- 1Department of Oncology of the First Affiliated Hospital & Cancer Institute, Hainan Medical University, Haikou, China
- 2Key Laboratory of Natural Products Research and Development from Li Folk Medicine of Hainan Province, Institute of Tropical Bioscience and Biotechnology, Chinese Academy of Tropical Agricultural Sciences, Haikou, China
- 3Key Laboratory of Emergency and Trauma of Ministry of Education & Key Laboratory of Tropical Cardiovascular Diseases Research of Hainan Province & Hainan Women and Children’s Medical Center, Hainan Medical University, Haikou, China
Background: Dendrobium nobile (D. nobile), a traditional Chinese medicine, has received attention as an anti-tumor drug, but its mechanism is still unclear. In this study, we applied network pharmacology, bioinformatics, and in vitro experiments to explore the effect and mechanism of Dendrobin A, the active ingredient of D. nobile, against pancreatic ductal adenocarcinoma (PDAC).
Methods: The databases of SwissTargetPrediction and PharmMapper were used to obtain the potential targets of Dendrobin A, and the differentially expressed genes (DEGs) between PDAC and normal pancreatic tissues were obtained from The Cancer Genome Atlas and Genotype-Tissue Expression databases. The protein-protein interaction (PPI) network for Dendrobin A anti-PDAC targets was constructed based on the STRING database. Molecular docking was used to assess Dendrobin A anti-PDAC targets. PLAU, one of the key targets of Dendrobin A anti-PDAC, was immunohistochemically stained in clinical tissue arrays. Finally, in vitro experiments were used to validate the effects of Dendrobin A on PLAU expression and the proliferation, apoptosis, cell cycle, migration, and invasion of PDAC cells.
Results: A total of 90 genes for Dendrobin A anti-PDAC were screened, and a PPI network for Dendrobin A anti-PDAC targets was constructed. Notably, a scale-free module with 19 genes in the PPI indicated that the PPI is highly credible. Among these 19 genes, PLAU was positively correlated with the cachexia status while negatively correlated with the overall survival of PDAC patients. Through molecular docking, Dendrobin A was found to bind to PLAU, and the Dendrobin A treatment led to an attenuated PLAU expression in PDAC cells. Based on clinical tissue arrays, PLAU protein was highly expressed in PDAC cells compared to normal controls, and PLAU protein levels were associated with the differentiation and lymph node metastatic status of PDAC. In vitro experiments further showed that Dendrobin A treatment significantly inhibited the proliferation, migration, and invasion, inducing apoptosis and arresting the cell cycle of PDAC cells at the G2/M phase.
Conclusion: Dendrobin A, a representative active ingredient of D. nobile, can effectively fight against PDAC by targeting PLAU. Our results provide the foundation for future PDAC treatment based on D. nobile.
Introduction
Pancreatic ductal adenocarcinoma (PDAC) is a highly aggressive malignancy with poor clinical prognosis and treatment outcomes, and its incidence and mortality rates have been increasing (GBD, 2017 Pancreatic Cancer Collaborators, 2019). Near 80%–90% of patients who suffered from PDAC had unresectable tumors or metastatic disease at the time of the first diagnosis, with a 5-year overall survival (OS) rate of 10%, despite the development of the radical surgical treatment. Although gemcitabine is a recognized first-line chemotherapy drug for PDAC, it is still unclear whether there is an advantage for survival (Zhang et al., 2022). Therefore, new cancer treatments are urgently needed for the treatment of metastatic or incurable PDAC (Von Hoff et al., 2013; Chen et al., 2020).
Cancer cachexia is a multifactorial combination of disease symptoms characterized by muscle wasting, which can lead to significant weight loss and affect patients’ quality of life, treatment-tolerant response, and survival (Vaughan et al., 2013). Freire et al. (2020) reported that variants in cachexia-inducible factors and their associated regulatory genes had the highest frequency in pancreatic cancer patients, and the expression levels of key cachexia genes had predictive prognostic value in pancreatic cancer. Meanwhile, specifically targeting PLA2G7 can alleviate cachexia levels in tumor-bearing mice (Morigny et al., 2021). These findings suggest that identification of cachexia-related factors and targeted intervention of cachexia play an important role in the treatment of malignant tumors, such as pancreatic cancer.
The use of traditional Chinese medicine (TCM) in pancreatic cancer treatment is gradually attracting the attention of clinicians. Dendrobium nobile (D. nobile), as a typical representative of TCM, has recently been reported in the field of anti-tumor drugs. Reports have increasingly shown that bibenzyl compounds extracted from D. nobile displayed promising effects against cancers derived from the lung (Losuwannarak et al., 2020; Putri et al., 2021), skin (Cardile et al., 2020), bladder (Zhu et al., 2019), liver (Chen et al., 2017), and breast (Yu et al., 2018). Dendrobin A (4,5-dihydroxy-3,3′-dimetheoxybibenzyl) is a new bibenzyl, and there is a lack of studies related to its role in pancreatic cancer; therefore, further elucidation of the anti-pancreatic cancer effect of Dendrobin A and other Dendrobium species is of great significance for the development of TCM anti-tumor therapy.
Network pharmacology is a novel approach that integrates systems biology and bioinformatics methods to predict drug targets using biological networks and big data technologies (Hopkins, 2008; Nogales et al., 2022), which has transformed the drug mechanism of action from a single-target, single-drug model to a network-target, multi-component therapeutic model, and has shown exciting results, especially in the field of TCM target prediction (Zhang et al., 2019). In this study, we integrated PDAC transcriptome expression data by network pharmacology technology and preferentially selected drug targets based on biological network characterization and survival analysis. Additionally, we performed in vitro experiments to validate the effects of Dendrobin A extracted from D. nobile on PDAC cells. Our results provide novel insights into Dendrobin A in treating PDAC and support the use of D. nobile in conventional medicine.
Materials and methods
Extraction and isolation of D. nobile gradient Dendrobin A
Dendrobin A was extracted and isolated from the D. nobile plant as per previously described protocols (Cao et al., 2021). Briefly, the air-dried stems of D. nobile (13.0 kg) were powdered and extracted with 95% EtOH three times. Then, the concentrated ethanolic extract (716.2 g) was suspended in H2O and successively partitioned with petroleum ether, EtOAc, and n-BuOH. Subsequently, the EtOAc extract (87.9 g) was fractionated by silica gel vacuum liquid column chromatography (CC) eluted with petroleum ether-EtOAc (20:1, 10:1, 5:1, 2:1, 1:1, 0:1, v/v) to generate 16 fractions (Fr.1–16). Fr.8 was separated by Rp-18 CC eluted with methanol-H2O (3:7→1:0) to obtain ten subfractions (Fr.8-1–Fr.8-10). Fr.8-6 was applied to the Sephadex LH-20 with chloroform-methanol (1:1) as eluent to produce three subfractions (Fr.8-6-1–Fr.8-6-3). Fr.8-6-1 was purified by silica gel CC eluted with petroleum ether-acetone (20:1→0:1) to give compound Dendrobin A.
Screening potential targets of Dendrobin A
To identify the potential targets of Dendrobin A, we first collected the drug compound information from PubChem (https://pubchem.ncbi.nlm.nih.gov/) with PubChem CID 44418770. The Dendrobin A canonical SMILES ‘COC1 = CC = CC (=C1) CCC2 = CC(=C(C(=C2)OC)O)O’ was submitted into the drug target predictions web server SwissTargetPrediction (Gfeller et al., 2014) and PharmMapper (Wang et al., 2017). After redundancy analysis and standardization, 353 well-reported pharmacological targets of Dendrobin A were obtained.
Differential expression genes (DEGs) of PDAC patients
Due to the limitation of adjacent normal samples in The Cancer Genome Atlas (TCGA) datasets, we performed DEG analysis by integrating the transcriptome of PDAC tumor samples in TCGA and normal pancreas tissues in the Genotype-Tissue Expression (GTEx) datasets according to the algorithm proposed in a previous report (Vivian et al., 2017). Data were obtained from the “TCGA TARGET GTEx” cohort in UCSC Xena (http://xena.ucsc.edu/), including sequencing counts and Transcripts Per Million (TPM) normalized expression matrices. All data were normalized and batch-corrected; the datasets used in this study included 178 cancer and 167 normal samples. The R package edgeR 3.30.3 (Robinson et al., 2010) was applied for DEGs analysis based on the gene read counts matrix. Only genes with |log2FC| > 2 and FDR <0.05 were considered significantly differentially expressed. An external dataset of PDAC was also downloaded from the GEO database (https://www.ncbi.nlm.nih.gov/geo/) with accession number GSE62452 (Yang et al., 2016), which included 69 pancreatic tumor and adjacent non-tumor tissues to validate the DEGs and clinical significance.
