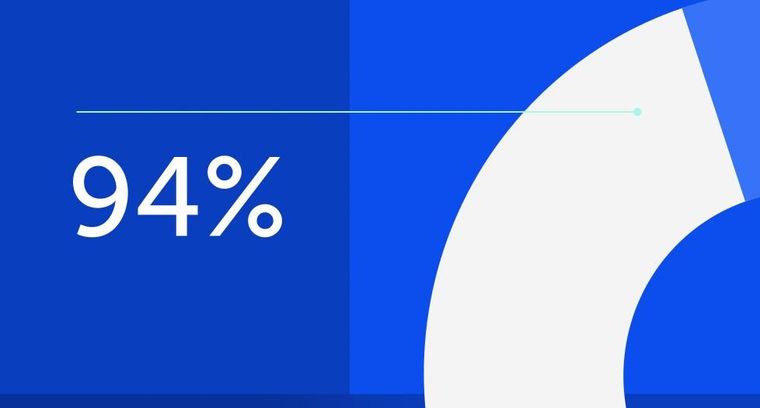
94% of researchers rate our articles as excellent or good
Learn more about the work of our research integrity team to safeguard the quality of each article we publish.
Find out more
SYSTEMATIC REVIEW article
Front. Pharmacol., 20 June 2022
Sec. Drugs Outcomes Research and Policies
Volume 13 - 2022 | https://doi.org/10.3389/fphar.2022.908538
This article is part of the Research TopicPharmacoepidemiology in Diabetes Mellitus and its Complications: Focus on Response to Medications, Volume IIView all 4 articles
Introduction: In human pharmacology, there are two important scientific branches: clinical pharmacology and pharmacoepidemiology. Pharmacokinetic/pharmacodynamic (PK/PD) modeling is important in preclinical studies and randomized control trials. However, it is rarely used in pharmacoepidemiological studies on the effectiveness and medication safety where the target population is heterogeneous and followed for longer periods. The objective of this literature review was to investigate how far PK/PD modeling is utilized in observational studies on glucose-lowering and antiarrhythmic drugs.
Method: A systematic literature search of MEDLINE, Embase, and Web of Science was conducted from January 2010 to 21 February 2020. To calculate the utilization of PK/PD modeling in observational studies, we followed two search strategies. In the first strategy, we screened a 1% random set from 95,672 studies on glucose-lowering and antiarrhythmic drugs on inclusion criteria. In the second strategy, we evaluated the percentage of studies in which PK/PD modeling techniques were utilized. Subsequently, we divided the total number of included studies in the second search strategy by the total number of eligible studies in the first search strategy.
Results: The comprehensive search of databases and the manual search of included references yielded a total of 29 studies included in the qualitative synthesis of our systematic review. Nearly all 29 studies had utilized a PK model, whereas only two studies developed a PD model to evaluate the effectiveness of medications. In total, 16 out of 29 studies (55.1%) used a PK/PD model in the observational setting to study effect modification. The utilization of PK/PD modeling in observational studies was calculated as 0.42%.
Conclusion: PK/PD modeling techniques were substantially underutilized in observational studies of antiarrhythmic and glucose-lowering drugs during the past decade.
There are two important scientific branches in human pharmacology, namely, clinical pharmacology with pharmacokinetic/pharmacodynamic modeling among small patient groups and pharmacoepidemiology with observational studies with effect modeling of medications in large populations or healthcare databases. The question arises as to how far these branches interact with a mutual exchange of information and expertise to attain some synergy by combining and integrating methodology and scientific output. Because in clinical pharmacology, the individual is the study subject, whereas pharmacoepidemiology focuses on effects in study populations, it seems that there is much to be gained where an overlap exists.
