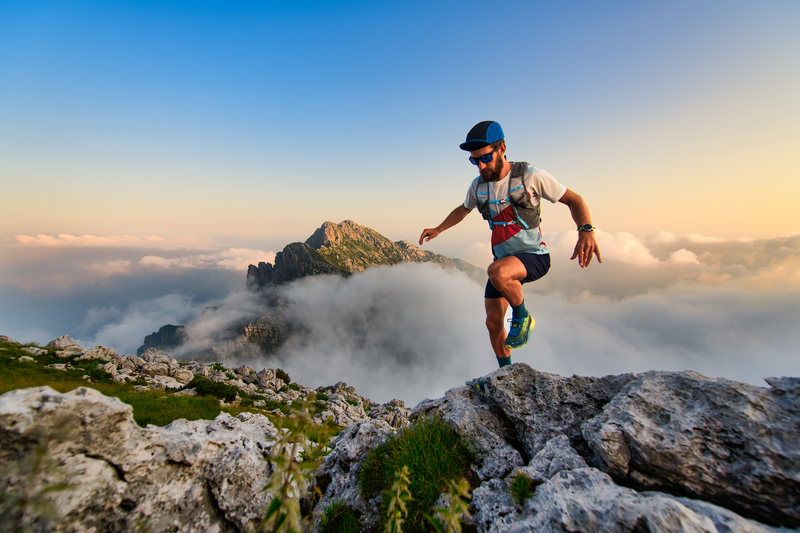
94% of researchers rate our articles as excellent or good
Learn more about the work of our research integrity team to safeguard the quality of each article we publish.
Find out more
ORIGINAL RESEARCH article
Front. Pharmacol. , 07 September 2022
Sec. Ethnopharmacology
Volume 13 - 2022 | https://doi.org/10.3389/fphar.2022.924478
This article is part of the Research Topic From ancient civilization to the present day: the diversity of traditional medicinal plant in the Middle East View all 5 articles
Type 2 diabetes mellitus (T2DM) is a heterogeneous disease with numerous abnormal targets and pathways involved in insulin resistance, low-grade inflammation, oxidative stress, beta cell dysfunction, and epigenetic factors. Botanical drugs provide a large chemical space that can modify various targets simultaneously. Matricaria aurea (MA, golden chamomile) is a widely used herb in Middle Eastern communities for many ailments, including diabetes mellitus, without any scientific basis to support this tradition. For the first time, this study aimed to investigate the possible antidiabetic activity of MA in a type 2 diabetic rat model, identify chemical constituents by LC-MS/MS, and then elucidate the molecular mechanism(s) using enzyme activity assays, q-RTPCR gene expression analysis, network pharmacology analysis, and molecular docking simulation. Our results demonstrated that only the polar hydroethanolic extract of MA had remarkable antidiabetic activity. Furthermore, it improved dyslipidemia, insulin resistance status, ALT, and AST levels. LC-MS/MS analysis of MA hydroethanolic extract identified 62 compounds, including the popular chamomile flavonoids apigenin and luteolin, other flavonoids and their glycosides, coumarin derivatives, and phenolic acids. Based on pharmacokinetic screening and literature, 46 compounds were chosen for subsequent network analysis, which linked to 364 candidate T2DM targets from various databases and literature. The network analysis identified 123 hub proteins, including insulin signaling and metabolic proteins: IRS1, IRS2, PIK3R1, AKT1, AKT2, MAPK1, MAPK3, and PCK1, inflammatory proteins: TNF and IL1B, antioxidant enzymes: CAT and SOD, and others. Subsequent filtering identified 40 crucial core targets (major hubs) of MA in T2DM treatment. Functional enrichment analyses of the candidate targets revealed that MA targets were mainly involved in the inflammatory module, energy-sensing/endocrine/metabolic module, and oxidative stress module. q-RTPCR gene expression analysis showed that MA hydroethanolic extract was able to significantly upregulate PIK3R1 and downregulate IL1B, PCK1, and MIR29A. Moreover, the activity of the antioxidant hub enzymes was substantially increased. Molecular docking scores were also consistent with the networks’ predictions. Based on experimental and computational analysis, this study revealed for the first time that MA exerted antidiabetic action via simultaneous modulation of multiple targets and pathways, including inflammatory pathways, energy-sensing/endocrine/metabolic pathways, and oxidative stress pathways.
Type 2 Diabetes Mellitus T2DM is a multifarious metabolic syndrome that has a strong association with hyperglycemia, hyperlipidemia, and obesity. It encompasses a lot of disturbances, including defective metabolism of carbohydrates, lipids, and proteins; oxidative stress; chronic low-grade inflammation; and epigenetic factors, such as regulatory microRNAs. T2DM has two major pathophysiological arms: resistance to the action of insulin, primarily in the liver and other insulin-sensitive tissues; and pancreatic beta cell secretory dysfunction (Czech, 2017; Galicia-Garcia et al., 2020). Abnormal Changes in signaling proteins associated with insulin signaling clearly play a crucial part in the insulin resistance state. Diabetes patients have insulin signal transduction pathway changes or defects, which are associated with lower levels of the molecules of insulin signaling such as “Insulin receptor substrate-1 (IRS-1) and phosphatidylinositol 3-kinase PI3K” (Dhanya et al., 2017). Hepatic insulin resistance causes increased glucose production from the liver owning to the failure of insulin signal to modulate the transcription factor foxo1, and thus fails to suppress transcription of phosphoenolpyruvate carboxy kinase (PEPCK or PCK1) and the gluconeogenesis pathway (Peng et al., 2020; Ge et al., 2021). Upregulation of PEPCK, the rate limiting enzyme of gluconeogenesis, takes place in almost all diabetes models as a result of a lack of insulin and/or its action. A large body of evidence suggests a direct correlation between PEPCK expression, insulin resistance, and glycemic status (Elo et al., 2007; Gómez-Valadés et al., 2008; Xu et al., 2019).
Oxidative stress linked to obesity and overnutrition has been proposed as a single unifying mechanism underlying insulin resistance, beta cell dysfunction, glucose intolerance, development of T2DM, and progression of the disease leading to macro and micro complications (Ceriello and Motz, 2004; Wright et al., 2006; Tangvarasittichai, 2015; Burgos-Morón et al., 2019). Oxidative stress is a covariate of glucose in both the development of diabetes and recovery during antidiabetic therapy. In addition, oxidative stress and antioxidant biomarkers show a strong correlation with glycemic status and glucose metabolism (Kulkarni et al., 2014). Moreover, oxidative stress increases PEPCK expression in the liver, which is a hallmark of hepatic insulin resistance (Ito et al., 2006). Overnutrition and its consequences, such as oxidative stress, potentiate the production of inflammatory cytokines, particularly IL-1β, TNF-α, IL-6, and many other cytokines and chemokines that are IL-1β-dependent. The activation of nuclear factor kappa B “NF-κB” in the liver, which controls the generation of proinflammatory cytokines like IL-1β, is thought to cause local and systemic insulin resistance (Cai et al., 2005; Rehman and Akash, 2016; Oguntibeju, 2019).
Another player in the arena of T2DM biology is microRNAs, which have emerged as etiological agents, diagnostic and prognostic biomarkers in T2DM, and promising therapeutic targets. MicroRNA 29a has been reported to be one of the strongest candidate Mirna regulatory hubs in the T2DM gene network, as well as one of the most upregulated miRNAs in various insulin-sensitive tissues in T2DM patients and rodent models of diabetes. Several studies have found that abnormally high expression of hepatic miRNA 29a in high-fat diet and genetic animal models is causally linked to insulin resistance, hyperlipidemia, and prevents insulin from downregulating PEPCK, resulting in unrestrained gluconeogenesis. Molecularly, Mirna 29a targets the regulatory unit p85 of PI3-K, a key signaling molecule in the insulin transduction pathway, causing insulin signal truncation, and contributing to insulin resistance (He et al., 2007; Pandey et al., 2011; Baran-Gale et al., 2013; Kurtz et al., 2014, 2015; Bhatia et al., 2015; Zhu and Leung, 2015; Dooley et al., 2016).
Given the increased incidence of T2DM (Khan et al., 2020) and the failure of current drugs to control the multiple pathophysiological processes in the long term, it is necessary to move forward in the search for novel medications that provide better solutions for the multiple abnormal targets and pathways of this disease (Gomez-Peralta and Abreu Padín, 2014). Plants reported in traditional medicine to possess antidiabetic activity provide an interesting and wealthy resource to find new drugs in their hugely diverse compounds. Historically, the development of the majority of established drugs, including the blockbuster drug metformin, was based on the identification of compounds from natural sources (Witters, 2001; Li and Vederas, 2009). Since ancient times, the Egyptians, Greeks, Romans, and Arabs have used chamomile, one of the most popular herbs in the world. Matricaria aurea, also known as golden chamomile (Soubra et al., 2018), is reported to be used in traditional medicine in some Middle Eastern countries as an antidiabetic herb (Yaniv et al., 1987; Al-Mustafa and Al-Thuniba, 2008). It is also widely used as a therapy for a variety of other conditions, including inflammation, respiratory disorders, facilitating delivery during pregnancy, and infant health care (Ali-Shtayeh et al., 2015; Abdelhalim et al., 2017). Previous experimental work, which is mainly in vitro, reported the antioxidant (Mohammad Al-Ismail and Aburjai, 2004), anti-inflammatory (Khodadadi et al., 2011), anti-ulcerative colitis (Minaiyan et al., 2011), analgesic (Qnais, 2011), antibacterial, and anticancer (Kheder et al., 2014; Ahmad et al., 2020, 2021) biological activity of Matricaria aurea. To the best of our knowledge, there is no scientific study that has investigated the antidiabetic activity of this plant.
Botanical extracts with numerous constituents may elicit pharmacological activity by modulating a variety of targets and signaling pathways, which could help in the treatment of T2DM. However, the complexities of herbal extracts may render the mission to discover the underlying molecular mechanisms more difficult. In order to overcome this problem, a new research methodology called network pharmacology has been developed to find the targets of drugs and elucidate mechanism(s) of action. Network pharmacology is a new theoretical framework and methodology grounded on the advancement of omics technologies, bioinformatics, systems biology, mathematics, and pharmacology to examine the impact of drugs on both the interactome and diseasome levels. Instead of the old paradigm of “one drug-one target-one disease,” network pharmacology adopts a new paradigm of “one or multi drugs-multi targets or multiple-component-therapeutics/network-targets.” This approach makes it extremely useful for analyzing drug combinations, especially botanical formulas (Chandran et al., 2017; Li et al., 2017; Zhang et al., 2019).
In this article, we report for the first time the antidiabetic activity of the polar hydroethanolic extract of Matricaria aurea. Furthermore, it improved dyslipidemia, oxidative stress status, and gene expression of important genes related to T2DM in an experimental rat model, and the active constituents were identified using the UPLC-Q-TOF MS/MS system. Moreover, we used network pharmacology principles and methods to decipher the potential mechanism of action of MA in T2DM treatment. Finally, we checked the reliability of network predictions against molecular docking simulation, q-RTPCR data, and enzyme activity assay data, as shown in Figure 1. Our findings lay the theoretical basis for the development of new antidiabetic drugs, more detailed mechanistic studies, and clinical trials.
FIGURE 1. Graphical abstract. Adjusting the size of the images, resolution, and combining the images was carried out using Photoshop.
The aerial parts of Matricaria aurea (Loefl.) Sch. Bip. (Family: Asteraceae) were collected from its wild habitat in Wadi Habis (Latitude 31° 22′ 81″N and Longitude 27° 3′ 54″E), Mersa Matruh governorate, Northwestern Coast, Egypt. The plant identity was identified and authenticated by Dr. Omran Ghaly, Head of the Plant Taxonomy Unit, Desert Research Center, Matria, Cairo, Egypt. A voucher specimen was deposited at the herbarium of the Desert Research Center (CAIH) with code number: CAIH-970-R.
MA aerial parts were air-dried in the shade then crushed into a powder (3.45 kg). The MA powder was successively extracted as described by (Pérez-Bonilla et al., 2013). In a Soxhlet apparatus (500 ml), four different solvents were used in ascending order of polarity (n-hexane, dichloromethane, ethyl acetate, and ethanol (95%)). All extracts of various organic solvents were dried by evaporating each solvent at 40°C using a rotary evaporator (R-205-Buchi, Germany), yielding 102.4 g of ethanol 95% extract, 24 g of ethyl acetate extract, 27.9 g of dichloromethane extract, and 45.2 g of hexane extract, respectively. In this study, two doses of 100 and 200 mg/kg were selected and used (Minaiyan et al., 2011; Kaseb et al., 2018; Hajizadeh-Sharafabad et al., 2020). The two doses were prepared by dissolving an appropriate amount of the dried extracts in 1 ml of Tween 20. Following that, 9 ml of 0.9% NaCl was added to each mixture. To prepare the vehicle, 1 ml of Tween 20 was dissolved in 9 ml of 0.9% NaCl.
Healthy male Wistar rats, 5 weeks old and weighing 90–110 g were obtained from the national research center (Cairo, Egypt). Rats were left for 1 week for acclimatization before beginning the experiment. Throughout the experiment, rats were maintained on 12 h light/dark cycle and temperature control (20–24°C). The animals were fed a standard chow diet and given water on an ad libitum basis throughout the experimental period, except when otherwise stated. Animal handling and experimental procedures were approved by the Research Ethics Committee of the Faculty of Pharmacy, Suez Canal University, Ismailia, Egypt (Code: 201803RA1), which are in accordance with the Guide for the Care and Use of Laboratory Animals (National Research Council, 2011).
Rats were divided into two dietary regimens at the beginning of the experiment: normal pellet diet (NPD; 67% carbohydrate, 21% protein, and 12% calories) and high fat diet (HFD; 17% carbohydrate, 25% protein, and 58% fat, as a percentage of total kcal) (detailed composition and energy density for both diets are presented in Supplementary Data Sheet S1) (Abo-elmatty et al., 2013). After 4 weeks of diet manipulation, rats fed on the HFD, were given a single intraperitoneal injection of 40 mg/kg/body weight of streptozotocin (STZ; Cat No: SLBJ7785V, Sigma Aldrich, CHEMIE) dissolved in di-sodium citrate buffer (pH 4.5), while normal non-diabetic rats received a single intraperitoneal injection of the vehicle (Skovsø, 2014). Ten days following STZ injection, fasting glucose levels were estimated in blood samples from the tail tip using the glucometer method (Accutrend alpha-Roche Diagnostic, Germany). Only rats with fasting blood glucose level >200 mg/dl were considered diabetic and included in the study.
