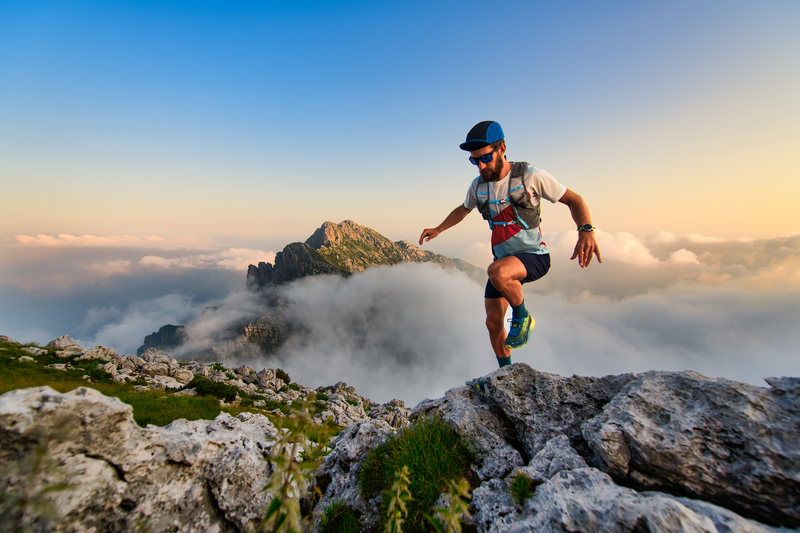
95% of researchers rate our articles as excellent or good
Learn more about the work of our research integrity team to safeguard the quality of each article we publish.
Find out more
ORIGINAL RESEARCH article
Front. Pharmacol. , 08 June 2022
Sec. Experimental Pharmacology and Drug Discovery
Volume 13 - 2022 | https://doi.org/10.3389/fphar.2022.930520
This article is part of the Research Topic Computational Intelligence in Personalized Medicine View all 11 articles
Background: Artificial intelligence (AI) has been used in the research of ophthalmic disease diagnosis, and it may have an impact on medical and ophthalmic practice in the future. This study explores the general application and research frontier of artificial intelligence in ophthalmic disease detection.
Methods: Citation data were downloaded from the Web of Science Core Collection database to evaluate the extent of the application of Artificial intelligence in ophthalmic disease diagnosis in publications from 1 January 2012, to 31 December 2021. This information was analyzed using CiteSpace.5.8. R3 and Vosviewer.
Results: A total of 1,498 publications from 95 areas were examined, of which the United States was determined to be the most influential country in this research field. The largest cluster labeled “Brownian motion” was used prior to the application of AI for ophthalmic diagnosis from 2007 to 2017, and was an active topic during this period. The burst keywords in the period from 2020 to 2021 were system, disease, and model.
Conclusion: The focus of artificial intelligence research in ophthalmic disease diagnosis has transitioned from the development of AI algorithms and the analysis of abnormal eye physiological structure to the investigation of more mature ophthalmic disease diagnosis systems. However, there is a need for further studies in ophthalmology and computer engineering.
Artificial intelligence (AI) is a broad term that refers to the use of computers to simulate intelligent behavior with little or no human intervention (Hamet and Tremblay, 2017). It is a multifaceted technology that includes complex algorithms, machine learning, and deep learning, transfer learning, among other components (Balyen and Peto, 2019; Kora et al., 2022). Medicine has long been identified as one of the most promising fields for the application of AI. Many clinical decision support systems have been proposed and developed by researchers since the mid-twentieth century (Miller, 1994). AI has been used in ophthalmology to diagnose diseases in conjunction with imaging technologies such as optical coherence tomography, and fundus fluorescein angiography (Ruiz Hidalgo et al., 2017; Hemalakshmi et al., 2020; Wan et al., 2021c; Ran et al., 2021). In addition, several simple and low-cost diagnostic system models have been under development (Bourouis et al., 2014; Metha et al., 2021). As a possible solution for the screening of major ophthalmic diseases and telemedicine, AI has been applied to the study of ophthalmic disease diagnosis. For example, diabetic retinopathy, glaucoma, hypertensive retinopathy, high myopia, age-related macular degeneration, familial amyloidosis, cataract, and other related conditions have been investigated using this technology (Fang et al., 2017; Asaoka et al., 2019; Araujo et al., 2020; Juneja et al., 2020; Kessel et al., 2020; Zhou et al., 2020; Wan et al., 2021a; Grzybowski and Brona, 2021; Szeskin et al., 2021; Xu et al., 2022). AI may have an impact on medical and ophthalmic practice in the coming decades, based on the results of several published reports (Ting et al., 2019; Dai et al., 2021).
Previous studies have used bibliometric methods to study the application of artificial intelligence in ophthalmic diseases in China (Dong et al., 2021; Koh et al., 2021; Saeed et al., 2021; Boudry et al., 2022). However, there is no bibliometric research on the application of artificial intelligence in ophthalmic disease diagnosis. This study aimed to gain a comprehensive understanding of the general use and research frontier of artificial intelligence in ocular illness detection by examining multiple aspects. For example, Scientific Citation Index (SCI) papers on the application of artificial intelligence to ocular illness diagnosis were analyzed using bibliometric approaches. The analysis emphasized data related to countries, regions, institutions, journals, research categories, keywords, and references. A critical aspect of our study was the development of a repeatable and unbiased strategy for exploring the active knowledge frontier in the research field. In particular, we examined the active areas of applied artificial intelligence, future development areas, and potential hurdles, relative to ocular disease diagnostics. This report is intended to serve as a resource for artificial intelligence professionals, ophthalmologists, diagnosticians, and medical imaging researchers.
