- 1BGI College and Henan Institute of Medical and Pharmaceutical Sciences, Zhengzhou University, Zhengzhou, China
- 2BGI-Shenzhen, Shenzhen, China
- 3College of Life Sciences, University of Chinese Academy of Sciences, Beijing, China
Cancer is one of the leading causes of death worldwide, bringing a significant burden to human health and society. Accurate cancer diagnosis and biomarkers that can be used as robust therapeutic targets are of great importance as they facilitate early and effective therapies. Shared etiology among cancers suggests the existence of pan-cancer biomarkers, performance of which could benefit from the large sample size and the heterogeneity of the studied patients. In this study, we conducted a systematic RNA-seq study of 9,213 tumors and 723 para-cancerous tissue samples of 28 solid tumors from the Cancer Genome Atlas (TCGA) database, and 7,008 normal tissue samples from the Genotype-Tissue Expression (GTEx) database. By differential gene expression analysis, we identified 214 up-regulated and 186 downregulated differentially expressed genes (DEGs) in more than 80% of the studied tumors, respectively, and obtained 20 highly linked up- and downregulated hub genes from them. These markers have rarely been reported in multiple tumors simultaneously. We further constructed pan-cancer diagnostic models to classify tumors and para-cancerous tissues using 10 up-regulated hub genes with an AUC of 0.894. Survival analysis revealed that these hub genes were significantly associated with the overall survival of cancer patients. In addition, drug sensitivity predictions for these hub genes in a variety of tumors obtained several broad-spectrum anti-cancer drugs targeting pan-cancer. Furthermore, we predicted immunotherapy sensitivity for cancers based on tumor mutational burden (TMB) and the expression of immune checkpoint genes (ICGs), providing a theoretical basis for the treatment of tumors. In summary, we identified a set of biomarkers that were differentially expressed in multiple types of cancers, and these biomarkers can be potentially used for diagnosis and used as therapeutic targets.
Introduction
Cancer is a serious threat to human life and health and is an important public health problem worldwide. According to the World Health Organization 2021, cancer is the first or second leading cause of human death (Siegel et al., 2021). Cancer arises from genetic mutations and dysregulation of transcriptional processes (Huntsman and Ladanyi, 2018), and its development is influenced by a variety of factors, including cancer cell development, differentiation, and epigenetic regulation (Graham and Sottoriva, 2017).
Early detection and accurate diagnosis of cancer based on robust biomarkers are of great value as they dramatically improve the therapeutic outcome (Sharma, 2018). Reduced costs through molecularly targeted therapies and improved accessibility of early and accurate diagnosis for cancer patients can ultimately lead to better clinical decision-making and additional possibilities for the precision treatment of tumors. Many studies have identified markers that can be used for cancer diagnosis, however, most of them have focused on diagnostic markers for a single type of cancer.
In recent years, pan‐cancer analysis brought us to a new level of cancer research, which overcomes the limitation in the sample size of single-cancer studies, and it is powerful for studying a highly heterogeneous disease like cancer. These pan-cancer studies have led to an increasing understanding of the complexity and heterogeneity of tumors. For example, in human pan-cancer studies, overexpression of BRCA1-associated protein (BRAP) was associated with poor prognosis and immune infiltration in a variety of cancers (Ju et al., 2020a). PINK1 was down-regulated in most tumors and may play a protective role in cancer patients (Zhu et al., 2020). NFE2L2 was positively associated with immune infiltration in pan-cancer (Ju et al., 2020b). Pan-cancer research has allowed us to understand that the same cancer may be very different at the molecular level (Hu et al., 2017), while diverse cancers may share the same molecular profile (Comprehensive genomic cha, 2008). Thereby deepening the pan-cancer level studies with a large sample size will hopefully discover new biomarkers which can be used to develop new cancer treatment strategies.
Currently, the TCGA (Weinstein et al., 2013) project provides sufficient transcriptome-level data to allow a systematic analysis of a wide range of cancers. However, early diagnostic studies of tumors also require a large amount of transcriptomic data of matched normal tissues to conduct the differential analysis. Although the TCGA has matched some para-cancerous tissues transcriptome datasets, the sample size is limited. GTEx (Human genomics. The Genot, 2015; Melé et al., 2015) samples can serve as alternative high-quality matched tissue controls, which provides an excellent opportunity to elucidate the transcriptional variation between normal and tumor tissues and the underlying genetic basis of the normal-to-tumor transition.
There are different treatment modalities for each tumor at present, while fewer broad-spectrum anti-cancer drugs are available, and it is urgent to find more broad-spectrum anti-cancer drugs. In addition, except for traditional treatment modalities like chemotherapy, immunotherapy represented by immune-checkpoint inhibitors (ICIs) has significantly improved the survival status of cancer patients, which is now changing the way of cancer treatment. However, the response to ICIs varies dramatically among patients with different malignancies. Therefore, screening the appropriate immunotherapy population before treatment is crucial to achieving precise treatment (Wang et al., 2019a).
In this study, we identified DEGs that are consistently expressed differentially in different cancers by performing differential gene expression analysis on transcriptomic data from TCGA and GTEx databases. A pan-cancer diagnostic model was further constructed to classify pan-cancers and normal samples with good performance. We also investigated the feasibility of using hub genes for prognostic assessment and drug sensitivity prediction. This study identified a set of candidate pan-cancer biomarkers, brought new insight into the etiology of tumors, and potentially provided new therapeutic targets for some cancers.
Materials and Methods
Data Acquisition
The transcriptome data of tumor samples and part of the matched control samples were obtained from the TCGA, including 9,213 tumor tissue samples and 723 para-cancerous tissue samples. In addition, we matched 7,008 tissue samples from GTEx (https://gtexportal.org/home/) database to obtain the transcriptomic data of normal tissues. The related normalized mRNA data and clinical data were downloaded from the UCSC Xena database (https://xena.ucsc.edu/) and used for subsequent differential gene expression analysis.
Differential Expression Gene Analysis
The DEGs were identified using the DESeq2 R package (1.30.1). Genes were defined as differentially expressed by thresholds of adjusted p-value < 0.05 and absolute log2 fold change (FC) > 1.0. When readings for genes are not detected in half or more of the samples, these genes will be filtered out by quality control.
Pathway Enrichment Analysis
Functional enrichment analysis based on the Kyoto Encyclopaedia of Genes and Genomes (KEGG) database (Kanehisa and Goto, 2000) was performed using Kobas3.0 (Bu et al., 2021). The top 30 items of the KEGG pathway enrichment analysis were sorted and presented as bar charts, which were plotted with the ggplot2 R package based on the p values of the statistical software R (version 4.0.4). p < 0.05 was considered to be statistically significant.
Protein-Protein Interaction Network Construction
The interaction between the DEGs was identified by the Search Tool for the Retrieval of Interacting Genes/Proteins database (STRING v10.5) (https://string-db.org/) and visualized using Cytoscape software (Shannon et al., 2003). The top 10 genes with the highest degree of connectivity were then selected as the target hub genes by using the Cytoscape plugin cytoHubba. The criteria of the protein-protein interaction (PPI) network included a confidence score ≥ 0.4.
Survival Analysis
Patient survival and related analysis were performed on Gene Expression Profiling Interactive Analysis 2 (Tang et al., 2019) (GEPIA2, http://gepia2.cancer-pku.cn), which is a web server built for analyzing the RNA expression data of tumors and normal samples from the TCGA and the GTEx projects, using a standard processing pipeline. GEPIA2 was also used for generating survival heatmaps of hub genes.
Drug Response Prediction
Drug response prediction was carried out using Gene Set Cancer Analysis (GSCA, http://bioinfo.life.hust.edu.cn/GSCA/) with 20 up- and downregulated hub genes as input, respectively. GSCA is an integrated database for genomic and immunogenomic gene set cancer analysis, which predicts the drug response based on the calculated correlation between mRNA expression and drug IC50 (Liu et al., 2018). The IC50s of more than 700 small molecule drugs in over 1,000 cell lines were obtained from the database of Genomics of Drug Sensitivity in Cancer (GDSC) and the mRNA expression of over 10,000 samples corresponding to more than 30 cancer types from the database of the Genomics of Therapeutics Response Portal (CTRP), respectively.
