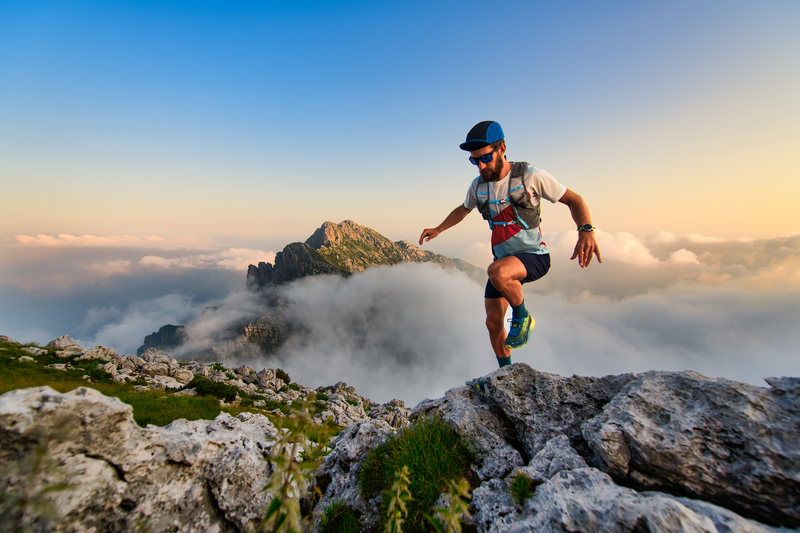
95% of researchers rate our articles as excellent or good
Learn more about the work of our research integrity team to safeguard the quality of each article we publish.
Find out more
EDITORIAL article
Front. Pharmacol. , 20 May 2022
Sec. Pharmacogenetics and Pharmacogenomics
Volume 13 - 2022 | https://doi.org/10.3389/fphar.2022.928527
This article is part of the Research Topic The Potential of Machine-learning in Pharmacogenetics, Pharmacogenomics and Pharmacoepidemiology View all 5 articles
Editorial on the Research Topic
The Potential of Machine-learning in Pharmacogenetics, Pharmacogenomics and Pharmacoepidemiology
Recent advances in deep learning, natural language processing, and information retrieval show great potential for enhancing the knowledge and processes in the fields of pharmacogenetics, pharmacogenomics, and pharmacoepidemiology. These techniques allow for the unprecedented analysis of large unstructured datasets that would otherwise be intractable, such as finding relevant patterns and clusters in the data being analyzed (e.g. free-text notes in Electronic Health Records, or Pubmed articles or abstracts). From predicting patient outcomes and guiding drug choices that prevent adverse reactions to drug discovery and repurposing, artificial intelligence holds great promise when it comes to the development of support systems to aid clinical decision making in the coming years.
In this Frontiers Research Topic, we invited potential contributors to submit their work and latest advances in deep and machine learning applied to pharmacogenetics, pharmacogenomics, and pharmacoepidemiology.
One of the potential applications of ML in pharmacogenomics is to improve dose prediction accuracy, improving outcomes and reducing adverse drug events (ADEs). In this context, Steiner et al. employ regression to predict stable warfarin dosages in a diverse cohort that includes US Latinos and Latin Americans, concluding that the inclusion of ethnicity and warfarin indication, in addition to the International Warfarin Pharmacogenetics Consortium’s recommended set of variables can result in a small but significant improvement in correct dose prediction.
Also in the field of ADE avoidance, Kang et al. aim to predict the risk of fetal teratogenicity caused by prescribed antiseizure medications (ASMs), which is especially relevant in novel ASMs where prior evidence is limited. To this end, they employ a Support Vector Machine on a series of curated FDA-approved ASMs with risk for teratogenicity, using data from the FDA Spontaneous Adverse Events Reporting System (FAERS) as ground truth and outperforming state-of-the-art approaches.
Pandi et al. propose text-mining to automatically compute potential pharmacogenomic associations. To achieve this, PubMed abstracts and articles are selected, PubTator Central annotations are used for entity recognition. Extracted sentences are then used to extract genome-chemical pairs using different classifiers.
Finally, Fusaroli et al. propose Adversome, a new approach to detect ADEs and other disease- and comorbidity-related syndromes in rapidly changing and low-resource environments such as the COVID epidemic. This network also uses FAERS as their data source to better pinpoint ADEs caused by drugs repurposed for COVID, better adapted than conventional approaches in the aforementioned context.
We are happy to announce that this Research Topic has been re-launched and we look forward to new contributions in the coming months.
All authors listed have made a substantial, direct, and intellectual contribution to the work and approved it for publication.
The authors declare that the research was conducted in the absence of any commercial or financial relationships that could be construed as a potential conflict of interest.
All claims expressed in this article are solely those of the authors and do not necessarily represent those of their affiliated organizations, or those of the publisher, the editors and the reviewers. Any product that may be evaluated in this article, or claim that may be made by its manufacturer, is not guaranteed or endorsed by the publisher.
Keywords: machine learning, artificial intellegence, pharmacogenetics, pharmacoepidemiology, pharmacogenomics
Citation: Garcia-Agundez A, García-Martín E and Eickhoff C (2022) Editorial: The Potential of Machine Learning in Pharmacogenetics, Pharmacogenomics and Pharmacoepidemiology. Front. Pharmacol. 13:928527. doi: 10.3389/fphar.2022.928527
Received: 25 April 2022; Accepted: 05 May 2022;
Published: 20 May 2022.
Edited and reviewed by:
Volker Martin Lauschke, Karolinska Institutet (KI), SwedenCopyright © 2022 Garcia-Agundez, García-Martín and Eickhoff. This is an open-access article distributed under the terms of the Creative Commons Attribution License (CC BY). The use, distribution or reproduction in other forums is permitted, provided the original author(s) and the copyright owner(s) are credited and that the original publication in this journal is cited, in accordance with accepted academic practice. No use, distribution or reproduction is permitted which does not comply with these terms.
*Correspondence: Augusto Garcia-Agundez, YXVndXN0b19nYXJjaWFAYnJvd24uZWR1
Disclaimer: All claims expressed in this article are solely those of the authors and do not necessarily represent those of their affiliated organizations, or those of the publisher, the editors and the reviewers. Any product that may be evaluated in this article or claim that may be made by its manufacturer is not guaranteed or endorsed by the publisher.
Research integrity at Frontiers
Learn more about the work of our research integrity team to safeguard the quality of each article we publish.