- 1Liver Research Center, Beijing Friendship Hospital, Capital Medical University, National Clinical Research Center for Digestive Diseases, Beijing, China
- 2Department of Pathology, Yong Loo Lin School of Medicine, National University of Singapore, Singapore, Singapore
- 3Department of Pathology and Molecular Medicine, Faculty of Health Sciences, McMaster University, Hamilton, ON, Canada
- 4Fourth Department of Liver Disease (Difficult and Complicated Liver Diseases and Artificial Liver Center), Beijing You’an Hospital, Capital Medical University, Beijing, China
- 5Clinical Epidemiology and Evidence Base Medicine Unit, Beijing Friendship Hospital, Capital Medical University, Beijing, China
- 6Department of Critical Liver Diseases, Liver Research Center, Beijing Friendship Hospital, Capital Medical University, National Clinical Research Center for Digestive Diseases, Beijing, China
- 7Senior Department of Hepatology, The Fifth Medical Center of PLA General Hospital, Beijing, China
Aims: To develop, optimize, and validate a novel model using alanine aminotransferase (ALT) and total bilirubin (TB) dynamic evolution patterns in predicting acute liver failure (ALF) in drug-induced liver injury (DILI) patients.
Methods: The demographics, clinical data, liver biopsy, and outcomes of DILI patients were collected from two hospitals. According to the dynamic evolution of ALT and TB after DILI onset, the enrolled patients were divided into ALT-mono-peak, TB-mono-peak, double-overlap-peak, and double-separate-peak (DSP) patterns and compared. Logistic regression was used to develop this predictive model in both discovery and validation cohorts.
Results: The proportion of ALF was significantly higher in patients with the DSP pattern than in the ALT-mono-peak pattern and DOP pattern (10.0 vs. 0.0% vs. 1.8%,p < 0.05). The area under receiver operating characteristic curve (AUROC) of the DSP pattern model was 0.720 (95% CI: 0.682–0.756) in the discovery cohort and 0.828 (95% CI: 0.788–0.864) in the validation cohort in predicting ALF, being further improved by combining with international normalized ratio (INR) and alkaline phosphatase (ALP) (AUROC in the discovery cohort: 0.899; validation cohort: 0.958). Histopathologically, patients with the DSP pattern exhibited a predominantly cholestatic hepatitis pattern (75.0%, p < 0.05) with a higher degree of necrosis (29.2%, p = 0.084).
Conclusion: DILI patients with the DSP pattern are more likely to progress to ALF. The predictive potency of the model for ALF can be improved by incorporating INR and ALP. This novel model allows for better identification of high-risk DILI patients, enabling timely measures to be instituted for better outcome.
Introduction
There have been dramatic changes in recent decades in the spectrum of liver diseases with a far-reaching impact on healthcare systems worldwide. The majority of chronic hepatitis C patients can be cured (Dennis et al., 2021), and chronic hepatitis B can be effectively controlled (European Association for the Study of the Liver, 2017). Drug-induced liver injury (DILI) has gradually emerged as a relatively common clinical liver disease with significant derangement of liver biochemical tests. Epidemiological data suggest that the annual incidence of DILI is 2.7–13.9 per 100,000 in Europe and North America (Vega et al., 2017; Sgro et al., 2002); it is even higher in the Asia-Pacific region (Suk et al., 2012; Shen et al., 2019) with an annual incidence of 13.9–23.8 per 100,000.
DILI is the most common cause of acute liver failure (ALF) in Europe and North America (Khandelwal et al., 2011; Reuben et al., 2016; Stravitz and Lee, 2019), and the mortality associated with DILI-induced ALF is as high as 80% if liver transplantation had not been performed (Stravitz and Lee, 2019). A recent prospective study from the Drug-Induced Liver Injury Network (DILIN) found that 10% of patients died or required liver transplantation within 2 years of DILI onset, in 80% of which DILI played a major or contributory role. (Hayashi et al., 2017).
Early identification of DILI-induced ALF is critical in clinical practice so that timely measures can be adopted to improve the final outcome. Various predictive models of ALF have been established. Hyman Zimmerman’s model (Hy’s Law), the most impactful, was used for early prediction of ALF during drug development and in clinical settings. It was validated by the Spanish DILI registry (Andrade et al., 2005) Swedish Adverse Drug Reactions Advisory Committee (SADRAC) database (Björnsson and Olsson, 2005) and the US DILIN (Chalasani et al., 2008; Andrade and Robles-Díaz, 2020). Subsequently, Spanish scholars updated Hy’s law and proposed a novel independent prognostic algorithm (named Robles-Diaz Model in our study) for DILI-induced ALF to achieve a better balance between sensitivity and specificity (Robles-Diaz et al., 2014; Lo Re et al., 2015). However, these models are based on specific values of liver biochemical parameter(s) at a single time point (onset/peak), and their predictive capability can certainly be improved. Whether the dynamic evolution patterns of ALT and TB in patients with DILI can be used as a new model to predict DILI-induced ALF has not been studied yet.
In this study, a novel model based on the dynamic evolution of ALT and TB in predicting DILI-induced ALF was established, optimized, and validated. The significance was to assist in early and accurate identification of high-risk DILI patients for timely intervention to improve clinical outcome.
Patients and Methods
Study Subjects
The study population was divided into the discovery cohort and the validation cohort. From January 2016 to December 2018, the medical records of patients with DILI were retrieved as the discovery cohort at the Senior Department of Hepatology, the Fifth Medical Center of PLA General Hospital, Beijing, China. Additionally, patients between January 2013 and December 2020 at the Liver Research Center, Beijing Friendship Hospital, Capital Medical University, Beijing, China, were included in the external validation cohort.
1.1 Inclusion criteria: 1) Age ≥ 18 years; 2) the chronological sequence between drug and liver injury was clear; 3) the Roussel–Uclaf Causality Assessment Method (RUCAM) score is ≥6.
1.2 Exclusion criteria: 1) Acute viral hepatitis A to E, Epstein-Barr virus or cytomegalovirus infection, autoimmune liver diseases (autoimmune hepatitis, primary biliary cholangitis, and primary sclerosing cholangitis), non-alcoholic steatohepatitis, alcoholic liver disease, hereditary and metabolic liver diseases, biliary obstruction, and ischemic hepatitis; 2) systemic infections (such as sepsis); 3) organ transplantation; and 4) malignant tumor of the liver, bile duct, or pancreas.
