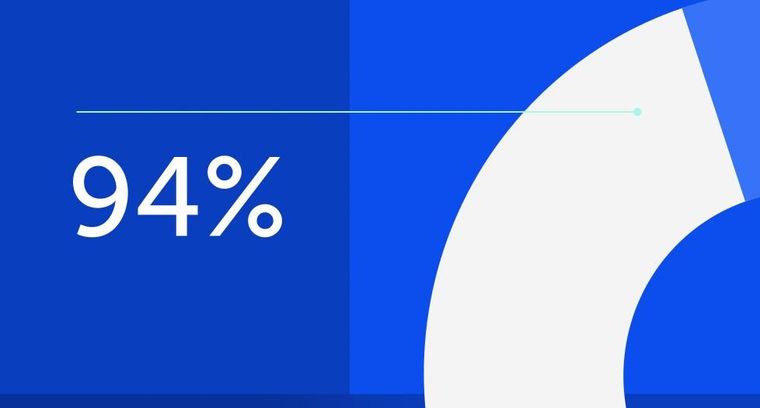
94% of researchers rate our articles as excellent or good
Learn more about the work of our research integrity team to safeguard the quality of each article we publish.
Find out more
ORIGINAL RESEARCH article
Front. Pharmacol., 19 May 2022
Sec. Gastrointestinal and Hepatic Pharmacology
Volume 13 - 2022 | https://doi.org/10.3389/fphar.2022.891410
This article is part of the Research TopicAdvances in Non-Alcoholic Fatty Liver Disease Therapeutics: Pathogenic Mechanisms and TargetsView all 15 articles
Background: Inflammatory bowel disease (IBD) and non-alcoholic fatty liver disease (NAFLD) usually co-exist clinically. However, whether such association is causal is still unknown.
Methods: Genetic variants were extracted as instrumental variables from the largest genome-wide association study (GWAS) of IBD, Crohn’s disease (CD) and ulcerative colitis (UC) with 25,042 cases and 34,915 controls (GWAS p-value < 5 × 10−8). Information of genetic variants in NAFLD was extracted from a GWAS with 1,483 cases and 17,781controls. Also, liver fat content (LFC) was included as the outcome. Then, a bi-direction Mendelian randomization (MR) was carried out to appraise the causal relationship between NAFLD on IBD. Besides, a multivariable MR (MVMR) design was carried to adjust for body mass index (BMI) and type 2 diabetes (T2D) as well.
Results: Generally, IBD might not affect the risk of NAFLD (OR = 0.994 [0.970, 1.019]), together with its subtypes including UC and CD. However, genetically-elevated risk of IBD might cause liver fat accumulation (beta = 0.019, p-value = 0.016) while turning insignificant at Bonferroni correction. Besides, no causal effect of NAFLD on IBD was observed (OR = 0.968 [0.928, 1.009]), together with UC and CD. Also, genetically-elevated LFC could not impact IBD, UC and CD either. The MR CAUSE analysis supported these null associations and MVMR analysis also supported such null associations even after adjusting for BMI and T2D.
Conclusion: This MR study ruled out the causal relationship between IBD and NAFLD, suggesting therapeutics targeting NAFLD might not work for IBD and vice versa.
Non-alcoholic fatty liver disease (NAFLD), a disease characterized with liver steatosis and determined by liver fat content (LFC), is amongst the most important causes of liver diseases even in lean patients and its global prevalence is estimated to reach over 24% (Younossi et al., 2018). Inflammatory bowel disease (IBD), including ulcerative colitis (UC) and Crohn’s disease (CD), is a chronic intestinal inflammation which can reduce patient’s life expectancy from age-related comorbidities like cardiovascular diseases, and its global prevalence will be as high as 1% by 2030 in many regions (Kaplan and Windsor, 2021). Considerable epidemiological evidence has linked these two diseases together where IBD was associated with increased risk of NAFLD and they usually coexist (McHenry et al., 2019; Zou et al., 2019; Lin et al., 2021). However, an observational study suggested there were no significant differences in terms of IBD characteristics between IBD patients with and without NAFLD, indicating the interplay between NAFLD and IBD is no easy (Magrì et al., 2019). Besides, it should be noted that all the available evidence is based on observational studies, which might be biased by unavoidable potential confounders and reverse causation (Piovani et al., 2021). There is a paucity of evidence illustrating whether the observed association is causal.