Pharmacokinetics (PK) generally describes the time–concentration relationship of the drug in body fluids and tissues in order to investigate the effect of the body on drugs to learn how drugs are absorbed, metabolized, and excreted. PK studies help identify recommendable dosage schemes, as well as predict different toxicity profiles, maximum drug concentration, and food/drug–drug interactions (Derendorf et al., 2000; Charles, 2014; Tamargo et al., 2015; Garralda et al., 2017). Pharmacodynamics (PD) refers to identifying the potential effects of a drug on the body and its physiology, including therapeutic effects and adverse effects. PD studies investigate the relationship between drug concentration at the receptor site and the receptor’s response to different drug concentrations. PK and PD demonstrate the link between the dose–response curves of a particular drug (Derendorf et al., 2000; Felmlee et al., 2012; Garralda et al., 2017). Physiologically based pharmacokinetic (PBPK) modeling predicts both systemic and tissue concentration–time profiles in individuals (Tan et al., 2019) as the basis of individual pharmacodynamic effects. Clinical pharmacologists are constantly moving this forward from individuals to groups with population-based PK/PD studies to evaluate the intra- and inter-individual variability, for instance, variability by gender (Harris et al., 1995), race (Johnson, 2000), or body mass index (BMI) (Hanley et al., 2010) in a specific population and to investigate its associated effects on the PK profile of the medication and its therapeutic response and dosing optimization (Vinks, 2002; Mould and Upton, 2013; Charles, 2014; Bensalem and Ternant, 2020). This information should be a basis for individualized therapy and developing a proper dosage regimen when a favorable therapeutic target goal is set (Vinks, 2002; Jayachandran et al., 2015). Utilization of PK/PD modeling is not only warranted in preclinical studies and randomized control trials (RCTs) as a part of new drug applications, but also PK/PD modeling data are essential for widespread and large-scale use in heterogeneous populations such as the elderly and children and long-term follow-up. The therapeutic window of antiarrhythmic drugs is narrow, and hence, a perfect target for PK/PD studies as small changes in the dosage will lead to a considerable PD alteration (Blix et al., 2010; Tamargo et al., 2015). Furthermore, PK/PD modeling is not only important for drugs with a narrow therapeutic window but also for other medications with a wider index. Glucose-lowering drugs have large therapeutic indexes and are safe. However, diabetic patients may encounter several complications during their life, and therefore, it is important to optimize and individualize the treatment for these patients throughout PK/PD modeling (Landersdorfer and Jusko, 2008). In observational settings, the effects of drugs are studied on a population-based scale. Hence, one might expect that population PK/PD modeling is an important part of pharmacoepidemiology. It should be possible to integrate both branches of human pharmacology to overcome the discrepancy between clinical and real-world medication outcomes. Unfortunately, although applying PK/PD modeling is possible in observational/pharmacoepidemiological studies, it seems that it was only scantily performed until now (Ette et al., 2003; Herland et al., 2005; Charles, 2014; Standing, 2017). Therefore, the objective of this literature review was to investigate how far PK/PD modeling has been utilized in pharmacoepidemiological studies during the past decade and to identify the different types of models and their specific objectives.
A systematic literature review was performed according to the most recently updated PRISMA (Preferred Reporting Items for Systematic Review and Meta-Analysis) guideline (Page et al., 2021). The study was performed in a work package as part of an EU-sponsored IMI-project [grant agreement 116,030], and the study focused on antiarrhythmic and glucose-lowering drugs.
A literature search of MEDLINE, Embase, and Web of Science was conducted from January 2010 to 21 February 2021 based on all MeSH terms and keywords, including but not limited to [observational studies] AND [glucose-lowering agents OR antiarrhythmic drug]. According to a large number of retrieved studies with this strategy and consequently impractical screening, we followed a sampling method. We selected 1% (956 studies) of the total number of studies (95,672) that were selected using the ENDNOTE–Excel random sampling method (Wanner, 2017). After that, by screening the 956 studies in the random set, the number of studies fulfilling the inclusion criteria was identified. Eventually, the proportion of true-positive identified studies out of 956 was used to estimate the number of all observational studies on glucose-lowering medications and antiarrhythmic drugs within the last 10 years (denominator).
To evaluate the percentage of PK/PD modeling utilization among the aforementioned observational studies, MeSH terms describing a PK/PD model were added to the previous search query, including ([observational studies] AND [glucose-lowering agents OR antiarrhythmic drug] AND [Pharmacokinetic OR Pharmacodynamics], (Supplementary Table) (numerator to calculate the utilization of PK/PD modeling). The summary of both search strategies is presented in Table1. In addition, the references of the included studies were manually searched for any further appropriate studies. The prevalence of utilizing PK/PD modeling in observational studies was calculated by dividing the numerator by the denominator.
Two researchers (SMJ and PP) independently screened articles by their titles and abstracts, and any discrepancies were solved by consensus. Afterward, articles were screened by their full text in the second eligibility assessment round and then highlighted as eligible if they had fulfilled the inclusion criteria. Any disagreement in this step was resolved by a third researcher (FA). Furthermore, the data of eligible studies were extracted by study design, study year, type of PK/PD model, effect modifiers, medication name, and PK/PD parameters using a designated form.
The most recent 10-year period [2010–2020] was applied. Inclusion criteria were observational studies (cohorts, cross-sectional, and case–control studies) and human studies on glucose-lowering or antiarrhythmic medications with no language restriction. For the second search strategy, additionally, we included a study if a PK/PD model was developed or utilized (Table 1).
In total, 95,672 records were identified within the literature search. After establishing a 1% random set, 956 studies were screened for their title/abstract, and 860 were eliminated according to the inclusion criteria. The full text of the remaining 96 studies was assessed for eligibility resulting in 68 qualified articles meeting our inclusion criteria. Consequently, by generalizing the 1% sampling to the entire identified records, 6,805 studies fulfilled our aforementioned criteria. This result indicates that approximately 6,805 observational studies (of any type) investigated glucose-lowering and antiarrhythmic medications between 2010 and 2020. The retrieved number was used as the denominator of the utilization proportion. The PRISMA flowchart of the randomization and selection procedure is depicted in Figure 1.