Study Methods
Retrieval of Onset Data and Hospitalization Data
Demographic, clinical, and laboratory data at the onset and hospital admission were retrieved, including blood routine, liver biochemical tests, lipid profiles, international normalized ratio (INR), viral hepatitis, and autoimmune markers. The dynamic evolution of ALT and TB during the course of the disease was recorded, and the corresponding patterns were established.
Clinical Classification, Causality, and Severity of Drug-Induced Liver Injury
Clinical classification of DILI was based on the Council for International Organizations of Medical Sciences (CIOMS) criteria: hepatocellular injury type: R ≥ 5, cholestatic: R ≤ 2, and mixed: 2 < R < 5 (Aithal et al., 2011).
The causality of the drug to liver injury was assessed using the Roussel Ucalaf causality assessment (RUCAM) (Danan and Teschke, 2015).
Severity of cases was graded as mild, moderate, severe, acute liver failure, and fatal according to the Chinese 2015 DILI guidelines (Yu et al., 2017).
Criteria for Prediction Models of Drug-Induced Liver Injury-Induced Acute Liver Failure
The current prediction models are as follows:
(i) Hy’s law: ALT or AST >3×ULN and TB > 2×ULN, ALP <2×ULN (Temple, 2006);
(ii) New Hy’s law (nHy’s law): TB > 2×ULN, nR ≥ 5 [nR value defined as (measured highest ALT or AST/their ULN)/(measured ALP/ALP ULN)] (Robles-Diaz et al., 2014); and
(iii) Robles-Diaz Model (Robles-Diaz et al., 2014): AST >17.3×ULN and TB > 6.6×ULN, or AST ≤17.3×ULN, but AST/ALT >1.5 (Björnsson and Olsson, 2005).
Definition of Alanine Aminotransferase-Total Bilirubin Dynamic Evolution Patterns
In order to establish a new prediction model for predicting ALF after the onset of DILI, the ALT-TB dynamic evolution patterns are defined as the following four patterns (Figure 1): 1) ALT-mono-peak pattern: ALT ≥3×ULN and TB < 2.5×ULN; 2) TB-mono-peak pattern: ALT <3×ULN and TB ≥ 2.5×ULN; 3) ALT and TB double overlap peak (DOP) pattern: ALT ≥3×ULN and TB ≥ 2.5×ULN, with the time interval between ALT and TB peaks being <3 days; and 4) ALT and TB double separate peak (DSP) pattern: ALT ≥3×ULN and TB ≥ 2.5×ULN, with the time interval between ALT and TB peaks being ≥3 days.
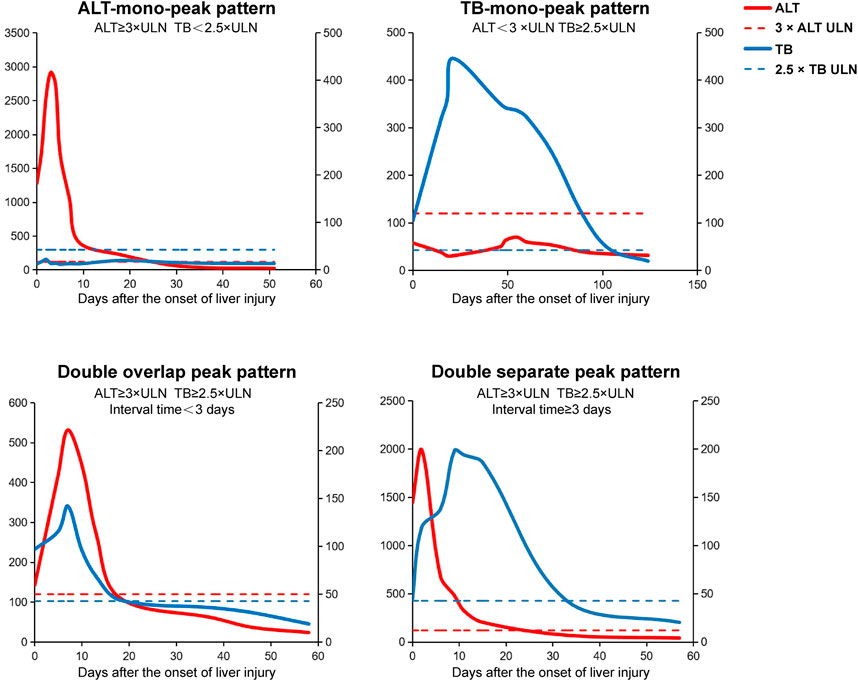
FIGURE 1. Schematic diagram of the four different dynamic evolution patterns of ALT and TB in DILI. The ALT-TB dynamic evolution pattern is defined as the following four patterns: (1) ALT-mono-peak pattern, ALT ≥ 3×ULN, and TB < 2.5×ULN; (2) TB-mono-peak pattern, ALT < 3×ULN and TB ≥ 2.5×ULN; (3) ALT and TB double overlap peak pattern, ALT ≥ 3×ULN and TB ≥ 2.5×ULN, and the time interval between ALT and TB peak <3 days; (4) ALT and TB double separate peak pattern, ALT ≥ 3×ULN and TB ≥ 2.5×ULN, and the time interval between ALT and TB peaks ≥3 days. Abbreviations: DILI, drug-induced liver injury; ALT, alanine aminotransferase; TB, total bilirubin.
Assessment of Liver Pathology
The liver biopsies were stained with hematoxylin and eosin (H&E), reticulin, Masson trichrome, periodic acid-Schiff with diastase (PAS-D), cytokeratin 7 (CK7), and CK19. Liver biopsies of enrolled patients (if any) were reviewed and classified by a clinical liver pathologist (XYZ) according to the pathological classification of DILI by Kleiner et al. (2014) and Wang et al. (2019).
Follow-Up and Definition of Clinical Outcomes for Drug-Induced Liver Injury
Follow-up within 1 year of DILI onset was achieved by means of a hospital management system (HIS) query, telephone consultation, and post-discharge laboratory test records. The clinical outcomes included ALF or death/liver transplantation.
The definition of ALF is according to the American Association for the Study of Liver Diseases (AASLD) guideline for the management of ALF (Polson and Lee, 2005). Liver-related deaths include (Hayashi et al., 2017) 1) DILI directly causing death and 2) aggravation of DILI or induction of another fatal disease (e.g., sepsis, multiorgan failure, and others).
Statistical Analysis
All data were analyzed using R version 3.3.3 and SPSS software (version 21.0; IBM Corp., Armonk, NY). Differences between groups were analyzed by ANOVA analysis for normally distributed variables, the Kruskal–Wallis H-test for non-normally distributed continuous variables, and the Chi-square test for categorical data. Mann–Whitney U tests were performed for multiple comparisons. Bilateral p < 0.05 was regarded as statistical difference.
