- 1Department of Molecular Pharmacology and Experimental Therapeutics, Mayo Clinic, Rochester, MN, United States
- 2Department of Molecular and Integrative Physiology, University of Illinois at Urbana-Champaign, Urbana, IL, United States
- 3Department of Psychiatry and Behavioral Sciences, Department of Medicine, Duke Institute for Brain Sciences, Duke University, Durham, NC, United States
- 4Department of Psychiatry and Psychology, Mayo Clinic, Rochester, MN, United States
- 5Department of Quantitative Health Sciences, Mayo Clinic, Rochester, MN, United States
- 6Peter O’Donnell Jr. Brain Institute and the Department of Psychiatry at the University of Texas Southwestern Medical Center, Dallas, TX, United States
- 7Department Population and Data Sciences, University of Texas Southwestern Medical Center, Dallas, TX, United States
- 8Department of Internal Medicine, Division of Gastroenterology and Hepatology, Mayo Clinic, Rochester, MN, United States
- 9Department of Psychiatry and Psychology, Mayo Clinic, Jacksonville, FL, United States
Background: Individuals with major depressive disorder (MDD) and a lifetime history of attempted suicide demonstrate lower antidepressant response rates than those without a prior suicide attempt. Identifying biomarkers of antidepressant response and lifetime history of attempted suicide may help augment pharmacotherapy selection and improve the objectivity of suicide risk assessments. Towards this goal, this study sought to use network science approaches to establish a multi-omics (genomic and metabolomic) signature of antidepressant response and lifetime history of attempted suicide in adults with MDD.
Methods: Single nucleotide variants (SNVs) which associated with suicide attempt(s) in the literature were identified and then integrated with a) p180-assayed metabolites collected prior to antidepressant pharmacotherapy and b) a binary measure of antidepressant response at 8 weeks of treatment using penalized regression-based networks in 245 ‘Pharmacogenomics Research Network Antidepressant Medication Study (PGRN-AMPS)’ and 103 ‘Combining Medications to Enhance Depression Outcomes (CO-MED)’ patients with major depressive disorder. This approach enabled characterization and comparison of biological profiles and associated antidepressant treatment outcomes of those with (N = 46) and without (N = 302) a self-reported lifetime history of suicide attempt.
Results: 351 SNVs were associated with suicide attempt(s) in the literature. Intronic SNVs in the circadian genes CLOCK and ARNTL (encoding the CLOCK:BMAL1 heterodimer) were amongst the top network analysis features to differentiate patients with and without a prior suicide attempt. CLOCK and ARNTL differed in their correlations with plasma phosphatidylcholines, kynurenine, amino acids, and carnitines between groups. CLOCK and ARNTL-associated phosphatidylcholines showed a positive correlation with antidepressant response in individuals without a prior suicide attempt which was not observed in the group with a prior suicide attempt.
Conclusion: Results provide evidence for a disturbance between CLOCK:BMAL1 circadian processes and circulating phosphatidylcholines, kynurenine, amino acids, and carnitines in individuals with MDD who have attempted suicide. This disturbance may provide mechanistic insights for differential antidepressant pharmacotherapy outcomes between patients with MDD with versus without a lifetime history of attempted suicide. Future investigations of CLOCK:BMAL1 metabolic regulation in the context of suicide attempts may help move towards biologically-augmented pharmacotherapy selection and stratification of suicide risk for subgroups of patients with MDD and a lifetime history of attempted suicide.
Introduction
Annually, 700,000 people die by suicide worldwide, and there are 10–20 times as many non-fatal suicide attempts (Hannah et al., 2015; WHO, 2021). Patients with major depressive disorder (MDD) and prior suicide attempt(s) demonstrate lower antidepressant remission and response rates and increased antidepressant intolerance compared to those with no history of suicide attempt (Perroud et al., 2010; Kim et al., 2011). Furthermore, evaluating suicidal patients is challenging, as standard clinical risk assessments are inherently subjective (Isometsa et al., 1995; Mann et al., 2006; Smith et al., 2013). Quantitative biomarkers that identify individuals at risk for suicide attempts would assist in personalized assessment and monitoring to a) move towards pharmacotherapy individualization for subgroups of patients with MDD and a history of suicide attempt, and b) hasten referrals to suicide prevention programs.
Biological risk factors for suicide attempts include alterations in stress-response, neuronal plasticity, serotonergic signaling, lipids, and inflammatory markers such as C-reactive protein (CRP) (De Berardis et al., 2008; Oquendo et al., 2014; Sudol and Mann, 2017; De Berardis et al., 2020). However, there are currently no biomarkers (laboratory or imaging) that are sufficiently valid for routine clinical stratification of suicide risk (Ryan and Oquendo, 2020). Enhanced understanding of the interplay between biomarkers for lifetime suicide attempt(s) and biomarkers for outcomes to antidepressant pharmacotherapy represents a first step towards personalized MDD care for individuals with a lifetime history of suicide attempts.
The biological factors which contribute to a) suicide risk and b) antidepressant treatment outcomes likely comprise multiple pathological processes interacting together as a network (Kouter and Videtic Paska, 2021). Integrative omics methods (i.e., genomics, metabolomics, transcriptomics, epigenomics), therefore, are novel and promising approaches for elucidating important molecular mechanisms underlying suicidality and other complex behavioral phenotypes (Barabasi et al., 2011; Bhak et al., 2019; Joyce et al., 2021; Liu et al., 2021; Grant et al., 2022). Integrating genomics and metabolomics has been particularly informative for identifying novel biomarkers of MDD risk and antidepressant response (Gupta et al., 2016; Athreya et al., 2018; Liu et al., 2018; Athreya et al., 2019; Liu et al., 2020). Together, the genome and metabolome capture complimentary information of heritable and environmentally driven suicide attempt risk factors. The heritability of suicide attempts is high, estimated at 17%–55% (Mullins et al., 2022), and the metabolome shows long-term alterations following traumatic events, (e.g., suicide attempts) (Bernini et al., 2009; Ghini et al., 2015). The current study, therefore, used a partial-least squares regression-based network science approach to integrate genomics, metabolomics, lifetime suicide attempt history, and treatment outcomes to a) understand if genetically driven metabolomic differences exist in individuals with a lifetime history of attempted suicide, and b) assess if such biological differences may have potential implications for antidepressant treatment outcomes (Figure 1 for conceptual overview).
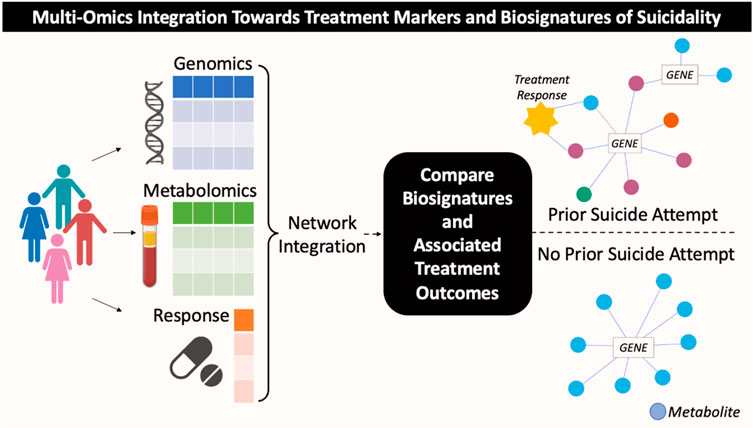
FIGURE 1. Conceptual overview of multi-omics integration analysis towards identification of candidate treatment markers for subgroups of patients with MDD based on history of suicide attempt.
This work hypothesized that biological signatures for suicide attempters within a sample of patients with MDD could be developed by integrating metabolomics and genomics data. Integration of -omic data with treatment outcomes demonstrates that the top metabolomic and genomic features to differentiate MDD patients with and without a lifetime history of attempted suicide correlate with antidepressant treatment response. The findings of this work would generate implications for potential therapeutic utility for the biomarkers which distinguish patients with MDD with a lifetime history of suicide attempts.
Materials and methods
Data sources
This was a cross-sectional secondary analysis of clinical and blood biomarker data from adults with MDD who participated in the Pharmacogenomics Research Network Antidepressant Medication Study (PGRN-AMPS) and Combining Medications to Enhance Depression Outcomes (CO-MED) studies. Subjects were included if they had metabolomics, genomics, and a binary clinical measure (yes/no) of prior suicide attempt (Table 1; see Supplementary Figure S1 for sample inclusion). Both the PGRN-AMPS and CO-MED trials have been previously described (Rush et al., 2011; Ji et al., 2013). Briefly, the Mayo Clinic PGRN-AMPS trial (NCT00613470) enrolled 927 outpatients with nonpsychotic MDD who scored ≥14 on the 17-item Hamilton Depression Rating Scale (HAMD-17 (Hamilton, 1967)) at enrollment; of these 245 participants had metabolomics, genomics, and a self-reported lifetime history of at least one attempted suicide. The CO-MED trial (NCT00590863), conducted at 15 sites throughout the United States, enrolled 665 outpatients who met DSM-IV-TR criteria for recurrent or chronic (current episode lasting ≥2 years) nonpsychotic MDD and scored ≥16 on the HAMD-17 at study entry; of these 103 participants had metabolomics, genomics, and a self-reported lifetime history of at least one attempted suicide. Thus, for these analyses, a total of 348 participants were included. All PGRN-AMPS and CO-MED participants provided written informed consent and each study was approved by their respective Institutional Review Boards.