The comprehensive search of databases, in addition to the manual search of included references, yielded a total of 1,256 citations by limiting the previous result (95,672 studies) to the PK/PD mesh terms. In contrast, 1,074 studies were excluded after the title/abstract screening. Of the 182 remaining studies undergoing full-text screening, 29 were included in the qualitative synthesis of our systematic review. Other studies were excluded because they did not apply a PK/PD model—these 29 pharmacometric studies were used as the numerator of the utilization proportion. The process of study identification and eligibility is shown in Figure 1.
By dividing the retrieved numerator by the denominator, the utilization proportion of PK/PD modeling in observational studies was calculated as 0.42% (Figure 2).
While the majority of the 29 included studies (21) investigated glucose-lowering medications, eight studies pertained to antiarrhythmic medications (Araki et al., 2011; Hsu et al., 2011; van den Broek et al., 2011; Shiga et al., 2013; Salem et al., 2016; Bursi et al., 2017; Dallefeld et al., 2018; Riff et al., 2018). As shown in Figure 3, nearly half (48%) of the studies on glucose-lowering drugs focused on metformin and half of the studies on antiarrhythmic drugs concerned lidocaine.
FIGURE 3. Frequency distribution of medications. Abbreviations: OADMs, Oral Anti-diabetic Medications; GLP1 Agonist, glucagon-like peptide 1 Agonist; SGL2 Inhibitors, Sodium-glucose Cotransporter-2 Inhibitors.
Nearly all studies had utilized a PK model. In contrast, only two studies by Salem et al. (2016) and Stringer et al. (2015) developed a PD model to evaluate the effect of medications in a cohort and case–control study design, respectively. Of the 182 studies included for full-text screening, twenty studies focused on antiarrhythmic, while eight (40%) applied PK/PD models. In total, 21 (14.5%) out of 144 studies on glucose-lowering medications applied PK/PD models.
Also, 11 out of 27 studies used a two-compartmental PK model to describe the action of medications. Contrarily, only 1 study (Bursi et al., 2017) developed a model using four-compartmental dispositions (Figure 4).
Also, 16 out of 29 studies (55.1%) used a PK/PD model in the observational setting with the aim of treatment individualization according to different patients’ clinical characteristics and deriving the safest and the most effective dose of the treatment, not only in a small group of patients but also in a large population. A total of 9 studies have evaluated the effect of population demographics (age, sex, race, and BMI, etc.) and 4 articles evaluated the outcome of genetic variation by applying a PK/PD model. The characteristics and purpose of developing a PK/PD model for each included study have been described in Table2.
This analysis of almost 100,000 publications on glucose-lowering and antiarrhythmic drugs over 10 years showed that PK/PD modeling studies and observational studies are in two distinct worlds. This is somewhat disappointing because both clinical pharmacology and pharmacoepidemiology intend to study drug effects in humans, and many of the pharmacokinetic and dynamic determinants are important effect modifiers in pharmacoepidemiological studies. This systematic review is the first appraisal of PK/PD utilization in observational studies, to the best of our knowledge. We showed that, in the past decade, the application of PK/PD modeling has been substantially underutilized in pharmacoepidemiological studies. This is unfortunate because PK/PD modeling gives an insight into important effect modifiers influencing drug metabolism and efficacy and would facilitate risk estimates in pharmacoepidemiology in subgroups and high-risk strata. By taking advantage of PK/PD modeling in large populations, the effects of different covariates (confounders or effect modifiers) on the pharmacokinetic profile of specific medications could be straightforwardly predicted in the real-world setting, which also will be of value for personalized medicine concepts (Boyko, 2013).
Our study showed no obvious growing trend in PK/PD modeling utilization in the past 10 years. The different scientific roots of clinical pharmacology and pharmacoepidemiology might partly explain this. PK/PD modeling was developed in animal studies and human volunteers to investigate drugs’ pharmacokinetics and pharmacodynamics effect on human biology. Subsequent PK/PD modeling in clinical trials in homogenous patient groups to optimize dosing schedules was nevertheless considered of limited value in predicting the response in large and heterogeneous populations (Vinks, 2002). Although pharmacoepidemiology started mainly quantifying adverse reactions and their risk factors after marketing, the increasing size of healthcare databases nowadays stimulates comparative studies on effectiveness. Unfortunately, such databases rarely include information that can be used for personalized fine-tuning of results within various risk groups (Van Boxtel and Wang, 1997). For instance, information on body mass index or smoking is often missing, while such determinants are often relevant. Another important reason for this underutilization may be because blood sampling and in-patient observations are often not feasible in a pharmacoepidemiological setting. In clinical trials, detailed patient information is more easily obtained. Consequently, observational studies have some limitations which can be avoided in randomized control trials to a great extent by complete data collection.
