- 1School of Pharmacy, Taylor’s University, Subang Jaya, Selangor, Malaysia
- 2Faculty of Pharmacy, University of Malaya, Kuala Lumpur, Malaysia
- 3International Institute for Halal Research and Training (INHART), International Islamic University Malaysia (IIUM), Gombak, Selangor, Malaysia
- 4Center for Drug Discovery and Molecular Pharmacology (CDDMP), School of Pharmacy, Taylor’s University, Subang Jaya, Selangor, Malaysia
- 5Infection Program and Department of Microbiology, Biomedicine Discovery Institute, Monash University, Clayton, VIC, Australia
- 6School of Medicine, Taylor’s University, Subang Jaya, Selangor, Malaysia
Multidrug-resistant (MDR) Klebsiella pneumoniae is a top-prioritized Gram-negative pathogen with a high incidence in hospital-acquired infections. Polymyxins have resurged as a last-line therapy to combat Gram-negative “superbugs”, including MDR K. pneumoniae. However, the emergence of polymyxin resistance has increasingly been reported over the past decades when used as monotherapy, and thus combination therapy with non-antibiotics (e.g., metabolites) becomes a promising approach owing to the lower risk of resistance development. Genome-scale metabolic models (GSMMs) were constructed to delineate the altered metabolism of New Delhi metallo-β-lactamase- or extended spectrum β-lactamase-producing K. pneumoniae strains upon addition of exogenous metabolites in media. The metabolites that caused significant metabolic perturbations were then selected to examine their adjuvant effects using in vitro static time–kill studies. Metabolic network simulation shows that feeding of 3-phosphoglycerate and ribose 5-phosphate would lead to enhanced central carbon metabolism, ATP demand, and energy consumption, which is converged with metabolic disruptions by polymyxin treatment. Further static time–kill studies demonstrated enhanced antimicrobial killing of 10 mM 3-phosphoglycerate (1.26 and 1.82 log10 CFU/ml) and 10 mM ribose 5-phosphate (0.53 and 0.91 log10 CFU/ml) combination with 2 mg/L polymyxin B against K. pneumoniae strains. Overall, exogenous metabolite feeding could possibly improve polymyxin B activity via metabolic modulation and hence offers an attractive approach to enhance polymyxin B efficacy. With the application of GSMM in bridging the metabolic analysis and time–kill assay, biological insights into metabolite feeding can be inferred from comparative analyses of both results. Taken together, a systematic framework has been developed to facilitate the clinical translation of antibiotic-resistant infection management.
1 Introduction
The emergence of multidrug-resistant (MDR) bacterial pathogens, including carbapenem-resistant Klebsiella pneumoniae, has garnered regular warnings of the World Health Organization (World Health Organization, 2020) and the U.S. Centers for Disease Control and Prevention (U.S. Centers for Disease Control and Prevention, 2021). Polymyxins (i.e., polymyxin B and colistin) are a group of lipopeptide antibiotics that are used as a last resort to treat severe infections caused by Gram-negative “superbugs”. Resistance can emerge during polymyxin monotherapy, which is mainly mediated by lipid A modifications in K. pneumoniae (Baron et al., 2016). Recently, the increasing prevalence of the mobile resistance gene mcr in Enterobacterales places critical challenges to polymyxin use (Liu et al., 2016; Yang et al., 2018; Hadjadj et al., 2019), underlining the urgent need for a novel antimicrobial therapeutic strategy. In clinics, colistin and polymyxin B are either used alone or in combination with other antimicrobials to treat life-threatening infections due to carbapenem-resistant K. pneumoniae (Nang et al., 2021; Yang et al., 2021). The emergence of polymyxin resistance in K. pneumoniae clinical isolates through diverse genetic adaptation has renewed the research focus on the importance of combination therapy. Furthermore, polymyxin dosage is limited by its nephrotoxicity and neurotoxicity (Aggarwal and Dewan, 2018). Combination therapies of polymyxin antibiotics are often employed to inhibit the resistance emergence and minimize the potential toxicity (Bergen et al., 2019). Among the combination treatments, using non-antibiotic adjuvants such as exogenous metabolites together with polymyxin B is a promising approach as the use of metabolites at low concentrations is generally non-toxic to the host (Chengxue et al., 2014; Zeng et al., 2017; Jiang et al., 2020; Rosenberg, Fang and Allison, 2020; Wang et al., 2020).
Recent studies have demonstrated that the cellular metabolism of bacterial pathogens is critical for antimicrobial efficacy (Liu et al., 2019). Modulation of cellular metabolism via exogenous metabolite feeding could significantly elevate antibiotic susceptibility of drug-resistant bacteria (Zeng et al., 2017; Su et al., 2018; Yang et al., 2019). However, the complicated interplay of multiple metabolic pathways underlying the synergy of metabolite–antimicrobial combination remains unclear, thus hampering the discovery of effective metabolite adjuvants to improve antimicrobial efficacy, including the last-line polymyxins. A genome-scale metabolic model (GSMM) serves as a systematic tool to simulate metabolic flux changes in response to antimicrobial treatment and metabolite feeding (Wadhwa et al., 2018; Rizvi et al., 2019; Zhou et al., 2021), and thus it can assist in delineating the mechanisms of enhanced bacterial killing by exogenous metabolite feeding.
The primary aim of this study was to identify promising polymyxin B–metabolite combinations against MDR K. pneumoniae using GSMM coupled with time–kill studies. Four GSMMs were constructed to elucidate the metabolic adaptation of K. pneumoniae strains upon addition of metabolites. We reveal that rewiring of metabolic flux distribution occurred owing to the feeding of additional metabolites. We also show that increased antimicrobial activity was demonstrated by the combination of 3-phosphoglycerate (3PG) and ribose 5-phosphate (R5P) with polymyxin B against New Delhi metallo-β-lactamase (NDM)- and extended spectrum β-lactamase (ESBL)-producing isolates.
2 Materials and methods
2.1 Bacterial isolates
Four K. pneumoniae American Type Culture Collection (ATCC) isolates were analyzed: ATCC 10031, 700603 (ST489, Pasteur scheme, same for following strains), 700721 (ST38, also known as K. pneumoniae MGH78578), and BAA-2146 (ST11). The strains were selected to represent a mixture of strains susceptible and resistant to polymyxin B (Table 1) and MDR strains. Strain ATCC 700603 was originally isolated from a urine sample of a hospitalized patient in 1994 (Elliott et al., 2016) and produces multiple ESBLs, especially beta-lactamase SHV-18. Strain ATCC BAA-2146 is an NDM-producing reference strain. All strains were purchased from ATCC and were stored in tryptone soy broth with 20% glycerol at −80°C.
2.2 Genome-scale metabolic modeling
The draft models were initially constructed by CarveMe (Machado et al., 2018) using genome annotation and coded in System Biology Markup Language Level 3 Version 1 (Smith et al., 2010). Manual curation and metabolic simulations were performed using COBRApy (Ebrahim et al., 2013). Transport and exchange reactions were added to allow nutrient uptake and metabolite transport across membranes according to the BiGG database (Norsigian et al., 2020). The manually added metabolites were complemented with specific properties including compartment localization, charge, formula, name, and database identifier according to the BiGG database.
