- 1 Shenzhen Hospital of Integrated Traditional Chinese and Western Medicine, Shenzhen, China
- 2 The First Affiliated Hospital of Guangzhou University of Chinese Medicine, Guangzhou, China
- 3 The First Clinical Medical School, Guangzhou University of Chinese Medicine, Guangzhou, China
- 4 Shenzhen Bao’an District Traditional Chinese Medicine Hospital, Guangzhou University of Chinese Medicine, Shenzhen, China
- 5 Lingnan Medical Research Center of Guangzhou University of Chinese Medicine, Guangzhou, China
Objective: People suffering from coronavirus disease 2019 (COVID-19) are prone to develop pulmonary fibrosis (PF), but there is currently no definitive treatment for COVID-19/PF co-occurrence. Kaempferol with promising antiviral and anti-fibrotic effects is expected to become a potential treatment for COVID-19 and PF comorbidities. Therefore, this study explored the targets and molecular mechanisms of kaempferol against COVID-19/PF co-occurrence by bioinformatics and network pharmacology.
Methods: Various open-source databases and Venn Diagram tool were applied to confirm the targets of kaempferol against COVID-19/PF co-occurrence. Protein-protein interaction (PPI), MCODE, key transcription factors, tissue-specific enrichment, molecular docking, Gene ontology (GO) and Kyoto Encyclopedia of Genes and Genomes (KEGG) enrichment analyses were used to clarify the influential molecular mechanisms of kaempferol against COVID-19 and PF comorbidities.
Results: 290 targets and 203 transcription factors of kaempferol against COVID-19/PF co-occurrence were captured. Epidermal growth factor receptor (EGFR), proto-oncogene tyrosine-protein kinase SRC (SRC), mitogen-activated protein kinase 3 (MAPK3), mitogen-activated protein kinase 1 (MAPK1), mitogen-activated protein kinase 8 (MAPK8), RAC-alpha serine/threonine-protein kinase (AKT1), transcription factor p65 (RELA) and phosphatidylinositol 4,5-bisphosphate 3-kinase catalytic subunit alpha isoform (PIK3CA) were identified as the most critical targets, and kaempferol showed effective binding activities with the above critical eight targets. Further, anti-COVID-19/PF co-occurrence effects of kaempferol were associated with the regulation of inflammation, oxidative stress, immunity, virus infection, cell growth process and metabolism. EGFR, interleukin 17 (IL-17), tumor necrosis factor (TNF), hypoxia inducible factor 1 (HIF-1), phosphoinositide 3-kinase/AKT serine/threonine kinase (PI3K/AKT) and Toll-like receptor signaling pathways were identified as the key anti-COVID-19/PF co-occurrence pathways.
Conclusion: Kaempferol is a candidate treatment for COVID-19/PF co-occurrence. The underlying mechanisms may be related to the regulation of critical targets (EGFR, SRC, MAPK3, MAPK1, MAPK8, AKT1, RELA, PIK3CA and so on) and EGFR, IL-17, TNF, HIF-1, PI3K/AKT and Toll-like receptor signaling pathways. This study contributes to guiding development of new drugs for COVID-19 and PF comorbidities.
Introduction
The outbreak of coronavirus disease 2019 (COVID-19) in December 2019, with rising incidence and prevalence worldwide, has caused more than six million deaths (World Health Organization, 2022). Severe acute respiratory syndrome coronavirus-2 (SARS-CoV-2) is the trigger for COVID-19 pandemic, and belongs to the same coronavirus lineage that causes SARS (Zhu et al., 2020). Common clinical symptoms of SARS-CoV-2 infection include fever, cough, tiredness, shortness of breath and even death with exacerbation (Wu and McGoogan, 2020). Independent risk factors associated with COVID-19 include hypertension, diabetes, chronic obstructive pulmonary disease, and cardiovascular and cerebrovascular diseases (Wang et al., 2020). Although vaccine use has reduced the incidence of COVID-19, vaccinated people are still at risk of contracting SARS-CoV-2 and the number of COVID-19 cases remains high (Soleimanpour and Yaghoubi, 2021). Drugs against SARS-CoV-2 have been developed that reduce the risk of COVID-19 developing into severe COVID-19, but drug-resistant variants of SARS-CoV-2 may still emerge (Hammond et al., 2022). These shows that COVID-19 remains a serious threat to global health.
Pulmonary fibrosis (PF) is a pathological event caused by acute and chronic interstitial lung injury. PF causes chronic dyspnea, long-term disability and affects the quality of life of the patients (Lechowicz et al., 2020). PF is characterized by alveolar epithelium damage, inflammation infiltration, myofibroblasts activation and excessive deposition of extracellular matrix (ECM) (Giacomelli et al., 2021). Of note, CT images of 62 COVID-19 patients in Wuhan show vacuolar sign in more than half of them (Zhou et al., 2020). Diffuse alveolar damage, fibroblast proliferation and fibrosis are also found in autopsies of COVID-19 patients (Schaller et al., 2020). Alveolar epithelial type II (ATII) cells show a decreasing trend in SARS-CoV-2 infected patients (Delorey et al., 2021). The spike (S) protein of SARS-CoV-2 binds to angiotensin-converting enzyme 2 (ACE2) expressed in ATII cells to enter host cells (Ziegler et al., 2020; Celik et al., 2021). Damaged ATII cells can release transforming growth factor-β (TGF-β) (Tatler and Jenkins, 2012), platelet derived growth factor (Antoniades et al., 1990), connective tissue growth factor (Pan et al., 2001) and interluekin-6 (IL-6) (Crestani et al., 1994), thereby activating lung fibroblasts to increase ECM deposition and promote the development of PF (Sisson et al., 2010). The above researches reveal that COVID-19 patients are at high risk of developing PF (George et al., 2020). Obviously, COVID-19/PF co-occurrence is a catastrophic threat to global health, and it is unclear whether the damage caused by COVID-19/PF co-occurrence can be reversed (John et al., 2021). Therefore, it is an urgent need to find an influential treatment for COVID-19/PF co-occurrence.