On 1 April 2022, citation data published between 1 January 2012, to 31 December 2021, were retrieved from the Web of Science Core Collection (WoSCC). These data were independently verified by two authors (Weihua Yang and Yi Lu). The search formula was TS= (retinal or ophthalmology or eye or ophthalmic or corneal or eyelid or orbital or uveal or scleral) AND (AI or “Artificial Intelligence” or “neural network” or “transfer learning” or “Machine Learning” or “Deep Learning”) AND (diagnos* or grad* or classification). The search selected English literature and articles and excluded early access, proceedings papers, book chapters, data papers, and retracted publications. To obtain the most accurate analysis results, we manually deleted data after reading the title and abstract of each literature. The criteria for manual exclusion are as follows: 1) the research discipline does not include medicine; 2) The study organ is an organ other than the eye; 3) The research method does not use artificial intelligence method; 4) The study disease is not an eye disease. All the data we included in the analysis were the research of artificial intelligence in the diagnosis of ophthalmic diseases. For each publication, we extracted the title, publication year, country or region, institution, journal, references, and keywords. The detailed search and analysis processes are depicted in Figure 1.
FIGURE 1. Frame flow diagram showing the detailed selection criteria and bibliometric analysis steps for the study of the application of AI in the diagnosis of ophthalmic diseases.
Collaborative networks of countries, institutions, journals, keywords, references, and research categories were analyzed using CiteSpace.5.8. R3 and Vosviewer. The article describes all citation features.
This study analyzed 1,498 papers that were published between 2012 and 2021 that focused on the use of AI in the diagnosis of ophthalmic diseases. Using the Web of Science (WoS) citation analyzer to count the annual number of citations retrieved and using the duplicate removal function of CiteSpace software to verify the data of the number of citations. The number of annually published reports for this period is shown in Figure 2. Since 2018, the annual number of articles on the application of AI in the diagnosis of ophthalmic diseases has exceeded 100 and has increased rapidly in subsequent years.
FIGURE 2. Annual number of publications on the application of AI in the diagnosis of ophthalmic diseases from 2012 to 2021.
The citation analyzer of WoS database is used to count the number of documents sent by countries or regions, and the default setting of CiteSpace software analyzes the cooperative relationship between countries and regions. These citations involve a total of 95 countries or regions. The size of each label and green node area in Figure 3 represents the number of documents sent. Countries with large green node areas include the people’s Republic of China (415 articles), the United States (365 articles) and India (263 articles). The connection between nodes represents the cooperative relationship between regions. Countries with more connecting lines have regions with strong influence, The area of purple circle indicates the influence of national documents, which is expressed by the centrality in Table 1. The purple circle in the United States has the largest area (0.25), indicating that the articles published in the United States in the field of ophthalmic diagnosis have the greatest overall influence. The data in Table 1 objectively substantiate these conclusions. The H index can accurately reflect academic achievement (Hirsch, 2005). The higher the centrality and H index, the greater the influence of a paper. In general, China had the largest number of articles and the United States had the greatest influence.
FIGURE 3. Cooperation of countries or regions that contributed to publications on the use of AI for the diagnosis of ophthalmic diseases from 2012 to 2021.
TABLE 1. Top 10 countries or regions with publications on the application of the use of AI in the diagnosis of ophthalmic diseases from 2012 to 2021.
Table 2 lists the top 10 institutions for published articles that were analysed. The displayed data is outcome from the default settings of CiteSpace software and vosviewer software. These included three American institutions, three England institutions, three Chinese institutions, and one Singapore institution. Two of the top five institutions with the highest h-index were from the United States and England. The connection between the tags in Figure 4 shows the inter-agency cooperation. The node size indicates the number of documents sent.
TABLE 2. Top 10 Institutions with publications on the application or the use of AI in the diagnosis of ophthalmic diseases from 2012 to 2021.
FIGURE 4. Cooperation of institutions that contributed to publications on the use of AI in the diagnosis of ophthalmic diseases from 2012 to 2021.
The documents in the cited journals constitute the knowledge base of the referenced articles. The research fields in highly cited journals constitute an active area of interest or hotspot. We use CiteSpace to draw the citation relationship in the field of journal research. The paths of the two colors shown in Figure 5 represent the citation relationship of highly active research fields. Among them, the red path represents the classification of the journals with the most papers. The research field of the citing journals is represented on the left, and the research field of the cited journals is shown on the right. The knowledge-based research fields of AI application in ophthalmic disease diagnosis in the recent 10 years include systems/computing/computer/molecular/biology/genetics/health/nursing/medicine/ophthalmology, which constitute the hotspot subjects involved in the research frontier such as mathematics/systems/mathematical/neurology/sports/ophthalmology. Tables 3, 4 list the discipline categories of the citing journals and cited journals/proceedings that rank among the top ten in terms of citations. The most common research field of the citing journals includes engineering technology/computers. The discipline that was most involved in the extracted version was classified as medicine/ophthalmology.
FIGURE 5. Dual map overlay of journals that contributed to publications on the use of AI in the diagnosis of ophthalmic diseases from 2012 to 2021.
TABLE 3. Top 10 citing journals of publications on the use of AI in the diagnosis of ophthalmic diseases from 2012 to 2021.
TABLE 4. Top 10 cited journals of publications on the use of AI in the diagnosis of ophthalmic diseases from 2012 to 2021.
To better understand the adoption of AI in the field of ophthalmic disease diagnosis in the recent 10 years based on an analysis diagram of keyword co-occurrence cooperation network, the emerging keywords that progressed over time were analyzed. This represented the migration of research hotspots. The default setting of CiteSpace is changed to the following mode: “Year Per Slice” = 2, “Top N%" = 30.0%, and “Minimum Duration” = 1. We get the result of Figure 6. The red square in Figure 6 represents emerging keywords for the investigated timeline. The bursts keywords from 2012 to 2021 included machine learning classifier (2012–2015), artificial neural network (2012–2015), nerve fiber layer (2012–2015), retinal layer (2018–2019), head (2018–2019), macular degeneration (2018–2019), cup (2018–2019), system (2020–2021), disease (2020–2021), and model (2020–2021).
