139–143.
De La Rosa, G., Corrales-García, L. L., Rodriguez-Ruiz, X., López-Vera, E., and Corzo, G. (2018). Short-chain consensus alpha-neurotoxin: A synthetic 60-mer peptide with generic traits and enhanced immunogenic properties. Amino Acids 50, 885–895. doi:10.1007/s00726-018-2556-0
De Vries, S. J., Van Dijk, M., and Bonvin, A. M. (2010). The HADDOCK web server for data-driven biomolecular docking. Nat. Protoc. 5, 883–897. doi:10.1038/nprot.2010.32
Denzin, L. K., and Cresswell, P. (1995). HLA-DM induces CLIP dissociation from MHC class II alpha beta dimers and facilitates peptide loading. Cell 82, 155–165. doi:10.1016/0092-8674(95)90061-6
Dickhaut, K., Hoepner, S., Eckhard, J., Wiesmueller, K. H., Schindler, L., Jung, G., et al. (2009). Enhancement of tumour-specific immune responses in vivo by 'MHC loading-enhancer' (MLE). PLoS One 4, e6811. doi:10.1371/journal.pone.0006811
Eisenberg, D., Luthy, R., and Bowie, J. U. (1997). VERIFY3D: Assessment of protein models with three-dimensional profiles. Methods Enzymol. 277, 396–404. doi:10.1016/s0076-6879(97)77022-8
Engmark, M., Andersen, M. R., Laustsen, A. H., Patel, J., Sullivan, E., De Masi, F., et al. (2016). High-throughput immuno-profiling of mamba (Dendroaspis) venom toxin epitopes using high-density peptide microarrays. Sci. Rep. 6, 36629. doi:10.1038/srep36629
Faisal, T., Tan, K. Y., Tan, N. H., Sim, S. M., Gnanathasan, C. A., and Tan, C. H. (2021). Proteomics, toxicity and antivenom neutralization of Sri Lankan and Indian Russell's viper (Daboia russelii) venoms. J. Venom. Anim. Toxins Incl. Trop. Dis. 27, e20200177. doi:10.1590/1678-9199-JVATITD-2020-0177
Ferrante, A., and Gorski, J. (2010). Cutting edge: HLA-DM-mediated peptide exchange functions normally on MHC class II-peptide complexes that have been weakened by elimination of a conserved hydrogen bond. J. Immunol. 184, 1153–1158. doi:10.4049/jimmunol.0902878
Fung-Leung, W. P., Surh, C. D., Liljedahl, M., Pang, J., Leturcq, D., Peterson, P. A., et al. (1996). Antigen presentation and T cell development in H2-M-deficient mice. Science 271, 1278–1281. doi:10.1126/science.271.5253.1278
Gupta, S., Hopner, S., Rupp, B., Gunther, S., Dickhaut, K., Agarwal, N., et al. (2008). Anchor side chains of short peptide fragments trigger ligand-exchange of class II MHC molecules. PLoS One 3, e1814. doi:10.1371/journal.pone.0001814
Hilhorst, M., Shirai, T., Berry, G., Goronzy, J. J., and Weyand, C. M. (2014). T cell-macrophage interactions and granuloma formation in vasculitis. Front. Immunol. 5, 432. doi:10.3389/fimmu.2014.00432
Hoogeboom, R., and Tolar, P. (2016). Molecular mechanisms of B cell antigen gathering and endocytosis. Curr. Top. Microbiol. Immunol. 393, 45–63. doi:10.1007/82_2015_476
Hunt, J. D., Jackson, D. C., Wood, P. R., Stewart, D. J., and Brown, L. E. (1995). Immunological parameters associated with antigenic competition in a multivalent footrot vaccine. Vaccine 13, 1649–1657. doi:10.1016/0264-410x(95)00145-q
Irais, C. M., Maria-De-La-Luz, S. G., Dealmy, D. G., Agustina, R. M., Nidia, C. H., Mario-Alberto, R. G., et al. (2020). Plant phenolics as pathogen-carrier immunogenicity modulator haptens. Curr. Pharm. Biotechnol. 21, 897–905. doi:10.2174/1389201021666200121130313
Kolaskar, A. S., and Tongaonkar, P. C. (1990). A semi-empirical method for prediction of antigenic determinants on protein antigens. FEBS Lett. 276, 172–174. doi:10.1016/0014-5793(90)80535-q
Laskowski, R. A., Macarthur, M. W., Moss, D. S., and Thornton, J. M. (1993). Procheck: A program to check the stereochemical quality of protein structures. J. Appl. Crystallogr. 26, 283–291. doi:10.1107/s0021889892009944