Seventy diabetic rats were randomly assigned into 10 groups of seven animals each. Nine groups received either pioglitazone (standard drug, PIO) at a dose of 20 mg/kg (Abo-elmatty et al., 2013) or one of two doses at 100 and 200 mg/kg of MA extracts: 95% ethanol extract (ETH) or ethyl acetate extract (EA) or dichloromethane extract (DCM) or hexane extract (HEX). The remaining group served as the diabetic control group (DC). In addition, another group (seven rats) without induction of diabetes served as a normal control group (NC) and received the vehicle. Starting on the 11th day after diabetes was induced, the vehicle, the standard drug, and plant extracts were given once a day by oral gavage for 4 weeks.
Rats were fasted overnight, anesthetized with diethyl ether, and euthanized at the end of the experiment. Retroorbital blood samples were taken, and liver samples were collected and kept at −80°C for RNA extraction and enzyme activity assays. To obtain serum samples, blood samples were subjected to centrifugation at 3000 rpm for 15 min, and then the serum was stored at −80°C until the biochemical assay.
Fasting blood glucose and serum insulin were estimated using the glucometer method (Accutrend alpha, Roche Diagnostic GMbH, Germany) and rat insulin ELISA kit (Wuhan Fine Biotech Co., Ltd., China), respectively. Systemic insulin resistance was estimated using the homeostasis model assessment index for insulin resistance (HOMA-IR) using the following equation:
Serum total cholesterol (TC), triglycerides (TGs), high density lipoprotein-cholesterol (HDL-C), low density lipoprotein-cholesterol (LDL-C), alanine aminotransferase (ALT), and aspartate aminotransferase (AST) were calorimetrically quantified using commercial kits from (Biodiagnostic, Giza, Egypt) according to the spectrophotometric methods described in the manufacturer’s protocol.
To determine oxidative stress and antioxidant markers in the liver tissue, malondialdehyde (MDA) (Ohkawa et al., 1979), reduced glutathione (GSH) (Owens and Belcher, 1965) levels as well as catalase (Fossati et al., 1980) and superoxide dismutase (SOD) (Nishikimi et al., 1972) activities were measured using commercial kits from (Biodiagnostic, Giza, Egypt) according to the manufacturer’s protocol.
Isolation of total RNA, including miRNAs, from liver tissue was carried out using the Qiagen miRNeasy Mini kit (Cat No. 217004; Qiagen, Hilden, Germany) according to the supplied manufacturer’s protocol. RNA concentration and purity were measured spectrophotometrically using the Nano Drop ND-1000 spectrophotometer (NanoDrop Tech., Inc., Wilmington, DE, United States). RNA was reverse transcribed to cDNA by the High-Capacity cDNA Reverse Transcription Kit, which uses the MultiScribe™ Reverse Transcriptase (Cat. No. 4368814, Applied Biosystems, Egypt). RT was carried out in a Mastercycler Gradient Thermocycler (Eppendorf, Hamburg, Germany) using the following parameter values: 25°C for 10 min, followed by 37°C for 120 min, and finally, 85°C for 5 min, then held at 4°C. While mirna-29a was reverse transcribed using the TaqMan™ MicroRNA Reverse Transcription kit and a miRNA-29a specific stem–loop primer (Applied Biosystem, assay ID 002112, Egypt). The Thermocycler was programmed according to the following conditions: 16°C for 30 min, 42°C for 30 min, and 85°C for 5 min, then held at 4°C to perform miRNA RT. The relative expression of PCK1, p85α PIK3R1, IL-1β and miRNA-29a was quantified using the TaqMan primers and probes with the following assay IDs (Applied Biosystems, assay IDs Rn01529014_m1 for PCK1, Rn01644964_m1 for Pik3r1, Rn00580432_m1 for IL-1β and 002112 for miRNA-29a). 18s rRNA was used as an endogenous control (Applied Biosystems, assay ID Hs99999901_s1). The PCR was performed on the StepOne™ Real-Time PCR System (Applied Biosystems) with the following conditions: 50°C for 2 min, then 95°C for 10 min, followed by 40 cycles of 95°C for 15 s and 60°C for 1 min. Relative gene expression was calculated by the 2−ΔΔCt method after normalization to 18s rRNA (Toraih et al., 2015, 2019; Abd Allah et al., 2021).
A stock solution of the extract was made by dissolving 50 mg of aq.-ethanolic extract (5:95) in 1 ml of a (2:1:1) solvent mixture of water, methanol, and acetonitrile (H2O: MeOH: ACN). To obtain complete solubility of the stock solution, the sample was vortexed and ultra-sonicated at 30 kHz for 10 min. 20 µl aliquot of the stock solution was again diluted with 1000 µl of the (2:1:1) H2O: MeOH: ACN, centrifuged at 10,000 rpm for 5 min before injection, and 10 µl (1 μg/ml) was used. For experiment confidence, the LC-MS analysis was also performed on blank and quality control samples/internal standard (IS). In both positive and negative modes, the sample was injected.
UPLC separation of phytochemicals was carried out on a reversed-phase Exion Xbridge C18 column (2.1 50 mm, 3.5 m) from (Waters Corporation, Milford, MA, United States), which was preceded by an in-line filter disks pre-column (0.5 µm × 3.0 mm, Phenomenex, Torrance, CA, United States). For each mode, the mobile phase contained two solvents: solvent (A) 5 mM ammonium formate in 1% methanol, pH adjusted to 3.0 by using formic acid, solvent (B) 5 mM ammonium formate in 1% methanol, adjusted to pH 8 using sodium hydroxide, and solvent (C) 100% acetonitrile. For the positive ion mode, solvents (A) and (C) were used, while for the negative ion mode, solvents (B) and (C) were used. The column temperature was maintained at 40°C, and the flow rate was set to 300 μl/min. The gradient elution was performed with the following parameters: 0–20 min, (90% (A) or (B), 10% (C); 21–25 min, (10% (A) or (B), 90% (C); 25.01–28 min, (90% (A) or (B), 10% (C)). (A) was used for the positive ion mode only, while solvent (B) was used for the negative ion mode only.
The mass spectrometry (MS) was carried out on a Triple TOF 5600+ system with a Duo-Spray source in the ESI mode (AB SCIEX, Concord, ON, Canada). In the positive mode, the sprayer capillary and declustering potential voltages were 4500 and 80 eV, respectively, and in the negative mode, -4500 and −80 V. The collision energies of 35 V (positive mode) and −35 V (negative mode) were used, together with CE spreading of 20 V and ion tolerance of 10 ppm. The TripleTOF5600+ was operated using an information-dependent acquisition (IDA) protocol. Analyst-TF 1.7.1 was used to collect batches of MS and MS/MS data. The IDA method was utilized to simultaneously collect full-scan MS and MS/MS information. The method used high-resolution survey spectra ranging from 50 to 1100 m/z, and the mass spectrometer was programmed to detect a 50-ms survey scan. Following that, the top 15 most intense ions were chosen for MS/MS fragmentation spectra acquisition after each scan (El Sayed et al., 2020; Mohammed et al., 2021).
For non-targeting, small molecule comprehensive analysis of the sample, MS-DIAL 3.70 open-source software was utilized. ReSpect positive (2737 records) or ReSpect negative (1573 records) databases were used as reference databases, depending on the acquisition mode. The search parameters were set as MS1 and MS2 mass tolerance: 0.01 and 0.05 Da for data collection, for peak detection; minimum peak height: 100 amplitude, mass slice width: 0.05 Da, smoothing level: two scans, minimum peak width: six scans, for identification MS1 and MS2 tolerance: 0.2Da/each, for alignment; retention time tolerance: 0.05 min and MS1 tolerance: 0.25 Da (El Sayed et al., 2020; Mohammed et al., 2021).
Compounds identified based on UPLC/MS-MS were further screened for oral bioavailability and drug likeness. Using accurate searching canonical SMILES, important pharmacology-related properties were obtained from the Swiss ADME tool (http://www.swissadme.ch/), including molecular weight, Log Po/w, hydrogen bond acceptor count, hydrogen bond donor count, and number of rotatable bonds. Based on the rule of five, these properties were applied to the drug likeness evaluation. The candidate bioactive chemicals were selected if they complied with two or more of the five drug-likeness filters (Lipinski, Veber, Ghose, Muegge, Egan) and had a bioavailability score of 0.55 or more, as proposed by the Swissadme tool, which allows for the computation of physicochemical properties and prediction of druglike nature, pharmacokinetic properties, and ADME parameters (Daina et al., 2017).
Six databases containing information about drug or chemical interactions with biological targets were used in this study to retrieve targets of MA active constituents. These databases include: Binding Database (https://www.bindingdb.org/bind/chemsearch/marvin/FMCT.jsp) retrieving targets matched only to “exact” compound and affinity filter of no more than 10,000 nM, Swiss Target Prediction (http://www.swisstargetprediction.ch/) with a score cutoff = 0.5, STITCH database (http://stitch.embl.de/) with a score cutoff 0.4, Therapeutic Target database (TTD, http://db.idrblab.net/ttd/), Comparative Toxicogenomics database (CTD, http://ctdbase.org/), and the first bioinformatics analysis tool for molecular mechanism of traditional Chinese medicine BATMAN-TCM (http://bionet.ncpsb.org.cn/batman-tcm/) with a target prediction score cutoff 20 (details of this work are presented in Supplementary Table S4).
To retrieve the known targets associated with T2DM, we used the key words “Type 2 Diabetes Mellitus” and “Insulin Resistance” to search in the following four databases: Online Mendelian Inheritance in Man (OMIM, https://www.omim.org/), DisGeNET (version 7.0) with a score cutoff of 0.2 (https://www.disgenet.org/), Therapeutic Target Database (TTD, http://db.idrblab.net/ttd/), and DRUGBANK (only targets for FDA approved drugs) (http://www.drugbank.ca). Targets were converted into HUGO Gene Nomenclature Committee (HGNC) approved gene symbols and then merged (details of this work are presented in Supplementary Table S4).
To get overlapping targets between T2DM disease targets and MA chemical constituents’ targets, we used the online tool “calculate and draw custom Venn diagrams” (https://bioinformatics.psb.ugent.be/cgi-bin/liste/Venn/calculate_venn.htpl) to create a Venn diagram and analyze the intersection between the two sets of targets. The intersection between MA targets and T2DM targets was subsequently utilized to build our compound-target network and protein-protein interaction network and was considered as MA candidate targets against T2DM. To get protein-protein interaction data, overlapping targets were further used in the STRING database (version 11.5, https://string-db.org/), and the screening parameters used were “Homo sapiens,” with a high interaction score of 0.7 as the minimum interaction score.
To decode the mechanism of action of MA against T2DM, we constructed the following three main networks: 1) The Compounds-targets (C-T) network, which links MA compounds and their overlapping targets with T2DM. 2) The Target-Target or Protein-Protein interaction (T-T) network of common targets for both MA and T2DM common targets. 3) Compound-Core key target-Pathway (C-T-P) network.
All the networks were constructed and visualized using Cytoscape (version 3.9.1), which is an open-source software for visualizing interactions between molecules, biomolecules, and biological pathways. In addition, many apps can work efficiently with cystoscope to analyze the network based on the equations of graph theory. Topological analysis was done using the network analyzer plug-in in Cytoscape. The fundamental units of a network are nodes and edges. Nodes represent drugs, proteins, or pathways, and edges represent interactions between them. Three topological properties, including degree centrality, closeness centrality, and betweenness centrality, were used to judge the importance of targets and compounds in the networks. The standard definition of degree is the number of direct neighbors of a node. The greater the number of a node’s direct links or edges, the greater its degree and influence in the network. A node’s betweenness centrality is defined as the number of shortest paths between all pairs of nodes that pass through the node. It represents the extent to which nodes stand between each other and is often used to find nodes that serve as a bridge from one part of a graph to another. Closeness centrality is considered a measure of how long it will take to spread information sequentially from one node to all other nodes. The higher the degree/closeness/betweenness centrality of a protein, the greater its importance in the protein-protein interaction network (Zhang et al., 2020; Que et al., 2021).
Enrichment of Gene ontology (GO) terms; biological process (BP), molecular function (MF), and cellular component (CC) to understand genes’ functions was performed using Enricher (https://maayanlab.cloud/Enrichr). To understand the molecular mechanisms and pathways of our targets, Kyoto Encyclopedia of Genes and Genome (KEGG) pathway enrichment analysis was conducted on all 364 candidate targets by the David tool (https://david.ncifcrf.gov/home.jsp).
The ligands’ SDF files were retrieved from PubChem and converted to PDB format using open Babel software. The protein data bank (https://www.rcsb.org) was utilized to retrieve 11 PDB file formats of the core target proteins predicted by network analysis. The protein names and PDB IDs are as follows: AKT1 (1unq), AKT2 (1o6l), HIF1A (1h2m), IL6 (1alu), MAPK1 (6rq4), MAPK3 (4qtb), PPARA (2p54), PPARG (5y2o), SIRT1 (4zzi), TNF (2az5), and RELA (2ram). Ligands and proteins were further prepared for docking and converted to PDPQT file format using Biovia Discovery Studio and Autodock Tools. The Autodock Vina software was used to perform the docking simulation process, and the ligands with the lowest binding energy scores were chosen to visualize their interactions with proteins in the Biovia Discovery Studio software (Oh et al., 2020; Ren et al., 2021).
Experimental data were presented as Mean ± SEM after analysis by the Statistical Package of Social Sciences (SPSS) program version 22, (Chicago, IL, United States). One-way analysis of variance (ANOVA) followed by a Tukey’s multiple comparison test was performed for comparisons among groups. For a specific value to be regarded a statistically significant, the p value must be less than 0.05. Graphs were created by GraphPad prism 9. Adjusting the size of the images and resolution, adding the panels’ letters, combining the images, and putting numbers on peaks were done using Adobe Photoshop CC (2019, V20.07.28362).