Mendelian randomization (MR) is an emerging epidemiological method of causal inference and has made great contribution to detection of causal risk factors for diseases. For instance, Voight et al. ruled out the possibility that high-density lipoprotein cholesterol (HDL-C) could lower risk of myocardial infarction using MR design, challenging the traditional concept (Voight et al., 2012). MR design utilizes genetic variants as instrument variables (IVs), usually single nucleotide polymorphisms (SNPs) and can largely evade bias caused by potential confounders as SNPs are allocated randomly at conception and free from influence of confounders (Davey Smith and Hemani, 2014). Thanks to the rapid development of genome-wide association study (GWAS) and accumulation of publicly available GWAS summary statistics, MR design based on two-sample setting is becoming more flexible and accessible. Several MR studies have identified causal risk factors of IBD, such as body fat percentage (Carreras-Torres et al., 2020) and ankylosing spondylitis (Cui et al., 2020). Also, a MR study clarified the causal relationship between NAFLD, type 2 diabetes (T2D) and obesity (Liu et al., 2020).
However, there is no MR study exploring the causal relationship between NAFLD and IBD. In this study, we aim to explore the causal relationship between NAFLD and IBD, hoping to disentangle their complex interplay and provide useful advice in clinical practice.
The GWAS summary statistics of NAFLD were obtained from a recent published GWAS, with 1,483 European NAFLD cases and 17,781 genetically matched controls, and this study included the first five principal components as covariates (Anstee et al., 2020). Considering NAFLD is closely associated with LFC, we also selected a recent LFC GWAS which included 32,858 European participants from United Kingdom Biobank and adjusted for age at imaging visit, age squared, sex, imaging center, scan date, scan time, genotyping batch, and genetic relatedness (Liu et al., 2021). The IBD data were downloaded from an IBD meta-GWAS which included a total of 59,957 subjects, with 12,194 Crohn’s disease and 12,366 ulcerative colitis, and this study adjusted for the first ten principal components for each cohort (de Lange et al., 2017). As for the IBD GWAS, there were 25,042 European and unknown ancestry cases, together with 34,915 European and unknown ancestry controls (de Lange et al., 2017). Genomic control has been applied to all these studies. Each GWAS has been approved by corresponding Ethics Committees.
MR study should be carried out under three principal assumptions: (Younossi et al., 2018): the genetic variants should be closely associated with the exposure; (Kaplan and Windsor, 2021) the genetic variants should not be associated with any potential confounders that might mediate the way from exposure to outcome; (McHenry et al., 2019) the genetic variants should not be associated with outcome if conditioned on exposure (Emdin et al., 2017) (Figure 1). Besides, additional assumptions should be satisfied as well, such as linearity and no interaction between mediator and outcome.
FIGURE 1. The basic principles of Mendelian randomization (MR) study. A represents the three principal assumptions; B represents the bi-direction MR design. IV is instrumental variable; IBD is inflammatory bowel disease; UC is ulcerative colitis; CD is Crohn’s disease; NAFLD is non-alcoholic fatty liver disease; LFC is liver fat content.
Genetic variants were selected as IVs if reaching the genome-wide significance (GWAS p-value < 5 × 10−8) and were further clumped based on linkage disequilibrium (LD, r2 = 0.01) and genomic region (clump window 1,000 kilobases). Also, SNP with lower minor allele frequency (MAF <0.01) would be removed in the following analysis. In the two-sample setting, we harmonized summary statistic data to ensure each IV was aligned with the same effect allele.
Preliminarily, the IBD, UC and CD were treated as the exposures to estimate their causal effect on NAFLD and LFC, resulting in six pairs of causal relationships including IBD-NAFLD, IBD-LFC, UC-NAFLD, UC-LFC, CD-NAFLD and CD-LFC. Then, a bi-directional MR analysis was performed where the NFALD and LFC were set to be the exposures, generating another six pairs of causal relationship namely NAFLD-IBD, NAFLD-UC, NAFLD-CD, LFC-IBD, LFC-UC and LFC-CD (Figure 1). Furthermore, a multivariable MR (MVMR) design was elaborated with adjusting for body mass index (BMI) and type 2 diabetes (T2D), two potential confounders according to a recent meta-analysis (Lin et al., 2021). Therein, the GWAS summary statistics of BMI was from the Genetic Investigation of ANthropometric Traits (GIANT) consortium (Locke et al., 2015) and these of T2D was from the DIAbetes Genetics Replication And Meta-analysis (DIAGRAM) consortium (Mahajan et al., 2014).
Initially, Wald ratio estimation was utilized to obtain the effect size of exposure on outcome for each IV and then each IV’s causal effect size was combined using an inverse-variance weighted (IVW) method. F statistics were calculated for each IV to ensure a sufficient power. The Cochrane’s Q value was calculated to appraise heterogeneity and the multiplicative random effect model would be adopted if there exists heterogeneity. The MR Steiger test has been performed to judge whether the IVs affect exposure more than outcome and we would eliminate the IV if it explained outcome more than exposure (Hemani et al., 2017).