Construction of the Classification Model
Classification of tumors and para-cancerous samples was performed using LASSO regression analysis. When the ratio of the number of tumors and para-cancerous samples is severely imbalanced, the accuracy of classification may be affected. Therefore, when the number of tumor samples exceeded 1.5 times the number of para-cancerous tissue samples, we randomly sampled the tumor samples according to the minimum sample size to make sure that the sample sizes of the two groups were approximately equal and repeated 10 times to verify the accuracy of classification.
Result
Characteristics of the Studied Samples
Transcriptome data were obtained from TCGA and GTEx databases and included 9,213 tumor tissue samples, 723 para-cancerous tissue samples, and GTEx 7,008 normal tissue samples. The tumor types of the samples used in this study, as well as their corresponding sample sizes, and AJCC pathologic stages, are described in Table 1 and Supplementary Figure S1. We set out to identify genes that are consistently differentially expressed in different tumors as a basis for early tumor diagnosis and the prediction of drug targets. The analyses performed in the study were shown as a flowchart diagram in Figure 1A.
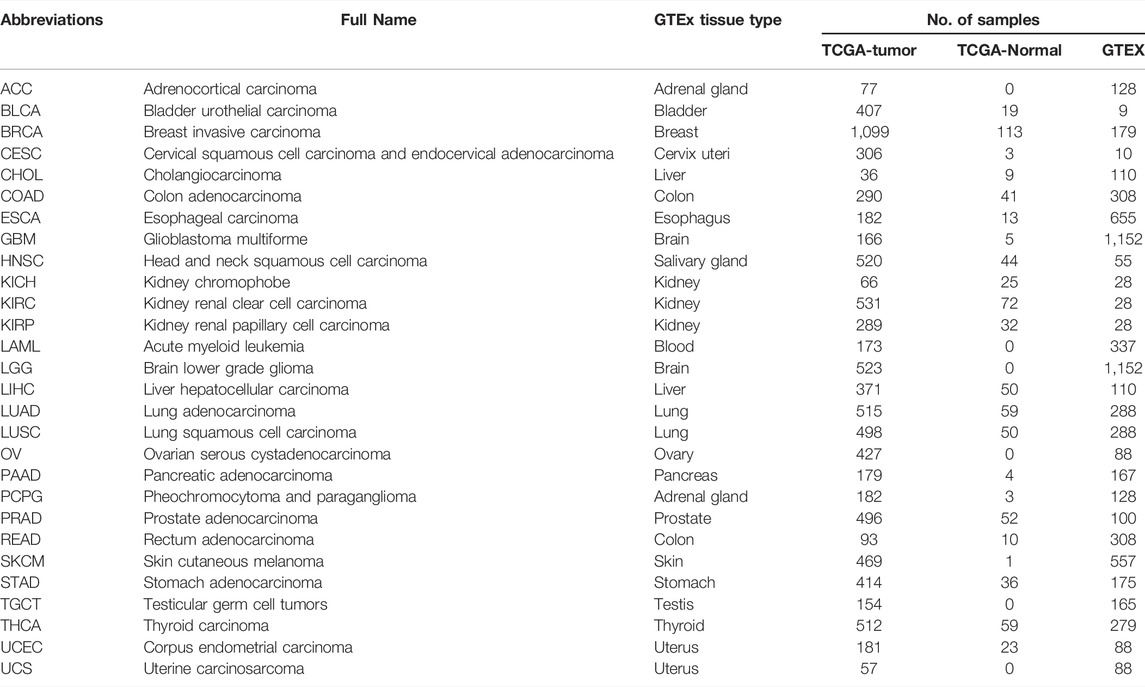
TABLE 1. 28 cancer types from TCGA and corresponding normal tissue samples from GTEx used for gene expression profiling.
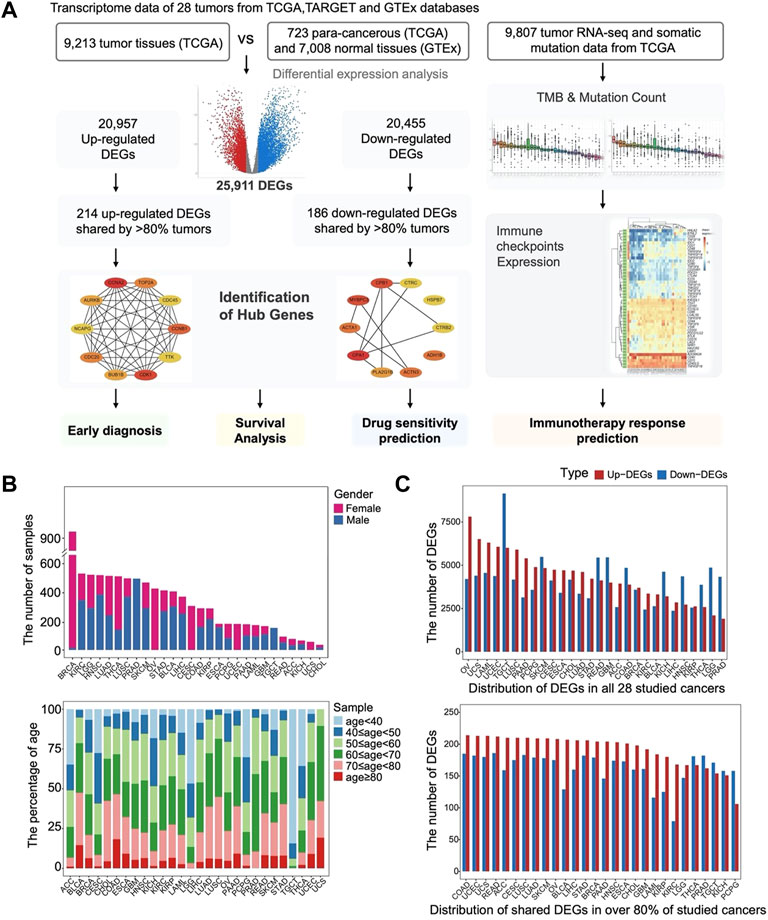
FIGURE 1. The landscape of distribution of DEGs among all tumors. (A) Workflow depicting a collection of TCGA, GTEx datasets, and processing of bioinformatic analysis for RNA-seq of pan-cancer. (B) Age and gender distribution of the 28 tumor samples. (C) Distribution of DEGs in all 28 studied cancers and distribution of shared DEGs in over 80% of studied cancers.
Overall, the most abundant malignancies in men are PRAD, HNSC, LUSC, and KIRC, while in women, the most common cancers are BRCA, OV, THCA, CESC, and LUAD (Figure 1B). The sample sizes of the studied tumors are approximately consistent with the incidence of the diseases (Siegel et al., 2021). For most malignant tumors, the frequencies of the studied samples increase with age (Figure 1B), which are consistent with the statistic that malignant tumor incidence is low among young people under 40 years of age, but increases rapidly after 40 years of age, and peaks at over 60 years of age. Notably, the age distribution is not uniform across different malignant tumors. The malignant tumors with a younger age of onset are TGCT, LGG, ACC, and THCA, while the other tumors are mainly from patients with age >50. Overall, the data from the study were selected to be representative in terms of sample size and its distribution among different tumors, and the findings can reflect the tumor and pan-cancer related patterns to a certain extent.
Identification of DEGs in Each Tumor and Shared DEGs in More Than 80% of Tumors
We first explored the unique and common gene expression dysregulation in different tumors by identifying the DEGs in each tumor. We identified a total of 25,911 DEGs in 28 tumors. A histogram shows the occurrence of total DEGs in different tumors (Figure 1C). The number of DEGs varies in different cancers, and the mean and median number of DEGs in each tumor are 8,440 and 8,300, respectively.
