- Department of Clinical Pharmacy and Toxicology, Leiden University Medical Center, Leiden, Netherlands
Cytochrome P450 3A (CYP3A) subfamily enzymes are involved in the metabolism of 40% of drugs in clinical use. Twin studies have indicated that 66% of the variability in CYP3A4 activity is hereditary. Yet, the complexity of the CYP3A locus and the lack of distinct drug metabolizer phenotypes has limited the identification and clinical application of CYP3A genetic variants compared to other Cytochrome P450 enzymes. In recent years evidence has emerged indicating that a substantial part of the missing heritability is caused by low frequency genetic variation. In this review, we outline the current pharmacogenomics knowledge of CYP3A activity and discuss potential future directions to improve our genetic knowledge and ability to explain CYP3A variability.
Introduction
The Cytochrome P450 CYP3A subgroup forms the largest proportion of CYP protein in the human liver and small intestine (Shimada et al., 1994; Rendic, 2002) and is involved in the metabolism of up to 60% of currently used drugs (Plant, 2007; Zanger et al., 2008). The CYP3A locus is located on Chromosome seven and consists of four distinct genes CYP3A4, CYP3A5, CYP3A7, CYP3A43, and three pseudogenes (Finta and Zaphiropoulos, 2000; Gellner et al., 2001). Notably, the four coding genes share more than 85% of their amino-acid sequence and have a partially overlapping substrate spectrum (Williams et al., 2002). However, the contribution to drug metabolism varies considerably between the CYP3A genes (Ozdemir et al., 2000; Lamba et al., 2002). Of the entire CYP3A enzyme family, the CYP3A4 and CYP3A5 enzymes are the most abundantly expressed proteins accounting for more than 95% of total CYP3A mRNA pool (Koch et al., 2002). CYP3A7, on the other hand, is most prominent in fetal liver cells and slowly diminishes after birth. CYP3A43 expression is almost negligible compared to the other enzymes (<5% of total mRNA).
CYP3A activity is characterized by substantial inter-individual heterogeneity. For instance, CYP3A4, the predominant isoform of CYP3A, shows a 10–100-fold inter-individual variability in enzyme activity (Perera, 2010). One of the causes of the variability in CYP3A activity is the presence of genetic variants in the genes encoding the CYP3A enzymes. The clinical importance of several of these variants has indeed been reported in many patients (Evans and Relling, 1999; Zanger et al., 2008; Zanger and Schwab, 2013). Interestingly, there are currently only two haplotypes that are used in clinical practice, the CYP3A5*3 allele resulting in a complete loss-of-function, and the CYP3A4*22 allele resulting in a decreased enzyme activity (Kuehl et al., 2001; Wang et al., 2011). By combining CYP3A5*3 and CYP3A4*22 more than 60% and 20% of the observed variability in tacrolimus and cyclosporine trough blood level could be explained respectively (Elens et al., 2011). Recommendations on how to apply CYP3A5 genotyping results to optimize drug and dose selection are included in clinical guidelines from the CPIC (Clinical Pharmacogenetic Implementation Consortium) (Birdwell et al., 2015) and for CYP3A4 and CYP3A5 by the DPWG (Dutch Pharmacogenomics Working Group) (Swen et al., 2011) (https://www.knmp.nl/index.php/media/1058).
Besides the genetic variants that form the CYP3A5*3 and CYP3A4*22 alleles, the CYP3A locus contains many more rare variants which are expected to play a role in enzyme activity and drug response. Using a repeated drug administration method Ozdemir et al. compared standard deviations for inter- and intra-person variation in the disposition of 10 different CYP3A4 substrates, including midazolam, and cyclosporine. Analyses of the disposition parameters of these orally administered substrates suggested that at least 60% of the variability in composite CYP3A4 activity is under genetic control (Ozdemir et al., 2000). Furthermore, substantial missing heritability in CYP3A pharmacogenomics has been reported (Klein and Zanger, 2013). A twin study compared the differences of metabolic similarity between monozygotic (MZ) and dizygotic (DZ) twins (Rahmioglu et al., 2011). In this cohort of 367 healthy twins, 66% (confidence interval: 50%–77%) of the induced CYP3A4 activity variation was found to be hereditary, while only ∼20% was explained by current clinical PGx based on a GWAS study (Oetting et al., 2018). In this review, we outline the current pharmacogenetics knowledge of CYP3A activity and discuss potential future directions to improve our genetic knowledge and ability to explain CYP3A variability.
Known CYP3A4 and CYP3A5 Haplotypes
There are 34 defined *-haplotypes for the CYP3A4 and five in the CYP3A5 gene designated by PharmVar (https://www.pharmvar.org/). Recently, three CYP3A5 alleles (CYP3A5*2, *4, and *5) have been reclassified as part of the CYP3A5*3 suballeles (Rodriguez-Antona et al., 2022). Among those haplotypes, the most widely studied genetic variant is the CYP3A5*3 allele (rs776746), characterized by a splice defect in intron 3. This loss-of-function variant generates a premature stop codon that causes a lower amount of functional protein (Kuehl et al., 2001). Notably, CYP3A5*3 allele is common but the allele frequency differs between ethnicities, with the frequency of approximately up to 0.92 in European Americans (EA) and fluctuating between 0.24 and 0.84 in non-European populations according to the PharmGKB frequency table (https://www.pharmgkb.org/page/cyp3a5RefMaterials). A CYP3A5*3/*3 diplotype results in a 10–30 fold lower CYP3A5 expression level compared to CYP3A5*1/*3 (Hustert et al., 2001a; Lin et al., 2002). In clinical practice, individuals are categorized into three distinct CYP3A5 activity groups: CYP3A5 expressors (carrying two *1 alleles), heterozygous expressors (carrying one *1 allele and one *3 allele) and non-expressors (carrying two *3 alleles) of which the last group is the most common. These predicted metabolizer phenotypes can help explain differences in drug metabolism. For example, the dose corrected tacrolimus concentration after transplantation in patients who carried at least one CYP3A5*1 allele was significantly lower compared to CYP3A5 non-expressor after the first month following transplantation (1.49 ± 0.88 vs. 3.11 ± 4.27, p = 0.01), which lasted for the first year post transplantation (Zheng et al., 2004). For this reason, the CYP3A5*3 allele is included in the CPIC, DPWG, RNPGx (French National Network of Pharmacogenetics), and IATDMCT (International Association of Therapeutic Drug Monitoring and Clinical Toxicity) guidelines as being associated with tacrolimus metabolism leading to the recommendation to increase 1.5—2.5 fold initial dosage in *1 carriers (Swen et al., 2011; Birdwell et al., 2015; Picard et al., 2017). In addition, two other key haplotypes CYP3A5*6 (rs10264272, c.624G > A) and CYP3A5*7 (rs41303343, c.1035dup) are included in the guidelines (Birdwell et al., 2015). CYP3A5*6 causes a splicing defect, and CYP3A5*7 results in a frameshift. These two alleles have a frequency of 11%–19% in the African population but have not been observed or with extremely low frequency in non-African populations (https://www.pharmgkb.org/page/cyp3a5RefMaterials). Both haplotypes are associated with no CYP3A5 catalytic activity and contribute to tacrolimus pharmacokinetics variability (Campagne et al., 2018), explaining about 6% of the variability in tacrolimus trough concentrations in African American patients (Oetting et al., 2016).
Besides CYP3A5*3, CYP3A4*22, located in the most predominant isoform of CYP3A, is the second *-allele that is often used in clinical practice. The CYP3A4*22 allele (rs35599367) is characterized by a G > A substitution in intron 6, resulting in an increased formation of a truncated alternative splice variant in vitro (Wang and Sadee, 2016). The allele frequency in the Caucasian population ranges from 3% to −5%, which is higher than compared to other populations, for example less than 1% in the Asian population. Notably, patients carrying CYP3A4*22 had a 1.7 to five fold decreased CYP3A4 mRNA or protein expression level (Elens et al., 2011; Wang et al., 2011; Wang and Sadee, 2016), explaining 12% of CYP3A4 activity variability (Wang et al., 2011). Moreover, the reduced activity caused by the CYP3A4*22 allele was verified with several CYP3A substrates in vivo (Elens et al., 2012; Elens et al., 2013a; de Jonge et al., 2015), and its contribution to variability in CYP3A activity and its potential clinical usage were summarized by Elens et al. and Mulder et al. (Elens et al., 2013b; Mulder et al., 2021). While CYP3A4 is involved in the metabolism of many drugs, there are currently no clinical guidelines available that include CYP3A4 genetic variants. This is potentially explained by the low frequency of the variants in combination with a relatively modest effect on enzyme function which makes it more difficult to ascertain a connection between genotypes and drug metabolism and clinical outcomes.