Considering the horizontal pleiotropy can largely mislead the MR estimation, various methods have been utilized to minimize the bias caused by it, including both correlated and uncorrelated horizontal pleiotropy. For correlated horizontal pleiotropy, MR-Egger regression (Bowden et al., 2015) and MR-PRESSO (Verbanck et al., 2018) were utilized. The MR-Egger regression uses the intercept obtained from regression analysis to judge the correlated horizontal pleiotropy and we assume there is no correlated horizontal pleiotropy if the intercept equals to zero (Bowden et al., 2015). The MR-PRESSO uses distortion test to detect outliers that might manifest horizontal pleiotropy and further corrects the IVW estimation with removal of outliers (Verbanck et al., 2018). For uncorrelated horizontal pleiotropy, another two methods were adopted, including weighted median (Bowden et al., 2016) and CAUSE (Morrison et al., 2020). Therein, CAUSE can allow for both correlated and uncorrelated horizontal pleiotropy, and it was functioned based on full summary statistics (Morrison et al., 2020). In CAUSE analysis, the threshold of SNP-exposure p-value was 1 × 10−3, ensuring enough IVs to estimate nuisance parameters.
All statistical analyses were performed using R packages, including “TwoSampleMR” (Hemani et al., 2018), “MRPRESSO” (Verbanck et al., 2018)and “cause” (Morrison et al., 2020) in R software 3.6.0 (https://www.r-project.org/). The data visualization was conducted using R packages, including “TwoSampleMR” (Hemani et al., 2018) and “forestplot”.
The leave-on-out sensitivity analysis was performed to find the IV that might drive the main results, guaranteeing the MR results were robust.
Generally, our MR study indicated there might be no causal relationship between NAFLD and IBD although the genetic liability to IBD might elevate the LFC slightly. The number of IVs for each phenotype varied from 4 to 145 and each F statistic was greater than the empirical threshold 10, indicating less bias caused by weak instruments (Table 1).
Preliminarily, genetic predisposition to IBD could not elevate the risk of NAFLD (OR = 0.994 [0.970, 1.019], IVW p-value = 0.645), including two subtypes of IBD as UC (OR = 1.007 [0.952, 1.066], IVW p-value = 0.800) and CD (OR = 0.996 [0.986, 1.007], IVW p-value = 0.491) (Figure 2). After adjusting for BMI and T2D, the genetic predisposition to IBD could not affect the risk of NAFLD (OR = 1.057 [0.993, 1.125], IVW p-value = 0.082). Similar results were obtained for UC and CD in MVMR analysis as well (IVW p-value > 0.05). However, we observed a slight causal effect of IBD on LFC where genetically-elevated risk of IBD could lead to liver fat accumulation (beta = 0.019, se = 0.008, IVW p-value = 0.016). Considering LFC is a continuous variable, we used beta value to represent the effect size. It should be noted that the causal effect of IBD on LFC turned insignificant after Bonferroni correction (Bonferroni-corrected IVW p-value = 0.096). Besides, genetically-driven UC (beta = 0.010, se = 0.007, IVW p-value = 0.164) and CD (beta = 0.006, se = 0.007, IVW p-value = 0.363) could not alter LFC either (Figure 2). The impact of genetic predisposition to IBD on LFC was insignificant after adjusting for BMI and T2D (beta = -0.018, se = 0.010, p-value = 0.065). The MVMR results were similar in UC-LFC and CD-LFC associations (IVW p-value > 0.05). All pairs of causal association were insignificant in the MR-Egger regression and weighted-median method (Table 2). Although slight heterogeneity was detected for CD-NAFLD, CD-LFC and IBD-LFC pairs, the conclusions still held after removal of outliers. Also, genetic liability to CD could not affect the risk of NAFLD yet after correcting horizontal pleiotropy (OR = 0.024 [0.792, 1.008], MR-Egger p-value = 0.070).
FIGURE 2. Mendelian randomization (MR) results where non-alcoholic fatty liver disease and liver fat content as the outcomes. NAFLD is non-alcoholic fatty liver disease; LFC is liver fat content; CD is Crohn’s disease; IBD is inflammatory bowel disease; UC is ulcerative colitis; NSNP is the number of single nucleotide polymorphisms used in MR analysis; OR is odds ratio; 95% LCI is the lower limit of 95% confidence interval of OR/BETA; 95% UCI is the upper limit of 95% confidence interval of OR/BETA; SE is standard error; P is the p-value of OR/BETA. BETA is for the continuous variable LFC.