Leong, P. K., Fung, S. Y., Tan, C. H., Sim, S. M., and Tan, N. H. (2015). Immunological cross-reactivity and neutralization of the principal toxins of Naja sumatrana and related cobra venoms by a Thai polyvalent antivenom (Neuro Polyvalent Snake Antivenom). Acta Trop. 149, 86–93. doi:10.1016/j.actatropica.2015.05.020
Leong, P. K., Sim, S. M., Fung, S. Y., Sumana, K., Sitprija, V., and Tan, N. H. (2012). Cross neutralization of Afro-Asian cobra and Asian krait venoms by a Thai polyvalent snake antivenom (Neuro Polyvalent Snake Antivenom). PLoS Negl. Trop. Dis. 6, e1672. doi:10.1371/journal.pntd.0001672
Leong, P. K., Tan, C. H., Sim, S. M., Fung, S. Y., Sumana, K., Sitprija, V., et al. (2014). Cross neutralization of common Southeast Asian viperid venoms by a Thai polyvalent snake antivenom (Hemato Polyvalent Snake Antivenom). Acta Trop. 132, 7–14. doi:10.1016/j.actatropica.2013.12.015
Lingam, T. M. C., Tan, K. Y., and Tan, C. H. (2020). Proteomics and antivenom immunoprofiling of Russell's viper (Daboia siamensis) venoms from Thailand and Indonesia. J. Venom. Anim. Toxins Incl. Trop. Dis. 26, e20190048. doi:10.1590/1678-9199-JVATITD-2019-0048
Mcfarland, B. J., Katz, J. F., Beeson, C., and Sant, A. J. (2001). Energetic asymmetry among hydrogen bonds in MHC class II*peptide complexes. Proc. Natl. Acad. Sci. U. S. A. 98, 9231–9236. doi:10.1073/pnas.151131498
Menzies, S. K., Dawson, C. A., Crittenden, E., Edge, R. J., Hall, S. R., Alsolaiss, J., et al. (2022). Virus-like particles displaying conserved toxin epitopes stimulate polyspecific, murine antibody responses capable of snake venom recognition. Sci. Rep. 12, 11328. doi:10.1038/s41598-022-13376-x
Morris, P., Shaman, J., Attaya, M., Amaya, M., Goodman, S., Bergman, C., et al. (1994). An essential role for HLA-DM in antigen presentation by class II major histocompatibility molecules. Nature 368, 551–554. doi:10.1038/368551a0
Murthy, V. L., and Stern, L. J. (1997). The class II MHC protein HLA-DR1 in complex with an endogenous peptide: Implications for the structural basis of the specificity of peptide binding. Structure 5, 1385–1396. doi:10.1016/s0969-2126(97)00288-8
Nielsen, M., Lund, O., Buus, S., and Lundegaard, C. (2010). MHC class II epitope predictive algorithms. Immunology 130, 319–328. doi:10.1111/j.1365-2567.2010.03268.x
O'boyle, N. M., Banck, M., James, C. A., Morley, C., Vandermeersch, T., and Hutchison, G. R. (2011). Open Babel: An open chemical toolbox. J. Cheminform 3, 33. doi:10.1186/1758-2946-3-33
Offor, B. C., Muller, B., and Piater, L. A. (2022). A review of the proteomic profiling of african viperidae and elapidae snake venoms and their antivenom neutralisation. Toxins 14, 723. doi:10.3390/toxins14110723
Oh, A. M. F., Tan, K. Y., Tan, N. H., and Tan, C. H. (2021). Proteomics and neutralization of Bungarus multicinctus (Many-banded Krait) venom: Intra-specific comparisons between specimens from China and Taiwan. Comp. Biochem. Physiology Part C Toxicol. Pharmacol. 247, 109063. doi:10.1016/j.cbpc.2021.109063
Painter, C. A., Negroni, M. P., Kellersberger, K. A., Zavala-Ruiz, Z., Evans, J. E., and Stern, L. J. (2011). Conformational lability in the class II MHC 310 helix and adjacent extended strand dictate HLA-DM susceptibility and peptide exchange. Proc. Natl. Acad. Sci. U. S. A. 108, 19329–19334. doi:10.1073/pnas.1108074108