For simulation of bacterial growth in minimal media (M9), the maximum uptake rates of nutrient ingredients were set to 10 mmol·gDW−1·h−1 (Zhu et al., 2018) whereas for Mueller–Hinton (MH) medium, the maximum uptake rates of nutrient ingredients were empirically constrained to 1 mmol·gDW−1·h−1 (Zhu et al., 2019). Non-growth-associated maintenance ATP consumption was set to 10 mmol·gDW−1·h−1 according to the previous study (Zhu et al., 2018).
Seven exogenous metabolites tested in this study are phenylpyruvate (PHPYR), orotate (OROT), 3-phosphohydroxypyruvate (3PHP), glycerol 3-phosphate (GLYC3P), 3PG, R5P, and uridine 5ʹ-diphospho-N-acetylglucosamine (UACGAM). The MH medium was used for metabolic modeling. For each metabolite, additional transport reactions were incorporated into the draft model, and the maximum uptake rate was constrained to 10 mmol·gDW−1·h−1. The metabolic solution space was sampled with 10,000 random points using OptGpSampler (Megchelenbrink, Huynen and Marchiori, 2014). Flux distributions of metabolite feeding were then compared with those of non-feeding conditions.
2.3 Antibiotic and exogenous metabolites
Polymyxin B was purchased from Merck (Darmstadt, Hesse) and was prepared by dissolving with Milli-Q water to obtain a final concentration of 512 mg/L. The exogenous metabolites (10 mM PHPYR, 1 mM OROT, 5 mM 3PHP, 10 mM 3PG, 10 mM R5P, and 1 mM UACGAM) were individually examined, alone and in combination with 2 mg/L polymyxin B against the four K. pneumoniae strains by static time–kill studies. The concentrations of exogenous metabolites were normalized to deliver 60 mM carbon except OROT, 3PHP, and 3PG due to their poor aqueous solubility. All metabolites were purchased from Sigma-Aldrich (Saint Louis, Missouri).
2.4 Static time–kill studies
Static time–kill studies were conducted over 24 h to study antimicrobial activity and the emergence of resistance after treatment with polymyxin B (Lin et al., 2019; Wistrand-Yuen et al., 2020). K. pneumoniae isolates were investigated at an initial inoculum of 106 CFU/ml [standard inoculum, as per the Clinical and Laboratory Standards Institute (CLSI) guidelines]. Log-phase cultures of K. pneumoniae isolates were prepared prior to the experiments.
Before spiking in antimicrobial agents, a sample of t = 0 h was collected. Clinically relevant free unbound concentration of polymyxin B 2 mg/L was used. After spiking in antimicrobial agents, further samples (∼700 μl) at t = 1, 4, and 24 h were collected aseptically, diluted appropriately in 0.9% saline solution, and plated manually. Upon incubation at 35°C for 24 h, viable cell counting was conducted. The final cell viability was expressed in log10 CFU/ml.
Polymyxin B exerted rapid bactericidal activity within 1 h, but significant bacterial regrowth was observed following 24 h exposure to polymyxin B monotherapy (Lin et al., 2019). Hence, the pharmacodynamic effect of the combination treatment was assessed over 24 h to investigate bacterial regrowth. Findings from polymyxin B pharmacokinetic studies suggest that the currently recommended mean polymyxin B maximum serum concentration at steady-state ranges from ∼2 to 14 mcg/ml (Avedissian et al., 2019). The polymyxin B concentrations selected were based on the clinical dosing regimens (Tsuji et al., 2019).
2.5 Pharmacodynamic analysis
Pharmacodynamic analysis was carried out to determine microbiological response to antimicrobial treatment (Lin et al., 2019). The log change method (log change = [log10 (CFUt)−log10 (CFU0)]) was used, comparing the change in bacterial count from 0 h to time point of interest. For static time–kill studies, antibacterial activity involves a reduction of ≥1 log10 CFU/ml from the initial inoculum. Bactericidal activity was defined as ≥ 3 log10 CFU/ml reduction from the starting inoculum. Additivity and synergy were defined as 1.0 to <2 log10 CFU/ml and ≥2 log10 CFU/ml reduction with the combination relative to its most active single agent, respectively (Sharma et al., 2017). Antagonism was defined as a ≥1 log10 CFU/ml increase between the combination and the most active single agent (Shields et al., 2018; Barber et al., 2021).
3 Results
3.1 Construction of genome-scale metabolic models for selected K. pneumoniae strains
With the aim of identifying promising metabolite adjuvants to increase antimicrobial activity of polymyxin B against K. pneumoniae, we have studied polymyxin-resistant strain ATCC 10031, polymyxin-susceptible strain ATCC 700721, and polymyxin-susceptible but MDR K. pneumoniae strains (ATCC 700603 and BAA-2146) (Table 1). In addition, GSMMs were constructed to simulate flux changes upon metabolite addition. Initial draft models were developed for the four K. pneumoniae isolates based on genome annotation. During manual curation against the literature and databases, a total of 10–12 metabolites and 20–23 reactions were added to each model (Supplementary Table S1), enabling metabolite uptake and secretion. The resulting models were designated iKpne_ATCC10031_21 (ATCC 10031), iKpne_ATCC700603_21 (ATCC 700603), iKpne_ATCCBAA2146_21 (ATCC BAA-2146), and iKpne_ATCC700721_21 (ATCC 700721) according to naming convention, and each of them contains 2,531–2,713 reactions, 1,695–1,778 metabolites, and 1,292–1,612 genes (Table 2).
3.2 Genome-scale metabolic modeling
The four models predicted the maximum specific growth rate (μmax) of 0.92 and 1.05 h−1 in M9 and MH media, respectively. The predicted μmax in MH media is similar to the calculated μmax using time–kill data, which varied between 1.02–1.16 h−1 for the K. pneumoniae isolates.
The metabolites were selected based on the previous transcriptomic and metabolomic findings (Maifiah et al., 2017; Han et al., 2018; Hussein et al., 2018; Abdul Rahim et al., 2021), which indicated that the intracellular levels of metabolites R5P, UACGAM, and GLYC3P were significantly perturbed by polymyxin. Furthermore, metabolites PHPYR, OROT, 3PG, and 3PHP have also been identified as significant metabolites perturbed by the combination (Abdul Rahim et al., 2021). Although many significant metabolites were identified from the studies, the selected metabolites were those that demonstrated perturbations to both gene expression and metabolism of the same pathway [e.g., gnd and R5P in the pentose phosphate pathway (PPP); pgk and 3PG in gluconeogenesis] by the combination (Abdul Rahim et al., 2020; 2021). For instance, transcriptomics and metabolomic results revealed that the expression of gene gnd and abundance level of R5P were downregulated and decreased in response to the polymyxin combination treatment, respectively. Thus, these observations were believed to further strengthen the basis of selection.
GSMM simulation results show that the addition of PHPYR, OROT, and 3PHP resulted in limited impact on non-central metabolic pathways, whereas feeding of 3PG, GLYC3P, R5P, and UACGAM induced significant metabolic perturbations to multiple pathways, including central metabolism (Figure 1). GLYC3P was excluded for further analyses due to its similar impact as 3PG. The perturbed reaction specific flux values under control and metabolite feeding treatment are denoted in the format fluxcontrol/fluxmetabolite in brackets in Sections 3.2.1, 3.2.2.