Our review was limited to two pharmacotherapeutic groups, that is, glucose-lowering and antiarrhythmic drugs as representative agents of medications with a wide and narrow therapeutic window, respectively. Due to the striking global prevalence of diabetes (6.28% in 2017) in comparison to cardiac arrhythmia (0.51% in 2017) (Khan et al., 2020; Lippi et al., 2021), the use of glucose-lowering agents is higher than that of antiarrhythmic drugs. In line with this, the selection of articles from the current study sample also shows that the total number of studies on glucose-lowering medications (21 studies) is higher than that on antiarrhythmic (8 studies). However, in addition to this lower number, our literature search suggests that more effort has been put into applying PK/PD models for antiarrhythmic drugs. An important reason for this higher PK/PD modeling yield in studies on antiarrhythmic drugs is their narrower therapeutic window (Muller and Milton, 2012). After all, the narrower the therapeutic window, the higher the probability of toxicity or adverse effect, and therefore, more PK/PD profile monitoring is required (Tamargo et al., 2015). The study by Caruso et al. (2014) also confirmed the importance of applying the PK/PD model to studies on antiarrhythmic drugs to enable a more accurate prediction of the medication’s clinical effects.
PK/PD modeling is highly encouraged in other vital therapeutic processes, not only in medications with a narrower therapeutic index but also in pediatric clinical research (De Cock et al., 2011). Furthermore, De Cock et al. explained the pivotal role of PK/PD modeling in studies on children to predict the possible therapeutic failure and occurrence of adverse effects or death.
Our systematic review observed that most studies developed the PK/PD model to evaluate the effect of different covariates on therapeutic outcomes in small- or medium-sized populations. Our result is in line with a previous study (Standing, 2017) that showed that using a PK/PD model can improve the approach to personalized medicine where the effect of variables such as age, sex, BMI, and genetic variation could be adjusted.
It should be taken into account that most included studies applied a PK/PD model to the secondary data. Secondary data are data which cannot be traced back to the level of individual cases of statistical units. These data sources can be retrieved by collecting the primary data from trials with matching population characteristics. For instance, the data had been collected for a particular purpose/research question but again utilized to answer another research question. The utilization of secondary data is more practical than collecting primary data from large populations, and it is also more economical (Schneeweiss and Avorn, 2005; Suissa and Garbe, 2007). Moreover, with the recent advances in electronic healthcare databases, pharmacoepidemiologists have easier access to these data to boost their investigations on medications’ effectiveness and individualized dosage optimization (Hennessy, 2006). For instance, clinical trial data on the incidence of cardiovascular endpoints in age-, sex-, and BMI-stratified analyses matched to observational studies could improve the quality of effectiveness studies in pharmacoepidemiology. In the future, individual pharmacogenetic passports can be used to tailor individual pharmacotherapy, especially if genetic analyses in responders/non-responders would become a common practice in drug trials. PK/PD modeling thereby will play a crucial role in the efficacy and safety assessment of recently developed medications and optimizing treatment. The synergy between clinical pharmacology and pharmacoepidemiology could be substantially improved if more effect- and risk-stratification in clinical trials would occur to investigate which subgroups’ response and toxicity are the highest. In this way, recognizing determinants of drug response and toxicity would provide important information on effect modifiers available in observational datasets. This would improve the validity and efficiency of real-life effectiveness studies.
Overall, there is a lack of synergy between clinical pharmacology and pharmacoepidemiology, especially large-sized observational studies make only limited use of information on effect modification from PK/PD studies. On the other hand, clinical trials might pay more attention to risk stratification, for instance, by pharmacogenetic analyses in responders/non-responders. Personalized pharmacotherapy will favor increased cooperation between both branches of human pharmacology.
The original contributions presented in the study are included in the article/Supplementary Material; further inquiries can be directed to the corresponding author.
SMJ, PP, and MNS screened and extracted the eligible studies. SMJ has drafted the manuscript. All authors revised it for important intellectual content and approved the final version of the manuscript to be published. BS and FA developed the concept and design of the study.
This project received funding from the Innovative Medicines Initiative 2 joint undertaking under grant agreement no 116030. This joint undertaking receives support from the European Union’s Horizon 2020 Research and Innovation Programme and EFPIA.
The authors declare that the research was conducted in the absence of any commercial or financial relationships that could be construed as a potential conflict of interest.
All claims expressed in this article are solely those of the authors and do not necessarily represent those of their affiliated organizations, or those of the publisher, the editors, and the reviewers. Any product that may be evaluated in this article, or claim that may be made by its manufacturer, is not guaranteed or endorsed by the publisher.