In addition to these two well-recognized haplotypes, there are 37 additional *-allele haplotypes defined for CYP3A4 and CYP3A5. A recently published review presented a comprehensive summarization of all CYP3A5 *-alleles (Rodriguez-Antona et al., 2022). Here we give an overview of all CYP3A4 *-alleles. Notably, for the CYP3A4, most of *-alleles are characterized by core variants which are rare (Minor allele frequency (MAF) < 1%), or very rare (MAF <0.1%). The only exception is CYP3A4*36, for which the global frequency is up to 0.42 based on the 1,000 Genomes. All CYP3A4 alleles and in vivo evidence of their potential impact on enzyme activity are shown in Table 1. As the impact of these alleles are not assigned in PharmVar, the summarized impact of the alleles is based on available literature with in vivo evidence. CYP3A4*8 was detected with whole-exome sequencing in a patient with a severe paclitaxel-induced peripheral neuropathy due to diminished CYP3A4 enzyme activity (Apellániz-Ruiz et al., 2015b). Notably, the CYP3A4*18 haplotype is associated with a decreased midazolam metabolism but also results in a gain-of-function in the clearance of certain substrates such as sex steroids. These substrate specific effects may be attributed to structural changes in substrate recognition sites that results in catalytic activity variation (Kang et al., 2009). Besides, CYP3A4*1G, which has been redesignated as CYP3A4*36, has been reported a substate-dependent impact on CYP3A4 activity as well (Yuan et al., 2011; Dong et al., 2012; He et al., 2014). Notably, CYP3A4*1G has high linkage disequilibrium with CYP3A5*3 and significantly related with a lncRNA, AC069294.1, that caused down-regulated CYP3A4 and CYP3A5 expression (Collins and Wang, 2022). Furthermore, the interaction of CYP3A4*1G and CYP3A5*3 on drug pharmacokinetics, for instance, tacrolimus (Miura et al., 2011; Zuo et al., 2013) and sirolimus (Zhang et al., 2017) has been reported. For CYP3A4*20, a loss-of -function allele caused by a frameshift variant, it has been suggested that this variant causes an equal functional alteration as CYP3A4*22, given that in CYP3A5 non-expressors the phenotype of a heterozygous CYP3A4*20 carrier was close to that of a CYP3A poor metabolizer (Lloberas et al., 2018). Moreover, CYP3A4*20 presents a higher frequency and founder effect in the Spanish population, which highlights the contribution of rare CYP3A functional alleles in a specific population (Apellániz-Ruiz et al., 2015a). However, given the extremely low frequency of most of those additional *-alleles, there is not enough evidence for their functionality in vivo and for the clinical impact of these *-alleles. Several studies assess activities of these alleles in vitro (Fang et al., 2017; Xu et al., 2018; Li et al., 2019; Lin et al., 2019; Yang et al., 2019; Kumondai et al., 2021). Notably, their predicted function is shown to differ between substrates. For instance, the intrinsic clearance values of CYP3A4*14 and CYP3A4*15 haplotypes were higher compared to wild type for regorafenib (Li et al., 2019) and cabozantinib (Lin et al., 2019). By contrast, intrinsic clearance values were decreased for ibrutinib (Xu et al., 2018). Moreover, Ketoconazole, a CYP3A4 inhibitor alters the function of CYP3A4*14 and CYP3A4*15 in vitro (Lin et al., 2019), which warranted drug-drug interaction plays a significant role in observed enzyme activity. Besides, the variability of enzyme activity caused by some of those *-alleles might be too moderate to be identified, especially when influenced by diverse genetic and non-genetic factors in vivo. As a result, most of the currently reported haplotypes in CYP3A4 and CYP3A5 failed to be taken into account in clinical practice.
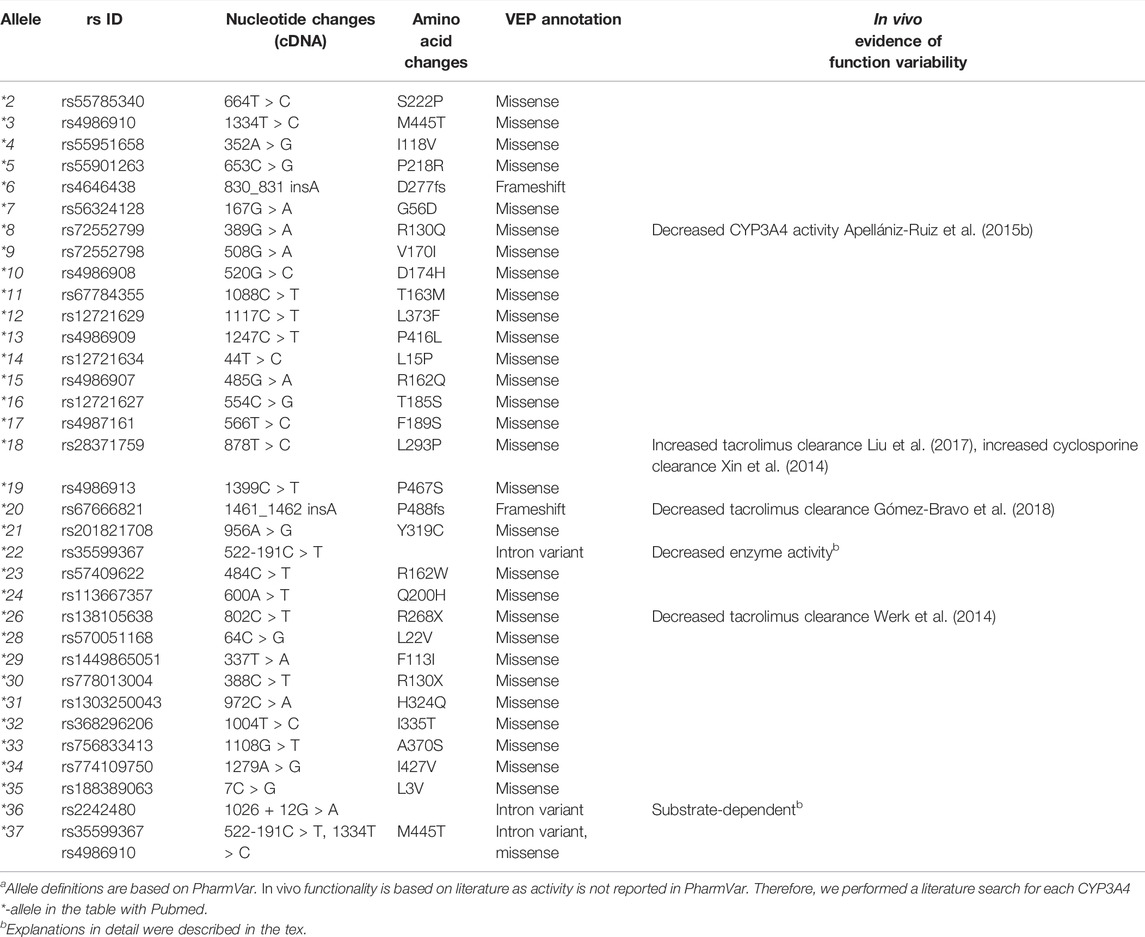
TABLE 1. Overview of core variants of CYP3A4 haplotypea.
Rare Variants in the CYP3A Locus
As mentioned, genetic variants in the CYP3A genes are related to CYP3A enzyme activity and, thereby drug response. However, currently used PGx approaches focusing on the well-known *-alleles, explain only a small proportion of the overall variability in the pharmacokinetics of CYP3A substrates. A recently published twin study revealed that up to 73% of the variability in CYP3A activity is attributed to genetic factors but only a part of this could be explained by PGx (Matthaei et al., 2020). This missing heritability suggests a role of more impactful variants inside and outside the CYP3A locus beyond the currently known and used haplotypes. For instance, a study showed that rare variants in CYP3A4 may account for up to 99% of the functional variability (Kozyra et al., 2017). This proportion is much higher than some other pharmacogenes, in which rare variants contribute to 30%–40% of the variability.
Numerous genetic variants have been detected in the CYP3A locus, as shown in the data from The Genome Aggregation Database (gnomAD) (https://gnomad.broadinstitute.org/). More than 90% of genetic variants in this locus are rare, with a MAF of less than 1% (Table 2). In CYP3A4, a total of 5,082 variants are identified. Of these, only 85 are common (MAF >1%) (Figures 1A,B). All common variants are either located in non-coding regions or are synonymous variants, which do not result in amino acid changes. By contrast, all 285 non-synonymous variants are rare (MAF <1%) of these 218 are missense variants. In addition, there are 32 and 20 splice region variants and frameshift variants, respectively, which is more than the rest of the Variant Effect Predictor (VEP) annotation groups. Finally, there are over four thousand rare intronic variants. For CYP3A5 (Figures 1A,C,D), a total of 6,151 variants are identified, of which 116 are common. Those common variants were mostly intronic or in the 3′untranslated region (3′-UTR) except for four variants which were non-synonymous (Figure 1D; Supplemental Figure S1). Moreover, 199 missense variants were detected in CYP3A5, all of which are rare, which is much more than other variant types. Notably, over 51% of all rare variants in the CPY3A4 and CYP3A5 genes were detected only in non-European populations. On the other hand, around 31% of all rare variants are limited to the European (non-Finnish) population [1576 (31.5%) and 1873 (31.0%) for CYP3A4 and CYP3A5 respectively]. These findings highlight the differences between ethnicities (https://gnomad.broadinstitute.org/).
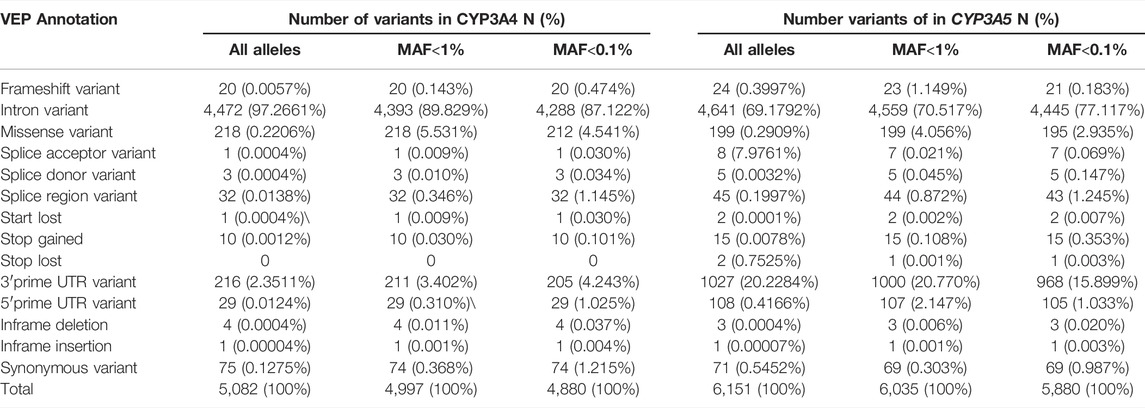
TABLE 2. Summary of CYP3A4 and CYP3A5 allele number classified by VEP (variant effect predictor) annotation.
