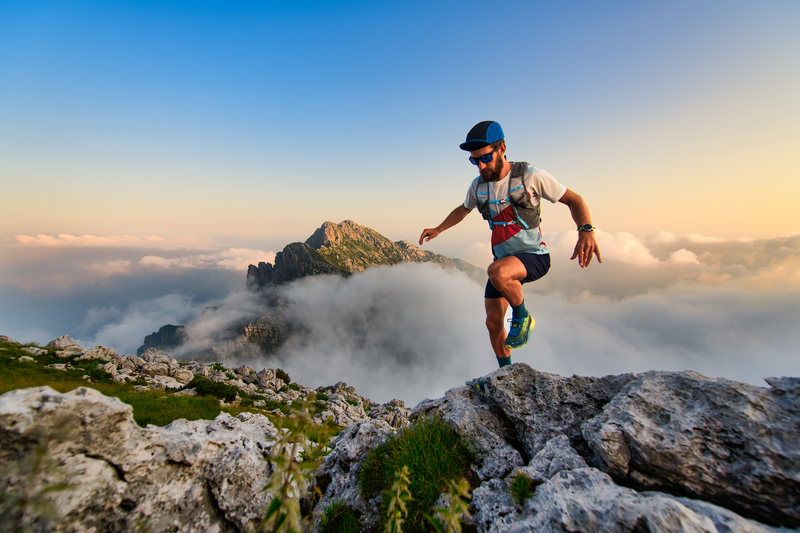
95% of researchers rate our articles as excellent or good
Learn more about the work of our research integrity team to safeguard the quality of each article we publish.
Find out more
DATA REPORT article
Front. Pharmacol. , 18 March 2022
Sec. Experimental Pharmacology and Drug Discovery
Volume 13 - 2022 | https://doi.org/10.3389/fphar.2022.869983
This article is part of the Research Topic Novel Approaches to The Treatment of Multidrug-Resistant Bacteria, volume II View all 8 articles
Antimicrobial resistance (AMR) is a pressing public health concern (Lv et al., 2021; Murray et al., 2022). It is estimated that by 2050, 10 million people will die from antimicrobial resistance, leading to economic losses of 100 billion U.S. dollars annually (O’neill, 2014). Much of this problem comes from the lack of innovation in antibiotic discovery. Most antibiotics were discovered within a few decades after the Second World War (Lv et al., 2021). Moreover, many pharmaceutical companies have abandoned projects searching for new antibiotics due to costs and market regulation. Therefore, alternative strategies for the treatment of bacterial infections are urgently required.
Drug combinations provide an effective strategy to combat antimicrobial resistance (Ryall and Tan, 2015; Tyers and Wright, 2019; Lv et al., 2021). Compared to monotherapy, drug combinations can provide improved efficacy with lower doses and/or slow the development of resistance (Tyers and Wright, 2019) and thus attracts the attention of both researchers and pharmaceutical companies (Ramsay et al., 2018). Previously, the effectiveness of drug combinations was determined through clinical trials. However, this approach is both expensive and time-consuming. In recent years, with the development of high-throughput screening (HTS) technology (Bajorath, 2002), it has been possible to simultaneously evaluate hundreds of drug combinations. Therefore, datasets of drug combinations are becoming more prevalent, and they also present an excellent opportunity for data-driven artificial intelligence (AI) models. However, these existing models often have “curse of dimensionality” problems, resulting in moderate accuracy (Chandrasekaran et al., 2016; Mason et al., 2017). Specifically, if the amount of available training data is fixed, then overfitting occurs if the number of features is much greater than the number of training sets. Therefore, a database to collect and integrate the growing antibiotic combination data is required. Wu et al. (Wu et al., 2021) summarized existing databases for drug combinations. These databases focus on a specific field, such as, anticancer (Liu et al., 2019; Seo et al., 2020; Zheng et al., 2021) or anti-fungal drug combinations (Chen et al., 2014). To our knowledge, a database for antibiotic combinations is not yet available.