The Supplementary Material for this article can be found online at: https://www.frontiersin.org/articles/10.3389/fphar.2022.908538/full#supplementary-material
Araki, R., Yukawa, E., Nakashima, M. N., Fukuchi, H., Sasaki, H., Yano, K., et al. (2011). Population Pharmacokinetic Investigation for Optimization of Amiodarone Therapy in Japanese Patients. Ther. Drug Monit. 33, 750–756. doi:10.1097/FTD.0b013e318239a728
Bardin, C., Nobecourt, E., Larger, E., Chast, F., Treluyer, J. M., and Urien, S. (2012). Population Pharmacokinetics of Metformin in Obese and Non-obese Patients with Type 2 Diabetes Mellitus. Eur. J. Clin. Pharmacol. 68, 961–968. doi:10.1007/s00228-011-1207-0
Bensalem, A., and Ternant, D. (2020). Pharmacokinetic Variability of Therapeutic Antibodies in Humans: A Comprehensive Review of Population Pharmacokinetic Modeling Publications. Clin. Pharmacokinet. 59, 857–874. doi:10.1007/s40262-020-00874-2
Blix, H. S., Viktil, K. K., Moger, T. A., and Reikvam, A. (2010). Drugs with Narrow Therapeutic Index as Indicators in the Risk Management of Hospitalised Patients. Pharm. Pract. (Granada) 8, 50–55. doi:10.4321/s1886-36552010000100006
Boyko, E. J. (2013). Observational Research-Oopportunities and Limitations. J. Diabetes Complicat. 27, 642–648. doi:10.1016/j.jdiacomp.2013.07.007
Bursi, R., Piana, C., Grevel, J., Huntjens, D., and Boesl, I. (2017). Evaluation of the Population Pharmacokinetic Properties of Lidocaine and its Metabolites after Long-Term Multiple Applications of a Lidocaine Plaster in Post-herpetic Neuralgia Patients. Eur. J. Drug Metab. Pharmacokinet. 42, 801–814. doi:10.1007/s13318-017-0400-7
Carlsson Petri, K. C., Ingwersen, S. H., Flint, A., Zacho, J., and Overgaard, R. V. (2018). Semaglutide s.C. Once-Weekly in Type 2 Diabetes: A Population Pharmacokinetic Analysis. Diabetes Ther. 9, 1533–1547. doi:10.1007/s13300-018-0458-5
Caruso, A., Frances, N., Meille, C., Greiter-Wilke, A., Hillebrecht, A., and Lavé, T. (2014). Translational PK/PD Modeling for Cardiovascular Safety Assessment of Drug Candidates: Methods and Examples in Drug Development. J. Pharmacol. Toxicol. Methods 70, 73–85. doi:10.1016/j.vascn.2014.05.004
Ceacareanu, A. C., Brown, G. W., Moussa, H. A., and Wintrob, Z. A. P. (2018). Application of a Pharmacokinetic Model of Metformin Clearance in a Population with Acute Myeloid Leukemia. J. Res. Pharm. Pract. 7, 41–45. doi:10.4103/jrpp.JRPP_17_53
Charles, B. (2014). Population Pharmacokinetics: an Overview. Aust. Prescr. 37, 210–213. doi:10.18773/austprescr.2014.078
Chitnis, S. D., Han, Y., Yamaguchi, M., Mita, S., Zhao, R., Sunkara, G., et al. (2016). Population Pharmacokinetic Modeling and Noncompartmental Analysis Demonstrated Bioequivalence between Metformin Component of Metformin/vildagliptin Fixed-Dose Combination Products and Metformin Immediate-Release Tablet Sourced from Various Countries. Clin. Pharmacol. Drug Dev. 5, 40–51. doi:10.1002/cpdd.191
Dallefeld, S. H., Atz, A. M., Yogev, R., Sullivan, J. E., Al-Uzri, A., Mendley, S. R., et al. (2018). A Pharmacokinetic Model for Amiodarone in Infants Developed from an Opportunistic Sampling Trial and Published Literature Data. J. Pharmacokinet. Pharmacodyn. 45, 419–430. doi:10.1007/s10928-018-9576-y
De Cock, R. F., Piana, C., Krekels, E. H., Danhof, M., Allegaert, K., and Knibbe, C. A. (2011). The Role of Population PK-PD Modelling in Paediatric Clinical Research. Eur. J. Clin. Pharmacol. 67 Suppl 1, 5–16. doi:10.1007/s00228-009-0782-9
Derendorf, H., Lesko, L. J., Chaikin, P., Colburn, W. A., Lee, P., Miller, R., et al. (2000). Pharmacokinetic/pharmacodynamic Modeling in Drug Research and Development. J. Clin. Pharmacol. 40, 1399–1418.