FIGURE 6. Keywords with the strongest citation bursts for publications on the use of AI in the diagnosis of ophthalmic diseases from 2012 to 2021.
The cited documents were highly related to the research topics. Using the default setting of CiteSpace to cluster the co cited documents and choose label clusters with indexing terms. The cluster labels that represented the research frontiers of the co-cited documents were obtained from these documents. The cited literature constituted the knowledge base of the research, and the size of the clusters obtained from the literature are listed in order from top to bottom on the right side of Figure 7. The largest cluster label #0“Brownian motion” was obtained during the pre-period of the application of AI for ophthalmic diagnosis, which was 2007–2017, and was a research hotspot. Table 5 lists the top ten citing literature from “times cited in all databases “among the relevant literature based on the application of AI for ophthalmic diagnosis. It was determined that AI techniques are promising for use in the diagnosis of ophthalmic diseases although there are some limitations associated with their use.
FIGURE 7. Co-cited reference timeline map of publications on the use of AI in the diagnosis of ophthalmic diseases from 2012 to 2021.
TABLE 5. Top 10 citing articles on the application of the use of AI in the diagnosis of ophthalmic diseases from 2012 to 2021.
Based on the preceding results, it is apparent that the published literature on the application of AI in the diagnosis of ophthalmic diseases has increased sharply in the past 5 years. This indicates that this research field has gradually attracted significant interest in recent years. The World Economic 2016 Forum identified the open artificial intelligence ecosystem as one of the ten most important emerging technologies (President et al., 2016). Gulshan, V et al. established that deep learning algorithms had high sensitivity and specificity for detecting diabetic retinopathy and macular edema (Gulshan et al., 2016). Although it is impossible to predict the number of literature on AI for ophthalmic disease diagnosis that will be published in the future, it is still a promising research field and the efficacy of the application of AI has been confirmed by many studies (Giardini and Livingstone, 2020).
In terms of the number of national documents, the United States has the highest centrality and h-index. This indicates that this country has a leading position in this research field. In addition, developed countries such as Britain, Spain, and Singapore exhibited strong centrality and influence. Although China published a large number of papers, it lacked highly cited articles. The top five research institutions in terms of the number of publications are in the United States and the United Kingdom. From the perspective of the h-index, the national unity of Singapore had a strong impact. This was observed in the analysis results for national document issuance. From the analysis of the research field of the journal, it is evident that in recent years, research focused on the use of computer engineering technology combined with a knowledge base of ophthalmology to develop more suitable ophthalmic disease detection systems. AI is widely used to identify ophthalmic diseases, which is typically based on the analysis of ophthalmic images (Xu et al., 2021a; Wan et al., 2021b; Xu et al., 2021b). In addition, this research also includes the detection of genes related to ophthalmic diseases (Saikia and Nirmala, 2022), ocular metabolites (Myer et al., 2020), and pathology ocular metabolites (Nezu et al., 2020). Areas of active interest and the research frontiers of AI in ophthalmic disease diagnosis can be identified based on the clustering timeline of emerging keywords and co-cited references. The titles and abstracts of 936 articles published in 2020 and 2021 were examined.
Table 6 lists the top ten diseases in the recent 2 years, among it we can see that the hottest disease with the ophthalmic diagnosis using AI technology is “diabetic retinopathy”. A classic study in 2016 was that Gulshan, V and others developed a deep machine learning algorithm based on 128,175 retinal images, which has high sensitivity and specificity in detecting diabetes retinopathy (Gulshan et al., 2016). In 2017, Ting, DSW and others obtained high sensitivity and specificity when using the deep learning system to evaluate the diagnostic images of patients with diabetes retinopathy and related eye diseases from multiple ethnic groups (Ting et al., 2017). Li, SC and others pointed out that the conditions for using artificial intelligence system to replace ophthalmologists are not mature (Li et al., 2022). Therefore, using AI technology to diagnose diabetes retinopathy or other ophthalmic diseases requires more research.
According to the results of the cluster analysis of CO cited references, it is not difficult to determine during the early stage of the sharp increase in the number of studies, the most important knowledge base is “Brownian motion.”
Previous studies have shown that using fractional Brownian motion to model medical examination images can provide better global texture indicators than traditional texture feature-based measurement methods (Mcgarry and Deriche, 1997). Early detection of glaucoma is important in the prevention of blindness. Yun, WL et al. used digital fundus images to extract texture features based on fractal dimension and Brownian motion. Specifically, they performed two-dimensional two-level discrete wavelet transform on the images, extracted energy and entropy data, and finally developed a highly specific and sensitive early glaucoma diagnosis model (Yun et al., 2014b). They also proposed a final stage of automatic detection of diabetic retinopathy using Brownian motion characteristics, namely, proliferative diabetic retinopathy (Yun et al., 2014a). Earlier literature also confirmed that the Brownian motion model can be used to classify normal and abnormal ultrasound liver images (Wu et al., 1992).
The research hotspots can be identified based on an analysis of emerging keywords. The emergent keywords in different periods represent different research hotspots.