The receiver operating characteristic (ROC) curves were used to analyze the prognostic performance of the previously published models and our novel model, and the area under ROC curve (AUROC) of the different models was compared by the Delong method. Logistic regression and the bootstrap method were used to develop and validate the optimized model in discovery and validation cohorts. In logistic regression, variables with p < 0.05 in the univariate analysis were screened as input variables and independent variables were screened using a likelihood ratio-based forward method to establish a logistic regression model.
This study has been approved by the Medical Ethics Committee of Beijing Friendship Hospital, Capital Medical University (Approval No.: 2020-P2-071-01), and the informed consent form has been waived.
Results
Demographic and Clinical Characteristics According to Alanine Aminotransferase-Total Bilirubin Dynamic Evolution Patterns
Patients diagnosed with DILI at the Fifth Medical Center of PLA General Hospital were enrolled in the discovery cohort (604 cases) from January 2016 to December 2018, and patients diagnosed with DILI at the Beijing Friendship Hospital, Capital Medical University, were enrolled in the external validation cohort (402 cases) from January 2013 to December 2020 (Figure 2).
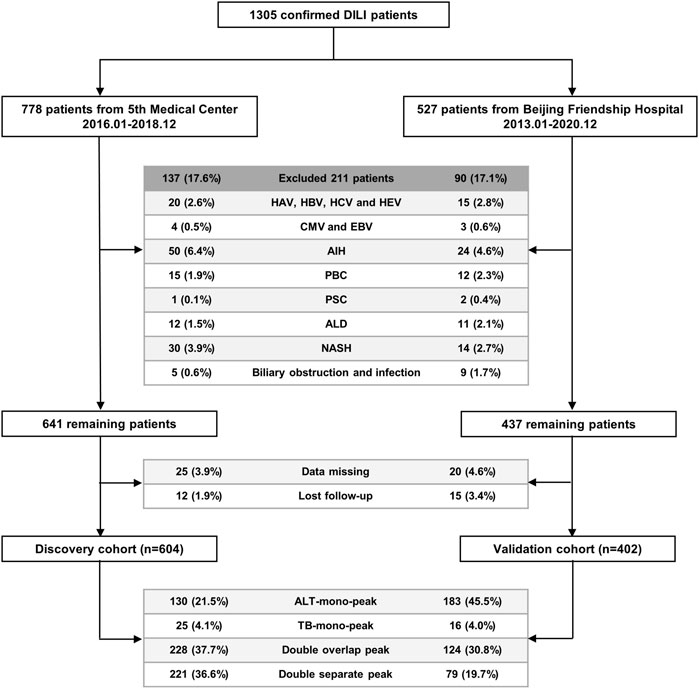
FIGURE 2. Flowchart of the enrolled patients with DILI in the discovery and validation cohorts. Abbreviations: DILI, drug-induced liver injury; HAV, hepatitis A virus; HBV, hepatitis B virus; HCV, hepatitis C virus; HEV, hepatitis E virus; CMV, cytomegalovirus; EBV, Epstein-Barr virus; AIH, autoimmune hepatitis; PBC, primary biliary cholangitis; PSC, primary sclerosing cholangitis; ALD, alcoholic liver disease; NASH, non-alcoholic steatohepatitis; ALT, alanine aminotransferase; TB, Total bilirubin.
In the discovery cohort, 372 out of 604 cases (61.6%) were female, with a median age of 49 years. The common clinical symptoms were jaundice (78.6%), fatigue (74.2%), and poor appetite (70.5%). Hepatocellular injury was predominant (80.0%). There was no significant difference in age, sex, latency, and BMI among the four dynamic evolution patterns (p > 0.05) (Table 1). The median hospitalization time was significantly longer (23.0 days) in patients with the DSP pattern.
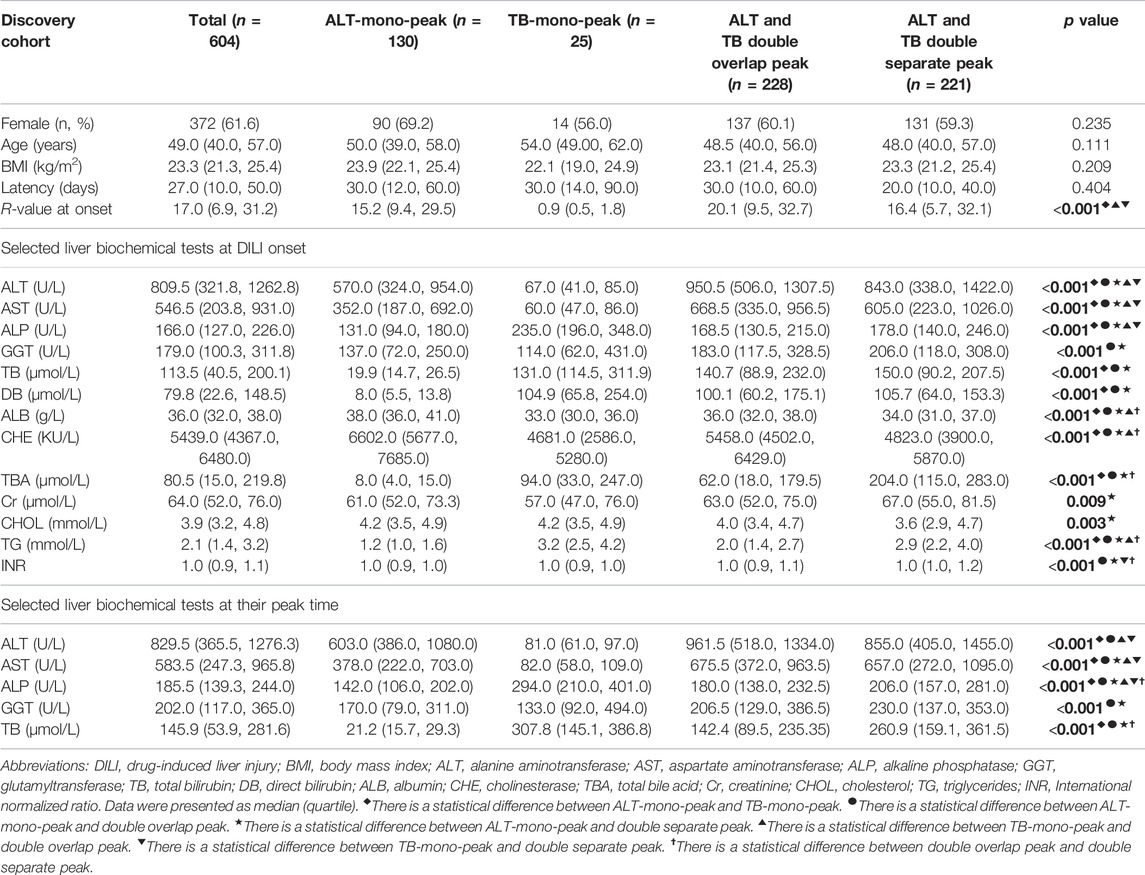
TABLE 1. Comparison of the demographic data and liver biochemical parameters among the four ALT-TB dynamic evolution patterns in DILI.