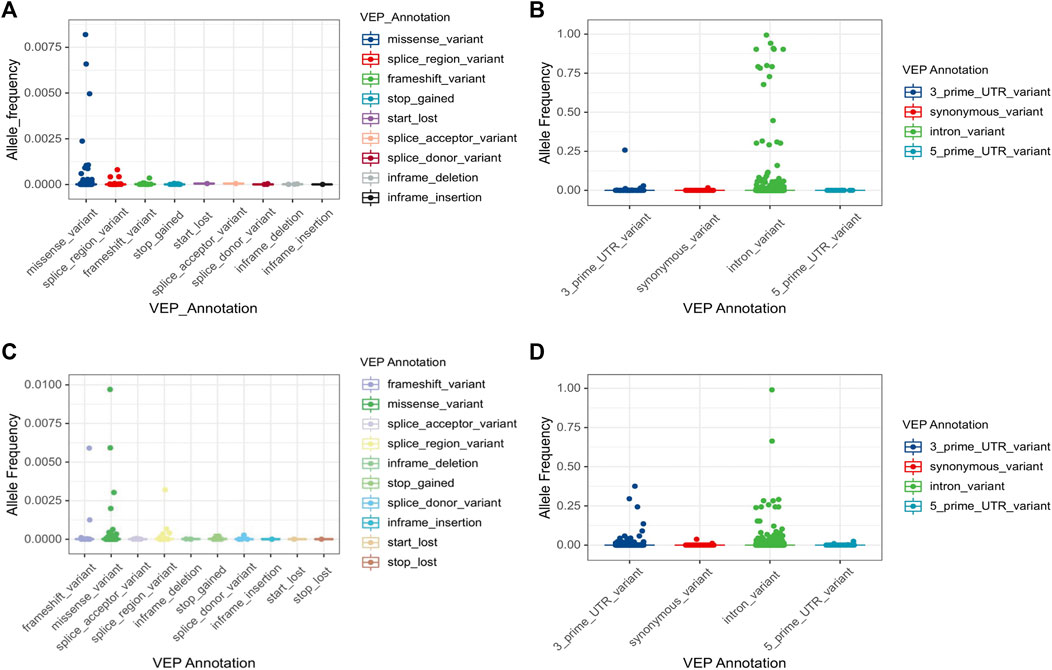
FIGURE 1. Allele frequency of CYP3A4 and CYP3A5 variations by VEP annotation. Total 5,082 variants in CYP3A4 (A,B) and 6,035 variants in CYP3A5 (C,D) are classified by VEP annotation. VEP annotations are separated by the impact on the coding sequence. Four common variants (MAF>1%) in CYP3A5 are marked separately in Supplementary Figure S1. Note that stop lost variants were only reported in CYP3A5. VEP: Variant effect predictor.
For both CYP3A4 and CYP3A5, intronic variants are the most common type of variants, accounting for 97% and 69% of the single nucleotide variants (SNVs), respectively (Table 2). Although an intron is not translated, intronic variants are still of importance as they can influence splicing. For example, the core variant of the CYP3A4*22 allele is a deleterious intronic variant. Furthermore, rs4646450, located in the CYP3A5 intron region, was associated with decreased protein expression and CYP3A4 activity, explaining 3%–5% of the variability (Klein et al., 2012). By contrast, most of common variants do not seem to cause alterations which can influence CYP3A activity. Moreover, a genome-wide associated study failed to identify any common variants related to induced CYP3A4 activity within 310 twins (Rahmioglu et al., 2013). Therefore, in the CYP3A locus, rare variants are the most likely to contribute profoundly to the variability of CYP3A enzyme activity. Notably, the log-transformed distribution of midazolam clearance showed a unimodal pattern (Lin et al., 2001), which suggests that the genetic impact on CYP3A activity could be attributed to a large number of small impact variants rather than only a few high impact ones.
Genetic Factors Outside the CYP3A Locus
While variants within the genes in the CYP3A locus can influence CYP3A enzyme activity, variability in expression can also play a role. Transcriptional regulation of CYP3A expression has been reported in many studies (Yuan et al., 2020). Among those transcriptional factors, two predominant nuclear receptors, pregnane X receptor (PXR, NR1I2) and constitutive androstane receptor (CAR, NR1I3), have been repeatedly associated with altered CYP3A activity (Lolodi et al., 2017). These are ligand-activated nuclear receptors which, after being activated by specific ligands, heterodimerize with the retinoic acid receptor (RXR; NR2B1). Subsequently, they bind to the 5′ regulation region of target genes at the hormone-responsive elements (HREs) motif (Chen et al., 2012; Banerjee and Chen, 2013; Tebbens et al., 2018). Several HREs share the typical nuclear structure, implying the overlapping of downstream genes. But the expression regulation effect varies between target genes (Timsit and Negishi, 2007). Studies have shown that CYP3A isoforms are mainly regulated by PXR (Mbatchi et al., 2018). More functional nuclear receptor SNVs related to CYP3A activity and CYP3A substrate metabolism are summarized in Table 3. Moreover, the interaction between nuclear receptors also plays a role in CYP3A activity variation. Hepatocyte nuclear factor-4α (HNF4α) is a liver-enriched nuclear receptor and is associated with CYP3A4 and CYP3A5 expression (Jover et al., 2001). HNF4α not only regulates CYP3A4 transcription directly by binding to two CYP3A4 5′ upstream regions featured with direct repeat (DR) 1-type motifs (Tegude et al., 2007) but also serves as a coactivator that interacts with the other two CYP3A4 regulators PXR and CAR, resulting in CYP3A4 expression variability. One of the HNF-4α polymorphisms (rs2071197), combined with PXR*1B, was related to the concentration-to-dose (C/D) ratios of carbamazepine, wild type HNF-4α carriers had higher C/D ratios in PXR*1B genotype rather than PXR*1B non-carriers (Saruwatari et al., 2014). Furthermore, the CYP3A4 mRNA expression in pediatric livers could be better predicted with the model, including the expression level of HNF-4α, PXR, CAR, and their heterodimer partner RXRα (Vyhlidal et al., 2006). The interaction of transcriptional factors implies that the expression of CYP3A is the combined effect of multiple transcription factors. More CYP3A transcription regulators were reported recently. For instance, the TSPYL family suppressed the CYP3A4 expression and one SNV in TSPYL1, rs3828743, reversed the suppression effect (Qin et al., 2018). In addition to transcriptional regulation, the CYPP450 oxidoreductase (POR) is reported to influence CYP3A activity by participating in electron transfer to the CYP3A enzyme (Masters, 2005). Indeed several studies showed that a common variant POR*28 (rs1057868) was associated with lower C/D ratios and higher dose requirement of tacrolimus (de Jonge et al., 2011; Suetsugu et al., 2019; Nakamura et al., 2020) and cyclosporin A (Cvetković et al., 2017). In addition, epigenetic regulation of the CYP3A enzymes is an emerging research field (Tang and Chen, 2015). Several mechanisms have been revealed particularly in microRNA (miRNA) involved in transcriptional and post-transcriptional regulation (Wei et al., 2014; Ekström et al., 2015). Notably, miRNA not only targets the 3′-untranslated region (3′UTR) of CYP3A4 directly (Pan et al., 2009) but also impacts the function of CYP3A4 transcriptional factors, for instance, HNF4α (Takagi et al., 2010) and VDR (Pan et al., 2009). More mechanisms of epigenetic regulation and related miRNA have been reviewed but a detailed description is outside the scope of this manuscript (Dluzen and Lazarus, 2015; Lolodi et al., 2017). Overall, while some genetic factors outside the CYP3A locus have been linked to the variability of CYP3A4 expression or CYP3A activity, conflicting data are present and for none of the variants there is sufficient evidence to support clinical application.
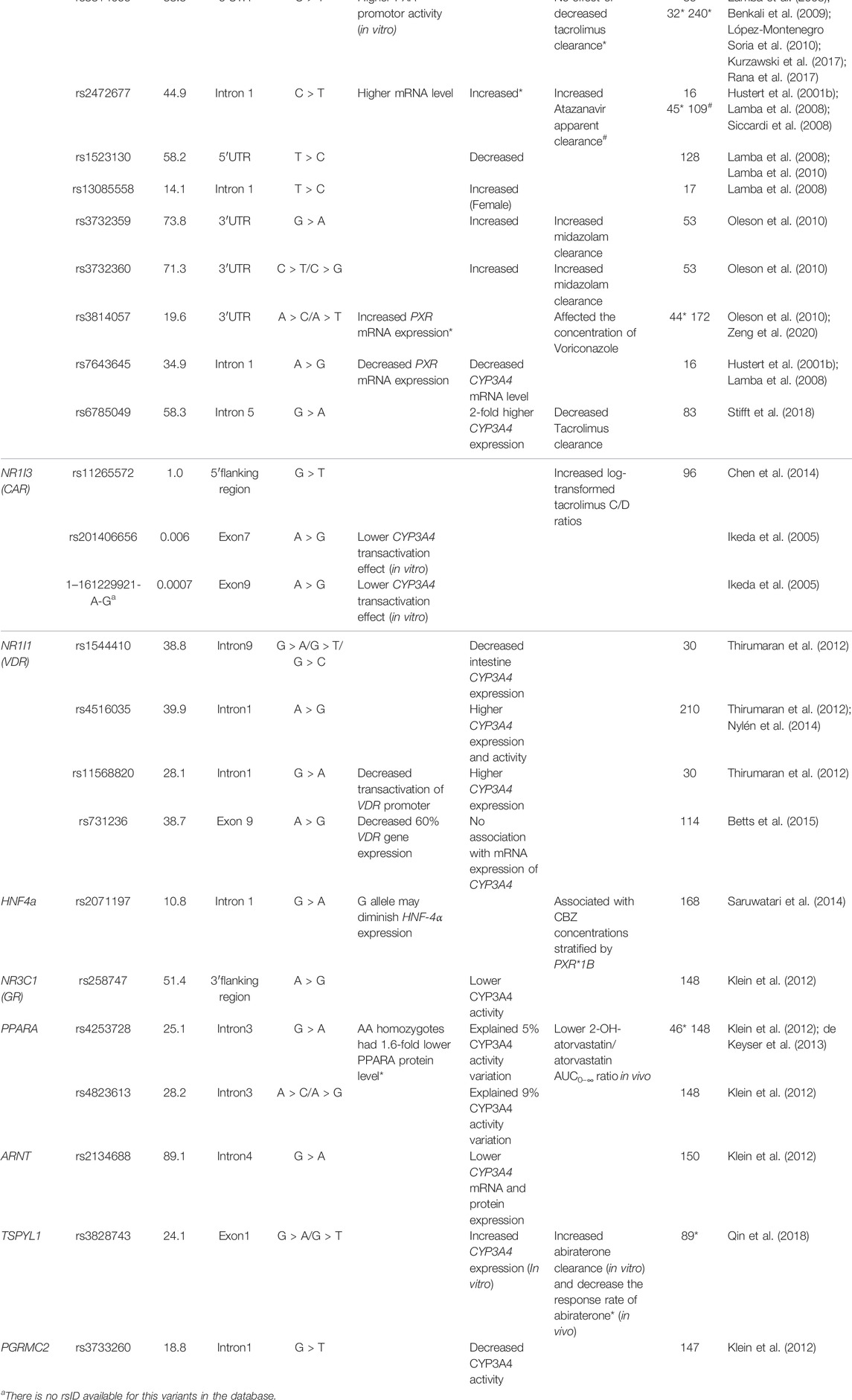
TABLE 3. Summary of single nucleotide variants in CYP3A4 transcriptional factors associated with variability of CYP3A4 activity.