Construction and topological analysis of protein-protein interaction (PPI) network
The putative target genes of Dendrobin A and DEGs in PDAC samples were overlapped to identify the shared target genes for Dendrobin A in treating PDAC. These essential genes were next input into the STRING V11.5 (Szklarczyk et al., 2019) to construct the PPI network with default parameters. We performed a Pearson correlation analysis for each gene pair of the PPI network in PDAC samples to obtain the actual relationships in the tumor context. Correlation coefficients above 0.2 and p-value <0.05 were identified as significant. The PDAC-related PPI network was visualized using Cytoscape V3.7.2 (Shannon et al., 2003). The MCODE plugin was applied to identify the network modules. The PPI network’s degree distribution, clustering coefficient, and characteristic path length were analyzed using R package igraph 1.2.6. To explore the potential biological function of the PPI network, we input the genes to Metascape (https://metascape.org/gp/index.html#/main/step1) with the setting of species (“Homo sapiens”) (Zhou et al., 2019).
Survival prognosis analysis
The survival analysis of OS for essential genes of PDAC tumors in TCGA cohorts was performed through GEPIA2 (http://gepia2.cancer-pku.cn/) (Tang et al., 2019). The median expression of genes was used to divide the patients into high-expression and low-expression groups. Then, the OS of these groups was compared by log-rank test.
Estimation of the association between genes and cachexia
The information on 25 cachexia-inducing factors (CIFs) was collected from a previous study (Freire et al., 2020). The single-sample GSEA (ssGSEA) algorithm (Barbie et al., 2009) was applied to estimate the cachexia score for each sample. Wilcoxon’s rank sum tests evaluated the cachexia score between PDAC in TCGA and normal samples in GTEx. Pearson correlation analysis was performed to estimate the association between the expression level of genes and cachexia score.
Molecular docking
In this study, the drug-protein binding patterns were predicted by molecular docking, which was performed using AutoDock Vina 1.1.21 software (The Scripps Research Institute, La Jolla, CA, United States) (Trott and Olson, 2010). Before the start of docking, the PLAU protein crystal structure was obtained from the PDB database with the protein search number 6AG7. The 3D structure of Dendrobin A was obtained from the PubChem database download, and energy minimization of the small molecule was performed using the MMFF94 force field. Before the start of formal docking, the protein was prepared using PyMol 2.5.2 software, including dehydrogenation, dehydration molecules, and non-liganded small molecules. The docking box was then defined to wrap the protein activity pocket. Then, the small molecules in PDB format and the receptor proteins were converted to PDBQT format using ADFRsuite 1.02 (Ravindranath et al., 2015). Finally, the docking work was performed, visualized, and analyzed using PyMol 2.5.2.
Assessment of Dendrobin A on cell proliferation by cell counting Kit-8 (CCK-8) assay
The human PDAC cell lines Panc-1 and Aspc-1, as well as the normal pancreatic cell line HPDE6-C7, were purchased from the Shanghai Chinese Academy of Sciences Cell bank. All cells were tested by short tandem repeat to confirm that there was no cross-contamination, and routine mycoplasma and chlamydia contamination tests were performed before the experiments. Cells were cultured in Dulbecco’s modified eagle medium (HyClone, Beijing, China) supplemented with 10% fetal bovine serum (HyClone), 100 μg/mL streptomycin, and 100 U/mL penicillin (Beyotime, Nanjing, China), at 37°C in 5% CO2. Dendribin A was dissolved in dimethyl sulfoxide (DMSO), and the concentration of DMSO was <0.1%. The proliferation and cytotoxicity of three pancreatic cell lines were measured by a cell counting kit (CCK-8, Beyotime); the cells were seeded into a 96-well plate (2,000 cells per well) and incubated overnight. Cells were treated with 10, 20, 30, 40, 50, 60, 70, 80, 90, and 100 µM of Dendrobin A for 24 h at 37°C. After the CCK-8 reagent was added and incubated for 1 h, the optical density was detected at the 450 nm wavelength. Five replicate wells were used for each group, and experiments were repeated three times.
Clone formation assay
PDAC cells at a density of 3,000 cells/well were seeded into 6-cm dishes overnight. The cells were treated with a medium containing Dendrobin A or DMSO for 24 h, and then the medium was replaced with Dendrobin A-free completed medium and cultured for 15 days. The culture medium was discarded, and then cells were washed twice with PBS, fixed in methanol for 20 min, and air dried after discarding methanol. The cells were stained with 0.3% crystal violet solution for 15 min and washed gently with ddH2O to remove the staining solution. The number of clones with >50 cells was counted after photographing. Statistical analysis was performed using Image J software (version 1.8.0). Experiments were repeated three times.
Wound healing assay
PDAC cells were seeded on 12-well plates to reach the monolayer, and a linear wound was formed by scraping with a 200 μL micropipette tip, and the cells were treated with Dendrobin A for 24 h. The cell scratch area was observed with a ×20 microscope objective and photographed, and the wound distance was used to assess the cell migration rate. The degree of wound healing was calculated by analysis with Image J software (version 1.8.0). Experiments were repeated three times.
Invasive and migration assay
The ability of PDAC cells to invade and migrate was assayed using the Transwell method. Matrigel gel (BD, China, Shanghai) and serum-free medium were diluted at a ratio of 1:8, and 100 μL per well was added in the upper chamber of the Transwell (Corning, NC, United States) and incubated at 37°C for 3 h. The migration assay was performed without Matrigel gel, and the procedure was the same. 4 × 104 Aspc-1 cells and 1 × 105 Panc-1 cells were loaded into the upper chamber with 200 µL of serum-free medium, while medium containing 20% serum was added to the lower chamber after 24 h of incubation. The upper chamber was fixed in 4% paraformaldehyde and stained with 0.1% crystal violet. Invaded or migrated cells were counted and photographed, and differences in the number of cells that penetrated the membrane represented the altered cell motility. Statistical analysis was performed using Image J software (version 1.8.0). Experiments were repeated three times.
Flow cytometry analysis of cell cycle and apoptosis
PDAC cells were added in 6-well plates allowing recovery overnight, then Dendrobin A was added for 24 h, and the cells were harvested for cell cycle and apoptosis analysis. PDAC cells were stained with propidium iodide (PI) for cell cycle analysis. Cell Cycle Assay Kit-PI/RNase staining (BD, China) was followed by the detection of cell ratios in the G0/G1, S, and G2/M phases. For apoptosis, PDAC cells were stained with Annexin V fluorescein isothiocyanate (FITC) and PI using the Annexin V-FITC Apoptosis Detection Kit (BD, China). Three wells were used for each group. Experiments were repeated three times.
Tissue microarrays and immunohistochemical staining
Human tissue microarrays of PDAC (#HPanA180Su03, Shanghai Outdo Biotech Company, China) were used to detect the expression of PLAU protein. Tissue arrays include 81 cancer tissues and 81 paracancerous tissues from surviving PDAC patients. Immunohistochemical staining (IHC) was performed using an antibody against PLAU (1:500 dilution, #17968-1-AP, Proteintech, Wuhan, China). The immunohistochemical staining protocol was performed according to the literature (Bai et al., 2022). The scores of PLAU were calculated briefly as staining intensity: 0, no staining; 1, light yellow; 2, brown; and 3, dark brown; staining positive rate: 0%–100%. The total score was calculated as follows: “staining intensity score” × “staining positive rate” ×10. The mean value of a total score of 15.75 for all PDAC samples was used as the grouping criterion, and samples with a score of ≥15.75 belonged to the high-expression group, while samples with a score of <15.75 were included in the low-expression group.
Western blot
Total proteins were extracted using RIPA buffer (Thermo Fisher Scientific, MA, United States). A total of 30 μg proteins was subjected to 10% Sodium dodecyl sulfate-polyacrylamide gel electrophoresis (SDS-PAGE), and proteins were transferred to a polyvinylidene fluoride membrane (Merk Millipore, Darmstadt, Germany). The membranes were blocked in 5% bovine serum albumin and incubated with primary antibodies anti-PLAU (1:2,000, Proteintech) and β-actin (1:2000, #66009-1-Ig, Proteintech) at 4°C overnight. The membranes were incubated with HRP-conjugated IgGs (1:1,000, #7074, CST, China, Shanghai) for 1 h at room temperature, and the protein was detected using enhanced chemiluminescence (Bio-Rad, China, Shanghai). Protein bands were analyzed using Image J software (version 1.8.0). Experiments were repeated three times.