At DILI onset, ALT, AST, ALP, GGT, TB, DB, and INR were significantly higher in the two double-peak patterns than in the ALT-mono-peak pattern (p < 0.05) (Table 1). Furthermore, INR levels were significantly higher in the DSP pattern than in the DOP pattern. Total bile acid (TBA) levels were significantly higher in the DSP pattern than in the ALT-mono-peak pattern and DOP pattern (p < 0.05), and its median values were 20 times higher than the upper limit of normal. Albumin (ALB) and cholinesterase (CHE) levels were significantly lower in the DSP pattern than that in the ALT-mono-peak pattern and DOP pattern (p < 0.05). At the peak level of biochemical tests, TB and ALP were significantly higher in the DSP pattern than in the ALT-mono-peak pattern and DOP pattern (p < 0.05). The proportion of ALF was significantly higher in patients with the DSP pattern than in the ALT-mono-peak pattern and DOP pattern (10.0 vs. 0.0% vs. 1.8%, p < 0.05) (Table 2).
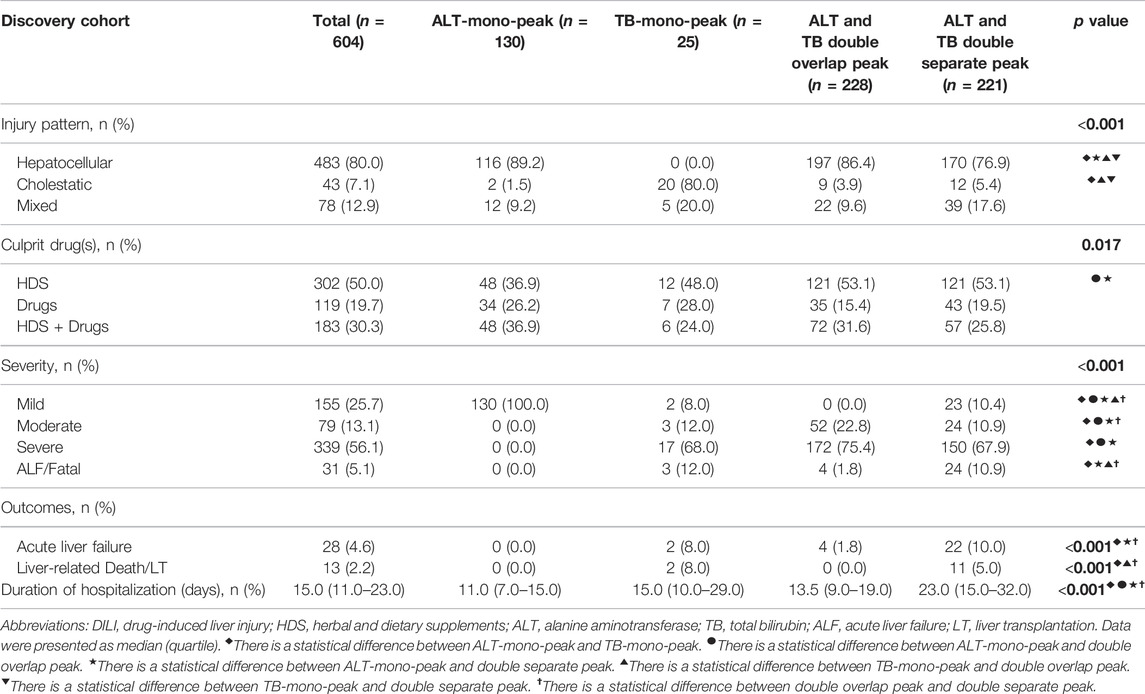
TABLE 2. Comparison of clinical classification, severity, and outcomes among the four ALT-TB dynamic evolution patterns in DILI.
Comparison of Clinical Characteristics and Laboratory Data at Drug-Induced Liver Injury Onset Between the ALF/non-ALF Group and Drug-Induced Liver Injury With/Without Non-Alcoholic Fatty Liver Disease Group
The laboratory tests at DILI onset showed that INR, TB, and TBAs were significantly higher, while ALB was significantly lower in the ALF group than in the non-ALF group (p < 0.05) (Supplementary Table S4). No significant difference in ALT, AST, ALP, and GGT was found between the two groups (Supplementary Table S4). In the discovery cohort, 99 of 604 cases (16.3%) had underline NAFLD. No significant difference was found in the outcomes of ALF and liver-related death/LT between the two groups (Supplementary Table S5). There was no significant difference between the DILI with NAFLD and DILI without the NAFLD group except for BMI, GGT, and the proportion of females (Supplementary Table S5).
Prediction of Acute Liver Failure According to Alanine Aminotransferase-Total Bilirubin Dynamic Evolution Patterns
As shown in Table 2, the two double-peak pattern groups had a significantly higher proportion of ALF than the two mono-peak pattern groups: 22 cases (10.0%) in the DSP and four (1.8%) in the DOP patterns but none in the ALT-mono-peak pattern (p < 0.001). The DSP pattern had the worst outcomes—22 patients (10.0%) developed ALF and 11 (5%) developed liver-related death.
As a novel model for the prediction of ALF, the sensitivity and specificity of the DSP pattern were 78.6 and 65.5%, respectively. The AUROC of the DSP model was 0.720 (95% CI: 0.682–0.756), whereas the AUROCs of Hy’s law, nHy’s law, and Robles-Diaz Model were 0.515 (95% CI: 0.474–0.555), 0.583 (95% CI: 0.543–0.623), and 0.635 (95% CI: 0.595–0.673), respectively (Table 3). The AUROC of the DSP model was significantly superior to the Hy’s law and nHy’s law models (Figure 3) (Z = 3.386, p < 0.001 or Z = 2.757, p = 0.006), comparable with the Robles-Diaz Model (Z = 1.296, p = 0.195).
