- 1Department of Pharmacy, Ruijin Hospital, School of Medicine, Shanghai Jiaotong University, Shanghai, China
- 2Department of Pharmaceutical Sciences, University of Michigan, Ann Arbor, MI, United States
- 3Department of Clinical Pharmacology, Nanjin Jinling Hospital, Nanjing, China
- 4Department of Clinical Pharmacy and Pharmaceutical Management, School of Pharmacy, Fudan University, Shanghai, China
Objective: We aimed to establish a population pharmacokinetic (PPK) model for isoniazid (INH) and its major metabolite Acetylisoniazid (AcINH) in healthy Chinese participants and tuberculosis patients and assess the role of the NAT2 genotype on the transformation of INH to AcINH. We also sought to estimate the INH exposure that would achieve a 90% effective concentration (EC90) efficiency for patients with various NAT2 genotypes.
Method: A total of 45 healthy participants and 157 tuberculosis patients were recruited. For healthy subjects, blood samples were collected 0–14 h after administration of 300 mg or 320 mg of the oral dose of INH; for tuberculosis patients who received at least seven days therapy with INH, blood samples were collected two and/or six hours after administration. The plasma concentration of INH and AcINH was determined by the reverse-phase HPLC method. NAT2 genotypes were determined by allele-specific amplification. The integrated PPK model of INH and AcINH was established through nonlinear mixed-effect modeling (NONMEM). The effect of NAT2 genotype and other covariates on INH and AcINH disposition was evaluated. Monte Carlo simulation was performed for estimating EC90 of INH in patients with various NAT2 genotypes.
Results: The estimated absorption rate constant (Ka), oral clearance (CL/F), and apparent volume of distribution (V2/F) for INH were 3.94 ± 0.44 h−1, 18.2 ± 2.45 L⋅h−1, and 56.8 ± 5.53 L, respectively. The constant of clearance (K30) and the volume of distribution (V3/F) of AcINH were 0.33 ± 0.11 h−1 and 25.7 ± 1.30 L, respectively. The fraction of AcINH formation (FM) was 0.81 ± 0.076. NAT2 genotypes had different effects on the CL/F and FM. In subjects with only one copy of NAT2 *5, *6, and *7 alleles, the CL/F values were approximately 46.3%, 54.9%, and 74.8% of *4/*4 subjects, respectively. The FM values were approximately 48.7%, 63.8%, and 86.9% of *4/*4 subjects, respectively. The probability of target attainment of INH EC90 in patients with various NAT2 genotypes was different.
Conclusion: The integrated parent-metabolite PPK model accurately characterized the disposition of INH and AcINH in the Chinese population sampled, which may be useful in the individualized therapy of INH.
Introduction
Tuberculosis remains a serious public health concern in many countries (Ferguson and Rhoads, 2009; Sandhu, 2011). Although the incidence of tuberculosis has been decreasing recently, approximately 10 million cases, along with approximately 1.2 million deaths associated with the disease have been reported (Chakaya et al., 2021). China accounted for 8.4% of total global tuberculosis cases in 2019 (Chakaya et al., 2021). Isoniazid (INH), inhibits the synthesis of long-chain mycolic acids, which are indispensable components of mycobacterial cell walls. As such, it is an essential component of standard first-line prophylactic treatment/measures of tuberculosis (Winder and Collins, 1970; Takayama et al., 1972). INH is also effective in preventing resistance to co-administered anti-tuberculosis drugs (Mitchison, 1979). A fixed-dose combination (FDC) anti-tuberculosis medication is thought to be more effective and convenient, with less drug resistence occurring (Blomberg et al., 2001). The use of FDCs from different manufacturers may cause high pharmacokinetic (PK) variability, along with inter-individual differences in efficacy and toxicity of INH (Shishoo et al., 2001; McIlleron et al., 2006; Hao et al., 2014).
Therapeutic drug monitoring (TDM) of anti-tuberculosis drugs, including INH, can be used to ensure adequate treatment effect, as well as reduce toxic effects (Peloquin, 2002; Weiner et al., 2003; Zuur et al., 2016). A peak INH concentration (Cmax) of <3 μg ml−1 after a daily administration, or <9 μg ml−1 after biweekly administration is considered ineffective (Peloquin, 2002). Some other studies suggested that a minimum area of 10.52 μg⋅h⋅ml−1 under the concentration-time curve (AUC0–24) is a suitable marker of clinical efficacy (Donald et al., 2007; Kiser et al., 2012).
The majority of INH is acetylated by N-acetyltransferase (NAT2) to form acetylisoniazid (AcINH), acetylhydrazine, and other metabolites, and only approximately 7–30% of INH is excreted intact as the parent drug. Although there is no evidence on the toxicity of AcINH, it may be converted to other toxic metabolites. The ratio of AcINH and INH levels in plasma at the peak time is considered to be an index of INH toxicity (Timbrell et al., 1980). NAT2 activity exhibits remarkable polymorphism, primarily due to NAT2 genotypes. A total of seven single nucleotide polymorphisms (SNPs) have been determined in the human NAT2 gene, consisting of more than 27 different NAT2 alleles (Lauterburg et al., 1985a; Bell et al., 1993; Loktionov et al., 2002; Walker et al., 2009). Two of these SNPs are silent, and the other five lead to amino acid substitutions. Hein et al. found that the C341→T (rs1801280) of *5, G590→A (rs1799930) of *6, and G857→A (rs1799931) of *7 led to substitutions of Ile114→Thr, Arg197→Gln, Gly286→Glu in the NAT2 enzyme, respectively, leading to a remarkable decrease in NAT2 activity (Hein et al., 1994). NAT2 alleles *5, *6, and *7 comprise the most important factor of slow acetylators in Whites and Asisans. The frequencies of these alleles are different among various populations (Lin et al., 1993; Agundez et al., 1996; Chen et al., 2006). Polymorphism of NAT2 has been considered as the primary reason for inter-individual differences in PK and pharmacodynamics (PD), which may further influence the INH dosing regimen (Horai et al., 1982; Deguchi et al., 1990; Parkin et al., 1997; Denti et al., 2015).
Population PK (PPK) is suitable for characterizing the disposition of the drug in a large group of participants with limited observations for each. PPK has the ability to provide quantitative estimates of the inter- and intra-patient variability, and also to determine the influence of demographic, clinical, and genetic factors on the PKs. PPK is also used in the simulation of PKs of parent drugs and their metabolites simultaneously (Seng et al., 2015; Sundell et al., 2020). However, to our knowledge, no study has been published on the integrated PPK model of INH and AcINH in Chinese patients. Based on the PPK parameters of INH, and the distribution of minimum inhibitory concentration (MIC) in clinical isolates, the PK/PD model can be established to identify an optimal dosing regimen of INH, which is helpful in the appropriate treatment of tuberculosis (Pasipanodya and Gumbo, 2011).
The purpose of this study is to establish a PPK model for INH and its major metabolite AcINH in tuberculosis patients and healthy Chinese participants,and assess the influence of NAT2 polymorphism on the PK/PD of INH.
Materials and Methods
Subjects
All healthy participants were enrolled in two different PK studies of INH. In the first study, 24 healthy male subjects with different NAT2 genotypes were recruited from 215 participants with known NAT2 genotypes (Chen et al., 2009). In the second study, 21 healthy subjects were enrolled from a group that participated in a bioequivalence study. All subjects were Han nationals. Each subject was physically healthy and had no history of significant medical illness or hypersensitivity to any drugs. A physical examination was performed before the study. This included a complete blood chemistry, urinalysis, and an electrocardiogram. All subjects were drug-free for two weeks before, and during the study. The study protocol was approved by the Jinling Hospital Ethics Committee and all subjects gave written consent to participate.
A total of 157 tuberculosis patients were also recruited. The subjects consisted of 89 males and 68 females (age: 42.2 ± 11.5 y, weight: 56.5 ± 9.71 kg) patients. Sixty-seven patients had taken three anti-tuberculosis drugs simultaneously, 48 had consumed four drugs simultaneously, while consumed two drugs simultaneously. The drugs used in combination with INH were rifampicin, rifapentine, pyrazinamide, and ethambutol. Demographic and clinical data were recorded.
Study Protocol
For the first PK study, 24 healthy volunteers were enrolled in the study center at 8:00 p.m. on the day before the study began. After fasting overnight, three tablets of INH (100 mg/tablet, Qianjin Pharmaceutical Company, Hunan, China) were taken with 200 ml of water at 8:00 a.m. Food was provided four hours after the INH intake. Three ml of blood was drawn into a sterile anticoagulation tube just before the administration of INH, and 0.25, 0.5, 1, 1.5, 2, 3, 4, 6, 8, 10 and 14 h after.