When treating NAFLD as the exposure, no causal association was detected, including IBD (OR = 0.968 [0.928, 1.009], IVW p-value = 0.123), UC (OR = 0.953 [0.878, 1.034], IVW p-value = 0.247) and CD (OR = 0.983 [0.935,1.034], IVW p-value = 0.516) (Figure 3). The MVMR suggested there was no causation between NAFLD and IBD (UC and CD) after adjusting for BMI and T2D (IBD OR = 1.024 [0.983, 1.066], IVW p-value = 0.255). Also, the liver fat accumulation appeared not to affect the risk of IBD (OR = 0.954 [0.861, 1.058], IVW p-value = 0.373), UC (OR = 0.961 [0.855, 1.081], IVW p-value = 0.511) or CD (OR = 0.932 [0.784, 1.109], IVW p-value = 0.426). The MVMR suggested genetically-predicted LFC could not alter the risk of IBD, UC or CD after adjusting for BMI and T2D (IVW p-value > 0.05). No significant association was observed in MR-Egger regression and weighted-median method (Table 2). No horizontal pleiotropy was detected and there existed heterogeneity in NAFLD-UC and LFC-CD pairs. Also, the conclusions still held after removing outliers.
FIGURE 3. Mendelian randomization (MR) results where non-alcoholic fatty liver disease and liver fat content as the exposures. NAFLD is non-alcoholic fatty liver disease; LFC is liver fat content; CD is Crohn’s disease; IBD is inflammatory bowel disease; UC is ulcerative colitis; NSNP is the number of single nucleotide polymorphisms used in MR analysis; OR is odds ratio; 95% LCI is the lower limit of 95% confidence interval of OR; 95% UCI is the upper limit of 95% confidence interval of OR; P is the p-value of OR.
The MR CAUSE analysis indicated the causal model did not hold in estimating the causal associations abovementioned as all p-values of causal model were greater than 0.05 (Table 3). However, it should be paid attention to that the direction of gamma values from CASUE were all positive in estimating causal associations if treating NAFLD and LFC as the outcomes (gamma >0) while these gamma values turned negative if treating IBD, UC and CD as the outcomes (gamma <0). These results suggested the order of disease initiation might lead to opposite outcomes unexpectedly.
After removal of outliers detected in MR-PRESSO, no SNP that might drive the results was identified in leave-one-out sensitivity analysis.
Although genetic liability to IBD might contribute to liver fat accumulation slightly, this MR study indicated there might be no causal link between NAFLD and IBD and it should be noted that the direction of NAFLD’s effect on IBD is negative, contrary to previous findings where NAFLD and IBD usually co-existed.
The observed causal effect of IBD on LFC might be a false positive one as this result was insignificant in both MR-Egger regression and weighted-median method. Besides, it was still insignificant in MR CAUSE analysis, a suitable method that can control false positive rate in MR analysis with consideration of both correlated and uncorrelated horizontal pleiotropy. However, we cannot completely rule out such causation as high-fat diet has impact on the quality of the intestinal barrier and the composition of the intestinal microbiome, influencing the pathogenesis of IBD (Ruemmele, 2016) and another study suggested LFC might be associated with UC as well (Jamali et al., 2017). Thus, further investigations should be carried out to elucidate such association.
As for the null associations, several reasons can be utilized to explain them. Metabolic syndrome, usually characterized by obesity, hyperglycemia, dyslipidemia and systemic hypertension, is currently the strongest risk factor of NAFLD (Friedman et al., 2018). Over 70% NAFLD patients are usually presented with high triglycerides (TG), high total cholesterol (TC), high low density lipoprotein cholesterol (LDL-C) and low high density lipoprotein cholesterol (HDL-C), most of which are primarily synthesized in liver, indicating dysregulated lipid metabolism in these patients, while the serum lipid profile is remarkably different in terms of serum TC and LDL-C in IBD patients at active stage, which can be rescued after intestinal surgery. Another study supported that less than 5% IBD patients are presented with dyslipidemia (Hoffmann et al., 2020), meanwhile, 25.0%–69.7% IBD patients at active stage developed malnutrition rather than obesity (Mijac et al., 2010). Therefore, we speculate that IBD brings out a specific metabolic state that might not be suitable for NAFLD.
Lean NAFLD is a special obesity resistant classification of NAFLD and believed to be with a distinct pathophysiological feature, characterized by higher serum secondary bile acid, increased expression of FGF19 and a shifted gut microbiota profile compared with non-lean NAFLD (Chen et al., 2020). IBD patients with NAFLD are often in absence of metabolic syndrome (Carr et al., 2017). There is still no report identifying the association between IBD and lean NAFLD. We cannot exclude that IBD and lean NAFLD have causal relationship, for no publicly available GWAS database can be utilized to address this problem until now.