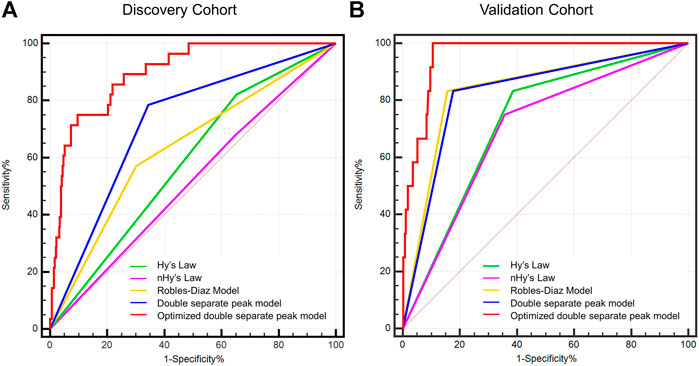
FIGURE 3. Potency comparison among different models in predicting DILI-induced ALF. The AUROC of the DSP model was significantly superior to that of Hy’s law and nHy’s law models and comparable with the Robles-Diaz Model. The prediction potency of ALF was further improved when incorporated with INR and ALP at DILI onset, which was significantly better than the three previous models with an AUROC for predicting DILI-induced ALF of 0.899 (95% CI: 0.87–0.921) in the discovery cohort and 0.958 (95% CI: 0.933–0.975) in the validation cohort, respectively [Figure (A) and (B)]. Abbreviations: AUROC, area under receiver operating characteristic; DSP, ALT, and TB double separate peak patterns; nHy’s Law, new Hy’s Law; DILI, drug-induced liver injury; ALT, alanine aminotransferase; TB, total bilirubin.
The AUROC of the DSP pattern in DILI with NAFLD patients was 0.859 (95% CI: 0.775–0.921), with a sensitivity of 100.0% and a specificity of 71.9%.
Verification of Alanine Aminotransferase-Total Bilirubin Dynamic Evolution Patterns in the Prediction of Acute Liver Failure
We conducted both internal and external verification on the potency of the DSP model in predicting DILI-induced ALF. A validity evaluation of the DSP model using internal validation was performed by bootstrap methods. The AUROC of the DSP model was 0.720 (95% CI:0.682–0.756), and the Brier score used to assess the calibration of the model was 0.044.
An additional independent 402 patients were enrolled in the external validation cohort. The patients were also mainly females (70.1%), the median age of onset was 57.0 years, and the main clinical type was hepatocellular injury (60.9%) (Supplementary Table S1). The median hospitalization time was significantly longer (14.0 days) in patients with the DSP pattern. The rates of ALF (12.7%) and DILI-induced deaths or liver transplantation (3.8%) in the DSP group were higher than that in other groups but without significance (Supplementary Table S2). The AUROC of the DSP model for predicting ALF in the validation cohort was 0.828 (95% CI: 0.788–0.864) with a Brier score of 0.027 (Table 3).
Optimization of the Double Separate Peak Model by Incorporation of International Normalized Ratio and Alkaline Phosphatase at Drug-Induced Liver Injury Onset
In light of the role of laboratory parameters other than ALT and TB in the prognostic assessment of DILI, the logistic regression model was used to screen for risk factors for the development of ALF in patients with DILI. When the variables with p value <0.05 were included in the multivariate analysis, it was found that INR (OR = 11.8, p < 0.001) and ALP at DILI onset (OR = 1.004, p = 0.002), complementary with the DSP model (OR = 3.906, p = 0.007), were independent risk factors for the development of ALF in patients with DILI, and the logistic regression model was as follows: Logistic (p) = 1.347 × DSP pattern + 8.363 × INR + 0.004 × ALP (Figure 4).
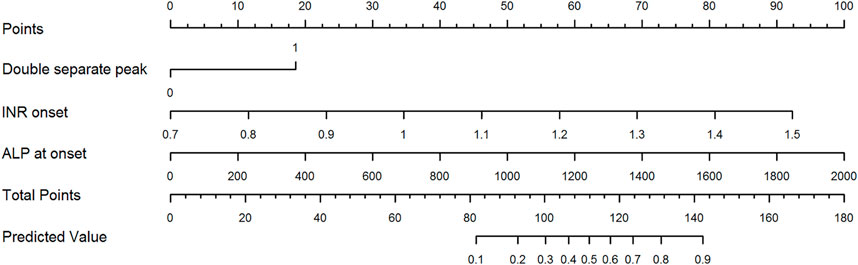
FIGURE 4. Optimized double separate peak model with INR and ALP at DILI onset. Points are assigned for double separate peak, INR, and ALP at DILI onset using the linear points scale at the top of the figure. The risk of acute liver failure correlating with the total points is on the two linear scales at the bottom of the figure. Abbreviations: ALP, alkaline phosphatase; INR, international normalized ratio.
Then, we investigated the predictive potency for ALF of the incorporated DSP model with INR and ALP at DILI onset. In the discovery cohort, the AUROC of the optimized model was found to be 0.899 (95% CI: 0.872–0.922), with a sensitivity of 75.0% and a specificity of 90.5%, which was superior to the DSP model alone and the three previous models (Figure 3; Table 3). Internal validation by bootstrap shows that the AUROC was 0.892 with a Brier score of 0.038. The AUROC and Brier scores in the independent validation cohort were 0.958 (95% CI: 0.933–0.975) and 0.022, respectively. Additionally, the AUROC in DILI with NAFLD patients of the optimized model was 0.896 (95% CI: 0.818–0.948), with a sensitivity of 100.0% and a specificity of 81.3%. The value of this new model in the subgroup of DILI with NAFLD has similar predictive potency compared to all DILI patients in terms of ALF.
Comparison of Pathological Classification According to Alanine Aminotransferase-Total Bilirubin Dynamic Evolution Patterns
A total of 227 patients enrolled in the discovery cohort underwent liver biopsy during hospitalization. Histological injury patterns were shown in Figure 5. The patients with the DSP pattern had predominantly cholestatic hepatitis (75.0%), which was significantly higher than that in the ALT-mono-peak pattern (p < 0.05) (Supplementary Table S3). The histological degree of moderate or severe and severe necrosis trended higher in the DSP pattern (29.2%) than in the ALT-mono-peak (11.8), TB-mono-peak (20.0), and DOP (23.2%) patterns.