Statistical analysis
For network pharmacology and bioinformatics, the statistical analyses were performed using R software. The associations between clinical features and PLAU expression were evaluated using the Wilcoxon signed-rank test and the chi-square test. Clinical features related to OS in PDAC patients were identified using the Kaplan-Meier method. For in vitro experiments, data were shown as mean ± SD. Statistical analysis was performed with GraphPad Prism 8.0 software, and the significance was analyzed with Student’s t-test or ANOVA. p < 0.05 was considered statistically significant.
Results
Extraction, isolation, and identification of Dendrobin A
According to our previously described protocols (Cao et al., 2021), we successfully obtained 548.4 mg Dendrobin A from 13.0 kg D. nobile. The structure of Dendrobin A is shown in Figure 1. In addition, the 1H NMR (500 MHz) and 13C NMR (125 MHz) data of Dendrobin A in CDCl3 were included in Table 1.
Identification and analysis of Dendrobin A potential targets in PDAC
Based on SwissTargetPrediction and PharmMapper databases, 353 pharmacological targets of Dendrobin A were identified. To explore the expression pattern of these putative drug targets in a tumor context, we performed DEG analysis by integrating the transcriptome of pancreas tumor in TCGA and normal pancreatic samples in GTEx datasets. We identified 3,131 DEGs, comprising 2,358 upregulated and 773 downregulated genes in PDAC samples (Figure 2A). By overlapping 3,131 DEGs and 353 drug targets, we found 90 genes related to Dendrobin A treatment and expressed differently in PDAC samples. Among them, 76 were upregulated, and 14 were downregulated (Figure 2B). Further functional enrichment analysis indicated that these genes were significantly enriched in several metabolism-related biological processes like collagen catabolic, organic hydroxyl compound metabolic process, and response to the drug (Figure 2C). For KEGG pathways, cancer-related pathways like transcriptional misregulation, PPAR signaling, and drug metabolism were significantly enriched (Figure 2D). Next, we obtained 504 genes related to PDAC from MalaCards and intersected with the 90 essential genes mentioned above. We identified 13 shared genes, namely, CCNA2, AURKA, ALB, LCN2, NQO1, CDA, PLAU, MMP7, REG1A, MMP9, LGALS3, MMP2, and PIK3CG (Figure 2E).
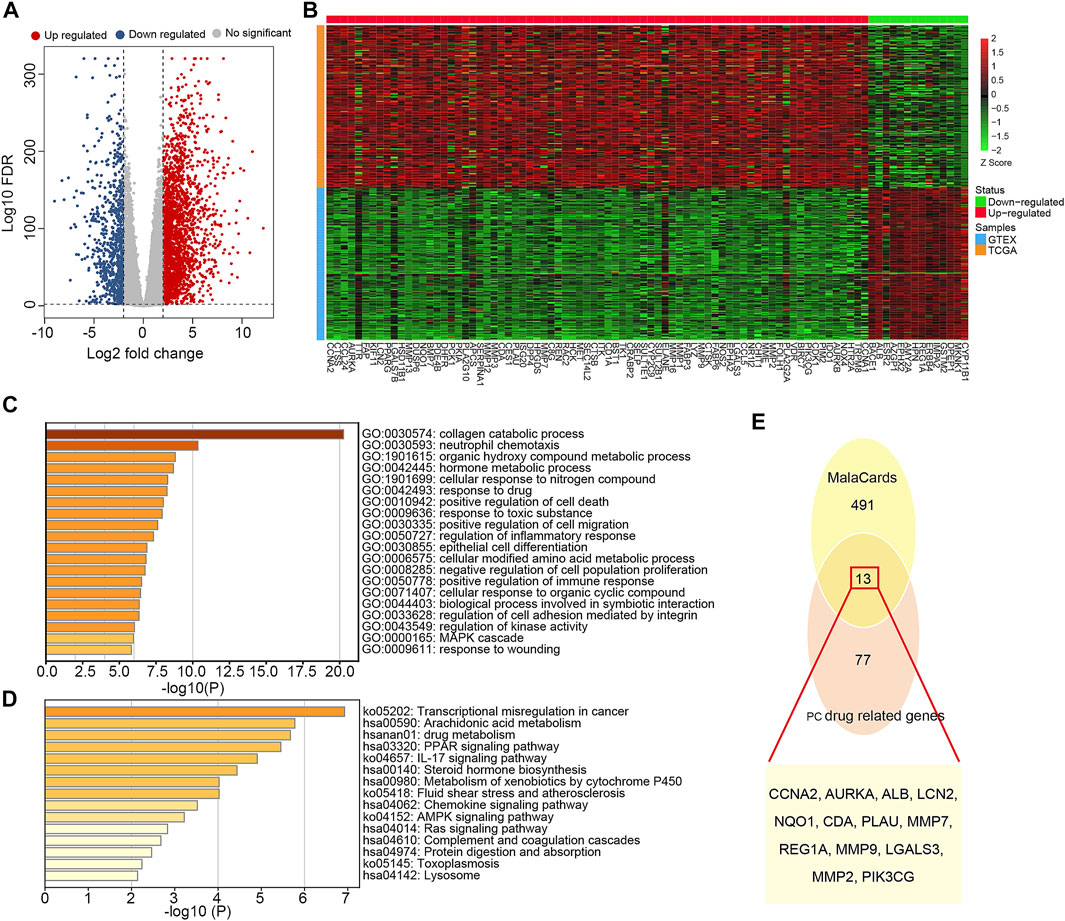
FIGURE 2. Identification and functional annotation of the overlapped targets of pancreatic ductal adenocarcinoma (PDAC) and Dendrobin A. (A) Volcano plot of differentially expressed genes related to PDAC; red dots represent upregulated genes, and blue dots represent downregulated genes. (B) Heat map of Dendrobin A target genes involved in PDAC; red boxes represent upregulated genes, and green boxes represent downregulated genes. (C) The biological process of Dendrobin A target genes involved in PDAC in GO annotation. (D) KEGG pathway enrichment of Dendrobin A target genes involved in PDAC. (E) Dendrobin A target genes involved in PDAC based on MalaCards.
Dendrobin A-related PPI network
The 90 essential Dendrobin A-related DEGs were input into STRING to obtain the interactions of proteins. As a result, a PPI network containing 81 nodes and 299 edges was constructed. Through co-expression analysis and based on the threshold of |Pearson r| > 0.2 & p < 0.05, we found 57.86% (173/299) positive correlation edges and 7.02% (21/299) negative correlation edges in PDAC samples (Figure 3A). We utilized the hierarchical model that linked genes, biological processes, and previously described cancer hallmarks to determine the association between the PPI network and tumorigenesis (Poulia et al., 2020). Many genes in the PPI network were associated with multiple cancer hallmarks (Figure 3B). These results suggest that Dendrobin A-related genes play essential roles in cancer processes. Through topological feature analysis, we found that the PPI network displayed scale-free distribution with R2 = 0.682 (Supplementary Figure S1A), suggesting that the network showed scale-free characteristics. Moreover, the PPI network’s clustering coefficient and characteristic path length were significantly increased when compared with random networks, as expected for reduced global efficiency and module characteristics (p-value <0.001, Supplementary Figures S1B, C). Therefore, a critical PPI network module with 19 genes was extracted through the MCODE plugin in Cytoscape software (score: 5.556, Figure 3C). The functional enrichment results showed that this module was strongly associated with histone phosphorylation, collagen catabolic process, and pathways in cancer (Figure 3D).