Opportunities for Pharmacogenomics Studies on CYP3A Missing Heritability
Based on a genome-wide association study (GWAS) consisting of 1,446 kidney transplant recipients, 12.5% of tacrolimus trough concentration variability can be explained by CYP3A5*3 variants, including both donor factors and recipient factors. The explained variability increases to 16.9% after taking CYP3A4*22 into account (Oetting et al., 2018). This study combined with the two twin studies mentioned above encourages further exploration of the missing heritability in CYP3A4. And as discussed in this review, many of the variants in the CYP3A locus are of unknown impact and are not included in the clinical *-nomenclature. Moreover, not for all known *-haplotypes the impact is known. This limits the implementation of these variants and haplotypes in clinical practice. As shown in Figure 2, in our opinion, these future developments should focus on a unifying approach that incorporates all (genetic) factors which can influence CYP3A activity. For example, advanced prediction models, e.g., neural network models, which incorporate rare variants, expression regulation and non-genetic contributions, are expecting as a promising strategy for future developments.
However, currently studies in genetic variants detection and interpretation have inevitable limitations. GWAS analysis identified some genetic variants associated with the variability of CYP3A4 activity. However, by design GWAS analyses exclude rare variants and sometimes even low-frequency variants (MAF<5%) for reasons of quality control. As a result, GWAS is incapable to identify rare variants associated with clinical outcomes. Moreover, GWAS analysis generally relies on SNV screening array, which has difficulty detecting variants in complex genes and cannot detect novel variants. However, these types of variants are abundantly present in the CYP3A locus.
The complexity of the CYP3A locus encourages the application of advanced sequencing technologies. First of all, next-generation sequencing (NGS) provides us with almost all of the SNVs in the CYP3A locus. However, NGS generally relies on short reads (100–200 bp), which makes it limited in characterizing complex regions extending that length. Moreover, short-read sequencing still has limitations in exploring the structural variation and providing phasing information directly (Russell and Schwarz, 2020; van der Lee et al., 2020). Besides NGS, long-read sequencing is a promising solution for comprehensive genetic information collection (Ameur et al., 2019). With long-read sequencing, all single-nucleotide variants and most of the structural variants can be detected and those variants can often be phased directly, which is crucial for phenotype prediction (van der Lee et al., 2020). The accuracy and size of phased haploblocks of long-read sequencing seems most prominent in the complex pharmacogenes. This information can also be used to optimize models to predict drug response by taking phasing and full gene sequencing into account. Indeed, recently a continuous scale model based on full gene variants data explored by long-read sequencing and neural network improved the explanation of CYP2D6 activity variability from 54% to 79% compared with the conventional phenotype classification (van der Lee et al., 2021).
Nonetheless, these sequencing technologies come with higher costs and significantly more data to analyze, resulting in consideration of cost-benefit balance and the challenging of novel variant interpretation. To assess the individual impact of a large number of low frequency variants, and in the absence of in vivo data, several in silico tools, including SIFT, PolyPhen-2, and CADD have been developed. However, the predicted effect of variants differed among in silico tools (Gulilat et al., 2019). Moreover, compared with in vitro models, the accuracy of those in silico prediction only reaches up to 80% for pharmacogenetic purposes (Han et al., 2017). SIFT, PolyPhen-2 show a higher false-negative rate in predicting gain-of-function variants compared to loss-of-function (Flanagan et al., 2010). Remarkably, neural network models have been developed to predict the unknown function *-alleles in CYP2D6. This model explains 47.5% of unknown function variants in *-alleles with 88% accuracy (McInnes et al., 2020), which encouraged the further implementation of neural network based approaches. By contrast, in vitro models provide us a better understanding of the effects of variants, especially for rare variants (Kumondai et al., 2021). However, it is a costly and time-consuming process to establish in vitro models for each individual CYP3A variant, which can probably never be realized and hampers their clinical implementation. Furthermore, by-design an in vitro system is not useful to assess the effect of variants located in non-coding regions, which occur frequently in the CYP3A locus.
Given the broad substrate spectrum of drugs affected by the CYP3A enzymes and the presence of substrate-specific effects, functional prediction based on in vitro data with only a limited number of substrates are controversial. Therefore, in vivo evidence is considered the gold-standard to establish if variants have a significant effect on enzyme activity, especially for non-coding and synonymous SNVs. For novel missense SNVs, drug-related clinical information is highly recommended. However, particularly for the frequently occurring low-frequency non-synonymous variants in the CYP3A locus, this evidence is hard to generate. Indeed, quite a few studies failed to detect functional variants or identify their associations with clinical outcomes (Belmonte et al., 2018; Riera et al., 2018). Moreover, lacking of clinical significance prevent their further study only based on in vitro evidence.
Conventional drug metabolizer phenotypes distinguish three or four metabolizer phenotype groups based on a limited number of variants. This classification assumes a fixed effect of each variant, where the predicted phenotype depends on the combined effects of those several well-known variants. This same method is used for almost all CYP-enzymes with in the end only five phenotype categories (poor-, intermediate-, normal-, rapid- and ultra-rapid metabolizers) (Caudle et al., 2017). However, it has been demonstrated that enzyme activity is not categorical but continuous. As a result, the conventional variant to metabolizer phenotype interpretation which only includes a limited number of well-known variants with fixed effects inevitably leads to missing information. Previous studies have shown that, at least for CYP2D6, a continuous model is able to better explain the enzyme activity (McInnes et al., 2020; van der Lee et al., 2021). It can be expected that the same principle holds true for CYP3A as well. Furthermore, in the CYP3A locus more than one coding isoforms with considerable overlapped substrate specificity contributes to the overall CYP3Aenzyme activity, complicating the predictions even more. As mentioned previously, CYP3A mediated clearance shows a unimodal distribution which does not fit the current categorical phenotype system. In the current system, the function of variants that cause slight or moderate CYP3A activity variability could be obscured by variants that have predominant effects, which further complicates the analysis of the contribution of every variant in every CYP3A gene. To deal with the complexity of the CYP3A locus, the use of artificial intelligence (AI) is an opportunity. AI models could include all variants detected to predict both variant effect as well as overall enzyme activity without the use of the categorical models (Zou et al., 2019).
Besides genetic variation, environmental factors also contribute to variability in CYP3A activity. For instance, 20% of induced CYP3A4 activity variation was attributed to BMI, alcohol use, and smoking (Rahmioglu et al., 2011). Also, inflammation has been shown to affect CYP3A activity through modulation of the expression (Vet et al., 2016). Moreover, some clinical variables, including transplant recipient age, glomerular filtration rate, anti-cytomegalovirus drug use, simultaneous pancreas-kidney transplant and antibody induction, account for 19.8% of the variability in tacrolimus trough concentrations (Oetting et al., 2016). Finally, CYP3A inducers and inhibitors have been shown to cause a ∼400-fold fluctuation in CYP3A activity and drug clearance (Hohmann et al., 2016). Zanger et al. summarized these and other non-genetic factors which are beyond the scope of this review (Zanger and Schwab, 2013). In summary, genetic variability in the CYP3A locus has been consistently linked with the inter-individual variability of drug metabolism and differences in drug response. Despite indications for clinical relevance, only a limited number of variants are included in CYP3A metabolizer phenotype prediction and clinical practice. The abundance of rare variants in the CYP3A locus and possible multi-genic regulations of CYP3A expression combined with the rapid technological advances in sequencing technologies present an opportunity for future investigations and offer a potential explanation for the observed missing heritability.
Author Contributions
Writing—original draft preparation, QZ and ML; Writing—review and editing, JS and TG. All authors have read and agreed to the published version of the manuscript.
Conflict of Interest
The authors declare that the research was conducted in the absence of any commercial or financial relationships that could be construed as a potential conflict of interest.
Publisher’s Note
All claims expressed in this article are solely those of the authors and do not necessarily represent those of their affiliated organizations, or those of the publisher, the editors and the reviewers. Any product that may be evaluated in this article, or claim that may be made by its manufacturer, is not guaranteed or endorsed by the publisher.
Supplementary Material
The Supplementary Material for this article can be found online at: https://www.frontiersin.org/articles/10.3389/fphar.2022.912618/full#supplementary-material
Supplementary Figure S1 | The allele frequency of four common CYP3A5 variants with potential impact VEP annotation.3.