As shown in Table 1, rats on HFD + STZ showed a significant increase in blood glucose level compared to normal non-diabetic control group. The curative benefits of the four plant extracts were investigated in HFD + STZ-induced diabetic rats, and only MA hydroethanolic extract treatment at two different doses demonstrated a promising antihyperglycemic effect, as evidenced by a significant drop in fasting blood glucose levels. After 4 weeks of therapy, MA ethanolic extracts and pioglitazone treated rats had considerably lower glucose levels than the diabetic control. In contrast, the hexane, dichloromethane, and ethyl acetate extracts did not show significant improvement in blood glucose levels. This result strongly suggested that the antidiabetic activity of MA was concentrated in the ethanolic extract, which directed biochemical and phytochemical analysis to focus on it. The antihyperglycemic effect of pioglitazone did not show a statistical difference from MA ethanolic extracts. In addition, diabetic rats showed a significant reduction in fasting serum insulin, secretory capacity of the pancreas (HOMA-B index), and the index of insulin sensitivity QUICKI, while the index of insulin resistance HOMA-IR, triglyceride and glucose (TyG) index, and the markers of liver injury ALT and AST increased significantly in comparison to the normal control rats, as shown in Table 2 and Figure 2A, respectively. Moreover, as depicted in Figure 2B, serum lipids, including triglycerides, cholesterol, and LDL-C increased significantly, whereas HDL-C decreased in diabetic control rats compared to normal control rats. Treatment with either MA ethanolic extract or pioglitazone was successful in ameliorating insulin resistance, hyperlipidemia, and fatty liver without having a significant effect on fasting insulin levels or pancreatic secretory capacity. Furthermore, it increased liver protection from injury associated with T2DM, as evidenced by a significant decrease in ALT and AST.
FIGURE 2. MA ethanolic extracts ameliorated the liver damage and dyslipidemia (A) liver enzymes: ALT, alanine aminotransferase; AST, aspartate aminotransferase, #p < 0.05 versus normal control, *p < 0.05 versus diabetic control. (B) Serum lipids: TG, triglycerides; TC, total cholesterol; LDL-C, low density lipoprotein cholesterol; HDL-C, high density lipoprotein cholesterol. #p < 0.05 versus normal control, *p < 0.05 versus diabetic control. Data are expressed as Mean ± SEM, n = 7. Adjusting the size of the images and resolution, adding the panels’ letters, and combining the images was carried out using Photoshop.
The cellular antioxidant defense mechanism was significantly diminished in diabetic control animals. In liver homogenate, the lipid peroxidation and oxidative stress parameter MDA was dramatically enhanced, while antioxidant indicators such as GSH, SOD, and CAT activity were significantly reduced. Administration of either Pioglitazone or MA extract significantly increased the activity of antioxidant enzymes CAT and SOD, along with GSH levels, while causing a significant decrement in MDA levels, as shown in Table 3.
TABLE 3. MA ethanolic extracts increase the antioxidant enzymes’ activity and decrease oxidative stress parameters.
To explore the molecular mechanism of MA in the treatment of T2DM, we selected PIK3R1, PCK1, IL1B, and MIR 29A to investigate their expression level changes by qRT-PCR. As exhibited in Figure 3, the expression level of PIK3R1 was significantly downregulated, whereas the expression of PCK1, IL1B, and MIR 29A were significantly upregulated in diabetic control rats compared to normal rats. Administration of either MA extract or pioglitazone for 4 weeks was able to significantly increase PIK3R1 expression and decrease PCK1, IL1B, and MIR 29a in the treated groups compared to diabetic control rats.
FIGURE 3. Effect of MA ethanolic extract on gene expression (A) MA ethanoic extract decreased the expression of the inflammatory cytokine IL1B (B) MA ethanoic extract increased the expression of PIK3R1. MA ethanolic extract decreased the expression of (C) PCK1 and (D) MIR -29a. Data are expressed as Mean ± SEM, n = 7, #p < 0.05 versus normal control, *p < 0.05 versus diabetic control. Adjusting the size of the images and resolution, adding the panels’ letters, and combining the images was carried out using Photoshop.
A total of 62 compounds were identified by utilizing UPLC/MS-MS analysis. The total ion chromatogram (TIC) is depicted in Supplementary Data Sheet S2, and the names of compounds, retention time, molecular ion and characteristic fragment masses, molecular formula, and bioavailability score are shown in Table 4. The compounds included many flavonoids and their glycosides, coumarin derivatives, stilbene derivatives, and phenolic acids. 34 compounds complied well with the drug likeness and bioavailability criteria. 28 compounds did not show good pharmacokinetic properties by violating either the rule of five or the bioavailability score or both. We ultimately retained 13 compounds out of the 28 predicted to have bad pharmacokinetic behavior because they showed important biological activity in the published literature. In addition, 15 compounds predicted to have bad pharmacokinetic behavior and one without biological targets were excluded. The final list of potential bioactive compounds in MA included 46 compounds (See Supplementary Table S1 for details on pharmacokinetics).
TABLE 4. Characterization of the chemical constituents in MA ethanolic extract using LC-ESI-MS/MS analysis.
Data mining of six databases led to the collection of 6931 targets (in Supplementary Table S2), which corresponded to 46 active compounds of MA, while the total targets related to T2DM was 534 targets from four databases (in Supplementary Table S3). As shown in Figure 4A, after overlapping the herb targets with the disease targets using a Venn diagram, 364 common targets were obtained. (MA targets associated with T2DM are presented in Supplementary Table S4).
FIGURE 4. Venn diagram and compounds-targets interaction network. (A) Venn diagram Shows the intersection between MA targets and T2DM targets (B) network shows the interaction between MA phytochemicals and T2DM targets. Yellow nodes represent compounds with degrees more than the network’s 2-fold median, while orange represent compounds with degrees are less than that. Blue nodes represent targets with more than the network’s 2-fold median, while green nodes represent targets whose degrees are less than that. Adjusting the size of the images and resolution, adding the panels’ letters, and combining the images was carried out using Photoshop.
The overlapped 364 targets were considered the potential candidate targets of MA against type 2 diabetes mellitus. 46 compounds of MA were connected to their corresponding 364 targets in a large compound-target network using cystoscope software, as shown in Figure 4B. The mathematical analysis of network topology revealed interesting information about the potential mechanisms of action. 87 therapeutic targets showed a degree ≥7 (mor than the 2-fold median degree of the network). These targets are enriched in multiple pathways related to type 2 diabetes mellitus, including adipocytokine, AMPK, TNF, HIF-1, PI3K-AKT, NOD and TOLL-like receptors, mTOR, glucagon, and insulin signaling pathways. Some of the important targets (with high degree) of MA compounds are shared with the FDA-approved medications, such as thiazolidinedione targets PPARG and PPARA, biguanides targets (AMPK) PRKAA1 and PRKAA2, alpha glucosidase inhibitors targets AMY2A and GAA, and DPP4 inhibitors targets. 37 ingredients of MA interact with ≥7 targets, suggesting a complex mechanism of MA in ameliorating type 2 diabetes mellitus and involving multi-compounds/multi-targets/multi-pathways modulation. The top 21 compounds showed a high degree ≥40 and included quercetin, apigenin, luteolin, resveratrol, epicatechin, naringenin, myricetin, etc. For a detailed look at compounds and target degrees, see Supplementary Table S5.
To gain more insight about the importance of each target and its contribution to the pharmacological mechanism of action, a protein-protein interaction network was created by importing the 364 common targets in STRING db. As shown in Figure 5A, the PPI network consisted of 363 nodes and 2856 edges (one target; CASP 12 was not identified in the String db. and was excluded). We screened the most important targets from this network in three discrete steps based on the topological properties (degree centrality, closeness centrality, and betweenness centrality) of each node. Firstly, we picked out 123 hub targets by taking nodes whose centrality values are greater than the median, as depicted in Figure 5B. Secondly, we selected 51 major hub targets whose centrality values are greater than the median value of the hubs, as shown in Figure 5C. Finally, we excluded 11 targets from the major hubs, which were affected by less than seven compounds, to end up with 40 key major hubs or core key targets for the MA mechanism in the treatment of type 2 diabetes mellitus, as shown in Figure 6. (For more details, see Supplementary Table S6).
FIGURE 5. Protein-protein interaction networks. (A) PPI network of 363 targets of MA. Yellow nodes represent the hubs that will form the following network (B) represents PPI of 123 hubs whose centrality values are greater than the median. The yellow nodes represent the major hubs that will form the following network (C) represents PPI of the major hubs or core key targets whose centrality values are greater than the median of the hub nodes. The yellow nodes represent the core key targets that are affected by more than six compounds and will form the network of the following figure. The blue nodes in (C) represent targets that affected by less than seven compounds. Adjusting the size of the images and resolution, adding the panels’ letters, and combining the images was carried out using Photoshop.
FIGURE 6. The PPI of 40 major hubs or core key targets of MA in T2DM treatment is ordered by color and size based on degree, from big and green to small and yellow, after the exclusion of the core targets affected by fewer than seven compounds. Adjusting the size of the images and resolution was carried out using Photoshop.
Enrichment analysis based on GO and KEGG databases was performed to investigate the biological functions and pathways of MA putative target for T2DM treatment. GO analysis results suggested that MA targets are enriched in multiple biological processes linked directly to metabolism and T2DM, including glucose homeostasis, carbohydrate homeostasis, regulation of metabolic processes, cellular response to insulin stimulus, cytokine stimulus, reactive oxygen species, and oxidative stress. Figure 7A shows the top 25 enriched GO (BP) terms, and the detailed results of GO enrichment analysis, including GO (BP), GO (MF), and GO (CC), are found in Supplementary Table S7.
FIGURE 7. GO and KEGG pathway enrichment analyses (A) represents the top 25 enriched GO biological processes terms. (B) Represents the top 25 enriched KEGG pathways. Adjusting the size of the images and resolution, adding the panels’ letters, and combining the images was carried out using Photoshop.
To further uncover the potential pathways involved in the antidiabetic effects of MA, KEGG pathway enrichment analysis was carried out. A total of 25 top enriched pathways are identified and presented in Figure 7B. More than half of the top 25 enriched pathways are well established T2DM-linked pathways. The enriched genes were linked to a variety of signaling and metabolic pathways, including energy sensing and metabolic pathways like the AMPK signaling pathway, insulin and glucagon signaling pathways, the FoXO signaling pathway, which promotes glucose homeostasis and combats oxidative stress, the HIF-1 signaling pathway, which mediates metabolic adaptation to oxygen, and immunoinflammatory pathways like the Adipocytokine and TNF signaling pathways, the PI3k-Akt signaling pathway, and pathways related to apoptosis. (For details on all KEGG enriched pathways see Supplementary Table S8).
To get a deeper look at the enrichment analysis of all MA targets, we managed to see the relationship between the 40 core targets (major hubs) extracted from the PPI network and the key pathways related to T2DM from the enrichment analysis of all MA targets and we asked how many core targets are found in each pathway. To answer this question, we constructed a compound-core target-pathway (C-T-P) network linking MA active compounds, core targets, and pathways, as depicted in Figure 8. The analysis of the network showed that it contained 101 nodes (43 compounds, 40 core targets, and 15 pathways) and 937 edges. The importance of each pathway in this network stemmed from the number of core targets it contained, judged by degree (the number of edges between pathway node and core targets). Table 5 shows these pathways and the percentage of core targets they contain, ordered based on degree. Together, the results of computational network pharmacology and experimental work suggested that MA ameliorated T2DM through modulation of insulin resistance by multiple mechanisms, including modulation of oxidative stress pathways, inflammatory pathways, energy sensing/endocrine/metabolic pathways.
FIGURE 8. Represents compounds-core key targets-pathways network. The yellow triangles represent compounds, the blue circles represent targets, the pink Hexagons represent pathways, and the green squares represent collections of functionally related pathways. The larger the size of the node, the greater its degree. Adjusting the size of the images and resolution, adding the panels’ letters, and combining the images was carried out using Photoshop.
Checking the validity of network predictions against other channels of evidence, such as experimental data and computational chemistry simulation is a critical step in elucidating the mechanism of action. Molecular docking simulation is one of the most important and commonly used tools for exploring the biological activity of drugs on proteins, with affinity or binding energy being the most important parameter. The molecule with the highest biological activity has the lowest value of this parameter. The docking score values determine the binding potential or affinity of drugs to their targets, as well as the stability of conformations. A docking score value of less than −7 indicates strong binding activity, values between −5 and −7 demonstrate good binding activity, and values in the range of −4.25 to −5 signify a certain binding activity (Dong et al., 2021). Out of 40 core targets, we selected 11 proteins involved in different mechanisms to perform docking with the top 21 MA compounds (with ≥40) in terms of degree. The results are presented in Figure 9 as a heat map, and they indicate that the majority of targets predicted by network analysis have strong binding activities with the majority of tested compounds. Strong binding activity was prevalent with MAPK1, MAPK3, SIRT1, PPARA, PPARG, AKT2, TNF, and HIF1A, whereas AKT1, IL6, and RELA had good binding activity with most of the tested compounds. Generally, phenolic acids and coumarin derivatives, including p-coumaric acid, ferulic acid, protocatechuic acid, and esculetin, showed lower affinity than flavonoids. The exceptions were rosmarinic acid and chlorogenic acid, which showed a binding score pattern comparable to flavonoids. Figure 10 shows the interaction of apigenin with the active sites of MAPK1, TNF, and PPARG.
FIGURE 9. Heatmap represents the docking results and affinity of 21 compounds with 11 proteins from the core targets. The more bluish the rectangle is, the stronger the affinity. Adjusting the size of the images and resolution was carried out using Photoshop.
FIGURE 10. Molecular docking of apigenin with three proteins. The left images and the right images represent the 3D and the 2D structures, respectively (A) Docking of apigenin with MAPK1. (B) Docking of apigenin with PPARG. (C) Docking of apigenin with TNF. Adjusting the size of the images and resolution, adding the panels’ letters, and combining the images was carried out using Photoshop.
In humans, the progression of type 2 diabetes could be divided into two distinct stages. The first stage is the transition from a healthy metabolism to a state of declining insulin action on peripheral tissues (insulin resistance) and prediabetes. Nutritional overload and obesity are thought to be associated with the development of this state by increasing the levels of circulating triglycerides and free fatty acids that deposit in the liver, muscle, and adipose tissue, resulting in insulin resistance. Despite all the abnormalities that occur in stage 1, the pancreatic beta cells are still able to compensate for insulin resistance by producing more insulin (hyperinsulinemia). To develop frank hyperglycemia and type 2 diabetes, there must be a second stage of transition from hyperfunctional beta cells that compensate for insulin resistance to beta cell failure/death that is characterized by normal or hypoinsulinemia and the inability to compensate for insulin resistance. In animal models, a high-fat diet is typically used to cause the first stage of insulin resistance and prediabetes, while chemical inducers such as STZ are usually used to cause the second stage of pancreatic B-cell death and hypoinsulinemia. Several studies have shown that a combination of HFD and STZ for induction of T2DM in rats is a better model for mimicking the natural history of the disease (Srinivasan et al., 2005; Islam and Choi, 2007; Skovsø, 2014; Barrière et al., 2018). T2DM is a heterogeneous disorder with high morbidity and a plethora of associated abnormalities. Therefore, several proteins or pathways are linked to the development of T2DM. Antidiabetic botanical extracts contain many compounds that could induce pharmacological actions through multiple targets and signaling pathways and may help in T2DM therapy (Li et al., 2017).