In this study, we constructed a comprehensive database (Antibiotic Combination DataBase, ACDB) focused on antibiotic combinations (Figure 1). This current release of ACDB includes 6,175 antibiotic combinations that were manually collected from the literature, covering 304 unique compounds and 460 bacterial strains. In addition, we also provided descriptors (e.g., LogP, molecular weight), molecular fingerprints (e.g., MACCS Keys, Morgan fingerprints), targets for each compound and chemogenomic data. Such data are a valuable resource for data-driven AI models. We developed a user-friendly website where the data can easily be acquired and analyzed further by users. In this way, it should be useful in predicting new antibiotic combinations and, in turn, combatting antimicrobial resistance.
Many studies of antibiotic combinations have been reported in PubMed. To obtain high-quality antibiotic combinations, we manually collected literature-reported antibiotic combinations from thousands of studies. Specifically, the keywords “antibiotic (s),” “fractional inhibitory concentration,” “synerg*,” and “combination (s)” were used to retrieve related literature in PubMed, and we finally obtained 6,175 antibiotic combinations from 6,121 publications (Dec. 2021).
We then filtered out duplicate content and 304 unique compounds were obtained. We normalized them into a standard format (PubChem CID) because they are well-known and easily linked to external databases. Simplified Molecular Input Line Entry System (SMILES) of each compound was obtained from PubChem, and we used SMILES to calculate descriptors (e.g., LogP, molecular weight, Lipinski’s five rules, Table 1). Moreover, we provided an additional Python script that converts SMILES into Protein Data Bank (PDB) format for download and further studies (molecular docking and/or molecular dynamics simulations). Targets of each compound were collected from DrugBank (Wishart et al., 2007).
ACDB is a relational database. It is hosted on a cloud server (CentOS Linux release 8.5.2111), which employs Apache (version 2.4.37) and MySQL (version 8.1) as the web and database server, respectively. The website is built with PHP, HTML and CSS and it can be freely accessed at http://www.acdb.plus.
There are six sections (Home, About, Download, Contact, Help and Visualization) on the ACDB website. A user-family retrieval system for antibiotic combinations was available on the homepage. The retrieval system allows users to find antibiotic combinations of interest to them. The About page contained the overview and motivation of ACDB. The Download page contained many useful datasets. These datasets can be used for AI-based models, and we will cover these usages in detail later. On the Help page, users can learn how to use ACDB. On the Visualization page, users can upload the dose-effect matrix, and then it will be fitted with the Loewe model. Finally, a heatmap and an isosurface will be shown on the webpage. If users have any further questions, they can find our contact information in the Contact page.
ACDB aims to help researchers obtain datasets of antibiotic combinations for further applications and analysis. ACDB contains a large amount of data on structure, pharmacology, and well-documented antibiotic combinations, which contribute to the development of combination therapy. In this section, we list some potential applications of ACDB.
Since experimental approaches for distinguishing antibiotic combinations are expensive and time-consuming, an increasing number of researchers are using machine learning methods to predict potential antibiotic combinations (Chandrasekaran et al., 2016; Mason et al., 2017; Wu et al., 2021). To make it easier for users, ACDB incorporated a series of features including Lipinski’s five rules, molecular fingerprints (e.g., MACCS Keys, Morgan fingerprint) and chemogenomic data. These features have been successfully applied in previous work to predict potential antibiotic combinations. For example, Yilancioglu et al. (Yilancioglu et al., 2014) investigated the relationships between Lipinski’s five rules and synergistic drug combinations, and they found a significant correlation (r = 0.51, p = 3.6
While machine learning models can offer satisfactory outcomes, the mechanisms underlying the synergy effect are still poorly understood. As such, mechanism-driven methods are needed in order to predict antibiotic combinations. Network pharmacology provides a new paradigm to explore intricate relationships between drugs, genes, and diseases (Hopkins, 2008). ACDB provides targets for each compound and several common protein-protein interaction (PPI) networks. Furthermore, Cytoscape (Shannon et al., 2003) and the Python package networkx can be used to draw the PPI network and calculate topological parameters (e.g. degree, betweenness, eigenvector centrality) for each node. Zou et al. (Zou et al., 2012) used these topological parameters to explore the underlying mechanisms of drug combinations. Network-based proximity (Cheng et al., 2019; Lv et al., 2022) can also be used to measure the relationship of two drugs. Based on the ACDB, comprehensive studies of antibiotic combinations at the system level can be undertaken.