Pirfenidone is one of the FDA-approved anti-fibrotic agents to treat idiopathic pulmonary fibrosis (IPF). Compared with methylprednisolone alone, pirfenidone and methylprednisolone combination therapy improves PF in hospitalized patients diagnosed with severe COVID-19 pneumonia (Acat et al., 2021). However, pirfenidone cannot prevent or reverse the progression of PF, which also limits its use in COVID-19/PF co-occurrence (Lancaster et al., 2019; Noble et al., 2011). There is no reported effective treatment for COVID-19/PF co-occurrence so far, thus the discovery of effective drugs against COVID-19/PF co-occurrence will contribute to improving patient prognosis and reducing social burdens. Surprisingly, it is confirmed that natural products have the effect of suppressing viral replication and transcription, and can inhibit cytokine storm and improve immunodeficiency (An et al., 2021). Natural product is also increasingly recognized as an alternative source for inhibiting fibrosis (Bahri et al., 2017). Natural products can reduce fibrosis by inhibiting inflammation, myofibroblast activation, ECM accumulation and epithelial-mesenchymal transition (EMT) (Chen et al., 2018). Natural products are a treasure trove for discovering new therapeutic drugs for COVID-19/PF co-occurrence. A natural product with dual antiviral and antifibrotic effects may have the great potential to become a therapeutic agent for COVID-19 and PF comorbidities.
Kaempferol, a natural flavonoid that widely exists in many fruits, vegetables and herbal medicine, is known as an antimicrobial, anti-inflammatory and antioxidant compound (Devi et al., 2015; Imran et al., 2019; Ren et al., 2019). Main protease (Mpro), a potential drug target for treating COVID-19, is found to be potentially inhibited by kaempferol (Khaerunnisa et al., 2020; Mahmud et al., 2021). Moreover, it is reported that kaempferol may directly target SARS-CoV-2 main protease (3CL pro) to perform anti-COVID effect (Shaldam et al., 2021; Zhang et al., 2021). Simultaneously, kaempferol inhibits the progression of silica-induced PF and attenuates fibrotic airway remodeling via modulating protease-activated receptor-1 activation (Gong et al., 2014; Liu et al., 2019). The above researches suggest that kaempferol has dual effects against COVID-19/PF co-occurrence, but the molecular mechanisms have not been investigated. Therefore, drug-target, disease-target and critical targets among COVID-19, PF and kaempferol were captured. Protein-protein interaction (PPI), MCODE, transcription factors, tissue-specific enrichment, molecular docking, Kyoto Encyclopedia of Genes and Genomes (KEGG) pathways and Gene Ontology (GO) analyses were performed. The detailed strategy of exploring the targets and mechanisms of kaempferol against COVID-19/PF co-occurrence by bioinformatics and network pharmacology is shown in Figure 1.
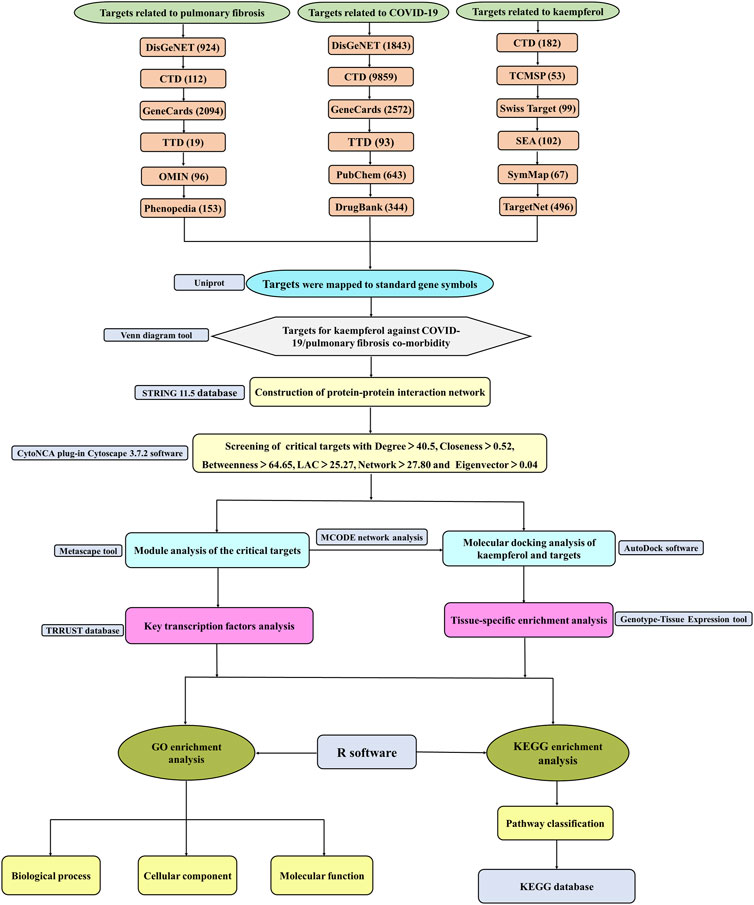
FIGURE 1. The flow diagram of a pragmatic strategy for identifying the pharmacological mechanism of kaempferol against COVID-19/pulmonary fibrosis co-occurrence based on system pharmacology and bioinformatics analysis.
Materials and Methods
Screening for Drug-Related Targets
The targets associated with kaempferol were retrieved from Comparative Toxicoomics Database (CTD, http://ctdbase.org/, accessed date: 3 September 2021) (Davis et al., 2021), Traditional Chinese Medicine Systems Pharmacology Database and Analysis Platform (TCMSP, https://tcmspe.com/, accessed date: 2 September 2021) (Ru et al., 2014), Swiss Target Prediction (http://swisstargetprediction.ch/, accessed date: 2 September 2021) (Daina et al., 2019), Similarity Ensemble Approach (SEA, https://sea.bkslab.org/, accessed date: 2 September 2021) (Keiser et al., 2007), SymMap (https://www.symmap.org/, accessed date: 2 September 2021) (Wu et al., 2019) and TargetNet (http://targetnet.scbdd.com/, accessed date: 2 September 2021) (Yao et al., 2016).
Collection of Disease-Related Targets
Targets related to COVID-19 were obtained from DisGeNET (https://www.disgenet.org/home/, accessed date: 2 September 2021) (Pinero et al., 2017), CTD, GeneCards (https://www.genecards.org/, accessed date: 3 September 2021) (Rebhan et al., 1997), Therapeutic Target Database (TTD, http://db.idrblab.net/ttd/, accessed date: 3 September 2021) (Wang et al., 2020), PubChem (https://pubchem.ncbi.nlm.nih.gov/, accessed date: 2 September 2021) (Kim et al., 2021) and DrugBank database (https://www.drugbank.com/, accessed date: 4 September 2021) (Wishart et al., 2018).