Palasuberniam, P., Chan, Y. W., Tan, K. Y., and Tan, C. H. (2021). Snake venom proteomics of samar cobra (Naja samarensis) from the southern Philippines: Short alpha-neurotoxins as the dominant lethal component weakly cross-neutralized by the philippine cobra antivenom. Front. Pharmacol. 12, 727756. doi:10.3389/fphar.2021.727756
Patikorn, C., Blessmann, J., Nwe, M. T., Tiglao, P. J. G., Vasaruchapong, T., Maharani, T., et al. (2022). Estimating economic and disease burden of snakebite in ASEAN countries using a decision analytic model. PLoS neglected Trop. Dis. 16, e0010775. doi:10.1371/journal.pntd.0010775
Pos, W., Sethi, D. K., and Wucherpfennig, K. W. (2013). Mechanisms of peptide repertoire selection by HLA-DM. Trends Immunol. 34, 495–501. doi:10.1016/j.it.2013.06.002
Pruksaphon, K., Yuvaniyama, J., and Ratanabanangkoon, K. (2022). Immunogenicity of snake alpha-neurotoxins and the CD4 T cell epitopes. Toxicon 214, 136–144. doi:10.1016/j.toxicon.2022.05.009
Ramos, H. R., Junqueira-De-Azevedo Ide, L., Novo, J. B., Castro, K., Duarte, C. G., Machado-De-Avila, R. A., et al. (2016). A heterologous multiepitope DNA prime/recombinant protein boost immunisation strategy for the development of an antiserum against Micrurus corallinus (coral snake) venom. PLoS Negl. Trop. Dis. 10, e0004484. doi:10.1371/journal.pntd.0004484
Ranawaka, U. K., Lalloo, D. G., and De Silva, H. J. (2013). Neurotoxicity in snakebite--the limits of our knowledge. PLoS Negl. Trop. Dis. 7, e2302. doi:10.1371/journal.pntd.0002302
Ratanabanangkoon, K. (2021). A quest for a universal plasma-derived antivenom against all elapid neurotoxic snake venoms. Front. Immunol. 12, 668328. doi:10.3389/fimmu.2021.668328
Ratanabanangkoon, K., Tan, K. Y., Eursakun, S., Tan, C. H., Simsiriwong, P., Pamornsakda, T., et al. (2016). A simple and novel strategy for the production of a pan-specific antiserum against elapid snakes of asia. PLoS Negl. Trop. Dis. 10, e0004565. doi:10.1371/journal.pntd.0004565
Ratanabanangkoon, K., Tan, K. Y., Pruksaphon, K., Klinpayom, C., Gutierrez, J. M., Quraishi, N. H., et al. (2020). A pan-specific antiserum produced by a novel immunization strategy shows a high spectrum of neutralization against neurotoxic snake venoms. Sci. Rep. 10, 11261. doi:10.1038/s41598-020-66657-8
Robbins, P. F., Lu, Y. C., El-Gamil, M., Li, Y. F., Gross, C., Gartner, J., et al. (2013). Mining exomic sequencing data to identify mutated antigens recognized by adoptively transferred tumor-reactive T cells. Nat. Med. 19, 747–752. doi:10.1038/nm.3161
Rodrigues, J. P., Trellet, M., Schmitz, C., Kastritis, P., Karaca, E., Melquiond, A. S., et al. (2012). Clustering biomolecular complexes by residue contacts similarity. Proteins 80, 1810–1817. doi:10.1002/prot.24078
Schulze, M. S., Anders, A. K., Sethi, D. K., and Call, M. J. (2013). Disruption of hydrogen bonds between major histocompatibility complex class II and the peptide N-terminus is not sufficient to form a human leukocyte antigen-DM receptive state of major histocompatibility complex class II. PLoS One 8, e69228. doi:10.1371/journal.pone.0069228
Sette, A., Vitiello, A., Reherman, B., Fowler, P., Nayersina, R., Kast, W. M., et al. (1994). The relationship between class I binding affinity and immunogenicity of potential cytotoxic T cell epitopes. J. Immunol. 153, 5586–5592. doi:10.4049/jimmunol.153.12.5586
Sidney, J., Southwood, S., Moore, C., Oseroff, C., Pinilla, C., Grey, H. M., et al. (2013). Measurement of MHC/peptide interactions by gel filtration or monoclonal antibody capture. Curr. Protoc. Immunol. 18, doi:10.1002/0471142735.im1803s100