Measures and outcomes
Self-reported lifetime history of suicide attempts (yes/no) was collected via a baseline case report form in PGRN-AMPS (“Has patient ever attempted suicide?“) and via the SAD PERSONS scale (Patterson et al., 1983) in CO-MED. Depression severity was assessed with the 16-item Quick Inventory of Depressive Symptomatology—Clinician-Rated (QIDS-C) (Trivedi et al., 2004). The QIDS-C tallies responses to 16 questions to derive a total score that ranges from 0 (no depression) to 27 (very severe depression). These 16 questions cover the nine diagnostic and statistical manual (DSM)-IV MDD symptom criterion domains: 1) sad mood; 2) concentration; 3) self-criticism; 4) suicidal ideation; 5) interest; 6) energy/fatigue; 7) sleep disturbance (initial, middle, and late insomnia or hypersomnia); 8) decrease/increase in appetite/weight; and 9) psychomotor agitation/retardation. Participants were classified as responders or non-responders at 8 weeks after initiating treatment with citalopram, escitalopram, bupropion plus citalopram, or venlafaxine plus mirtazapine. Responders were defined as demonstrating ≥50% reduction in total QIDS-C score at (Table 1).
Genomics
PGRN-AMPS patients were genotyped with Illumina human 610-Quad BeadChips (Illumina, San Diego, CA, United States), with quality control and imputation as previously reported (Gupta et al., 2016). CO-MED patients were genotyped, as previously published, with Illumina Quad, Human Omni 2.5 bead chips (Gadad et al., 2018). Imputation was performed using the Michigan Imputation Server (Das et al., 2016). All CO-MED single nucleotide variants (SNVs) included in this analysis met imputation R2 and call rates of >0.95, while genotyped SNVs met LooRsq >0.95.
Candidate single nucleotide variant search strategy
Candidate SNVs that best differentiated individuals with and without a prior suicide attempt were identified by a PubMed Search and a GWAS Catalog (Buniello et al., 2019) Search (Illustrated in Figure 2). These complimentary strategies enabled the identification of SNVs which associated with suicide attempts in both targeted (e.g., candidate gene) and unbiased (e.g., genome-wide association study (GWAS)) studies. These searches were conducted agnostic of clinical diagnoses, as the heritability of suicidal behavior is transdiagnostic (Oquendo et al., 2014; Mann and Rizk, 2020). The PubMed Search, conducted on 07/09/2021, included the following criteria: ((SNP) OR (SNPs) OR (single nucleotide polymorphism) OR (single nucleotide polymorphisms) OR (SNV) OR (SNVs) OR (single nucleotide variant) OR (single nucleotide variants)) AND ((suicide attempt) OR (suicide attempts)). SNVs which reached significance according to their respective study definitions were retained for evaluation in the PGRN-AMPS and CO-MED cohorts. The GWAS Catalog search was conducted on 07/20/2021 using the gwasrappid R package (Magno and Maia, 2020), which enabled efficient querying and downloading of SNVs associated with suicide attempts. GWAS Catalog SNVs meeting the catalogue’s definition of suggestive significance (p < 1.0 × 10−5) were retained for further evaluation. Studies were reviewed by two independent raters. Studies and SNVs were excluded based upon criteria outlined in Figure 2. SNVs were annotated with genomic location and nearest gene according to the National Center for Biotechnology Information (NCBI) Reference Sequence Database (RefSeq) using haploR (Zhbannikov et al., 2017), implemented in R 4.0.3 (R Foundation for Statistical Computing, 2020) using RStudio version 1.3 (RStudio, 2020).
Single nucleotide variant associations with suicide attempt history
Candidate SNVs meeting the quality control criteria (outlined in the Genomics section) and a minor allele frequency threshold of 5% were assessed for association with lifetime history of attempted suicide in the combined PGRN-AMPS and CO-MED cohorts using chi-squared tests with two degrees of freedom. As this was intended as a discovery step to identify SNVs which may contribute to multi-omics differentiation of patients by lifetime history of suicide attempt, a nominal p-value of ≤0.05 was considered significant.
Metabolomics
In both the PGRN-AMPS and CO-MED cohorts, plasma metabolites were assayed with the AbsoluteIDQ p180 platform (BIOCRATES Life Science AG, Innsbruck, Austria) (Sciences, 2010). This targeted assay detects metabolites from five analyte classes (acylcarnitines, amino acids, biogenic amines, glycerophospholipids and sphingolipids) by triple quadrupole tandem mass spectrometry operated in Multiple Reaction Monitoring mode. Metabolomic profiling and quality control were conducted as previously published (Czysz et al., 2019; Ahmed et al., 2020; MahmoudianDehkordi et al., 2021), and metabolites with ≥10% missingness were excluded. 153 metabolites met quality control criteria in both the PGRN-AMPS and CO-MED datasets (Supplementary Table S1). The p180 platform has demonstrated utility in characterizing related psychiatric phenotypes, including MDD and antidepressant response (Gupta et al., 2016; Neavin et al., 2016; Athreya et al., 2018; Bhattacharyya et al., 2019; Czysz et al., 2019; Ahmed et al., 2020; Joyce et al., 2021; MahmoudianDehkordi et al., 2021; Brydges et al., 2022), schizophrenia (Parksepp et al., 2020), and psychosis (Kriisa et al., 2017; Balotsev et al., 2019), but has not yet been investigated in the context of suicidality. Therefore, all metabolites meeting quality control criteria were included in the current integration analysis. This enabled the assessment of promising analyte classes in the characterization of suicide attempt, with the limitation that the p180 platform does not capture other metabolites of potential etiologic significance for neuropsychiatric disorders or drug response [i.e., dopamine, γ-aminobutyric acid (GABA)].
Network science for the integration of multi-omics and antidepressant outcomes
Integrative network analysis was performed to a) characterize the multi-omics profiles of patients with and without a lifetime history of suicide attempt, b) identify SNVs, metabolites, and associated treatment outcomes which best differentiate the profiles of patients with and without a lifetime history of suicide attempt, and c) detect sub-networks (i.e., communities) of tightly correlated SNVs, metabolites, and treatment outcomes to elucidate novel biological associations which may reflect biological mechanisms of attempted suicide. Subnetworks are hypothesized to consist of functionally related biomolecules (Barabasi et al., 2011; Lichtblau et al., 2017).
This network science approach, based on partial least squares regression, represents a multi-variate dimensionality reduction tool that is becoming increasingly popular in integrative omics analyses (Ruiz-Perez et al., 2020). Input features included: a) SNVs associated with prior suicide attempt in the PGRN-AMPS and CO-MED cohorts (p ≤ 0.05), b) all 153 metabolites meeting quality control criteria, and c) treatment response measured at 8 weeks. The resulting networks comprise SNVs, metabolites, and associated outcomes which are significantly correlated (|r|>0.1, p < 0.05) in patients with and without a lifetime history of suicide attempt. The derived networks were compared by calculating the delta centrality (change in eigenvector centrality) for each SNV and metabolite across networks. Delta centrality ranges from 0 to 1, with 1 indicating significant differences between patients with and without a history of suicide attempt in the number and quality of network correlations (Lichtblau et al., 2017).
Prior to integration analysis, the sole missing datapoint (one taurine value) was imputed with K-nearest neighbor’s imputation. Metabolites were transformed using Yeo-Johnson transformation, centered at zero, and scaled to unit variance. Preprocessing was performed using the tidymodels package version 0.1.2 (Tidymodels, 2020) and integration analysis was performed using xMWAS (Uppal et al., 2018), implemented in R 4.0.338 using RStudio version 1.339.
Resource availability
The PGRN-AMPS data has been deposited in dbGaP (study accession phs000670. v1. p.1). Funding for the CO-MED project was exclusively provided through institutional resources. Data supporting the conclusions of this article will be made available by contacting the authors, without undue reservation.
Results
Participant characteristics
Participants were predominantly female (66%) and Caucasian (92%). Of the 348 participants included, 46 (13%) had a lifetime history of a suicide attempt. Individuals who reported a prior suicide attempt were more likely to have a depression onset prior to age 18 (65% vs. 37%; p < 0.05) and had higher QIDS-C scores (16.5 vs.14.9) compared with individuals without a lifetime history of attempted suicide. Table 1 provides the demographics and clinical characteristics of the sample.
Candidate single nucleotide variant search
The PubMed Search for SNVs associated suicide attempts yielded 227 articles, and 113 were retained following title, abstract, and full text screening. The GWAS Catalog Search yielded 31 articles, 13 of which were retained following identical screening (Figure 2). In total, 351 unique SNVs were associated with suicide attempts in a transdiagnostic manner in the literature, with some replicating in multiple studies (for a complete table of SNVs, SNV annotations, and studies see Supplementary Table S2). SNVs which associated with suicide attempt(s) in the GWAS catalog most frequently localized outside the open reading frame (N = 110 SNVs), while SNVs derived from the PubMed search most frequently localized to intronic genomic regions (N = 125 SNVs) (Table 2). The GWAS catalog studies most frequently reported associations of RUNX1 with suicide attempt(s), while the PubMed studies most frequently reported associations with FKBP5 (Table 2). The initial literature associations of these SNVs and suicide attempts were discovered in cohorts with a variety of psychiatric diagnoses: bipolar disorders, substance dependence, childhood onset mood disorders, MDD, eating disorders, schizophrenia, post-traumatic stress disorder, mixed cohorts, and no specified diagnosis (Table 2).