In the second PK study, Rifater, containing INH 80 mg/tablet (Gruppo Lepetit SPA, Italy) was used as the reference formulation, and INH 80 mg/tablet or 80 mg/capsule (LanBen, Nanjing, China) was used as the test formulation. Twenty-one subjects received a single dose of 320 mg test or reference tablets or capsules after an overnight fast. Both formulations were administered with 200 ml water at 8:00 a.m. Standard meals were provided four and ten hours after drug administration. No other food or drinks containing Xanthine (e.g., tea, coffee, cola) were allowed for subjects during their time in the study center. A total of three ml of blood was collected via veins, and then transferred into sterile EDTA anticoagulation tube before dosing, and 0.25, 0.5, 1, 1.5, 1, 2, 4, 6, 9, 12 h after.
All patients received anti-tuberculosis therapy for 7–14 consecutive days before sample collection. For all patients, 3 ml of blood was drawn 2 and/or 6 h after INH administration. Blood samples were centrifuged at 1610 × g for 10 min. Since AcINH is not stable in plasma samples, plasma was immediately separated and stored at −70°C while awaiting further analysis.
Genotyping
NAT2 C341→T, G590→A, and G857→A represent *5, *6, and *7 alleles, respectively. These SNPs are sufficient for the reflection of the important NAT2 genotypes have impaction on NAT2 activity. We developed allele-specific PCR (ASA-PCR) to determine the three aforementioned SNPs (Chen et al., 2004). The method was designed based on the principles of amplification refractory mutation systems. Briefly stated, allele-specific primers for different NAT2 alleles were designed. ASA-PCR was performed using two tubes for the detection of the wild and mutant genotype of each allele. The primers were mixed with Taq DNA enzyme, dNTPs, Mg2+, buffer, and genomic DNA. Upon 30 amplification cycles, the NAT2 genotypes were analyzed after electrophoresis.
Quantification of INH and AcINH
A previously established reverse phase HPLC was used to determine plasma concentrations of INH and AcINH simultaneously (Cao et al., 2005). Perchloric acid was added to the plasma for precipitation of protein. The supernatant was separated after centrifugation and eluted with 2 mmol⋅L−1 sodium heptane sulfonate-acetonitrile (98:2) on a Lichrospher C18 column and detected at a wavelength of 266 nm. The calibration curve was linear in the range of 0.12–15.89 mg⋅L−1 (0.88–115.9 μmol⋅L−1) for INH and in the range of 0.13–17.08 mg⋅L−1 (0.72–95.4 μmol⋅L−1) for AcINH (r2 > 0.99).
Non-Compartmental Analysis
The PK parameters of INH and AcINH in healthy subjects were determined with the WinNonlin 5.01 (Pharsight Corp., Mountain View, CA, United States). Cmax and Tmax were obtained from observation. The Ke was calculated using least-square regression analysis performed using the terminal phase of the concentration–time curve. The AUC was calculated by using the trapezoidal method.
Compartmental Pharmacokinetic Analysis
Data Splitting
For the 202 subjects in the present study, 122 were assigned to the model-building set (index group) and 80 to the test set (validation group), respectively. The index group included 15 subjects with one full PK profile, 12 subjects with three full PK profiles, and 95 patients with sparse sampling data. The validation group includednine subjects with one full PK profile, nine with three full PK profiles, and 62 with sparse sampling data.
Model Development
PPK modeling of INH and AcINH was constructed based on the of modeling group data. The final PPK model was constructed by using data from both the modeling group and validation group. One- and two-compartment models for INH or AcINH were evaluated during model construction. The first absorption, with or without lag time, was also tested. NONMEM (Version 6, GloboMax, Hanover, MD) was used for the model construction. The first-order conditional estimation method (FOCE) was applied. Model selection was based on objective function value (OFV), parameter estimates, and standard error. OFV is proportional to −2 log likelihood of the relevant model. The distribution of empirical Bayes estimates and ability of the model to determine reasonable INH and AcINH concentration-time profiles were also important for model assessment.
Interindividual and Residual Error Model
The inter-individual variability of the parameters was assessed using an exponential function (Eq. 1)
Pi denotes the PK parameter value, θTV denotes the population value for the parameter described, and ηi denotes the random deviation of Pi from θTV. The values of ηi were assumed to be independently normally distributed with a mean of 0 and a variance of ω2.
A proportional model was used for residual error analysis of INH and AcINH as (Eq. 2)
Cobs is the observed concentration, Cpred is the predicted concentration, and ε is a residual error with a mean of 0 and a variance of σ2.
Covariates
The characteristics of the subjects, including body weight, age, clearance of creatinine (CLcr), aspartate aminotransferase (ASP), alanine aminotransferase (AST), and NAT2 genotype, were tested as covariates in the population analysis. The model of continuous covariates on the Pi was as follows (Eqs 3–6)
For categorical covariate of the NAT2 genotype, three methods representing NAT2 genotyping were tested:
1) Subjects were placed into extensive metabolizer (EM) and poor metabolizer (PM) groups according to the NAT2 genotype, where scores of “1” and “0” were assigned to the EM and PM subjects, respectively.
2) Subjects were characterized as homogenous wild type (wt/wt), heterozygous mutant (m/wt), and homogenous mutant (m/m) based on their genotypes. Scores of “0,” “1,” and “2” were assigned to the three groups, respectively. Pi was modeled according to the following equation (Eq. 7)
3) For each allele of *5, *6 and *7, scores of 0, 1 and 2 were assigned to the w/w, m/w, and m/m genotype. Hence, subjects with *4/*4, *4/*6, *4/*7, *6/*6, *6/*7, *7/*7, and *5/*7 genotype were assigned the scores of 0/0/0, 0/1/0, 0/0/1, 0/2/0, 0/1/1, 0/0/2, and 1/0/1, respectively. Pi was modeled according to the following equation (Eq. 8)
Various covariates were included in a stepwise manner, followed by a stepwise elimination for the final regression model. Changes in the OFV were used to approximate the χ2 distribution, with degrees of freedom (df) equal to the number of covariates introduced. When the OFV was reduced by 6.64 or higher (p < 0.01, df = 1), the covariate included was considered statistically significant. After all covariates significantly decreasing OFV were included, each covariate was fixed as zero in turn. This procedure was repeated until the value of the objective function failed to increase by more than 10.9 (p < 0.001, df = 1).
Data Analysis and Model Evaluation
The final model was tested by using a visual predictive check. A total of 1,000 new datasets for patients with different NAT2 genotypes were simulated based on estimation of the parameters with their inter- and intra-individual variety, and NAT2 genotypes of healthy subjects and tuberculosis patients. A 90% prediction interval was obtained by extracting the 5% and 95% quantiles of their simulated distributions. The quality of the evaluation was assessed by graphical presentation of the observed concentration vs. predictions. The stability and performance of the final model were also assessed through a nonparametric bootstrap, with resampling and replacement. In this study, 1,000 bootstrap samples were generated, and the PPK parameters were estimated for each sample using the final model.
Bayesian Estimation of AUC of INH
Based on the Bayesian approach, the POSTHOC subroutine of NONMEM without an estimation step (MAXEVAL = 0) was used to estimate the individual PK parameters of each patient in the validation group. The PPK parameters, inter-individual variation (IIV), and residual variability were set as the data obtained from the index group. Models of INH C2, C2-C4, and C2-C6 plasma concentration-time points following INH administration were selected as the Bayesian estimators for estimation of the AUC of the validation group. AUC0-24 was estimated through the equation: AUC0-24 = dose/(CL/F). AUC0-24 obtained from the Bayesian estimators was compared with observed values. The predictive performance was evaluated as bias and precision, which are expressed as MPE (%) and RMSE (%), respectively.
Monte Carlo Simulations
In line with previous studies, AUC0–24/MIC indicated a better PK/PD index compared with Cmax/MIC and TMIC. The 90% maximal kill Mycobacterium tuberculosis (90% effective concentration, EC90) is considered the therapeutic target of INH. AUC0–24/MIC that mediates EC90 was 567 (Gumbo et al., 2007). The MIC of INH was obtained from previous studies on Mycobacterium tuberculosis strains (MIC values were 0.01, 0.02, 0.05, 0.10, and 0.25 μg/ml) (Zhao and Lu, 2019). The INH dosing regimen included 300, 600, and 900 mg daily. AUC0–24 of INH was estimated based on the integrated PPK model with estimated uncertainty (standard error). The simulation was performed with the data of 10,000 subjects with various NAT2 genotypes using the Monte Carlo method (Gumbo. 2010). The probability of target attainment (PTA) of patients with various NAT2 genotypes was summarized based on the estimated proportions of isolates.