The emerging keywords from 2012 to 2015 were “Machine Learning Classifier,” “artificial neural network,” and “nerve fiber layer.” This suggests that the performance of different intelligent algorithms was under investigation for application to ophthalmic disease diagnosis. A subfield of artificial intelligence technology is machine learning. It employs algorithms in a systematic manner to synthesize the potential relationship between data and information (Awad and Khanna, 2015). Andersson, S et al. used the visual field print output of 99 glaucoma patients and 66 healthy people to compare the sensitivity and specificity of the results obtained for a glaucoma diagnosis system based on artificial neural network and direct diagnosis by ophthalmologists. This study confirmed that an artificial neural network has higher specificity and sensitivity and fewer classification errors compared to doctors (Andersson et al., 2013). Yousefi, S et al. compared the detection of glaucoma progression using different machine learning classifiers based on longitudinal structure data sequences extracted from retinal nerve fiber layer thickness measurement and visual function data obtained from standard automatic visual field examination and evaluated the performance of these classifiers (Yousefi et al., 2014). To improve the effectiveness of treating open-angle glaucoma, Ein Oh et al. investigated the application of a screening method to distinguish open-angle glaucoma from suspected glaucoma without visual field testing. They used five open-angle glaucoma risk prediction models that were created based on 8,958 subjects (including patients with suspected open-angle glaucoma) using an artificial neural network. It was established that the artificial neural network method was a cost-effective screening tool for distinguishing between patients with open-angle glaucoma and glaucoma suspect subjects (Oh et al., 2015).
The emerging keywords from 2018 to 2019 were “retinal layer,” “head,” “macular degeneration,” and “cup.” This indicates that the research focus transitioned to the study of various ophthalmic diseases and anatomical structures. Color fundus photography facilitates the examination of the optic disc to determine the cup-to-disc ratio, which is important in the diagnosis of glaucoma. Al-Bander et al. proposed a new method based on deep learning, which used the combination of a convolution network and DenseNet to segment optical discs and optical cups. Four data sets for image detection were then used to evaluate its effectiveness (Al-Bander et al., 2018). Although the image quality of optical coherence tomography (OCT) needs to be improved and the scanning duration leads to patient discomfort, this imaging modality has become an established clinical routine for in vivo imaging of optic nerve head tissue, which is very important in the diagnosis of various ophthalmic diseases (Du et al., 2014). Devalla, SK et al. developed a customized deep learning method to remove the noise in a single frame OCT B-scan. The network proposed in this study performs denoising in less than 20 milliseconds (Devalla et al., 2019). Based on the analysis of chorioretinal OCT images, clinicians and researchers have a better understanding of the diagnosis of a series of ophthalmic diseases under different conditions (Bussel et al., 2014). In practical applications, choroidal boundary segmentation usually requires manual segmentation, which is time-consuming. Kugelman, J et al. proposed several depth learning methods based on complete convolution to accurately determine the location of the choroidal boundary of interest. Artificial intelligence technology was compared with manual boundary segmentation and standard image analysis technology as part of the study. Furthermore, the investigation established the advantage of deep learning methods in chorioretinal boundary analysis and the segmentation of OCT images (Kugelman et al., 2019).
The emerging keywords from 2020 to 2021 were “system,” “disease,” and “model,” which indicate that researchers have begun to develop various systematic diagnostic models for the study of ophthalmic diseases. The proportion of doctors and patients in China is unbalanced, and the regional distribution of medical capital is uneven (National Health Commission, 2020). To minimize these biases, Zheng, B et al. designed five intelligent models for the diagnosis of fundus diseases using transfer learning. These models can detect normal eyes and four common fundus diseases including retinal vein occlusion, high myopia, glaucoma, and diabetic retinopathy (Zheng et al., 2021). However, the limitation of this study is that the models can only diagnose four common fundus diseases, which may be misdiagnosed in the case of other ophthalmic diseases. Ahn, H used machine-learning artificial intelligence to develop a model to classify the severity of emerging ophthalmic diseases, with an accuracy of nearly 100% (Ahn, 2020). The authors reported that the relative lack of data set samples was a major limitation of the study. To prevent vision loss caused by corneal diseases and to improve the early diagnosis of corneal diseases, Elsawy, A et al. proposed a deep learning network based on corneal OCT images, Fuchs’ cochlear dystrophy, and keratoconus. The developed algorithm was then used to evaluate the data set of 16721 OCT images. It was determined that the algorithm was superior to other network learning procedures (Elsawy and Abdel-Mottaleb, 2021).
As the adoption of artificial intelligence increases and the technology is continually applied to a wide array of medical fields, more intelligent detection methods will be required for ophthalmic diseases diagnosis.
By summarizing the limitations of the top ten cited literature, it was determined that the constraints of AI in ophthalmic disease diagnosis can be divided into the following five categories: 1) The clinical examination standard is highly subjective, which leads to difficulties in the design of intelligent auxiliary diagnosis system; 2) Limited detectability of ophthalmic diseases in intelligent auxiliary diagnosis systems; 3) The data set used in most training models included a limited examination population; 4) The diagnosis of artificial intelligence of ophthalmic diseases is still in the stage of auxiliary diagnosis, and requires the use of traditional clinical examination tools; 5) The scope limitations of validating intelligent auxiliary diagnosis systems.
To develop a more robust and usable diagnostic system, it is necessary to obtain more types and larger data sets. For example, the collection of ophthalmic examination data from different races, countries, or regions (Bellemo et al., 2019; Raumviboonsuk et al., 2019; Al Turk et al., 2020). More disease types should also be included in these studies, such as pterygium, familial amyloidosis, and thyroid-associated ophthalmopathy (Kessel et al., 2020; Zamani et al., 2020; Xu W. et al., 2021; Song et al., 2021). In addition, more ophthalmologists with different levels of training should participate in the screening stage of the data set and the examination stage of the algorithm to obtain clinically-based diagnoses. Finally, by increasing the number of clinical cases of the validation system and utilizing statistical approaches to achieve high sensitivity and specificity, the utilization rate of artificial intelligence models can be further improved.