Single nucleotide variant associations with suicide attempt history
Genotypes for 252 of the 351 candidate SNVs were obtained from PGRN-AMPS and CO-MED patients after quality control and applying a minor allele frequency threshold of 5% (Figure 2 for SNV exclusion). Chi-square tests were performed to associate SNVs with suicide attempt history in these depressed adults, with twelve SNVs meeting nominal significance (p ≤ 0.05) (Supplementary Table S3 for complete results). After pruning for linkage disequilibrium, eight significant SNVs from distinct genomic regions remained (Table 3). The initial literature associations of these SNVs and suicide attempts were discovered in cohorts with a variety of psychiatric diagnoses (Table 3) (Fudalej et al., 2010; Roy et al., 2010; Strauss et al., 2012; Pawlak et al., 2015; Penas-Lledo et al., 2015; Acikel et al., 2020; Russell et al., 2020).
Network science for the integration of multi-omics and antidepressant outcomes
Integrative network analysis was performed to characterize and compare the multi-omics profiles of patients with (Figure 3A) and without (Figure 3B) a lifetime history of attempted suicide. The analysis integrates measures and detects subnetworks of biological and clinical features (e.g., SNVs, metabolites, treatment outcomes) which are highly correlated within their subnetwork, but sparsely correlated to the rest of the network (Girvan and Newman, 2002; Uppal et al., 2018).
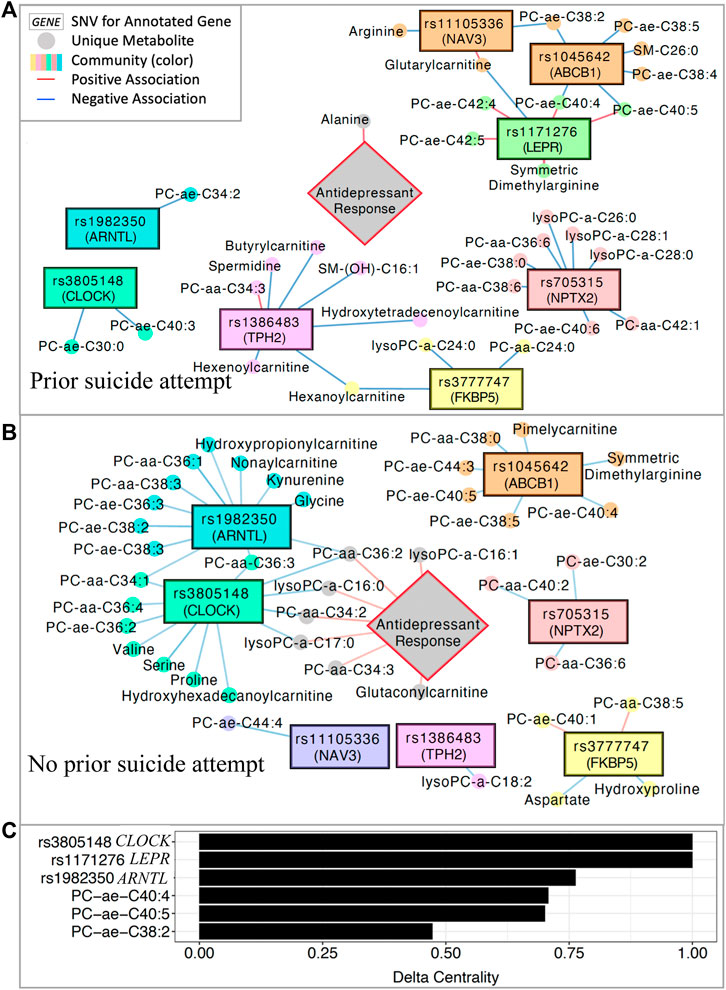
FIGURE 3. Multi-omics integration network. (A) Significantly associated SNVs and metabolites in patients with a history of suicide attempt and (B) patients with no history of suicide attempt. (C) Delta centrality scores for the five variables with the highest delta centrality values. Delta centrality is defined as the change in eigenvector centrality across networks (lifetime history of suicide attempt vs. no history). Delta centrality ranges from 0–1, with 1 indicating the greatest differences in number and quality of connections across networks. PC: phosphatidylcholine; SM: sphingomyelin.
After inputting all 153 metabolites, the eight significant SNVs, and treatment outcomes into xMWAS (Uppal et al., 2018), eight subnetworks of correlated SNVs and metabolites were detected in patients with and without a lifetime history of attempted suicide (Figures 3A,B). Delta centrality was calculated for each feature, with higher values representing larger differences across networks (history suicide attempt vs. no history of suicide attempt) in the strength and quality of network connections. The six SNVs and metabolites with the highest delta centrality are shown in Figure 3C. These included three intronic SNVs with high (>0.75) delta centrality in CLOCK, LEPR, and ARNTL (rs3805148, rs1171276, rs1982350, respectively).
In patients with a previous suicide attempt, the ARNTL and CLOCK SNVs were significantly correlated (p < 0.05) with only one and two phosphatidylcholines, respectively (Figure 3A). In such patients, antidepressant response correlated positively with alanine concentrations. Additionally, the LEPR SNV was positively correlated with long-chain phosphatidylcholines, symmetric dimethylarginine, and glutarylcarnitine in patients with a history of suicide attempt.
In contrast, in patients with no lifetime history of attempted suicide, the ARNTL and CLOCK SNVs shared significant negative correlations (p < 0.05) with three phosphatidylcholine metabolites (Figure 3B). Individually, the ARTNL and CLOCK SNVs were also negatively correlated (p < 0.05) with many additional phosphatidylcholines, kynurenine, amino acids (serine, proline, valine, glycine), and carnitines. Four phosphatidylcholine metabolites associated with ARNTL and CLOCK SNVs are positively correlated with treatment response in individuals with no prior suicide attempt. The LEPR SNV was not correlated with any metabolites in patients without a history of suicide attempt. Pearson correlation coefficients for all SNVs and metabolites represented in these networks are tabulated in Supplementary Table S4.
Discussion
This work using network science approaches identified integrative genomic-metabolomic signatures of antidepressant response and lifetime history of attempted suicide in adults with MDD. Importantly, the subnetworks of biomeasures which most differentiated individuals by their lifetime history of suicide attempt (CLOCK, ARNTL subnetworks) correlated with response to antidepressant pharmacotherapies exclusively in individuals without a prior suicide attempt. Future investigations of CLOCK:BMAL1 metabolic regulation in the context of suicide attempts may help move towards biologically-augmented pharmacotherapy selection and stratification of suicide risk and for patients with MDD based upon their lifetime history of attempted suicide.
The network science approach utilized in this work is a multivariate analysis that provides an analytical prioritization of biomarker subnetworks for future mechanistic investigation (Barabasi et al., 2011; Joyce et al., 2021). SNVs in the circadian genes CLOCK and ARNTL and their associated plasma metabolites discriminate individuals with and without a lifetime history of attempted suicide, despite all patients having the same diagnosis of MDD. Circadian genes including CLOCK and ARNTL have been implicated in suicidality previously, yet the underlying molecular mechanisms, including the downstream effects on metabolism, and the treatment implications are incompletely characterized (Benedetti et al., 2015; Levey et al., 2016).
The CLOCK and ARNTL genes encode for the CLOCK (circadian locomotor output cycles kaput) and BMAL1 (brain and muscle aryl hydrocarbon nuclear translocator (ARNT)-like 1) proteins, respectively. These are core components of the molecular circadian mechanism, which comprises the autonomous clocks of nearly every cell in the body and the “master circadian pacemaker” of the hypothalamic suprachiasmatic nucleus (Mohawk et al., 2012; Rijo-Ferreira and Takahashi, 2019). The sleep/wake cycle is perhaps the most evident function of circadian rhythms, but many additional physiological processes are regulated by circadian rhythms, including feeding, temperature, secretion of hormones, metabolism of drugs and xenobiotics, glucose homeostasis, and cell cycle progression (Rijo-Ferreira and Takahashi, 2019). The associations derived in this work suggest underlying biological differences between patients with and without a prior suicide attempt in the mechanistic processes which connect circadian rhythm variation (e.g., SNVs in CLOCK, ARNTL) with downstream associated metabolites (e.g., phosphatidylcholines, kynurenine, and more). Altered transcription regulation may represent one such mechanism, as these SNVs are expression quantitative trait loci (eQTLs) which alter messenger RNA (mRNA) levels (p < 0.001) of the ARNTL and CLOCK transcripts in multiple peripheral tissues according to the Genotype-Tissue Expression (GTEx) database (Consortium, 2020). While genomic, metabolomic, and clinical data was available for analysis in this study, the epigenomic, transcriptomic, proteomic, and environmental factors which may mediate the derived genomic and metabolomic associations must be examined in future work to mechanistically understand these correlations (Figure 4).
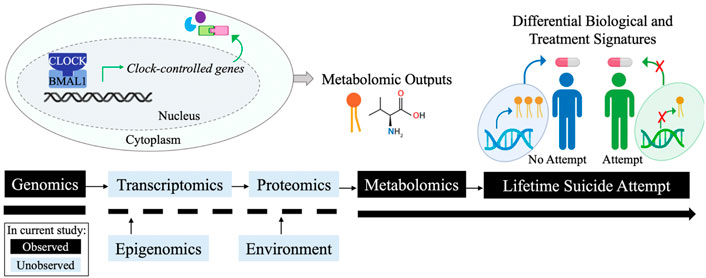
FIGURE 4. Conceptual summary. Simplified circadian rhythm loop. Tuning of such loops occurs by multiple mechanisms (blue boxes). Black boxes denote available data and blue boxes denote unavailable data in the current analysis. Figure created with BioRender.com.