Pharmacologically, an antibiotic combination may produce synergy effect, additive effect, and antagonism effect (Cokol et al., 2011). Every antibiotic combination has its own advantages. For synergistic antibiotic combinations, they are frequently used in clinics because they can provide improved efficacy at lower dosages (Yeh et al., 2009). The combination of trimethoprim and sulfamethoxazole, for example, can interfere with folic acid synthesis in a synergistic way (Yeh et al., 2006). For antagonistic antibiotic combinations, they have been shown to slow down the evolution of AMR (Chait et al., 2007; Michel et al., 2008). However, the potency of antibiotic combinations is not immutable and it is affected by metabolic conditions (Cokol et al., 2018), bacterial strains (Chandrasekaran et al., 2016), etc. This is one of the important drivers for development of ACDB. Through the “Organism Search” in ACDB, users can obtain a series of species-specific antibiotic combinations and their efficacy. Undoubtedly, ACDB combined with antimicrobial susceptibility testing can help clinicians tailor treatments based on the pathogen microenvironment and the patient’s condition.
We introduce a freely available database focusing on antibiotic combinations. To our knowledge, ACDB is currently the only database utilizing this approach. ACDB contains a great number of well-documented drug combinations and structural, physicochemical, pharmacological and chemogenomic data. It should benefit the performance of AI-based models and to explore the mechanism of synergy effects. In future versions, combinations of antibiotics, human-targeted drugs, and plant extracts and more applications will be incorporated into this web-based program.
ACDB can be freely available at http://www.acdb.plus and we will update it annually.
JL and WD: database development, investigation, writing manuscript. YJ and YS: database testing, validation. GL: supervision, project administration, editing the manuscript. All authors have read and approved the manuscript.
This work was supported by the National Nature Science Foundation of China (grant numbers 61772226 and 61862056); Science and Technology Development Program of Jilin Province (grant number 20210204133YY); The Natural Science Foundation of Jilin Province (Grant number No. 20200201159JC).
The authors declare that the research was conducted in the absence of any commercial or financial relationships that could be construed as a potential conflict of interest.
All claims expressed in this article are solely those of the authors and do not necessarily represent those of their affiliated organizations, or those of the publisher, the editors and the reviewers. Any product that may be evaluated in this article, or claim that may be made by its manufacturer, is not guaranteed or endorsed by the publisher.
Bajorath, J. (2002). Integration of Virtual and High-Throughput Screening. Nat. Rev. Drug Discov. 1 (11), 882–894. doi:10.1038/nrd941
Chait, R., Craney, A., and Kishony, R. (2007). Antibiotic Interactions that Select against Resistance. Nature 446 (7136), 668–671. doi:10.1038/nature05685