Dissanayake, A. M., Wheldon, M. C., and Hood, C. J. (2018). Pharmacokinetics of Metformin in Patients with Chronic Kidney Disease Stage 4 and Metformin-Naïve Type 2 Diabetes. Pharmacol. Res. Perspect. 6, e00424. doi:10.1002/prp2.424
Duong, J. K., Kroonen, M. Y. A. M., Kumar, S. S., Heerspink, H. L., Kirkpatrick, C. M., Graham, G. G., et al. (2017). A Dosing Algorithm for Metformin Based on the Relationships between Exposure and Renal Clearance of Metformin in Patients with Varying Degrees of Kidney Function. Eur. J. Clin. Pharmacol. 73, 981–990. doi:10.1007/s00228-017-2251-1
Duong, J. K., Kumar, S. S., Furlong, T. J., Kirkpatrick, C. M., Graham, G. G., Greenfield, J. R., et al. (2015). The Pharmacokinetics of Metformin and Concentrations of Haemoglobin A1C and Lactate in Indigenous and Non-indigenous Australians with Type 2 Diabetes Mellitus. Br. J. Clin. Pharmacol. 79, 617–623. doi:10.1111/bcp.12525
Ette, E. I., Williams, P. J., Kim, Y. H., Lane, J. R., Liu, M. J., and Capparelli, E. V. (2003). Model Appropriateness and Population Pharmacokinetic Modeling. J. Clin. Pharmacol. 43, 610–623. doi:10.1177/0091270003253624
Felmlee, M. A., Morris, M. E., and Mager, D. E. (2012). Mechanism-based pharmacodynamic modeling. In Computational toxicology. 583–600. doi:10.1007/978-1-62703-050-2_21
Garralda, E., Dienstmann, R., and Tabernero, J. (2017). Pharmacokinetic/Pharmacodynamic Modeling for Drug Development in Oncology. Am. Soc. Clin. Oncol. Educ. Book 37, 210–215. doi:10.1200/EDBK_180460
Geiser, J. S., Heathman, M. A., Cui, X., Martin, J., Loghin, C., Chien, J. Y., et al. (2016). Clinical Pharmacokinetics of Dulaglutide in Patients with Type 2 Diabetes: Analyses of Data from Clinical Trials. Clin. Pharmacokinet. 55, 625–634. doi:10.1007/s40262-015-0338-3
Gertz, M., Tsamandouras, N., Säll, C., Houston, J. B., and Galetin, A. (2014). Reduced Physiologically-Based Pharmacokinetic Model of Repaglinide: Impact of OATP1B1 and CYP2C8 Genotype and Source of In Vitro Data on the Prediction of Drug-Drug Interaction Risk. Pharm. Res. 31, 2367–2382. doi:10.1007/s11095-014-1333-3
Goswami, S., Yee, S. W., Stocker, S., Mosley, J. D., Kubo, M., Castro, R., et al. (2014). Genetic Variants in Transcription Factors Are Associated with the Pharmacokinetics and Pharmacodynamics of Metformin. Clin. Pharmacol. Ther. 96, 370–379. doi:10.1038/clpt.2014.109
Haidar, A., Duval, C., Legault, L., and Rabasa-Lhoret, R. (2013). Pharmacokinetics of Insulin Aspart and Glucagon in Type 1 Diabetes during Closed-Loop Operation. J. Diabetes Sci. Technol. 7, 1507–1512. doi:10.1177/193229681300700610
Hanley, M. J., Abernethy, D. R., and Greenblatt, D. J. (2010). Effect of Obesity on the Pharmacokinetics of Drugs in Humans. Clin. Pharmacokinet. 49, 71–87. doi:10.2165/11318100-000000000-00000
Harris, R. Z., Benet, L. Z., and Schwartz, J. B. (1995). Gender Effects in Pharmacokinetics and Pharmacodynamics. Drugs 50, 222–239. doi:10.2165/00003495-199550020-00003
Hennessy, S. (2006). Use of Health Care Databases in Pharmacoepidemiology. Basic Clin. Pharmacol. Toxicol. 98, 311–313. doi:10.1111/j.1742-7843.2006.pto_368.x
Herland, K., Akselsen, J. P., Skjønsberg, O. H., and Bjermer, L. (2005). How Representative Are Clinical Study Patients with Asthma or COPD for a Larger "real Life" Population of Patients with Obstructive Lung Disease? Respir. Med. 99, 11–19. doi:10.1016/j.rmed.2004.03.026
Hoeben, E., De Winter, W., Neyens, M., Devineni, D., Vermeulen, A., and Dunne, A. (2016). Population Pharmacokinetic Modeling of Canagliflozin in Healthy Volunteers and Patients with Type 2 Diabetes Mellitus. Clin. Pharmacokinet. 55, 209–223. doi:10.1007/s40262-015-0307-x
Hsu, Y. W., Somma, J., Newman, M. F., and Mathew, J. P. (2011). Population Pharmacokinetics of Lidocaine Administered during and after Cardiac Surgery. J. Cardiothorac. Vasc. Anesth. 25, 931–936. doi:10.1053/j.jvca.2011.03.008
Jayachandran, D., Laínez-Aguirre, J., Rundell, A., Vik, T., Hannemann, R., Reklaitis, G., et al. (2015). Model-based Individualized Treatment of Chemotherapeutics: Bayesian Population Modeling and Dose Optimization. PloS one 10, e0133244. doi:10.1371/journal.pone.0133244