Phosphatidylcholines were network correlates of ARNTL and CLOCK SNVs that differed between individuals with and without a lifetime history of attempted suicide. Phosphatidylcholines are a subclass of phospholipid and the main component of mammalian membranes and lipoproteins (van der Veen et al., 2017). Phosphatidylcholines are implicated in MDD risk (Liu et al., 2015; Knowles et al., 2017; Walther et al., 2018; Pu et al., 2020) and response to antidepressant treatments (Joyce et al., 2021; MahmoudianDehkordi et al., 2021), but the relationship between suicidality and phosphatidylcholines is yet to be understood. However, functionally related lipids, including cholesterol, have been implicated in suicidal behavior (Muldoon et al., 1990; Neaton et al., 1992; Salter, 1992; Morgan et al., 1993; Maes et al., 1997; Yang et al., 2003; Alghamdi et al., 2018). In some trials, the benefits of statins, which lower cholesterol in individuals with high lipid levels, have been offset by increased suicidality (Knowles et al., 2017). These findings encourage additional evaluation of the lipidome, including phosphatidylcholines, in suicidality and the risk of attempted suicide.
Kynurenine is a biogenic amine produced via tryptophan metabolism (Peters, 1991) which has been separately associated with a) circadian disturbance (e.g., sleep disruption) (Bhat et al., 2020), b) suicidal behavior and ideation (Rujescu et al., 2003; Lin and Tsai, 2004; Pitchot et al., 2005; Lopez et al., 2007; Malone et al., 2007; Yeh et al., 2014; Setoyama et al., 2016), and c) antidepressant response (Sun et al., 2020). Suicidal patients exhibit dysregulation along the tryptophan metabolism pathway at the level of enzymes (e.g., tryptophan hydroxylase I, II (TPH1, TPH2)) (Rujescu et al., 2003; Lopez et al., 2007), receptors (5-Hydroxytryptamine Receptors 5HT1A, 5-HT2A) (Pitchot et al., 2005; Malone et al., 2007), and transporters (serotonin transporter (5-HTTLPR)) (Lin and Tsai, 2004; Yeh et al., 2014). Sleep deprivation activates enzymatic degradation of tryptophan along the kynurenine pathway, resulting in decreased production of melatonin and neuroprotective metabolites (e.g., kynurenic acid) and increased production of inflammatory and neurotoxic metabolites (e.g., 3-hydroxykynurenine, quinolinic acid) (Bhat et al., 2020). The network analysis unifies these observations and identifies kynurenine as part of the ARNTL subnetwork of circadian SNVs associating with antidepressant response exclusively in MDD patients with a lifetime history of suicide attempt and not in those without a lifetime history of suicide attempt. This encourages future mechanistic investigation of the negative correlation between the circadian variant rs1982350 (in ARNTL), the subnetwork’s additional metabolites (e.g., phosphatidylcholines), and kynurenine in the context of antidepressant response and lifetime history of suicide attempts.
The results of this study also extend beyond circadian regulation of metabolism to identify additional promising biomarkers of suicide attempt. Eight SNVs were included in the network analysis because they associated with suicide attempts both in various psychiatric cohorts in the literature (Fudalej et al., 2010; Roy et al., 2010; Strauss et al., 2012; Pawlak et al., 2015; Penas-Lledo et al., 2015; Acikel et al., 2020; Russell et al., 2020) and in PGRN-AMPS and CO-MED adults with MDD. These SNVs may, therefore, represent promising transdiagnostic markers of suicide attempt, and may be validated across additional psychiatric cohorts in the future. An intronic SNV in LEPR (leptin receptor), which is an eQTL for LEPR in whole blood and lymphoblastoid cells, demonstrated high network delta centrality (delta centrality = 1), showing differential associations with plasma phosphatidylcholines, symmetric dimethylarginine, and hydroxyvalerylcarnitine in patients with and without a lifetime history of attempted suicide. Enhancing our understanding of these associations via future functional experimentation may assist in pharmacogenomic personalization of therapeutics which modulate these systems. While further evidence is needed to assess whether targeting these systems through pharmacotherapy might benefit subgroups of patients with MDD, compounds exist already which can modulate these systems. For example, orexin modulators such as Orexin A, can modulate leptin receptor signaling (Medrano et al., 2018). Orexin modulators have also demonstrated antidepressant and sleep-promoting benefits in patients with major depressive disorder (Recourt et al., 2019).
This study has limitations. Lifetime history of suicide attempt was self-reported, with no independent verification. Information on the severity of the suicide attempt and the number of past such attempts was not available for examination. A larger proportion of patients with a history of suicide attempt had depression onset prior to age 18 compared to those without a history of suicide attempt 65% vs. 37%), which aligns with epidemiological reports (Claassen et al., 2007). The enrollment criteria of the PGRN-AMPS and CO-MED trials indicated that participants had, on average, a moderate to severe MDD presentation (Rush et al., 2006). To evaluate the generalizability of our findings across the spectrum of MDD severity, our study’s biomarker associations must be evaluated in future large cohorts (e.g., treatment-resistant depression, very severe depression). This study’s samples did not have a sex difference between those with and without a lifetime history of suicide attempt, despite epidemiological evidence which suggests that females more frequently attempt suicide than males (Freeman et al., 2017). This may reflect the sample’s moderate depressive severity or additional factors related to study enrollment, indicating replication of biosignatures in additional cohorts is warranted. These analyses were not controlled for age at which the suicide attempt occurred (as this information was not collected) or sex (due to limited males with a lifetime of attempted suicide in these datasets, N = 12). There are known clinical and demographic differences in the suicide attempts of young, middle-aged, and older individuals (e.g., reason for attempt, propensity to re-attempt, occupation, alcohol-co-ingestion, psychiatric history, sex, and hospital discharge outcomes) (Freeman et al., 2017; Kim et al., 2020), but it is unknown whether these factors might influence the derived biosignatures. Future studies should utilize cohort designs to investigate the impact of sex, age, and additional clinical/demographic factors at suicide attempt on genomic-metabolomic biosignatures of suicide attempt. This could confirm whether the current findings generalize across patients with prior suicide attempts. Such future sex-stratified analyses are especially warranted given differential expression of clock genes in adipose tissue of men and women, which may also generalize to additional tissues (e.g., brain) (Gomez-Abellan et al., 2012; Levey et al., 2016; Labonte et al., 2017). The PGRN-AMPS and CO-MED cohorts largely comprise Caucasian individuals, and the SNVs identified via the literature search vary in minor allele frequency across populations (Supplementary Table S5). These network interactions should be replicated in larger cohorts and across ancestries to assess the generalizability of these findings. We lacked data on the latency from the attempted suicide to the plasma metabolomic assay. There is no benchmark for the optimal timeframe to assay metabolomics relative to suicide attempts. The characterization across time of the plasma metabolome following a suicide attempt may be addressed in future longitudinal studies. Metabolites were not collected in a systematic manner (e.g., under fasting conditions, at a uniform time of day), which may confer noise to the analyses, although fasting status may not significantly impact laboratory variability for most metabolites (Townsend et al., 2013). Other psychiatric metabolites, including dopamine and GABA, are not assayed by the p180 platform, and the platform was unable to detect serotonin at the quality control threshold employed. Lipids assayed by current mass spectrometry technology may actually reflect sum signals of all isomeric/isobaric compounds having the same parent and daughter ions (Sciences, 2020). Therefore, future studies should validate the identified lipid metabolites with additional assays.
Future studies may build on this work by examining whether biological variation in the top SNVs and metabolites can be used prospectively to predict suicide attempts. Those predictions may be bolstered by additional risk measures, such as assays of cerebrospinal fluid, brain imaging, and clinical history (Hayashi-Takagi et al., 2014). Neuroimaging studies suggest that complex interactions among ventral and dorsal prefrontal cortex systems produces a framework for suicidal ideation to progress to lethal behaviors (Schmaal et al., 2020). At present, however, distinct neural signatures with imaging are not sufficiently developed for clinical implementation (Mann and Rizk, 2020). Combinations of neuroimaging and peripheral biomarker measurements may yield more accurate biosignatures for suicidal behavior than either modality alone (Calati et al., 2020). Furthermore, given the circadian variants implicated in this work, future studies might assess the utility of peripheral sleep monitors in helping to predict suicidality (e.g., smartwatches). Sleep complaints and disorders are associated with suicide attempts (Bernert and Joiner, 2007; Mirsu-Paun et al., 2017; Benard et al., 2019; Mash et al., 2021). In our sample, QIDS-C measures of insomnia, hypersomnia, and energy/fatiguability did not significantly differentiate individuals with and without a history of suicide attempt in the current study, although sleep-onset insomnia and early-morning insomnia had marginal significance. These seemingly contradictory findings may be attributed to the discrepancy between self-reported sleep quality and sleep quality determined by wrist-worn actigraphy monitors in depressed patients (Orta et al., 2016). This calls for smartwatch sleep monitoring of depressed patients would provide richer, remote, and scalable data towards quantifying sleep patterns and sleep quality in individuals at risk of suicide. Biosignatures developed with such data could ultimately identify subgroups of patients with MDD who may benefit from care provided by specialty providers (e.g., psychiatrists specializing in suicidality). For example, biosignatures could inform on ideal candidates for the investigational circadian-based intervention for managing depressive symptoms, such as Circadian Reinforcement Therapy (CRT) (Dunker Svendsen et al., 2019). The network analysis suggests that biosignatures could also facilitate pharmacogenomic-guided antidepressant prescriptions aimed towards personalizing care.