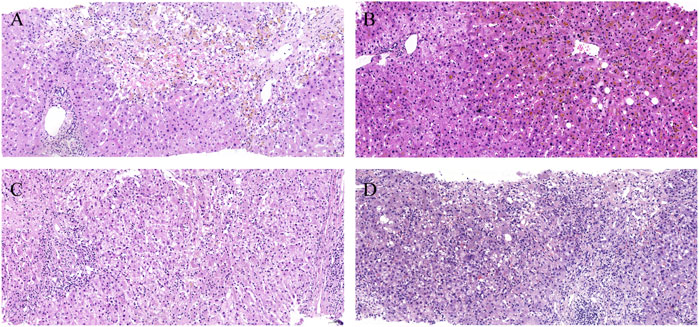
FIGURE 5. Histological injury patterns. (A) Histological acute hepatitis pattern (lobular necroinflammation with cholestasis) mainly correlated with the ALT-mono-peak pattern. (B) Histological acute cholestasis pattern (hepatocellular and canalicular cholestasis without obvious necroinflammation) mainly correlated with the TB-mono-peak pattern. (C) Histological cholestatic hepatitis pattern (mild to moderate lobular necroinflammation with mild cholestasis) mainly correlated with the ALT and TB double overlap peak pattern. (D) Histological cholestatic hepatitis pattern (moderate to severe lobular necroinflammation with moderate cholestasis) mainly correlated with ALT and TB double separate peak patterns. Abbreviations: ALT, alanine aminotransferase; TB, total bilirubin.
Discussion
DILI is the leading cause of ALF in Europe and North America (Stravitz and Lee, 2019) and the third leading cause in China (You et al., 2013; Medina-Caliz et al., 2016). Timely and accurate identification of DILI-induced ALF is the prerequisite for improving the prognosis. Currently, the prognostic models of DILI, such as Hy’s law, nHy’s law, and Robles-Diaz Model, are based on single-point biochemical markers at onset or peak time. In this study, we categorized the dynamic evolution patterns of ALT-TB after DILI onset into four patterns: ALT-mono-peak pattern, TB-mono-peak pattern, DOP pattern, and DSP pattern. The patients with the DSP pattern had significantly more severe disease with a significantly longer hospital stay than other patterns, with an AUROC for predicting DILI-induced ALF of 0.720 (95% CI: 0.682–0.756), a sensitivity of 0.79, and a specificity of 0.66. When we incorporated ALP and INR at DILI onset to the new dynamic model, the AUROC of the optimized model was 0.899 (95% CI: 0.872–0.922) with improvement in both sensitivity and specificity. Furthermore, our results had been validated by an independent external DILI cohort.
The advantages of the new model are the following: first, the most common liver biochemical parameters, namely, ALT and TB, were used—this was simple, not restricted by region, and especially useful in developing countries. The prediction potency can be further improved if incorporated with INR and ALP at DILI onset. Second, we defined the criteria for the dynamic biochemical patterns. DILI patients would usually have the results of a set of their liver biochemical tests before admission. According to these biochemical data, we can easily determine whether ALT or TB is solely or doubly elevated, that is, the mono-peak or double peak patterns. When the liver biochemical tests are checked again during hospitalization, any further increase in TB would implicate the DSP pattern. In the enrolled patients, 86.0% of the DSP pattern was confirmed within 1 week of admission thus implying timely determination of the dynamic patterns without any delay.
In comparison with other predictive models, the DSP model had significantly higher predictive capability of ALF. This is due to it being based not only on the key parameters at onset or peak level but also on the changes within a short period of time, depicting the whole picture along with the natural course of DILI. The kinetics of liver biochemical markers have been used in prediction of treatment response in alcoholic hepatitis, (Rachakonda et al., 2020), chronic hepatitis C (pegylated interferon and ribavirin) (Lee et al., 1998), and acute severe autoimmune hepatitis (Rahim et al., 2020). In the study, we employed them in the prediction of ALF in DILI.
Furthermore, our data showed that DILI patients with or without underlying NAFLD had similar clinical outcomes in terms of ALF and liver-related death/LT. However, the recent guideline suggested that the patients with NASH rather than NAFLD may have an increased risk of severe liver injury and adverse outcome (Regev et al., 2019). Since it is not possible to biopsy all patients with underlying NAFLD to discriminate between NAFL and NASH, the well-accepted non-invasive markers for this discrimination are highly warranted in the field.
Liver pathology determines the severity of liver injury by the lesion characteristic, injury degree, and the regeneration mode, which determines the clinical severity of DILI22 (Kleiner, 2018; Kleiner, 2017). Comparison of the histological patterns of DILI according to the various peak patterns revealed that the DSP pattern was associated with more instances of acute cholestatic hepatitis with a higher degree of necrosis and greater cholestasis. The results from the SADRAC database (Robles-Diaz et al., 2014) have shown that the extent of necrosis predicts low survival. Similarly, liver failure and death are associated with more severe necrosis (Robles-Diaz et al., 2014). This histologically accounts for why the DSP pattern is associated with more severe liver injury and hence more likely to be predictive of poorer outcome.
In the study, we proposed, optimized, and validated a novel model which can predict acute ALF after DILI onset with fairly good potency. However, enrolled cases of this study are all hospitalized patients, which are not representative of the entire DILI population. In order to overcome this limitation, we are planning to enroll a prospective DILI cohort to validate the model in a future study as well as the subgroup of DILI with NAFLD (Zhou et al., 2021).
Conclusion
The dynamic evolution patterns of ALT and TB are correlated with the prognosis of DILI. Patients with the DSP pattern (ALT ≥ 3 × ULN, TB ≥ 2.5 × ULN, with the peak time interval between ALT and TB being ≥3 days) are more likely to progress to ALF. The prediction potency of the model can be further improved by incorporating with INR and ALP at DILI onset so that extra care can be implemented in time for improving the outcomes in patients with DILI.
Data Availability Statement
The datasets presented in this article are not readily available because the datasets generated and/or analyzed during the current study are not publicly available due to the local policy but are available from the corresponding author on reasonable request. Requests to access the datasets should be directed to emhhb194aW55YW5AY2NtdS5lZHUuY24=.
Ethics Statement
The studies involving human participants were reviewed and approved by the Medical Ethics Committee of Beijing Friendship Hospital, Capital Medical University (Approval No. 2020-P2-071-01). The informed consent form has been waived.
Author Contributions
Study concept and design: XZ. Acquisition of data: RY, CZ, KL, YW, LL, YW, ZM, TW, JL, AH, YS, BC, and QL. Statistical Analysis and interpretation of data: CZ, KL, RY, and ML. Drafting of the manuscript: KL, CZ, and RY. Critical revision of the manuscript for important intellectual content: XZ, ZZ, JJ, AW, and JL. Obtained funding and study supervision: ML, YW, ZZ, and XZ.