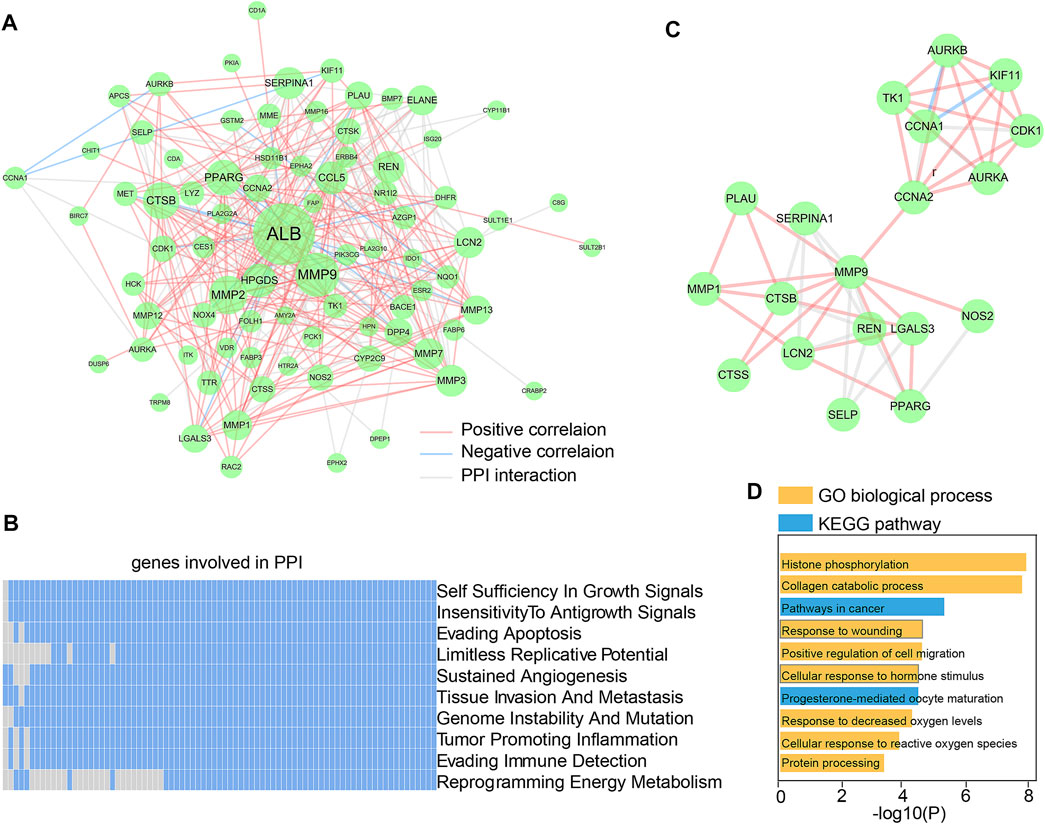
FIGURE 3. Construction and functional analysis of Dendrobin A-associated PPI network. (A) Dendrobin A-related PPI network in PDAC; red lines represent positively interacting genes in PDAC, blue lines represent negatively interacting genes in PDAC, and the node sizes represent connectivity. (B) Association of genes in PPI network with ten cancer hallmarks; blue indicates the positive relation to cancer hallmarks, and gray indicates non-relation. (C) Key network module based on MCODE. (D) Module function enrichment.
Expression and survival significance of Dendrobin A-related genes in PDAC
We next explored the significance of 19 Dendrobin A-related genes in the survival of PDAC patients. We found that patients with higher expression of AURKA, CCNA2, CDK1, KIF11, LGALS3, MMP1, and PLAU had worse OS in PDAC patients (HR > 1 and log-rank p < 0.05). At the same time, CCNA1 indicated a favorable prognosis in PDAC (HR < 1 and log-rank p < 0.05, Figure 4A), and the remaining 11 genes showed no significance with OS of PDAC (data not shown). Furthermore, the expression levels of these eight OS-related genes were displayed (Figure 4B). Therefore, these eight OS-related genes, except CCNA1, may play oncogenic roles in PDAC.
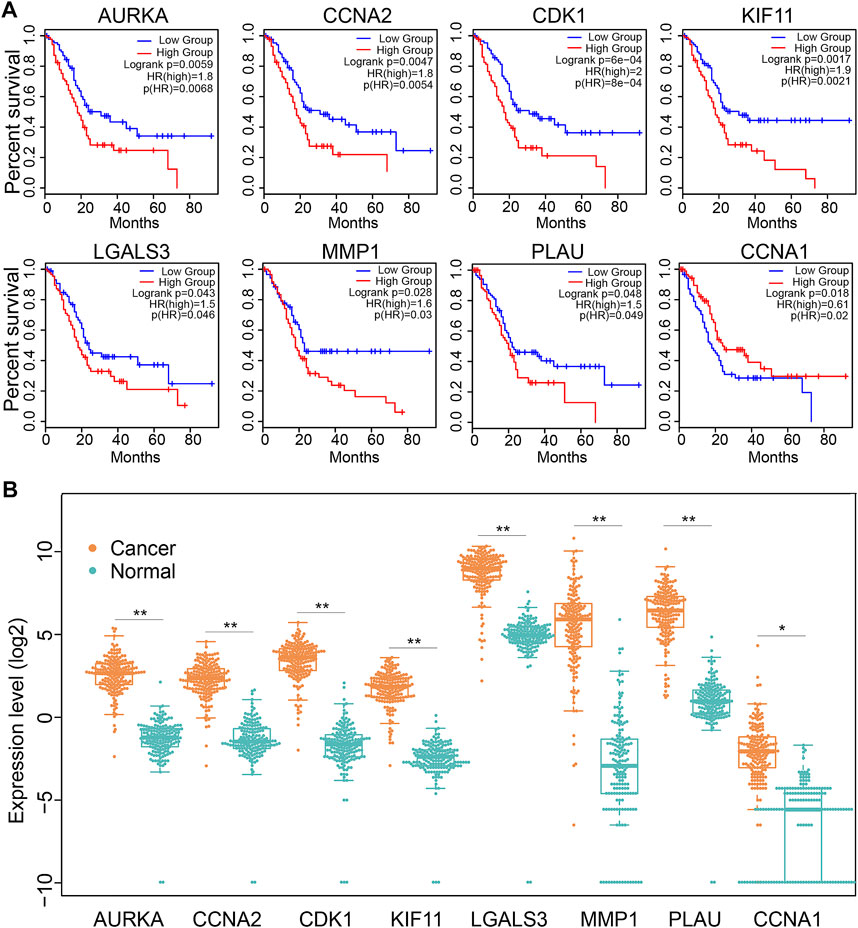
FIGURE 4. The expression and association of key Dendrobin A-related genes with OS in the PDAC cohort. (A) Overall survival analysis of key Dendrobin A-related genes in PDAC. (B) Expression of key Dendrobin A-related genes in PDAC and normal pancreatic tissues. Wilcoxon’s rank sum tests, *p < 0.05, **p < 0.01.
Association of Dendrobin A-related genes with cachexia in PDAC
Since cachexia is a classic feature of PDAC patients (Freire et al., 2020), we next explored whether the dysregulation of Dendrobin A-related genes was associated with cachexia in PDAC patients. DEG analysis showed that 18 of 25 cachexia-induced factors were upregulated in PDAC samples (Figure 5A). In addition, the cachexia score of cancerous pancreatic tissues was significantly higher than that of normal pancreatic tissues (Figure 5B). We thus evaluated the associations between risky prognostic genes and cachexia in PDAC patients. As a result, the expression level of PLAU was significantly positively correlated with the cachexia score (Figure 5C). Consistent with this finding, a similar upregulated expression pattern, prognostic risk, and the positive correlation with cachexia of PLAU levels in another PDAC dataset were observed (GSE62452, Figures 5D–G). We analyzed the association between PLAU mRNA levels and PDAC patients’ clinical parameters, including age, gender, stage, and nodal metastasis status. The results showed that PLAU was differentially expressed between stage 1 and stage 2 (Supplementary Figure S2).
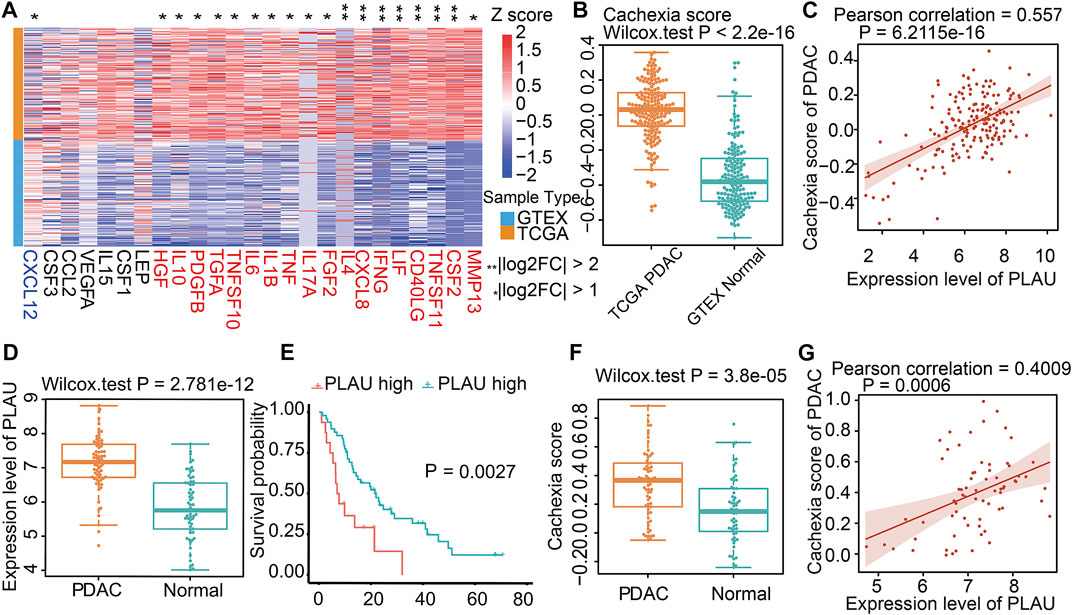
FIGURE 5. Cachexia-inducing factors in PDAC and validation. (A) Enrichment of Cachexia-inducing factors in PDAC based on TCGA and GTEX databases. Genes in red indicate a positive association with cachexia. (B) Comparison of cachexia scores for genes in red shown in Figure 5A for PDAC and normal tissue. (C) Correlation of PLAU gene with cachexia score in PDAC. (D–G) Validation of the expression, correlation PLAU in PDAC, and cachexia using external data from GEO dataset GSE62452.