Results
INH and AcINH in Relation to NAT2 Genotypes
The demographic and laboratory test data of all subjects obtained are shown in Table 1. In the first PK study performed with 24 healthy subjects, five different NAT2 genotypes were observed: *4/*4 (8), *4/*6 (6), *4/*7 (2), *5/*7 (1), and *6/*6 (7). A total of eight subjects were characterized with a wt/wt genotype (*4/*4);eight with m/wt genotype (*4/*6 and *4/*7); 8 subjects with m/m genotype (*5/*7 and *6/*6). There was a significant difference in INH PK parameters among various groups. The Cmax of INH in the wt/wt, m/wt, and m/m groups were 36.0 ± 13.5, 41.7 ± 14.2, and 60.4 ± 17.5 μmol⋅L−1, and AUC0–14h of INH in different groups were 71.5 ± 16.8, 115.6 ± 21.9, and 274.9 ± 55.5 μmol⋅h⋅L−1 (p < 0.001), respectively (Table 2). A significant difference was observed among various NAT2 genotypes.
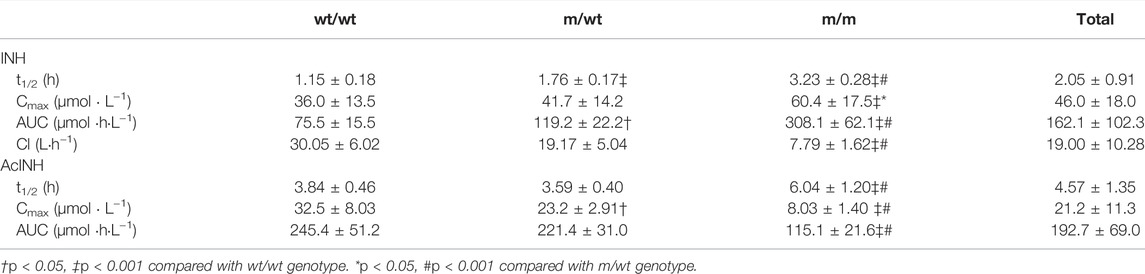
TABLE 2. Pharmacokinetic parameters of INH and AcINH in Chinese healthy subjects with different NAT2 genotypes.
For the bioequivalence study performed with 21 healthy subjects who received 320 mg of INH, five different NAT2 genotypes were observed, namely, *4/*4 (11), *4/*6 (6), *4/*7 (2), *4/*5 (1), and *6/*6 (1). A total of 11, 9, and 1 subjects were included in the NAT2 wt/wt, m/wt, and m/m groups, respectively. The INH Cmax in the wt/wt, m/wt, and m/m groups were 32.4 ± 13.7, 42.6 ± 11.0, and 71.6 μmol⋅L−1, and AUC0–24h of INH in different groups were 55.2 ± 10.1, 97.7 ± 16.7, and 319.9 μmol⋅h⋅L−1 (p < 0.001), respectively.
For 157 tuberculosis patients, seven different NAT2 genotypes including *4/*4 (n = 72), *4/*5 (n = 6), *4/*6 (n = 31), *4/*7 (n = 25), *6/*6 (n = 12) and *7/*7 (n = 4), *5/*7 (n = 2), and *6/*7 (n = 5) were observed. A total of 72 (45.8%) patients with *4/*4 (wt/wt) genotype and 23 patients (14.6%) with *5/*7, *6/*7, *6/*6 or *7/*7 genotypes were classified in the m/m group. The other 62 patients (39.5%) were classified in the m/wt group. The concentrations of INH 2 h (n = 109) after the administration in the wt/wt, m/wt, and m/m groups were 22.8 ± 17.3, 28.2 ± 23.2, and 46.8 ± 28.8 μmol⋅L−1 (p < 0.001), and the concentrations of AcINH in the three groups were 35.0 ± 18.8, 21.5 ± 15.8, and 11.9 ± 8.64 μmol⋅L−1 (p < 0.001), respectively. The concentrations of INH 6 h (n = 52) after administration in the wt/wt, m/wt, and m/m groups were 3.96 ± 1.74, 6.69 ± 3.56, and 24.2 ± 9.95 μmol⋅L−1 (p < 0.001), and the concentrations of AcINH in the three groups were 21.3 ± 13.5, 18.1 ± 15.2, and 9.87 ± 3.35 μmol⋅L−1 (p = 0.034), respectively.
Integrated Model of INH and AcINH
An integrated model describing INH and AcINH simultaneously was developed. The graphical representation of the model is provided in Figure 1. The structural model consisted of a one-compartment model obtained by first-order elimination process for both INH and AcINH. The PPK parameters of INH and AcINH are presented in Table 3. For the basic model, the Ka of INH was estimated to be 3.94 ± 0.44 h−1. The clearance of INH (CL/F) was estimated to be 18.2 ± 2.45 L⋅h−1, K30 (constant of clearance of AcINH) was 0.33 ± 0.11 h−1, Vd/F values of INH (V2) and AcINH (V3) were 56.8 ± 5.53 L and 25.7 ± 1.30 L, respectively, and the FM was 0.81 ± 0.076.
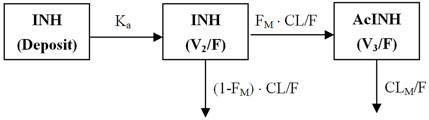
FIGURE 1. Schematic figure of the integrated PPK model of isoniazid (INH) and acetylisoniazid (AcINH). Ka: The absorption rate constant of INH. F: The oral bioavailability of INH. CL/F: clearance of INH. FM: The fraction of INH converted to AcINH. CLM/F: clearance of AcINH. V2 and V3: volume of distribution of INH and AcINH.
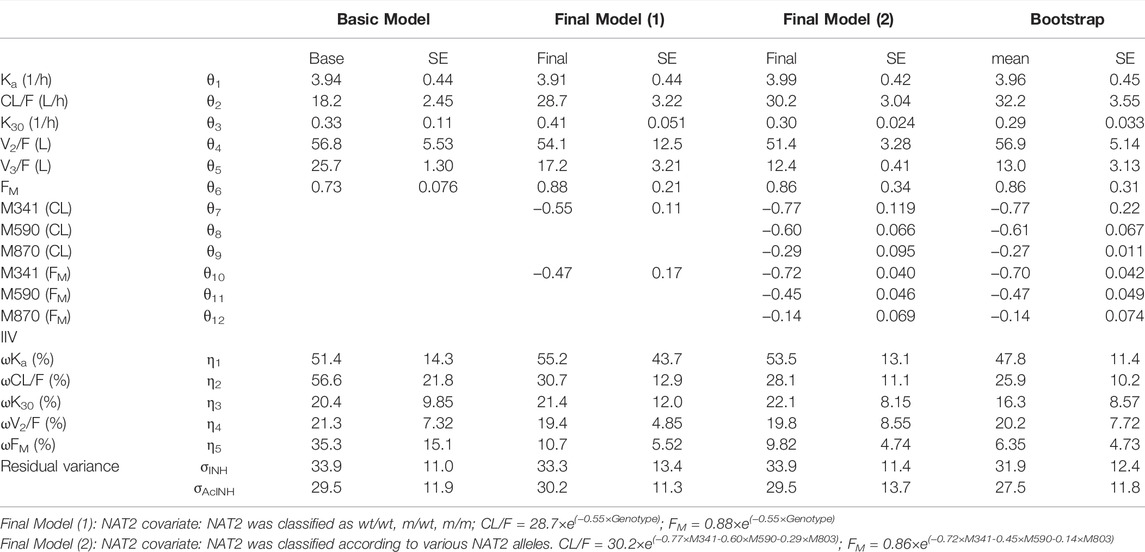
TABLE 3. Parameters of integrated PPK model of INH and AcINH in Chinese healthy subjects and tuberculosis patients.
After the forward inclusion and backward elimination step, only the effect of NAT2 genotypes remained in the model. When the subjects were characterized with the genotypes of wt/wt, m/wt, or m/m or as EM and PM, the ΔOFV values were -275.3 and -261.4, respectively. When *5, *6 and *7 alleles were used as covariates separately, the OFV value demonstrated the most significant reduction (ΔOFV was −315.5). In subjects with only one copy of NAT2 *5, *6, and *7 alleles, the CL/F values were approximately 46.3%, 54.9%, and 74.8% of *4/*4 subjects, and FM values were approximately 48.7%, 63.8%, and 86.9% of *4/*4 subjects. After inclusion of the NAT2 genotype as a covariate, IIV of CL/F and FM values were reduced from 56.6% to 28.1%, and 35.3% to 9.82%, respectively.