In conclusion, this study utilized a network science approach to derive molecular signatures of lifetime history of suicide attempt and antidepressant response. The subnetworks of genes and metabolites which best differentiate individuals with MDD by their lifetime history of suicide attempt also associate with antidepressant pharmacotherapy response exclusively in individuals with no lifetime history of suicide attempt. The interplay of genomics, metabolomics, treatment response, and lifetime history of suicide attempts in MDD indicates that multi-modal data integration is a critical step towards the goal of clinically-actionable, objective biomarkers to a) monitor patients at risk for suicide attempts and b) individualize therapy for subgroups of patients with MDD. The results encourage future studies to assay a wider range of -omics measures (e.g., transcriptomics, proteomics, and epigenomics) and to include digital technologies (e.g., smartwatches) to augment understanding of the associations derived in this work. Therefore, this work represents a starting point for additional multi-modal studies aimed at improving our mechanistic understanding of suicidality and antidepressant response in patients with MDD.
Data availability statement
The original contributions presented in the study are included in the article/Supplementary Materials, further inquiries can be directed to the corresponding authors.
Ethics statement
The studies involving human participants were reviewed and approved by the All PGRN-AMPS and CO-MED participants provided written informed consent and each study was approved by Institutional Review Boards at Mayo Clinic and Univ. of Texas Southwestern Medical Center. The patients/participants provided their written informed consent to participate in this study.
Author contributions
CG, AW, PC, and AA designed and conducted the study. MS, RW, LW, JB, and WB were part of the PGRN-AMPS study team, and TC, TM, and MT were part of the CO-MED study team that provided cohort-level insights in the study. RK-D generated metabolomics data of PGRN-AMPS study. KL and RW are genomic medicine experts and contributed to genomic resources at Mayo Clinic Center for Individualized Medicine. Finally, CG, AW, WB, PC, and AA drafted and wrote the manuscript and all authors reviewed and approved the manuscript.
Funding
This work is supported by the Harry C. and Debra A. Stonecipher Predoctoral Fellowship at the Mayo Clinic Graduate School of Biomedical Sciences, Mayo Clinic Summer Undergraduate Research Fellowship, National Science Foundation (NSF) under grant IIS-2041339; National Institutes of Health (NIH) under grants R01 GM028157, R01 AA027486, U19 AG063744, N01 MH90003, R01 AG046171, R01 MH113700, R01 MH124655, U01 AG061359, R01 AG057452, RF1 AG051550 and R01 MH108348; the Hersh Foundation, the Duke Proteomics and Metabolomics Shared Resource, the Blue Gator Foundation, and the Mayo Clinic Center for Individualized Medicine. The CO-MED study received medications at no cost from Forest Pharmaceuticals, GlaxoSmithKline, Organon, and Wyeth Pharmaceuticals. Any opinions, findings, and conclusions or recommendations expressed in this material are those of the author(s) and do not necessarily reflect the views of the NSF or the NIH.
Conflict of interest
LW and RMW are co-founders of and stockholders in OneOme, LLC. WVB research has been supported by the National Institute of Mental Health, the Agency for Healthcare Quality and Research, the National Science Foundation, the Myocarditis Foundation, and the Mayo Foundation for Medical Education and Research. He has contributed chapters to UpToDate concerning the pharmacological treatment of adults with bipolar major depression. PEC has received research grant support from Neuronetics, Inc., NeoSync, Inc., and Pfizer, Inc. He has received grant in-kind (equipment, supply, and genotyping support for research studies) from Assurex Health, Neuronetics, Inc., and MagVenture, Inc. PEC has served as a consultant for Engrail Therapeutics, Myriad Neuroscience, Procter & Gamble, and Sunovion. MHT has provided consulting services to Acadia Pharmaceuticals, Inc., Alkermes Inc, Alto Neuroscience Inc, Axsome Therapeutics, GH Research Limited, GreenLight VitalSign6 Inc, Janssen, Merck Sharp & Dohme Corp., Mind Medicine (MindMed) Inc., Neurocrine Biosciences Inc, Orexo US Inc, Otsuka, SAGE Therapeutics, Signant Health, Titan Pharmaceuticals, Inc. He has received grant/research funding from NIMH, NIDA, NCATS, American Foundation for Suicide Prevention, Patient-Centered Outcomes Research Institute (PCORI), and Blue Cross Blue Shield of Texas. Additionally, he has received editorial compensation from Oxford University Press. TC has provided consulting services to Alkermes, Inc. RK-D is an inventor on a series of patents on use of metabolomics for the diagnosis and treatment of CNS diseases and holds equity in Metabolon Inc., Chymia LLC and PsyProtix. All other authors declared no competing interests for this work.
Publisher’s note
All claims expressed in this article are solely those of the authors and do not necessarily represent those of their affiliated organizations, or those of the publisher, the editors and the reviewers. Any product that may be evaluated in this article, or claim that may be made by its manufacturer, is not guaranteed or endorsed by the publisher.
Supplementary material
The Supplementary Material for this article can be found online at: https://www.frontiersin.org/articles/10.3389/fphar.2022.984383/full#supplementary-material
References
Acikel, S. B., Eroglu, C., Ugras Dikmen, A., and Kurar, E. (2020). The association between leptin receptor polymorphism and suicidal behaviour in depressed adolescents. Int. J. Psychiatry Clin. Pract. 24, 120–126. doi:10.1080/13651501.2019.1711422
Ahmed, A. T., Mahmoudian Dehkordi, S., Bhattacharyya, S., Arnold, M., Liu, D., Neavin, D., et al. (2020). Acylcarnitine metabolomic profiles inform clinically-defined major depressive phenotypes. J. Affect. Disord. 264, 90–97. doi:10.1016/j.jad.2019.11.122
Alghamdi, J., Matou-Nasri, S., Alghamdi, F., Alghamdi, S., Alfadhel, M., and Padmanabhan, S. (2018). Risk of neuropsychiatric adverse effects of lipid-lowering drugs: A mendelian randomization study. Int. J. Neuropsychopharmacol. 21, 1067–1075. doi:10.1093/ijnp/pyy060
Athreya, A., Iyer, R., Neavin, D., Wang, L., Weinshilboum, R., Kaddurah-Daouk, R., et al. (2018). Augmentation of physician assessments with multi-omics enhances predictability of drug response: A case study of major depressive disorder. IEEE Comput. Intell. Mag. 13, 20–31. doi:10.1109/MCI.2018.2840660
Athreya, A. P., Neavin, D., Carrillo-Roa, T., Skime, M., Biernacka, J., Frye, M. A., et al. (2019). Pharmacogenomics-driven prediction of antidepressant treatment outcomes: A machine-learning approach with multi-trial replication. Clin. Pharmacol. Ther. 106, 855–865. doi:10.1002/cpt.1482
Balotsev, R., Haring, L., Koido, K., Leping, V., Kriisa, K., Zilmer, M., et al. (2019). Antipsychotic treatment is associated with inflammatory and metabolic biomarkers alterations among first-episode psychosis patients: A 7-month follow-up study. Early Interv. Psychiatry 13, 101–109. doi:10.1111/eip.12457
Barabasi, A. L., Gulbahce, N., and Loscalzo, J. (2011). Network medicine: A network-based approach to human disease. Nat. Rev. Genet. 12, 56–68. doi:10.1038/nrg2918
Benard, V., Etain, B., Vaiva, G., Boudebesse, C., Yeim, S., Benizri, C., et al. (2019). Sleep and circadian rhythms as possible trait markers of suicide attempt in bipolar disorders: An actigraphy study. J. Affect. Disord. 244, 1–8. doi:10.1016/j.jad.2018.09.054
Benedetti, F., Riccaboni, R., Dallaspezia, S., Locatelli, C., Smeraldi, E., and Colombo, C. (2015). Effects of CLOCK gene variants and early stress on hopelessness and suicide in bipolar depression. Chronobiol. Int. 32, 1156–1161. doi:10.3109/07420528.2015.1060603
Bernert, R. A., and Joiner, T. E. (2007). Sleep disturbances and suicide risk: A review of the literature. Neuropsychiatr. Dis. Treat. 3, 735–743. doi:10.2147/ndt.s1248
Bernini, P., Bertini, I., Luchinat, C., Nepi, S., Saccenti, E., Schafer, H., et al. (2009). Individual human phenotypes in metabolic space and time. J. Proteome Res. 8, 4264–4271. doi:10.1021/pr900344m