Chandrasekaran, S., Cokol-Cakmak, M., Sahin, N., Yilancioglu, K., Kazan, H., Collins, J. J., et al. (2016). Chemogenomics and Orthology-Based Design of Antibiotic Combination Therapies. Mol. Syst. Biol. 12 (5), 872. doi:10.15252/msb.20156777
Chen, X., Ren, B., Chen, M., Liu, M. X., Ren, W., Wang, Q. X., et al. (2014). ASDCD: Antifungal Synergistic Drug Combination Database. Plos One 9 (1), e86499. doi:10.1371/journal.pone.0086499
Cheng, F., Kovács, I. A., and Barabási, A. L. (2019). Network-based Prediction of Drug Combinations. Nat. Commun. 10, 1197. doi:10.1038/s41467-019-09186-x
Cokol, M., Chua, H. N., Tasan, M., Mutlu, B., Weinstein, Z. B., Suzuki, Y., et al. (2011). Systematic Exploration of Synergistic Drug Pairs. Mol. Syst. Biol. 7 (1), 544. doi:10.1038/msb.2011.71
Cokol, M., Li, C., and Chandrasekaran, S. (2018). Chemogenomic Model Identifies Synergistic Drug Combinations Robust to the Pathogen Microenvironment. Plos Comput. Biol. 14 (12), e1006677. doi:10.1371/journal.pcbi.1006677
Hopkins, A. L. (2008). Network Pharmacology: the Next Paradigm in Drug Discovery. Nat. Chem. Biol. 4 (11), 682–690. doi:10.1038/nchembio.118
Liu, H., Zhang, W., Zou, B., Wang, J., Deng, Y., and Deng, L. (2019). DrugCombDB: a Comprehensive Database of Drug Combinations toward the Discovery of Combinatorial Therapy. Nucleic Acids Res. 48 (D1), D871–D881. doi:10.1093/nar/gkz1007
Lv, J., Deng, S., and Zhang, L. (2021). A Review of Artificial Intelligence Applications for Antimicrobial Resistance. Biosafety and Health 3 (1), 22–31. doi:10.1016/j.bsheal.2020.08.003
Lv, J., Liu, G., Ju, Y., Sun, Y., and Guo, W. (2022). Prediction of Synergistic Antibiotic Combinations by Graph Learning. Front. Pharmacol. 13, 849006. doi:10.3389/fphar.2022.849006
Mason, D. J., Stott, I., Ashenden, S., Weinstein, Z. B., Karakoc, I., Meral, S., et al. (2017). Prediction of Antibiotic Interactions Using Descriptors Derived from Molecular Structure. J. Med. Chem. 60 (9), 3902–3912. doi:10.1021/acs.jmedchem.7b00204
Michel, J. B., Yeh, P. J., Chait, R., Moellering, R. C., and Kishony, R. (2008). Drug Interactions Modulate the Potential for Evolution of Resistance. Proc. Natl. Acad. Sci. U S A. 105 (39), 14918–14923. doi:10.1073/pnas.0800944105
Murray, C. J. L., Ikuta, K. S., Sharara, F., Swetschinski, L., Robles Aguilar, G., Gray, A., et al. (2022). Global Burden of Bacterial Antimicrobial Resistance in 2019: A Systematic Analysis. The Lancet 399 (10325), 629–655. doi:10.1016/S0140-6736(21)02724-0
Nichols, R. J., Sen, S., Choo, Y. J., Beltrao, P., Zietek, M., Chaba, R., et al. (2011). Phenotypic Landscape of a Bacterial Cell. Cell 144 (1), 143–156. doi:10.1016/j.cell.2010.11.052
O’neill, J. (2014). Antimicrobial Resistance: Tackling a Crisis for the Future Health and Wealth of Nations. London: Review on Antimicrobial Resistance. Available at: http://amr-review.org/sites/default/files/AMR%20Review%20Paper%20-%20Tackling%20a%20crisis%20for%20the%20health%20and%20wealth%20of%20nations_1.pdf (Accessed January 15, 2022).