The goodness of fit plots of the population predicted (PRED) and individual predicted (IPRE) vs. observed concentrations (CONC) of the integrated model and the individual weighted residuals (WRES) vs. PRED and WRES vs. time for INH and AcINH are presented in Figures 2, 3, respectively. The higher bias of INH is distributed in the first two hours. Hence, it seems that the absorption phase was not effectively estimated. The residual errors for INH and AcINH were 33.9% and 29.5%, respectively.
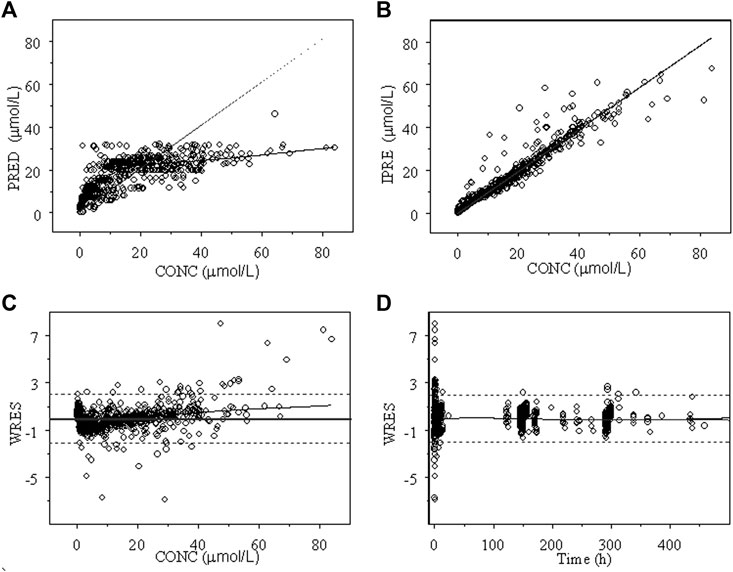
FIGURE 2. Goodness of fit of INH from the final integrated PPK model in Chinese healthy subjects and tuberculosis patients. (A) Population predicted concentration (PRED) vs. observed concentration (CONC); (B) individual predicted concentration (IPRE) vs. DV; (C) weighted residual error (WRES) vs. CONC; (D) WRES vs. time.
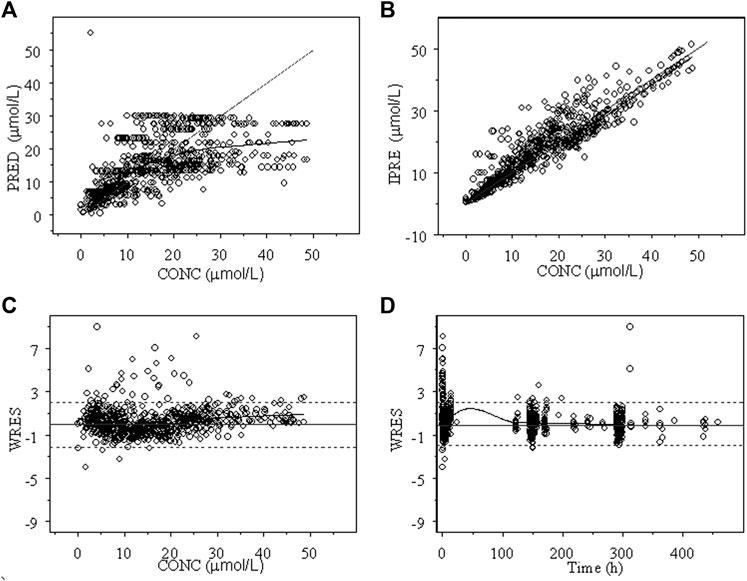
FIGURE 3. Goodness of fit of AcINH from the final integrated PPK model in Chinese healthy subjects and tuberculosis patients. (A) Population predicted concentration (PRED) vs. observed concentration (CONC); (B) individual predicted concentration (IPRE) vs. DV; (C) weighted residual error (WRES) vs. CONC; (D) WRES vs. time.
VPC from the data set simulated using the final modelisshown in Figure 4. The predicted medians and 5th and 95th data percentiles were similar to the observed medians and 90th percentiles for INH and AcINH. Bootstrap runs were performed successfully 89.7 percent (897 of 1000) of the time similar to the 95% CI calculated by NONMEM.
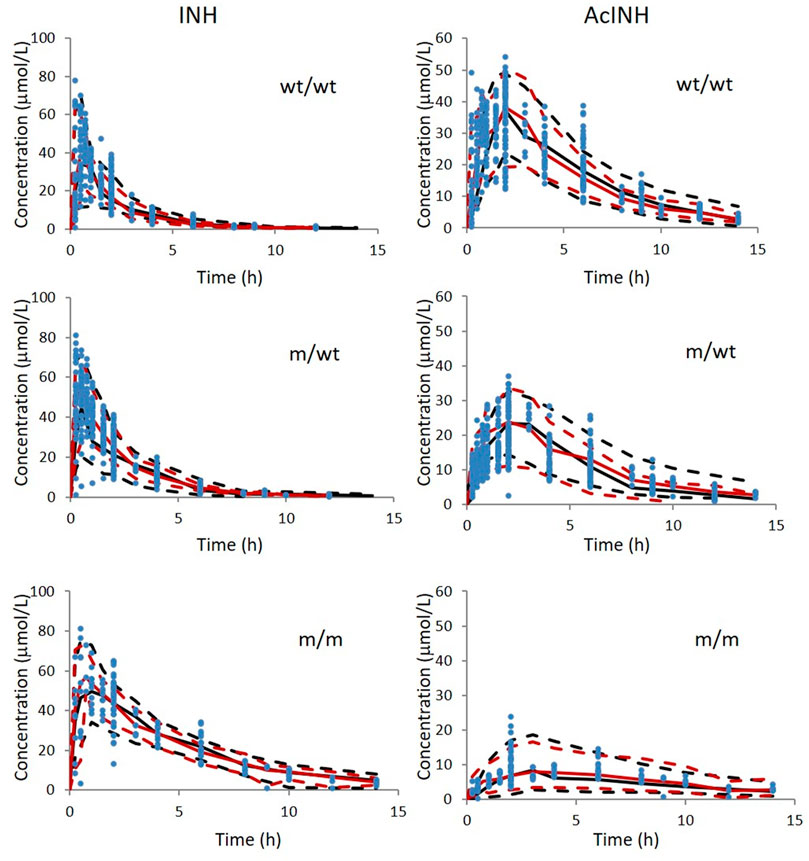
FIGURE 4. Visual predictive check of INH and AcINH based on the integrated PPK model in Chinese healthy subjects and tuberculosis patients with therapy of INH. The red and black sold lines represent 50th of observed data and simulated data; The upper and lower red dashed lines represent 95th and 5th of observed data; The upper and lower black dashed lines represent 95th and 5th of the simulated data. The blue solid cycles represent observed data wt/wt: NAT2 homogenous wild type; m/wt: NAT2 heterozygous mutant genotype; m/m: homogenous mutant genotype.
Bayesian Estimator
Using Bayesian estimation, the individually predicted INH and AcINH concentrations of the validation group (80 patients, 302 points) by correlated well with observed data. The MPE (95% CI) and MRSE % (95% CI) values were −1.16% (−4.35%, 6.68%) and 27.7% (22.2%, 33.2%) for INH and 2.46% (−0.39%, 5.31%) and 28.6% (23.0%, 34.3%) for AcINH, respectively.
Based on Bayesian method, CL/F of INH obtained from the index group was used to predict AUC0–24 in the validation group. The sampling strategies consisted of observation of plasma levels at 2 h (C2), 2 and 4 h (C2-C4), 2 and 6 h (C2-C6) after INH administration (Table 4). The AUC0–24 values of various strategies were 143.4 ± 85.6 μmol⋅h/L (19.7 ± 11.7 μg⋅h/ml) and 122.0 ± 88.1 μmol⋅h/L (16.7 ± 12.1 μg⋅h/ml).