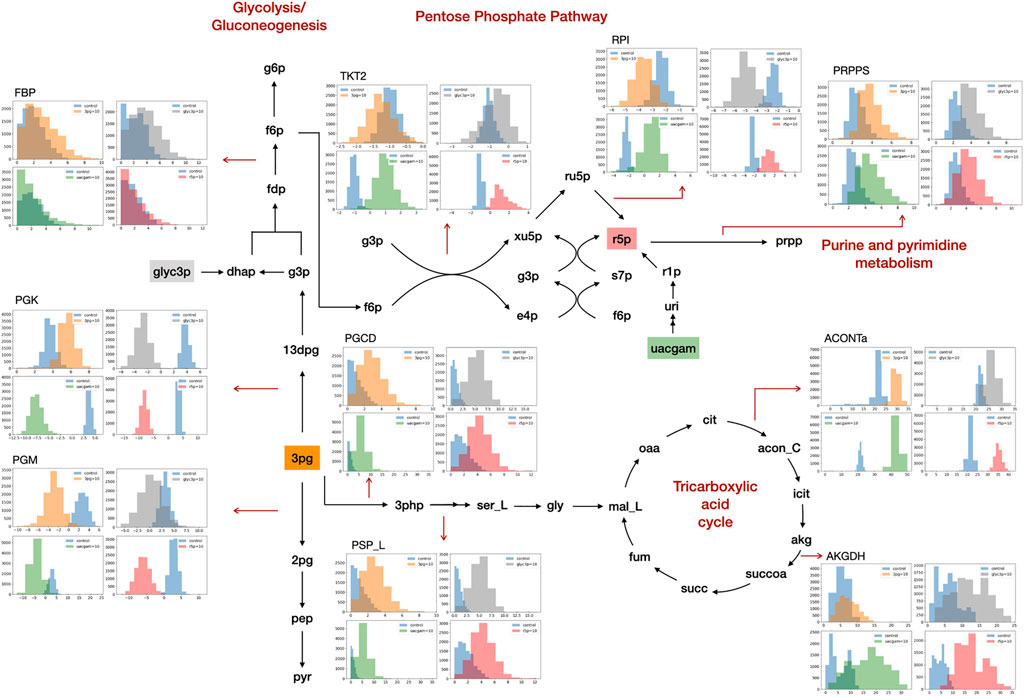
FIGURE 1. Metabolite feeding of 3PG, GLYC3P, R5P, and UACGAM induced metabolic alterations. The subgraphs indicate the distribution of sampled metabolic fluxes (mmol·gDW−1·h−1) in iKpne_ATCC700603_21 (blue, control; orange, 3PG; gray, GLYC3P; red, R5P; green, UACGAM). The metabolite abbreviations are as follows: g6p, D-glucose 6-phosphate; f6p, D-fructose 6-phosphte; fdp, D-fructose 1,6-biphosphate; dhap, dihydroxyacetone phosphate; g3p, glyceraldehyde 3-phosphate; 13dpg, 3-phospho-D-glyceroyl phosphate; 3pg, 3-phosphoglycerate; 2pg, D-glycerate 2-phosphate; pep, phosphoenolpyruvate; pyr, pyruvate; ru5p, D-ribulose 5-phosphate; xu5p, D-xylulose 5-phosphate; r5p, D-ribose 5-phosphate; s7p, sedoheptulose 7-phosphate; e4p, D-erythrose 4-phosphate; prpp, 5- phospho-alpha-D-ribose 1-diphosphate; 3php, 3-phosphohydroxypyruvate; ser_L, L-serine; gly, glycine; mal_L, L-malate; oaa, oxaloacetate; cit, citrate; acon_C, cis-aconitate; icit, isocitrate; akg, 2-oxoglutarate; succoa, succinyl-CoA; succ, succinate; fum, fumarate. The reaction abbreviations are as follows: FBP, fructose-bisphosphatase; PGK, phosphoglycerate kinase; PGM, phosphoglycerate mutase; TKT2, transketolase 2; RPI, ribose-5-phosphate isomerase; PRPPS, phosphoribosylpyrophosphate synthetase; PGCD, phosphoglycerate dehydrogenase; PSP_L, phosphoserine phosphatase; ACONTa, aconitase (half-reaction A); AKGDH, 2-oxoglutarate dehydrogenase.
3.2.1 Metabolic impact on non-central metabolism
The model simulations predict that the uptake of exogenous PHPYR was at a relatively low rate compared to that of other metabolites and exerted minimal effect on phenylalanine metabolism upon feeding. Generally, GSMM results show that the addition of OROT would increase pyrimidine biosynthesis. A higher flux distribution of orotate phosphoribosyltransferase (ORPT) (iKpne_ATCC10031_21: 0.41/0.54; iKpne_ATCC700603_21: 0.20/0.43; iKpne_ATCC700721_21: 0.22/0.48; and iKpne_ATCCBAA2146_21: 0.19/0.42), orotidine 5′-phosphate decarboxylase (OMPDC) (iKpne_ATCC10031_21: 0.41/0.54; iKpne_ATCC700603_21: 0.20/0.43; iKpne_ATCC700721_21: 0.22/0.48; and iKpne_ATCCBAA2146_21:0.19/0.42), and uridine 5′-monophosphate kinase (UMPK) (iKpne_ATCC10031_21: 1.67/1.76; iKpne_ATCC700603_21: 2.64/2.87; iKpne_ATCC700721_21: 2.57/2.58; and iKpne_ATCCBAA2146_21: 2.59/2.65) indicated elevated pyrimidine biosynthesis activity. Uridine diphosphate (UDP) was further converted to uridine-5′-triphosphate (UTP) via higher flux through nucleoside-diphosphate kinase (NDPK2) (iKpne_ATCC10031_21: 5.99/6.17; iKpne_ATCC700603_21: 4.92/5.20; iKpne_ATCC700721_21: 4.65/4.55; and iKpne_ATCCBAA2146_21: 5.18/5.14). Moreover, the addition of exogenous 3PHP was predicted to digest into serine and glycine metabolism to increase fluxes of phosphoserine transaminase (PSERT) (iKpne_ATCC10031_21: 2.29/11.43; iKpne_ATCC700603_21: 0.89/10.67; iKpne_ATCC700721_21: 1.47/10.62; and iKpne_ATCCBAA2146_21: 1.29/10.57), phosphoserine phosphatase (PSP_L) (iKpne_ATCC10031_21: 2.29/11.43; iKpne_ATCC700603_21: 0.89/10.67; iKpne_ATCC700721_21: 1.47/10.62; and iKpne_ATCCBAA2146_21: 1.29/10.57), and then glycine hydroxymethyltransferase (GHMT2r) (iKpne_ATCC10031_21: 1.11/6.00; iKpne_ATCC700603_21: 2.15/4.33; iKpne_ATCC700721_21: 1.27/4.98; and iKpne_ATCCBAA2146_21: 0.90/6.04) to form glycine.