Molecular docking
Molecular docking is a convenient and effective means to explore the interaction of small molecules with their targets (Trott and Olson, 2010; Ravindranath et al., 2015); we used Vina 1.1.21 software to perform a molecular docking study of Dendrobin A with PLAU proteins. Interestingly, Dendrobin A is bound within the PLAU active pocket (score: −6.9 kcal/mol) (Figure 6A). In addition, western blot results showed that Dendrobin A treatment reduced the expression level of PLAU protein in PDAC cells (Figure 6B).
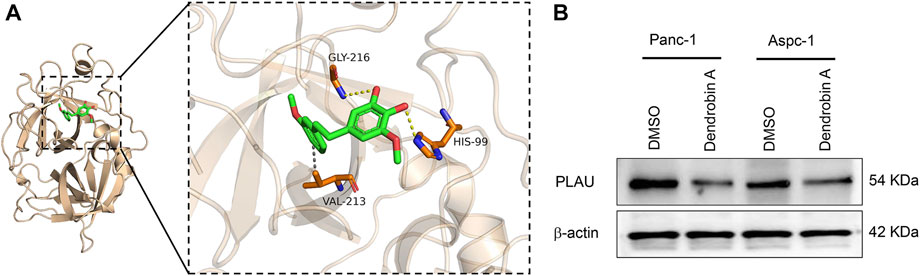
FIGURE 6. Molecular docking of Dendrobin A and PLAU. (A) The representative images for molecular docking of Dendrobin A and PLAU. Dendrobin A (green) binds with PLAU (orange) using hydrophobic interaction and hydrogen (dashed line). (B) Dendrobin A inhibiting PLAU protein expression in PDAC cells assessed by western blotting.
PLAU expression and association with clinical parameters in PDAC patients
We further examined PLAU protein expression in clinical PDAC samples by IHC. Commercial tissue arrays with 81 cases of PDAC and paired paraneoplastic tissues were used. The results showed that PDAC PLAU protein levels were significantly enhanced compared to paraneoplastic tissues (Figure 7A). Further analysis showed that higher PLAU protein expression in PDAC was positively correlated with the differentiation and lymph node metastasis status of PDAC (Figures 7B, C). Interestingly, PLAU protein expression in PDAC was associated with gender but not age, T classification, M classification, TNM stage, tumor size, and vascular and nerve invasion (Table 2).
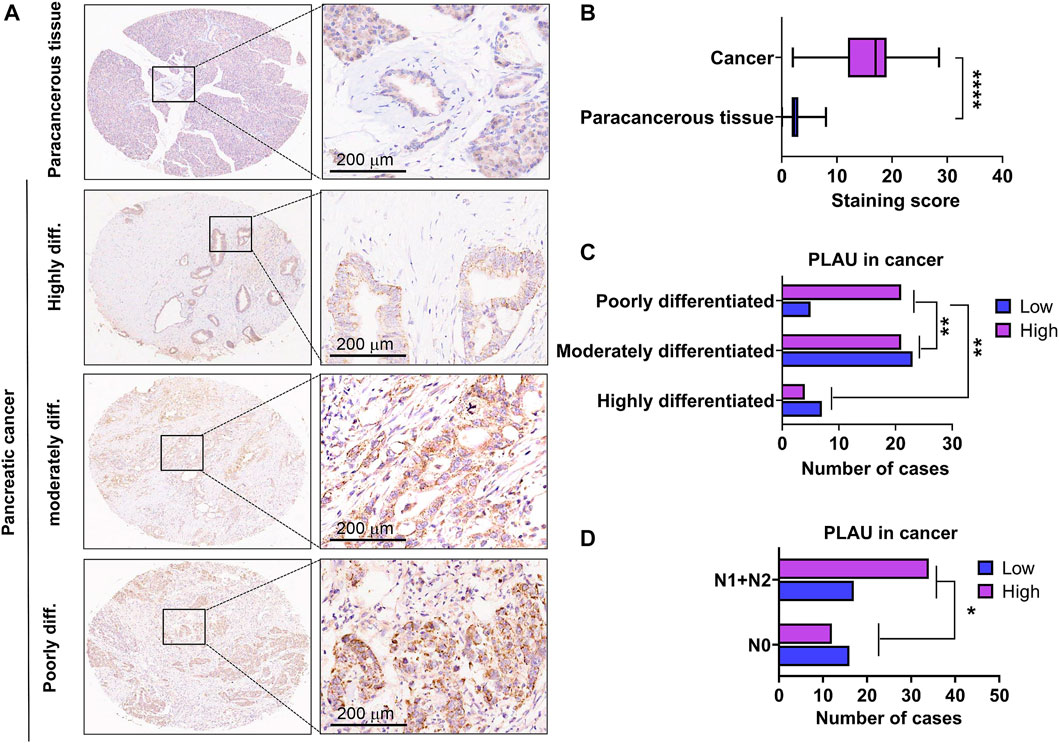
FIGURE 7. Expression levels of PLAU protein in PDAC and paraneoplastic tissues. (A) Representative images of PLAU protein in PDAC and control tissues by IHC staining. Bars = 200 μm. (B) Quantitative results of PLAU protein in PDACs and controls, ****p < 0.0001. (C) Correlation between PLAU protein expression in PDAC and tumor differentiation status of patients, **p < 0.01. (D) Correlation between PLAU protein expression in PDAC and lymph node metastatic status of patients, *p < 0.05.
In vitro validation of Dendrobin A on the proliferation, cycle, apoptosis, and migration in PDAC cells
Results of the CCK-8 assay indicated that Dendrobin A effectively inhibited the proliferation potentials of PDAC cells in a concentration-dependent manner but not for normal pancreatic cells (Figure 8A). Subsequent experiments were performed using Dendrobin A at 60 µM according to its IC 50 values. The colony formation assay results further confirmed the inhibitory effects of Dendrobin A on PDAC cells (Figure 8B). Considering that cell cycle and apoptosis are closely related to cell proliferation, we applied to flow cytometry to examine the effect of Dendrobin A on PDAC cell cycle and apoptosis. The results showed that the values of G2/M phases in PDAC cell lines Aspc-1 and Panc-1 have increased by 88.5% and 256% post-drug treatment, respectively (Figure 8C), and the total number of apoptotic cells in Dendrobin A-treated Aspc-1 and Panc-1 cells were increased to 77% and 79.5%, respectively (Figure 8D). We also tested the effects of Dendrobin A on PDAC cell migration and invasion. The wound healing and Transwell migration assay showed that Dendrobin A impaired PDAC cell migration and invasion abilities (Figures 8E, F).