Monte Carlo Simulation for Patients With Various NAT2 Genotypes
Using the Monte Carlo simulation method, PTAs for patients with various NAT2 genotypes were estimated with various INH regimens. It seemed that with a daily regimen including 300 mg of INH, the PTA target could be obtained in patients with wt/wt and m/wt genotypes, with the MIC lower than 0.01 μg/ml. With a daily regimen involving administration of 600 mg INH, target PTA can be obtained with MIC values lower than 0.02 μg/ml in wt/wt and m/wt patients. For m/m patients, target PTA was obtained with MIC values of 0.02, 0.05, and 0.1 μg/ml with 300 mg INH dosing regimen. When patients were characterized with *4/*4, *4/*5, *4/*6, *4/*7, *7/*7, *5/*7, *6/*7, and *6/*6 genotype, differences in PTA among various m/m genotypes were also observed (Figure 5).
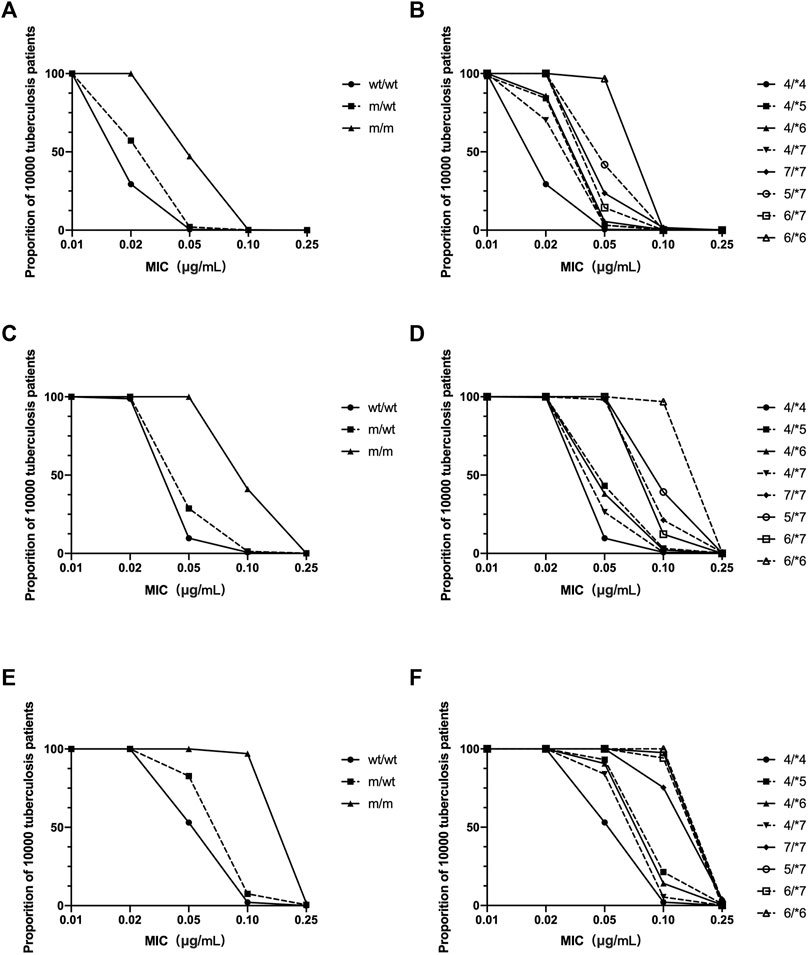
FIGURE 5. The estimated probabilities of target attainment in patients with various NAT2 genotype and various doing regimens by a Monte Carlo simulation method. INH dosing regimen: 300 mg daily (A,B); 600 mg daily (C,D); 900 mg daily (E,F). (A,C,E) NAT2 genotype was characterized as homogenous wild type (wt/wt), heterozygous mutant (m/wt), and homogenous mutant (m/m). (B,D,F) NAT2 genotype was characterized according to various NAT2 alleles.
Discussion
In this study, we established for the first time an integrated model for INH and AcINH, using the data derived from healthy Chinese subjects and Chinese tuberculosis patients. We found that various NAT2 alleles had different impact on INH and AcINH PKs. A Monte Carlo method was used to simulate INH dosing regimen for Chinese patients with different NAT2 genotypes.
Efficacy and adverse effects of INH and its metabolites in the anti-tuberculosis therapy has been demonstrated. The most important metabolite of INH is AcINH, which is converted to isonicotinic acid (INA) and acetylhydrazine (AcHz) (Boxenbaum and Riegelman, 1976; Lauterburg et al., 1985a). AcHz can be converted either to relatively non-toxic diacetylhydrazine, or a reactive intermediate metabolite through CYP2E1, which may cause toxic hepatitis. INH may also be hydrolyzed to hydrazine (Hz) and INA directly. Oxidation of Hz may also lead to transformation to hepatoxic metabolites; however, this pathway is considered a minor part of the metabolism process, especially in subjects with EM of NAT2 (Boxenbaum and Riegelman, 1976; Lauterburg et al., 1985b). For EM subjects, 7–9%, 39–43%, 0.4–1.6%, and 23% of INH and for PM subjects, 21–32%, 18–27%, 1.1–3.1%, and 3.1–5.1% of INH is excreted as INH, AcINH, AcHz, and diacetylhydrazine, respectively, 24 h after the administration of INH. In contrast, only 0.1–0.4% and 0.5–1.0% of INH is excreted as Hz in EM and PM subjects, respectively (Peretti et al., 1987). INH, AcINH, and its further metabolites account for the major amount of INH recovered from urine. Hence, PKs of INH and AcINH reflected the disposition of INH after administration in a Chinese population sample.
PPK models of INH in different populations have been developed. Both two-compartment (Zvada et al., 2014; Seng et al., 2015; Rodriguez et al., 2019; Jing et al., 2020; Sundell et al., 2020) and one-compartment models have been applied (Cojutti et al., 2017; Aruldhas et al., 2019). The Ka was 2.47–6.69 1/h, CL/F of various populations was 5.41–23.7 L/h, and Vd/F was 29.6–72.3 L. Models integrating INH and its metabolite are limited. Seng et al. (2015) established an integrated model for INH, AcINH and INA. A two-compartment model with the best description of the first-order absorption of INH PKs; A two- and one-compartment model was suitable for the description for AcINH and INA data, respectively. They found that NAT2 was a significant covariate for INH clearance, and creatinine clearance was a significant covariate for AcINH clearance. However, only healthy subjects were included in their study. In the present study, for the first time, an integrated model of INH and AcINH was established in both healthy Chinese subjects and tuberculosis patients . Most Chinese subjects could be classified as EM subjects. INH concentration cannot be obtained 12 h after INH administration in these subjects. We found that a one-compartment model was sufficient for most subjects, and the two-compartment model was suggested to be over parameterized. The final integrated model consisted of three compartments, including one deposit compartment and one compartment each for INH and AcINH (Figure 1).
The typical value of CL/F obtained from the integrated model was 18.2 ± 2.45 L⋅h−1, which was comparable with previous studies. CL/F of INH included two parts: INH converted to AcINH and direct elimination through urine (Figure 1). FM was used to reflect the part of INH converted to AcINH. It was a key parameter in the integrated model. Since in the present study, INH was administered orally and the part of INH excreted as the parent drug was not determined, FM could not be determined directly. It should be noted that the exact value of clearance (CLM/F) and Vd/F of AcINH is difficult to obtain. Using the present integrated model, CLM/F could be estimated by multiplying K30 with V3/F. The CLAcINH/F in the present study was estimated as 11.3 L⋅h−1, which was similar to that of previous studies.
Polymorphisms in NAT2 activity are responsible for the inter-individual differences in INH PKs (Donald et al., 2007; Seng et al., 2015; Sundell et al., 2020) (14, 29, 30). Most early studies on NAT2 phenotypes classified the subjects as EMs and PMs. Smith et al. found that the activity of NAT2 showed a trimodal distribution (Smith et al., 1997). Kita et al. found that the urinary recovery of INH was lower in healthy volunteers and tuberculosis patients with a higher number of active NAT2 alleles Kita et al. (2001). Kinzig-Schippers reported that the NAT2 genotypes accounted for 88% variation of INH clearance, which can be presented as: CL/F = 10 + 9 × (number of NAT2*4 alleles) (Kinzig-Schippers et al., 2005). Various NAT2 SNPs are related to decreased expression and enzyme instability. Hein et al. suggested that catalytic activity of recombinant NAT2 with 341C/C, 590A/A, and 857A/A genotypes were 10%, 19–42%, and 39–70% of that NAT2 wild type, respectively (Hein et al.,1994; Hein et al., 1995). It can be inferred that although both are classified as PM, the metabolic activity of NAT2 *7/*7 might be 3–6 times higher than that of *5/*5. In our previous study, the influence of various NAT2 SNPs on MR and INH PKs was analyzed using multiple regression. We found that *5(T341→C) allele had a more significant effect than *6(G590→A) and *7(G857→A) on MRSM2 and MRINH (Chen et al., 2009). The results showed that various NAT2 alleles had different impacts on NAT2 activity. In the present study, different NAT2 genotypes were tested as covariates of CL/F and FM. The results showed that OFV decreased, and the model simulation greatly improved. We found significant improvement upon classifying the NAT2 genotype as wt/wt, m/wt, or m/m, or classification according to various NAT2 alleles. In the final model, IIV of CL/F and FM was significantly decreased from the basic model (from 56.6% to 28.1%, and 35.3%–9.82%, respectively). CL/F values of *4/*7, *4/*6, *5/*7, and *6/*6 subjects were approximately 74.8%, 54.9%, 34.6%, and 30.1% of *4/*4 subjects. FM was 0.86 in *4/*4 subjects, which was 1.57 fold higher than that of the *4/*6 (0.55) subjects and more than two times higher than that of the *5/*7 (0.36) and *6/*6 (0.35) subjects. It can be deduced that more INH was converted to AcINH in subjects with more active NAT2 genes. In addition, various NAT2 mutant alleles have different influences on the PKs of INH and AcINH.