References
Ameur, A., Kloosterman, W. P., and Hestand, M. S. (2019). Single-Molecule Sequencing: Towards Clinical Applications. Trends Biotechnol. 37 (1), 72–85. doi:10.1016/j.tibtech.2018.07.013
Apellániz-Ruiz, M., Inglada-Pérez, L., Naranjo, M. E., Sánchez, L., Mancikova, V., Currás-Freixes, M., et al. (2015a). High Frequency and Founder Effect of the CYP3A4*20 Loss-Of-Function Allele in the Spanish Population Classifies CYP3A4 as a Polymorphic Enzyme. Pharmacogenomics J. 15 (3), 288–292. doi:10.1038/tpj.2014.67
Apellániz-Ruiz, M., Lee, M. Y., Sánchez-Barroso, L., Gutiérrez-Gutiérrez, G., Calvo, I., García-Estévez, L., et al. (2015b). Whole-exome Sequencing Reveals Defective CYP3A4 Variants Predictive of Paclitaxel Dose-Limiting Neuropathy. Clin. Cancer Res. 21 (2), 322–328. doi:10.1158/1078-0432.Ccr-14-1758
Banerjee, M., and Chen, T. (2013). Differential Regulation of CYP3A4 Promoter Activity by a New Class of Natural Product Derivatives Binding to Pregnane X Receptor. Biochem. Pharmacol. 86 (6), 824–835. doi:10.1016/j.bcp.2013.07.023
Belmonte, C., Ochoa, D., Román, M., Saiz-Rodríguez, M., Wojnicz, A., Gómez-Sánchez, C. I., et al. (2018). Influence of CYP2D6, CYP3A4, CYP3A5 and ABCB1 Polymorphisms on Pharmacokinetics and Safety of Aripiprazole in Healthy Volunteers. Basic Clin. Pharmacol. Toxicol. 122 (6), 596–605. doi:10.1111/bcpt.12960
Benkali, K., Prémaud, A., Picard, N., Rérolle, J. P., Toupance, O., Hoizey, G., et al. (2009). Tacrolimus Population Pharmacokinetic-Pharmacogenetic Analysis and Bayesian Estimation in Renal Transplant Recipients. Clin. Pharmacokinet. 48 (12), 805–816. doi:10.2165/11318080-000000000-00000
Betts, S., Björkhem-Bergman, L., Rane, A., and Ekström, L. (2015). Expression of CYP3A4 and CYP3A7 in Human Foetal Tissues and its Correlation with Nuclear Receptors. Basic Clin. Pharmacol. Toxicol. 117 (4), 261–266. doi:10.1111/bcpt.12392
Birdwell, K. A., Decker, B., Barbarino, J. M., Peterson, J. F., Stein, C. M., Sadee, W., et al. (2015). Clinical Pharmacogenetics Implementation Consortium (CPIC) Guidelines for CYP3A5 Genotype and Tacrolimus Dosing. Clin. Pharmacol. Ther. 98 (1), 19–24. doi:10.1002/cpt.113
Campagne, O., Mager, D. E., Brazeau, D., Venuto, R. C., and Tornatore, K. M. (2018). Tacrolimus Population Pharmacokinetics and Multiple CYP3A5 Genotypes in Black and White Renal Transplant Recipients. J. Clin. Pharmacol. 58 (9), 1184–1195. doi:10.1002/jcph.1118
Caudle, K. E., Dunnenberger, H. M., Freimuth, R. R., Peterson, J. F., Burlison, J. D., Whirl-Carrillo, M., et al. (2017). Standardizing Terms for Clinical Pharmacogenetic Test Results: Consensus Terms from the Clinical Pharmacogenetics Implementation Consortium (CPIC). Genet. Med. 19 (2), 215–223. doi:10.1038/gim.2016.87
Chen, D., Guo, F., Shi, J., Zhang, C., Wang, Z., Fan, J., et al. (2014). Association of Hemoglobin Levels, CYP3A5, and NR1I3 Gene Polymorphisms with Tacrolimus Pharmacokinetics in Liver Transplant Patients. Drug Metab. Pharmacokinet. 29 (3), 249–253. doi:10.2133/dmpk.dmpk-13-rg-095
Chen, Y., Tang, Y., Guo, C., Wang, J., Boral, D., and Nie, D. (2012). Nuclear Receptors in the Multidrug Resistance through the Regulation of Drug-Metabolizing Enzymes and Drug Transporters. Biochem. Pharmacol. 83 (8), 1112–1126. doi:10.1016/j.bcp.2012.01.030
Collins, J. M., and Wang, D. (2022). Regulation of CYP3A4 and CYP3A5 by a lncRNA: a Potential Underlying Mechanism Explaining the Association between CYP3A4*1G and CYP3A Metabolism. Pharmacogenet Genomics 32 (1), 16–23. doi:10.1097/fpc.0000000000000447
Cvetković, M., Zivković, M., Bundalo, M., Gojković, I., Spasojević-Dimitrijeva, B., Stanković, A., et al. (2017). Effect of Age and Allele Variants of CYP3A5, CYP3A4, and POR Genes on the Pharmacokinetics of Cyclosporin A in Pediatric Renal Transplant Recipients from Serbia. Ther. Drug Monit. 39 (6), 589–595. doi:10.1097/ftd.0000000000000442
de Jonge, H., Elens, L., de Loor, H., van Schaik, R. H., and Kuypers, D. R. (2015). The CYP3A4*22 C>T Single Nucleotide Polymorphism Is Associated with Reduced Midazolam and Tacrolimus Clearance in Stable Renal Allograft Recipients. Pharmacogenomics J. 15 (2), 144–152. doi:10.1038/tpj.2014.49
de Jonge, H., Naesens, C., Lambrechts, M., Kuypers, D., and au, D. R. (2011). The P450 Oxidoreductase *28 SNP Is Associated with Low Initial Tacrolimus Exposure and Increased Dose Requirements in CYP3A5-Expressing Renal Recipients. Pharmacogenomics 12 (9), 1281–1291. doi:10.2217/pgs.11.77
de Keyser, C. E., Becker, M. L., Uitterlinden, A. G., Hofman, A., Lous, J. J., Elens, L., et al. (2013). Genetic Variation in the PPARA Gene Is Associated with Simvastatin-Mediated Cholesterol Reduction in the Rotterdam Study. Pharmacogenomics 14 (11), 1295–1304. doi:10.2217/pgs.13.112
Dluzen, D. F., and Lazarus, P. (2015). MicroRNA Regulation of the Major Drug-Metabolizing Enzymes and Related Transcription Factors. Drug Metab. Rev. 47 (3), 320–334. doi:10.3109/03602532.2015.1076438
Dong, Z. L., Li, H., Chen, Q. X., Hu, Y., Wu, S. J., Tang, L. Y., et al. (2012). Effect of CYP3A4*1G on the Fentanyl Consumption for Intravenous Patient-Controlled Analgesia after Total Abdominal Hysterectomy in Chinese Han Population. J. Clin. Pharm. Ther. 37 (2), 153–156. doi:10.1111/j.1365-2710.2011.01268.x
Du, Q. Q., Wang, Z. J., He, L., Jiang, X. H., and Wang, L. (2013). PXR Polymorphisms and Their Impact on Pharmacokinetics/pharmacodynamics of Repaglinide in Healthy Chinese Volunteers. Eur. J. Clin. Pharmacol. 69 (11), 1917–1925. doi:10.1007/s00228-013-1552-2
Ekström, L., Skilving, I., Ovesjö, M. L., Aklillu, E., Nylén, H., Rane, A., et al. (2015). miRNA-27b Levels Are Associated with CYP3A Activity In Vitro and In Vivo. Pharmacol. Res. Perspect. 3 (6), e00192. doi:10.1002/prp2.192
Elens, L., Bouamar, R., Hesselink, D. A., Haufroid, V., van Gelder, T., and van Schaik, R. H. (2012). The New CYP3A4 Intron 6 C>T Polymorphism (CYP3A4*22) Is Associated with an Increased Risk of Delayed Graft Function and Worse Renal Function in Cyclosporine-Treated Kidney Transplant Patients. Pharmacogenet Genomics 22 (5), 373–380. doi:10.1097/FPC.0b013e328351f3c1
Elens, L., Nieuweboer, A., Clarke, S. J., Charles, K. A., de Graan, A. J., Haufroid, V., et al. (2013a). CYP3A4 Intron 6 C>T SNP (CYP3A4*22) Encodes Lower CYP3A4 Activity in Cancer Patients, as Measured with Probes Midazolam and Erythromycin. Pharmacogenomics 14 (2), 137–149. doi:10.2217/pgs.12.202
Elens, L., van Gelder, T., Hesselink, D. A., Haufroid, V., and van Schaik, R. H. (2013b). CYP3A4*22: Promising Newly Identified CYP3A4 Variant Allele for Personalizing Pharmacotherapy. Pharmacogenomics 14 (1), 47–62. doi:10.2217/pgs.12.187
Elens, L., van Schaik, R. H., Panin, N., de Meyer, M., Wallemacq, P., Lison, D., et al. (2011). Effect of a New Functional CYP3A4 Polymorphism on Calcineurin Inhibitors' Dose Requirements and Trough Blood Levels in Stable Renal Transplant Patients. Pharmacogenomics 12 (10), 1383–1396. doi:10.2217/pgs.11.90
Evans, W. E., and Relling, M. V. (1999). Pharmacogenomics: Translating Functional Genomics into Rational Therapeutics. Science 286 (5439), 487–491. doi:10.1126/science.286.5439.487
Fang, P., Tang, P. F., Xu, R. A., Zheng, X., Wen, J., Bao, S. S., et al. (2017). Functional Assessment of CYP3A4 Allelic Variants on Lidocaine Metabolism In Vitro. Drug Des. Devel Ther. 11, 3503–3510. doi:10.2147/dddt.S152366
Finta, C., and Zaphiropoulos, P. G. (2000). The Human Cytochrome P450 3A Locus. Gene Evolution by Capture of Downstream Exons. Gene 260 (1-2), 13–23. doi:10.1016/s0378-1119(00)00470-4
Flanagan, S. E., Patch, A. M., and Ellard, S. (2010). Using SIFT and PolyPhen to Predict Loss-Of-Function and Gain-Of-Function Mutations. Genet. Test. Mol. Biomarkers 14 (4), 533–537. doi:10.1089/gtmb.2010.0036
Gellner, K., Eiselt, R., Hustert, E., Arnold, H., Koch, I., Haberl, M., et al. (2001). Genomic Organization of the Human CYP3A Locus: Identification of a New, Inducible CYP3A Gene. Pharmacogenetics 11 (2), 111–121. doi:10.1097/00008571-200103000-00002
Gómez-Bravo, M. A., Apellaniz-Ruiz, M., Salcedo, M., Fondevila, C., Suarez, F., Castellote, J., et al. (2018). Influence of Donor Liver CYP3A4*20 Loss-Of-Function Genotype on Tacrolimus Pharmacokinetics in Transplanted Patients. Pharmacogenet Genomics 28 (2), 41–48. doi:10.1097/fpc.0000000000000321
Gulilat, M., Lamb, T., Teft, W. A., Wang, J., Dron, J. S., Robinson, J. F., et al. (2019). Targeted Next Generation Sequencing as a Tool for Precision Medicine. BMC Med. Genomics 12 (1), 81. doi:10.1186/s12920-019-0527-2
Han, S. M., Park, J., Lee, J. H., Lee, S. S., Kim, H., Han, H., et al. (2017). Targeted Next-Generation Sequencing for Comprehensive Genetic Profiling of Pharmacogenes. Clin. Pharmacol. Ther. 101 (3), 396–405. doi:10.1002/cpt.532
He, B. X., Shi, L., Qiu, J., Zeng, X. H., and Zhao, S. J. (2014). The Effect of CYP3A4*1G Allele on the Pharmacokinetics of Atorvastatin in Chinese Han Patients with Coronary Heart Disease. J. Clin. Pharmacol. 54 (4), 462–467. doi:10.1002/jcph.229
Hohmann, N., Haefeli, W. E., and Mikus, G. (2016). CYP3A Activity: towards Dose Adaptation to the Individual. Expert Opin. Drug Metab. Toxicol. 12 (5), 479–497. doi:10.1517/17425255.2016.1163337
Hustert, E., Haberl, M., Burk, O., Wolbold, R., He, Y. Q., Klein, K., et al. (2001a). The Genetic Determinants of the CYP3A5 Polymorphism. Pharmacogenetics 11 (9), 773–779. doi:10.1097/00008571-200112000-00005
Hustert, E., Zibat, A., Presecan-Siedel, E., Eiselt, R., Mueller, R., Fuss, C., et al. (2001b). Natural Protein Variants of Pregnane X Receptor with Altered Transactivation Activity toward CYP3A4. Drug Metab. Dispos. 29 (11), 1454–1459.