3.2.2 Metabolic impact on central metabolism
GSMM results show that feeding of 3PG resulted in increased glycolytic/gluconeogenetic fluxes in all four strains (Figure 1). Results show that 3PG influx bifurcates to form D-glycerate 2-phosphate (2PG) of glycolysis and 3-phospho-D-glyceroyl phosphate (13DPG) of gluconeogenesis; the latter in turn enhances PPP flux to generate R5P. Results show enhanced production of 5-phospho-alpha-D-ribose 1-diphosphate (PRPP), the starting metabolite of the nucleotide biosynthesis pathway (Figure 1) and increased fluxes of reactions ORPT, OMPDC, UMPK, and NDPK2 toward UTP biosynthesis. Furthermore, addition of 3PG was predicted to increase serine biosynthesis via enhanced fluxes of PSERT (iKpne_ATCC10031_21: 2.29/5.39; iKpne_ATCC700603_21: 0.89/3.70; iKpne_ATCC700721_21: 1.47/3.85; and iKpne_ATCCBAA2146_21: 1.29/4.43) and PSP_L (iKpne_ATCC10031_21: 2.29/5.39; iKpne_ATCC700603_21: 0.89/3.70; iKpne_ATCC700721_21: 1.47/3.85; and iKpne_ATCCBAA2146_21: 1.29/4.43). Increased tricarboxylic acid cycle (TCA) cycle flux was observed upon feeding of 3PG. Additionally, the overall fluxes within oxidative phosphorylation were increased (Figure 2) which potentially resulted in higher oxygen consumption and a higher ATP turnover rate.
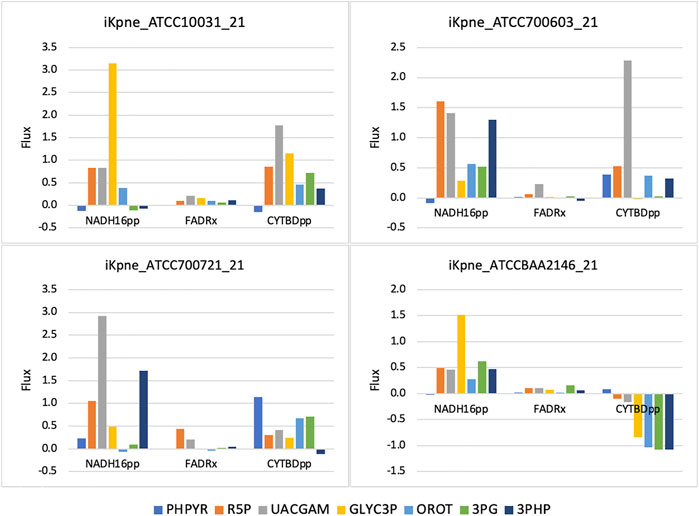
FIGURE 2. Oxidative phosphorylation fluxes changes upon metabolite addition. The reaction abbreviations are as follows: NADH16pp, NADH dehydrogenase (ubiquinone-8 and 3 protons) (periplasm); FADRx, FAD reductase; CYTBDpp, cytochrome oxidase bd (ubiquinol-8: 2 protons) (periplasm).
Furthermore, the GSMM predicted the exogenous GLYC3P formed dihydroxyacetone phosphate (DHAP) through enhanced dehydrogenation (iKpne_ATCC10031_21: 4.15/4.26; iKpne_ATCC700603_21: −4.92/1.80; iKpne_ATCC700721_21: −3.92/3.95; and iKpne_ATCCBAA2146_21: −4.15/3.06), which in turn flew down to glycolysis, serine metabolism, and eventually to the TCA cycle (Figure 1). The metabolic flux changes caused by GLYC3P feeding are similar to 3PG feeding. In addition, feeding of R5P was predicted to significantly affect central carbon metabolism flux. The addition of R5P would preferably form D-ribulose 5-phosphate (RU5P) than PRPP via isomerization (iKpne_ATCC10031_21: −0.44/5.80; iKpne_ATCC700603_21: −2.42/1.48; iKpne_ATCC700721_21: −2.59/0.54; and iKpne_ATCCBAA2146_21: 2.56/1.19). Increased flux of generating fructose 6-phosphate (F6P) from RU5P would enter glycolysis metabolism, and then the end product of glycolysis, acetyl CoA, would be fueled to the TCA cycle for cellular respiration. Furthermore, the GSMM results also reveal that feeding of UACGAM increases the fluxes of central and nucleotide metabolism. The exogenous UACGAM flows into PPP through the nucleotide salvage pathway (Figure 1) via increased flux of pyrimidine-nucleoside phosphorylase (iKpne_ATCC700603_21: −1.42/6.54; iKpne_ATCC700721_21: −1.32/6.40; and iKpne_ATCCBAA2146: −1.00/6.91) except for iKpne_ATCC10031_21. Model iKpne_ATCC10031 predicted exogenous UACGAM digested into PPP via increased flux of uridine hydrolase (URIH) (iKpne_ATCC10031_21: 0.44/9.85).
3.3 Validation of metabolite effects using in vitro time–kill studies
Polymyxin B (2 mg/L) monotherapy produced rapid and extensive killing within 1 h against all isolates except ATCC 10031 with ≥3 log10 CFU/ml killing (Figures 3A,B). Nevertheless, significant bacterial regrowth was observed at 24 h for all isolates treated with polymyxin B monotherapy.
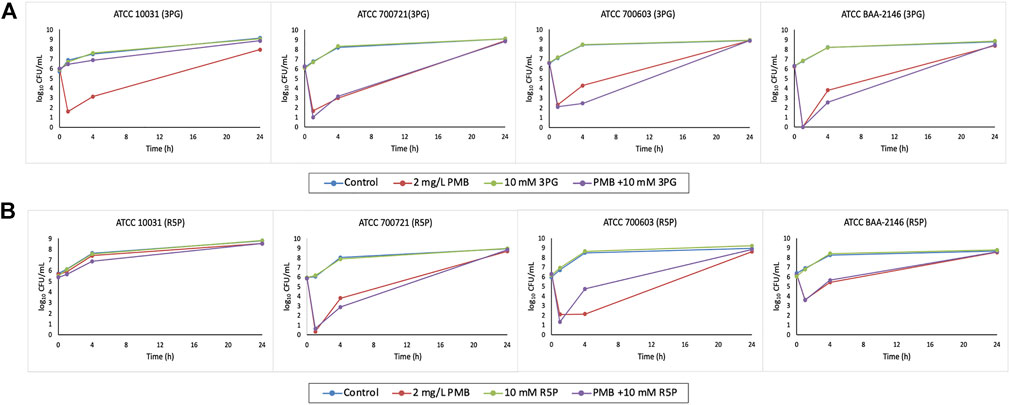
FIGURE 3. Time–kill curves of metabolite treatment with polymyxin B (PMB), alone and in combination. (A) 10 mM 3PG and (B) 10 mM R5P.
For the six metabolites tested, three metabolite–polymyxin B combinations demonstrated enhanced antimicrobial activity against MDR K. pneumoniae isolates even when NDM was present.