Johnson, J. A. (2000). Predictability of the Effects of Race or Ethnicity on Pharmacokinetics of Drugs. Int. J. Clin. Pharmacol. Ther. 38, 53–60. doi:10.5414/cpp38053
Khan, M. A. B., Hashim, M. J., King, J. K., Govender, R. D., Mustafa, H., and Al Kaabi, J. (2020). Epidemiology of Type 2 Diabetes - Global Burden of Disease and Forecasted Trends. J. Epidemiol. Glob. Health 10, 107–111. doi:10.2991/jegh.k.191028.001
Landersdorfer, C. B., and Jusko, W. J. (2008). Pharmacokinetic/pharmacodynamic Modelling in Diabetes Mellitus. Clin. Pharmacokinet. 47, 417–448. doi:10.2165/00003088-200847070-00001
Li, L., Guan, Z., Li, R., Zhao, W., Hao, G., Yan, Y., et al. (2020). Population Pharmacokinetics and Dosing Optimization of Metformin in Chinese Patients with Type 2 Diabetes Mellitus. Med. Baltim. 99, e23212. doi:10.1097/MD.0000000000023212
Lippi, G., Sanchis-Gomar, F., and Cervellin, G. (2021). Global Epidemiology of Atrial Fibrillation: An Increasing Epidemic and Public Health Challenge. Int. J. Stroke 16, 217–221. doi:10.1177/1747493019897870
Mould, D. R., and Upton, R. N. (2013). Basic Concepts in Population Modeling, Simulation, and Model-Based Drug Development-Part 2: Introduction to Pharmacokinetic Modeling Methods. CPT Pharmacometrics Syst. Pharmacol. 2, e38. doi:10.1038/psp.2013.14
Muller, P. Y., and Milton, M. N. (2012). The Determination and Interpretation of the Therapeutic Index in Drug Development. Nat. Rev. Drug Discov. 11, 751–761. doi:10.1038/nrd3801
Overgaard, R. V., Lindberg, S. Ø., and Thielke, D. (2019). Impact on HbA1c and Body Weight of Switching from Other GLP-1 Receptor Agonists to Semaglutide: A Model-Based Approach. Diabetes Obes. Metab. 21, 43–51. doi:10.1111/dom.13479
Page, M. J., Mckenzie, J. E., Bossuyt, P. M., Boutron, I., Hoffmann, T. C., Mulrow, C. D., et al. (2021). Updating Guidance for Reporting Systematic Reviews: Development of the PRISMA 2020 Statement. J. Clin. Epidemiol. 134, 103–112. doi:10.1016/j.jclinepi.2021.02.003
Petri, K. C., Jacobsen, L. V., and Klein, D. J. (2015). Comparable Liraglutide Pharmacokinetics in Pediatric and Adult Populations with Type 2 Diabetes: a Population Pharmacokinetic Analysis. Clin. Pharmacokinet. 54, 663–670. doi:10.1007/s40262-014-0229-z
Riff, C., Bourgoin, A., Marsot, A., Allanioux, L., Leone, M., Blin, O., et al. (2018). Population Pharmacokinetic Model for Tumescent Lidocaine in Women Undergoing Breast Cancer Surgery. Eur. J. Clin. Pharmacol. 74, 1309–1315. doi:10.1007/s00228-018-2503-8
Riggs, M. M., Staab, A., Seman, L., Macgregor, T. R., Bergsma, T. T., Gastonguay, M. R., et al. (2013). Population Pharmacokinetics of Empagliflozin, a Sodium Glucose Cotransporter 2 Inhibitor, in Patients with Type 2 Diabetes. J. Clin. Pharmacol. 53, 1028–1038. doi:10.1002/jcph.147
Salem, J. E., El-Aissaoui, M., Alazard, M., Hulot, J. S., Aissaoui, N., Le-Heuzey, J. Y., et al. (2016). Modeling of Amiodarone Effect on Heart Rate Control in Critically Ill Patients with Atrial Tachyarrhythmias. Clin. Pharmacokinet. 55, 991–1002. doi:10.1007/s40262-016-0372-9
Santoro, A. B., Stage, T. B., Struchiner, C. J., Christensen, M. M., Brosen, K., and Suarez-Kurtz, G. (2016). Limited Sampling Strategy for Determining Metformin Area under the Plasma Concentration-Time Curve. Br. J. Clin. Pharmacol. 82, 1002–1010. doi:10.1111/bcp.13049
Schneeweiss, S., and Avorn, J. (2005). A Review of Uses of Health Care Utilization Databases for Epidemiologic Research on Therapeutics. J. Clin. Epidemiol. 58, 323–337. doi:10.1016/j.jclinepi.2004.10.012
Shiga, T., Hashiguchi, M., Naganuma, M., Suzuki, A., and Hagiwara, N. (2013). Contributing Factors to the Apparent Clearance of Bepridil in Patients with Paroxysmal or Persistent Atrial Fibrillation: Analysis Using Population Pharmacokinetics. Ther. Drug Monit. 35, 367–373. doi:10.1097/FTD.0b013e318286ec33
Sokolov, V., Yakovleva, T., Ueda, S., Parkinson, J., Boulton, D. W., Penland, R. C., et al. (2019). Urinary Glucose Excretion after Dapagliflozin Treatment: An Exposure-Response Modelling Comparison between Japanese and Non-Japanese Patients Diagnosed with Type 1 Diabetes Mellitus. Diabetes Obes. Metab. 21, 829–836. doi:10.1111/dom.13586