Bhak, Y., Jeong, H. O., Cho, Y. S., Jeon, S., Cho, J., Gim, J. A., et al. (2019). Depression and suicide risk prediction models using blood-derived multi-omics data. Transl. Psychiatry 9, 262. doi:10.1038/s41398-019-0595-2
Bhat, A., Pires, A. S., Tan, V., Babu Chidambaram, S., and Guillemin, G. J. (2020). Effects of sleep deprivation on the tryptophan metabolism. Int. J. Tryptophan Res. 13, 1178646920970902. doi:10.1177/1178646920970902
Bhattacharyya, S., Dunlop, B. W., Mahmoudiandehkordi, S., Ahmed, A. T., Louie, G., Frye, M. A., et al. (2019). Pilot study of metabolomic clusters as state markers of major depression and outcomes to CBT treatment. Front. Neurosci. 13, 926. doi:10.3389/fnins.2019.00926
Brydges, C. R., Bhattacharyya, S., Dehkordi, S. M., Milaneschi, Y., Penninx, B., Jansen, R., et al. (2022). Metabolomic and inflammatory signatures of symptom dimensions in major depression. Brain Behav. Immun. 102, 42–52. doi:10.1016/j.bbi.2022.02.003
Buniello, A., MacArthur, J. A. L., Cerezo, M., Harris, L. W., Hayhurst, J., Malangone, C., et al. (2019). The NHGRI-EBI GWAS Catalog of published genome-wide association studies, targeted arrays and summary statistics 2019. Nucleic Acids Res. 47, D1005–D1012. doi:10.1093/nar/gky1120
Calati, R., Nemeroff, C. B., Lopez-Castroman, J., Cohen, L. J., and Galynker, I. (2020). Candidate biomarkers of suicide crisis syndrome: What to test next? A concept paper. Int. J. Neuropsychopharmacol. 23, 192–205. doi:10.1093/ijnp/pyz063
Claassen, C. A., Trivedi, M. H., Rush, A. J., Husain, M. M., Zisook, S., Young, E., et al. (2007). Clinical differences among depressed patients with and without a history of suicide attempts: Findings from the STAR*D trial. J. Affect. Disord. 97, 77–84. doi:10.1016/j.jad.2006.05.026
Consortium, G. T. (2020). The GTEx Consortium atlas of genetic regulatory effects across human tissues. Science 369, 1318–1330. doi:10.1126/science.aaz1776
Czysz, A. H., South, C., Gadad, B. S., Arning, E., Soyombo, A., Bottiglieri, T., et al. (2019). Can targeted metabolomics predict depression recovery? Results from the CO-med trial. Transl. Psychiatry 9, 11. doi:10.1038/s41398-018-0349-6
Das, S., Forer, L., Schonherr, S., Sidore, C., Locke, A. E., Kwong, A., et al. (2016). Next-generation genotype imputation service and methods. Nat. Genet. 48, 1284–1287. doi:10.1038/ng.3656
De Berardis, D., Fornaro, M., Valchera, A., Rapini, G., Di Natale, S., De Lauretis, I., et al. (2020). Alexithymia, resilience, somatic sensations and their relationships with suicide ideation in drug naive patients with first-episode major depression: An exploratory study in the "real world" everyday clinical practice. Early Interv. Psychiatry 14, 336–342. doi:10.1111/eip.12863
De Berardis, D., Serroni, N., Campanella, D., Carano, A., Gambi, F., Valchera, A., et al. (2008). Alexithymia and its relationships with C-reactive protein and serum lipid levels among drug naive adult outpatients with major depression. Prog. Neuropsychopharmacol. Biol. Psychiatry 32, 1982–1986. doi:10.1016/j.pnpbp.2008.09.022
Dunker Svendsen, S., Aggestrup, A. S., Norregaard, L. B., Loventoft, P., Præstegaard, A., Danilenko, K. V., et al. (2019). Circadian reinforcement therapy in combination with electronic self-monitoring to facilitate a safe post-discharge period of patients with depression by stabilizing sleep: Protocol of a randomized controlled trial. BMC Psychiatry 19, 124. doi:10.1186/s12888-019-2101-z
Freeman, A., Mergl, R., Kohls, E., Szekely, A., Gusmao, R., Arensman, E., et al. (2017). A cross-national study on gender differences in suicide intent. BMC Psychiatry 17, 234. doi:10.1186/s12888-017-1398-8
Fudalej, S., Ilgen, M., Fudalej, M., Kostrzewa, G., Barry, K., Wojnar, M., et al. (2010). Association between tryptophan hydroxylase 2 gene polymorphism and completed suicide. Suicide Life. threat. Behav. 40, 553–560. doi:10.1521/suli.2010.40.6.553
Gadad, B. S., Raj, P., Jha, M. K., Carmody, T., Dozmorov, I., Mayes, T. L., et al. (2018). Association of novel ALX4 gene polymorphisms with antidepressant treatment response: Findings from the CO-med trial. Mol. Neuropsychiatry 4, 7–19. doi:10.1159/000487321
Ghini, V., Saccenti, E., Tenori, L., Assfalg, M., and Luchinat, C. (2015). Allostasis and resilience of the human individual metabolic phenotype. J. Proteome Res. 14, 2951–2962. doi:10.1021/acs.jproteome.5b00275
Girvan, M., and Newman, M. E. (2002). Community structure in social and biological networks. Proc. Natl. Acad. Sci. U. S. A. 99, 7821–7826. doi:10.1073/pnas.122653799
Gomez-Abellan, P., Madrid, J. A., Lujan, J. A., Frutos, M. D., Gonzalez, R., Martinez-Augustin, O., et al. (2012). Sexual dimorphism in clock genes expression in human adipose tissue. Obes. Surg. 22, 105–112. doi:10.1007/s11695-011-0539-2
Grant, C. W., Barreto, E. F., Kumar, R., Kaddurah-Daouk, R., Skime, M., Mayes, T., et al. (2022). Multi-omics characterization of early- and adult-onset major depressive disorder. J. Pers. Med. 12, 412. doi:10.3390/jpm12030412
Gupta, M., Neavin, D., Liu, D., Biernacka, J., Hall-Flavin, D., Bobo, W. V., et al. (2016). TSPAN5, ERICH3 and selective serotonin reuptake inhibitors in major depressive disorder: Pharmacometabolomics-informed pharmacogenomics. Mol. Psychiatry 21, 1717–1725. doi:10.1038/mp.2016.6
Hamilton, M. (1967). Development of a rating scale for primary depressive illness. Br. J. Soc. Clin. Psychol. 6, 278–296. doi:10.1111/j.2044-8260.1967.tb00530.x
Hannah, R., Max, R., and Esteban, O. O. (2015). Suicide. Available at: https://ourworldindata.org/suicide.
Hayashi-Takagi, A., Vawter, M. P., and Iwamoto, K. (2014). Peripheral biomarkers revisited: Integrative profiling of peripheral samples for psychiatric research. Biol. Psychiatry 75, 920–928. doi:10.1016/j.biopsych.2013.09.035
Isometsa, E. T., Heikkinen, M. E., Marttunen, M. J., Henriksson, M. M., Aro, H. M., and Lonnqvist, J. K. (1995). The last appointment before suicide: Is suicide intent communicated? Am. J. Psychiatry 152, 919–922. doi:10.1176/ajp.152.6.919
Ji, Y., Biernacka, J. M., Hebbring, S., Chai, Y., Jenkins, G. D., Batzler, A., et al. (2013). Pharmacogenomics of selective serotonin reuptake inhibitor treatment for major depressive disorder: Genome-wide associations and functional genomics. Pharmacogenomics J. 13, 456–463. doi:10.1038/tpj.2012.32
Joyce, J. B., Grant, C. W., Liu, D., Mahmoudian Dehkordi, S., Kaddurah-Daouk, R., Skime, M., et al. (2021). Multi-omics driven predictions of response to acute phase combination antidepressant therapy: A machine learning approach with cross-trial replication. Transl. Psychiatry 11, 513. doi:10.1038/s41398-021-01632-z
Kim, S. H., Kim, H. J., Oh, S. H., and Cha, K. (2020). Analysis of attempted suicide episodes presenting to the emergency department: Comparison of young, middle aged and older people. Int. J. Ment. Health Syst. 14, 46. doi:10.1186/s13033-020-00378-3
Kim, S. W., Stewart, R., Kim, J. M., Shin, I. S., Yoon, J. S., Jung, S. W., et al. (2011). Relationship between a history of a suicide attempt and treatment outcomes in patients with depression. J. Clin. Psychopharmacol. 31, 449–456. doi:10.1097/JCP.0b013e3182217d51
Knowles, E. E. M., Huynh, K., Meikle, P. J., Goring, H. H. H., Olvera, R. L., Mathias, S. R., et al. (2017). The lipidome in major depressive disorder: Shared genetic influence for ether-phosphatidylcholines, a plasma-based phenotype related to inflammation, and disease risk. Eur. Psychiatry. 43, 44–50. doi:10.1016/j.eurpsy.2017.02.479
Kouter, K., and Videtic Paska, A. (2021). Omics' of suicidal behaviour: A path to personalised psychiatry. World J. Psychiatry 11, 774–790. doi:10.5498/wjp.v11.i10.774
Kriisa, K., Leppik, L., Balotsev, R., Ottas, A., Soomets, U., Koido, K., et al. (2017). Profiling of acylcarnitines in first episode psychosis before and after antipsychotic treatment. J. Proteome Res. 16, 3558–3566. doi:10.1021/acs.jproteome.7b00279
Labonte, B., Engmann, O., Purushothaman, I., Menard, C., Wang, J., Tan, C., et al. (2017). Sex-specific transcriptional signatures in human depression. Nat. Med. 23, 1102–1111. doi:10.1038/nm.4386