Ramsay, R. R., Popovic-Nikolic, M. R., Nikolic, K., Uliassi, E., and Bolognesi, M. L. (2018). A Perspective on Multi-Target Drug Discovery and Design for Complex Diseases. Clin. Transl. Med. 7 (1), 3. doi:10.1186/s40169-017-0181-2
Ryall, K. A., and Tan, A. C. (2015). Systems Biology Approaches for Advancing the Discovery of Effective Drug Combinations. J. Cheminform 7 (1), 7. doi:10.1186/s13321-015-0055-9
Seo, H., Tkachuk, D., Ho, C., Mammoliti, A., Rezaie, A., Madani Tonekaboni, S. A., et al. (2020). SYNERGxDB: an Integrative Pharmacogenomic portal to Identify Synergistic Drug Combinations for Precision Oncology. Nucleic Acids Res. 48 (W1), W494–W501. doi:10.1093/nar/gkaa421
Shannon, P., Markiel, A., Ozier, O., Baliga, N. S., Wang, J. T., Ramage, D., et al. (2003). Cytoscape: a Software Environment for Integrated Models of Biomolecular Interaction Networks. Genome Res. 13 (11), 2498–2504. doi:10.1101/gr.1239303
Tyers, M., and Wright, G. D. (2019). Drug Combinations: a Strategy to Extend the Life of Antibiotics in the 21st century. Nat. Rev. Microbiol. 17 (3), 141–155. doi:10.1038/s41579-018-0141-x
Whiteside, M. D., Winsor, G. L., Laird, M. R., and Brinkman, F. S. (2012). OrtholugeDB: a Bacterial and Archaeal Orthology Resource for Improved Comparative Genomic Analysis. Nucleic Acids Res. 41 (D1), D366–D376. doi:10.1093/nar/gks1241
Wishart, D. S., Knox, C., Guo, A. C., Cheng, D., Shrivastava, S., Tzur, D., et al. (2007). DrugBank: a Knowledgebase for Drugs, Drug Actions and Drug Targets. Nucleic Acids Res. 36 (Suppl. l_1), D901–D906. doi:10.1093/nar/gkm958
Wu, L., Wen, Y., Leng, D., Zhang, Q., Dai, C., Wang, Z., et al. (2021). Machine Learning Methods, Databases and Tools for Drug Combination Prediction. Brief. Bioinf 23, bbab355. doi:10.1093/bib/bbab355
Yeh, P., Tschumi, A. I., and Kishony, R. (2006). Functional Classification of Drugs by Properties of Their Pairwise Interactions. Nat. Genet. 38 (4), 489–494. doi:10.1038/ng1755
Yeh, P. J., Hegreness, M. J., Aiden, A. P., and Kishony, R. (2009). Drug Interactions and the Evolution of Antibiotic Resistance. Nat. Rev. Microbiol. 7 (6), 460–466. doi:10.1038/nrmicro2133
Yilancioglu, K., Weinstein, Z. B., Meydan, C., Akhmetov, A., Toprak, I., Durmaz, A., et al. (2014). Target-independent Prediction of Drug Synergies Using Only Drug Lipophilicity. J. Chem. Inf. Model. 54 (8), 2286–2293. doi:10.1021/ci500276x
Zheng, S., Aldahdooh, J., Shadbahr, T., Wang, Y., Aldahdooh, D., Bao, J., et al. (2021). DrugComb Update: a More Comprehensive Drug Sensitivity Data Repository and Analysis portal. Nucleic Acids Res. 49 (W1), W174–W184. doi:10.1093/nar/gkab438
Keywords: antimicrobial resistance, antibiotic combinations, synergy effect, fractional inhibitory concentration index, database
Citation: Lv J, Liu G, Dong W, Ju Y and Sun Y (2022) ACDB: An Antibiotic Combination DataBase. Front. Pharmacol. 13:869983. doi: 10.3389/fphar.2022.869983
Received: 05 February 2022; Accepted: 28 February 2022;
Published: 18 March 2022.
Edited by:
Priyia Pusparajah, Monash University Malaysia, MalaysiaReviewed by:
Stephen Zinner, Harvard Medical School, United StatesCopyright © 2022 Lv, Liu, Dong, Ju and Sun. This is an open-access article distributed under the terms of the Creative Commons Attribution License (CC BY). The use, distribution or reproduction in other forums is permitted, provided the original author(s) and the copyright owner(s) are credited and that the original publication in this journal is cited, in accordance with accepted academic practice. No use, distribution or reproduction is permitted which does not comply with these terms.
*Correspondence: Guixia Liu, bGl1Z3hAamx1LmVkdS5jbg==
Disclaimer: All claims expressed in this article are solely those of the authors and do not necessarily represent those of their affiliated organizations, or those of the publisher, the editors and the reviewers. Any product that may be evaluated in this article or claim that may be made by its manufacturer is not guaranteed or endorsed by the publisher.
Research integrity at Frontiers
Learn more about the work of our research integrity team to safeguard the quality of each article we publish.