Intriguingly, there was direct evidence illustrating that glucocorticoid for IBD could promote the initiation and progression of NAFLD instead of inhibiting NAFLD, and the use of azathioprine for CD was also determined as one risk factor for NAFLD (Woods et al., 2015). Similarly, bowel resection, a therapeutic strategy for severe IBD, was regarded as the risk factor for NAFLD in CD (Hoffmann et al., 2020), seemingly indicating inhibition of IBD could precipitate NAFLD. However, it is worthwhile to note that glucocorticoid as well as bowel surgery per se have the potential to result in liver steatosis or cholestasis, even without IBD history (Sasdelli et al., 2019). Thus, the observed co-existence of NAFLD and IBD might result from the effect of treatments, especially for the impact of IBD therapies on NAFLD (Restellini et al., 2017). Furthermore, Magri et al. reported there are no significant associations between NAFLD and IBD-related factors in IBD patients (Magrì et al., 2019). In a word, it is very difficult to demonstrate that IBD can contribute to the development of NAFLD based on previous studies.
On the other hand, accumulating evidence pointed to it that alteration of gut microbiota should have potential influence on the various risk factors of metabolic syndrome (Dabke et al., 2019). IBD is a chronic immunologically-mediated disease at the intersection of complex interactions between genetics, environment and gut microbiota (Ananthakrishnan, 2015), and gut microbiota have been reported to play an important role in pathogenesis of IBD (Franzosa et al., 2019). Metformin therapy for NAFLD could interact with gut microbiota (Vallianou et al., 2019) and so did the glucocorticoid therapy for IBD (Wu et al., 2018). Therefore, we postulate that gut microbiota may exert their effects on NAFLD and IBD concomitantly, and it can be comprehended as the pleiotropy of gut microbiota. As a result, NAFLD and IBD usually co-exist in clinical observation although there should be no causal relationship between them. Further investigations are needed to elucidate this hypothesis and corroborate our findings.
As no causal relationship was observed between NAFLD and IBD, it is still possible the underlying causal effect might be cancelled out due to opposite direct and indirect effects as omega 3 (ω3) fatty acids could alleviate intestinal inflammation (Marton et al., 2019). Therefore, it should be possible that the effects of “bad” lipids and “good” lipids can cancel out each other. Additionally, the negative results of MR study cannot completely rule out the causal relationship as the genetically-driven exposure cannot equals to the exposure and the negative results usually happen as the strict selection of IV.
As abovementioned, the pleiotropic effect of gut microbiota, impact of therapeutic treatments and opposite direct and indirect effects might help to explain the null causal relationship between NAFLD and IBD.
Our study has several strengths as follows: (Younossi et al., 2018) MR design was used to detect the causal relationship between and it could free this study from potential bias and reverse causation; (McHenry et al., 2019) both correlated and uncorrelated horizontal pleiotropy were controlled in this MR study; (McHenry et al., 2019) a bi-directional MR analysis was carried out to clarify the causation. However, some limitations should also be pointed out: (Younossi et al., 2018) horizontal pleiotropy should also be a major concern in MR study as various statistical methods fail to rule out horizontal pleiotropy caused by undetected biological mechanism; (Kaplan and Windsor, 2021) the proportion of NAFLD cases is relatively slow which might reduce the statistical power; (McHenry et al., 2019) the exclusion-restriction the selection might be violated as the binary phenotype was treated as the exposure due to data limitation; (Zou et al., 2019) the selection bias caused by competing risk factors could not be assessed as individual-level data was unavailable.
This MR study ruled out the causal relationship between IBD and NAFLD, suggesting therapeutics targeting NAFLD might not work for IBD and vice versa.
Publicly available datasets were analyzed in this study. This data can be found here: https://www.ebi.ac.uk/gwas/.
All analyses were based on summary-level GWAS statistics and all subjects are de-identified. Each GWAS was approved by its corresponding ethical committee and the summary statistics can be freely used without restriction. The patients/participants provided their written informed consent to participate in this study.
GL proposed the conception, designed the study and supervised the whole analysis. LC acquired the data and performed statistical analysis. LC drafted the article. ZF checked the statistical analysis and substantially revised the draft. XS and WQ read the whole manuscript and revised it. YC and JZ provided some necessary statistical suggestions and improved the English writing. All authors gave final approval of the version to be submitted.
The work is supported by National Natural Science Foundation of China (Grant No. 81602059) and Jilin Provincial Finance Department (Grant No. 2018SCZWSZX-042, 2018SCZWSZX-033).