Six databases were used to obtained PF-related targets including DisGeNET, CTD, GeneCards, TTD, Online Mendelian Inheritance in Man (OMIM, https://omim.org/, accessed date: 2 September 2021) (Amberger et al., 2015) and Phenopedia (https://phgkb.cdc.gov/PHGKB/startPagePhenoPedia.action, accessed date: 2 September 2021) (Yu et al., 2010). Targets were mapped to standard symbols by using Uniprot database (https://www.uniprot.org/, accessed date: 2 September 2021) (UniProt, 2015).
Analysis of Overlapping Targets Between Drug and Diseases
The Venn package of R 3.6.2 software was used to draw the petal map. Venn diagram showing the intersection of the targets of kaempferol against COVID-19/PF co-morbidity was plotted by the Venn Diagram tool (http://bioinformatics.psb.ugent.be/webtools/Venn/) and Microsoft Excel.
Protein-Protein Interaction Network Construction and Critical Targets Analysis
The shared targets between diseases and drug were put into the STRING 11.5 database (https://string-db.org/, accessed date: 6 September 2021) (Szklarczyk et al., 2021) to construct a PPI network. The organism was set to “Homo sapiens” and the minimum required interaction score was set to 0.4. Then the PPI network was visualized by Cytoscape 3.7.2 software (https://cytoscape.org/) (Otasek et al., 2019). The CytoNCA plug-in Cytoscape 3.7.2 software was applied to calculate topological parameters including degree, closeness, betweenness, LAC, network and eigenvector (Tang et al., 2015). Regarding the medians of topological parameters as the screening threshold, the overlapping targets above the threshold were identified as critical targets.
Module Analysis of Critical Targets
Metascape (http://metascape.org/, accessed date: 7 September 2021) was used to perform module analysis of critical targets (Zhou et al., 2019). MCODE score (Bader and Hogue, 2003) was applied to cluster the most significant modules. Code score was calculated on the connection density of the adjacent area, and the target in MCODE module with greater degree value was considered to play a more important role in treating COVID-19/PF co-morbidity. Of note, the top five targets with the highest degree values in MCODE modules were selected to perform molecular docking analysis.
Key Transcription Factors Analysis of Critical Targets
Transcriptional Regulatory Relationships Unraveled by Sentence-based Text mining (TRRUST, https://www.grnpedia.org/trrust/, accessed date: 7 September 2021) is a useful tool for predicting transcriptional regulatory network (Han et al., 2018). The TRRUST database provides abundant information of 8,444 transcription factors (TFs)-target network. Critical targets were input to TRRUST database with the species of “Human.” The top 10 TFs ranking based on p value from small to large were selected to construct the TFs-target network by using Cytoscape 3.7.2 software.
Tissue-Specific Enrichment Analysis of Critical Targets
Genotype-Tissue Expression (GETx) (https://www.gtexportal.org/, accessed date: 7 September 2021) is an online tool to study genetic variation and expression of human tissues (Consortium, 2013). The top 50 targets ranking based on modules’ degree values from high to low were selected for tissue-specific enrichment analysis. The heat map showed the correlation between different samples and targets, and more the important tissues corresponding to the targets would show darker colors.
Gene Ontology and Kyoto Encyclopedia of Genes and Genomes Enrichment Analyses of Critical Targets
GO enrichment analysis included biological process (BP), molecular function (MF) and cellular component (CC), as well as KEGG pathway enrichment analysis were conducted in R 3.6.2 software. “Org.hs.eg.db” (https://www.bioconductor.org/packages/org.Hs.eg.db, accessed date: 7 September 2021) was used to match the gene ID corresponding to critical targets. Then “cluster Profiler” package (Wu et al., 2021) was used to perform enrichment analysis with the criteria of pvalueCutoff = 0.05 and qvalueCutoff = 0.05. Based on adjusted p value in ascending order, the top 20 enrichment results were selected to display as a bubble chart by bioinformatics tool (http://www.bioinformatics.com.cn/). Furthermore, the KEGG pathways were classified based on KEGG databases and visualized by hiplot (https://hiplot.com.cn/).
Molecular Docking Analysis of the Top Five Targets
Molecular docking is widely applied in drug detection and is often used to predict the relationship between targets and ligand. Molecular docking was carried out between kaempferol and the top five targets via AutoDock software (Vina 1.5.6, http://autodock.scripps.edu/) (Shen et al., 2021; Trott and Olson, 2010), which was often used to calculate the molecular interaction force between protein and ligand. The small-molecule two-dimensional structure format information of kaempferol was obtained from PubChem database (https://pubchem.ncbi.nlm.nih.gov/) and saved in the SDF format. The SDF molecular structure file of kaempferol was converted into a PDB file by Open Babel software. The three-dimensional structure of key target proteins was downloaded from the RCSB PDB database (https://www.rcsb.org/) (Rose et al., 2021). The Auto Dock Tools 1.5.6 software was used to convert the molecular structure document into PDBQT format and perform molecular docking. The PyMol 2.3.2 software was used to visualize the results with higher docking scores and calculate the corresponding RMSD values.
Results
Targets of Kaempferol Against COVID-19/PF Co-Occurrence
As shown in Figure 2A unique PF-related targets were obtained from DisGeNET (924), CTD (112), GeneCards (2,094), TTD (19), OMIM (96) and Phenopedia (153). As shown in Figure 2B, 11,457 unique targets of COVID-19 were retrieved from DisGeNET (1,843), CTD (9,859), GeneCards (2,572), TTD (93), PubChem (643) and DrugBank (344). As shown in Figure 2C, 737 unique targets related to kaempferol were identified from CTD (182), TCMSP (53), Swiss Target Prediction (99), SEA (102), SymMap (67) and TargetNet (496). Finally, 290 targets of kaempferol against COVID-19/PF co-occurrence were obtained (Figure 2D).