The combination of polymyxin B (2 mg/L) with 10 mM 3PG resulted in strong bacterial killing at 1 h with 4.3–6.2 log10 CFU/ml reduction for isolate ATCC 700603 and BAA-2146 compared to initial inoculum (Figure 3A). At 4 h, the combination treatment increased the extent of antibacterial activity approximately to 2 log10 CFU/ml (1.82 log10 CFU/ml) reduction for isolate ATCC 700603 relative to its most active polymyxin B monotherapy (Figure 3A). A similar increased antibacterial effect was also observed for the combination treatment against MDR isolate BAA-2146 with 1.26 log10 CFU/ml reduction at 4 h (Figure 3A). However, bacterial regrowth was observed for both isolates at 24 h.
Metabolite feeding with 10 mM R5P combined with polymyxin B showed a bacterial count reduction of approximately 1 log10 CFU/ml (0.91 log10 CFU/ml) for isolate ATCC 700721 (Figure 3B). Interestingly, isolate ATCC 10031 is resistant to polymyxin B monotherapy and the addition of R5P to polymyxin B resulted in a modest improvement in antibacterial activity with 0.53 log10 CFU/ml reduction compared with polymyxin B monotherapy at 4 h (Figure 3B).
The antibacterial effect of UACGAM feeding was also tested against MDR isolates. For ESBL isolate ATCC 700603, addition of 1 mM UACGAM to polymyxin B treatment showed an increase of bacterial killing of 1 log10 CFU/ml reduction. The magnitude of antibacterial activity was further enhanced to 0.70 log10 CFU/ml reduction at 4 h in contrast to polymyxin B monotherapy (Supplementary Figure S1).
4 Discussion
The rapid spread of opportunistic K. pneumoniae that are resistant to last-resort polymyxins highlights the urgent requirement for novel antimicrobial adjuvant therapy to minimize the emergence of resistance. Polymyxin B combined with non-antibiotics, such as metabolites, offers an attractive approach to increase antibacterial activity without exceeding the clinically achieved concentration of polymyxin B. To this end, it is crucial to understand the reciprocal relationship between bacterial metabolic responses to exogenous metabolites and antimicrobial activity to optimize the combination therapy. GSMM is a powerful tool in studying bacterial metabolism, and it has been applied to elucidate the mechanism of antibiotic killing and development of resistance. Thus, integration with in vitro experiments enables a systematic framework for identifying novel exogenous metabolite–antibiotic combinations.
Simulation with the four GSMMs showed that additions of exogenous metabolites such as 3PG, 3PHP, GLYC3P, R5P, and UACGAM display an effect on increasing bacterial growth except for metabolites PHPYR and OROT. This could be explained by the flow of metabolic flux corresponding to the metabolite addition in which metabolite PHPYR was not digested in the metabolism; OROT addition only exerted minor effects on purine and pyrimidine metabolism. The highest growth induced by UACGAM feeding among the metabolites demonstrated the highest metabolic flux changes in model predictions. The uridine part of UACGAM can be digested to form nucleotides, whereas the amino sugar component (i.e., N-acetylglucosamine) can be utilized for cell envelope biosynthesis.
The growth rate is the primary variable that determines the phenotype of susceptibility to antibiotics of the bacterial populations (Martínez and Rojo, 2011). A slow growth rate was associated with low antibiotic activity (Yang, Bening and Collins, 2017; Zampieri et al., 2017; Lee et al., 2018). Thus, we hypothesize that the stagnant bacterial growth upon feeding of PHPYR and OROT would not exert antibacterial activity when treated together with polymyxin B against K. pneumoniae isolates. The time–kill studies supported this hypothesis where both combination therapies (i.e., polymyxin B with PHPYR; polymyxin B with OROT) did not show effect on antibacterial activity. In addition, minor metabolic flux changes in glucose metabolism and oxidative phosphorylation displayed by feeding of these two metabolites suggest that no metabolic regulation and modulation occur.
Prax et al. (2016) showed that glucose potentiated a membrane-active antimicrobial peptide, daptomycin, in which killing may be dependent on glucose metabolism. The attenuation of carbon catabolism associated with cellular respiration is the primary cause of metabolite-driven ciprofloxacin activity (Gutierrez et al., 2017). Recent metabolomics results showed polymyxin treatment-induced dramatic changes in central carbon metabolism in polymyxin-susceptible Gram-negative pathogens (Maifiah et al., 2017; Zhu et al., 2019). Our fluxomic data revealed that metabolite feeding of 3PG, R5P, UACGAM, and GLYC3P notably increased glycolysis, PPP, and TCA cycle fluxes. It is conceivable that exogenous metabolite feeding would further intensify the metabolic burden attributed to polymyxin B activity and cause increased cellular respiration. On top of that, polymyxin treatment also induced disruption of nucleotide biosynthesis (Zhu et al., 2018). In silico addition of the aforementioned four metabolites also upregulated purine and pyrimidine metabolism. Our time–kill result showed enhanced antimicrobial killing by the combination of 3PG, R5P, and UACGAM treated along with polymyxin B against K. pneumoniae (Figures 3A,B; Supplementary Figure S1). These results indicate that the surge of ATP is required to restore the disrupted nucleotide pool because of both antibiotic and metabolite treatments (Yang et al., 2019). The enhanced ATP demand stimulates the nucleotide biosynthesis metabolism and elevates the central carbon metabolism. The increased metabolic activity by metabolite feeding is likely to produce toxic metabolic by-products that reduce bacterial fitness (Stokes et al., 2019), hence increasing the killing effect of polymyxin B.
Another possible mechanism of metabolite feeding is increased production of reactive oxygen species (ROS) to enhance antibiotic activity (Brynildsen et al., 2013; Van Acker and Coenye, 2017). Increasing ROS production would increase bacterial sensitivity to oxidative attack (Brynildsen et al., 2013). The mechanism of polymyxin action involves free radical-induced death (Trimble et al., 2016). Abdul Rahim et al. (2021) postulated that an increase in nucleotide synthesis including R5P and OROT was an initial bacterial stress response to polymyxin combination treatment (Abdul Rahim et al., 2021). Such metabolic perturbation might be exacerbated driven by TCA activity upon metabolite feeding. Our results showed that metabolite feeding upregulated the TCA cycle and produced NADH which is utilized for facilitating the electron transport chain. This would induce the formation of ROS and cause oxidative damage, contributing to lethality. Altogether, the increased fluxes of NADH16pp, FADRx, and CYTBDpp (Figure 2), inducing an oxidative stress and concurrently increasing metabolic activity by metabolite feeding, may sensitize K. pneumoniae to polymyxin B killing.
For polymyxin B-resistant isolate ATCC 10031, evident in time–kill studies, the addition of metabolite R5P to polymyxin B resulted in a slight improvement (log change = 0.52 log10 CFU/ml) in antibacterial activity at 4 h compared with polymyxin B monotherapy (Figure 3B). This suggests that metabolite feeding may be a possible approach to restore antibiotic susceptibility of antibiotic-resistant isolates. Antibiotic-resistant strains are generally demonstrated to have weaker bacterial fitness and reduced metabolism due to evolution of mutation under selection pressure of antibiotics (Lázár et al., 2014). The addition of exogenous metabolites to restore the metabolic deprivation offers a hopeful approach to increase sensitivity to antibiotics of antibiotic-resistant bacteria (Cheng et al., 2019; Li et al., 2020). This enables better antimicrobial activity to be achieved with combinations containing clinically relevant polymyxin B concentrations given that polymyxin B-induced nephrotoxicity is a dose-limiting adverse effect (Avedissian et al., 2019).