We further looked for shared DEGs that expressed differentially in most types of cancers. Among them, a total of 12 genes were found to be differentially expressed in all 28 tumors, of which 6 genes (ASPM, KIF4A, NEIL3, DTL, UBE2C, and UBE2SP2) were upregulated and 2 genes (PLIN4 and ADH1B) were downregulated in expression. In addition, four genes were differentially expressed in opposite directions among 28 tumors, ABCA9 was upregulated in LAML and down-regulated in the remaining 27 tumors, NPPA was upregulated in GBM and LGG and down-regulated in the remaining 26 tumors, while PBK and H2AC11 were down-regulated in TGCT and up-regulated in the rest 27 tumors.
Most of these DEGs have been reported to have potential as diagnostic and prognostic biomarkers in a specific tumor or class of tumors (Xu et al., 2019; Zhao et al., 2021; Luo et al., 2022), while a few have been reported to have such potential in no less than 10 tumors (Jiang et al., 2021; Pan et al., 2021). For example, ASPM (Assembly Factor for Spindle Microtubules) facilitates the homologous recombination-mediated repair of DNA double-strand break (Xu et al., 2021), is essential for mitotic spindle function during cell replication, and plays a pivotal role in the invasiveness of bladder cancer and serves as a potential prognostic biomarker for them (Xu et al., 2019). The UBE2C (Ubiquitin Conjugating Enzyme E2 C) is a conjugating enzyme, that plays a crucial role in cancer progression and its upregulation has been found in various cancers, a recent study demonstrated that overexpression of UBE2C was associated with TMB, microsatellite instability, immune cell infiltration, and diverse drug sensitivities (Jiang et al., 2021).
In addition, there are 47 genes differentially expressed in 27 types of cancers, while 677 are differentially expressed in >80% of tumor types (Supplementary Tables S1, S2). The distribution of genes that were consistently up- or downregulated in more than 80% of tumors was shown in Figure 1C.
Functional Annotation of Shared DEGs
We explored similarities in expression using DEGs that were identified in more than 80% of tumors for clustering, and up- and downregulated genes were used separately (Figures 2A–C). Among these studied tumors, some of them with similar expression dysregulation features clustered together, such as COAD and READ were clustered together, probably because they both belonged to colorectal cancer (CRC) (The Lancet Oncology, 2017), while UCS, OV, and UCEC, which are uterine and ovarian cancers, clustered together.
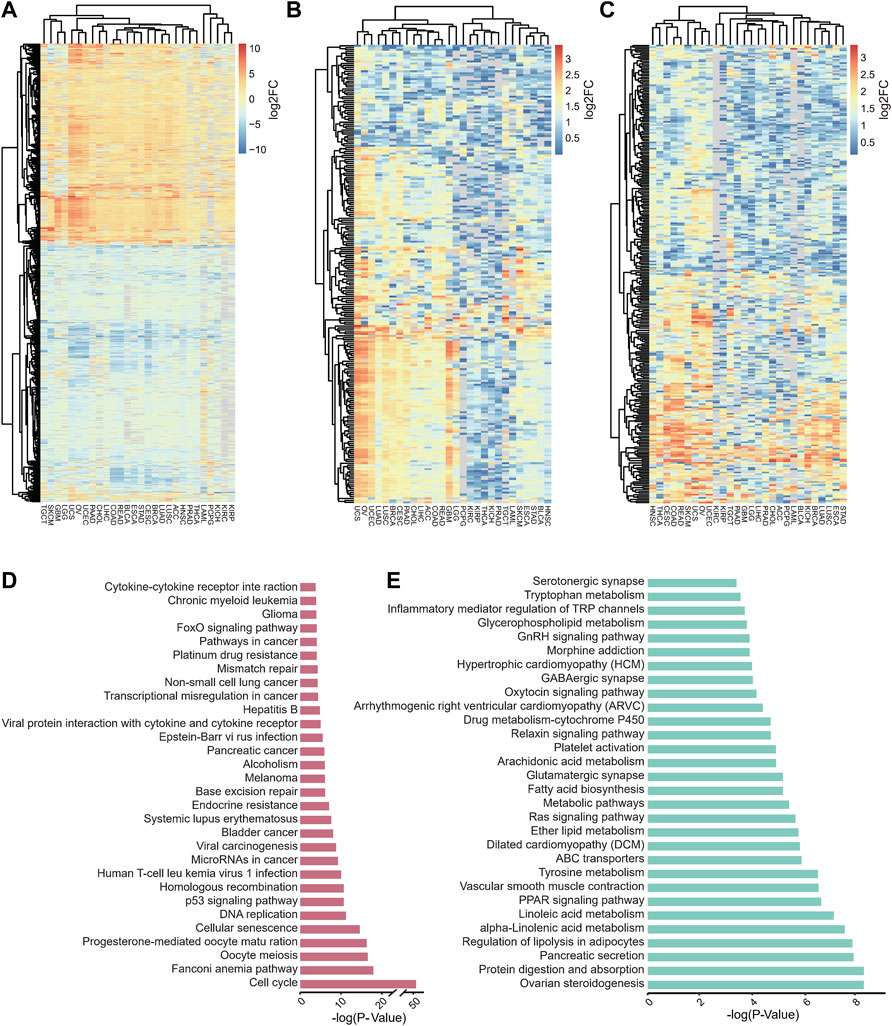
FIGURE 2. Identified DEGs shared in more than 80% of cancers and pathways significantly associated with these DEGs. (A–C) Overview of identified DEGs (A), identical up-regulated (B) and down-regulated (C) DEGs shared by over 80% of tumors in 28 tumors. (D–E) Barplot represents the top 30 enriched pathways of identical up-regulated (D) and identical down-regulated (E) of DEGs that are shared by over 80% of tumors, analysis was performed using KOBAS 3.0.
Furthermore, we examined the shared dysregulated pathways of DEGs by KEGG pathway enrichment analysis and found that the shared up-regulated genes among 28 tumors are functionally associated with pathways that are related to oncogenesis and cell cycle (Figure 2D), such as cell cycle, cellular senescence, p53 signaling pathway, human T-cell leukemia virus 1 infection, microRNAs in cancer, transcriptional dysregulation in cancer (Liu et al., 2020; Hirons et al., 2021; Lee and Dutta, 2009). In contrast, the differentially down-regulated expressed genes shared by 28 tumors are mainly involved in nutrient metabolism-related pathways (Figure 2E), such as protein digestion and absorption, regulation of lipolysis in adipocytes, alpha-linolenic acid metabolism, linoleic acid metabolism, tyrosine metabolism, and fatty acid biosynthesis (Burak et al., 2019; Yang and Mottillo, 2020).
Networks Analysis and Hub Genes Screening
Systematic exploration of the relationships between genes can help to explain the relationship between genotype and phenotype (Kuzmin et al., 2018). We performed protein-protein interaction (PPI) network analysis of the shared DEGs that existed in more than 80% of the tumors using the STRING database and screened the up- and downregulated hub genes using Cytoscape plugin cytoHubba, respectively, based on the amount of connectivity (Figures 3A,B). The highly connected genes in a network are hub genes, and the 10 upregulated hub genes interact much more intensively with each other compared to downregulated hub genes (Figures 3C,D), suggesting that the majority of DEGs in cancers are upregulated, and these hub genes are closely coordinated and interact tremendously to participate in certain oncogenic pathways leading to carcinogenesis.