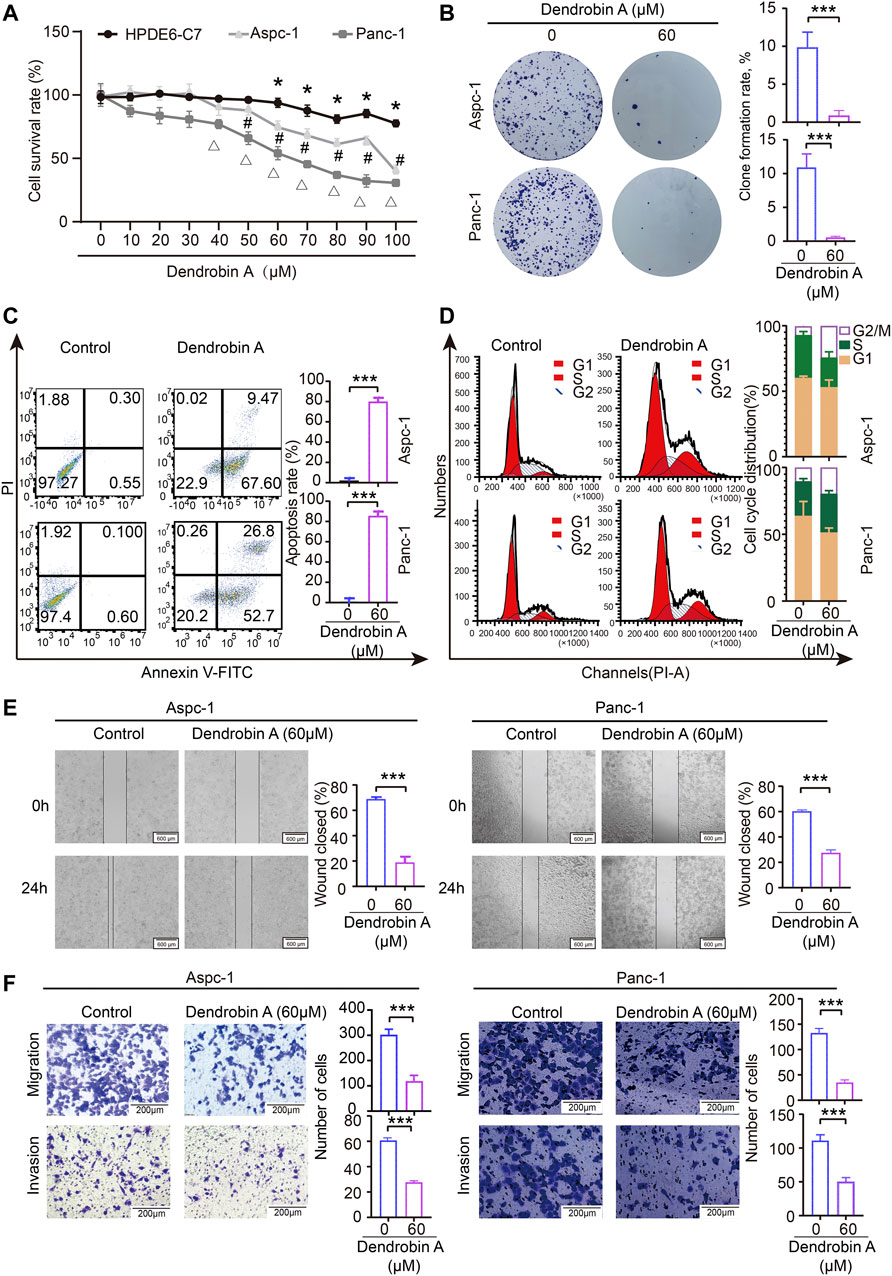
FIGURE 8. Effects of Dendrobin A on PDAC cell proliferation, cycle, apoptosis, migration, and invasion. (A) Results of CCK-8 assay; compared with 0 μM group, *p < 0.05, #p < 0.05, and Δp < 0.05. (B) Results of plate clone formation assay, ***p < 0.001. (C) Results of apoptosis, ***p < 0.001. (D) Results of the cell cycle analysis. (E). Results of cell migration; ***p < 0.001, bars = 600 μm. (F) Results of invasion; ***p < 0.001, bars = 200 μm.
Discussion
TCM plays a special role in human life and health. Network pharmacology is an emerging technology to explore and identify the key targets of drugs, which provides an alternative way of exploring the application of TCM in the field of tumor treatment (Tang and Aittokallio, 2014; Asakawa et al., 2020). As one of the effective extracts of D. nobile, bibenzyl has various pharmacological activities such as anti-tumor, -inflammatory, and -diabetic (Zhao et al., 2018; Asakawa et al., 2020). Dendrobin A is a recently identified type of bibenzyl extracted from D. nobile (He et al., 2020), which has anti-cancer activities. However, its effects and mechanism of action on specified tumors remain unsolved. The goal of this work was to uncover the effects and mechanisms of Dendrobin A against PDAC.
Using SwissTargetPrediction and PharmMapper platform, 353 targets for Dendrobin A were identified. Subsequently, based on TCGA and GTXs databases, 3,131 DEGs between PDAC and normal pancreatic tissues were obtained. Finally, 90 potential targets of Dendrobin A against PDAC were identified. GO annotation indicated that these 90 genes were involved in metabolism-related biological processes and responses to drugs, while KEGG signals enrichment showed that cancer-related pathways and drug metabolism were enriched. To further explore the signatures of targets for Dendrobin A against PDAC, we overlapped these 90 genes with targets related to PDAC from the MalaCards database, and 13 key genes previously reported in PDAC were found, namely, AURKA, CCNA2, ALB, LCN2, NQO1, CDA, PLAU, LGALS3, MMP2, REG1A, MMP7, MMP9, and PIK3CG. Therefore, these 90 genes indicated that the role of Dendrobin A on PDAC is multifaceted.
Next, we established a PPI for these 90 genes using the STRING database. As a result, a close link among the PPIs was identified. We also found that a specific module consisted of 19 key genes, namely, AURKA, KIF11, TK1, CDK1, CCNA1, AURKB, CCNA2, PLAU, SERPINA1, STSB, CTSS, REN, SELP, PPARG, LGALS3, MMP1, MMP9, NOS2, and LCN2. The functional analysis further indicated that these 19 genes were indeed involved in pathways in cancer. Thus, these 19 genes provided a cue for Dendrobin A against PDAC.
Given that these 19 genes play a critical role in PDAC carcinogenesis, we analyzed the significance of these 19 genes in the OS of PDAC patients. However, eight genes displayed the OS-predicated values in PDAC. Among them, high expression of AURKA, CCNA2, CDK1, KIF11, LGALS3, MMP1, and PLAU is the risk factor, while high expression of CCNA1 is a protective factor for PDAC. Indeed, AURKA (Gomes-Filho et al., 2020), CCNA2 (Dong et al., 2019), CDK1 (Piao et al., 2019), KIF11 (Gu et al., 2022), LGALS3 (Yi et al., 2020), MMP1 (Chen et al., 2019), and PLAU (Kisling et al., 2021; Wang et al., 2022) are significantly associated with the prognosis of PDAC patients. Our current study provides focus targets of Dendrobin A against PDAC.
Cachexia, a typical characteristic of malignant tumors, especially for pancreatic cancer patients, is significantly associated with PDAC prognosis, treatment, and quality of life. Studies have reported the positive role of TCM in improving the cachexia status of tumor patients (Xu et al., 2021). In this study, we found a high association of Dendrobin A targets with PDAC cachexia. Among these genes, PLAU highlights the association with PDAC cachexia. Increasing reports showed that high expression of PLAU is a risk factor in various tumors, including PDAC (Ai et al., 2020; Fang et al., 2021; Li et al., 2021; Wang et al., 2022). Additionally, molecular docking found that Dendrobin A could be bound within the PLAU active pocket. In addition, after treating the PDAC cells with Dendrobin A, PLAU expression in PDAC cell lines was also dramatically changed. Thus, PLAU may have important molecular targeting value in anti-PDAC treatment. Indeed, an early report has proposed that PLAU is a potent target for the anti-PDAC using TCM (Zhao et al., 2020) and a potential prognostic marker in PDAC (Kisling et al., 2021; Wang et al., 2022). Since PLAU was one of the representative key targets identified in this study, the expression level of PLAU protein in PDAC and control paraneoplastic tissues in tissue microarrays was detected by immunohistochemistry. The results showed that the expression of PLAU was significantly higher in PDAC tissues, and its expression level correlated with the differentiation and lymph node metastasis status of PDAC. We also observed that PLAU protein levels were higher in female PDAC patients than in male PDAC patients, though the reason for this is unknown. Together, these results highlight the pathophysiological role of PLAU in PDAC.
Finally, we performed several in vitro experiments to test the feasibility of anti-PDAC using Dendrobin A. Similar to the expected results, we found that administration of PDAC cells with Dendrobin A led to substantially anti-cancer effects, as evidenced by the attenuated cell viability, migration, invasion, and enhanced apoptosis, and changed the cell cycle. Thus, the anti-PDAC properties of Dendrobin A are versatile.
In summary, our current study integrated network pharmacology, bioinformatics, and validation experiments, and found that Dendrobin A may exert its anti-PDAC action through multiple targets, and that PLAU may be a potential target for Dendrobin A against PDAC. Our study provides alternative evidence for the use of TCM to treat PDAC. However, a larger cohort and animal experiments are needed to verify these results.
Data availability statement
The original contributions presented in the study are included in the article/Supplementary Material, further inquiries can be directed to the corresponding authors.
Ethics statement
Ethical review and approval was not required for the study on human participants in accordance with the local legislation and institutional requirements. Written informed consent for participation was not required for this study in accordance with the national legislation and the institutional requirements.