The authors declare that the research was conducted in the absence of any commercial or financial relationships that could be construed as a potential conflict of interest.
All claims expressed in this article are solely those of the authors and do not necessarily represent those of their affiliated organizations, or those of the publisher, the editors and the reviewers. Any product that may be evaluated in this article, or claim that may be made by its manufacturer, is not guaranteed or endorsed by the publisher.
We would like to thank all investigators who made these GWAS summary statistics publicly available.
Ananthakrishnan, A. N. (2015). Epidemiology and Risk Factors for IBD. Nat. Rev. Gastroenterol. Hepatol. 12, 205–217. doi:10.1038/nrgastro.2015.34
Anstee, Q. M., Darlay, R., Cockell, S., Meroni, M., Govaere, O., Tiniakos, D., et al. (2020). Genome-wide Association Study of Non-alcoholic Fatty Liver and Steatohepatitis in a Histologically Characterised Cohort☆. J. Hepatol. 73, 505–515. doi:10.1016/j.jhep.2020.04.003
Bowden, J., Davey Smith, G., and Burgess, S. (2015). Mendelian Randomization with Invalid Instruments: Effect Estimation and Bias Detection through Egger Regression. Int. J. Epidemiol. 44, 512–525. doi:10.1093/ije/dyv080
Bowden, J., Davey Smith, G., Haycock, P. C., and Burgess, S. (2016). Consistent Estimation in Mendelian Randomization with Some Invalid Instruments Using a Weighted Median Estimator. Genet. Epidemiol. 40, 304–314. doi:10.1002/gepi.21965
Carr, R. M., Patel, A., Bownik, H., Oranu, A., Kerner, C., Praestgaard, A., et al. (2017). Intestinal Inflammation Does Not Predict Nonalcoholic Fatty Liver Disease Severity in Inflammatory Bowel Disease Patients. Dig. Dis. Sci. 62, 1354–1361. doi:10.1007/s10620-017-4495-0
Carreras-Torres, R., Ibáñez-Sanz, G., Obón-Santacana, M., Duell, E. J., and Moreno, V. (2020). Identifying Environmental Risk Factors for Inflammatory Bowel Diseases: a Mendelian Randomization Study. Sci. Rep. 10, 19273. doi:10.1038/s41598-020-76361-2
Chen, F., Esmaili, S., Rogers, G. B., Bugianesi, E., Petta, S., Marchesini, G., et al. (2020). Lean NAFLD: A Distinct Entity Shaped by Differential Metabolic Adaptation. Hepatology 71, 1213–1227. doi:10.1002/hep.30908
Cui, Z., Hou, G., Meng, X., Feng, H., He, B., and Tian, Y. (2020). Bidirectional Causal Associations between Inflammatory Bowel Disease and Ankylosing Spondylitis: A Two-Sample Mendelian Randomization Analysis. Front. Genet. 11, 587876. doi:10.3389/fgene.2020.587876
Dabke, K., Hendrick, G., and Devkota, S. (2019). The Gut Microbiome and Metabolic Syndrome. J. Clin. Invest. 129, 4050–4057. doi:10.1172/JCI129194
Davey Smith, G., and Hemani, G. (2014). Mendelian Randomization: Genetic Anchors for Causal Inference in Epidemiological Studies. Hum. Mol. Genet. 23, R89–R98. doi:10.1093/hmg/ddu328
de Lange, K. M., Moutsianas, L., Lee, J. C., Lamb, C. A., Luo, Y., Kennedy, N. A., et al. (2017). Genome-wide Association Study Implicates Immune Activation of Multiple Integrin Genes in Inflammatory Bowel Disease. Nat. Genet. 49, 256–261. doi:10.1038/ng.3760
Emdin, C. A., Khera, A. V., and Kathiresan, S. (2017). Mendelian Randomization. Jama 318, 1925–1926. doi:10.1001/jama.2017.17219
Franzosa, E. A., Sirota-Madi, A., Avila-Pacheco, J., Fornelos, N., Haiser, H. J., Reinker, S., et al. (2019). Gut Microbiome Structure and Metabolic Activity in Inflammatory Bowel Disease. Nat. Microbiol. 4, 293–305. doi:10.1038/s41564-018-0306-4
Friedman, S. L., Neuschwander-Tetri, B. A., Rinella, M., and Sanyal, A. J. (2018). Mechanisms of NAFLD Development and Therapeutic Strategies. Nat. Med. 24, 908–922. doi:10.1038/s41591-018-0104-9
Hemani, G., Zheng, J., Elsworth, B., Wade, K. H., Haberland, V., Baird, D., et al. (2018). The MR-Base Platform Supports Systematic Causal Inference across the Human Phenome. Elife 7. doi:10.7554/eLife.34408
Hemani, G., Tilling, K., and Davey Smith, G. (2017). Orienting the Causal Relationship between Imprecisely Measured Traits Using GWAS Summary Data. Plos Genet. 13, e1007081. doi:10.1371/journal.pgen.1007081
Hoffmann, P., Jung, V., Behnisch, R., and Gauss, A. (2020). Prevalence and Risk Factors of Nonalcoholic Fatty Liver Disease in Patients with Inflammatory Bowel Diseases: A Cross-Sectional and Longitudinal Analysis. World J. Gastroenterol. 26, 7367–7381. doi:10.3748/wjg.v26.i46.7367
Jamali, R., Biglari, M., Seyyed Hosseini, S. V., Shakouri Rad, A., and Kosari, F. (2017). The Correlation between Liver Fat Content and Ulcerative Colitis Disease Severity. Acta Med. Iran 55, 333–339.