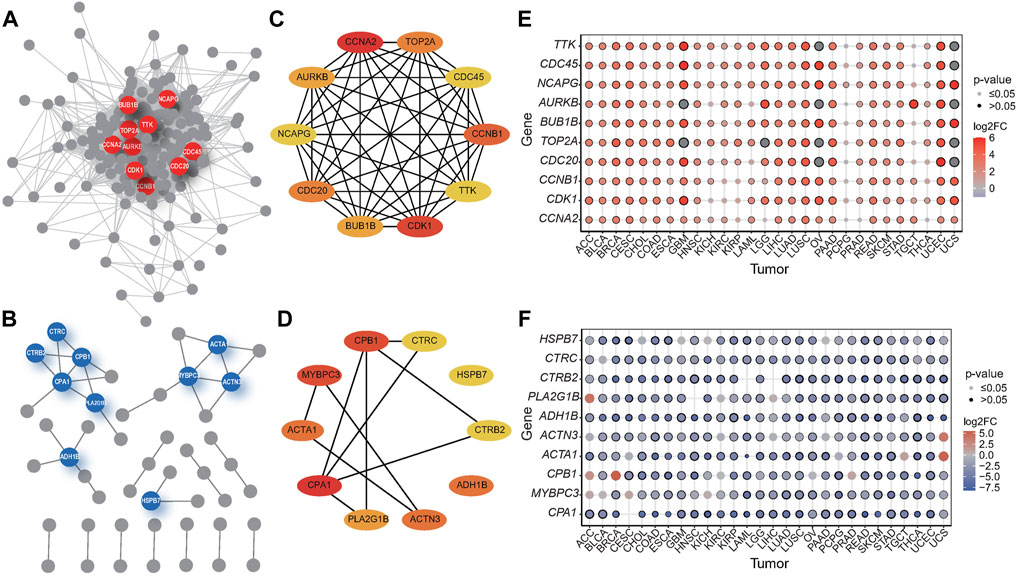
FIGURE 3. PPI network of the hub genes in tumors and the expression profile of hub genes. (A–B) PPI networks of up-regulated (A) and down-regulated (B) DEGs shared by 28 tumors. The hubs genes were in the center of the network, represented by colored circles. (C–D) PPI among top 10 identical up- (C) and down-regulated (D) DEGs in various cancers. (E–F) Gene expression of hub genes in identical up- (E) and down-regulated (F) DEGs in 28 tumors.
Next, we mapped the expression levels of the hub genes among these overlapping expression genes in all the studied tumors and presented them as bubble plots (Figures 3E,F) to visualize the differential expression of these genes in specific tumors and para-cancerous tissues. The results showed that NCAPG was significantly overexpressed in almost all tumors except TGCT, while CDC45, TTK, BUB1B, and TOP2A were overexpressed in all tumors other than PCPG and TGCT.
Non-structural maintenance of chromosomes condensin I complex subunit G (NCAPG) is responsible for the condensation and stabilization of chromosomes during mitosis and meiosis (Murphy and Sarge, 2008). The previous study suggests that the overexpression of NCAPG is significantly associated with unfavorable survival in diverse human malignancies. And the high expression of NCAPG may play an essential role in tumorigenesis and progression (Xiao et al., 2020), serving as a promising molecular target for cancer treatment and prognostic biomarkers for hepatocellular carcinoma (HCC) (Wang et al., 2019b; Xiao et al., 2020).
Cytokinesis cycle protein 45 (CDC45) was thought to be associated with tumorigenesis, and its low proteomic levels were associated with poor prognosis in HCC patients, suggesting that CDC45 may be a novel prognostic marker for HCC (Yang et al., 2021). In addition, knockdown of CDC45 expression inhibited the proliferation of non-small cell lung cancer (NSCLC) cells in vitro and in vivo and arrested cells in the G2/M phase of the cell cycle, which could be a novel therapeutic target for NSCLC (Huang et al., 2019). A component of the Spindle Assembly Checkpoint, TTK protein kinase whose inhibition could be a novel therapeutic target for the treatment of triple-negative breast cancer (TNBC) (Maia et al., 2015) and pancreatic ductal adenocarcinoma (PDAC) (Kaistha et al., 2014), is overexpressed in several cancers. BUB1 Mitotic Checkpoint Serine/Threonine Kinase B (BUB1B) is an essential component of the mitotic checkpoint, and its high expression is thought to be associated with the progression and recurrence of several cancers (Dong et al., 2019). Topoisomerase II alpha (TOP2A), highly expressed in various human cancers, is a potential prognostic and predictive marker as well as a therapeutic target in combating HCC (Panvichian et al., 2015; Wang et al., 2022).
Hub Genes as Potential Diagnostic Markers for Cancers
We used these identified hub genes as features to construct a pan-cancer diagnostic model, and the receiver operating characteristic (ROC) curve illustrated the diagnostic ability and the area under the curve (AUC) showed the performance of a classification model. Based on the Lasso regression, the classifier performed excellently with an AUC value close to 89.4% (Figure 4A) using the top 10 upregulated hub genes (CCNA2, CDK1, CCNB1, CDC20, TOP2A, BUB1B, AURKB, NCAPG, CDC45, and TTK). We replicated these hub genes as features for tumor and para-cancerous tissues classification using transcriptomic data from 14 additional datasets for different tumors, all with good accuracy and an AUC of at least 77.6% (Figure 4B). These datasets include BLCA (GSE13507), BRCA (GSE27562), CESC (GSE63514), CHOL (GSE76297), COAD (GSE39582), ESCA (GSE23400), STAD (GSE27342), HCC (GSE14520), KIRC (GSE40435), LUAD (GSE31547), PAAD (GSE62452), PRAD (GSE46602), SKCM (GSE15605), and PATC (GSE33630) (Supplementary Table S3).
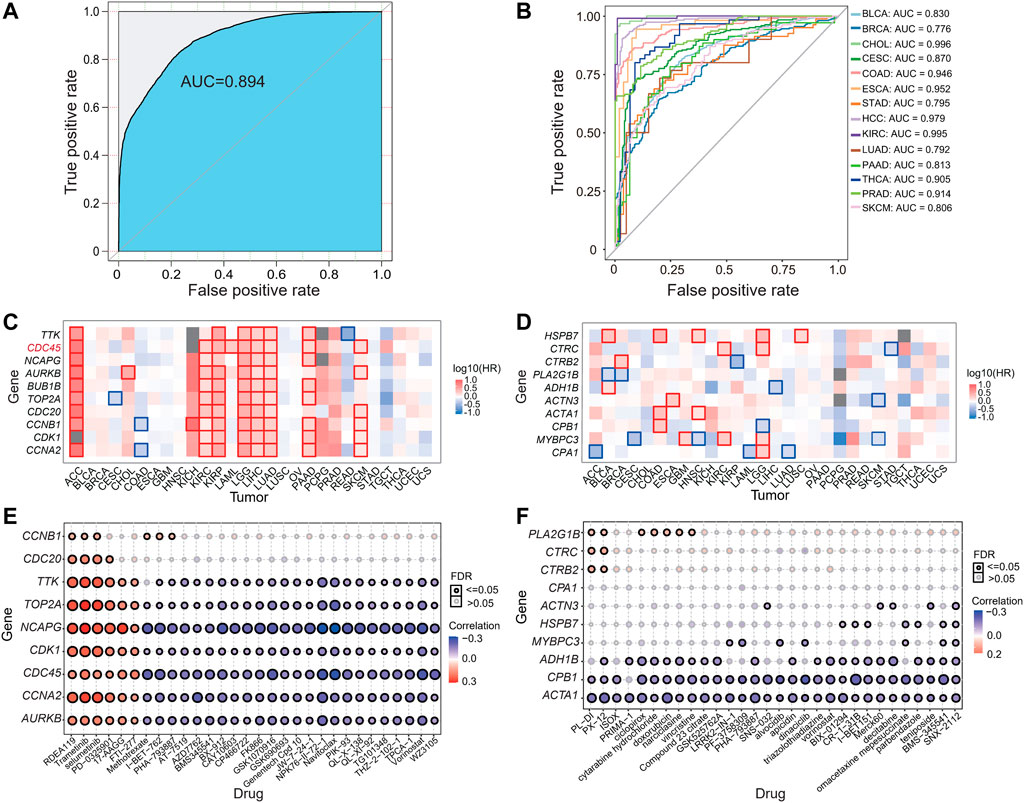
FIGURE 4. Performance of classification model, prognostic assessment and drug sensitivity evaluation based on hub genes. (A–B) Area under the ROC curve (AUC) plots of the training dataset (A) and external datasets (B). (C–D) Survival analysis of hub genes in identical up- (C) and down-regulated (D) DEGs in various cancers. (E–F) The bubble plots showed the correlations of mRNA expression levels of hub genes with GDSC (E) and CTRP (F) drug sensitivities.