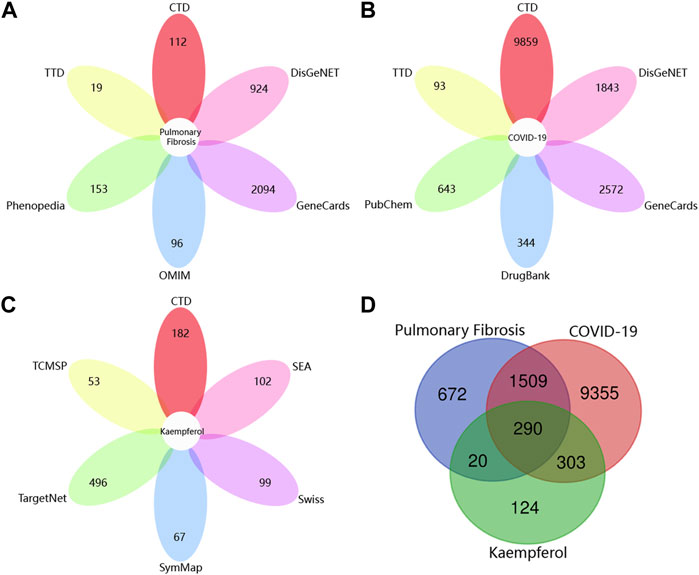
FIGURE 2. Collection of targets related to drug and diseases from various open-source databases. (A) The number of pulmonary fibrosis-related targets from six open-source databases. (B) The number of targets related to COVID-19 from six open-source databases. (C) The number of targets associated with kaempferol from six open-source databases. (D) Venn diagram depicting common targets between COVID-19, pulmonary fibrosis and kaempferol.
Protein-Protein Interaction Network Construction and Critical Targets Acquisition
The nodes represented shared targets and the edges indicated protein-protein interactions between shared targets in PPI network. PPI network of 290 common targets shown in Figure 3A contained 290 nodes and 7,431 edges. Through the topological identification and calculation of PPI network, the medians of the topological parameter were degree = 40.5, closeness = 0.52, betweenness = 64.65, LAC = 25.27, network = 27.80 and eigenvector = 0.04. Then 115 critical targets with the topological parameters greater than the medians of above six topological factors were screened out to construct PPI network of critical targets. There were 115 nodes and 3,639 edges in the PPI network of critical targets as shown in Figure 3B.
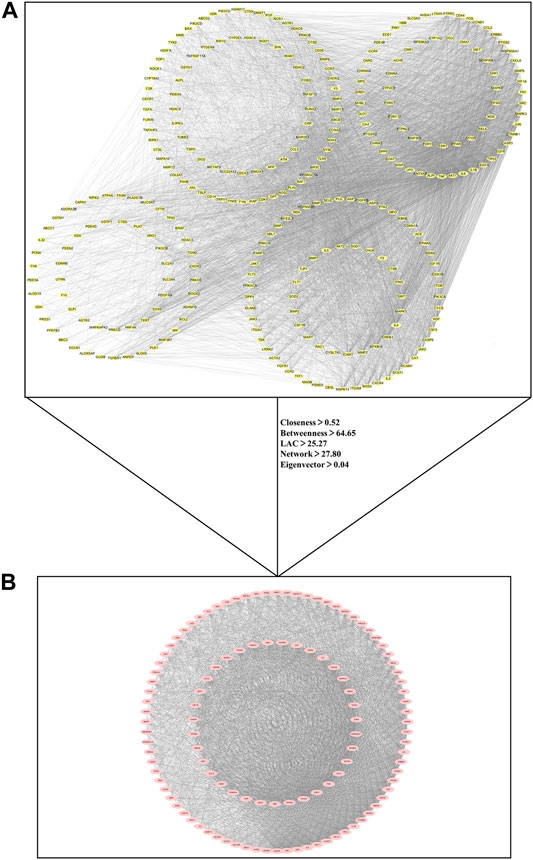
FIGURE 3. Protein-protein interaction (PPI) network for critical targets of kaempferol against COVID-19/pulmonary fibrosis co-occurrence. Nodes represent targets and edges represent protein-protein interactions. (A) PPI network of 290 common targets between COVID-19, pulmonary fibrosis and kaempferol. (B) PPI network of 115 critical targets for kaempferol against COVID-19/pulmonary fibrosis co-occurrence.
Investigation of Important Modules
Module analysis was carried out by using Metascape tool and five functional clusters were shown in Figures 4A–E. Module 1 included 28 nodes and 132 edges with MCODE score = 4.714. Module 2 contained 24 nodes and 205 edges with MCODE score = 8.541. Module 3 included 21 nodes and 62 edges with MCODE score = 2.952. Module 4 comprised of 4 nodes and 4 edges with MCODE score = 1.000. Module 5 included 3 nodes and 3 edges with MCODE score = 1.000. The top five targets with the highest degree scores were epidermal growth factor receptor (EGFR, degree = 23), proto-oncogene tyrosine-protein kinase SRC (SRC, degree = 21), mitogen-activated protein kinase 3 (MAPK3, degree = 21), mitogen-activated protein kinase 1 (MAPK1, degree = 21), mitogen-activated protein kinase 8 (MAPK8, degree = 20), RAC-alpha serine/thre onine-protein kinase (AKT1, degree = 20), transcription factor p65 (RELA, degree = 19) and phosphatidylinositol 4,5-bisphosphate 3-kinase catalytic subunit alpha isoform (PIK3CA, degree = 18).
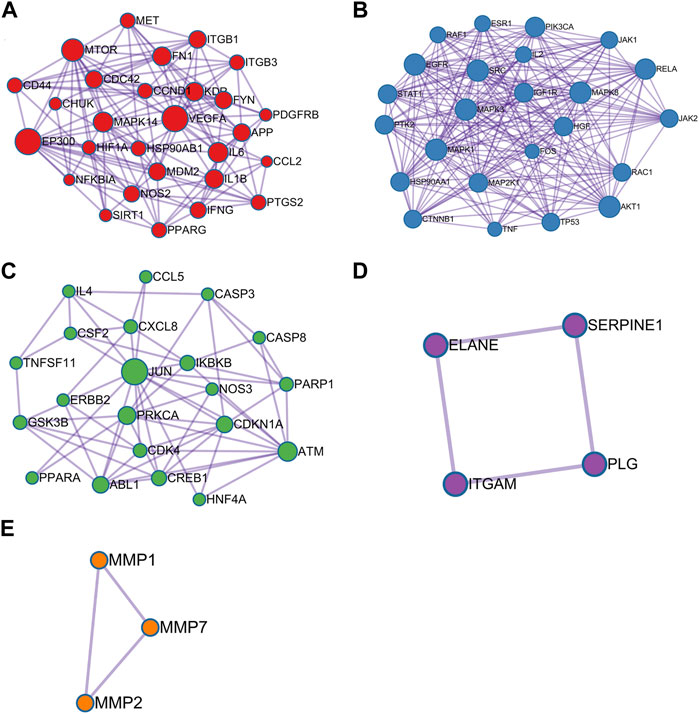
FIGURE 4. Module analysis of 115 critical genes is performed by the Metascape tool. Each module demonstrats different biological process functions. (A) Module 1; (B) Module 2; (C) Module 3; (D) Module 4; (E) Module 5.