Matricaria aurea (MA, Golden Chamomile) is a widely used herb in Middle Eastern communities for a variety of ailments, including diabetes mellitus, despite a lack of scientific evidence to support such use (Yaniv et al., 1987; Al-Mustafa and Al-Thuniba, 2008). Furthermore, a few previous studies, which were mainly in vitro, reported the antioxidant (Mohammad Al-Ismail and Aburjai, 2004), anti-inflammatory (Khodadadi et al., 2011), anti-ulcerative colitis (in vivo) (Minaiyan et al., 2011), analgesic (Qnais, 2011), antibacterial, and anticancer (Kheder et al., 2014; Ahmad et al., 2020, 2021) biological activities of Matricaria aurea. As far as we know, this is the first scientific study to investigate the anti-diabetic activity of MA extracts in an established type 2 diabetic rat model using HFD + STZ. The first key finding of our study is that only the polar ethanolic extract was able to reduce fasting blood glucose in diabetic rats, whereas other less polar extracts did not show any promising activity. This finding is in harmony with previous research experience that polar hydroalcoholic extraction of chamomile’s polyphenolics and flavonoids is more effective than non-polar solvents (Molnar et al., 2017; Pereira et al., 2018), and it has directed the entire research process to investigate the biological and chemical roles of MA ethanolic extract in T2DM treatment. The second key finding of our study is that MA ethanolic extract was able to significantly reduce the insulin resistance index (HOMA-IR) and the triglyceride and glucose (TyG) index. In addition, our results showed that MA ethanolic extract was not able to significantly increase the basal insulin secretion and the secretory capacity of the pancreas, as evidenced by the low level of fasting insulin and the HOMA-B index. These results indicate that MA ethanolic extract was successful in reducing insulin resistance without having a significant effect on insulin levels or the secretory capacity of the pancreas. It also suggests that MA could improve T2DM by increasing cell sensitivity to existing insulin without insulin secretagogue activity. In accordance with our findings, a previous interesting study on Roman chamomile showed that it lowers the blood glucose levels in both normal and STZ-diabetic rats without increasing the basal insulin secretion (Eddouks et al., 2005). In addition, another study examined the antihyperglycemic effect of German chamomile using STZ-diabetic rats and found that it reduces hyperglycemia without affecting insulin levels (Al-Musa and AL-Hashem, 2014). While Weidner et al. showed that German chamomile was able to reduce hyperglycemia, hyperinsulinemia, and insulin resistance in a diabetic obese model induced by long-term HFD feeding (Weidner et al., 2013). Zemestani et al. showed that chamomile can reduce insulin resistance and hyperinsulinemia in type 2 diabetic patients (Zemestani et al., 2016). Therefore, it seems that the findings of our investigation and the four previous studies support the notion that different chamomile varieties ameliorate type 2 diabetes without increasing insulin secretion or the secretory capacity of the pancreas. MA ethanolic extract was also able to significantly alleviate dyslipidemia and liver damage indicators ALT and AST, indicating an improvement in lipid metabolism and protection of the liver. The TyG index is a sensitive marker for the status of hepatic steatosis, insulin resistance, and fatty liver. Groups that received MA showed a significant improvement in their TyG index, which indicates an improvement in hepatic insulin resistance and lipid metabolism and a reduction in the lipid content of the liver (Zhang et al., 2017; Lee et al., 2019). Previous studies in HFD animal models and human clinical trials showed the ability of German chamomile to improve dyslipidemia in mice and human blood (Rafraf et al., 2015; Bayliak et al., 2021). Furthermore, it has been shown that chamomile can increase the hepatic expression of genes involved in the beta oxidation of fatty acids, including carnitine palmitoyltransferase 2, Acyl-Coenzyme A Oxidase 2, HMG-CoA synthase 2, and enoyl CoA hydratase genes. Liver damage markers in type 2 diabetes models are typically associated with steatohepatitis in liver tissue caused by a high-fat diet. The observed liver protection in MA-treated groups has also been demonstrated in several illness models, including diabetes treated with different chamomile varieties. Jabri et al. showed that chamomile can protect the liver from the lipotoxicity and oxidative stress caused by HFD in mice (Jabri et al., 2017). It has been shown that chamomile can ameliorate steatohepatitis induced by HFD, reduce the liver content of triglycerides, and prevent the rise of ALT (Mannaa et al., 2015; Bayliak et al., 2021).
Establishing the antidiabetic activity of MA ethanolic extract prompted us to search for the molecular players that might contribute to the mechanism of MA’s antidiabetic effect. Firstly, we hypothesized that the chemical components of MA ethanolic extract could influence critical T2DM targets. Using UPLC QTOF MS/MS, we identified a total of 62 chemical compounds in MA ethanolic extract. Many of the identified compounds have previously been reported to be the major flavonoids and phenolics in chamomile, including Apigenin and its glycosides, Luteolin and its glycosides, Quercetin and its glycosides, p-coumaric acid, chlorogenic acid, and ferulic acid (Aboul-Ela et al., 2012; Khan et al., 2019; Yousefbeyk et al., 2022). Then, we filtered these compounds according to their ADME properties and published pharmacological activities to obtain a final list of 46 active compounds. In this study, it was found that 46 compounds from MA could interact with 364 candidate targets related to T2DM. The analysis of the protein-protein interaction network identified 123 targets as hub proteins, including AKT1, AKT2, PIK3R1, MAPK1, RELA, PCK1, SOD1, and SOD2, etc. Further filtering to obtain the network’s crucial key targets revealed a total of 40 crucial core targets through which MA may exert its effect, including AKT1, AKT2, IRS1, IRS2, PIK3R1, TNF, ILB, IL6, MAPK1, MAPK3, HIF-1, and FOXO. From the rigorous interpretation of these results, some important themes started to emerge. 1) Firstly, a single compound can regulate multiple targets on its own, whereas a single target can be regulated by many compounds; for instance, the inflammatory cytokine IL1B was modulated by more than half of all identified compounds. This observation highlights the essence of network polypharmacology and shows that simultaneously targeting numerous proteins associated with the disease may be more beneficial than targeting a single protein selectively. Moreover, the targeting of a single protein by numerous bioactive compounds suggested that MA bioactive compounds may have a synergistic effect in treating T2DM. 2) The major constituents of MA, including apigenin, luteolin, quercetin, chlorogenic acid, p-coumaric acid, and ferulic acid, were found to target about 80% of the total targets in the network model. We think that MA’s antidiabetic activity is very likely to be mediated by the major constituents’ actions on these targets. The minor constituents might contribute to the biological activity by synergistic or additive interactions with the same targets. Experimental studies of synergy are needed to validate or rule out this assumption. 3) Analysis of the relationships between the crucial core targets predicted by network analysis and the enriched pathways revealed that each of the top enriched pathways contains a large number of the core targets; for example, more than one-third of the core targets are found in the insulin signaling pathway and approximately half of the core targets are found in the Foxo signaling pathway. To emphasize this point, we think that the aggregation of a large proportion of the core targets predicted by PPI network analysis in the top pathways reflects the powerful prediction capability and reliability of such networks for inferring action mechanisms. 4) The fourth pattern revealed by network analysis was the ability of one target to be involved in more than one pathway, implying crosstalk and a close relationship between these pathways. For example, PIK3R1 is involved in nine of the most important pathways related to T2DM, such as the insulin signaling pathway, the FoxO signaling pathway, and so on. This observation captured network biology’s realization of the modularity nature of complex diseases such as diabetes. The importance of targets in this model stems from their importance in the disease module, whereas the importance of drugs stems from their ability to modulate essential hubs in the disease module. Moreover, GO and KEGG pathway enrichment analyses of the putative targets identified multiple pathways, mainly inflammatory pathways, oxidative stress pathways, and endocrine/energy-sensing/metabolic pathways.
Inflammation is widely recognized as a crucial factor in the pathogenesis of T2DM and insulin resistance. Local inflammation in the peripheral tissues plays a significant role in the vicious cycle of diabetes abnormalities (Cai et al., 2005; Catrysse and van Loo, 2017). Reducing inflammation by blocking or decreasing the effect of the inflammatory mediator IL-1B has drawn the attention of many researchers interested in discovering newer antidiabetics. Treatment with IL1 receptor antagonists has been shown to decrease hyperglycemia, enhance B cell function and increase insulin sensitivity in peripheral tissues, notably the liver (Ehses et al., 2009). Our network analysis predicted that the inflammatory cytokines IL1B, IL6, and TNF are crucial targets for MA in treating T2DM. This prediction is consistent with the mRNA expression of IL1B that was downregulated in the MA treated groups compared with the diabetic non-treated animals. In agreement with our experimental results and network predictions, a previous study showed that chamomile was able to mitigate inflammation and reduce the expression of inflammatory genes in the liver of insulin-resistant obese mice (Weidner et al., 2013). In addition, previous studies showed that chamomile reduces the inflammatory markers in the blood of animal models and human type 2 diabetes patients (Shelbaya, 2017; Nargesi et al., 2018; Zemestani et al., 2018). Furthermore, several studies showed the effectiveness of chamomile in mitigating inflammation in various inflammatory disease models, including macrophages, brain, skin, colon, and adipocytes. (Srivastava et al., 2009; Bhaskaran et al., 2010; Curra et al., 2013; Menghini et al., 2016; Ortiz et al., 2017; Russo et al., 2021; Wang et al., 2021; Jabri et al., 2022). A deeper explanation for the observed anti-inflammatory effect of MA emerged from the appearance of RELA (NFKB 65), NFKB1, MAPK1, and MAPK3 as core targets in the network. Obesity, nutritional overload, and oxidative stress associated with T2DM have been shown to activate these signaling proteins, leading to the development of inflammation and insulin resistance in peripheral tissues (Catrysse and van Loo, 2017). Inhibition of RELA, NFKB1, MAPK1, and MAPK3 may mitigate inflammation and reduce inflammatory cytokines expression. In accord with our model predictions, previous studies showed that major chamomile flavonoids, including apigenin, luteolin, and quercetin, have powerful anti-inflammatory activity through inhibiting the activation of RELA (NFKB65) and MAPK proteins and reducing their expression (Malik et al., 2017; Choy et al., 2019). Furthermore, ferulic acid and chlorogenic acid have been shown to reduce proinflammatory mediators by inhibiting the MAPK and NFKB pathways (Bao et al., 2018; Ghosh et al., 2018; Chowdhury et al., 2019; Yan et al., 2020). In addition, the anti-inflammatory activity and reduction of IL1B and TNF expression are known with p-coumaric acid treatment (Alam et al., 2016; Mozaffari Godarzi et al., 2020). Molecular docking simulation gave a booster support for this mechanism, showing a strong binding energy with MAPK1, MAPK3 (less than −8.5 with most compounds), and TNF, and a good binding energy with IL6 and RELA. Furthermore, the study of Bhaskaran et al. on the effect of chamomile on inflammatory mechanisms in macrophages lent great support to our predicted mechanism through RELA/NFKB65. This study demonstrated that chamomile was able to reduce LPS-induced NO production and block IL1B, TNF, and IL6-induced NO formation in macrophages through inhibition of RELA/NFKB65 activation and iNOS gene expression (Bhaskaran et al., 2010). MMP9 is another inflammatory protein predicted to be a major hub modulated by MA in the PPI network. This prediction is consistent with an in vitro study that showed the anti-inflammatory activity of MA hydroethanolic extract via inhibiting MMPs proteins (Khodadi et al., 2011). Bulgari and others showed that the anti-inflammatory effect of chamomile at the gastric level was mediated through inhibition of metalloproteinase 9 (MMP9) and the NFKB pathway (Bulgari et al., 2012). According to the results of GO and KEGG pathway enrichment analyses, the pathways related to inflammation, including TNF, Adipocytokine, Toll-like receptor, and MAPK signaling pathways, are major pathways through which MA compounds can modulate the inflammatory processes. The combined experimental and computational evidence suggests that an anti-inflammatory mechanism is involved in ameliorating T2DM by MA ethanolic extract.
Oxidative stress is one of the major factors in the pathogenesis of T2DM. Flavonoids and polyphenols’ actions are frequently attributed to their antioxidative capacity. The outcomes of our study showed a significant improvement in the antioxidant enzymes CAT and SOD activity, as well as GSH content in the liver. In agreement with our results, many studies have shown the antioxidative stress potential of different Matricaria species in either in vitro or in vivo models. It has been shown that chamomile diminishes the lipotoxicity and oxidative stress markers in the liver of HFD-fed rats (Mohammad Al-Ismail and Aburjai, 2004; Cemek et al., 2008; Jabri et al., 2017; Al-Dabbagh et al., 2019). Moreover, chamomile tea was successful in reducing oxidative stress markers in the blood of diabetic human subjects (Zemestani et al., 2016). Network analysis provided a deeper underlying mechanism for increasing the antioxidant potential through the induction of Nrf2 (gene name: NFE2L2). Nrf2 is a transcription factor that induces antioxidant genes via interaction with the antioxidant response element (ARE) in DNA, and hence plays a central role in the defense system of the cell in combating oxidative stress. Nrf2 regulates the transcription of antioxidative stress enzymes such as CAT, SOD, HMOX1, and glutathione peroxidase (David et al., 2017). More than 20 of the identified compounds were shown to modulate Nrf2. Another probable mechanism involves modulation of FoxO signaling and SIRT1. In accordance with our results, previous studies showed the ability of various flavonoids, including Apigenin, Luteolin, quercetin, naringenin, and myricetin, to act as modulators of Nrf2 and FoxO (Pallauf et al., 2017). Furthermore, hydroxycinnamic acid derivatives such as chlorogenic acid and ferulic acid have been shown to protect against hyperglycemia-induced oxidative stress via activation of Nrf2 (Song et al., 2016; Bao et al., 2018). On the other hand, Baicalin has been demonstrated to upregulate SOD and CAT through modulation of FoxO phosphorylation (Kim et al., 2012). In the case of Formononetin, it has been shown to indirectly upregulate Nrf2 via SIRT1 (Zhuang et al., 2021). In accord with our model predictions, a couple of studies have shown that chamomile can protect macrophages from oxidative stress through induction of Nrf2 accumulation, leading to an increase in antioxidant enzymes levels (Bhaskaran et al., 2012, 2013). The correlation between the experimental antioxidative potential of MA extract and network prediction of important proteins that regulate oxidative stress refers to an antioxidant mechanism involved in the amelioration of T2DM.