Funding
This work was funded by grants from the National Natural Science Foundation of China-Youth Science Fund (No. 82103902; 81900526) and the China Hepatitis Prevention and Treatment Foundation of Wang Baoen Liver Fibrosis Research Fund (No. 2021060).
Conflict of Interest
The authors declare that the research was conducted in the absence of any commercial or financial relationships that could be construed as a potential conflict of interest.
Publisher’s Note
All claims expressed in this article are solely those of the authors and do not necessarily represent those of their affiliated organizations or those of the publisher, the editors, and the reviewers. Any product that may be evaluated in this article or claim that may be made by its manufacturer is not guaranteed or endorsed by the publisher.
Supplementary Material
The Supplementary Material for this article can be found online at: https://www.frontiersin.org/articles/10.3389/fphar.2022.934467/full#supplementary-material
References
Aithal, G. P., Watkins, P. B., Andrade, R. J., Larrey, D., Molokhia, M., Takikawa, H., et al. (2011). Case Definition and Phenotype Standardization in Drug-Induced Liver Injury. Clin. Pharmacol. Ther. 89, 806–815. doi:10.1038/clpt.2011.58
Andrade, R. J., Lucena, M. I., Fernández, M. C., Pelaez, G., Pachkoria, K., García-Ruiz, E., et al. (2005). Drug-induced Liver Injury: an Analysis of 461 Incidences Submitted to the Spanish Registry over a 10-year Period. Gastroenterology 129, 512–521. doi:10.1016/j.gastro.2005.05.006
Andrade, R. J., and Robles-Díaz, M. (2020). Diagnostic and Prognostic Assessment of Suspected Drug-Induced Liver Injury in Clinical Practice. Liver Int. 40, 6–17. doi:10.1111/liv.14271
Björnsson, E., and Olsson, R. (2005). Outcome and Prognostic Markers in Severe Drug-Induced Liver Disease. Hepatology 42, 481–489. doi:10.1002/hep.20800
Chalasani, N., Fontana, R. J., Bonkovsky, H. L., Watkins, P. B., Davern, T., Serrano, J., et al. (2008). Causes, Clinical Features, and Outcomes from a Prospective Study of Drug-Induced Liver Injury in the United States. Gastroenterology 135, 1924–1934. e1-4. doi:10.1053/j.gastro.2008.09.011
Danan, G., and Teschke, R. (2015). RUCAM in Drug and Herb Induced Liver Injury: The Update. Int. J. Mol. Sci. 17, 14. doi:10.3390/ijms17010014
Dennis, B. B., Naji, L., Jajarmi, Y., Ahmed, A., and Kim, D. (2021). New Hope for Hepatitis C Virus: Summary of Global Epidemiologic Changes and Novel Innovations over 20 Years. World J. Gastroenterol. 27, 4818–4830. doi:10.3748/wjg.v27.i29.4818
European Association for the Study of the Liver (2017). EASL 2017 Clinical Practice Guidelines on the Management of Hepatitis B Virus Infection. J. Hepatol. 67:370–398.doi:10.1016/j.jhep.2017.03.021
Hayashi, P. H., Rockey, D. C., Fontana, R. J., Tillmann, H. L., Kaplowitz, N., Barnhart, H. X., et al. (2017). Death and Liver Transplantation within 2 Years of Onset of Drug-Induced Liver Injury. Hepatology 66, 1275–1285. doi:10.1002/hep.29283
Khandelwal, N., James, L. P., Sanders, C., Larson, A. M., and Lee, W. M. (2011). Unrecognized Acetaminophen Toxicity as a Cause of Indeterminate Acute Liver Failure. Hepatology 53, 567–576. doi:10.1002/hep.24060
Kleiner, D. E., Chalasani, N. P., Lee, W. M., Fontana, R. J., Bonkovsky, H. L., Watkins, P. B., et al. (2014). Hepatic Histological Findings in Suspected Drug-Induced Liver Injury: Systematic Evaluation and Clinical Associations. Hepatology 59, 661–670. doi:10.1002/hep.26709
Kleiner, D. E. (2017). Drug-induced Liver Injury: The Hepatic Pathologist's Approach. Gastroenterol. Clin. North Am. 46, 273–296. doi:10.1016/j.gtc.2017.01.004
Kleiner, D. E. (2018). Histopathological Challenges in Suspected Drug-Induced Liver Injury. Liver Int. 38, 198–209. doi:10.1111/liv.13584
Lee, W. M., Reddy, K. R., Tong, M. J., Black, M., van Leeuwen, D. J., Hollinger, F. B., et al. (1998). Early Hepatitis C Virus-RNA Responses Predict Interferon Treatment Outcomes in Chronic Hepatitis C. Hepatology 28, 1411–1415. doi:10.1002/hep.510280533
Lo Re, V., Haynes, K., Forde, K. A., Goldberg, D. S., Lewis, J. D., Carbonari, D. M., et al. (2015). Risk of Acute Liver Failure in Patients with Drug-Induced Liver Injury: Evaluation of Hy's Law and a New Prognostic Model. Clin. Gastroenterol. Hepatol. 13, 2360–2368. doi:10.1016/j.cgh.2015.06.020
Medina-Caliz, I., Robles-Diaz, M., Garcia-Muñoz, B., Stephens, C., Ortega-Alonso, A., Garcia-Cortes, M., et al. (2016). Definition and Risk Factors for Chronicity Following Acute Idiosyncratic Drug-Induced Liver Injury. J. Hepatol. 65, 532–542. doi:10.1016/j.jhep.2016.05.003
Polson, J., and Lee, W. M. (2005). AASLD Position Paper: the Management of Acute Liver Failure. Hepatology 41, 1179–1197. doi:10.1002/hep.20703
Rachakonda, V., Bataller, R., and Duarte-Rojo, A. (2020). Recent Advances in Alcoholic Hepatitis. F1000Res 9, F1000. doi:10.12688/f1000research.20394.1
Rahim, M. N., Miquel, R., and Heneghan, M. A. (2020). Approach to the Patient with Acute Severe Autoimmune Hepatitis. JHEP Rep. 2, 100149. doi:10.1016/j.jhepr.2020.100149
Regev, A., Palmer, M., Avigan, M. I., Dimick-Santos, L., Treem, W. R., Marcinak, J. F., et al. (2019). Consensus: Guidelines: Best Practices for Detection, Assessment and Management of Suspected Acute Drug-Induced Liver Injury during Clinical Trials in Patients with Nonalcoholic Steatohepatitis. Aliment. Pharmacol. Ther. 49, 702–713. doi:10.1111/apt.15153