Key Transcription Factors Acquisition
115 critical targets were input to the TRRUST database and 203 TFs were obtained. TFs-target network contained 97 nodes including 10 TFs, 87 targets and 278 edges (Figure 5). Red nodes represented TFs and purple nodes represented corresponding targets, and the edge indicated the relevance between TFs and corresponding targets. The size of the red node was negatively correlated with p value, the larger the size of the red node was, the more important it is in the TFs-target network. Especially, there were four critical targets that were also predicted as TFs, including signal transducerand activator of transcription 1 (STAT1), tumor protein P53 (TP53), JUN proto-oncogene, AP-1 transcription factor subunit (JUN) and RELA. The detailed information of the top 10 TFs were listed in Table 1.
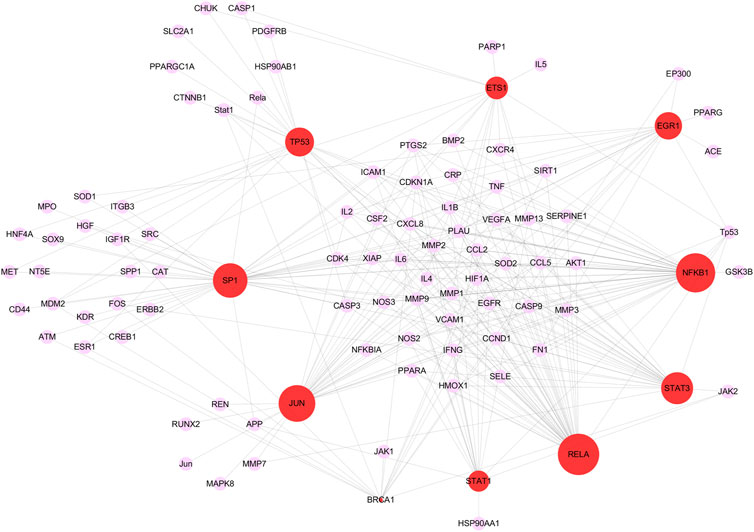
FIGURE 5. The top 10 key transcription factors (TFs) of 115 critical targets. The red nodes represent TFs and the purple nodes represent corresponding targets. The edges represent the connection between TFs and targets. The sizes of red nodes present negative correlation with p values and a node with larger shape represents the more important role in treating COVID-19/pulmonary fibrosis co-occurrence.
Critical Targets Were Mostly Enriched in Lung Tissue
Tissues were represented on the abscissa and targets were indicated on the ordinate (Figure 6). The data was presented as a heat map and the color indicated the level of enrichment. The darker the color was, the higher the expression level of critical target in corresponding tissue was. The result indicated that most critical targets were highly expressed in lung tissue, especially fibronectin 1 (FN1), heat shock protein 90 alpha family class B member 1 (HSP90AB1), fos proto-oncogene, AP-1 transcription factor subunit (FOS), JUN, RAC family small GTPase 1 (RAC1), vascular endothelial growth factor A (VEGFA), ABL proto-oncogene 1, non-receptor tyrosine kinase (ABL1), RELA, heat shock protein 90 alpha family class A member 1 (HSP90AA1) and so on.
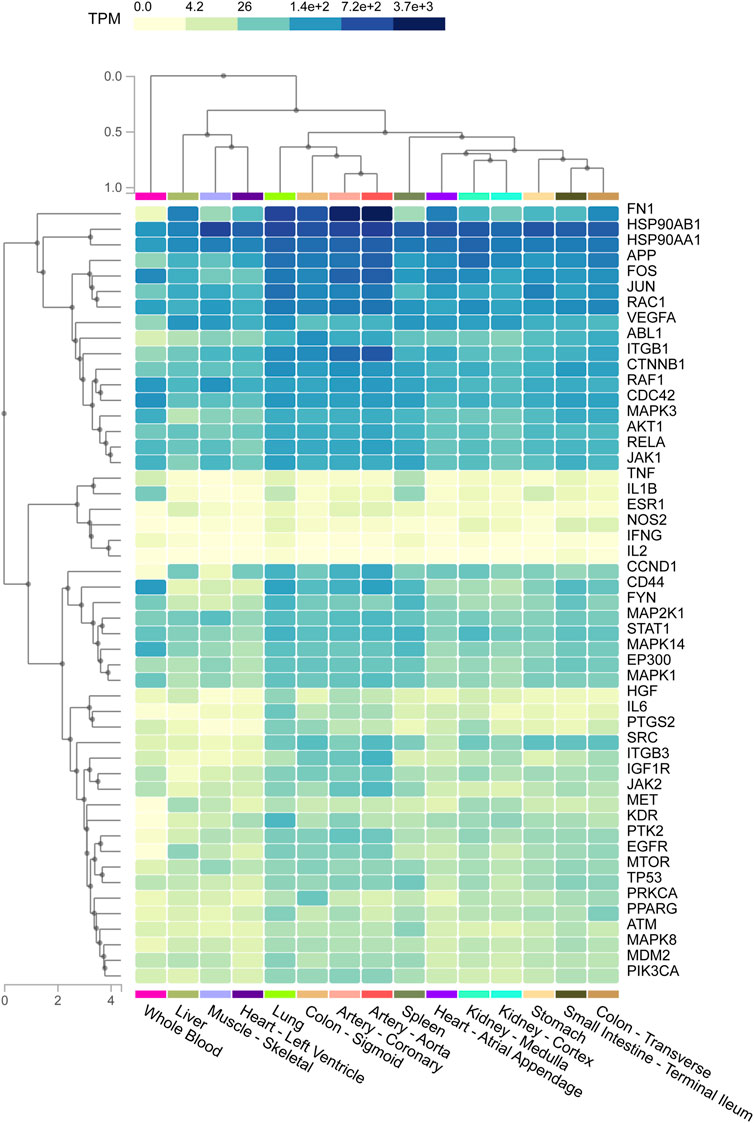
FIGURE 6. The heat map shows the relationship between different tissue samples and critical targets. Column represents critical targets and row represents enriched tissues. The shades of colors represent the levels of enrichment of critical targets in tissues, and the darker the color indicates the more significant enrichment of targets in corresponding tissues.