Author contributions
SZ, WJ, and WM developed the concept and designed the study. LY and HD extracted and isolated Dendrobin A from D. nobile. XX, YY, BW, BR, and YF performed most of the research. WJ and SZ supervised the research. XX and WJ wrote the manuscript. BR, BW, and XZ helped in the investigation and data analysis. All authors proofread and approved the final manuscript.
Funding
This work was supported by Hainan Province Science and Technology special fund (ZDYF2020132, ZDYF2022SHFZ065 to SZ; ZDYF2021SHFZ098 to XZ); the National Natural Science Foundation of China (81960528 to SZ); the specific research fund of the Innovation Platform for Academicians of Hainan Province (YSPTZX202208 to SZ, YSPTZX202037 to WM); and Hainan Province Clinical Medical Center (QWYH2021276 to SZ).
Acknowledgments
We thank LetPub (www.letpub.com) for its linguistic assistance during the preparation of this manuscript.
Conflict of interest
The authors declare that the research was conducted in the absence of any commercial or financial relationships that could be construed as a potential conflict of interest.
Publisher’s note
All claims expressed in this article are solely those of the authors and do not necessarily represent those of their affiliated organizations, or those of the publisher, the editors and the reviewers. Any product that may be evaluated in this article, or claim that may be made by its manufacturer, is not guaranteed or endorsed by the publisher.
Supplementary material
The Supplementary Material for this article can be found online at: https://www.frontiersin.org/articles/10.3389/fphar.2023.1079539/full#supplementary-material
References
Ai, C., Zhang, J., Lian, S., Ma, J., Gyorffy, B., Qian, Z., et al. (2020). FOXM1 functions collaboratively with PLAU to promote gastric cancer progression. J. Cancer 11, 788–794. doi:10.7150/jca.37323
Asakawa, Y., Nagashima, F., and Ludwiczuk, A. (2020). Distribution of bibenzyls, prenyl bibenzyls, bis-bibenzyls, and terpenoids in the liverwort genus radula. J. Nat. Prod. 83, 756–769. doi:10.1021/acs.jnatprod.9b01132
Bai, J., Zheng, A., Ha, Y., Xu, X., Yu, Y., Lu, Y., et al. (2022). Comprehensive analysis of LAMC1 expression and prognostic value in kidney renal papillary cell carcinoma and clear cell carcinoma. Front. Mol. Biosci. 9, 988777. doi:10.3389/fmolb.2022.988777
Barbie, D. A., Tamayo, P., Boehm, J. S., Kim, S. Y., Moody, S. E., Dunn, I. F., et al. (2009). Systematic RNA interference reveals that oncogenic KRAS-driven cancers require TBK1. Nature 462, 108–112. doi:10.1038/nature08460
Cao, X., Yang, L., Dai, H., Mei, Y., Huang, S., Wang, H., et al. (2021). One new lignan and one new fluorenone from Dendrobium nobile Lindl. Phytochem. Lett. 44, 164–168. doi:10.1016/j.phytol.2021.06.022
Cardile, V., Avola, R., Graziano, A. C. E., and Russo, A. (2020). Moscatilin, a bibenzyl derivative from the orchid Dendrobium loddigesii, induces apoptosis in melanoma cells. Chem. Biol. Interact. 323, 109075. doi:10.1016/j.cbi.2020.109075
Chen, H., Huang, Y., Huang, J., Lin, L., and Wei, G. (2017). Gigantol attenuates the proliferation of human liver cancer HepG2 cells through the PI3K/Akt/NF-κB signaling pathway. Oncol. Rep. 37, 865–870. doi:10.3892/or.2016.5299
Chen, P., Wu, Q., Feng, J., Yan, L., Sun, Y., Liu, S., et al. (2020). Erianin, a novel dibenzyl compound in Dendrobium extract, inhibits lung cancer cell growth and migration via calcium/calmodulin-dependent ferroptosis. Signal Transduct. Target Ther. 5, 51. doi:10.1038/s41392-020-0149-3
Chen, Y., Peng, S., Cen, H., Lin, Y., Huang, C., Chen, Y., et al. (2019). MicroRNA hsa-miR-623 directly suppresses MMP1 and attenuates IL-8-induced metastasis in pancreatic cancer. Int. J. Oncol. 55, 142–156. doi:10.3892/ijo.2019.4803
Dong, S., Huang, F., Zhang, H., and Chen, Q. (2019). Overexpression of BUB1B, CCNA2, CDC20, and CDK1 in tumor tissues predicts poor survival in pancreatic ductal adenocarcinoma. Biosci. Rep. 39, BSR20182306. doi:10.1042/BSR20182306
Fang, L., Che, Y., Zhang, C., Huang, J., Lei, Y., Lu, Z., et al. (2021). PLAU directs conversion of fibroblasts to inflammatory cancer-associated fibroblasts, promoting esophageal squamous cell carcinoma progression via uPAR/Akt/NF-κB/IL8 pathway. Cell. Death Discov. 7, 32. doi:10.1038/s41420-021-00410-6
Freire, P. P., Fernandez, G. J., de Moraes, D., Cury, S. S., Dal Pai-Silva, M., Dos Reis, P. P., et al. (2020). The expression landscape of cachexia-inducing factors in human cancers. J. Cachexia Sarcopenia Muscle 11, 947–961. doi:10.1002/jcsm.12565
GBD 2017 Pancreatic Cancer Collaborators (2019). The global, regional, and national burden of pancreatic cancer and its attributable risk factors in 195 countries and territories, 1990-2017: A systematic analysis for the global burden of disease study 2017. Lancet Gastroenterol. Hepatol. 4, 934–947. doi:10.1016/S2468-1253(19)30347-4
Gfeller, D., Grosdidier, A., Wirth, M., Daina, A., Michielin, O., and Zoete, V. (2014). SwissTargetPrediction: A web server for target prediction of bioactive small molecules. Nucleic Acids Res. 42, W32–W38. doi:10.1093/nar/gku293
Gomes-Filho, S. M., Dos Santos, E. O., Bertoldi, E. R. M., Scalabrini, L. C., Heidrich, V., Dazzani, B., et al. (2020). Aurora A kinase and its activator TPX2 are potential therapeutic targets in KRAS-induced pancreatic cancer. Cell. Oncol. (Dordr) 43, 445–460. doi:10.1007/s13402-020-00498-5
Gu, X., Zhu, Q., Tian, G., Song, W., Wang, T., Wang, A., et al. (2022). KIF11 manipulates SREBP2-dependent mevalonate cross talk to promote tumor progression in pancreatic ductal adenocarcinoma. Cancer Med. 11, 3282–3295. doi:10.1002/cam4.4683
He, L., Su, Q., Bai, L., Li, M., Liu, J., Liu, X., et al. (2020). Recent research progress on natural small molecule bibenzyls and its derivatives in Dendrobium species. Eur. J. Med. Chem. 204, 112530. doi:10.1016/j.ejmech.2020.112530
Hopkins, A. L. (2008). Network pharmacology: The next paradigm in drug discovery. Nat. Chem. Biol. 4, 682–690. doi:10.1038/nchembio.118
Kisling, S. G., Natarajan, G., Pothuraju, R., Shah, A., Batra, S. K., and Kaur, S. (2021). Implications of prognosis-associated genes in pancreatic tumor metastasis: Lessons from global studies in bioinformatics. Cancer Metastasis Rev. 40, 721–738. doi:10.1007/s10555-021-09991-1
Li, Z., Chen, C., Wang, J., Wei, M., Liu, G., Qin, Y., et al. (2021). Overexpressed PLAU and its potential prognostic value in head and neck squamous cell carcinoma. PeerJ 9, e10746. doi:10.7717/peerj.10746
Losuwannarak, N., Roytrakul, S., and Chanvorachote, P. (2020). Gigantol targets MYC for ubiquitin-proteasomal degradation and suppresses lung cancer cell growth. Cancer Genomics Proteomics 17, 781–793. doi:10.21873/cgp.20232
Morigny, P., Kaltenecker, D., Zuber, J., Machado, J., Mehr, L., Tsokanos, F. F., et al. (2021). Association of circulating PLA2G7 levels with cancer cachexia and assessment of darapladib as a therapy. J. Cachexia Sarcopenia Muscle 12, 1333–1351. doi:10.1002/jcsm.12758
Nogales, C., Mamdouh, Z. M., List, M., Kiel, C., Casas, A. I., and Schmidt, H. (2022). Network pharmacology: Curing causal mechanisms instead of treating symptoms. Trends Pharmacol. Sci. 43, 136–150. doi:10.1016/j.tips.2021.11.004