Levey, D. F., Niculescu, E. M., Le-Niculescu, H., Dainton, H. L., Phalen, P. L., Ladd, T. B., et al. (2016). Towards understanding and predicting suicidality in women: Biomarkers and clinical risk assessment. Mol. Psychiatry 21, 768–785. doi:10.1038/mp.2016.31
Lichtblau, Y., Zimmermann, K., Haldemann, B., Lenze, D., Hummel, M., and Leser, U. (2017). Comparative assessment of differential network analysis methods. Brief. Bioinform. 18, 837–850. doi:10.1093/bib/bbw061
Lin, P. Y., and Tsai, G. (2004). Association between serotonin transporter gene promoter polymorphism and suicide: Results of a meta-analysis. Biol. Psychiatry 55, 1023–1030. doi:10.1016/j.biopsych.2004.02.006
Liu, A., Dai, Y., Mendez, E. F., Hu, R., Fries, G. R., Najera, K. E., et al. (2021). Genome-wide correlation of DNA methylation and gene expression in postmortem brain tissues of opioid use disorder patients. Int. J. Neuropsychopharmacol. 24, 879–891. doi:10.1093/ijnp/pyab043
Liu, D., Ray, B., Neavin, D. R., Zhang, J., Athreya, A. P., Biernacka, J. M., et al. (2018). Beta-defensin 1, aryl hydrocarbon receptor and plasma kynurenine in major depressive disorder: Metabolomics-informed genomics. Transl. Psychiatry 8, 10. doi:10.1038/s41398-017-0056-8
Liu, D., Zhuang, Y., Zhang, L., Gao, H., Neavin, D., Carrillo-Roa, T., et al. (2020). ERICH3: Vesicular association and antidepressant treatment response. Mol. Psychiatry 26, 2415–2428. doi:10.1038/s41380-020-00940-y
Liu, X., Zheng, P., Zhao, X., Zhang, Y., Hu, C., Li, J., et al. (2015). Discovery and validation of plasma biomarkers for major depressive disorder classification based on liquid chromatography-mass spectrometry. J. Proteome Res. 14, 2322–2330. doi:10.1021/acs.jproteome.5b00144
Lopez, V. A., Detera-Wadleigh, S., Cardona, I., Kassem, L., and McMahon, F. J.National Institute of Mental Health Genetics Initiative Bipolar Disorder Consortium (2007). Nested association between genetic variation in tryptophan hydroxylase II, bipolar affective disorder, and suicide attempts. Biol. Psychiatry 61, 181–186. doi:10.1016/j.biopsych.2006.03.028
Maes, M., Smith, R., Christophe, A., Vandoolaeghe, E., Van Gastel, A., Neels, H., et al. (1997). Lower serum high-density lipoprotein cholesterol (HDL-C) in major depression and in depressed men with serious suicidal attempts: Relationship with immune-inflammatory markers. Acta Psychiatr. Scand. 95, 212–221. doi:10.1111/j.1600-0447.1997.tb09622.x
Magno, R., and Maia, A. T. (2020). gwasrapidd: an R package to query, download and wrangle GWAS catalog data. Bioinformatics 36, 649–650. doi:10.1093/bioinformatics/btz605
MahmoudianDehkordi, S., Ahmed, A. T., Bhattacharyya, S., Han, X., Baillie, R. A., Arnold, M., et al. (2021). Alterations in acylcarnitines, amines, and lipids inform about the mechanism of action of citalopram/escitalopram in major depression. Transl. Psychiatry 11, 153. doi:10.1038/s41398-020-01097-6
Malone, K. M., Ellis, S. P., Currier, D., and Mann, J. (2007). Platelet 5-HT2A receptor subresponsivity and lethality of attempted suicide in depressed in-patients. Int. J. Neuropsychopharmacol. 10, 335–343. doi:10.1017/S1461145706006997
Mann, J. J., Currier, D., Stanley, B., Oquendo, M. A., Amsel, L. V., and Ellis, S. P. (2006). Can biological tests assist prediction of suicide in mood disorders? Int. J. Neuropsychopharmacol. 9, 465–474. doi:10.1017/S1461145705005687
Mann, J. J., and Rizk, M. M. (2020). A brain-centric model of suicidal behavior. Am. J. Psychiatry 177, 902–916. doi:10.1176/appi.ajp.2020.20081224
Mash, H. B. H., Ursano, R. J., Kessler, R. C., Naifeh, J. A., Fullerton, C. S., Aliaga, P. A., et al. (2021). Predictors of suicide attempt within 30 Days after first medically documented suicidal ideation in U.S. Army soldiers. Am. J. Psychiatry 178, 1050–1059. doi:10.1176/appi.ajp.2021.20111570
Medrano, M., Aguinaga, D., Reyes-Resina, I., Canela, E. I., Mallol, J., Navarro, G., et al. (2018). Orexin A/hypocretin modulates leptin receptor-mediated signaling by allosteric modulations mediated by the ghrelin GHS-r1a receptor in hypothalamic neurons. Mol. Neurobiol. 55, 4718–4730. doi:10.1007/s12035-017-0670-8
Mirsu-Paun, A., Jaussent, I., Komar, G., Courtet, P., and Lopez-Castroman, J. (2017). Sleep complaints associated with wish to die after a suicide crisis-an exploratory study. J. Sleep. Res. 26, 726–731. doi:10.1111/jsr.12537
Mohawk, J. A., Green, C. B., and Takahashi, J. S. (2012). Central and peripheral circadian clocks in mammals. Annu. Rev. Neurosci. 35, 445–462. doi:10.1146/annurev-neuro-060909-153128
Morgan, R. E., Palinkas, L. A., Barrett-Connor, E. L., and Wingard, D. L. (1993). Plasma cholesterol and depressive symptoms in older men. Lancet 341, 75–79. doi:10.1016/0140-6736(93)92556-9
Muldoon, M. F., Manuck, S. B., and Matthews, K. A. (1990). Lowering cholesterol concentrations and mortality: A quantitative review of primary prevention trials. BMJ 301, 309–314. doi:10.1136/bmj.301.6747.309
Mullins, N., Kang, J., Campos, A. I., Coleman, J. R. I., Edwards, A. C., Galfalvy, H., et al. (2022). Dissecting the shared genetic architecture of suicide attempt, psychiatric disorders, and known risk factors. Biol. Psychiatry 91, 313–327. doi:10.1016/j.biopsych.2021.05.029
Neaton, J. D., Blackburn, H., Jacobs, D., Kuller, L., Lee, D. J., Sherwin, R., et al. (1992). Serum cholesterol level and mortality findings for men screened in the multiple risk factor intervention trial. Multiple risk factor intervention trial research group. Arch. Intern. Med. 152, 1490–1500. doi:10.1001/archinte.152.7.1490
Neavin, D., Kaddurah-Daouk, R., and Weinshilboum, R. (2016). Pharmacometabolomics informs pharmacogenomics. Metabolomics 12, 121. doi:10.1007/s11306-016-1066-x
Oquendo, M. A., Sullivan, G. M., Sudol, K., Baca-Garcia, E., Stanley, B. H., Sublette, M. E., et al. (2014). Toward a biosignature for suicide. Am. J. Psychiatry 171, 1259–1277. doi:10.1176/appi.ajp.2014.14020194
Orta, O. R., Barbosa, C., Velez, J. C., Gelaye, B., Chen, X., Stoner, L., et al. (2016). Associations of self-reported and objectively measured sleep disturbances with depression among primary caregivers of children with disabilities. Nat. Sci. Sleep. 8, 181–188. doi:10.2147/NSS.S104338
Parksepp, M., Leppik, L., Koch, K., Uppin, K., Kangro, R., Haring, L., et al. (2020). Metabolomics approach revealed robust changes in amino acid and biogenic amine signatures in patients with schizophrenia in the early course of the disease. Sci. Rep. 10, 13983. doi:10.1038/s41598-020-71014-w
Patterson, W. M., Dohn, H. H., Bird, J., and Patterson, G. A. (1983). Evaluation of suicidal patients: The SAD PERSONS scale. Psychosomatics 24, 343. doi:10.1016/S0033-3182(83)73213-5
Pawlak, J., Dmitrzak-Weglarz, M., Maciukiewicz, M., Wilkosc, M., Leszczynska-Rodziewicz, A., Zaremba, D., et al. (2015). Suicidal behavior in the context of disrupted rhythmicity in bipolar disorder--data from an association study of suicide attempts with clock genes. Psychiatry Res. 226, 517–520. doi:10.1016/j.psychres.2015.01.010
Penas-Lledo, E., Guillaume, S., Delgado, A., Naranjo, M. E. G., Jaussent, I., LLerena, A., et al. (2015). ABCB1 gene polymorphisms and violent suicide attempt among survivors. J. Psychiatr. Res. 61, 52–56. doi:10.1016/j.jpsychires.2014.12.005
Perroud, N., Uher, R., Hauser, J., Rietschel, M., Henigsberg, N., Placentino, A., et al. (2010). History of suicide attempts among patients with depression in the GENDEP project. J. Affect. Disord. 123, 131–137. doi:10.1016/j.jad.2009.09.001
Peters, J. C. (1991). Tryptophan nutrition and metabolism: An overview. Adv. Exp. Med. Biol. 294, 345–358. doi:10.1007/978-1-4684-5952-4_32
Pitchot, W., Hansenne, M., Pinto, E., Reggers, J., Fuchs, S., and Ansseau, M. (2005). 5-Hydroxytryptamine 1A receptors, major depression, and suicidal behavior. Biol. Psychiatry 58, 854–858. doi:10.1016/j.biopsych.2005.05.042
Pu, J., Liu, Y., Zhang, H., Tian, L., Gui, S., Yu, Y., et al. (2020). An integrated meta-analysis of peripheral blood metabolites and biological functions in major depressive disorder. Mol. Psychiatry 26, 4265–4276. doi:10.1038/s41380-020-0645-4
R Foundation for Statistical Computing (2020). A language and environment for statistical computing. Vienna: R Foundation for Statistical Computing.