A combination of different anti-tuberculosis drugs is usually used for the treatment of tuberculosis; Hence, drug-drug interaction should be considered. According to previous research, rifamycins may induce several metabolic pathways, including CYP3A4, uridine diphosphate glucuronosyltransferases (UGTs), and many transporters. However, there is no evidence of the impact of rifamycin on NAT2 activity and INH PK. On the other hand, pyrazinamide and ethambutol appear to have few significant drug interactions on INH. In our study, 21 healthy subjects were administered a compound preparation including isoniazid, rifampin and pyrazinamide. There was no significant difference in INH PK parameters of these subjects compared with 24 subjects given only INH.
TDM in INH could be helpful in achieving the desired therapeutic target. INH is a concentration-dependent antibiotic; AUC0–24 or Cmax of INH is suggested as the TDM index. Limited sampling strategy models, based on multiple linear regression (MLR) or maximum a posteriori (MAP) analysis, were established for the estimation of AUC in patients who were administered INH. Alshaikheid et al. (2020)established an LSS model, based on 25 patients using the MLR analysis. They found that C3, C2-C6, C0- C1-C6 are suitable for estimation of INH AUC0–24h. Cojutti et al. (2017)established a PPK model based on 185 adult tuberculosis patients. LSS models were estimated by the Bayesian method, and C1-C2-C5 and C1-C2-C9 were found to be most suitable. In the present study, concentration-time points were selected based on previous studies using the MAP method. For developed models including C2 and C2-C6, the MPE % were −10.4% and −5.46%, respectively, and MRSE % ranged from 18.5% to 12.4%, indicating that the sampling strategies including C2 and C2-C6 were clinically acceptable. The AUC0–24 values were 8.31 ± 4.12, 12.2 ± 5.16, and 27.0 ± 11.4 μg⋅h/ml for patients with wt/wt, m/wt, and m/m genotypes.
AUC0–24/MIC is considered as the most important PK/PD index for INH. Dose-scheduling studies demonstrated that antimicrobial capacity of the INH was linked to the AUC0–24/MIC (r2 = 0.83) (Donald et al., 1997; Jayaram et al., 2004). In the present study, the AUC0–24 of INH in Chinese patients with various NAT2 genotypes was estimated by the Bayesian method. A Monte Carlo method was used to simulate the effect of the standard dose of 300 mg, 600 mg, or 900 mg INH in tuberculosis patients. We found that, for each NAT2 genotype, the probability of achieving the therapeutic goal was different (Figure 5). Ninety percent of patients with various NAT2 genotypes achieved the target ratio only when the MIC of INH fell below 0.01 μg/ml. For Mycobacterium tuberculosis with an MIC of 0.02 μg/ml, EC90 could be achieved for m/m patients who were administered 300 mg of the drug, m/wt patients who were administered 600 mg of the drug, and wt/wt patients who were administered 900 mg of INH. We also found a difference in PTA among patients with NAT2 m/m genotype. The results showed that different INH dosage regimens could be designed for patients with different NAT2 genotypes. As there are many more patients with a NAT2 wt/wt genotypes in the Chinese population, the INH dose should be higher for Chinese subjects. Due to differences in tissue penetration and immunity, INH levels in the site of action, including epithelial cells and macrophages in pulmonary compartments, may exceed those found in plasma. The optimal values for the AUC0–24/MIC ratios may be different in the pulmonary compartment and more patients could achieve PTA (Honeybourne et al., 2001). When determining the optimal dose of INH, the efficacy and safety profiles of the drug should also be taken into account in the context of the contributions from other anti-tuberculosis drugs co-administered.
The present study does have a few limitations. First, only INH and AcINH concentration data were obtained. Although AcINH is the most important metabolite, if other metabolites, such as AcHZ and isonicortic acid were included, the established model might have provided more information. Second, there is still high bias of INH or AcINH prediction, especially in the first two hours. Because there were limited samples in the absorption phase, more elaborate absorption models were not applied, causing the PPK model to be inaccurate in predicting Ka and Cmax. In addition, factors other than NAT2 genotype were not introduced as covariates in the final model. The influence of those missing factors may partly explain interindividual variation and residual error. Third, the correlation between INH or AcINH and adverse effects may be more valuable. However, we did not obtain that data from patients. Finally, although the determination of the MIC of patients is more valuable for dosing regimen design, we did not do this; hence, only reference data can be used.
Conclusion
The integrate parent-metabolite population PK model accurately characterized the PKs of INH and AcINH in a Chinese population sample. In a study with a larger number of patients, personalized medicine regimens can be designed, which may be used to increase efficiency and diminish the side effects of INH therapy in Chinese turboculosis patients.
Data Availability Statement
The original contributions presented in the study are included in the article/Supplementary Materials, further inquiries can be directed to the corresponding author.
Ethics Statement
The studies involving human participants were reviewed and approved by Jinling Hospital Ethics Committee. The patients/participants provided their written informed consent to participate in this study.
Author Contributions
BC and H-QS contributed to conception and design of the study and PK study. MF performed the statistical analysis. X-HW organized the database. X-MC determined isoniazid and NAT2 genotying. W-MC contributed to manuscript.
Funding
This study was supported by the National Natural Science Foundation of China (grant number: 81973387).
Conflict of Interest
The authors declare that the research was conducted in the absence of any commercial or financial relationships that could be construed as a potential conflict of interest.
Publisher’s Note
All claims expressed in this article are solely those of the authors and do not necessarily represent those of their affiliated organizations, or those of the publisher, the editors and the reviewers. Any product that may be evaluated in this article, or claim that may be made by its manufacturer, is not guaranteed or endorsed by the publisher.
References
Agúndez, J. A., Olivera, M., Martínez, C., Ladero, J. M., and Benítez, J. (1996). Identification and Prevalence Study of 17 Allelic Variants of the Human NAT2 Gene in a White Population. Pharmacogenetics 6, 423–428.