Reuben, A., Tillman, H., Fontana, R. J., Davern, T., McGuire, B., Stravitz, R. T., et al. (2016). Outcomes in Adults with Acute Liver Failure between 1998 and 2013: An Observational Cohort Study. Ann. Intern Med. 164, 724–732. doi:10.7326/M15-2211
Robles-Diaz, M., Lucena, M. I., Kaplowitz, N., Stephens, C., Medina-Cáliz, I., González-Jimenez, A., et al. (2014). Use of Hy's Law and a New Composite Algorithm to Predict Acute Liver Failure in Patients with Drug-Induced Liver Injury. Gastroenterology 147, 109–118. e5. doi:10.1053/j.gastro.2014.03.050
Sgro, C., Clinard, F., Ouazir, K., Chanay, H., Allard, C., Guilleminet, C., et al. (2002). Incidence of Drug-Induced Hepatic Injuries: a French Population-Based Study. Hepatology 36, 451–455. doi:10.1053/jhep.2002.34857
Shen, T., Liu, Y., Shang, J., Xie, Q., Li, J., Yan, M., et al. (2019). Incidence and Etiology of Drug-Induced Liver Injury in Mainland China. Gastroenterology 156, 2230. e11. doi:10.1053/j.gastro.2019.02.002
Stravitz, R. T., and Lee, W. M. (2019). Acute Liver Failure. Lancet 394, 869–881. doi:10.1016/S0140-6736(19)31894-X
Suk, K. T., Kim, D. J., Kim, C. H., Park, S. H., Yoon, J. H., Kim, Y. S., et al. (2012). A Prospective Nationwide Study of Drug-Induced Liver Injury in Korea. Am. J. Gastroenterol. 107, 1380–1387. doi:10.1038/ajg.2012.138
Temple, R. (2006). Hy's Law: Predicting Serious Hepatotoxicity. Pharmacoepidemiol Drug Saf. 15, 241–243. doi:10.1002/pds.1211
Vega, M., Verma, M., Beswick, D., Bey, S., Hossack, J., Merriman, N., et al. (2017). The Incidence of Drug- and Herbal and Dietary Supplement-Induced Liver Injury: Preliminary Findings from Gastroenterologist-Based Surveillance in the Population of the State of Delaware. Drug Saf. 40, 783–787. doi:10.1007/s40264-017-0547-9
Wang, T., Zhao, X., Shao, C., Ye, L., Guo, J., Peng, N., et al. (2019). A Proposed Pathologic Sub-classification of Drug-Induced Liver Injury. Hepatol. Int. 13, 339–351. doi:10.1007/s12072-019-09940-9
You, S., Rong, Y., Zhu, B., Zhang, A., Zang, H., Liu, H., et al. (2013). Changing Etiology of Liver Failure in 3,916 Patients from Northern China: a 10-year Survey. Hepatol. Int. 7, 714–720. doi:10.1007/s12072-013-9424-5
Yu, Y. C., Mao, Y. M., Chen, C. W., Chen, J. J., Chen, J., Cong, W. M., et al. (2017). CSH Guidelines for the Diagnosis and Treatment of Drug-Induced Liver Injury. Hepatol. Int. 11, 221–241. doi:10.1007/s12072-017-9793-2
Zhou, Y. J., Wong, V. W., and Zheng, M. H. (2021). Consensus Scoring Systems for Nonalcoholic Fatty Liver Disease: an Unmet Clinical Need. Hepatobiliary Surg. Nutr. 10, 388–390. doi:10.21037/hbsn-21-80
Glossary
AASLD American Association for the Study of Liver Diseases
ALB albumin
ALF acute liver failure
ALP alkaline phosphatase
ALT alanine aminotransferase
AST aspartate aminotransferase
AUROC area under receiver operating characteristic
BMI body mass index
CHE cholinesterase
CK7 cytokeratin 7
CIOMS Council for International Organizations of Medical Sciences
CI confidence interval
Cr creatinine
DILI drug-induced liver injury
DILIN drug-induced liver injury network
DOP ALT and TB double overlap peak pattern
DSP ALT and TB double separate peak pattern
GGT gamma glutamyltransferase
HDS herbal and dietary supplements
H&E hematoxylin and eosin
HGB hemoglobin
HIS hospital management system
INR international normalized ratio
LT liver transplantation
NAFLD non-alcoholic fatty liver disease
nHy’s Law new Hy’s Law
NSAIDs non-steroidal anti-inflammatory drugs
PAS-D periodic acid-Schiff with diastase
PLT platelets
ROC receiver operating characteristic
RUCAM Roussel Uclaf causality assessment method
SADRAC Swedish Adverse Drug Reactions Advisory Committee
TBA total bile acid
TB total bilirubin
ULN upper limit of normal
WBC white blood cell
Keywords: drug toxicity, predictive model, dynamic evolution pattern, clinical characteristic, clinical outcome
Citation: Yang R, Li K, Zou C, Wee A, Liu J, Liu L, Li M, Wu T, Wang Y, Ma Z, Wang Y, Liu J, Huang A, Sun Y, Chang B, Liang Q, Jia J, Zou Z and Zhao X (2022) Alanine Aminotransferase and Bilirubin Dynamic Evolution Pattern as a Novel Model for the Prediction of Acute Liver Failure in Drug-Induced Liver Injury. Front. Pharmacol. 13:934467. doi: 10.3389/fphar.2022.934467
Received: 02 May 2022; Accepted: 08 June 2022;
Published: 22 July 2022.
Edited by:
Laura Grasa, University of Zaragoza, SpainReviewed by:
Hartmut Jaeschke, University of Kansas Medical Center Research Institute, United StatesMing-Hua Zheng, First Affiliated Hospital of Wenzhou Medical University, China
Copyright © 2022 Yang, Li, Zou, Wee, Liu, Liu, Li, Wu, Wang, Ma, Wang, Liu, Huang, Sun, Chang, Liang, Jia, Zou and Zhao. This is an open-access article distributed under the terms of the Creative Commons Attribution License (CC BY). The use, distribution or reproduction in other forums is permitted, provided the original author(s) and the copyright owner(s) are credited and that the original publication in this journal is cited, in accordance with accepted academic practice. No use, distribution or reproduction is permitted which does not comply with these terms.
*Correspondence: Xinyan Zhao, emhhb194aW55YW5AY2NtdS5lZHUuY24=
†These authors share first authorship