Ikeda, S., Kurose, K., Jinno, H., Sai, K., Ozawa, S., Hasegawa, R., et al. (2005). Functional Analysis of Four Naturally Occurring Variants of Human Constitutive Androstane Receptor. Mol. Genet. Metab. 86 (1-2), 314–319. doi:10.1016/j.ymgme.2005.05.011
Jover, R., Bort, R., Gómez-Lechón, M. J., and Castell, J. V. (2001). Cytochrome P450 Regulation by Hepatocyte Nuclear Factor 4 in Human Hepatocytes: a Study Using Adenovirus-Mediated Antisense Targeting. Hepatology 33 (3), 668–675. doi:10.1053/jhep.2001.22176
Kang, Y. S., Park, S. Y., Yim, C. H., Kwak, H. S., Gajendrarao, P., Krishnamoorthy, N., et al. (2009). The CYP3A4*18 Genotype in the Cytochrome P450 3A4 Gene, a Rapid Metabolizer of Sex Steroids, Is Associated with Low Bone Mineral Density. Clin. Pharmacol. Ther. 85 (3), 312–318. doi:10.1038/clpt.2008.215
King, C. R., Xiao, M., Yu, J., Minton, M. R., Addleman, N. J., Van Booven, D. J., et al. (2007). Identification of NR1I2 Genetic Variation Using Resequencing. Eur. J. Clin. Pharmacol. 63 (6), 547–554. doi:10.1007/s00228-007-0295-3
Klein, K., Thomas, M., Winter, S., Nussler, A. K., Niemi, M., Schwab, M., et al. (2012). PPARA: a Novel Genetic Determinant of CYP3A4 In Vitro and In Vivo. Clin. Pharmacol. Ther. 91 (6), 1044–1052. doi:10.1038/clpt.2011.336
Klein, K., and Zanger, U. M. (2013). Pharmacogenomics of Cytochrome P450 3A4: Recent Progress toward the "Missing Heritability" Problem. Front. Genet. 4, 12. doi:10.3389/fgene.2013.00012
Koch, I., Weil, R., Wolbold, R., Brockmöller, J., Hustert, E., Burk, O., et al. (2002). Interindividual Variability and Tissue-Specificity in the Expression of Cytochrome P450 3A mRNA. Drug Metab. Dispos. 30 (10), 1108–1114. doi:10.1124/dmd.30.10.1108
Kozyra, M., Ingelman-Sundberg, M., and Lauschke, V. M. (2017). Rare Genetic Variants in Cellular Transporters, Metabolic Enzymes, and Nuclear Receptors Can Be Important Determinants of Interindividual Differences in Drug Response. Genet. Med. 19 (1), 20–29. doi:10.1038/gim.2016.33
Kuehl, P., Zhang, J., Lin, Y., Lamba, J., Assem, M., Schuetz, J., et al. (2001). Sequence Diversity in CYP3A Promoters and Characterization of the Genetic Basis of Polymorphic CYP3A5 Expression. Nat. Genet. 27 (4), 383–391. doi:10.1038/86882
Kumondai, M., Gutiérrez Rico, E. M., Hishinuma, E., Ueda, A., Saito, S., Saigusa, D., et al. (2021). Functional Characterization of 40 CYP3A4 Variants by Assessing Midazolam 1'-Hydroxylation and Testosterone 6β-Hydroxylation. Drug Metab. Dispos. 49 (3), 212–220. doi:10.1124/dmd.120.000261
Kurzawski, M., Malinowski, D., Dziewanowski, K., and Droździk, M. (2017). Analysis of Common Polymorphisms within NR1I2 and NR1I3 Genes and Tacrolimus Dose-Adjusted Concentration in Stable Kidney Transplant Recipients. Pharmacogenet Genomics 27 (10), 372–377. doi:10.1097/fpc.0000000000000301
Lamba, J., Lamba, V., Strom, S., Venkataramanan, R., and Schuetz, E. (2008). Novel Single Nucleotide Polymorphisms in the Promoter and Intron 1 of Human Pregnane X receptor/NR1I2 and Their Association with CYP3A4 Expression. Drug Metab. Dispos. 36 (1), 169–181. doi:10.1124/dmd.107.016600
Lamba, J. K., Lin, Y. S., Schuetz, E. G., and Thummel, K. E. (2002). Genetic Contribution to Variable Human CYP3A-Mediated Metabolism. Adv. Drug Deliv. Rev. 54 (10), 1271–1294. doi:10.1016/s0169-409x(02)00066-2
Lamba, V., Panetta, J. C., Strom, S., and Schuetz, E. G. (2010). Genetic Predictors of Interindividual Variability in Hepatic CYP3A4 Expression. J. Pharmacol. Exp. Ther. 332 (3), 1088–1099. doi:10.1124/jpet.109.160804
Li, Y. H., Lin, Q. M., Pang, N. H., Zhang, X. D., Huang, H. L., Cai, J. P., et al. (2019). Functional Characterization of 27 CYP3A4 Protein Variants to Metabolize Regorafenib In Vitro. Basic Clin. Pharmacol. Toxicol. 125 (4), 337–344. doi:10.1111/bcpt.13246
Lin, Q. M., Li, Y. H., Lu, X. R., Wang, R., Pang, N. H., Xu, R. A., et al. (2019). Characterization of Genetic Variation in CYP3A4 on the Metabolism of Cabozantinib In Vitro. Chem. Res. Toxicol. 32 (8), 1583–1590. doi:10.1021/acs.chemrestox.9b00100
Lin, Y. S., Dowling, A. L., Quigley, S. D., Farin, F. M., Zhang, J., Lamba, J., et al. (2002). Co-regulation of CYP3A4 and CYP3A5 and Contribution to Hepatic and Intestinal Midazolam Metabolism. Mol. Pharmacol. 62 (1), 162–172. doi:10.1124/mol.62.1.162
Lin, Y. S., Lockwood, G. F., Graham, M. A., Brian, W. R., Loi, C. M., Dobrinska, M. R., et al. (2001). In-vivo Phenotyping for CYP3A by a Single-point Determination of Midazolam Plasma Concentration. Pharmacogenetics 11 (9), 781–791. doi:10.1097/00008571-200112000-00006
Liu, F., Ou, Y. M., Yu, A. R., Xiong, L., and Xin, H. W. (2017). Long-Term Influence of CYP3A5, CYP3A4, ABCB1, and NR1I2 Polymorphisms on Tacrolimus Concentration in Chinese Renal Transplant Recipients. Genet. Test. Mol. Biomarkers 21 (11), 663–673. doi:10.1089/gtmb.2017.0088
Lloberas, N., Hesselink, D. A., van Schaik, R. H., Grinyò, J. M., Colom, H., Gelder, T. V., et al. (2018). Detection of a Rare CYP3A4 Variant in a Transplant Patient Characterized by a Tacrolimus Poor Metabolizer Phenotype. Pharmacogenomics 19 (4), 305–310. doi:10.2217/pgs-2017-0301
Lolodi, O., Wang, Y. M., Wright, W. C., and Chen, T. (2017). Differential Regulation of CYP3A4 and CYP3A5 and its Implication in Drug Discovery. Curr. Drug Metab. 18 (12), 1095–1105. doi:10.2174/1389200218666170531112038
López-Montenegro Soria, M. A., Kanter Berga, J., Beltrán Catalán, S., Milara Payá, J., Pallardó Mateu, L. M., and Jiménez Torres, N. V. (2010). Genetic Polymorphisms and Individualized Tacrolimus Dosing. Transpl. Proc. 42 (8), 3031–3033. doi:10.1016/j.transproceed.2010.08.001
Masters, B. S. (2005). The Journey from NADPH-Cytochrome P450 Oxidoreductase to Nitric Oxide Synthases. Biochem. Biophys. Res. Commun. 338 (1), 507–519. doi:10.1016/j.bbrc.2005.09.165
Matthaei, J., Bonat, W. H., Kerb, R., Tzvetkov, M. V., Strube, J., Brunke, S., et al. (2020). Inherited and Acquired Determinants of Hepatic CYP3A Activity in Humans. Front. Genet. 11, 944. doi:10.3389/fgene.2020.00944
Mbatchi, L. C., Brouillet, J. P., and Evrard, A. (2018). Genetic Variations of the Xenoreceptors NR1I2 and NR1I3 and Their Effect on Drug Disposition and Response Variability. Pharmacogenomics 19 (1), 61–77. doi:10.2217/pgs-2017-0121