Survival Analysis and Drug Sensitivity Prediction of Hub DEGs
Given that most of the hub genes have been reported to be associated with a variety of tumorigenesis and prognosis, to further explore the role of these genes in the prognosis of all studied tumors, we performed a survival analysis based on these hub genes (Figures 4C,D). We found that 10 up-regulated hub genes were significantly associated with survival in most tumors. For instance, CDC45 plays a key role in DNA replication was significantly associated with survival in 9 tumors, and AURKB was significantly associated with survival in 8 tumors (Figure 4C). We further investigated the expression of CDC45 in tumors at different stages and found that in all 28 studied tumors, CDC45 was highly expressed in most tumors compared to normal controls, and its expression level gradually increased with progressive tumor stage (Supplementary Figure S2). Together with the previous finding that CDC45 affected the survival of most tumors, we speculated that the upregulation of these specific DEGs may have an impact on patients’ survival.
Lastly, to explore the potential of the screened hub genes for clinical application, we calculated the correlation between the hub gene expression and drug sensitivity associated expression profiles from the GSCA database, which merged GDSC and CTRP databases and screened for potential anti-cancer drugs that interacted with it. The result showed that overexpression of these hub genes is positively correlated with the resistance of some drugs, vice verse (Figures 4E,F), implying that these drugs may be able to reduce the expression level of these genes and act as an anti-tumor agent. And some drugs are already used in clinical, such as RDEA119, trametinib, selumetinib, PD-0325901, 17-AAG, and FTI-277 (Figures 4E,F). RDEA119/BAY 869766 is highly potent in inhibiting cell proliferation in several tumor cell lines in vitro. It has also shown potent activity in xenograft models of melanoma, colon, and epidermoid carcinoma in vivo (Iverson et al., 2009). Trametinib (GSK1120212) is an oral mitogen-activated protein kinase (MEK) inhibitor that is selective for MEK1 and MEK2. It has been approved by the FDA in combination for the treatment of metastatic melanoma with BRAF inhibitors (Zeiser et al., 2018). Selumetinib is a mitogen-activated protein kinase 1 and 2 (MEK1/2) inhibitor, used to treat neurofibromatosis and various cancers. It can also be used as adjuvant therapy for thyroid cancer and the treatment of type 1 neurofibromas (Markham and Keam, 2020). In a word, our analysis and predictions will hopefully be informative for the clinical management of these cancers.
The Prediction of Immunotherapy Sensitivity
Higher TMB and somatic mutation rates were associated with better anti-cancer immune responses (Castle et al., 2019). We calculated the TMB (Figure 5A) and somatic mutation counts (Figure 5B) for all tumor samples based on mutect2 results for 33 tumors in the TCGA. In contrast, patients affected by several tumors with high TMB and mutation counts, such as SKCM, LUSC, and LUAD, may have a higher sensitivity to immunotherapy.
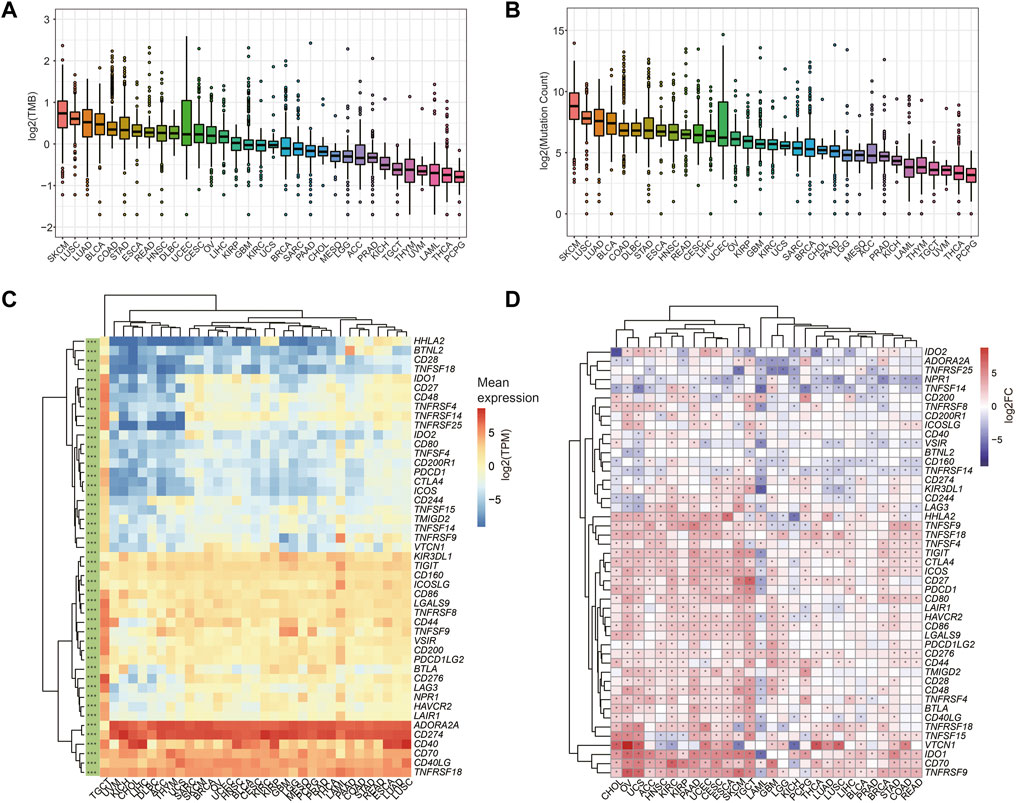
FIGURE 5. The prediction of immunotherapy sensitivity. (A–B) Tumor mutational burden (TMB) (A) and mutation count (B) across 33 cancer types. (C) Gene expression profiling of ICGs in different tumors in the TCGA cohort (* represents that ICGs are significantly differentially expressed in different tumors, Kruskal-Wallis test was used, and *** means p < 0.0001). (D) Differential expression of ICGs constructed in 28 TCGA cancers with tumor-normal paired samples (* indicates ICGs are differentially expressed in tumor and normal tissues).
We also collected 47 ICGs reported in the previous study (Huang et al., 2021), and these ICGs are mainly involved in ligand-receptor interactions and have different effects on immune activity, including inhibition, stimulation, or a combination of both. We investigated the expression profiles of ICGs in all tumor tissues of the TCGA cohort (Figure 5C), as well as the differential expression of these genes in 28 tumors and para-cancerous tissues (Figure 5D). Significant differential expression of ICGs was observed in tumors (Figure 5C), and these ICGs had a distinct cancer-specific profile compared to normal controls (Figure 5D). We found that the expression of most ICGs was up-regulated in KIRC, KIRP, ESCA, SKCM, and HNSC compared to para-cancerous tissues, suggesting a potential response to immunotherapy in the corresponding tumors (Zhang et al., 2022).
In addition, we found the ICGs expression was higher in tumors with high TMB and having more mutations such as LUSC, LUAD, ESCA, and STAD (Figure 5C), reinforcing the point that these tumors may have surprising efficacious against prevailing ICIs (Liu et al., 2017; Tung et al., 2021; Xie et al., 2021; Zhang et al., 2021).
Discussion
Integrating transcriptomic data from a large variety of cancers to study cancer characteristics is an important and valuable direction of research in cancer biology. A substantial number of studies have shown similarities between different cancers, such as key driver mutations (Martincorena and Campbell, 2015), immune (Desrichard et al., 2016), and microbial signatures (Poore et al., 2020), suggesting the possibility of common features of different cancers for tumor diagnosis and clinical recommendations. However, cancer cell heterogeneity is a challenging concept in cancer biology. Therefore, we herein sought to find potential biomarkers by selecting dysregulated genes in most types of cancer from a large cohort and validating these biomarkers in additional independent datasets to reduce the impact of heterogeneity across cancers.