Kaplan, G. G., and Windsor, J. W. (2021). The Four Epidemiological Stages in the Global Evolution of Inflammatory Bowel Disease. Nat. Rev. Gastroenterol. Hepatol. 18, 56–66. doi:10.1038/s41575-020-00360-x
Lin, A., Roth, H., Anyane-Yeboa, A., Rubin, D. T., and Paul, S. (2021). Prevalence of Nonalcoholic Fatty Liver Disease in Patients with Inflammatory Bowel Disease: A Systematic Review and Meta-Analysis. Inflamm. Bowel Dis. 27, 947–955. doi:10.1093/ibd/izaa189
Liu, Y., Basty, N., Whitcher, B., Bell, J. D., Sorokin, E. P., van Bruggen, N.., et al. (2021). Genetic Architecture of 11 Organ Traits Derived from Abdominal MRI Using Deep Learning. Elife, 10. doi:10.7554/elife.65554
Liu, Z., Zhang, Y., Graham, S., Wang, X., Cai, D., Huang, M., et al. (2020). Causal Relationships between NAFLD, T2D and Obesity Have Implications for Disease Subphenotyping. J. Hepatol. 73, 263–276. doi:10.1016/j.jhep.2020.03.006
Locke, A. E., Kahali, B., Berndt, S. I., Justice, A. E., Pers, T. H., Day, F. R., et al. (2015). Genetic Studies of Body Mass index Yield New Insights for Obesity Biology. Nature 518, 197–206. doi:10.1038/nature14177
Magrì, S., Paduano, D., Chicco, F., Cingolani, A., Farris, C., Delogu, G., et al. (2019). Nonalcoholic Fatty Liver Disease in Patients with Inflammatory Bowel Disease: Beyond the Natural History. World J. Gastroenterol. 25, 5676–5686. doi:10.3748/wjg.v25.i37.5676
Mahajan, A., Go, M. J., Zhang, W., Below, J. E., Gaulton, K. J., Mahajan, A., et al. (2014). Genome-wide Trans-ancestry Meta-Analysis Provides Insight into the Genetic Architecture of Type 2 Diabetes Susceptibility. Nat. Genet. 46, 234–244. doi:10.1038/ng.2897
Marton, L. T., Goulart, R. A., Carvalho, A. C. A., and Barbalho, S. M. (2019). Omega Fatty Acids and Inflammatory Bowel Diseases: An Overview. Int. J. Mol. Sci. 20. doi:10.3390/ijms20194851
McHenry, S., Sharma, Y., Tirath, A., Tsai, R., Mintz, A., Fraum, T. J., et al. (2019). Crohn's Disease Is Associated with an Increased Prevalence of Nonalcoholic Fatty Liver Disease: A Cross-Sectional Study Using Magnetic Resonance Proton Density Fat Fraction Mapping. Clin. Gastroenterol. Hepatol. 17, 2816–2818. doi:10.1016/j.cgh.2019.02.045
Mijac, D. D., Janković, G. L., Jorga, J., and Krstić, M. N. (2010). Nutritional Status in Patients with Active Inflammatory Bowel Disease: Prevalence of Malnutrition and Methods for Routine Nutritional Assessment. Eur. J. Intern. Med. 21, 315–319. doi:10.1016/j.ejim.2010.04.012
Morrison, J., Knoblauch, N., Marcus, J. H., Stephens, M., and He, X. (2020). Mendelian Randomization Accounting for Correlated and Uncorrelated Pleiotropic Effects Using Genome-wide Summary Statistics. Nat. Genet. 52, 740–747. doi:10.1038/s41588-020-0631-4
Piovani, D., Pansieri, C., Peyrin-Biroulet, L., Danese, S., and Bonovas, S. (2021). Confounding and Bias in Observational Studies in Inflammatory Bowel Disease: a Meta-Epidemiological Study. Aliment. Pharmacol. Ther. 53, 712–721. doi:10.1111/apt.16222
Restellini, S., Chazouillères, O., and Frossard, J. L. (2017). Hepatic Manifestations of Inflammatory Bowel Diseases. Liver Int. 37, 475–489. doi:10.1111/liv.13265
Ruemmele, F. M. (2016). Role of Diet in Inflammatory Bowel Disease. Ann. Nutr. Metab. 68 Suppl 1 (Suppl. 1), 33–41. doi:10.1159/000445392