Southwood, S., Sidney, J., Kondo, A., Del Guercio, M. F., Appella, E., Hoffman, S., et al. (1998). Several common HLA-DR types share largely overlapping peptide binding repertoires. J. Immunol. 160, 3363–3373. doi:10.4049/jimmunol.160.7.3363
Stern, L. J., Brown, J. H., Jardetzky, T. S., Gorga, J. C., Urban, R. G., Strominger, J. L., et al. (1994). Crystal structure of the human class II MHC protein HLA-DR1 complexed with an influenza virus peptide. Nature 368, 215–221. doi:10.1038/368215a0
Stratikos, E., Wiley, D. C., and Stern, L. J. (2004). Enhanced catalytic action of HLA-DM on the exchange of peptides lacking backbone hydrogen bonds between their N-terminal region and the MHC class II alpha-chain. J. Immunol. 172, 1109–1117. doi:10.4049/jimmunol.172.2.1109
Takemura, K., and Kitao, A. (2019). More efficient screening of protein-protein complex model structures for reducing the number of candidates. Biophys. Physicobiol 16, 295–303. doi:10.2142/biophysico.16.0_295
Tan, C. H., Palasuberniam, P., Blanco, F. B., and Tan, K. Y. (2021). Immunoreactivity and neutralization capacity of Philippine cobra antivenom against Naja philippinensis and Naja samarensis venoms. Trans. R. Soc. Trop. Med. Hyg. 115, 78–84. doi:10.1093/trstmh/traa087
Tan, C. H., and Tan, K. Y. (2021). De novo venom-gland transcriptomics of spine-bellied sea snake (Hydrophis curtus) from penang, Malaysia—Next-generation sequencing, functional annotation and toxinological correlation. Toxins 13, 127. doi:10.3390/toxins13020127
Tan, C. H., Tan, K. Y., Fung, S. Y., and Tan, N. H. (2015a). Venom-gland transcriptome and venom proteome of the Malaysian king cobra (Ophiophagus hannah). BMC Genomics 16, 687. doi:10.1186/s12864-015-1828-2
Tan, C. H., Tan, K. Y., Lim, S. E., and Tan, N. H. (2015b). Venomics of the beaked sea snake, Hydrophis schistosus: A minimalist toxin arsenal and its cross-neutralization by heterologous antivenoms. J. Proteomics 126, 121–130. doi:10.1016/j.jprot.2015.05.035
Tan, C. H., Tan, K. Y., Ng, T. S., Sim, S. M., and Tan, N. H. (2018). Venom proteome of spine-bellied sea snake (Hydrophis curtus) from penang, Malaysia: Toxicity correlation, immunoprofiling and cross-neutralization by sea snake antivenom. Toxins 11, 3. doi:10.3390/toxins11010003
Tan, C. H., Tan, K. Y., and Tan, N. H. (2016a). Revisiting Notechis scutatus venom: On shotgun proteomics and neutralization by the "bivalent" sea snake antivenom. J. Proteomics 144, 33–38. doi:10.1016/j.jprot.2016.06.004
Tan, C. H., Wong, K. Y., Chong, H. P., Tan, N. H., and Tan, K. Y. (2019a). Proteomic insights into short neurotoxin-driven, highly neurotoxic venom of Philippine cobra (Naja philippinensis) and toxicity correlation of cobra envenomation in Asia. J. Proteomics 206, 103418. doi:10.1016/j.jprot.2019.103418
Tan, K. Y., Liew, J. L., Tan, N. H., Quah, E. S., Ismail, A. K., and Tan, C. H. (2019b). Unlocking the secrets of banded coral snake (Calliophis intestinalis, Malaysia): A venom with proteome novelty, low toxicity and distinct antigenicity. J. proteomics 192, 246–257. doi:10.1016/j.jprot.2018.09.006
Tan, K. Y., Tan, C. H., Fung, S. Y., and Tan, N. H. (2016b). Neutralization of the principal toxins from the venoms of Thai Naja kaouthia and Malaysian Hydrophis schistosus: Insights into toxin-specific neutralization by two different antivenoms. Toxins (Basel) 8, 86. doi:10.3390/toxins8040086
Tan, K. Y., Tan, C. H., Fung, S. Y., and Tan, N. H. (2015c). Venomics, lethality and neutralization of Naja kaouthia (monocled cobra) venoms from three different geographical regions of Southeast Asia. J. Proteomics 120, 105–125. doi:10.1016/j.jprot.2015.02.012