T2DM is associated with insulin resistance, defective insulin signaling, and unrestrained gluconeogenesis. PCK1 is the rate-limiting enzyme in gluconeogenesis, which is transcriptionally controlled by insulin. In the diabetic state, the normal insulin signal that goes through INSR, IRS1, IRS2, PI3K, AKT, and Foxo to inhibit PCK1 transcription and reduce gluconeogenesis is aborted (Peng et al., 2020; Ge et al., 2021). Our study showed that the insulin signaling pathway was modulated by MA administration. Our network analysis indicated that the majority of this pathway’s components are either hubs, such as INSR and PCK1, or core targets, such as IRS1, IRS2, PIK3R1, AKT1, AKT2, and Foxo. Treatment with MA led to significant upregulation of PIK3R1 mRNA expression and significant downregulation of PCK1 expression compared to diabetic control, which signifies improvement in insulin signaling and inhibition of gluconeogenesis. In agreement with our results, a previous mechanistic study found that popular chamomile flavonoids Apigenin and luteolin were able to inhibit gluconeogenesis and PCK1 expression via modulation of FOXO1, AKT, and Nrf2 (Bumke-Vogt et al., 2014), while Liu et al. demonstrated that Daidzein-8-C-glucoside (Puerarin) could upregulate PI3K, AKT, pAKT and pfoxo1, leading to downregulation of PCK1 and inhibition of gluconeogenesis (Liu et al., 2021). Ferulic acid has been shown to attenuate glucose production by inhibiting PCK1, G6Pase, and glycogen phosphorylase and increasing the activity of glucokinase and glycogen synthase, while chlorogenic acid inhibits glucose 6-phosphatase and gluconeogenesis (Alam et al., 2016). The effect of chlorogenic acid was mediated through modulation of IRS1, PI3K, AKT, GSK3β, and FOXO1 signaling proteins (Gao et al., 2018; Yan et al., 2020). Other previous studies have shown that luteolin, quercetin, and apigenin could improve insulin signaling via modulation of PI3K (Nepali et al., 2015; Kalivarathan et al., 2020; Eraky et al., 2022). The second important mechanism predicted by network analysis was the activation of the AMPK signaling pathway, which contributes to explaining the observed modulation of glucose and lipid metabolism. Insulin-independent activation of AMPK suppresses the transcription factors Foxo1 and CRTC2, which are responsible for PCK1 transcription and gluconeogenesis. Furthermore, AMPK signaling inhibits gluconeogenesis by decreasing PPAR coactivator 1α (gene name: PPARGC1A) expression and increasing phosphorylation of glycogen synthase kinase 3 (GSK-3) (Joshi et al., 2019). Moreover, AMPK increases fatty acid oxidation in the liver and decreases de novo fatty acid synthesis, cholesterol synthesis, and lipogenesis by inhibiting sterol regulatory element binding protein 1c (SREBF1), HMG CoA reductase (HMGCR), and Acetyl-coA carboxylase (ACACA). In agreement with our proposal, previous studies have shown that MA ingredients, including Apigenin, luteolin, quercetin, ferulic acid, chlorogenic acid, isoorientin, naringenin, and resveratrol, ameliorate hyperglycemia and hyperlipidemia through activation of the AMPK signaling pathway and SIRT1 (Ong et al., 2012; Jin et al., 2015; Tsai et al., 2018; Joshi et al., 2019; DiNicolantonio et al., 2022). Our network analysis also revealed an increase in adiponectin and leptin production, which may activate AMPK signaling. In agreement with our predictions, previous studies have shown that apigenin, quercetin, and chlorogenic acid increase the adiponectin and leptin levels (Nisha et al., 2014; Jin et al., 2015; Zhang et al., 2018). Our study added a new layer of complexity to the process of improving the insulin signaling pathway through modulation of epigenetic mechanisms. Mir29A has been reported to be a critical regulatory hub in T2DM and its upregulation inhibits the insulin signal by targeting PIK3R1 and IRS1, resulting in uncontrolled gluconeogenesis and PCK1 upregulation (Pandey et al., 2011; Kurtz et al., 2014; Yang et al., 2014; Dooley et al., 2016). The q-RTPCR expression analysis revealed that both pioglitazone and MA extracts were effective in normalizing MIR29A expression in the liver, suggesting another epigenetic modulation of insulin resistance by MA. A recent study identified morin, a bioflavonoid closely related to MA flavonoids, as an inhibitor of MIR29A upregulation in human HepG2 cells (Razavi et al., 2019). The experimental and computational data suggest that MA ethanolic extract ameliorates T2DM by modulating multiple metabolic pathways, including insulin, FoxO, AMPK, and PI3K-AKT signaling pathways.
Network pharmacology analysis showed that some popular metabolic targets for the FDA-approved antidiabetic drugs, such as PPARG and PPARA, the targets of pioglitazone, might play a great role in MA activity against insulin resistance and dyslipidemia associated with T2DM. In the cases of PPARG and PPARA, the molecular docking scores referred to a strong binding with the majority of tested compounds (less than −7). In perfect agreement with our model’s predictions, a mechanistic study showed that chamomile ameliorates insulin resistance through transcriptional stimulation of PPARG and PPARA in the adipose tissue and liver, respectively (Weidner et al., 2013). In addition, previous research has shown that flavonoids can modulate PPARG and PPARA activity, which is consistent with our prediction. According to Feng et al., apigenin improves insulin resistance in muscle and liver and attenuates metabolic inflammation through PPARG activation (Feng et al., 2016), while Beekman et al. indicated that quercetin and kaempferol increase PPARG receptor mRNA expression. Other studies using apigenin, luteolin, chlorogenic acid, naringenin, formononetin, myricetin, diosmetin, and baicalin found a similar activation of PPARG-mediated gene expression (Beekmann et al., 2015; Sanchez et al., 2017; Peng et al., 2018). Moreover, previous studies showed that apigenin, quercetin, chlorogenic acid, rosmarinic acid, naringenin, Hesperitin, daidzein, formononetin, and umbelliferone can activate and/or increase the expression of PPARA (Shi et al., 2015; Alam et al., 2016; Rigano et al., 2017; Sanchez et al., 2017; Wang et al., 2019; Doi et al., 2022). Network analysis showed that alpha glucosidase and alpha amylase enzymes are potential targets for a large number of MA compounds. Prior investigation showed the ability of apigenin, quercetin, luteolin, chlorogenic acid, ferulic acid, and protocatechuic acid to inhibit alpha glucosidase and alpha amylase in in vitro studies (Malunga et al., 2018; Takahama and Hirota, 2018; Şöhretoğlu and Sari, 2020; Aleixandre et al., 2022). In perfect agreement with our predictions, previous investigations showed that chamomile can reduce carbohydrate digestion and absorption by inhibiting alpha glucosidase and alpha amylase enzymes (Kato et al., 2008; Villa-Rodriguez et al., 2018).
It is very interesting to find that natural compounds can modulate the targets of FDA-approved drugs, although they are chemically different from each other. According to Liu and others, the calculated chemical similarity scores between FDA-drugs and popular flavonoids were less than 0.6 for the majority of FDA-approved antidiabetic drugs, except some SGLT2 inhibitors (liu et al., 2020). We conducted a similarity score calculation using RDKIT (unpublished data) and found that it was less than 0.6.
Hypoxia and impaired adaptive responses to hypoxia in diabetic tissues as a result of inadequate HIF-1 stimulation seem to be pathogenic agents in the development of diabetes and its afflictions. However, the detailed mechanism has not yet been discovered (Catrina and Zheng, 2021). The outcomes of network analysis showed that HIF-1 is a major hub in the PPI network and might contribute to the antidiabetic mechanisms of MA. The molecular docking scores of MA compounds with HIF-1 were very good and suggested a probable activity. A previous study indicated that quercetin activates and increases the accumulation of HIF-1α in normoxia. However, other studies in cancer models showed the opposite effect with apigenin and quercetin, suggesting a complex role of HIF-1 in different health states (Wilson and Poellinger, 2002; Park et al., 2008; Samec et al., 2021). More studies are needed to detect the exact role and molecular mechanism of HIF-1 in the diabetic state and how it could be exploited as a therapeutic target.
The limitation of our study lies in its inability to dictate the extent to which every single mechanism contributes to the final observed phenotype. It is possible that the proposed different mechanisms collectively contribute and cooperate to improve the disease state. However, it is very likely that some regulated mechanisms contribute more to treatment than their rivals. These issues can be resolved in the future by further detailed mechanistic studies. Another limitation is the small number of animals used in the experiment. However, if we consider the large effect size needed to be detected (in blood glucose and other parameters), we will find that the small sample size is sufficient. Before considering this plant for advanced clinical trials in humans, larger studies using a higher number of animals are warranted in future studies. In addition, the network model in our study was based on the chemical profile of MA ethanolic extract, taking into account the minor constituents’ interactions. The role of minor constituents needs to be validated or refuted in further synergy studies.
In this study, we established for the first time the antidiabetic potential of Matricaria aurea. Integrating different channels of evidence: experimental, computational biology, and computational chemistry, helped us to elucidate the general action mechanisms that mediate MA antidiabetic activity at the molecular level from a systems biology perspective. This mechanism can be summarized in modulation of inflammatory pathways, energy sensing/endocrine/metabolic pathways, as well as oxidative stress pathways. MA can serve as a source of new antidiabetics and nutraceutical formulations to improve diabetic state.
The original contributions presented in the study are included in the article/Supplementary Material, further inquiries can be directed to the corresponding author.
The animal study was reviewed and approved by the Research Ethics Committee of the Faculty of Pharmacy, Suez Canal University, Ismailia, Egypt.
YI, DA, SS, and DF contributed to conception and design. YI and DF prepared the plant extracts. YI, DF, and WZ performed the experiments. YI and MA performed network pharmacology analysis. YI and MA performed literature review and data analysis. YI and MG wrote the article. All authors read and approved the final manuscript.
This study was supported by the Desert Research Center (DRC), for which the first, second, and fourth authors work. The DRC is a governmental research center that provides funds for its members and staff. The funds only cover the research materials, such as kits and chemicals. However, no specific grants were used to fund the study.
The authors greatly acknowledge the lab of proteomics and metabolomics at Children’s Cancer Hospital (57357) for performing the LC-MS/MS.
The authors declare that the research was conducted in the absence of any commercial or financial relationships that could be construed as a potential conflict of interest.
All claims expressed in this article are solely those of the authors and do not necessarily represent those of their affiliated organizations, or those of the publisher, the editors and the reviewers. Any product that may be evaluated in this article, or claim that may be made by its manufacturer, is not guaranteed or endorsed by the publisher.