Recourt, K., de Boer, P., Zuiker, R., Luthringer, R., Kent, J., van der Ark, P., et al. (2019). The selective orexin-2 antagonist seltorexant (JNJ-42847922/MIN-202) shows antidepressant and sleep-promoting effects in patients with major depressive disorder. Transl. Psychiatry 9, 216. doi:10.1038/s41398-019-0553-z
Rijo-Ferreira, F., and Takahashi, J. S. (2019). Genomics of circadian rhythms in health and disease. Genome Med. 11, 82. doi:10.1186/s13073-019-0704-0
Roy, A., Gorodetsky, E., Yuan, Q., Goldman, D., and Enoch, M. A. (2010). Interaction of FKBP5, a stress-related gene, with childhood trauma increases the risk for attempting suicide. Neuropsychopharmacology 35, 1674–1683. doi:10.1038/npp.2009.236
Ruiz-Perez, D., Guan, H., Madhivanan, P., Mathee, K., and Narasimhan, G. (2020). So you think you can PLS-DA? BMC Bioinforma. 21, 2. doi:10.1186/s12859-019-3310-7
Rujescu, D., Giegling, I., Sato, T., Hartmann, A. M., and Moller, H. J. (2003). Genetic variations in tryptophan hydroxylase in suicidal behavior: Analysis and meta-analysis. Biol. Psychiatry 54, 465–473. doi:10.1016/s0006-3223(02)01748-1
Rush, A. J., Bernstein, I. H., Trivedi, M. H., Carmody, T. J., Wisniewski, S., Mundt, J. C., et al. (2006). An evaluation of the quick inventory of depressive symptomatology and the Hamilton rating scale for depression: A sequenced treatment alternatives to relieve depression trial report. Biol. Psychiatry 59, 493–501. doi:10.1016/j.biopsych.2005.08.022
Rush, A. J., Trivedi, M. H., Stewart, J. W., Nierenberg, A. A., Fava, M., Kurian, B. T., et al. (2011). Combining medications to enhance depression outcomes (CO-med): Acute and long-term outcomes of a single-blind randomized study. Am. J. Psychiatry 168, 689–701. doi:10.1176/appi.ajp.2011.10111645
Russell, A. E., Ford, T., Gunnell, D., Heron, J., Joinson, C., Moran, P., et al. (2020). Investigating evidence for a causal association between inflammation and self-harm: A multivariable mendelian randomisation study. Brain Behav. Immun. 89, 43–50. doi:10.1016/j.bbi.2020.05.065
Ryan, E. P., and Oquendo, M. A. (2020). Suicide risk assessment and prevention: Challenges and opportunities. Focus (Am Psychiatr. Publ. 18, 88–99. doi:10.1176/appi.focus.20200011
Salter, M. (1992). Low serum cholesterol and suicide. Lancet 339, 727–729. doi:10.1016/0140-6736(92)90609-7
Schmaal, L., van Harmelen, A. L., Chatzi, V., Lippard, E. T. C., Toenders, Y. J., Averill, L. A., et al. (2020). Imaging suicidal thoughts and behaviors: A comprehensive review of 2 decades of neuroimaging studies. Mol. Psychiatry 25, 408–427. doi:10.1038/s41380-019-0587-x
Sciences, B. L. (2020). Annotation of potential isobaric and isomeric lipid species analyzed using the MxP® Quant 500 Kit. Available at: https://biocrates.com/wp-content/uploads/2020/02/Biocrates_Q500_isomers_isobars.pdf.
Setoyama, D., Kato, T. A., Hashimoto, R., Kunugi, H., Hattori, K., Hayakawa, K., et al. (2016). Plasma metabolites predict severity of depression and suicidal ideation in psychiatric patients-A multicenter pilot analysis. PLoS One 11, e0165267. doi:10.1371/journal.pone.0165267
Smith, E. G., Kim, H. M., Ganoczy, D., Stano, C., Pfeiffer, P. N., and Valenstein, M. (2013). Suicide risk assessment received prior to suicide death by Veterans Health Administration patients with a history of depression. J. Clin. Psychiatry 74, 226–232. doi:10.4088/JCP.12m07853
Strauss, J., McGregor, S., Freeman, N., Tiwari, A., George, C. J., Kovacs, M., et al. (2012). Association study of early-immediate genes in childhood-onset mood disorders and suicide attempt. Psychiatry Res. 197, 49–54. doi:10.1016/j.psychres.2011.11.022
Sudol, K., and Mann, J. J. (2017). Biomarkers of suicide attempt behavior: Towards a biological model of risk. Curr. Psychiatry Rep. 19, 31. doi:10.1007/s11920-017-0781-y
Sun, Y., Drevets, W., Turecki, G., and Li, Q. S. (2020). The relationship between plasma serotonin and kynurenine pathway metabolite levels and the treatment response to escitalopram and desvenlafaxine. Brain Behav. Immun. 87, 404–412. doi:10.1016/j.bbi.2020.01.011
Tidymodels (2020). A collection of packages for modeling and machine learning using tidyverse principles.
Townsend, M. K., Clish, C. B., Kraft, P., Wu, C., Souza, A. L., Deik, A. A., et al. (2013). Reproducibility of metabolomic profiles among men and women in 2 large cohort studies. Clin. Chem. 59, 1657–1667. doi:10.1373/clinchem.2012.199133
Trivedi, M. H., Rush, A. J., Ibrahim, H. M., Carmody, T. J., Biggs, M. M., Suppes, T., et al. (2004). The inventory of depressive symptomatology, clinician rating (IDS-C) and self-report (IDS-SR), and the quick inventory of depressive symptomatology, clinician rating (QIDS-C) and self-report (QIDS-SR) in public sector patients with mood disorders: A psychometric evaluation. Psychol. Med. 34, 73–82. doi:10.1017/s0033291703001107
Uppal, K., Ma, C., Go, Y. M., Jones, D. P., and Wren, J. (2018). xMWAS: a data-driven integration and differential network analysis tool. Bioinformatics 34, 701–702. doi:10.1093/bioinformatics/btx656
van der Veen, J. N., Kennelly, J. P., Wan, S., Vance, J. E., Vance, D. E., and Jacobs, R. L. (2017). The critical role of phosphatidylcholine and phosphatidylethanolamine metabolism in health and disease. Biochim. Biophys. Acta. Biomembr. 1859, 1558–1572. doi:10.1016/j.bbamem.2017.04.006
Walther, A., Cannistraci, C. V., Simons, K., Duran, C., Gerl, M. J., Wehrli, S., et al. (2018). Lipidomics in major depressive disorder. Front. Psychiatry 9, 459. doi:10.3389/fpsyt.2018.00459
Yang, C. C., Jick, S. S., and Jick, H. (2003). Lipid-lowering drugs and the risk of depression and suicidal behavior. Arch. Intern. Med. 163, 1926–1932. doi:10.1001/archinte.163.16.1926
Yeh, Y. W., Ho, P. S., Chen, C. Y., Kuo, S. C., Liang, C. S., Ma, K. H., et al. (2014). Incongruent reduction of serotonin transporter associated with suicide attempts in patients with major depressive disorder: A positron emission tomography study with 4-[18F]-ADAM. Int. J. Neuropsychopharmacol. 18, pyu065. doi:10.1093/ijnp/pyu065
Keywords: machine learning, suicide, depression, genomics, circadian rhythm, multi-omics
Citation: Grant CW, Wilton AR, Kaddurah-Daouk R, Skime M, Biernacka J, Mayes T, Carmody T, Wang L, Lazaridis K, Weinshilboum R, Bobo WV, Trivedi MH, Croarkin PE and Athreya AP (2022) Network science approach elucidates integrative genomic-metabolomic signature of antidepressant response and lifetime history of attempted suicide in adults with major depressive disorder. Front. Pharmacol. 13:984383. doi: 10.3389/fphar.2022.984383
Received: 02 July 2022; Accepted: 15 August 2022;
Published: 03 October 2022.
Edited by:
Augusto Garcia-Agundez, Brown University, United StatesReviewed by:
Domenico De Berardis, Mental Health Center (CSM) and Psychiatric Service of Diagnosis and Treatment (SPDC), ItalyXi Wu, Shanghai Jiao Tong University, China
Copyright © 2022 Grant, Wilton, Kaddurah-Daouk, Skime, Biernacka, Mayes, Carmody, Wang, Lazaridis, Weinshilboum, Bobo, Trivedi, Croarkin and Athreya. This is an open-access article distributed under the terms of the Creative Commons Attribution License (CC BY). The use, distribution or reproduction in other forums is permitted, provided the original author(s) and the copyright owner(s) are credited and that the original publication in this journal is cited, in accordance with accepted academic practice. No use, distribution or reproduction is permitted which does not comply with these terms.
*Correspondence: Paul E. Croarkin, Y3JvYXJraW4ucGF1bEBtYXlvLmVkdQ==; Arjun P. Athreya, YXRocmV5YS5hcmp1bkBtYXlvLmVkdQ==
†These authors have contributed equally to this work