Standing, J. F. (2017). Understanding and Applying Pharmacometric Modelling and Simulation in Clinical Practice and Research. Br. J. Clin. Pharmacol. 83, 247–254. doi:10.1111/bcp.13119
Stringer, F., Dejongh, J., Enya, K., Koumura, E., Danhof, M., and Kaku, K. (2015). Evaluation of the Long-Term Durability and Glycemic Control of Fasting Plasma Glucose and Glycosylated Hemoglobin for Pioglitazone in Japanese Patients with Type 2 Diabetes. Diabetes Technol. Ther. 17, 215–223. doi:10.1089/dia.2014.0222
Suissa, S., and Garbe, E. (2007). Primer: Administrative Health Databases in Observational Studies of Drug Effects-Aadvantages and Disadvantages. Nat. Clin. Pract. Rheumatol. 3, 725–732. doi:10.1038/ncprheum0652
Tamargo, J., Le Heuzey, J. Y., and Mabo, P. (2015). Narrow Therapeutic Index Drugs: a Clinical Pharmacological Consideration to Flecainide. Eur. J. Clin. Pharmacol. 71, 549–567. doi:10.1007/s00228-015-1832-0
Tan, M. L., Zhao, P., Zhang, L., Ho, Y. F., Varma, M. V. S., Neuhoff, S., et al. (2019). Use of Physiologically Based Pharmacokinetic Modeling to Evaluate the Effect of Chronic Kidney Disease on the Disposition of Hepatic CYP2C8 and OATP1B Drug Substrates. Clin. Pharmacol. Ther. 105, 719–729. doi:10.1002/cpt.1205
van den Broek, M. P., Huitema, A. D., Van Hasselt, J. G., Groenendaal, F., Toet, M. C., Egberts, T. C., et al. (2011). Lidocaine (Lignocaine) Dosing Regimen Based upon a Population Pharmacokinetic Model for Preterm and Term Neonates with Seizures. Clin. Pharmacokinet. 50, 461–469. doi:10.2165/11589160-000000000-00000
van Noorden, B., Knopp, J. L., and Chase, J. G. (2019). A Subcutaneous Insulin Pharmacokinetic Model for Insulin Detemir. Comput. Methods Programs Biomed. 178, 1–9. doi:10.1016/j.cmpb.2019.06.007
Van Boxtel, C. J., and Wang, G. (1997). Some Observations on Pharmacoepidemiology in Europe. Neth J. Med. 51, 205–212. doi:10.1016/s0300-2977(97)00054-5
Vinks, A. A. (2002). The Application of Population Pharmacokinetic Modeling to Individualized Antibiotic Therapy. Int. J. Antimicrob. Agents 19, 313–322. doi:10.1016/s0924-8579(02)00023-7
Wanner, A. (2017). Finding a Random Set of Citations in EndNote. [Online]. Available: https://expertsearching.wordpress.com/2017/03/01/finding-a-random-set-of-citations-in-endnote/(Accessed.
Keywords: pharmacodynamics (PD), pharmacokinetics, pharmacoepidemiology, glucose-lowering agents, antiarrhythmic, PK/PD modeling
Citation: Mohammadi Jouabadi S, Nekouei Shahraki M, Peymani P, Stricker BH and Ahmadizar F (2022) Utilization of Pharmacokinetic/Pharmacodynamic Modeling in Pharmacoepidemiological Studies: A Systematic Review on Antiarrhythmic and Glucose-Lowering Medicines. Front. Pharmacol. 13:908538. doi: 10.3389/fphar.2022.908538
Received: 30 March 2022; Accepted: 04 May 2022;
Published: 20 June 2022.
Edited by:
Daniela Calina, University of Medicine and Pharmacy of Craiova, RomaniaReviewed by:
Aristidis M. Tsatsakis, University of Crete, GreeceCopyright © 2022 Mohammadi Jouabadi, Nekouei Shahraki, Peymani, Stricker and Ahmadizar. This is an open-access article distributed under the terms of the Creative Commons Attribution License (CC BY). The use, distribution or reproduction in other forums is permitted, provided the original author(s) and the copyright owner(s) are credited and that the original publication in this journal is cited, in accordance with accepted academic practice. No use, distribution or reproduction is permitted which does not comply with these terms.
*Correspondence: Bruno H. Stricker, Yi5zdHJpY2tlckBlcmFzbXVzbWMubmw=
Disclaimer: All claims expressed in this article are solely those of the authors and do not necessarily represent those of their affiliated organizations, or those of the publisher, the editors and the reviewers. Any product that may be evaluated in this article or claim that may be made by its manufacturer is not guaranteed or endorsed by the publisher.
Research integrity at Frontiers
Learn more about the work of our research integrity team to safeguard the quality of each article we publish.