In this study, we analyzed a large amount of transcriptomic data from public databases of tumor and para-cancerous tissues and obtained DEGs that were differentially expressed in most of the studied tumors compared to para-cancerous tissues, some of which have been reported can be used as diagnostic or prognostic markers for specific cancer (Kaistha et al., 2014; Maia et al., 2015; Wang et al., 2019b; Xu et al., 2019; Xiao et al., 2020; Zhao et al., 2021; Luo et al., 2022) and partly as biomarkers for pan-cancer diagnosis (Dong et al., 2019; Jiang et al., 2021; Pan et al., 2021). We performed PPI network analysis on genes that differentially expressed in more than 80% of the studied cancers and obtained 20 up- and downregulated hub genes. We further explored the possibility of using the ten upregulated hub genes as features to distinguish tumors from para-cancerous tissues, which achieved high accuracy and sensitivity. Furthermore, we found that some hub genes have the potential for the prognostic assessment of cancer patients. Additionally, we examined the drug sensitivity of some broad-spectrum anti-cancer drugs based on 20 hub genes and obtained a handful of drugs such as RDEA119, trametinib, and selumetinib possibly exert anti-tumor effects in pan-cancers with corresponding DEGs.
Finally, we found that some tumors such as SKCM, LUSC, LUAD, KIRC, KIRP, ESCA, HNSC, and STAD might be more suitable for immunotherapy by comparing the TMB, mutation count levels as well as the expression levels of ICGs in different tumors. Interestingly, from the rank of TMB and somatic mutation counts, TGCT may be unsuitable for immunotherapy. However, regarding the expression of ICGs, TGCT is possibly sensitive to ICIs, which needs to be explored in further studies (Kalavska et al., 2020). In addition, ICGs such as CD276 and CD70 are upregulated in most tumors, and gene expression profiles show that CD276 is highly expressed in tumors such as SKCM, HNSC, ESCA, and LUSC, while CD70 is highly expressed in the majority of tumors. High expression of the CD276 gene is thought to be associated with the development and metastasis of several cancers (Yuan et al., 2011; Boland et al., 2013; Mao et al., 2015), and CD70 is involved in the survival of tumor cells and regulatory T cells through interaction with its ligand CD27 (Jacobs et al., 2015). A previous study showed that tandem CAR-T cells targeting CD70 and CD276 exhibited potent preclinical activity against a variety of solid tumors (Yang et al., 2020), suggesting that these two genes could be candidate targets for immunotherapy. However, this may be influenced by resistance to anti-cancer immunotherapy, the development of which involves complex and diverse factors. Thus pre-treatment testing of oncology patients and assessment of possible resistance development would be beneficial in guiding the choice of anti-cancer immunotherapy. In addition, combinations of ICIs, or combinations of strategies (cancer immunotherapies with targeted mutagenic drugs) may be considered to overcome the resistance to anti-cancer immunotherapy and improve efficacy (Sharma et al., 2017).
Despite the compelling results found in our study, there are still some limitations to be noted. First, cancer is a highly heterogeneous disease with great variation between tumors. Although the hub genes we identified hereby can predict patient survival to some extent, the survival of specific cancer patients is influenced by many factors. Except for these genetic background factors, whether the patient can be diagnosed at an early stage, the status of mainstream treatment modalities, and the accessibility of new treatments (especially immunotherapy) will lead to differences in survival outcomes.
Second, although our work suggests several biomarkers that may be useful for pan-cancer diagnosis and broad-spectrum anti-cancer drug selection, it should be noted that the present study is a retrospective study without validation in independent wet-lab laboratory experiments, and the limited sample size for some cancers could potentially lead to inaccurate or false-positive results. Besides, ICG expression was quantified at the transcriptomic level, while proteins such as mutation-derived neoantigens are the components directly involved in tumor immunity, and the protein expression does not exactly match the RNA expression (Dybas et al., 2019), so the performance of RNA expression-based biomarker may be influenced by the inconsistency between the RNA expression and protein expression. We believe that further persuasive wet-lab experiments and clinical studies are still needed to validate these biomarkers and confirm their specificity and sensitivity.
Third, we mainly explored the DEGs between tumor and para-cancerous tissues but did not address the discrepancies among different tumors. Future studies on specific markers for different tumors can be considered, which are expected to distinguish metastatic tumors that cannot be determined from the primary lesions and achieve early diagnosis and precision medicine for indistinguishable tumors.
In summary, the hub genes identified in this study may serve as biomarkers to construct a pan-cancer diagnostic model that could effectively distinguish tumors from para-cancerous tissues and contribute to drug selection and development. Our findings provide new clues for pan-cancer classification in complex cancer biology and facilitate early diagnosis and precise treatment of cancer.
Data Availability Statement
The original contributions presented in the study are included in the article/Supplementary Material, further inquiries can be directed to the corresponding authors.
Ethics Statement
The studies involving human participants were reviewed and approved by the Ethical Clearance the Institutional Review Board of BGI. Written informed consent from the participants’ legal guardian/next of kin was not required to participate in this study in accordance with the national legislation and the institutional requirements.
Author Contributions
MF designed the research, LZ and YM performed data analysis and data interpretation, LZ and MF drafted the manuscript, FX and PJ revised the manuscript, LX and XJ supervised the study. All authors read and approved the final manuscript.
Funding
This study was supported by the National Natural Science Foundation of China (No.31800765) and the National Key Research and Development Program of China (No. 2020YFC2002902).
Conflict of Interest
All authors were employed by company BGI-Shenzhen.
Publisher’s Note
All claims expressed in this article are solely those of the authors and do not necessarily represent those of their affiliated organizations, or those of the publisher, the editors and the reviewers. Any product that may be evaluated in this article, or claim that may be made by its manufacturer, is not guaranteed or endorsed by the publisher.
Supplementary Material
The Supplementary Material for this article can be found online at: https://www.frontiersin.org/articles/10.3389/fphar.2022.870660/full#supplementary-material