Gene Ontology Enrichment Analysis
2,958 GO terms were obtained, of which 2,705 belonged to GO-BP, 94 to GO-CC and 159 to GO-MF. The top 20 GO terms were respectively shown in Figures 7A–C. As for GO-BP, critical targets were mainly enriched in response to lipopolysaccharide, response to molecule of bacterial origin, response to oxidative stress, cellular response to biotic stimulus, response to antibiotic, regulation of cell-cell adhesion and so on. As for GO-MF, critical targets were mainly enriched in cytokine receptor binding, phosphatase binding, protein tyrosine kinase activity, growth factor receptor binding, protein phosphatase binding and so on. As for GO-CC, critical targets were mainly enriched in membrane raft, membrane microdomain, membrane region, focal adhesion, cell-substrate adherens junction and so on.
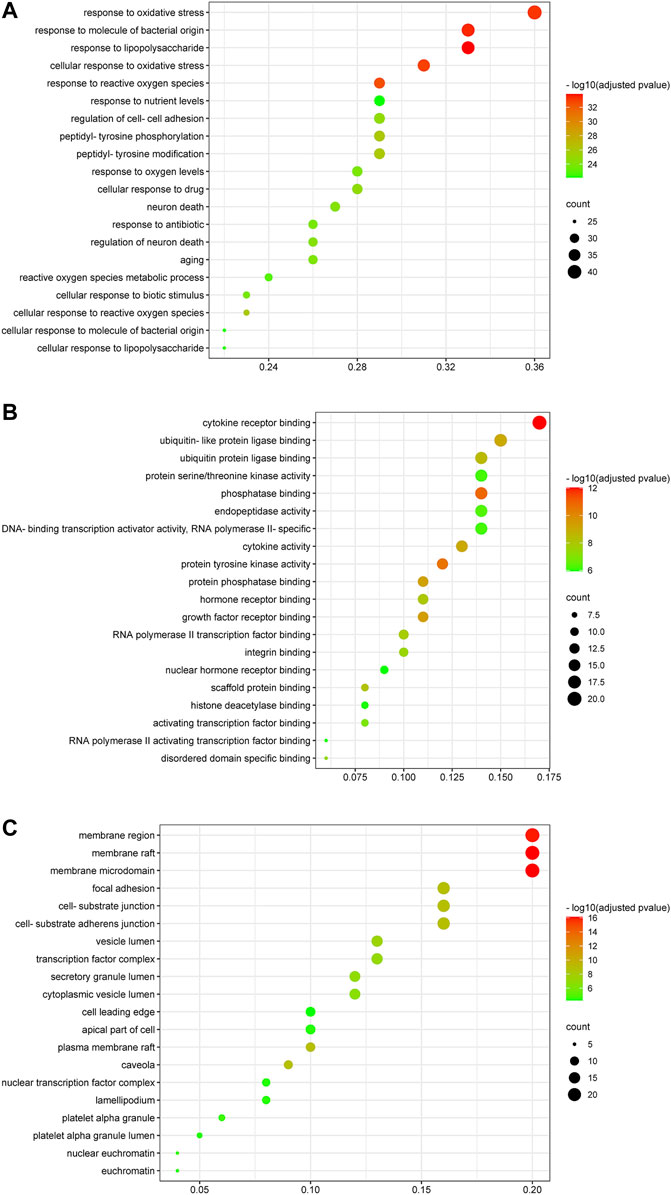
FIGURE 7. Gene ontology enrichment analysis of critical targets. The size of the node represents the number of genes involved in the GO term, and the color from green to red indicates the −log10 (adjusted p value) from small to large. (A) Biological process enrichment results of critical targets. (B) Molecular function enrichment results of critical targets. (C) Cellular components enrichment results of critical targets.
Kyoto Encyclopedia of Genes and Genomes Enrichment Analysis
174 KEGG terms were acquired and the top 20 KEGG terms were shown in Figure 8. Critical targets were mainly enriched in the EGFR tyrosine kinase inhibitor resistance, interleukin 17 (IL-17) signaling pathway, tumor necrosis factor (TNF) signaling pathway, Toll-like receptor signaling pathway, Yersinia infection, advanced glycation end product-receptor for advanced glycation end product (AGE-RAGE) signaling pathway in diabetic complications, hypoxia inducible factor 1 (HIF-1) signaling pathway, T cell receptor signaling pathway, C-type lectin receptor signaling pathway, Th17 cell differentiation, phosphoinositide 3-kinase/AKT serine/threonine kinase (PI3K/Akt) signaling pathway and so on. The results of KEGG pathway enrichment analysis were classified into five types, containing inflammation, oxidative stress, immunity, virus infection, cell growth processes and metabolism (Figure 9).
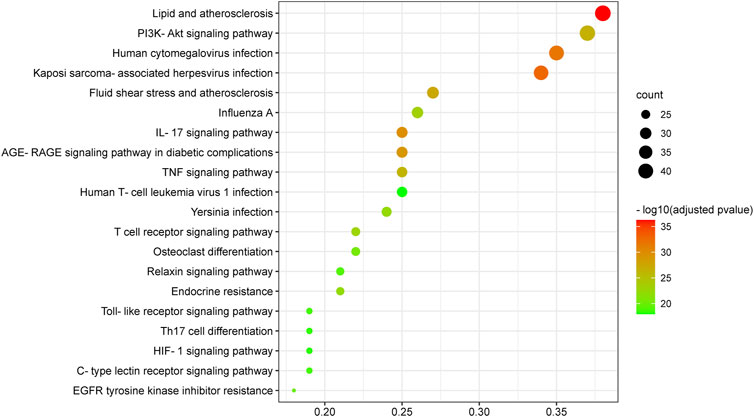
FIGURE 8. Kyoto Encyclopedia of Genes and Genomes enrichment analysis of critical targets. The size of the node represents the number of genes involved in the enrichment pathway, and the color from green to red indicates the −log10 (adjusted p value) from small to large.