McInnes, G., Dalton, R., Sangkuhl, K., Whirl-Carrillo, M., Lee, S. B., Tsao, P. S., et al. (2020). Transfer Learning Enables Prediction of CYP2D6 Haplotype Function. PLoS Comput. Biol. 16 (11), e1008399. doi:10.1371/journal.pcbi.1008399
Miura, M., Satoh, S., Kagaya, H., Saito, M., Numakura, K., Tsuchiya, N., et al. (2011). Impact of the CYP3A4*1G Polymorphism and its Combination with CYP3A5 Genotypes on Tacrolimus Pharmacokinetics in Renal Transplant Patients. Pharmacogenomics 12 (7), 977–984. doi:10.2217/pgs.11.33
Moes, D. J., Press, R. R., den Hartigh, J., van der Straaten, T., de Fijter, J. W., and Guchelaar, H. J. (2012). Population Pharmacokinetics and Pharmacogenetics of Everolimus in Renal Transplant Patients. Clin. Pharmacokinet. 51 (7), 467–480. doi:10.2165/11599710-000000000-00000
Mulder, T. A. M., van Eerden, R. A. G., de With, M., Elens, L., Hesselink, D. A., Matic, M., et al. (2021). CYP3A4∗22 Genotyping in Clinical Practice: Ready for Implementation? Front. Genet. 12, 711943. doi:10.3389/fgene.2021.711943
Nakamura, T., Fukuda, M., Matsukane, R., Suetsugu, K., Harada, N., Yoshizumi, T., et al. (2020). Influence of POR*28 Polymorphisms on CYP3A5*3-Associated Variations in Tacrolimus Blood Levels at an Early Stage after Liver Transplantation. Int. J. Mol. Sci. 21 (7), 2287. doi:10.3390/ijms21072287
Nylén, H., Björkhem-Bergman, L., Ekström, L., Roh, H. K., Bertilsson, L., Eliasson, E., et al. (2014). Plasma Levels of 25-hydroxyvitamin D3 and In Vivo Markers of Cytochrome P450 3A Activity in Swedes and Koreans: Effects of a Genetic Polymorphism and Oral Contraceptives. Basic Clin. Pharmacol. Toxicol. 115 (4), 366–371. doi:10.1111/bcpt.12230
Oetting, W. S., Schladt, D. P., Guan, W., Miller, M. B., Remmel, R. P., Dorr, C., et al. (2016). Genomewide Association Study of Tacrolimus Concentrations in African American Kidney Transplant Recipients Identifies Multiple CYP3A5 Alleles. Am. J. Transpl. 16 (2), 574–582. doi:10.1111/ajt.13495
Oetting, W. S., Wu, B., Schladt, D. P., Guan, W., Remmel, R. P., Mannon, R. B., et al. (2018). Genome-wide Association Study Identifies the Common Variants in CYP3A4 and CYP3A5 Responsible for Variation in Tacrolimus Trough Concentration in Caucasian Kidney Transplant Recipients. Pharmacogenomics J. 18 (3), 501–505. doi:10.1038/tpj.2017.49
Oleson, L., von Moltke, L. L., Greenblatt, D. J., and Court, M. H. (2010). Identification of Polymorphisms in the 3'-untranslated Region of the Human Pregnane X Receptor (PXR) Gene Associated with Variability in Cytochrome P450 3A (CYP3A) Metabolism. Xenobiotica 40 (2), 146–162. doi:10.3109/00498250903420243
Ozdemir, V., Kalow, W., Tang, B. K., Paterson, A. D., Walker, S. E., Endrenyi, L., et al. (2000). Evaluation of the Genetic Component of Variability in CYP3A4 Activity: a Repeated Drug Administration Method. Pharmacogenetics 10 (5), 373–388. doi:10.1097/00008571-200007000-00001
Pan, Y. Z., Gao, W., and Yu, A. M. (2009). MicroRNAs Regulate CYP3A4 Expression via Direct and Indirect Targeting. Drug Metab. Dispos. 37 (10), 2112–2117. doi:10.1124/dmd.109.027680
Perera, M. A. (2010). The Missing Linkage: what Pharmacogenetic Associations Are Left to Find in CYP3A? Expert Opin. Drug Metab. Toxicol. 6 (1), 17–28. doi:10.1517/17425250903379546
Picard, N., Boyer, J. C., Etienne-Grimaldi, M. C., Barin-Le Guellec, C., Thomas, F., and Loriot, M. A. (2017). Pharmacogenetics-based Personalized Therapy: Levels of Evidence and Recommendations from the French Network of Pharmacogenetics (RNPGx). Therapie 72 (2), 185–192. doi:10.1016/j.therap.2016.09.014
Plant, N. (2007). The Human Cytochrome P450 Sub-family: Transcriptional Regulation, Inter-individual Variation and Interaction Networks. Biochim. Biophys. Acta 1770 (3), 478–488. doi:10.1016/j.bbagen.2006.09.024
Qin, S., Liu, D., Kohli, M., Wang, L., Vedell, P. T., Hillman, D. W., et al. (2018). TSPYL Family Regulates CYP17A1 and CYP3A4 Expression: Potential Mechanism Contributing to Abiraterone Response in Metastatic Castration-Resistant Prostate Cancer. Clin. Pharmacol. Ther. 104 (1), 201–210. doi:10.1002/cpt.907
Rahmioglu, N., Heaton, J., Clement, G., Gill, R., Surdulescu, G., Zlobecka, K., et al. (2011). Genetic Epidemiology of Induced CYP3A4 Activity. Pharmacogenet Genomics 21 (10), 642–651. doi:10.1097/FPC.0b013e3283498ecf
Rahmioglu, N., Heaton, J., Clement, G., Gill, R., Surdulescu, G., Zlobecka, K., et al. (2013). Genome-wide Association Study Reveals a Complex Genetic Architecture Underpinning-Induced CYP3A4 Enzyme Activity. Eur. J. Drug Metab. Pharmacokinet. 38 (1), 63–67. doi:10.1007/s13318-012-0103-z
Rana, M., Coshic, P., Goswami, R., and Tyagi, R. K. (2017). Influence of a Critical Single Nucleotide Polymorphism on Nuclear Receptor PXR-Promoter Function. Cell Biol. Int. 41 (5), 570–576. doi:10.1002/cbin.10744
Rendic, S. (2002). Summary of Information on Human CYP Enzymes: Human P450 Metabolism Data. Drug Metab. Rev. 34 (1-2), 83–448. doi:10.1081/dmr-120001392
Riera, P., Salazar, J., Virgili, A. C., Tobeña, M., Sebio, A., Gallano, P., et al. (2018). Relevance of CYP3A4*20, UGT1A1*37 and UGT1A1*28 Variants in Irinotecan-Induced Severe Toxicity. Br. J. Clin. Pharmacol. 84 (6), 1389–1392. doi:10.1111/bcp.13574
Rodriguez-Antona, C., Savieo, J. L., Lauschke, V. M., Sangkuhl, K., Drögemöller, B. I., Wang, D., et al. (2022). PharmVar GeneFocus: CYP3A5. Clin. Pharmacol. Ther. doi:10.1002/cpt.2563
Russell, L. E., and Schwarz, U. I. (2020). Variant Discovery Using Next-Generation Sequencing and its Future Role in Pharmacogenetics. Pharmacogenomics 21 (7), 471–486. doi:10.2217/pgs-2019-0190
Saruwatari, J., Yoshida, S., Tsuda, Y., Okada, Y., Ogusu, N., Yoshida, K., et al. (2014). Pregnane X Receptor and Hepatocyte Nuclear Factor 4α Polymorphisms Are Cooperatively Associated with Carbamazepine Autoinduction. Pharmacogenet Genomics 24 (3), 162–171. doi:10.1097/fpc.0000000000000030
Shimada, T., Yamazaki, H., Mimura, M., Inui, Y., and Guengerich, F. P. (1994). Interindividual Variations in Human Liver Cytochrome P-450 Enzymes Involved in the Oxidation of Drugs, Carcinogens and Toxic Chemicals: Studies with Liver Microsomes of 30 Japanese and 30 Caucasians. J. Pharmacol. Exp. Ther. 270 (1), 414–423.