Tan, N. H., Wong, K. Y., and Tan, C. H. (2017). Venomics of Naja sputatrix, the javan spitting cobra: A short neurotoxin-driven venom needing improved antivenom neutralization. J. proteomics 157, 18–32. doi:10.1016/j.jprot.2017.01.018
Ten Broeke, T., Wubbolts, R., and Stoorvogel, W. (2013). MHC class II antigen presentation by dendritic cells regulated through endosomal sorting. Cold Spring Harb. Perspect. Biol. 5, a016873. doi:10.1101/cshperspect.a016873
Van Zundert, G. C. P., Rodrigues, J., Trellet, M., Schmitz, C., Kastritis, P. L., Karaca, E., et al. (2016). The HADDOCK2.2 web server: User-friendly integrative modeling of biomolecular complexes. J. Mol. Biol. 428, 720–725. doi:10.1016/j.jmb.2015.09.014
Wong, K. Y., Tan, C. H., and Tan, N. H. (2016). Venom and purified toxins of the spectacled cobra (Naja naja) from Pakistan: Insights into toxicity and antivenom neutralization. Am. J. Trop. Med. Hyg. 94, 1392–1399. doi:10.4269/ajtmh.15-0871
World Health Organization (2016). Guidelines for the management of snakebites. New Delhi: World Health Organization, Regional Office for South-East Asia.
Yadav, M., Jhunjhunwala, S., Phung, Q. T., Lupardus, P., Tanguay, J., Bumbaca, S., et al. (2014). Predicting immunogenic tumour mutations by combining mass spectrometry and exome sequencing. Nature 515, 572–576. doi:10.1038/nature14001
Yin, L., Trenh, P., Guce, A., Wieczorek, M., Lange, S., Sticht, J., et al. (2014). Susceptibility to HLA-DM protein is determined by a dynamic conformation of major histocompatibility complex class II molecule bound with peptide. J. Biol. Chem. 289, 23449–23464. doi:10.1074/jbc.M114.585539
Zhao, W., and Sher, X. (2018). Systematically benchmarking peptide-MHC binding predictors: From synthetic to naturally processed epitopes. PLoS Comput. Biol. 14, e1006457. doi:10.1371/journal.pcbi.1006457
Keywords: three-finger toxins, antivenom efficacy, bioinformatics, immunogenicity, antigenicity, molecular docking
Citation: Chan YW, Tan CH, Heh CH and Tan KY (2023) An immunoinformatic approach to assessing the immunogenic capacity of alpha-neurotoxins in elapid snake venoms. Front. Pharmacol. 14:1143437. doi: 10.3389/fphar.2023.1143437
Received: 13 January 2023; Accepted: 27 March 2023;
Published: 10 April 2023.
Edited by:
Cassandra M. Modahl, Liverpool School of Tropical Medicine, United KingdomReviewed by:
Rohit Patel, Liverpool School of Tropical Medicine, United KingdomCopyright © 2023 Chan, Tan, Heh and Tan. This is an open-access article distributed under the terms of the Creative Commons Attribution License (CC BY). The use, distribution or reproduction in other forums is permitted, provided the original author(s) and the copyright owner(s) are credited and that the original publication in this journal is cited, in accordance with accepted academic practice. No use, distribution or reproduction is permitted which does not comply with these terms.
*Correspondence: Kae Yi Tan, a3l0YW5fa2FlQHVtLmVkdS5teQ==
Disclaimer: All claims expressed in this article are solely those of the authors and do not necessarily represent those of their affiliated organizations, or those of the publisher, the editors and the reviewers. Any product that may be evaluated in this article or claim that may be made by its manufacturer is not guaranteed or endorsed by the publisher.
Research integrity at Frontiers
Learn more about the work of our research integrity team to safeguard the quality of each article we publish.