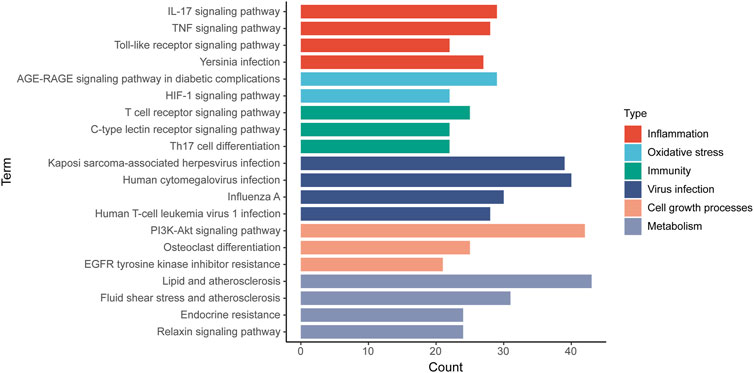
FIGURE 9. The classification of KEGG pathways. The results of KEGG pathway enrichment analysis are classified into five types and a color represents a type. Column represents KEGG pathway terms and row represents the number of targets enriched on the pathways.
Kaempferol Had Good Binding Activities With Critical Targets
To investigate whether kaempferol directly binds to EGFR, MAPK1, MAPK3, SRC, AKT1, MAPK8, RELA and PIK3CA (the top five targets with the highest degree values), molecular docking analysis was performed by Auto Dock Tools software. A binding energy less than 0 indicates spontaneous binding of ligand and receptor. The lower binding energy indicates a better binding effect. It is generally believed that binding energy < −5 kcal mol−1 indicates a good binding activity. Moreover, the stability of the simulated molecular docking systems was investigated by the root-mean-square deviation (RMSD), and it means the system is stable when RMSD is lower than 2 Å. The molecular docking results showed that the binding energies of kaempferol and the eight critical targets ranged from −6.23 to −8.15 kcal mol−1 (Table 2). All the simulated molecular docking reached the RMSD value range required for stability. The better docking result was selected for molecular docking visualization by using PyMol 2.3.2 software. The results showed that 2-5 hydrogen bonds could be formed between kaempferol and the eight critical targets (Figure 10). Molecular docking results proved that kaempferol had good binding activities with the eight critical targets.
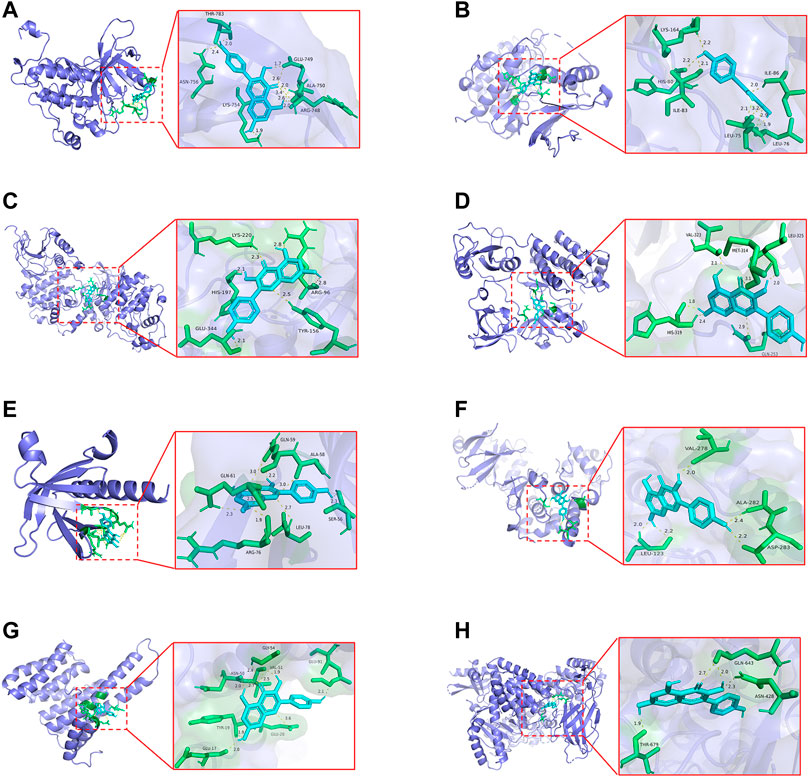
FIGURE 10. The docking models of kaempferol with the identified the top eight critical targets. (A) Docking results of kaempferol and EGFR. (B) Docking results of kaempferol and MAPK1. (C) Docking results of kaempferol and MAPK3. (D) Docking results of kaempferol and SRC. (E) Docking results of kaempferol and AKT1. (F)Docking results of kaempferol and MAPK8. (G)Docking results of kaempferol and RELA. (H)Docking results of kaempferol and PIK3CA.
Discussion
The prevention and treatment of COVID-19 related complications are public concerns. COVID-19/PF co-occurrence is a common and threatening condition, and early intervention is important for improving prognosis of pulmonary complications caused by SARS-CoV-2 infection (Pan et al., 2020). Traditional natural products have the effect of inhibiting viral replication and transcription, reducing cytokine storm and ameliorating immunodeficiency (An, et al., 2021). Further, growing evidence shows that natural products are alternative sources for improving fibrosis (Bahri, et al., 2017). Therefore, it reveals that natural product is a treasure trove for discovering new therapeutic drugs. It has been confirmed that kaempferol alleviates H9N2 influenza virus-induced inflammation and acute lung injury (Zhang et al., 2017). Kaempferol can also inhibit the virus replication of the pseudorabies virus in mice (Li et al., 2021). Moreover, kaempferol is proved to inhibit the activity of the Japanese encephalitis virus in BHK-21 cells (Care et al., 2020). Except for the antiviral effect, the anti-PF effect of kaempferol is also verified by a silica-induced PF mice model (Liu, et al., 2019). The above evidences indicate that kaempferol with dual antiviral and anti-PF effects may be the promising medicine for treating COVID-19/PF co-occurrence. Thus, this study analyzed potential targets and mechanisms of kaempferol against COVID-19/PF co-occurrence by integrating bioinformatics and system pharmacological tools.