In summary, the training of intelligent algorithms based on the analysis of images is of growing interest. There is ongoing worldwide research on the use of AI in ophthalmic diagnosis. In particular, the United States is the most influential country in this research field. The application of artificial intelligence technology to ophthalmic diagnosis has revolutionized the clinical landscape of ophthalmologists and patients. This technology facilitates more accurate diagnosis and remote diagnosis services. However, there are limitations associated with these approaches. For example, the credibility of the training model is questionable and is often not recognized by institutions for practical clinical work, even if it has been shown to have high sensitivity and specificity. In addition, contemporary research is focused on several relatively well-known diseases, whereas the number of studies on other diseases is small, and a mature diagnostic system has not been developed. At present, the research focus has shifted from the development of artificial intelligence algorithms and the analysis of the abnormal ocular physiological structure to research on ophthalmic disease diagnosis systems. To address the existing limitations, it is necessary to obtain more national and ethnic ophthalmic data to train and test the algorithm. This is a huge task. In addition to computer engineering experts who primarily develop algorithms, ophthalmologists from different regions and with different levels of experience need to participate in this endeavor.
The original contributions presented in the study are included in the article/Supplementary Material, further inquiries can be directed to the corresponding authors.
JZ and YL conceived and designed the analysis, performed the analysis, and wrote the manuscript; SZ performed the analysis and wrote the manuscript; WY, QJ and KL designed the research, acquired the article information, and revised the manuscript. All authors contributed to the article and approved the submitted version.
This research was funded by the Nanjing Enterprise Expert Team Project and, Medical Science and Technology Development Project Fund of Nanjing (Grant No. YKK21262) and Medical Big Data Clinical Research Project of Nanjing Medical University.
The authors declare that the research was conducted in the absence of any commercial or financial relationships that could be construed as a potential conflict of interest.
All claims expressed in this article are solely those of the authors and do not necessarily represent those of their affiliated organizations, or those of the publisher, the editors and the reviewers. Any product that may be evaluated in this article, or claim that may be made by its manufacturer, is not guaranteed or endorsed by the publisher.
Abràmoff, M. D., Lavin, P. T., Birch, M., Shah, N., and Folk, J. C. (2018). Pivotal Trial of an Autonomous AI-Based Diagnostic System for Detection of Diabetic Retinopathy in Primary Care Offices. NPJ Digit. Med. 1, 39. doi:10.1038/s41746-018-0040-6
Abràmoff, M. D., Lou, Y., Erginay, A., Clarida, W., Amelon, R., Folk, J. C., et al. (2016). Improved Automated Detection of Diabetic Retinopathy on a Publicly Available Dataset through Integration of Deep Learning. Invest. Ophthalmol. Vis. Sci. 57, 5200–5206. doi:10.1167/iovs.16-19964
Ahn, H. (2020). Artificial Intelligence Method to Classify Ophthalmic Emergency Severity Based on Symptoms: a Validation Study. Bmj Open 10, e037161. doi:10.1136/bmjopen-2020-037161
Al Turk, L., Wang, S., Krause, P., Wawrzynski, J., Saleh, G. M., Alsawadi, H., et al. (2020). Evidence Based Prediction and Progression Monitoring on Retinal Images from Three Nations. Transl. Vis. Sci. Technol. 9, 44. doi:10.1167/tvst.9.2.44
Al-Bander, B., Williams, B. M., Al-Nuaimy, W., Al-Taee, M. A., Pratt, H., and Zheng, Y. L. (2018). Dense Fully Convolutional Segmentation of the Optic Disc and Cup in Colour Fundus for Glaucoma Diagnosis. Symmetry-Basel 10, 16. doi:10.3390/sym10040087
Andersson, S., Heijl, A., Bizios, D., and Bengtsson, B. (2013). Comparison of Clinicians and an Artificial Neural Network Regarding Accuracy and Certainty in Performance of Visual Field Assessment for the Diagnosis of Glaucoma. Acta Ophthalmol. 91, 413–417. doi:10.1111/j.1755-3768.2012.02435.x
Araújo, T., Aresta, G., Mendonça, L., Penas, S., Maia, C., Carneiro, Â., et al. (2020). DR|GRADUATE: Uncertainty-Aware Deep Learning-Based Diabetic Retinopathy Grading in Eye Fundus Images. Med. Image Anal. 63, 101715. doi:10.1016/j.media.2020.101715
Asaoka, R., Murata, H., Hirasawa, K., Fujino, Y., Matsuura, M., Miki, A., et al. (2019). Using Deep Learning and Transfer Learning to Accurately Diagnose Early-Onset Glaucoma from Macular Optical Coherence Tomography Images. Am. J. Ophthalmol. 198, 136–145. doi:10.1016/j.ajo.2018.10.007
Awad, M., and Khanna, R. (2015). Efficient Learning Machines: Theories, Concepts, and Applications for Engineers and System Designers. Springer nature.