Alshaikheid, M., Chaabane, A., Ben Fredj, N., Ben Brahim, H., Ben Fadhel, N., Chadli, Z., et al. (2020). Limited Sampling Strategy for Predicting Isoniazid Exposure in Patients with Extrapulmonary Tuberculosis. J. Clin. Pharm. Ther. 45, 503–512. doi:10.1111/jcpt.13098
Aruldhas, B. W., Hoglund, R. M., Ranjalkar, J., Tarning, J., Mathew, S. K., Verghese, V. P., et al. (2019). Optimization of Dosing Regimens of Isoniazid and Rifampicin in Children with Tuberculosis in India. Br. J. Clin. Pharmacol. 85, 644–654. doi:10.1111/bcp.13846
Bell, D. A., Taylor, J. A., Butler, M. A., Stephens, E. A., Wiest, J., Brubaker, L. H., et al. (1993). Genotype/phenotype Discordance for Human Arylamine N-Acetyltransferase (NAT2) Reveals a New Slow-Acetylator Allele Common in African-Americans. Carcinogenesis 14, 1689–1692. doi:10.1093/carcin/14.8.1689
Blomberg, B., Spinaci, S., Fourie, B., and Laing, R. (2001). The Rationale for Recommending Fixed-Dose Combination Tablets for Treatment of Tuberculosis. Bull. World. Health. Organ. 79, 61–68. doi:10.1590/S0042-96862001000100012
Boxenbaum, H. G., and Riegelman, S. (1976). Pharmacokinetics of Isoniazid and Some Metabolites in Man. J. Pharmacokinet. Biopharm. 4, 287–325. doi:10.1007/BF01063121
Cao, X. M., Chen, B., Leng, W. W., and Li, J. H. (2005). Simultaneous Determination of Isoniazid and Acetylisoniazid in Plasma with HPLC. J. Med. Postgr. 18, 397–398. doi:10.3969/j.issn.1008-8199.2005.05.005
Chakaya, J., Khan, M., Ntoumi, F., Aklillu, E., Fatima, R., Mwaba, P., et al. (2021). Global Tuberculosis Report 2020 - Reflections on the Global TB Burden, Treatment and Prevention Efforts. Int. J. Infect. Dis. 113, S7–S12. doi:10.1016/j.ijid.2021.02.107
Chen, B., Cai, W., Li, J., and Cao, X. (2009). Estimating N-Acetyltransferase Metabolic Activity and Pharmacokinetic Parameters of Isoniazid from Genotypes in Chinese Subjects. Clin. Chim. Acta 405, 23–29. doi:10.1016/j.cca.2009.03.045
Chen, B., Zhang, W. X., and Cai, W. M. (2006). The Influence of Various Genotypes on the Metabolic Activity of NAT2 in a Chinese Population. Eur. J. Clin. Pharmacol. 62, 355–359. doi:10.1007/s00228-006-0110-6
Chen, B., Li, J. H., Huang, J., and Cao, X. M. (2004). A One-step Allele Specific Amplificaton for Genotyping of NAT2 in Chinese Subjects. Chin. J. Clin. Pharmacol. 20, 49–52. doi:10.3969/j.issn.1001-6821.2004.01.012
Cojutti, P., Giangreco, M., Isola, M., and Pea, F. (2017). Limited Sampling Strategies for Determining the Area Under the Plasma Concentration-Time Curve for Isoniazid Might Be a Valuable Approach for Optimizing Treatment in Adult Patients With Tuberculosis. Int. J. Antimicrob. Agents. 50, 23–28. doi:10.1016/j.ijantimicag.2017.01.036
Deguchi, T., Mashimo, M., and Suzuki, T. (1990). Correlation between Acetylator Phenotypes and Genotypes of Polymorphic Arylamine N-Acetyltransferase in Human Liver. J. Biol. Chem. 265, 12757–12760. doi:10.1016/s0021-9258(19)38219-5
Denti, P., Jeremiah, K., Chigutsa, E., Faurholt-Jepsen, D., PrayGod, G., Range, N., et al. (2015). Pharmacokinetics of Isoniazid, Pyrazinamide, and Ethambutol in Newly Diagnosed Pulmonary TB Patients in Tanzania. PLoS. One. 10, e0141002. doi:10.1371/journal.pone.0141002
Donald, P. R., Parkin, D. P., Seifart, H. I., Schaaf, H. S., van Helden, P. D., Werely, C. J., et al. (2007). The Influence of Dose and N-Acetyltransferase-2 (NAT2) Genotype and Phenotype on the Pharmacokinetics and Pharmacodynamics of Isoniazid. Eur. J. Clin. Pharmacol. 63, 633–639. doi:10.1007/s00228-007-0305-5
Donald, P. R., Sirgel, F. A., Botha, F. J., Seifart, H. I., Parkin, D. P., Vandenplas, M. L., et al. (1997). The Early Bactericidal Activity of Isoniazid Related to its Dose Size in Pulmonary Tuberculosis. Am. J. Respir. Crit. Care. Med. 156, 895–900. doi:10.1164/ajrccm.156.3.9609132
Ferguson, L. A., and Rhoads, J. (2009). Multidrug-resistant and Extensively Drug-Resistant Tuberculosis: The New Face of an Old Disease. J. Am. Acad. Nurse. Pract. 21, 603–609. doi:10.1111/j.1745-7599.2009.00458.x
Gumbo, T., Louie, A., Liu, W., Brown, D., Ambrose, P. G., Bhavnani, S. M., et al. (2007). Isoniazid Bactericidal Activity and Resistance Emergence: Integrating Pharmacodynamics and Pharmacogenomics to Predict Efficacy in Different Ethnic Populations. Antimicrob. Agents. Chemother. 51, 2329–2336. doi:10.1128/AAC.00185-07
Gumbo, T. (2010). New Susceptibility Breakpoints for First-Line Antituberculosis Drugs Based on Antimicrobial Pharmacokinetic/Pharmacodynamic Science and Population Pharmacokinetic Variability. Antimicrob. Agents. Chemother. 54, 1484–1491. doi:10.1128/AAC.01474-09
Hao, L. H., Guo, S. C., Liu, C. C., Zhu, H., Wang, B., Fu, L., et al. (2014). Comparative Bioavailability of Rifampicin and Isoniazid in Fixed-Dose Combinations and Single-Drug Formulations. Int. J. Tuberc. Lung. Dis. 18, 1505–1512. doi:10.5588/ijtld.13.0647
Hein, D. W., Doll, M. A., Rustan, T. D., and Ferguson, R. J. (1995). Metabolic Activation of N-Hydroxyarylamines and N-Hydroxyarylamides by 16 Recombinant Human NAT2 Allozymes: Effects of 7 Specific NAT2 Nucleic Acid Substitutions. Cancer. Res. 55, 3531–3536.
Hein, D. W., Ferguson, R. J., Doll, M. A., Rustan, T. D., and Gray, K. (1994). Molecular Genetics of Human Polymorphic N-Acetyltransferase: Enzymatic Analysis of 15 Recombinant Wild-type, Mutant, and Chimeric NAT2 Allozymes. Hum. Mol. Genet. 3, 729–734. doi:10.1093/hmg/3.5.729
Honeybourne, D., Banerjee, D., Andrews, J., and Wise, R. (2001). Concentrations of Gatifloxacin in Plasma and Pulmonary Compartments Following a Single 400 Mg Oral Dose in Patients Undergoing Fibre-Optic Bronchoscopy. J. Antimicrob. Chemother. 48, 63–66. doi:10.1093/jac/48.1.63
Horai, Y., Ishizaki, T., Sasaki, T., Koya, G., Matsuyama, K., and Iguchi, S. (1982). Isoniazid Disposition, Comparison of Isoniazid Phenotyping Methods in and Acetylator Distribution of Japanese Patients with Idiopathic Systemic Lupus Erythematosus and Control Subjects. Br. J. Clin. Pharmacol. 13, 361–374. doi:10.1111/j.1365-2125.1982.tb01387.x
Jayaram, R., Shandil, R. K., Gaonkar, S., Kaur, P., Suresh, B. L., Mahesh, B. N., et al. (2004). Isoniazid Pharmacokinetics-Pharmacodynamics in an Aerosol Infection Model of Tuberculosis. Antimicrob. Agents. Chemother. 48, 2951–2957. doi:10.1128/AAC.48.8.2951-2957.2004
Jing, W., Zong, Z., Tang, B., Wang, J., Zhang, T., Wen, S., et al. (2020). Population Pharmacokinetic Analysis of Isoniazid Among Pulmonary Tuberculosis Patients from China. Antimicrob. Agents. Chemother. 64, e01736–19. doi:10.1128/AAC.01736-19
Kinzig-Schippers, M., Tomalik-Scharte, D., Jetter, A., Scheidel, B., Jakob, V., Rodamer, M., et al. (2005). Should We Use N-Acetyltransferase Type 2 Genotyping to Personalize Isoniazid Doses? Antimicrob. Agents. Chemother. 49, 1733–1738. doi:10.1128/AAC.49.5.1733-1738.2005
Kiser, J. J., Zhu, R., DʼArgenio, D. Z., Cotton, M. F., Bobat, R., McSherry, G. D., et al. (2012). Isoniazid Pharmacokinetics, Pharmacodynamics, and Dosing in South African Infants. Ther. Drug. Monit. 34, 446–451. doi:10.1097/FTD.0b013e31825c4bc3
Kita, T., Tanigawara, Y., Chikazawa, S., Hatanaka, H., Sakaeda, T., Komada, F., et al. (2001). N-Acetyltransferase2 Genotype Correlated with Isoniazid Acetylation in Japanese Tuberculous Patients. Biol. Pharm. Bull. 24, 544–549. doi:10.1248/bpb.24.544
Lauterburg, B. H., Smith, C. V., Todd, E. L., and Mitchell, J. R. (1985b). Oxidation of Hydrazine Metabolites Formed from Isoniazid. Clin. Pharmacol. Ther. 38, 566–571. doi:10.1038/clpt.1985.225
Lauterburg, B. H., Smith, C. V., Todd, E. L., and Mitchell, J. R. (1985a). Pharmacokinetics of the Toxic Hydrazino Metabolites Formed from Isoniazid in Humans. J. Pharmacol. Exp. Ther. 235, 566–570.