The Supplementary Material for this article can be found online at: https://www.frontiersin.org/articles/10.3389/fphar.2022.924478/full#supplementary-material
Abd Allah, N. B., Toraih, E. A., Al Ageeli, E., Elhagrasy, H., Gouda, N. S., Fawzy, M. S., et al. (2021). MYD88, NFKB1, and IL6 transcripts overexpression are associated with poor outcomes and short survival in neonatal sepsis. Sci. Rep. 11, 13374. doi:10.1038/s41598-021-92912-7
Abdelhalim, A., Aburjai, T., Hanrahan, J., and Abdel-Halim, H. (2017). Medicinal plants used by traditional healers in Jordan, the tafila region. Pharmacogn. Mag. 13, S95–S101. doi:10.4103/0973-1296.203975
Abo-elmatty, D. M., Essawy, S. S., Badr, J. M., and Sterner, O. (2013). Antioxidant and anti-inflammatory effects of Urtica pilulifera extracts in type 2 diabetic rats. J. Ethnopharmacol. 145, 269–277. doi:10.1016/j.jep.2012.11.002
Aboul-Ela, M., El-Lakany, A., and Hijazi, M. (2012). “General introduction on family Asteracea,” in Phytochemicals—A Global Perspective of Their Role in Nutrition and Health. Editors V. Rao (Rijeka, Croatia: InTech), 375–400. doi:10.5772/28956
Ahmad, I., Mir, M. A., Srivastava, S., Shati, A. A., Elbehairi, S. E. I., Irfan, S., et al. (2021). Phytochemical screening and in-vitro antibacterial and anticancer activity of crude extract of Matricaria aurea. Curr. Pharm. Des. 27, 69–79. doi:10.2174/1381612826666201207105620
Ahmad, I., Wahab, S., Nisar, N., Dera, A. A., Alshahrani, M. Y., Abullias, S. S., et al. (2020). Evaluation of antibacterial properties of Matricaria aurea on clinical isolates of periodontitis patients with special reference to red complex bacteria. Saudi Pharm. J. 28, 1203–1209. doi:10.1016/j.jsps.2020.08.010
Al-Dabbagh, B., Elhaty, I. A., Elhaw, M., Murali, C., al Mansoori, A., Awad, B., et al. (2019). Antioxidant and anticancer activities of chamomile (Matricaria recutita L.). BMC Res. Notes 12, 3. doi:10.1186/s13104-018-3960-y
Al-Musa, H., and Al-Hashem, F. (2014). Hypoglycemic, hepato-renal and antioxidant potential effects of Chamomile recutita flowers ethanolic extract in streptozotocin-diabetic rats. Am. J. Pharmacol. Toxicol. 9, 1–12. doi:10.3844/ajptsp.2014.1.12
Al-Mustafa, A. H., and Al-Thuniba, O. Y. (2008). Antioxidant activity of some Jordanian medicinal plants used traditionally for treatment of diabetes. Pak. J. Biol. Sci. 11, 351–358. doi:10.3923/pjbs.2008.351.358
Alam, M. A., Subhan, N., Hossain, H., Hossain, M., Reza, H. M., Rahman, M. M., et al. (2016). Hydroxycinnamic acid derivatives: A potential class of natural compounds for the management of lipid metabolism and obesity. Nutr. Metab. 13, 27. doi:10.1186/s12986-016-0080-3
Aleixandre, A., Gil, J. V., Sineiro, J., and Rosell, C. M. (2022). Understanding phenolic acids inhibition of α-amylase and α-glucosidase and influence of reaction conditions. Food Chem. 372, 131231. doi:10.1016/j.foodchem.2021.131231
Ali-Shtayeh, M. S., Jamous, R. M., and Jamous, R. M. (2015). Plants used during pregnancy, childbirth, postpartum and infant healthcare in Palestine. Complement. Ther. Clin. Pract. 21, 84–93. doi:10.1016/j.ctcp.2015.03.004
Bao, L., Li, J., Zha, D., Zhang, L., Gao, P., Yao, T., et al. (2018). Chlorogenic acid prevents diabetic nephropathy by inhibiting oxidative stress and inflammation through modulation of the Nrf2/HO-1 and NF-ĸB pathways. Int. Immunopharmacol. 54, 245–253. doi:10.1016/j.intimp.2017.11.021
Baran-Gale, J., Fannin, E. E., Kurtz, C. L., and Sethupathy, P. (2013). Beta cell 5’-shifted isomiRs are candidate regulatory hubs in type 2 diabetes. PLoS One 8, e73240. doi:10.1371/journal.pone.0073240
Barrière, D. A., Noll, C., Roussy, G., Lizotte, F., Kessai, A., Kirby, K., et al. (2018). Combination of high-fat/high-fructose diet and low-dose streptozotocin to model long-term type-2 diabetes complications. Sci. Rep. 8, 424. doi:10.1038/s41598-017-18896-5
Bayliak, M. M., Dmytriv, T. R., Melnychuk, A. v., Strilets, N. v., Storey, K. B., and Lushchak, V. I. (2021). Chamomile as a potential remedy for obesity and metabolic syndrome. EXCLI J. 20, 1261–1286. doi:10.17179/excli2021-4013
Beekmann, K., Rubió, L., de Haan, L. H. J., Actis-Goretta, L., van der Burg, B., van Bladeren, P. J., et al. (2015). The effect of quercetin and kaempferol aglycones and glucuronides on peroxisome proliferator-activated receptor-gamma (PPAR-γ). Food Funct. 6, 1098–1107. doi:10.1039/c5fo00076a
Bhaskaran, N., Shukla, S., Kanwal, R., Srivastava, J. K., and Gupta, S. (2012). Induction of heme oxygenase-1 by chamomile protects murine macrophages against oxidative stress. Life Sci. 90, 1027–1033. doi:10.1016/j.lfs.2012.05.019
Bhaskaran, N., Shukla, S., Srivastava, J. K., and Gupta, S. (2010). Chamomile: An anti-inflammatory agent inhibits inducible nitric oxide synthase expression by blocking RelA/p65 activity. Int. J. Mol. Med. 26, 935–940. doi:10.3892/ijmm_00000545
Bhaskaran, N., Srivastava, J. K., Shukla, S., and Gupta, S. (2013). Chamomile confers protection against hydrogen peroxide-induced toxicity through activation of nrf2-mediated defense response. Phytother. Res. 27, 118–125. doi:10.1002/ptr.4701
Bhatia, P., Raina, S., Chugh, J., and Sharma, S. (2015). miRNAs: early prognostic biomarkers for Type 2 diabetes mellitus? Biomark. Med. 9, 1025–1040. doi:10.2217/bmm.15.69
Bulgari, M., Sangiovanni, E., Colombo, E., Maschi, O., Caruso, D., Bosisio, E., et al. (2012). Inhibition of neutrophil elastase and Metalloprotease-9 of human adenocarcinoma gastric cells by chamomile ( Matricaria recutita L.) infusion. Phytother. Res. 26, 1817–1822. doi:10.1002/ptr.4657
Bumke-Vogt, C., Osterhoff, M. A., Borchert, A., Guzman-Perez, V., Sarem, Z., Birkenfeld, A. L., et al. (2014). The flavones apigenin and luteolin induce FOXO1 translocation but inhibit gluconeogenic and lipogenic gene expression in human cells. PLoS One 9, e104321. doi:10.1371/journal.pone.0104321
Burgos-Morón, E., Abad-Jiménez, Z., de Marañón, A. M., Iannantuoni, F., Escribano-López, I., López-Domènech, I., et al. (2019). Relationship between oxidative stress, ER stress, and inflammation in type 2 diabetes: The battle continues. J. Clin. Med. 8, 1385. doi:10.3390/jcm8091385
Cai, D., Yuan, M., Frantz, D. F., Melendez, P. A., Hansen, L., Lee, J., et al. (2005). Local and systemic insulin resistance resulting from hepatic activation of IKK-beta and NF-kappaB. Nat. Med. 11, 183–190. doi:10.1038/nm1166
Catrina, S.-B., and Zheng, X. (2021). Hypoxia and hypoxia-inducible factors in diabetes and its complications. Diabetologia 64, 709–716. doi:10.1007/s00125-021-05380-z
Catrysse, L., and van Loo, G. (2017). Inflammation and the metabolic syndrome: The tissue-specific functions of NF-κB. Trends Cell. Biol. 27, 417–429. doi:10.1016/j.tcb.2017.01.006
Cemek, M., Kağa, S., Şimşek, N., Büyükokuroğlu, M. E., and Konuk, M. (2008). Antihyperglycemic and antioxidative potential of Matricaria chamomilla L. in streptozotocin-induced diabetic rats. J. Nat. Med. 62, 284–293. doi:10.1007/s11418-008-0228-1
Ceriello, A., and Motz, E. (2004). Is oxidative stress the pathogenic mechanism underlying insulin resistance, diabetes, and cardiovascular disease? The common soil hypothesis revisited. Arterioscler. Thromb. Vasc. Biol. 24, 816–823. doi:10.1161/01.ATV.0000122852.22604.78
Chandran, U., Mehendale, N., Patil, S., Chaguturu, R., and Patwardhan, B. (2017). “Network pharmacology,” in Innovative approaches in drug Discovery. Editors B. Patwardhan, and R. Chaguturu (Boston, MA: Academic Press), 127–164. doi:10.1016/B978-0-12-801814-9.00005-2
Chowdhury, S., Ghosh, S., Das, A. K., and Sil, P. C. (2019). Ferulic acid protects hyperglycemia-induced kidney damage by regulating oxidative insult, inflammation and autophagy. Front. Pharmacol. 10, 27. doi:10.3389/fphar.2019.00027
Choy, K. W., Murugan, D., Leong, X.-F., Abas, R., Alias, A., and Mustafa, M. R. (2019). Flavonoids as natural anti-inflammatory agents targeting nuclear factor-kappa B (NFκB) signaling in cardiovascular diseases: A Mini review. Front. Pharmacol. 10, 1295. doi:10.3389/fphar.2019.01295
Curra, M., Martins, M. A. T., Lauxen, I. S., Pellicioli, A. C. A., Sant’Ana Filho, M., Pavesi, V. C. S., et al. (2013). Effect of topical chamomile on immunohistochemical levels of IL-1β and TNF-α in 5-fluorouracil-induced oral mucositis in hamsters. Cancer Chemother. Pharmacol. 71, 293–299. doi:10.1007/s00280-012-2013-9
Czech, M. P. (2017). Insulin action and resistance in obesity and type 2 diabetes. Nat. Med. 23, 804–814. doi:10.1038/nm.4350
Daina, A., Michielin, O., and Zoete, V. (2017). SwissADME: A free web tool to evaluate pharmacokinetics, drug-likeness and medicinal chemistry friendliness of small molecules. Sci. Rep. 7, 42717. doi:10.1038/srep42717
David, J. A., Rifkin, W. J., Rabbani, P. S., and Ceradini, D. J. (2017). The nrf2/keap1/ARE pathway and oxidative stress as a therapeutic target in type II diabetes mellitus. J. Diabetes Res. 2017, 4826724. doi:10.1155/2017/4826724
Dhanya, R., Arya, A. D., Nisha, P., and Jayamurthy, P. (2017). Quercetin, a lead compound against type 2 diabetes ameliorates glucose uptake via AMPK pathway in skeletal muscle cell line. Front. Pharmacol. 8, 336. doi:10.3389/fphar.2017.00336
DiNicolantonio, J. J., McCarty, M. F., Assanga, S. I., Lujan, L. L., and O’Keefe, J. H. (2022). Ferulic acid and berberine, via Sirt1 and AMPK, may act as cell cleansing promoters of healthy longevity. Open Heart 9, e001801. doi:10.1136/openhrt-2021-001801
Doi, M., Morita, N., Okuzawa, T., Ohgiya, S., Okamoto, D., Sato, K., et al. (2022). Pinellic acid isolated from quercetin-rich onions has a peroxisome proliferator-activated receptor-alpha/gamma (PPAR-α/γ) transactivation activity. Planta Med. 88, 440–446. doi:10.1055/a-1345-9471
Dong, Y., Zhao, Q., and Wang, Y. (2021). Network pharmacology-based investigation of potential targets of astragalus membranaceous-angelica sinensis compound acting on diabetic nephropathy. Sci. Rep. 11, 19496. doi:10.1038/s41598-021-98925-6
Dooley, J., Garcia-Perez, J. E., Sreenivasan, J., Schlenner, S. M., Vangoitsenhoven, R., Papadopoulou, A. S., et al. (2016). The microRNA-29 family dictates the balance between homeostatic and pathological glucose handling in diabetes and obesity. Diabetes 65, 53–61. doi:10.2337/db15-0770
Eddouks, M., Lemhadri, A., Zeggwagh, N. A., and Michel, J.-B. (2005). Potent hypoglycaemic activity of the aqueous extract of Chamaemelum nobile in normal and streptozotocin-induced diabetic rats. Diabetes Res. Clin. Pract. 67, 189–195. doi:10.1016/j.diabres.2004.07.015
Ehses, J. A., Lacraz, G., Giroix, M.-H., Schmidlin, F., Coulaud, J., Kassis, N., et al. (2009). IL-1 antagonism reduces hyperglycemia and tissue inflammation in the type 2 diabetic GK rat. Proc. Natl. Acad. Sci. U. S. A. 106, 13998–14003. doi:10.1073/pnas.0810087106
El Sayed, A. M., Basam, S. M., El-Naggar, E.-M. B. A., Marzouk, H. S., and El-Hawary, S. (2020). LC-MS/MS and GC-MS profiling as well as the antimicrobial effect of leaves of selected Yucca species introduced to Egypt. Sci. Rep. 10, 17778. doi:10.1038/s41598-020-74440-y
Elo, B., Villano, C. M., Govorko, D., and White, L. A. (2007). Larval zebrafish as a model for glucose metabolism: Expression of phosphoenolpyruvate carboxykinase as a marker for exposure to anti-diabetic compounds. J. Mol. Endocrinol. 38, 433–440. doi:10.1677/JME-06-0037
Eraky, S. M., Ramadan, N. M., and Abo El-Magd, N. F. (2022). Antidiabetic effects of quercetin and liraglutide combination through modulation of TXNIP/IRS-1/PI3K pathway. Cell. biochem. Funct. 40, 90–102. doi:10.1002/cbf.3678
Feng, X., Weng, D., Zhou, F., Owen, Y. D., Qin, H., Zhao, J., et al. (2016). Activation of PPARγ by a natural flavonoid modulator, apigenin ameliorates obesity-related inflammation via regulation of macrophage polarization. EBioMedicine 9, 61–76. doi:10.1016/j.ebiom.2016.06.017
Fossati, P., Prencipe, L., and Berti, G. (1980). Use of 3, 5-dichloro-2-hydroxybenzenesulfonic acid/4-aminophenazone chromogenic system in direct enzymic assay of uric acid in serum and urine. Clin. Chem. 26, 227–231. doi:10.1093/clinchem/26.2.0227
Galicia-Garcia, U., Benito-Vicente, A., Jebari, S., Larrea-Sebal, A., Siddiqi, H., Uribe, K. B., et al. (2020). Pathophysiology of type 2 diabetes mellitus. Int. J. Mol. Sci. 21, E6275. doi:10.3390/ijms21176275
Gao, J., He, X., Ma, Y., Zhao, X., Hou, X., Hao, E., et al. (2018). Chlorogenic acid targeting of the AKT PH domain activates AKT/GSK3β/FOXO1 signaling and improves glucose metabolism. Nutrients 10, E1366. doi:10.3390/nu10101366
Ge, W., Zhao, Y., Yang, Y., Ding, Z., Xu, X., Weng, D., et al. (2021). An insulin-independent mechanism for transcriptional regulation of Foxo1 in type 2 diabetic mice. J. Biol. Chem. 297, 100846. doi:10.1016/j.jbc.2021.100846
Ghosh, S., Chowdhury, S., Sarkar, P., and Sil, P. C. (2018). Ameliorative role of ferulic acid against diabetes associated oxidative stress induced spleen damage. Food Chem. Toxicol. 118, 272–286. doi:10.1016/j.fct.2018.05.029
Gomez-Peralta, F., and Abreu Padín, C. (2014). Do we need new treatments for type 2 diabetes? Endocrinol. Nutr. (English Ed. 61, 323–328. doi:10.1016/j.endoen.2014.06.002
Gómez-Valadés, A. G., Méndez-Lucas, A., Vidal-Alabró, A., Blasco, F. X., Chillon, M., Bartrons, R., et al. (2008). Pck1 gene silencing in the liver improves glycemia control, insulin sensitivity, and dyslipidemia in db/db mice. Diabetes 57, 2199–2210. doi:10.2337/db07-1087
Hajizadeh-Sharafabad, F., Varshosaz, P., Jafari-Vayghan, H., Alizadeh, M., and Maleki, V. (2020). Chamomile (Matricaria recutita L.) and diabetes mellitus, current knowledge and the way forward: A systematic review. Complement. Ther. Med. 48, 102284. doi:10.1016/j.ctim.2019.102284
He, A., Zhu, L., Gupta, N., Chang, Y., and Fang, F. (2007). Overexpression of micro ribonucleic acid 29, highly up-regulated in diabetic rats, leads to insulin resistance in 3T3-L1 adipocytes. Mol. Endocrinol. 21, 2785–2794. doi:10.1210/me.2007-0167
Islam, Md. S., and Choi, H. (2007). Nongenetic model of type 2 diabetes: A comparative study. Pharmacology 79, 243–249. doi:10.1159/000101989
Ito, Y., Oumi, S., Nagasawa, T., and Nishizawa, N. (2006). Oxidative stress induces phosphoenolpyruvate carboxykinase expression in H4IIE cells. Biosci. Biotechnol. Biochem. 70, 2191–2198. doi:10.1271/bbb.60135
Jabri, M.-A., Rtibi, K., and Sebai, H. (2022). Chamomile decoction mitigates high fat diet-induced anxiety-like behavior, neuroinflammation and cerebral ROS overload. Nutr. Neurosci. 25, 1350–1361. doi:10.1080/1028415X.2020.1859727
Jabri, M.-A., Sakly, M., Marzouki, L., and Sebai, H. (2017). Chamomile (Matricaria recutita L.) decoction extract inhibits in vitro intestinal glucose absorption and attenuates high fat diet-induced lipotoxicity and oxidative stress. Biomed. Pharmacother. 87, 153–159. doi:10.1016/j.biopha.2016.12.043
Jin, S., Chang, C., Zhang, L., Liu, Y., Huang, X., and Chen, Z. (2015). Chlorogenic acid improves late diabetes through adiponectin receptor signaling pathways in db/db mice. PLoS One 10, e0120842. doi:10.1371/journal.pone.0120842
Joshi, T., Singh, A. K., Haratipour, P., Sah, A. N., Pandey, A. K., Naseri, R., et al. (2019). Targeting AMPK signaling pathway by natural products for treatment of diabetes mellitus and its complications. J. Cell. Physiol. 234, 17212–17231. doi:10.1002/jcp.28528
Kalivarathan, J., Kalaivanan, K., Chandrasekaran, S. P., Nanda, D., Ramachandran, V., and Carani Venkatraman, A. (2020). Apigenin modulates hippocampal CREB-BDNF signaling in high fat, high fructose diet-fed rats. J. Funct. Foods 68, 103898. doi:10.1016/j.jff.2020.103898
Kaseb, F., Yazdanpanah, Z., Biregani, A. N., Yazdi, N. B., and Yazdanpanah, Z. (2018). The effect of chamomile (Matricaria recutita L.) infusion on blood glucose, lipid profile and kidney function in type 2 diabetic patients: A randomized clinical trial. Prog. Nutr. 20, 110–118. doi:10.23751/pn.v20i1-S.5884
Kato, A., Minoshima, Y., Yamamoto, J., Adachi, I., Watson, A. A., and Nash, R. J. (2008). Protective effects of dietary chamomile tea on diabetic complications. J. Agric. Food Chem. 56, 8206–8211. doi:10.1021/jf8014365
Khan, M., Abdullah, M. M. S., Mahmood, A., Al-Mayouf, A. M., and Alkhathlan, H. Z. (2019). Evaluation of Matricaria aurea extracts as effective anti-corrosive agent for Mild steel in 1.0 M HCl and isolation of their active ingredients. Sustainability 11, 7174. doi:10.3390/su11247174
Khan, M. A. B., Hashim, M. J., King, J. K., Govender, R. D., Mustafa, H., and al Kaabi, J. (2020). Epidemiology of type 2 diabetes - global burden of disease and forecasted trends. J. Epidemiol. Glob. Health 10, 107–111. doi:10.2991/jegh.k.191028.001
Kheder, F. B. H., Mahjoub, M. A., Zaghrouni, F., Kwaja, S., Helal, A. N., and Mighri, Z. (2014). Chemical composition antioxidant and antimicrobial activities of the essential oils of Matricaria aurea loefl. Growing in Tunisia. J. Essent. Oil Bear. Plants 17, 493–505. doi:10.1080/0972060X.2014.884777
Khodadadi, A., Pipelzadeh, M. H., Aghel, N., Esmaeilian, M., and Zali, I. (2011). A comparative study upon the therapeutic indices of some natural and synthetic anti-inflammatory agents. Iran. J. Basic Med. Sci. 14, 340–348.