Balyen, L., and Peto, T. (2019). Promising Artificial Intelligence-Machine Learning-Deep Learning Algorithms in Ophthalmology. Asia Pac J. Ophthalmol. (Phila) 8, 264–272. doi:10.22608/APO.2018479
Bellemo, V., Lim, Z. W., Lim, G., Nguyen, Q. D., Xie, Y., Yip, M. Y. T., et al. (2019). Artificial Intelligence Using Deep Learning to Screen for Referable and Vision-Threatening Diabetic Retinopathy in Africa: a Clinical Validation Study. Lancet Digit. Health 1, E35–E44. doi:10.1016/S2589-7500(19)30004-4
Boudry, C., Al Hajj, H., Arnould, L., and Mouriaux, F. (2022). Analysis of International Publication Trends in Artificial Intelligence in Ophthalmology. Graefes Arch. Clin. Exp. Ophthalmol. 260, 1779–1788. doi:10.1007/s00417-021-05511-7
Bourouis, A., Feham, M., Hossain, M. A., and Zhang, L. (2014). An Intelligent Mobile Based Decision Support System for Retinal Disease Diagnosis. Decis. Support Syst. 59, 341–350. doi:10.1016/j.dss.2014.01.005
Bussel, , Wollstein, G., and Schuman, J. S. (2014). OCT for Glaucoma Diagnosis, Screening and Detection of Glaucoma Progression. Br. J. Ophthalmol. 98 Suppl 2, ii15–9. doi:10.1136/bjophthalmol-2013-304326
Dai, Q., Liu, X., Lin, X., Fu, Y., Chen, C., Yu, X., et al. (2021). A Novel Meibomian Gland Morphology Analytic System Based on a Convolutional Neural Network. Ieee Access 9, 23083–23094. doi:10.1109/access.2021.3056234
Devalla, S. K., Subramanian, G., Pham, T. H., Wang, X., Perera, S., Tun, T. A., et al. (2019). A Deep Learning Approach to Denoise Optical Coherence Tomography Images of the Optic Nerve Head. Sci. Rep. 9, 14454. doi:10.1038/s41598-019-51062-7
Dong, Y., Liu, Y. L., Yu, J. G., Qi, S. X., and Liu, H. J. (2021). Mapping Research Trends in Diabetic Retinopathy from 2010 to 2019 A Bibliometric Analysis. Medicine 100, 12. doi:10.1097/md.0000000000023981
Du, Y., Liu, G., Feng, G., and Chen, Z. (2014). Speckle Reduction in Optical Coherence Tomography Images Based on Wave Atoms. J. Biomed. Opt. 19, 056009. doi:10.1117/1.JBO.19.5.056009
Elsawy, A., and Abdel-Mottaleb, M. (2021). A Novel Network with Parallel Resolution Encoders for the Diagnosis of Corneal Diseases. IEEE Trans. Biomed. Eng. 68, 3671–3680. doi:10.1109/TBME.2021.3082152
Fang, L., Cunefare, D., Wang, C., Guymer, R. H., Li, S., and Farsiu, S. (2017). Automatic Segmentation of Nine Retinal Layer Boundaries in OCT Images of Non-exudative AMD Patients Using Deep Learning and Graph Search. Biomed. Opt. Express 8, 2732–2744. doi:10.1364/BOE.8.002732
Fu, H., Cheng, J., Xu, Y., Wong, D. W. K., Liu, J., and Cao, X. (2018). Joint Optic Disc and Cup Segmentation Based on Multi-Label Deep Network and Polar Transformation. IEEE Trans. Med. Imaging 37, 1597–1605. doi:10.1109/TMI.2018.2791488
Gargeya, R., and Leng, T. (2017). Automated Identification of Diabetic Retinopathy Using Deep Learning. Ophthalmology 124, 962–969. doi:10.1016/j.ophtha.2017.02.008
Giardini, M. E., and Livingstone, I. A. T. (2020). “Extending the Reach and Task-Shifting Ophthalmology Diagnostics through Remote Visualisation,”. Biomedical Visualisation. Editor P. M. Rea (Cham: Springer International Publishing Ag), Vol. 8. doi:10.1007/978-3-030-47483-6_9
Grzybowski, A., and Brona, P. (2021). Analysis and Comparison of Two Artificial Intelligence Diabetic Retinopathy Screening Algorithms in a Pilot Study: IDx-DR and Retinalyze. J. Clin. Med. 10, 8. doi:10.3390/jcm10112352
Gulshan, V., Peng, L., Coram, M., Stumpe, M. C., Wu, D., Narayanaswamy, A., et al. (2016). Development and Validation of a Deep Learning Algorithm for Detection of Diabetic Retinopathy in Retinal Fundus Photographs. JAMA 316, 2402–2410. doi:10.1001/jama.2016.17216
Hamet, P., and Tremblay, J. (2017). Artificial Intelligence in Medicine. Metabolism 69S, S36–S40. doi:10.1016/j.metabol.2017.01.011
Hemalakshmi, G., Santhi, D., R. S. Mani, V., Geetha, A., and B. Prakash, N. (2020). Deep Residual Network Based on Image Priors for Single Image Super Resolution in FFA Images. Cmes-Computer Model. Eng. Sci. 125, 125–143. doi:10.32604/cmes.2020.011331
Hirsch, J. E. (2005). An Index to Quantify an Individual's Scientific Research Output. Proc. Natl. Acad. Sci. U. S. A. 102, 16569–16572. doi:10.1073/pnas.0507655102
Juneja, M., Singh, S., Agarwal, N., Bali, S., Gupta, S., Thakur, N., et al. (2020). Automated Detection of Glaucoma Using Deep Learning Convolution Network (G-Net). Multimed. Tools Appl. 79, 15531–15553. doi:10.1007/s11042-019-7460-4
Kessel, K., Mattila, J., Linder, N., Kivelä, T., and Lundin, J. (2020). Deep Learning Algorithms for Corneal Amyloid Deposition Quantitation in Familial Amyloidosis. Ocul. Oncol. Pathol. 6, 58–65. doi:10.1159/000500896
Koh, B. M. Q. R., Banu, R., Nusinovici, S., and Sabanayagam, C. (2021). 100 Most-Cited Articles on Diabetic Retinopathy. Br. J. Ophthalmol. 105, 1329–1336. doi:10.1136/bjophthalmol-2020-316609
Kora, P., Ooi, C. P., Faust, O., Raghavendra, U., Gudigar, A., Chan, W. Y., et al. (2022). Transfer Learning Techniques for Medical Image Analysis: A Review. Biocybern. Biomed. Eng. 42, 79–107. doi:10.1016/j.bbe.2021.11.004
Kugelman, J., Alonso-Caneiro, D., Read, S. A., Hamwood, J., Vincent, S. J., Chen, F. K., et al. (2019). Automatic Choroidal Segmentation in OCT Images Using Supervised Deep Learning Methods. Sci. Rep. 9, 13298. doi:10.1038/s41598-019-49816-4
Li, Q., Feng, B., Xie, L., Liang, P., Zhang, H., and Wang, T. (2016). A Cross-Modality Learning Approach for Vessel Segmentation in Retinal Images. IEEE Trans. Med. Imaging 35, 109–118. doi:10.1109/TMI.2015.2457891
Li, S., Zhao, R., and Zou, H. (2022). Artificial Intelligence for Diabetic Retinopathy. Chin. Med. J. 135, 253–260. doi:10.1097/cm9.0000000000001816
Li, Z., He, Y., Keel, S., Meng, W., Chang, R. T., and He, M. (2018). Efficacy of a Deep Learning System for Detecting Glaucomatous Optic Neuropathy Based on Color Fundus Photographs. Ophthalmology 125, 1199–1206. doi:10.1016/j.ophtha.2018.01.023
Liskowski, P., and Krawiec, K. (2016). Segmenting Retinal Blood Vessels with Deep Neural Networks. IEEE Trans. Med. Imaging 35, 2369–2380. doi:10.1109/TMI.2016.2546227
Mcgarry, G., and Deriche, M. (1997). “Modelling Mammographic Images Using Fractional Brownian Motion,” in IEEE Region 10 Annual Conference on Speech and Image Technologies for Computing and Telecommunications (IEEE TENCON 97), Brisbane, Australia, Dec 02-04 1997, 299–302.