Sasdelli, A. S., Agostini, F., Pazzeschi, C., Guidetti, M., Lal, S., and Pironi, L. (2019). Assessment of Intestinal Failure Associated Liver Disease According to Different Diagnostic Criteria. Clin. Nutr. 38, 1198–1205. doi:10.1016/j.clnu.2018.04.019
Vallianou, N. G., Stratigou, T., and Tsagarakis, S. (2019). Metformin and Gut Microbiota: Their Interactions and Their Impact on Diabetes. Hormones (Athens) 18, 141–144. doi:10.1007/s42000-019-00093-w
Verbanck, M., Chen, C. Y., Neale, B., and Do, R. (2018). Detection of Widespread Horizontal Pleiotropy in Causal Relationships Inferred from Mendelian Randomization between Complex Traits and Diseases. Nat. Genet. 50, 693–698. doi:10.1038/s41588-018-0099-7
Voight, B. F., Peloso, G. M., Orho-Melander, M., Frikke-Schmidt, R., Barbalic, M., Jensen, M. K., et al. (2012). Plasma HDL Cholesterol and Risk of Myocardial Infarction: a Mendelian Randomisation Study. Lancet 380, 572–580. doi:10.1016/S0140-6736(12)60312-2
Woods, C. P., Hazlehurst, J. M., and Tomlinson, J. W. (2015). Glucocorticoids and Non-alcoholic Fatty Liver Disease. J. Steroid Biochem. Mol. Biol. 154, 94–103. doi:10.1016/j.jsbmb.2015.07.020
Wu, T., Yang, L., Jiang, J., Ni, Y., Zhu, J., Zheng, X., et al. (2018). Chronic Glucocorticoid Treatment Induced Circadian Clock Disorder Leads to Lipid Metabolism and Gut Microbiota Alterations in Rats. Life Sci. 192, 173–182. doi:10.1016/j.lfs.2017.11.049
Younossi, Z., Anstee, Q. M., Marietti, M., Hardy, T., Henry, L., Eslam, M., et al. (2018). Global burden of NAFLD and NASH: Trends, Predictions, Risk Factors and Prevention. Nat. Rev. Gastroenterol. Hepatol. 15, 11–20. doi:10.1038/nrgastro.2017.109
Keywords: null association, causal inference, genetic epidemiology, mendelian randomization, NAFLD (non alcoholic fatty liver disease), IBD—inflammatory bowel disease
Citation: Chen L, Fan Z, Sun X, Qiu W, Chen Y, Zhou J and Lv G (2022) Mendelian Randomization Rules Out Causation Between Inflammatory Bowel Disease and Non-Alcoholic Fatty Liver Disease. Front. Pharmacol. 13:891410. doi: 10.3389/fphar.2022.891410
Received: 07 March 2022; Accepted: 05 April 2022;
Published: 19 May 2022.
Edited by:
Ana Blas-García, University of Valencia, SpainReviewed by:
Lorena Ortega Moreno, Autonomous University of Madrid, SpainCopyright © 2022 Chen, Fan, Sun, Qiu, Chen, Zhou and Lv. This is an open-access article distributed under the terms of the Creative Commons Attribution License (CC BY). The use, distribution or reproduction in other forums is permitted, provided the original author(s) and the copyright owner(s) are credited and that the original publication in this journal is cited, in accordance with accepted academic practice. No use, distribution or reproduction is permitted which does not comply with these terms.
*Correspondence: Guoyue Lv, bHZneUBqbHUuZWR1LmNu
†These authors have contributed equally to this work
Disclaimer: All claims expressed in this article are solely those of the authors and do not necessarily represent those of their affiliated organizations, or those of the publisher, the editors and the reviewers. Any product that may be evaluated in this article or claim that may be made by its manufacturer is not guaranteed or endorsed by the publisher.
Research integrity at Frontiers
Learn more about the work of our research integrity team to safeguard the quality of each article we publish.