Despite the positive antimicrobial effect of the combination treatment, an antagonistic effect was observed for the combination of 3PG and R5P with polymyxin B against ATCC 10031 and ATCC 700603, respectively (Figures 3A,B). Although the underlying mechanisms of these antagonism pairs remain unclear, they could be considered a potential target for the development of new antimicrobial therapy for these K. pneumoniae isolates. Alteration of related metabolic processes could thereby lead to a reversal of the antagonistic effect, thus improving the susceptibility of antibiotics. It would be interesting to investigate the metabolic perturbations in gene expression and metabolism in the K. pneumoniae isolates driven by the combination.
In summary, this is the first study incorporating GSMM findings to unveil mechanistic insights into metabolic flux changes following metabolite addition, correlated with antibiotic activity through in vitro studies. This will shed light on antimicrobial development of non-antibiotic combinations with polymyxin B to rescue the last-line resort. Further studies into transcriptomics and metabolomics analysis to delineate the complex metabolic responses to metabolite feeding are warranted for better model validation and accuracy. Apart from that, in vivo studies are crucial to evaluate the efficacy, concentration, and safety of metabolite adjuvants used in potentiating antibiotic activity against MDR K. pneumoniae infections.
Data availability statement
The original contributions presented in the study are included in the article/Supplementary Material; further inquiries can be directed to the corresponding authors.
Author contributions
WEH, NAR, and YZ supervised and designed this project. CWY performed the experiment and drafted the manuscript. All authors reviewed the manuscript and approved it for publication.
Funding
This work was supported by the Fundamental Research Grant Scheme, Ministry of Higher Education, Malaysia (FRGS/1/2019/SKK11/TAYLOR/03/1).
Conflict of interest
The authors declare that the research was conducted in the absence of any commercial or financial relationships that could be construed as a potential conflict of interest.
Publisher’s note
All claims expressed in this article are solely those of the authors and do not necessarily represent those of their affiliated organizations, or those of the publisher, the editors, and the reviewers. Any product that may be evaluated in this article, or claim that may be made by its manufacturer, is not guaranteed or endorsed by the publisher.
Supplementary material
The Supplementary Material for this article can be found online at: https://www.frontiersin.org/articles/10.3389/fphar.2022.880352/full#supplementary-material
References
Abdul Rahim, N., Cheah, S. E., Johnson, M. D., Zhu, Y., Yu, H. H., Sidjabat, H. E., et al. (2020). Transcriptomic responses of a New Delhi metallo-β-lactamase-producing Klebsiella pneumoniae isolate to the combination of polymyxin B and chloramphenicol. Int. J. Antimicrob. Agents 56 (2), 106061. doi:10.1016/J.IJANTIMICAG.2020.106061
Abdul Rahim, N., Zhu, Y., Cheah, S. E., Johnson, M. D., Yu, H. H., Sidjabat, H. E., et al. (2021). Synergy of the polymyxin-chloramphenicol combination against New Delhi Metallo-b-lactamase-producing Klebsiella pneumoniae is predominately driven by chloramphenicol. ACS Infectious Diseases 7 (6), 1584–1595. doi:10.1021/acsinfecdis.0c00661
Aggarwal, R., and Dewan, A. (2018). Comparison of nephrotoxicity of colistin with polymyxin B administered in currently recommended doses: A prospective study. Ann. Clin. Microbiol. Antimicrob. 17 (1), 15. doi:10.1186/s12941-018-0262-0
Avedissian, S. N., Liu, J., Rhodes, N. J., Lee, A., Pais, G. M., Hauser, A. R., et al. (2019). A review of the clinical pharmacokinetics of polymyxin B. Antibiotics 8 (1), E31. doi:10.3390/antibiotics8010031
Barber, K. E., Shammout, Z., Smith, J. R., Kebriaei, R., Morrisette, T., and Rybak, M. J. (2021). Biofilm time-kill curves to assess the bactericidal activity of daptomycin combinations against biofilm-producing vancomycin-resistant Enterococcus faecium and faecalis. Antibiotics 10 (8), 897. doi:10.3390/ANTIBIOTICS10080897
Baron, S., Hadjadj, L., Rolain, J. M., and Olaitan, A. O. (2016). Molecular mechanisms of polymyxin resistance: Knowns and unknowns. Int. J. Antimicrob. Agents 48 (6), 583–591. doi:10.1016/J.IJANTIMICAG.2016.06.023
Bergen, P. J., Smith, N. M., Bedard, T. B., Bulman, Z. P., Cha, R., and Tsuji, B. T. (2019). Rational combinations of polymyxins with other antibiotics. Adv. Exp. Med. Biol. 1145, 251–288. doi:10.1007/978-3-030-16373-0_16
Brynildsen, M. P., Winkler, J. A., Spina, C. S., MacDonald, I. C., and Collins, J. J. (2013). Potentiating antibacterial activity by predictably enhancing endogenous microbial ROS production. Nat. Biotechnol. 31 (2), 160–165. doi:10.1038/NBT.2458
Cheng, Z., Guo, C., Chen, Z. G., Yang, T. C., Zhang, J. Y., Wang, J., et al. (2019). Glycine, serine and threonine metabolism confounds efficacy of complement-mediated killing. Nat. Commun. 10 (11), 3325. doi:10.1038/s41467-019-11129-5
Chengxue, Z., Ma, Y. m., Li, H., and Peng, X. x. (2014). N-acetylglucosamine enhances survival ability of tilapias infected by Streptococcus iniae. Fish. Shellfish Immunol. 40 (2), 524–530. doi:10.1016/j.fsi.2014.08.008
Ebrahim, A., Lerman, J. A., Palsson, B. O., and Hyduke, D. R. (2013). COBRApy: COnstraints-based reconstruction and analysis for Python. BMC Syst. Biol. 7 (11), 74. doi:10.1186/1752-0509-7-74
Elliott, A. G., Ganesamoorthy, D., Coin, L., Cooper, M. A., and Cao, M. D. (2016). Complete genome sequence of Klebsiella quasipneumoniae subsp. similipneumoniae strain ATCC 700603. Genome Announc. 4 (3), e00438–16. doi:10.1128/GENOMEA.00438-16
Gutierrez, A., Jain, S., Bhargava, P., Hamblin, M., Lobritz, M. A., and Collins, J. J. (2017). Understanding and sensitizing density-dependent persistence to quinolone antibiotics. Mol. Cell 68 (6), 1147–1154. e3. doi:10.1016/j.molcel.2017.11.012
Hadjadj, L., Baron, S. A., Olaitan, A. O., Morand, S., and Rolain, J. M. (2019). Co-occurrence of variants of mcr-3 and mcr-8 genes in a Klebsiella pneumoniae isolate from Laos. Front. Microbiol. 10, 2720. doi:10.3389/fmicb.2019.02720
Han, M. L., Liu, X., Velkov, T., Lin, Y. W., Zhu, Y., Li, M., et al. (2018). Metabolic analyses revealed time-dependent synergistic killing by colistin and aztreonam combination against multidrug-resistant Acinetobacter baumannii. Front. Microbiol. 9, 2776. doi:10.3389/fmicb.2018.02776