Kim, D. H., Kim, J. M., Lee, E. K., Choi, Y. J., Kim, C. H., Choi, J. S., et al. (2012). Modulation of FoxO1 phosphorylation/acetylation by baicalin during aging. J. Nutr. Biochem. 23, 1277–1284. doi:10.1016/j.jnutbio.2011.07.008
Kulkarni, R., Acharya, J., Ghaskadbi, S., and Goel, P. (2014). Oxidative stress as a covariate of recovery in diabetes therapy. Front. Endocrinol. 5, 89. doi:10.3389/fendo.2014.00089
Kurtz, C. L., Fannin, E. E., Toth, C. L., Pearson, D. S., Vickers, K. C., and Sethupathy, P. (2015). Inhibition of miR-29 has a significant lipid-lowering benefit through suppression of lipogenic programs in liver. Sci. Rep. 5, 12911. doi:10.1038/srep12911
Kurtz, C. L., Peck, B. C. E., Fannin, E. E., Beysen, C., Miao, J., Landstreet, S. R., et al. (2014). MicroRNA-29 fine-tunes the expression of key FOXA2-activated lipid metabolism genes and is dysregulated in animal models of insulin resistance and diabetes. Diabetes 63, 3141–3148. doi:10.2337/db13-1015
Lee, S. B., Kim, M. K., Kang, S., Park, K., Kim, J. H., Baik, S. J., et al. (2019). Triglyceride glucose index is superior to the homeostasis model assessment of insulin resistance for predicting nonalcoholic fatty liver disease in Korean adults. Endocrinol. Metab. 34, 179–186. doi:10.3803/EnM.2019.34.2.179
Li, J. W.-H., and Vederas, J. C. (2009). Drug discovery and natural products: End of an era or an endless frontier? Science 325, 161–165. doi:10.1126/science.1168243
Li, W., Yuan, G., Pan, Y., Wang, C., and Chen, H. (2017). Network pharmacology studies on the bioactive compounds and action mechanisms of natural products for the treatment of diabetes mellitus: A review. Front. Pharmacol. 8, 74. doi:10.3389/fphar.2017.00074
Liu, R., Su, C., Xu, Y., Shang, K., Sun, K., Li, C., et al. (2020). Identifying potential active components of walnut leaf that action diabetes mellitus through integration of UHPLC-Q-Orbitrap HRMS and network pharmacology analysis. J. Ethnopharmacol. 253, 112659. doi:10.1016/j.jep.2020.112659
Liu, Y., Qiu, Y., Chen, Q., Han, X., Cai, M., and Hao, L. (2021). Puerarin suppresses the hepatic gluconeogenesis via activation of PI3K/Akt signaling pathway in diabetic rats and HepG2 cells. Biomed. Pharmacother. = Biomedecine Pharmacother. 137, 111325. doi:10.1016/j.biopha.2021.111325
Malik, S., Suchal, K., Khan, S. I., Bhatia, J., Kishore, K., Dinda, A. K., et al. (2017). Apigenin ameliorates streptozotocin-induced diabetic nephropathy in rats via MAPK-NF-κB-TNF-α and TGF-β1-MAPK-fibronectin pathways. Am. J. Physiol. Ren. Physiol. 313, F414–F422. doi:10.1152/ajprenal.00393.2016
Malunga, L. N., Joseph Thandapilly, S., and Ames, N. (2018). Cereal‐derived phenolic acids and intestinal alpha glucosidase activity inhibition: Structural activity relationship. J. Food Biochem. 42, e12635. doi:10.1111/jfbc.12635
Mannaa, F., Ibrahim, N., Ibrahim, S., Abdel-Wahhab, K., Hassan, N., and Mohammed, S. (2015). Preventive role of chamomile flowers and fennel seeds extracts against liver injury and oxidative stress induced by an immunosuppressant drug in rats. Hepatoma Res. 1, 125. doi:10.4103/2394-5079.167375
Menghini, L., Ferrante, C., Leporini, L., Recinella, L., Chiavaroli, A., Leone, S., et al. (2016). An hydroalcoholic chamomile extract modulates inflammatory and immune response in HT29 cells and isolated rat colon. Phytother. Res. 30, 1513–1518. doi:10.1002/ptr.5655
Minaiyan, M., ghassemi-Dehkordi, N., Mahzouni, P., and Ansari-Roknabady, M. (2011). Effect of Matricaria aurea (loefl.) shultz-bip. Hydroalcoholic extract on acetic acid-induced acute colitis in rats. Iran. J. Basic Med. Sci. 14, 67–74. doi:10.22038/ijbms.2011.4966
Mohammad Al-Ismail, K., and Aburjai, T. (2004). Antioxidant activity of water and alcohol extracts of chamomile flowers, anise seeds and dill seeds. J. Sci. Food Agric. 84, 173–178. doi:10.1002/jsfa.1625
Mohammed, H. A., Khan, R. A., Abdel-Hafez, A. A., Abdel-Aziz, M., Ahmed, E., Enany, S., et al. (2021). Phytochemical profiling, in vitro and in silico anti-Microbial and anti-cancer activity evaluations and staph GyraseB and h-TOP-iiβ receptor-docking studies of major constituents of zygophyllum coccineum L. Aqueous-ethanolic extract and its subsequent fractions: An approach to validate traditional phytomedicinal knowledge. Molecules 26, 577. doi:10.3390/molecules26030577
Molnar, M., Mendešević, N., Šubarić, D., Banjari, I., and Jokić, S. (2017). Comparison of various techniques for the extraction of umbelliferone and herniarin in Matricaria chamomilla processing fractions. Chem. Cent. J. 11, 78. doi:10.1186/s13065-017-0308-y
Mozaffari Godarzi, S., Valizade Gorji, A., Gholizadeh, B., Mard, S. A., and Mansouri, E. (2020). Antioxidant effect of p-coumaric acid on interleukin 1-β and tumor necrosis factor-α in rats with renal ischemic reperfusion. Nefrología 40, 311–319. doi:10.1016/j.nefro.2019.10.003
Nargesi, S., Moayeri, A., Ghorbani, A., Seifinejad, Y., and Shirzadpour, E. (2018). The effects of Matricaria chamomilla L.hydroalcoholic extract on atherosclerotic plaques, antioxidant activity, lipid profile and inflammatory indicators in rats. Biomed. Res. Ther. 5, 2752–2761. doi:10.15419/bmrat.v5i10.490
Nepali, S., Son, J. S., Poudel, B., Lee, J. H., Lee, Y. M., and Kim, D. K. (2015). Luteolin is a bioflavonoid that attenuates adipocyte-derived inflammatory responses via suppression of nuclear factor-κB/mitogen-activated protein kinases pathway. Pharmacogn. Mag. 11, 627–635. doi:10.4103/0973-1296.160470
Nisha, V. M., Anusree, S. S., Priyanka, A., and Raghu, K. G. (2014). Apigenin and quercetin ameliorate mitochondrial alterations by tunicamycin-induced ER stress in 3T3-L1 adipocytes. Appl. Biochem. Biotechnol. 174, 1365–1375. doi:10.1007/s12010-014-1129-2
Nishikimi, M., Appaji Rao, N., and Yagi, K. (1972). The occurrence of superoxide anion in the reaction of reduced phenazine methosulfate and molecular oxygen. Biochem. Biophys. Res. Commun. 46, 849–854. doi:10.1016/S0006-291X(72)80218-3
Oguntibeju, O. O. (2019). Type 2 diabetes mellitus, oxidative stress and inflammation: Examining the links. Int. J. Physiol. Pathophysiol. Pharmacol. 11, 45–63.