References
Boland, J. M., Kwon, E. D., Harrington, S. M., Wampfler, J. A., Tang, H., Yang, P., et al. (2013). Tumor B7-H1 and B7-H3 Expression in Squamous Cell Carcinoma of the Lung. Clin. Lung Cancer 14 (2), 157–163. doi:10.1016/j.cllc.2012.05.006
Bu, D., Luo, H., Huo, P., Wang, Z., Zhang, S., He, Z., et al. (2021). KOBAS-i: Intelligent Prioritization and Exploratory Visualization of Biological Functions for Gene Enrichment Analysis. Nucleic Acids Res. 49 (W1), W317–w325. doi:10.1093/nar/gkab447
Burak, C., Wolffram, S., Zur, B., Langguth, P., Fimmers, R., Alteheld, B., et al. (2019). Effect of Alpha-Linolenic Acid in Combination with the Flavonol Quercetin on Markers of Cardiovascular Disease Risk in Healthy, Non-obese Adults: A Randomized, Double-Blinded Placebo-Controlled Crossover Trial. Nutrition 58, 47–56. doi:10.1016/j.nut.2018.06.012
Castle, J. C., Uduman, M., Pabla, S., Stein, R. B., and Buell, J. S. (2019). Mutation-Derived Neoantigens for Cancer Immunotherapy. Front. Immunol. 10, 1856. doi:10.3389/fimmu.2019.01856
Comprehensive Genomic Characterization Defines Human Glioblastoma Genes and Core Pathways. Nature, 2008. 455(7216): p. 1061–1068.doi:10.1038/nature07385
Desrichard, A., Snyder, A., and Chan, T. A. (2016). Cancer Neoantigens and Applications for Immunotherapy. Clin. Cancer Res. 22 (4), 807–812. doi:10.1158/1078-0432.CCR-14-3175
Dong, S., Huang, F., Zhang, H., and Chen, Q. (2019). Overexpression of BUB1B, CCNA2, CDC20, and CDK1 in Tumor Tissues Predicts Poor Survival in Pancreatic Ductal Adenocarcinoma. Biosci. Rep. 39 (2). doi:10.1042/BSR20182306
Dybas, J. M., O'Leary, C. E., Ding, H., Spruce, L. A., Seeholzer, S. H., and Oliver, P. M. (2019). Integrative Proteomics Reveals an Increase in Non-degradative Ubiquitylation in Activated CD4+ T Cells. Nat. Immunol. 20 (6), 747–755. doi:10.1038/s41590-019-0381-6
Graham, T. A., and Sottoriva, A. (2017). Measuring Cancer Evolution from the Genome. J. Pathol. 241 (2), 183–191. doi:10.1002/path.4821
Hirons, A., Khoury, G., and Purcell, D. F. J. (2021). Human T-Cell Lymphotropic Virus Type-1: a Lifelong Persistent Infection, yet Never Truly Silent. Lancet Infect. Dis. 21 (1), e2–e10. doi:10.1016/S1473-3099(20)30328-5
Hu, W., Yang, Y., Li, X., and Zheng, S. (2017). Pan-organ Transcriptome Variation across 21 Cancer Types. Oncotarget 8 (4), 6809–6818. doi:10.18632/oncotarget.14303
Huang, J., Li, Y., Lu, Z., Che, Y., Sun, S., Mao, S., et al. (2019). Analysis of Functional Hub Genes Identifies CDC45 as an Oncogene in Non-small Cell Lung Cancer - a Short Report. Cel Oncol (Dordr) 42 (4), 571–578. doi:10.1007/s13402-019-00438-y
Huang, X., Tang, T., Zhang, G., and Liang, T. (2021). Identification of Tumor Antigens and Immune Subtypes of Cholangiocarcinoma for mRNA Vaccine Development. Mol. Cancer 20 (1), 50. doi:10.1186/s12943-021-01342-6
Human Genomics. The Genotype-Tissue Expression (GTEx) Pilot Analysis: Multitissue Gene Regulation in Humans. Science, 2015. 348(6235): p. 648–660.doi:10.1126/science.1262110
Huntsman, D. G., and Ladanyi, M. (2018). The Molecular Pathology of Cancer: from Pan-Genomics to post-genomics. J. Pathol. 244 (5), 509–511. doi:10.1002/path.5057
Iverson, C., Larson, G., Lai, C., Yeh, L. T., Dadson, C., Weingarten, P., et al. (2009). RDEA119/BAY 869766: a Potent, Selective, Allosteric Inhibitor of MEK1/2 for the Treatment of Cancer. Cancer Res. 69 (17), 6839–6847. doi:10.1158/0008-5472.CAN-09-0679
Jacobs, J., Deschoolmeester, V., Zwaenepoel, K., Rolfo, C., Silence, K., Rottey, S., et al. (2015). CD70: An Emerging Target in Cancer Immunotherapy. Pharmacol. Ther. 155, 1–10. doi:10.1016/j.pharmthera.2015.07.007
Jiang, X., Yuan, Y., Tang, L., Wang, J., Liu, Q., Zou, X., et al. (2021). Comprehensive Pan-Cancer Analysis of the Prognostic and Immunological Roles of the METTL3/lncRNA-SNHG1/miRNA-140-3p/UBE2C Axis. Front Cel Dev Biol 9, 765772. doi:10.3389/fcell.2021.765772
Ju, Q., Li, X., Zhang, H., Yan, S., Li, Y., and Zhao, Y. (2020). NFE2L2 Is a Potential Prognostic Biomarker and Is Correlated with Immune Infiltration in Brain Lower Grade Glioma: A Pan-Cancer Analysis. Oxid Med. Cel Longev 2020, 3580719. doi:10.1155/2020/3580719
Ju, Q., Li, X. M., Zhang, H., and Zhao, Y. J. (2020). BRCA1-Associated Protein Is a Potential Prognostic Biomarker and Is Correlated with Immune Infiltration in Liver Hepatocellular Carcinoma: A Pan-Cancer Analysis. Front. Mol. Biosci. 7, 573619. doi:10.3389/fmolb.2020.573619
Kaistha, B. P., Honstein, T., Müller, V., Bielak, S., Sauer, M., Kreider, R., et al. (2014). Key Role of Dual Specificity Kinase TTK in Proliferation and Survival of Pancreatic Cancer Cells. Br. J. Cancer 111 (9), 1780–1787. doi:10.1038/bjc.2014.460
Kalavska, K., Schmidtova, S., Chovanec, M., and Mego, M. (2020). Immunotherapy in Testicular Germ Cell Tumors. Front. Oncol. 10, 573977. doi:10.3389/fonc.2020.573977
Kanehisa, M., and Goto, S. (2000). KEGG: Kyoto Encyclopedia of Genes and Genomes. Nucleic Acids Res. 28 (1), 27–30. doi:10.1093/nar/28.1.27
Kuzmin, E., Vandersluis, B., Wang, W., Tan, G., Deshpande, R., Chen, Y., et al. (2018). Systematic Analysis of Complex Genetic Interactions. New York, N.Y.): Science, 360.6386
Lee, Y. S., and Dutta, A. (2009). MicroRNAs in Cancer. Annu. Rev. Pathol. 4, 199–227. doi:10.1146/annurev.pathol.4.110807.092222
Liu, C. J., Hu, F. F., Xia, M. X., Han, L., Zhang, Q., and Guo, A. Y. (2018). GSCALite: a Web Server for Gene Set Cancer Analysis. Bioinformatics 34 (21), 3771–3772. doi:10.1093/bioinformatics/bty411
Liu, J., Zhang, C., Wang, J., Hu, W., and Feng, Z. (2020). The Regulation of Ferroptosis by Tumor Suppressor P53 and its Pathway. Int. J. Mol. Sci. 21 (21). doi:10.3390/ijms21218387
Liu, X., Wu, S., Yang, Y., Zhao, M., Zhu, G., and Hou, Z. (2017). The Prognostic Landscape of Tumor-Infiltrating Immune Cell and Immunomodulators in Lung Cancer. Biomed. Pharmacother. 95, 55–61. doi:10.1016/j.biopha.2017.08.003
Luo, Y., He, Z., Liu, W., Zhou, F., Liu, T., and Wang, G. (2022). DTL Is a Prognostic Biomarker and Promotes Bladder Cancer Progression through Regulating the AKT/mTOR axis. Oxid Med. Cel Longev 2022, 3369858. doi:10.1155/2022/3369858
Maia, A. R., de Man, J., Boon, U., Janssen, A., Song, J. Y., Omerzu, M., et al. (2015). Inhibition of the Spindle Assembly Checkpoint Kinase TTK Enhances the Efficacy of Docetaxel in a Triple-Negative Breast Cancer Model. Ann. Oncol. 26 (10), 2180–2192. doi:10.1093/annonc/mdv293
Mao, Y., Li, W., Chen, K., Xie, Y., Liu, Q., Yao, M., et al. (2015). B7-H1 and B7-H3 Are Independent Predictors of Poor Prognosis in Patients with Non-small Cell Lung Cancer. Oncotarget 6 (5), 3452–3461. doi:10.18632/oncotarget.3097
Markham, A., and Keam, S. J. (2020). Selumetinib: First Approval. Drugs 80 (9), 931–937. doi:10.1007/s40265-020-01331-x
Martincorena, I., and Campbell, P. J. (2015). Somatic Mutation in Cancer and normal Cells. Science 349 (6255), 1483–1489. doi:10.1126/science.aab4082
Melé, M., Ferreira, P. G., Reverter, F., DeLuca, D. S., Monlong, J., Sammeth, M., et al. (2015). Human Genomics. The Human Transcriptome across Tissues and Individuals. Science 348 (6235), 660–665. doi:10.1126/science.aaa0355