Hussein, M., Han, M. L., Zhu, Y., Schneider-Futschik, E. K., Hu, X., Zhou, Q. T., et al. (2018). Mechanistic insights from global metabolomics studies into synergistic bactericidal effect of a polymyxin B combination with tamoxifen against cystic fibrosis MDR Pseudomonas aeruginosa. Comput. Struct. Biotechnol. J. 16, 587–599. doi:10.1016/J.CSBJ.2018.11.001
Jiang, M., Yang, L., Chen, Z. G., Lai, S. S., Zheng, J., and Peng, B. (2020). Exogenous maltose enhances Zebrafish immunity to levofloxacin-resistant Vibrio alginolyticus. Microb. Biotechnol. 13 (4), 1213–1227. doi:10.1111/1751-7915.13582
Lázár, V., Nagy, I., Spohn, R., Csorgo, B., Gyorkei, A., Nyerges, A., et al. (2014). Genome-wide analysis captures the determinants of the antibiotic cross-resistance interaction network. Nat. Commun. 5 (1), 4352. doi:10.1038/ncomms5352
Lee, A. J., Wang, S., Meredith, H. R., Zhuang, B., Dai, Z., and You, L. (2018). Robust, linear correlations between growth rates and β-lactam-mediated lysis rates. Proc. Natl. Acad. Sci. U. S. A. 115 (16), 4069–4074. doi:10.1073/pnas.1719504115
Li, L., Su, Y. B., Peng, B., Peng, X. X., and Li, H. (2020). Metabolic mechanism of colistin resistance and its reverting in Vibrio alginolyticus. Environ. Microbiol. 22 (10), 4295–4313. doi:10.1111/1462-2920.15021
Lin, Y. W., Abdul Rahim, N., Zhao, J., Han, M. L., Yu, H. H., Wickremasinghe, H., et al. (2019). Novel polymyxin combination with the antiretroviral zidovudine exerts synergistic killing against NDM-producing multidrug-resistant Klebsiella pneumoniae. Antimicrob. Agents Chemother. 63 (4), e02176–18. PDF. doi:10.1128/AAC.02176-18
Liu, Y., Li, R., Xiao, X., and Wang, Z. (2019). Bacterial metabolism-inspired molecules to modulate antibiotic efficacy. J. Antimicrob. Chemother. 74, 3409–3417. Article in. doi:10.1093/jac/dkz230
Liu, Y. Y., Wang, Y., Walsh, T. R., Yi, L. X., Zhang, R., Spencer, J., et al. (2016). Emergence of plasmid-mediated colistin resistance mechanism MCR-1 in animals and human beings in China: a microbiological and molecular biological study. Lancet. Infect. Dis. 16 (2), 161–168. doi:10.1016/S1473-3099(15)00424-7
Machado, D., Andrejev, S., Tramontano, M., and Patil, K. R. (2018). Fast automated reconstruction of genome-scale metabolic models for microbial species and communities. Nucleic Acids Res. 46 (15), 7542–7553. doi:10.1093/NAR/GKY537
Maifiah, M. H. M., Creek, D. J., Nation, R. L., Forrest, A., Tsuji, B. T., Velkov, T., et al. (2017). Untargeted metabolomics analysis reveals key pathways responsible for the synergistic killing of colistin and doripenem combination against Acinetobacter baumannii. Sci. Rep. 7 (February), 45527. doi:10.1038/srep45527
Martínez, J. L., and Rojo, F. (2011). Metabolic regulation of antibiotic resistance. FEMS Microbiol. Rev. 35 (5), 768–789. doi:10.1111/J.1574-6976.2011.00282.X
Megchelenbrink, W., Huynen, M., and Marchiori, E. (2014). optGpSampler: An improved tool for uniformly sampling the solution-space of genome-scale metabolic networks. PLoS ONE 9 (2), e86587. doi:10.1371/JOURNAL.PONE.0086587
Nang, S. C., Azad, M. A. K., Velkov, T., Zhou, Q. T., and Li, J. (2021). Rescuing the last-line polymyxins: Achievements and challenges. Pharmacol. Rev. 73 (2), 679–728. doi:10.1124/pharmrev.120.000020
Norsigian, C. J., Pusarla, N., McConn, J. L., Yurkovich, J. T., Drager, A., Palsson, B. O., et al. (2020). BiGG models 2020: multi-strain genome-scale models and expansion across the phylogenetic tree. Nucleic Acids Res. 48 (D1), D402–D406. doi:10.1093/NAR/GKZ1054
Prax, M., Mechler, L., Weidenmaier, C., and Bertram, R. (2016). Glucose augments killing efficiency of daptomycin challenged Staphylococcus aureus persisters. Plos One 11 (3), e0150907. doi:10.1371/JOURNAL.PONE.0150907
Rizvi, A., Shankar, A., Chatterjee, A., More, T. H., Bose, T., Dutta, A., et al. (2019). Rewiring of metabolic network in Mycobacterium tuberculosis during adaptation to different stresses. Front. Microbiol. 10 (OCT), 2417. doi:10.3389/fmicb.2019.02417
Rosenberg, C. R., Fang, X., and Allison, K. R. (2020). Potentiating aminoglycoside antibiotics to reduce their toxic side effects. PLOS ONE 15 (9), e0237948. doi:10.1371/JOURNAL.PONE.0237948
Sharma, R., Patel, S., Abboud, C., Diep, J., Ly, N. S., Pogue, J. M., et al. (2017). Polymyxin B in combination with meropenem against carbapenemase-producing Klebsiella pneumoniae: pharmacodynamics and morphological changes. Int. J. Antimicrob. Agents 49 (2), 224–232. doi:10.1016/J.IJANTIMICAG.2016.10.025
Shields, R. K., Nguyen, M. H., Hao, B., Kline, E. G., and Clancy, C. J. (2018). Colistin does not potentiate ceftazidime-avibactam killing of carbapenem-resistant enterobacteriaceae in vitro or suppress emergence of ceftazidime-avibactam resistance. Antimicrob. Agents Chemother. 62 (8), e01018-18. JPEG. doi:10.1128/AAC.01018-18
Smith, L., Wilkinson, D., Hucka, M., Bergmann, F., Hoops, S., Keating, S., et al. (2010). The systems Biology Markup Language (SBML): Language specification for level 3 version 1 core. Nat. Prec. 2010, 1. doi:10.1038/npre.2010.4959.1
Stokes, J. M., Lopatkin, A. J., Lobritz, M. A., and Collins, J. J. (2019). Bacterial metabolism and antibiotic efficacy. Cell Metab. 30 (2), 251–259. doi:10.1016/j.cmet.2019.06.009
Su, Y. Bin, Peng, B., Li, H., Cheng, Z. X., Zhang, T. T., Zhu, J. X., et al. (2018). Pyruvate cycle increases aminoglycoside efficacy and provides respiratory energy in bacteria. Proc. Natl. Acad. Sci. U. S. A. 115 (7), E1578–E1587. doi:10.1073/pnas.1714645115
Trimble, M. J., Mlynarcik, P., Kolar, M., and Hancock, R. E. W. (2016). Polymyxin: alternative mechanisms of action and resistance. Cold Spring Harb. Perspect. Med. 6 (10), a025288. doi:10.1101/CSHPERSPECT.A025288
Tsuji, B. T., Pogue, J. M., Zavascki, A. P., Paul, M., Daikos, G. L., Forrest, A., et al. (2019). International consensus guidelines for the optimal use of the polymyxins: Endorsed by the American college of clinical pharmacy (ACCP), European society of clinical microbiology and infectious Diseases (ESCMID), infectious Diseases society of America (IDSA), international society for anti-infective Pharmacology (ISAP), society of critical care medicine (SCCM), and society of infectious Diseases pharmacists (SIDP)..Pharmacotherapy 39 (1), 10–39. doi:10.1002/PHAR.2209
U.S. Centers for Disease Control and Prevention (2021) About antibiotic resistance | antibiotic/antimicrobial resistance CDC. Available at: https://www.cdc.gov/drugresistance/about.html (Accessed: 25 November 2021).