Piao, J., Zhu, L., Sun, J., Li, N., Dong, B., Yang, Y., et al. (2019). High expression of CDK1 and BUB1 predicts poor prognosis of pancreatic ductal adenocarcinoma. Gene 701, 15–22. doi:10.1016/j.gene.2019.02.081
Poulia, K. A., Sarantis, P., Antoniadou, D., Koustas, E., Papadimitropoulou, A., Papavassiliou, A. G., et al. (2020). Pancreatic cancer and cachexia-metabolic mechanisms and novel insights. Nutrients 12, 1543. doi:10.3390/nu12061543
Putri, H. E., Nutho, B., Rungrotmongkol, T., Sritularak, B., Vinayanuwattikun, C., and Chanvorachote, P. (2021). Bibenzyl analogue DS-1 inhibits MDM2-mediated p53 degradation and sensitizes apoptosis in lung cancer cells. Phytomedicine 85, 153534. doi:10.1016/j.phymed.2021.153534
Ravindranath, P. A., Forli, S., Goodsell, D. S., Olson, A. J., and Sanner, M. F. (2015). AutoDockFR: Advances in protein-ligand docking with explicitly specified binding site flexibility. PLoS Comput. Biol. 11, e1004586. doi:10.1371/journal.pcbi.1004586
Robinson, M. D., McCarthy, D. J., and Smyth, G. K. (2010). edgeR: a Bioconductor package for differential expression analysis of digital gene expression data. Bioinformatics 26, 139–140. doi:10.1093/bioinformatics/btp616
Shannon, P., Markiel, A., Ozier, O., Baliga, N. S., Wang, J. T., Ramage, D., et al. (2003). Cytoscape: A software environment for integrated models of biomolecular interaction networks. Genome Res. 13, 2498–2504. doi:10.1101/gr.1239303
Szklarczyk, D., Gable, A. L., Lyon, D., Junge, A., Wyder, S., Huerta-Cepas, J., et al. (2019). STRING v11: Protein-protein association networks with increased coverage, supporting functional discovery in genome-wide experimental datasets. Nucleic Acids Res. 47, D607–D613. doi:10.1093/nar/gky1131
Tang, J., and Aittokallio, T. (2014). Network pharmacology strategies toward multi-target anticancer therapies: From computational models to experimental design principles. Curr. Pharm. Des. 20, 23–36. doi:10.2174/13816128113199990470
Tang, Z., Kang, B., Li, C., Chen, T., and Zhang, Z. (2019). GEPIA2: An enhanced web server for large-scale expression profiling and interactive analysis. Nucleic Acids Res. 47, W556–W560. doi:10.1093/nar/gkz430
Trott, O., and Olson, A. J. (2010). AutoDock Vina: Improving the speed and accuracy of docking with a new scoring function, efficient optimization, and multithreading. J. Comput. Chem. 31, 455–461. doi:10.1002/jcc.21334
Vaughan, V. C., Martin, P., and Lewandowski, P. A. (2013). Cancer cachexia: Impact, mechanisms and emerging treatments. J. Cachexia Sarcopenia Muscle 4, 95–109. doi:10.1007/s13539-012-0087-1
Vivian, J., Rao, A. A., Nothaft, F. A., Ketchum, C., Armstrong, J., Novak, A., et al. (2017). Toil enables reproducible, open source, big biomedical data analyses. Nat. Biotechnol. 35, 314–316. doi:10.1038/nbt.3772
Von Hoff, D. D., Ervin, T., Arena, F. P., Chiorean, E. G., Infante, J., Moore, M., et al. (2013). Increased survival in pancreatic cancer with nab-paclitaxel plus gemcitabine. N. Engl. J. Med. 369, 1691–1703. doi:10.1056/NEJMoa1304369
Wang, H., Lu, L., Liang, X., and Chen, Y. (2022). Identification of prognostic genes in the pancreatic adenocarcinoma immune microenvironment by integrated bioinformatics analysis. Cancer Immunol. Immunother. 71, 1757–1769. doi:10.1007/s00262-021-03110-3
Wang, X., Shen, Y., Wang, S., Li, S., Zhang, W., Liu, X., et al. (2017). PharmMapper 2017 update: A web server for potential drug target identification with a comprehensive target pharmacophore database. Nucleic Acids Res. 45, W356–W360. doi:10.1093/nar/gkx374
Xu, B., Cheng, Q., and So, W. K. W. (2021). Review of the effects and safety of traditional Chinese medicine in the treatment of cancer cachexia. Asia Pac J. Oncol. Nurs. 8, 471–486. doi:10.4103/apjon.apjon-2130
Yang, S., He, P., Wang, J., Schetter, A., Tang, W., Funamizu, N., et al. (2016). A novel MIF signaling pathway drives the malignant character of pancreatic cancer by targeting NR3C2. Cancer Res. 76, 3838–3850. doi:10.1158/0008-5472.CAN-15-2841
Yi, N., Zhao, X., Ji, J., Xu, M., Jiao, Y., Qian, T., et al. (2020). Serum galectin-3 as a biomarker for screening, early diagnosis, prognosis and therapeutic effect evaluation of pancreatic cancer. J. Cell. Mol. Med. 24, 11583–11591. doi:10.1111/jcmm.15775
Yu, S., Wang, Z., Su, Z., Song, J., Zhou, L., Sun, Q., et al. (2018). Gigantol inhibits Wnt/β-catenin signaling and exhibits anticancer activity in breast cancer cells. BMC Complement. Altern. Med. 18, 59. doi:10.1186/s12906-018-2108-x
Zhang, R., Zhu, X., Bai, H., and Ning, K. (2019). Network pharmacology databases for traditional Chinese medicine: Review and assessment. Front. Pharmacol. 10, 123. doi:10.3389/fphar.2019.00123
Zhang, Z., He, S., Wang, P., and Zhou, Y. (2022). The efficacy and safety of gemcitabine-based combination therapy vs. gemcitabine alone for the treatment of advanced pancreatic cancer: A systematic review and meta-analysis. J. Gastrointest. Oncol. 13, 1967–1980. doi:10.21037/jgo-22-624
Zhao, G. Y., Deng, B. W., Zhang, C. Y., Cui, Y. D., Bi, J. Y., and Zhang, G. G. (2018). New phenanthrene and 9, 10-dihydrophenanthrene derivatives from the stems of Dendrobium officinale with their cytotoxic activities. J. Nat. Med. 72, 246–251. doi:10.1007/s11418-017-1141-2
Zhao, X., Liu, Z., Ren, Z., Wang, H., Wang, Z., Zhai, J., et al. (2020). Triptolide inhibits pancreatic cancer cell proliferation and migration via down-regulating PLAU based on network pharmacology of Tripterygium wilfordii Hook F. Eur. J. Pharmacol. 880, 173225. doi:10.1016/j.ejphar.2020.173225
Zhou, Y., Zhou, B., Pache, L., Chang, M., Khodabakhshi, A. H., Tanaseichuk, O., et al. (2019). Metascape provides a biologist-oriented resource for the analysis of systems-level datasets. Nat. Commun. 10, 1523. doi:10.1038/s41467-019-09234-6
Keywords: pancreatic ductal adenocarcinoma, Dendrobium nobile, Dendrobin A, network pharmacology, bioinformatics, PLAU
Citation: Xu X, Yu Y, Yang L, Wang B, Fan Y, Ruan B, Zhang X, Dai H, Mei W, Jie W and Zheng S (2023) Integrated analysis of Dendrobium nobile extract Dendrobin A against pancreatic ductal adenocarcinoma based on network pharmacology, bioinformatics, and validation experiments. Front. Pharmacol. 14:1079539. doi: 10.3389/fphar.2023.1079539
Received: 25 October 2022; Accepted: 20 February 2023;
Published: 01 March 2023.
Edited by:
Liang Feng, China Pharmaceutical University, ChinaReviewed by:
Weikun Qian, The First Affiliated Hospital of Xi’an Jiaotong University, ChinaShunda Wang, Chinese Academy of Medical Sciences and Peking Union Medical College, China
Copyright © 2023 Xu, Yu, Yang, Wang, Fan, Ruan, Zhang, Dai, Mei, Jie and Zheng. This is an open-access article distributed under the terms of the Creative Commons Attribution License (CC BY). The use, distribution or reproduction in other forums is permitted, provided the original author(s) and the copyright owner(s) are credited and that the original publication in this journal is cited, in accordance with accepted academic practice. No use, distribution or reproduction is permitted which does not comply with these terms.
*Correspondence: Wenli Mei, bWVpd2VubGlAaXRiYi5vcmcuY24=; Wei Jie, d2VpX2ppZUBoYWlubWMuZWR1LmNu; Shaojiang Zheng, emhlbmdzajIwMDhAMTYzLmNvbQ==
†These authors have contributed equally to this work