Lin, H. J., Han, C. Y., Lin, B. K., and Hardy, S. (1993). Slow Acetylator Mutations in the Human Polymorphic N-Acetyltransferase Gene in 786 Asians, Blacks, Hispanics, and Whites: Application to Metabolic Epidemiology. Am. J. Hum. Genet. 52, 827–834.
Loktionov, A., Moore, W., Spencer, S. P., Vorster, H., Nell, T., O'Neill, I. K., et al. (2002). Differences in N-Acetylation Genotypes between Caucasians and Black South Africans: Implications for Cancer Prevention. Cancer. detect. Prev. 26, 15–22. doi:10.1016/s0361-090x(02)00010-7
McIlleron, H., Wash, P., Burger, A., Norman, J., Folb, P. I., and Smith, P. (2006). Determinants of Rifampin, Isoniazid, Pyrazinamide, and Ethambutol Pharmacokinetics in a Cohort of Tuberculosis Patients. Antimicrob. Agents. Chemother. 50, 1170–1177. doi:10.1128/AAC.50.4.1170-1177.2006
Mitchison, D. A. (1979). Basic Mechanisms of Chemotherapy. Chest 76 (Suppl. l), 771–781. doi:10.1378/chest.76.6_supplement.771
Parkin, D. P., Vandenplas, S., Botha, F. J., Vandenplas, M. L., Seifart, H. I., van Helden, P. D., et al. (1997). Trimodality of Isoniazid Elimination: Phenotype and Genotype in Patients with Tuberculosis. Am. J. Respir. Crit. Care. Med. 155, 1717–1722. doi:10.1164/ajrccm.155.5.9154882
Pasipanodya, J., and Gumbo, T. (2011). An Oracle: Antituberculosis Pharmacokinetics-Pharmacodynamics, Clinical Correlation, and Clinical Trial Simulations to Predict the Future. Antimicrob. Agents. Chemother. 55, 24–34. doi:10.1128/AAC.00749-10
Peloquin, C. A. (2002). Therapeutic Drug Monitoring in the Treatment of Tuberculosis. Drugs 62, 2169–2183. doi:10.2165/00003495-200262150-00001
Peretti, E., Karlaganis, G., and Lauterburg, B. H. (1987). Increased Urinary Excretion of Toxic Hydrazino Metabolites of Isoniazid by Slow Acetylators. Effect of a Slow-Release Preparation of Isoniazid. Eur. J. Clin. Pharmacol. 33, 283–286. doi:10.1007/BF00637563
Rodriguez, C. A., Zuluaga, A. F., Neely, M. N., Sierra, Y., Morales-Gutierrez, J., Zapata, J., et al. (2019). Nonparametric Population Pharmacokinetic Modeling of Isoniazid in Colombian Patients With Tuberculosis. Ther. Drug. Monit. 41, 719–725. doi:10.1097/FTD.0000000000000661
Sandhu, G. K. (2011). Tuberculosis: Current Situation, Challenges and Overview of its Control Programs in India. J. Glob. Infect. Dis. 3, 143–150. doi:10.4103/0974-777X.81691
Seng, K. Y., Hee, K. H., Soon, G. H., Chew, N., Khoo, S. H., and Lee, L. S. (2015). Population Pharmacokinetic Analysis of Isoniazid, Acetylisoniazid, and Isonicotinic Acid in Healthy Volunteers. Antimicrob. Agents. Chemother. 59, 6791–6799. doi:10.1128/AAC.01244-15
Shishoo, C. J., Shah, S. A., Rathod, I. S., Savale, S. S., and Vora, M. J. (2001). Impaired Bioavailability of Rifampicin in Presence of Isoniazid from Fixed Dose Combination (FDC) Formulation. Int. J. Pharm. 228, 53–67. doi:10.1016/s0378-5173(01)00831-6
Smith, C. A., Wadelius, M., Gough, A. C., Harrison, D. J., Wolf, C. R., and Rane, A. (1997). A Simplified Assay for the Arylamine N-Acetyltransferase 2 Polymorphism Validated by Phenotyping with Isoniazid. J. Med. Genet. 34, 758–760. doi:10.1136/jmg.34.9.758
Sundell, J., Bienvenu, E., Janzén, D., Birgersson, S., Äbelö, A., and Ashton, M. (2020). Model-Based Assessment of Variability in Isoniazid Pharmacokinetics and Metabolism in Patients Co-Infected With Tuberculosis and HIV: Implications for a Novel Dosing Strategy. Clin. Pharmacol. Ther. 108, 73–80. doi:10.1002/cpt.1806
Takayama, K., Wang, L., and David, H. L. (1972). Effect of Isoniazid on the In Vivo Mycolic Acid Synthesis, Cell Growth, and Viability of Mycobacterium tuberculosis. Antimicrob. Agents Chemother. 2, 29–35. doi:10.1128/AAC.2.1.29
Timbrell, J. A., Mitchell, J. R., Snodgrass, W. R., and Nelson, S. D. (1980). Isoniazid Hepatoxicity: the Relationship between Covalent Binding and Metabolism In Vivo. J. Pharmacol. Exp. Ther. 213, 364–369.
Walker, K., Ginsberg, G., Hattis, D., Johns, D. O., Guyton, K. Z., and Sonawane, B. (2009). Genetic Polymorphism in N-Acetyltransferase (NAT): Population Distribution of NAT1 and NAT2 Activity. J. Toxicol. Environ. Health. B. Crit. Rev. 12, 440–472. doi:10.1080/10937400903158383
Weiner, M., Burman, W., Vernon, A., Benator, D., Peloquin, C. A., Khan, A., et al. (2003). Low Isoniazid Concentrations and Outcome of Tuberculosis Treatment with Once-Weekly Isoniazid and Rifapentine. Am. J. Respir. Crit. Care. Med. 167, 1341–1347. doi:10.1164/rccm.200208-951OC
Winder, F. G., and Collins, P. B. (1970). Inhibition by Isoniazid of Synthesis of Mycolic Acids in Mycobacterium tuberculosis. J. Gen. Microbiol. 63, 41–48. doi:10.1099/00221287-63-1-41
Zhao, J. J., and Lu, Y. (2019). Research and Progress of PK/PD for Anti-tuberculosis Drugs. Chin. J. Antituberc. 41, 700–704. doi:10.3969/j.issn.1000-6621.2019.06.020
Zuur, M. A., Bolhuis, M. S., Anthony, R., den Hertog, A., van der Laan, T., Wilffert, B., et al. (2016). Current Status and Opportunities for Therapeutic Drug Monitoring in the Treatment of Tuberculosis. Expert. Opin. Drug. Metab. Toxicol. 12, 509–521. doi:10.1517/17425255.2016.1162785
Zvada, S. P., Denti, P., Donald, P. R., Schaaf, H. S., Thee, S., Seddon, J. A., et al. (2014). Population Pharmacokinetics of Rifampicin, Pyrazinamide and Isoniazid in Children With Tuberculosis: In Silico Evaluation of Currently Recommended Doses. J. Antimicrob. Chemother. 69, 1339–1349. doi:10.1093/jac/dkt524
Keywords: isoniazid, acetylisoniazid, population pharmacokinetics, N-acetyltransferase 2, genetic polymorphism, pharmacodynamics
Citation: Chen B, Shi H-Q, Feng MR, Wang X-H, Cao X-M and Cai W-M (2022) Population Pharmacokinetics and Pharmacodynamics of Isoniazid and its Metabolite Acetylisoniazid in Chinese Population. Front. Pharmacol. 13:932686. doi: 10.3389/fphar.2022.932686
Received: 30 April 2022; Accepted: 07 June 2022;
Published: 19 July 2022.
Edited by:
Zipeng Gong, Guizhou Medical University, ChinaCopyright © 2022 Chen, Shi, Feng, Wang, Cao and Cai. This is an open-access article distributed under the terms of the Creative Commons Attribution License (CC BY). The use, distribution or reproduction in other forums is permitted, provided the original author(s) and the copyright owner(s) are credited and that the original publication in this journal is cited, in accordance with accepted academic practice. No use, distribution or reproduction is permitted which does not comply with these terms.
*Correspondence: Bing Chen, Y2hjaGVuYmluZ0AxNjMuY29t
†These authors have contributed equally to this work