Van Acker, H., and Coenye, T. (2017). The role of reactive oxygen species in antibiotic-mediated killing of bacteria. Trends Microbiol. 25 (6), 456–466. doi:10.1016/J.TIM.2016.12.008
Wadhwa, M., Srinivasan, S., Bachhawat, A. K., and Venkatesh, K. V. (2018). Role of phosphate limitation and pyruvate decarboxylase in rewiring of the metabolic network for increasing flux towards isoprenoid pathway in a TATA binding protein mutant of Saccharomyces cerevisiae. Microb. Cell Fact. 17 (1), 152. doi:10.1186/s12934-018-1000-1
Wang, M., Chan, E. W. C., Yang, C., Chen, K., So, P. K., and Chen, S. (2020). N-Acetyl-D-Glucosamine acts as adjuvant that re-sensitizes starvation-induced antibiotic-tolerant population of E. Coli to β-lactam. iScience 23 (11), 101740. doi:10.1016/J.ISCI.2020.101740
Wistrand-Yuen, P., Olsson, A., Skarp, K. P., Friberg, L. E., Nielsen, E. I., Lagerback, P., et al. (2020). Evaluation of polymyxin B in combination with 13 other antibiotics against carbapenemase-producing Klebsiella pneumoniae in time-lapse microscopy and time-kill experiments. Clin. Microbiol. Infect. 26 (9), 1214–1221. doi:10.1016/J.CMI.2020.03.007
World Health Organization (2020) Antibiotic resistance. Available at: https://www.who.int/news-room/fact-sheets/detail/antibiotic-resistance (Accessed: 25 November 2021).
Yang, J. H., Bening, S. C., and Collins, J. J. (2017). Antibiotic efficacy — context matters. Curr. Opin. Microbiol. 39, 73–80. doi:10.1016/J.MIB.2017.09.002
Yang, J. H., Wright, S. N., Hamblin, M., McCloskey, D., Alcantar, M. A., Schrubbers, L., et al. (2019). A white-box machine learning approach for revealing antibiotic mechanisms of action. Cell 177 (6), 1649–1661. e9. doi:10.1016/j.cell.2019.04.016
Yang, X., Dong, N., Chan, E. W. C., Zhang, R., and Chen, S. (2021). Carbapenem resistance-encoding and virulence-encoding conjugative plasmids in Klebsiella pneumoniae. Trends Microbiol. 29 (1), 65–83. doi:10.1016/J.TIM.2020.04.012
Yang, Y. Q., Li, Y. X., Lei, C. W., Zhang, A. Y., and Wang, H. N. (2018). Novel plasmid-mediated colistin resistance gene mcr-7.1 in Klebsiella pneumoniae. J. Antimicrob. Chemother. 73 (7), 1791–1795. doi:10.1093/JAC/DKY111
Zampieri, M., Zimmermann, M., Claassen, M., and Sauer, U. (2017). Nontargeted metabolomics reveals the multilevel response to antibiotic perturbations. Cell Rep. 19 (6), 1214–1228. doi:10.1016/J.CELREP.2017.04.002
Zeng, Z. hai, Du, C. C., Liu, S. R., Li, H., Peng, X. X., and Peng, B. (2017). Glucose enhances tilapia against Edwardsiella tarda infection through metabolome reprogramming. Fish. Shellfish Immunol. 61, 34–43. doi:10.1016/j.fsi.2016.12.010
Zhou, J., Liu, P., Xia, J., and Zhuang, Y. (2021). Advances in the development of constraint-based genome-scale metabolic network models. Chin. J. Biotechnol. 37 (5), 1526–1540. doi:10.13345/J.CJB.200498
Zhu, Y., Czauderna, T., Zhao, J., Klapperstueck, M., Maifiah, M. H. M., Han, M. L., et al. (2018). Genome-scale metabolic modeling of responses to polymyxins in Pseudomonas aeruginosa. GigaScience 7 (4), 1–18. doi:10.1093/gigascience/giy021
Zhu, Y., Zhao, J., Maifiah, M. H. M., Velkov, T., Schreiber, F., and Li, J. (2019). Metabolic responses to polymyxin treatment in Acinetobacter baumannii ATCC 19606: Integrating transcriptomics and metabolomics with Genome-scale metabolic modeling. mSystems 4 (1), e00157–18. doi:10.1128/msystems.00157-18
Keywords: Klebsiella pneumoniae, polymyxin, metabolite, genome-scale metabolic modeling, time–kill, metabolic modulation, antimicrobial resistance
Citation: Chung WY, Abdul Rahim N, Mahamad Maifiah MH, Hawala Shivashekaregowda NK, Zhu Y and Wong EH (2022) In silico genome-scale metabolic modeling and in vitro static time-kill studies of exogenous metabolites alone and with polymyxin B against Klebsiella pneumoniae. Front. Pharmacol. 13:880352. doi: 10.3389/fphar.2022.880352
Received: 21 February 2022; Accepted: 28 June 2022;
Published: 04 August 2022.
Edited by:
Hong Zhou, Zunyi Medical University, ChinaReviewed by:
Jason H. Yang, The State University of New Jersey, United StatesSameh AbdelGhani, Beni-Suef University, Egypt
Copyright © 2022 Chung, Abdul Rahim, Mahamad Maifiah, Hawala Shivashekaregowda, Zhu and Wong. This is an open-access article distributed under the terms of the Creative Commons Attribution License (CC BY). The use, distribution or reproduction in other forums is permitted, provided the original author(s) and the copyright owner(s) are credited and that the original publication in this journal is cited, in accordance with accepted academic practice. No use, distribution or reproduction is permitted which does not comply with these terms.
*Correspondence: Yan Zhu, yan.zhu@monash.edu; Eng Hwa Wong, enghwa.wong@taylors.edu.my