Oh, K. K., Adnan, M., and Cho, D. H. (2020). Network pharmacology of bioactives from Sorghum bicolor with targets related to diabetes mellitus. PLoS One 15, e0240873. doi:10.1371/journal.pone.0240873
Ohkawa, H., Ohishi, N., and Yagi, K. (1979). Assay for lipid peroxides in animal tissues by thiobarbituric acid reaction. Anal. Biochem. 95, 351–358. doi:10.1016/0003-2697(79)90738-3
Ong, K. W., Hsu, A., and Tan, B. K. H. (2012). Chlorogenic acid stimulates glucose transport in skeletal muscle via AMPK activation: A contributor to the beneficial effects of coffee on diabetes. PLoS ONE 7, e32718. doi:10.1371/journal.pone.0032718
Ortiz, M. I., Cariño-Cortés, R., Ponce-Monter, H. A., González-García, M. P., Castañeda-Hernández, G., and Salinas-Caballero, M. (2017). Synergistic interaction of Matricaria chamomilla extract with diclofenac and indomethacin on carrageenan-induced paw inflammation in rats. Drug Dev. Res. 78, 360–367. doi:10.1002/ddr.21401
Owens, C. W., and Belcher, R. V. (1965). A colorimetric micro-method for the determination of glutathione. Biochem. J. 94, 705–711. doi:10.1042/bj0940705
Pallauf, K., Duckstein, N., Hasler, M., Klotz, L.-O., and Rimbach, G. (2017). Flavonoids as putative inducers of the transcription factors Nrf2, FoxO, and PPARγ. Oxid. Med. Cell. Longev. 2017, 4397340. doi:10.1155/2017/4397340
Pandey, A. K., Verma, G., Vig, S., Srivastava, S., Srivastava, A. K., and Datta, M. (2011). miR-29a levels are elevated in the db/db mice liver and its overexpression leads to attenuation of insulin action on PEPCK gene expression in HepG2 cells. Mol. Cell. Endocrinol. 332, 125–133. doi:10.1016/j.mce.2010.10.004
Park, S.-S., Bae, I., and Lee, Y. J. (2008). Flavonoids-induced accumulation of hypoxia-inducible factor (HIF)-1alpha/2alpha is mediated through chelation of iron. J. Cell. Biochem. 103, 1989–1998. doi:10.1002/jcb.21588
Peng, S.-G., Pang, Y.-L., Zhu, Q., Kang, J.-H., Liu, M.-X., and Wang, Z. (2018). Chlorogenic acid functions as a novel agonist of PPARγ2 during the differentiation of Mouse 3T3-L1 preadipocytes. Biomed. Res. Int. 2018, 8594767. doi:10.1155/2018/8594767
Peng, S., Li, W., Hou, N., and Huang, N. (2020). A review of FoxO1-regulated metabolic diseases and related drug discoveries. Cells 9, 184. doi:10.3390/cells9010184
Pereira, S. V., Reis, R. A. S. P., Garbuio, D. C., and de Freitas, L. A. P. (2018). Dynamic maceration of Matricaria chamomilla inflorescences: Optimal conditions for flavonoids and antioxidant activity. Rev. Bras. Farmacogn. 28, 111–117. doi:10.1016/j.bjp.2017.11.006
Pérez-Bonilla, M., Salido, S., Sánchez, A., van Beek, T. A., and Altarejos, J. (2013). Effect of extraction conditions on the antioxidant activity of olive wood extracts. Int. J. Food Sci. 2013, 719593. doi:10.1155/2013/719593
Qnais, E. (2011). The analgesic effect of the ethanolic extract of Matricaria aurea. Turkish J. Biol. 35, 347–352. doi:10.3906/biy-0909-22
Que, W., Chen, M., Yang, L., Zhang, B., Zhao, Z., Liu, M., et al. (2021). A network pharmacology-based investigation on the bioactive ingredients and molecular mechanisms of Gelsemium elegans Benth against colorectal cancer. BMC Complement. Med. Ther. 21, 99. doi:10.1186/s12906-021-03273-7
Rafraf, M., Zemestani, M., and Asghari-Jafarabadi, M. (2015). Effectiveness of chamomile tea on glycemic control and serum lipid profile in patients with type 2 diabetes. J. Endocrinol. Invest. 38, 163–170. doi:10.1007/s40618-014-0170-x
Razavi, T., Kouhsari, S. M., and Abnous, K. (2019). Morin exerts anti-diabetic effects in human HepG2 cells via down-regulation of miR-29a. Exp. Clin. Endocrinol. Diabetes 127, 615–622. doi:10.1055/a-0650-4082
Rehman, K., and Akash, M. S. H. (2016). Mechanisms of inflammatory responses and development of insulin resistance: How are they interlinked? J. Biomed. Sci. 23, 87. doi:10.1186/s12929-016-0303-y
Ren, Y., Long, S., and Cao, S. (2021). Molecular docking and virtual screening of an influenza virus inhibitor that disrupts protein–protein interactions. Viruses 13, 2229. doi:10.3390/v13112229
Rigano, D., Sirignano, C., and Taglialatela-Scafati, O. (2017). The potential of natural products for targeting PPAR α. Acta Pharm. Sin. B 7, 427–438. doi:10.1016/j.apsb.2017.05.005
Russo, C., Edwards, K. D., Margetts, G., Kleidonas, S., Zaibi, N. S., Clapham, J. C., et al. (2021). Effects of Salvia officinalis L. and Chamaemelum nobile (L.) extracts on inflammatory responses in two models of human cells: Primary subcutaneous adipocytes and neuroblastoma cell line (SK-N-SH). J. Ethnopharmacol. 268, 113614. doi:10.1016/j.jep.2020.113614
Samec, M., Liskova, A., Koklesova, L., Mersakova, S., Strnadel, J., Kajo, K., et al. (2021). Flavonoids targeting HIF-1: Implications on cancer metabolism. Cancers (Basel) 13, E130. doi:10.3390/cancers13010130
Sanchez, M. B., Miranda-Perez, E., Verjan, J. C. G., de Los Angeles Fortis Barrera, M., Perez-Ramos, J., and Alarcon-Aguilar, F. J. (2017). Potential of the chlorogenic acid as multitarget agent: Insulin-secretagogue and PPAR α/γ dual agonist. Biomed. Pharmacother. = Biomedecine Pharmacother. 94, 169–175. doi:10.1016/j.biopha.2017.07.086
Shelbaya, L. (2017). Hypoglycemic and hypolipidemic effects of chamomile powder and oil with high fat high fructose diet in rats. Bull. Natl. Nutr. Inst. Arab Repub. Egypt 50, 170–195. doi:10.21608/bnni.2017.6728
Shi, T., Zhuang, R., Zhou, H., Wang, F., Shao, Y., and Cai, Z. (2015). Effect of apigenin on protein expressions of PPARs in liver tissues of rats with nonalcoholic steatohepatitis. Zhonghua Gan Zang Bing Za Zhi 23, 124–129. doi:10.3760/cma.j.issn.1007-3418.2015.02.010
Skovsø, S. (2014). Modeling type 2 diabetes in rats using high fat diet and streptozotocin. J. Diabetes Investig. 5, 349–358. doi:10.1111/jdi.12235
Şöhretoğlu, D., and Sari, S. (2020). Flavonoids as alpha-glucosidase inhibitors: Mechanistic approaches merged with enzyme kinetics and molecular modelling. Phytochem. Rev. 19, 1081–1092. doi:10.1007/s11101-019-09610-6
Song, Y., Wen, L., Sun, J., Bai, W., Jiao, R., Hu, Y., et al. (2016). Cytoprotective mechanism of ferulic acid against high glucose-induced oxidative stress in cardiomyocytes and hepatocytes. Food Nutr. Res. 60, 30323. doi:10.3402/fnr.v60.30323
Soubra, N., Yazbek, M. M., Noun, J., Talhouk, R., Tanios, S., and Karam, N. (2018). Evaluation of diversity and conservation status of Matricaria chamomilla (L.) and Matricaria aurea (Loefl.) Sch. Bip. in Lebanon. J. Biodivers. Endanger. Species 06, 1–10. doi:10.4172/2332-2543.1000206
Srinivasan, K., Viswanad, B., Asrat, L., Kaul, C. L., and Ramarao, P. (2005). Combination of high-fat diet-fed and low-dose streptozotocin-treated rat: A model for type 2 diabetes and pharmacological screening. Pharmacol. Res. 52, 313–320. doi:10.1016/j.phrs.2005.05.004
Srivastava, J. K., Pandey, M., and Gupta, S. (2009). Chamomile, a novel and selective COX-2 inhibitor with anti-inflammatory activity. Life Sci. 85, 663–669. doi:10.1016/j.lfs.2009.09.007
Takahama, U., and Hirota, S. (2018). Interactions of flavonoids with α-amylase and starch slowing down its digestion. Food Funct. 9, 677–687. doi:10.1039/c7fo01539a
Tangvarasittichai, S. (2015). Oxidative stress, insulin resistance, dyslipidemia and type 2 diabetes mellitus. World J. Diabetes 6, 456–480. doi:10.4239/wjd.v6.i3.456
Toraih, E. A., El-Wazir, A., Hussein, M. H., Khashana, M. S., Matter, A., Fawzy, M. S., et al. (2019). Expression of long intergenic non-coding RNA, regulator of reprogramming, and its prognostic value in patients with glioblastoma. Int. J. Biol. Markers 34, 69–79. doi:10.1177/1724600818814459
Toraih, E. A., Mohammed, E. A., Farrag, S., Ramsis, N., and Hosny, S. (2015). Pilot study of serum MicroRNA-21 as a diagnostic and prognostic biomarker in Egyptian breast cancer patients. Mol. Diagn. Ther. 19, 179–190. doi:10.1007/s40291-015-0143-6
Tsai, K.-L., Hung, C.-H., Chan, S.-H., Hsieh, P.-L., Ou, H.-C., Cheng, Y.-H., et al. (2018). Chlorogenic acid protects against oxLDL-induced oxidative damage and mitochondrial dysfunction by modulating SIRT1 in endothelial cells. Mol. Nutr. Food Res. 62, 1700928. doi:10.1002/mnfr.201700928
Villa-Rodriguez, J. A., Kerimi, A., Abranko, L., Tumova, S., Ford, L., Blackburn, R. S., et al. (2018). Acute metabolic actions of the major polyphenols in chamomile: An in vitro mechanistic study on their potential to attenuate postprandial hyperglycaemia. Sci. Rep. 8, 5471. doi:10.1038/s41598-018-23736-1
Wang, M., Xiao, F. L., Mao, Y. J., Ying, L. L., Zhou, B., and Li, Y. (2019). Quercetin decreases the triglyceride content through the PPAR signalling pathway in primary hepatocytes of broiler chickens. Biotechnol. Biotechnol. Equip. 33, 1000–1010. doi:10.1080/13102818.2019.1635528
Wang, W., Wang, Y., Zou, J., Jia, Y., Wang, Y., Li, J., et al. (2021). The mechanism action of German chamomile (Matricaria recutita L.) in the treatment of eczema: Based on dose–effect weight coefficient network pharmacology. Front. Pharmacol. 12, 706836. doi:10.3389/fphar.2021.706836
Weidner, C., Wowro, S. J., Rousseau, M., Freiwald, A., Kodelja, V., Abdel-Aziz, H., et al. (2013). Antidiabetic effects of chamomile flowers extract in obese mice through transcriptional stimulation of nutrient sensors of the peroxisome proliferator-activated receptor (PPAR) family. PLoS ONE 8, e80335. doi:10.1371/journal.pone.0080335
Wilson, W. J., and Poellinger, L. (2002). The dietary flavonoid quercetin modulates HIF-1 alpha activity in endothelial cells. Biochem. Biophys. Res. Commun. 293, 446–450. doi:10.1016/S0006-291X(02)00244-9
Witters, L. A. (2001). The blooming of the French lilac. J. Clin. Invest. 108, 1105–1107. doi:10.1172/JCI14178
Wright, E., Scism-Bacon, J. L., and Glass, L. C. (2006). Oxidative stress in type 2 diabetes: The role of fasting and postprandial glycaemia. Int. J. Clin. Pract. 60, 308–314. doi:10.1111/j.1368-5031.2006.00825.x
Xu, S., Wang, G., Peng, W., Xu, Y., Zhang, Y., Ge, Y., et al. (2019). Corosolic acid isolated from Eriobotrya japonica leaves reduces glucose level in human hepatocellular carcinoma cells, zebrafish and rats. Sci. Rep. 9, 4388. doi:10.1038/s41598-019-40934-7
Yan, Y., Zhou, X., Guo, K., Zhou, F., and Yang, H. (2020). Use of chlorogenic acid against diabetes mellitus and its complications. J. Immunol. Res. 2020, 9680508. doi:10.1155/2020/9680508
Yang, W.-M., Jeong, H.-J., Park, S.-Y., and Lee, W. (2014). Induction of miR-29a by saturated fatty acids impairs insulin signaling and glucose uptake through translational repression of IRS-1 in myocytes. FEBS Lett. 588, 2170–2176. doi:10.1016/j.febslet.2014.05.011
Yaniv, Z., Dafni, A., Friedman, J., and Palevitch, D. (1987). Plants used for the treatment of diabetes in Israel. J. Ethnopharmacol. 19, 145–151. doi:10.1016/0378-8741(87)90038-9
Yoon, H., Jeon, D. J., Park, C. E., You, H. S., and Moon, A. E. (2016). Relationship between homeostasis model assessment of insulin resistance and beta cell function and serum 25-hydroxyvitamin D in non-diabetic Korean adults. J. Clin. Biochem. Nutr. 59, 139–144. doi:10.3164/jcbn.15-143
Yousefbeyk, F., Hemmati, G., Gholipour, Z., Ghasemi, S., Evazalipour, M., Schubert, C., et al. (2022). Phytochemical analysis, antioxidant, cytotoxic, and antimicrobial activities of golden chamomile (Matricaria aurea (Loefl.) Schultz Bip). Z. Naturforsch. C J. Biosci. 77, 331–342. doi:10.1515/znc-2021-0269
Zemestani, M., Rafraf, M., and Asghari-Jafarabadi, M. (2016). Chamomile tea improves glycemic indices and antioxidants status in patients with type 2 diabetes mellitus. Nutrition 32, 66–72. doi:10.1016/j.nut.2015.07.011
Zemestani, M., Rafraf, M., and Asghari-Jafarabadi, M. (2018). Effects of chamomile tea on inflammatory markers and insulin resistance in patients with type 2 diabetes mellitus. Trends Gen. Pract. 1. doi:10.15761/TGP.1000117
Zhang, J., Zhao, L., Cheng, Q., Ji, B., Yang, M., Sanidad, K. Z., et al. (2018). Structurally different flavonoid subclasses attenuate high-fat and high-fructose diet induced metabolic syndrome in rats. J. Agric. Food Chem. 66, 12412–12420. doi:10.1021/acs.jafc.8b03574
Zhang, L., Shi, X., Huang, Z., Mao, J., Mei, W., Ding, L., et al. (2020). Network pharmacology approach to uncover the mechanism governing the effect of radix achyranthis bidentatae on osteoarthritis. BMC Complement. Med. Ther. 20, 121. doi:10.1186/s12906-020-02909-4
Zhang, R., Zhu, X., Bai, H., and Ning, K. (2019). Network pharmacology databases for traditional Chinese medicine: Review and assessment. Front. Pharmacol. 10, 123. doi:10.3389/fphar.2019.00123
Zhang, S., Du, T., Zhang, J., Lu, H., Lin, X., Xie, J., et al. (2017). The triglyceride and glucose index (TyG) is an effective biomarker to identify nonalcoholic fatty liver disease. Lipids Health Dis. 16, 15. doi:10.1186/s12944-017-0409-6
Zhu, H., and Leung, S. W. (2015). Identification of microRNA biomarkers in type 2 diabetes: A meta-analysis of controlled profiling studies. Diabetologia 58, 900–911. doi:10.1007/s00125-015-3510-2
Keywords: network pharmacology, LC-MS/MS, Matricaria aurea (MA), diabetes, metabolic pathways, oxidative stress
Citation: Ismail Y, Fahmy DM, Ghattas MH, Ahmed MM, Zehry W, Saleh SM and Abo-elmatty DM (2022) Integrating experimental model, LC-MS/MS chemical analysis, and systems biology approach to investigate the possible antidiabetic effect and mechanisms of Matricaria aurea (Golden Chamomile) in type 2 diabetes mellitus. Front. Pharmacol. 13:924478. doi: 10.3389/fphar.2022.924478
Received: 20 April 2022; Accepted: 11 August 2022;
Published: 07 September 2022.
Edited by:
Gokhan Zengin, Selcuk University, TurkeyReviewed by:
Bruno Araujo Serra Pinto, Federal University of Maranhão, BrazilCopyright © 2022 Ismail, Fahmy, Ghattas, Ahmed, Zehry, Saleh and Abo-elmatty. This is an open-access article distributed under the terms of the Creative Commons Attribution License (CC BY). The use, distribution or reproduction in other forums is permitted, provided the original author(s) and the copyright owner(s) are credited and that the original publication in this journal is cited, in accordance with accepted academic practice. No use, distribution or reproduction is permitted which does not comply with these terms.
*Correspondence: Yassin Ismail, eWFzc2luaXNtYWlsMjIyQHBoYXJtLnN1ZXouZWR1LmVn
Disclaimer: All claims expressed in this article are solely those of the authors and do not necessarily represent those of their affiliated organizations, or those of the publisher, the editors and the reviewers. Any product that may be evaluated in this article or claim that may be made by its manufacturer is not guaranteed or endorsed by the publisher.
Research integrity at Frontiers
Learn more about the work of our research integrity team to safeguard the quality of each article we publish.