Siccardi, M., D'Avolio, A., Baietto, L., Gibbons, S., Sciandra, M., Colucci, D., et al. (2008). Association of a Single-Nucleotide Polymorphism in the Pregnane X Receptor (PXR 63396C-->T) with Reduced Concentrations of Unboosted Atazanavirwith Reduced Concentrations of Unboosted Atazanavir. Clin. Infect. Dis. 47 (9), 1222–1225. doi:10.1086/592304
Stifft, F., van Kuijk, S. M. J., Bekers, O., and Christiaans, M. H. L. (2018). Increase in Tacrolimus Exposure after Steroid Tapering Is Influenced by CYP3A5 and Pregnane X Receptor Genetic Polymorphisms in Renal Transplant Recipients. Nephrol. Dial. Transpl. 33 (9), 1668–1675. doi:10.1093/ndt/gfy096
Suetsugu, K., Mori, Y., Yamamoto, N., Shigematsu, T., Miyamoto, T., Egashira, N., et al. (2019). Impact of CYP3A5, POR, and CYP2C19 Polymorphisms on Trough Concentration to Dose Ratio of Tacrolimus in Allogeneic Hematopoietic Stem Cell Transplantation. Int. J. Mol. Sci. 20 (10), 2413. doi:10.3390/ijms20102413
Swen, J. J., Nijenhuis, M., de Boer, A., Grandia, L., Maitland-van der Zee, A. H., Mulder, H., et al. (2011). Pharmacogenetics: from Bench to Byte-Aan Update of Guidelines. Clin. Pharmacol. Ther. 89 (5), 662–673. doi:10.1038/clpt.2011.34
Takagi, S., Nakajima, M., Kida, K., Yamaura, Y., Fukami, T., and Yokoi, T. (2010). MicroRNAs Regulate Human Hepatocyte Nuclear Factor 4alpha, Modulating the Expression of Metabolic Enzymes and Cell Cycle. J. Biol. Chem. 285 (7), 4415–4422. doi:10.1074/jbc.M109.085431
Tang, X., and Chen, S. (2015). Epigenetic Regulation of Cytochrome P450 Enzymes and Clinical Implication. Curr. Drug Metab. 16 (2), 86–96. doi:10.2174/138920021602150713114159
Tebbens, J. D., Azar, M., Friedmann, E., Lanzendörfer, M., and Pávek, P. (2018). Mathematical Models in the Description of Pregnane X Receptor (PXR)-Regulated Cytochrome P450 Enzyme Induction. Int. J. Mol. Sci. 19 (6), 1785. doi:10.3390/ijms19061785
Tegude, H., Schnabel, A., Zanger, U. M., Klein, K., Eichelbaum, M., and Burk, O. (2007). Molecular Mechanism of Basal CYP3A4 Regulation by Hepatocyte Nuclear Factor 4alpha: Evidence for Direct Regulation in the Intestine. Drug Metab. Dispos. 35 (6), 946–954. doi:10.1124/dmd.106.013565
Thirumaran, R. K., Lamba, J. K., Kim, R. B., Urquhart, B. L., Gregor, J. C., Chande, N., et al. (2012). Intestinal CYP3A4 and Midazolam Disposition In Vivo Associate with VDR Polymorphisms and Show Seasonal Variation. Biochem. Pharmacol. 84 (1), 104–112. doi:10.1016/j.bcp.2012.03.017
Timsit, Y. E., and Negishi, M. (2007). CAR and PXR: the Xenobiotic-Sensing Receptors. Steroids 72 (3), 231–246. doi:10.1016/j.steroids.2006.12.006
van der Lee, M., Allard, W. G., Vossen, R., Baak-Pablo, R. F., Menafra, R., Deiman, B., et al. (2021). Toward Predicting CYP2D6-Mediated Variable Drug Response from CYP2D6 Gene Sequencing Data. Sci. Transl. Med. 13 (603), 3637. doi:10.1126/scitranslmed.abf3637
van der Lee, M., Kriek, M., Guchelaar, H. J., and Swen, J. J. (2020). Technologies for Pharmacogenomics: A Review. Genes (Basel) 11 (12), 1456. doi:10.3390/genes11121456
Vet, N. J., Brussee, J. M., de Hoog, M., Mooij, M. G., Verlaat, C. W., Jerchel, I. S., et al. (2016). Inflammation and Organ Failure Severely Affect Midazolam Clearance in Critically Ill Children. Am. J. Respir. Crit. Care Med. 194 (1), 58–66. doi:10.1164/rccm.201510-2114OC
Vyhlidal, C. A., Gaedigk, R., and Leeder, J. S. (2006). Nuclear Receptor Expression in Fetal and Pediatric Liver: Correlation with CYP3A Expression. Drug Metab. Dispos. 34 (1), 131–137. doi:10.1124/dmd.105.005967
Wang, D., Guo, Y., Wrighton, S. A., Cooke, G. E., and Sadee, W. (2011). Intronic Polymorphism in CYP3A4 Affects Hepatic Expression and Response to Statin Drugs. Pharmacogenomics J. 11 (4), 274–286. doi:10.1038/tpj.2010.28
Wang, D., and Sadee, W. (2016). CYP3A4 Intronic SNP Rs35599367 (CYP3A4*22) Alters RNA Splicing. Pharmacogenet Genomics 26 (1), 40–43. doi:10.1097/fpc.0000000000000183
Wang, Z. P., Zhao, M., Qu, Q. S., and Miao, S. Z. (2016). Effect of Pregnane X Receptor Polymorphisms on Tacrolimus Blood Concentrations and the Resulting Adverse Reactions in Kidney Transplantation Recipients. Genet. Mol. Res. 15 (3), 8464. doi:10.4238/gmr.15038464
Wei, Z., Jiang, S., Zhang, Y., Wang, X., Peng, X., Meng, C., et al. (2014). The Effect of microRNAs in the Regulation of Human CYP3A4: a Systematic Study Using a Mathematical Model. Sci. Rep. 4, 4283. doi:10.1038/srep04283
Werk, A. N., Lefeldt, S., Bruckmueller, H., Hemmrich-Stanisak, G., Franke, A., Roos, M., et al. (2014). Identification and Characterization of a Defective CYP3A4 Genotype in a Kidney Transplant Patient with Severely Diminished Tacrolimus Clearance. Clin. Pharmacol. Ther. 95 (4), 416–422. doi:10.1038/clpt.2013.210
Williams, J. A., Ring, B. J., Cantrell, V. E., Jones, D. R., Eckstein, J., Ruterbories, K., et al. (2002). Comparative Metabolic Capabilities of CYP3A4, CYP3A5, and CYP3A7. Drug Metab. Dispos. 30 (8), 883–891. doi:10.1124/dmd.30.8.883
Xin, H. W., Liu, H. M., Li, Y. Q., Huang, H., Zhang, L., Yu, A. R., et al. (2014). Association of CYP3A4*18B and CYP3A5*3 Polymorphism with Cyclosporine-Related Liver Injury in Chinese Renal Transplant Recipients. Int. J. Clin. Pharmacol. Ther. 52 (6), 497–503. doi:10.5414/cp202042
Xu, R. A., Wen, J., Tang, P., Wang, C., Xie, S., Zhang, B. W., et al. (2018). Functional Characterization of 22 CYP3A4 Protein Variants to Metabolize Ibrutinib In Vitro. Basic Clin. Pharmacol. Toxicol. 122 (4), 383–387. doi:10.1111/bcpt.12934
Yang, C. C., Zheng, X., Liu, T. H., Wang, C. C., Tang, P. F., Chen, Z., et al. (2019). Functional Characterization of 21 CYP3A4 Variants on Amiodarone Metabolism In Vitro. Xenobiotica 49 (1), 120–126. doi:10.1080/00498254.2017.1414971
Yuan, R., Zhang, X., Deng, Q., Wu, Y., and Xiang, G. (2011). Impact of CYP3A4*1G Polymorphism on Metabolism of Fentanyl in Chinese Patients Undergoing Lower Abdominal Surgery. Clin. Chim. Acta 412 (9-10), 755–760. doi:10.1016/j.cca.2010.12.038
Yuan, X., Lu, H., Zhao, A., Ding, Y., Min, Q., and Wang, R. (2020). Transcriptional Regulation of CYP3A4 by Nuclear Receptors in Human Hepatocytes under Hypoxia. Drug Metab. Rev. 52 (2), 225–234. doi:10.1080/03602532.2020.1733004
Zanger, U. M., and Schwab, M. (2013). Cytochrome P450 Enzymes in Drug Metabolism: Regulation of Gene Expression, Enzyme Activities, and Impact of Genetic Variation. Pharmacol. Ther. 138 (1), 103–141. doi:10.1016/j.pharmthera.2012.12.007
Zanger, U. M., Turpeinen, M., Klein, K., and Schwab, M. (2008). Functional Pharmacogenetics/genomics of Human Cytochromes P450 Involved in Drug Biotransformation. Anal. Bioanal. Chem. 392 (6), 1093–1108. doi:10.1007/s00216-008-2291-6
Zeng, G., Wang, L., Shi, L., Li, H., Zhu, M., Luo, J., et al. (2020). Variability of Voriconazole Concentrations in Patients with Hematopoietic Stem Cell Transplantation and Hematological Malignancies: Influence of Loading Dose, Procalcitonin, and Pregnane X Receptor Polymorphisms. Eur. J. Clin. Pharmacol. 76 (4), 515–523. doi:10.1007/s00228-020-02831-1
Zhang, J., Dai, Y., Liu, Z., Zhang, M., Li, C., Chen, D., et al. (2017). Effect of CYP3A4 and CYP3A5 Genetic Polymorphisms on the Pharmacokinetics of Sirolimus in Healthy Chinese Volunteers. Ther. Drug Monit. 39 (4), 406–411. doi:10.1097/ftd.0000000000000415
Zheng, H., Zeevi, A., Schuetz, E., Lamba, J., McCurry, K., Griffith, B. P., et al. (2004). Tacrolimus Dosing in Adult Lung Transplant Patients Is Related to Cytochrome P4503A5 Gene Polymorphism. J. Clin. Pharmacol. 44 (2), 135–140. doi:10.1177/0091270003262108
Zou, J., Huss, M., Abid, A., Mohammadi, P., Torkamani, A., and Telenti, A. (2019). A Primer on Deep Learning in Genomics. Nat. Genet. 51 (1), 12–18. doi:10.1038/s41588-018-0295-5
Zuo, X. C., Ng, C. M., Barrett, J. S., Luo, A. J., Zhang, B. K., Deng, C. H., et al. (2013). Effects of CYP3A4 and CYP3A5 Polymorphisms on Tacrolimus Pharmacokinetics in Chinese Adult Renal Transplant Recipients: a Population Pharmacokinetic Analysis. Pharmacogenet Genomics 23 (5), 251–261. doi:10.1097/FPC.0b013e32835fcbb6
Keywords: CYP3A locus, CYP3A4, CYP3A5, genetic variants, enzyme activity, missing heritability, pharmacogenomics
Citation: Zhai Q, van der Lee M, van Gelder T and Swen JJ (2022) Why We Need to Take a Closer Look at Genetic Contributions to CYP3A Activity. Front. Pharmacol. 13:912618. doi: 10.3389/fphar.2022.912618
Received: 05 April 2022; Accepted: 30 May 2022;
Published: 16 June 2022.
Edited by:
Andrea Gaedigk, Children’s Mercy Hospital, United StatesReviewed by:
Todd Skaar, Indiana University, United StatesFrancesca Scionti, National Research Council (CNR), Italy
Copyright © 2022 Zhai, van der Lee, van Gelder and Swen. This is an open-access article distributed under the terms of the Creative Commons Attribution License (CC BY). The use, distribution or reproduction in other forums is permitted, provided the original author(s) and the copyright owner(s) are credited and that the original publication in this journal is cited, in accordance with accepted academic practice. No use, distribution or reproduction is permitted which does not comply with these terms.
*Correspondence: Jesse J. Swen, Si5KLlN3ZW5AbHVtYy5ubA==