Metha, P., Peterson, C. A., Wen, J. C., Banitt, M. R., Chen, P. P., Bojikian, K. D., et al. (2021). Automated Detection of Glaucoma with Interpretable Machine Learning Using Clinical Data and Multimodal Retinal Images. Am. J. Ophthalmol. 231, 154–169.
Miller, R. A. (1994). Medical Diagnostic Decision Support Systems-Ppast, Present, and Future: a Threaded Bibliography and Brief Commentary. J. Am. Med. Inf. Assoc. 1, 8–27. doi:10.1136/jamia.1994.95236141
Myer, C., Abdelrahman, L., Banerjee, S., Khattri, R. B., Merritt, M. E., Junk, A. K., et al. (2020). Aqueous Humor Metabolite Profile of Pseudoexfoliation Glaucoma Is Distinctive. Mol. Omics 16, 425–435. doi:10.1039/c9mo00192a
National Health Commission (2020). Transcript of the Regular Press Conference of the National Health Commission. Available at http://www.nhc.gov.cn/xcs/s3574/202006/1f519d91873948d88a77a35a427c3944.shtml.2020.6.5.
Nezu, N., Usui, Y., Asakage, M., Shimizu, H., Tsubota, K., Narimatsu, A., et al. (2020). Distinctive Tissue and Serum MicroRNA Profile of IgG4-Related Ophthalmic Disease and MALT Lymphoma. J. Clin. Med. 9, 18. doi:10.3390/jcm9082530
Oh, E., Yoo, T. K., and Hong, S. (2015). Artificial Neural Network Approach for Differentiating Open-Angle Glaucoma from Glaucoma Suspect without a Visual Field Test. Invest. Ophthalmol. Vis. Sci. 56, 3957–3966. doi:10.1167/iovs.15-16805
President, E. O. O. T., Holdren, J. P., and Smith, M. (2016). Preparing for the Future of Artificial Intelligence. Washington: National Science and Technology Council.
Ran, A. R., Tham, C. C., Chan, P. P., Cheng, C. Y., Tham, Y. C., Rim, T. H., et al. (2021). Deep Learning in Glaucoma with Optical Coherence Tomography: a Review. Eye (Lond) 35, 188–201. doi:10.1038/s41433-020-01191-5
Raumviboonsuk, P., Krause, J., Chotcomwongse, P., Sayres, R., Raman, R., Widner, K., et al. (2019). Deep Learning versus Human Graders for Classifying Diabetic Retinopathy Severity in a Nationwide Screening Program. NPJ Digit. Med. 2, 25. doi:10.1038/s41746-019-0099-8
Ruiz Hidalgo, I., Rozema, J. J., Saad, A., Gatinel, D., Rodriguez, P., Zakaria, N., et al. (2017). Validation of an Objective Keratoconus Detection System Implemented in a Scheimpflug Tomographer and Comparison with Other Methods. Cornea 36, 689–695. doi:10.1097/ICO.0000000000001194
Saeed, A. Q., Abdullah, S., Che-Hamzah, J., and Ghani, A. T. A. (2021). Accuracy of Using Generative Adversarial Networks for Glaucoma Detection: Systematic Review and Bibliometric Analysis. J. Med. Internet Res. 23, 32. doi:10.2196/27414
Saikia, S. J., and Nirmala, S. R. (2022). Identification of Disease Genes and Assessment of Eye-Related Diseases Caused by Disease Genes Using JMFC and GDLNN. Comput. Methods Biomech. Biomed. Engin 25, 359–370. doi:10.1080/10255842.2021.1955358
Song, X., Liu, Z., Li, L., Gao, Z., Fan, X., Zhai, G., et al. (2021). Artificial Intelligence CT Screening Model for Thyroid-Associated Ophthalmopathy and Tests under Clinical Conditions. Int. J. Comput. Assist. Radiol. Surg. 16, 323–330. doi:10.1007/s11548-020-02281-1
Szeskin, A., Yehuda, R., Shmueli, O., Levy, J., and Joskowicz, L. (2021). A Column-Based Deep Learning Method for the Detection and Quantification of Atrophy Associated with AMD in OCT Scans. Med. Image Anal. 72, 12. doi:10.1016/j.media.2021.102130
Ting, D. S. W., Cheung, C. Y., Lim, G., Tan, G. S. W., Quang, N. D., Gan, A., et al. (2017). Development and Validation of a Deep Learning System for Diabetic Retinopathy and Related Eye Diseases Using Retinal Images from Multiethnic Populations with Diabetes. JAMA 318, 2211–2223. doi:10.1001/jama.2017.18152
Ting, D. S. W., Pasquale, L. R., Peng, L., Campbell, J. P., Lee, A. Y., Raman, R., et al. (2019). Artificial Intelligence and Deep Learning in Ophthalmology. Br. J. Ophthalmol. 103, 167–175. doi:10.1136/bjophthalmol-2018-313173
Wan, C., Li, H., Cao, G. F., Jiang, Q., and Yang, W. H. (2021a). An Artificial Intelligent Risk Classification Method of High Myopia Based on Fundus Images. J. Clin. Med. 10, 13. doi:10.3390/jcm10194488
Wan, C., Wu, J., Li, H., Yan, Z., Wang, C., Jiang, Q., et al. (2021b). Optimized-Unet: Novel Algorithm for Parapapillary Atrophy Segmentation. Front. Neurosci. 15, 10. doi:10.3389/fnins.2021.758887
Wan, C., Zhou, X., You, Q., Sun, J., Shen, J., Zhu, S., et al. (2021c). Retinal Image Enhancement Using Cycle-Constraint Adversarial Network. Front. Med. (Lausanne) 8, 16.