Murphy, L. A., and Sarge, K. D. (2008). Phosphorylation of CAP-G Is Required for its Chromosomal DNA Localization during Mitosis. Biochem. Biophys. Res. Commun. 377 (3), 1007–1011. doi:10.1016/j.bbrc.2008.10.114
Pan, J., Lei, X., and Mao, X. (2021). Identification of KIF4A as a Pan-Cancer Diagnostic and Prognostic Biomarker via Bioinformatics Analysis and Validation in Osteosarcoma Cell Lines. PeerJ 9, e11455. doi:10.7717/peerj.11455
Panvichian, R., Tantiwetrueangdet, A., Angkathunyakul, N., and Leelaudomlipi, S. (2015). TOP2A Amplification and Overexpression in Hepatocellular Carcinoma Tissues. Biomed. Res. Int. 2015, 381602. doi:10.1155/2015/381602
Poore, G. D., Kopylova, E., Zhu, Q., Carpenter, C., Fraraccio, S., Wandro, S., et al. (2020). Microbiome Analyses of Blood and Tissues Suggest Cancer Diagnostic Approach. Nature 579 (7800), 567–574. doi:10.1038/s41586-020-2095-1
Shannon, P., Markiel, A., Ozier, O., Baliga, N. S., Wang, J. T., Ramage, D., et al. (2003). Cytoscape: a Software Environment for Integrated Models of Biomolecular Interaction Networks. Genome Res. 13 (11), 2498–2504. doi:10.1101/gr.1239303
Sharma, A. K. (2018). Emerging Trends in Biomarker Discovery: Ease of Prognosis and Prediction in Cancer. Semin. Cancer Biol. 52 (Pt 1), iii–iv. doi:10.1016/j.semcancer.2018.05.008
Sharma, P., Hu-Lieskovan, S., Wargo, J. A., and Ribas, A. (2017). Primary, Adaptive, and Acquired Resistance to Cancer Immunotherapy. Cell 168 (4), 707–723. doi:10.1016/j.cell.2017.01.017
Siegel, R. L., Miller, K. D., Fuchs, H. E., and Jemal, A. (2021). Cancer Statistics, 2021. CA A. Cancer J. Clin. 71 (1), 7–33. doi:10.3322/caac.21654
Tang, Z., Kang, B., Li, C., Chen, T., and Zhang, Z. (2019). GEPIA2: an Enhanced Web Server for Large-Scale Expression Profiling and Interactive Analysis. Nucleic Acids Res. 47 (W1), W556–w560. doi:10.1093/nar/gkz430
The Lancet Oncology, O. (2017). Colorectal Cancer: a Disease of the Young? Lancet Oncol. 18 (4), 413. doi:10.1016/S1470-2045(17)30202-4
Tung, C. B., Li, C. Y., and Lin, H. Y. (2021). Multi-Omics Reveal the Immunological Role and the Theragnostic Value of miR-216a/GDF15 Axis in Human Colon Adenocarcinoma. Int. J. Mol. Sci. 22 (24). doi:10.3390/ijms222413636
Wang, S., He, Z., Wang, X., Li, H., and Liu, X. S. (2019). Antigen Presentation and Tumor Immunogenicity in Cancer Immunotherapy Response Prediction. Elife 8. doi:10.7554/eLife.49020
Wang, T., Lu, J., Wang, R., Cao, W., and Xu, J. (2022). TOP2A Promotes Proliferation and Metastasis of Hepatocellular Carcinoma Regulated by miR-144-3p. J. Cancer 13 (2), 589–601. doi:10.7150/jca.64017
Wang, Y., Gao, B., Tan, P. Y., Handoko, Y. A., Sekar, K., Deivasigamani, A., et al. (2019). Genome-wide CRISPR Knockout Screens Identify NCAPG as an Essential Oncogene for Hepatocellular Carcinoma Tumor Growth. FASEB J. 33 (8), 8759–8770. doi:10.1096/fj.201802213RR
Weinstein, J. N., Weinstein, J. N., Collisson, E. A., Mills, G. B., Shaw, K. R., Ozenberger, B. A., et al. (2013). The Cancer Genome Atlas Pan-Cancer Analysis Project. Nat. Genet. 45 (10), 1113–1120. doi:10.1038/ng.2764
Xiao, C., Gong, J., Jie, Y., Cao, J., Chen, Z., Li, R., et al. (2020). NCAPG Is a Promising Therapeutic Target across Different Tumor Types. Front. Pharmacol. 11, 387. doi:10.3389/fphar.2020.00387
Xie, Y., Shi, X., Chen, Y., Wu, B., Gong, X., Lu, W., et al. (2021). The Intra-class Heterogeneity of Immunophenotyping and Immune Landscape in Oesophageal Cancer and Clinical Implications. Ann. Med. 53 (1), 626–638. doi:10.1080/07853890.2021.1912385
Xu, S., Wu, X., Wang, P., Cao, S. L., Peng, B., and Xu, X. (2021). ASPM Promotes Homologous Recombination-Mediated DNA Repair by Safeguarding BRCA1 Stability. iScience 24 (6), 102534. doi:10.1016/j.isci.2021.102534
Xu, Z., Zhang, Q., Luh, F., Jin, B., and Liu, X. (2019). Overexpression of the ASPM Gene Is Associated with Aggressiveness and Poor Outcome in Bladder Cancer. Oncol. Lett. 17 (2), 1865–1876. doi:10.3892/ol.2018.9762
Yang, A., and Mottillo, E. P. (2020). Adipocyte Lipolysis: from Molecular Mechanisms of Regulation to Disease and Therapeutics. Biochem. J. 477 (5), 985–1008. doi:10.1042/BCJ20190468
Yang, C., Xie, S., Wu, Y., Ru, G., He, X., Pan, H. Y., et al. (2021). Prognostic Implications of Cell Division Cycle Protein 45 Expression in Hepatocellular Carcinoma. PeerJ 9, e10824. doi:10.7717/peerj.10824
Yang, M., Tang, X., Zhang, Z., Gu, L., Wei, H., Zhao, S., et al. (2020). Tandem CAR-T Cells Targeting CD70 and B7-H3 Exhibit Potent Preclinical Activity against Multiple Solid Tumors. Theranostics 10 (17), 7622–7634. doi:10.7150/thno.43991
Yuan, H., Wei, X., Zhang, G., Li, C., Zhang, X., and Hou, J. (2011). B7-H3 over Expression in Prostate Cancer Promotes Tumor Cell Progression. J. Urol. 186 (3), 1093–1099. doi:10.1016/j.juro.2011.04.103
Zeiser, R., Andrlová, H., and Meiss, F. (2018). Trametinib (GSK1120212). Recent Results Cancer Res. 211, 91–100. doi:10.1007/978-3-319-91442-8_7
Zhang, J., Han, X., Lin, L., Chen, J., Wang, F., Ding, Q., et al. (2022). Unraveling the Expression Patterns of Immune Checkpoints Identifies New Subtypes and Emerging Therapeutic Indicators in Lung Adenocarcinoma. Oxid Med. Cel Longev 2022, 3583985. doi:10.1155/2022/3583985
Zhang, X., Wang, Y., A, G., Qu, C., and Chen, J. (2021). Pan-Cancer Analysis of PARP1 Alterations as Biomarkers in the Prediction of Immunotherapeutic Effects and the Association of its Expression Levels and Immunotherapy Signatures. Front. Immunol. 12, 721030. doi:10.3389/fimmu.2021.721030
Zhao, C., Liu, J., Zhou, H., Qian, X., Sun, H., Chen, X., et al. (2021). NEIL3 May Act as a Potential Prognostic Biomarker for Lung Adenocarcinoma. Cancer Cel Int 21 (1), 228. doi:10.1186/s12935-021-01938-4
Keywords: biomarkers, pan-cancer, transcriptome analyses, diagnosis, therapeutic
Citation: Zhu L, Miao Y, Xi F, Jiang P, Xiao L, Jin X and Fang M (2022) Identification of Potential Biomarkers for Pan-Cancer Diagnosis and Prognosis Through the Integration of Large-Scale Transcriptomic Data. Front. Pharmacol. 13:870660. doi: 10.3389/fphar.2022.870660
Received: 10 February 2022; Accepted: 24 March 2022;
Published: 23 May 2022.
Edited by:
Peixin Dong, Hokkaido University, JapanReviewed by:
Sridhar Muthusami, Karpagam Academy of Higher Education, IndiaFukang Sun, Shanghai Jiao Tong University, China
Copyright © 2022 Zhu, Miao, Xi, Jiang, Xiao, Jin and Fang. This is an open-access article distributed under the terms of the Creative Commons Attribution License (CC BY). The use, distribution or reproduction in other forums is permitted, provided the original author(s) and the copyright owner(s) are credited and that the original publication in this journal is cited, in accordance with accepted academic practice. No use, distribution or reproduction is permitted which does not comply with these terms.
*Correspondence: Mingyan Fang, ZmFuZ21pbmd5YW5AZ2Vub21pY3MuY24=; Xin Jin, amlueGluQGdlbm9taWNzLmNu
†These authors have contributed equally to this work