Wu, C. M., Chen, Y. C., and Hsieh, K. S. (1992). Texture Features for Classification of Ultrasonic Liver Images. IEEE Trans. Med. Imaging 11, 141–152. doi:10.1109/42.141636
Xu, J., Shen, J., Wan, C., Jiang, Q., Yan, Z., and Yang, W. (2022). A Few-Shot Learning-Based Retinal Vessel Segmentation Method for Assisting in the Central Serous Chorioretinopathy Laser Surgery. Front. Med. (Lausanne) 9, 821565. doi:10.3389/fmed.2022.821565
Xu, J., Wan, C., Yang, W., Zheng, B., Yan, Z., and Shen, J. (2021b). A Novel Multi-Modal Fundus Image Fusion Method for Guiding the Laser Surgery of Central Serous Chorioretinopathy. Math. Biosci. Eng. 18, 4797–4816. doi:10.3934/mbe.2021244
Xu, J., Shen, J., Jiang, Q., Wan, C., Yan, Z., and Yang, W. (2021a). Research on the Segmentation of Biomarker for Chronic Central Serous Chorioretinopathy Based on Multimodal Fundus Image. Dis. Markers 2021, 11. doi:10.1155/2021/1040675
Xu, W., Jin, L., Zhu, P. Z., He, K., Yang, W. H., and Wu, M. N. (2021c). Implementation and Application of an Intelligent Pterygium Diagnosis System Based on Deep Learning. Front. Psychol. 12, 759229. doi:10.3389/fpsyg.2021.759229
Yousefi, S., Goldbaum, M. H., Balasubramanian, M., Jung, T. P., Weinreb, R. N., Medeiros, F. A., et al. (2014). Glaucoma Progression Detection Using Structural Retinal Nerve Fiber Layer Measurements and Functional Visual Field Points. IEEE Trans. Biomed. Eng. 61, 1143–1154. doi:10.1109/TBME.2013.2295605
Yun, W. L., Mookiah, M. R. K., and Koh, J. E. W. (2014a). Automated Detection of Proliferative Diabetic Retinopathy Using Brownian Motion Features. J. Med. Imaging Hlth Inf. 4, 250–254. doi:10.1166/jmihi.2014.1248
Yun, W. L., Mookiah, M. R. K., and Koh, J. E. W. (2014b). Glaucoma Classification Using Brownian Motion and Discrete Wavelet Transform. J. Med. Imaging Hlth Inf. 4, 621–627. doi:10.1166/jmihi.2014.1299
Zamani, N. S. M., Zaki, W. M. D. W., Huddin, A. B., Hussain, A., Mutalib, H. A., and Ali, A. (2020). Automated Pterygium Detection Using Deep Neural Network. Ieee Access 8, 191659–191672. doi:10.1109/access.2020.3030787
Zheng, B., Jiang, Q., Lu, B., He, K., Wu, M. N. A., Hao, X. L., et al. (2021). Five-Category Intelligent Auxiliary Diagnosis Model of Common Fundus Diseases Based on Fundus Images. Transl. Vis. Sci. Technol. 10, 10. doi:10.1167/tvst.10.7.20
Keywords: ophthalmic disease, artificial intelligence, diagnosis, bibliometric, CiteSpace
Citation: Zhao J, Lu Y, Zhu S, Li K, Jiang Q and Yang W (2022) Systematic Bibliometric and Visualized Analysis of Research Hotspots and Trends on the Application of Artificial Intelligence in Ophthalmic Disease Diagnosis. Front. Pharmacol. 13:930520. doi: 10.3389/fphar.2022.930520
Received: 28 April 2022; Accepted: 23 May 2022;
Published: 08 June 2022.
Edited by:
Yuanpeng Zhang, Nantong University, ChinaReviewed by:
Huiying Liu, Institute for Infocomm Research (A∗STAR), SingaporeCopyright © 2022 Zhao, Lu, Zhu, Li, Jiang and Yang. This is an open-access article distributed under the terms of the Creative Commons Attribution License (CC BY). The use, distribution or reproduction in other forums is permitted, provided the original author(s) and the copyright owner(s) are credited and that the original publication in this journal is cited, in accordance with accepted academic practice. No use, distribution or reproduction is permitted which does not comply with these terms.
*Correspondence: Weihua Yang, YmVuYmVuMDYwNkAxMzkuY29t; Qin Jiang, anFpbjcxMEB2aXAuc2luYS5jb20=; Keran Li, a2F0aHlrZXJhbjg2MDMyN0AxMjYuY29t
†These authors have contributed equally to this work
Disclaimer: All claims expressed in this article are solely those of the authors and do not necessarily represent those of their affiliated organizations, or those of the publisher, the editors and the reviewers. Any product that may be evaluated in this article or claim that may be made by its manufacturer is not guaranteed or endorsed by the publisher.
Research integrity at Frontiers
Learn more about the work of our research integrity team to safeguard the quality of each article we publish.