First, 290 common targets between kaempferol, COVID-19 and PF were obtained, and then 115 critical targets with greater topological parameters in the PPI network were screened out. The top five targets from the 115 critical targets were identified, including EGFR (degree = 23), SRC (degree = 21), MAPK3 (degree = 21), MAPK1 (degree = 21), MAPK8 (degree = 20), AKT1 (degree = 20), RELA (degree = 19) and PIK3CA (degree = 18). Computer modelling approaches show that kaempferol has a high binding affinity to 3CLpro (Shaldam, et al., 2021; Zhang, et al., 2021). In vitro experiment confirms that kaempferol has strong inhibitory effects on 3CLpro (Khan et al., 2021). Of note, except for the direct effect on virus-produced proteins, downstream molecules or signaling pathways during the pathologic process are also potential mechanisms for kaempferol against COVID-19/PF co-occurrence. Surprisely, molecular docking analysis found that kaempferol showed promising binding activities with the top five targets (EGFR, SRC, MAPK3, MAPK1, MAPK8, AKT1, RELA and PIK3CA). EGFR inhibitors are proved to have antiviral and antifibrotic effects based on the Viral Fibrotic score, indicating that EGFR may be a critical regulator of COVID-19/PF co-occurrence (Vagapova et al., 2021). SRC is involved in the pathogenesis of PF by regulating EMT, myofibroblast differentiation and inflammation.
Xu et al. (2020), and a recent study reports that targeting SRC reduces titers of SARS-CoV-2 (Meyer et al., 2021). AKT shows an increased trend in various fibrotic diseases (Lu et al., 2010; Huang et al., 2011), and it also increases in fibroblasts of bleomycin-induced IPF in vivo and in vitro (Vittal et al., 2005; Xia et al., 2008; Le Cras et al., 2010). Moreover, deficiency of AKT1 significantly inhibits viral RNA expression (Esfandiarei et al., 2004), and PI3K/AKT kinase inhibitors are found to suppress the replication of middle east respiratory syndrome (MERS) (Kindrachuk et al., 2015). The first identified member of the MAPK pathway is extracellular signal-regulated kinase (ERK)1/2, which overexpresses in IPF (Antoniou et al., 2010). A study confirms that inhibition of ERK1/2 attenuates bleomycin-mediated PF by inhibiting EMT (Zou et al., 2020). In addition, MAPK is also involved in regulating virus replication, immune response and apoptosis of virus-infected cells (Bian et al., 2011; Gaur et al., 2011). It is worth noting that p38 MAPK inhibitor effectively prevents the phosphorylation of heat shock protein 27, cathelicidin antimicrobial peptide response element-binding protein and eukaryotic initiation factor 4E in SARS-CoV infected cells (Mizutani et al., 2004). RELA regulates the interferon IFN response during SARS-CoV-2 infection (Yin et al., 2021), and inhibition of RELA contributes to improving PF (Hou et al., 2018). PIK3CA belongs to the lipid kinase family and is responsible for coordinating functions such as proliferation, vesicle trafficking, and protein synthesis in various cells (Maheshwari et al., 2017). The above results reveal that targeting the critical targets especially the top five targets may be the potential therapeutic approach for kaempferol against COVID-19/PF co-occurrence.
Abnormal TFs activation and subsequent abnormal pathogenic genes expression play important roles in disease progression. The top 10 TFs were identified from 115 critical targets, and RELA was the most significant TF with the smallest p value among the top 10 TFs. The activation of RELA, a subtype of nuclear factor kappa-B (NF-κB), enhances the expression of TGF-β1 (Rameshwar et al., 2000). TGF-β1 is a key pro-fibrotic factor that has been proved to promote the transition of fibroblast to myofibroblast in PF (Andersson-Sjoland et al., 2008; Goodwin and Jenkins, 2009). ACT001 (NF-ĸB inhibitor) attenuates PF through decreasing the transition of fibroblast to myofibroblast, inhibiting IL-6 production and fibronectin deposition (Jaffaret al., 2021). Increased inflammatory cytokines and chemokines levels result in spontaneous haemorrhage, thrombocytopenia and systemic inflammation, which are the main manifestations of the fatal cytokine syndrome in advanced COVID-19 patients (Song et al., 2020; Xu et al., 2020). The activation of NF-ĸB enhances the expression of inflammatory cytokines and chemokines, including IL-1, IL-6, IL-8 and TNF-α (Liao et al., 2005; Wang et al., 2007). Selective bruton tyrosine kinase inhibitor inhibits NF-ĸB at the RELA phosphorylation stage, which leads to the reduction of C-reactive protein and IL-6 and an improvement of oxygen saturation (Roschewski et al., 2020). Further, to explore the association between tissues and critical targets, tissue-specific enrichment analysis was performed. The results showed that FN1, HSP90AB1, HSP90AA1 and so on were significantly enriched in the lung tissues. One of the characteristics of PF is excessive deposition of ECM proteins such as fibronectin (Liu et al., 2017). Elevated fibronectin deposition has been found in the lung tissues of PF patients (Liu et al., 2019), and it has been suggested that SARS-CoV-2 infection may promote the fibronectin expression in alveolar epithelial cells (Xu et al., 2020). HSP90 plays an important role in the folding, maturation and stabilization of proteins, and is therefore required for replication of multiple DNA and RNA viruses (Nagy et al., 2011). HSP90 inhibitor could inhibit virus replication, thus inhibition of HSP90 may be an effective strategy against SARS-CoV-2 infection (Li et al., 2020). In addition, increasing evidence shows that HSP90 is closely related to fibrogenesis (Bellaye et al., 2014), and overexpression of HSP90 emerges as a hallmark pathological step indicating the fibrogenesis progress (Sontake et al., 2017; Bellaye et al., 2018). Immunohistochemistry study reveals that HSP90α and HSP90β are overexpressed in the lungs of IPF patients (Sibinska et al., 2017). HSP90α participates in the PF progress through promoting the phosphorylation of AKT in P38 and ERK signaling pathways (Dong et al., 2017). The above descriptions indicate that targeting critical targets and TFs to regulate downstream genes may contribute to improving the condition of COVID-19/PF co-occurrence.
The biological process and molecular mechanisms of critical targets were further analyzed by GO and KEGG enrichment analyses. Critical targets were found to be strongly associated with regulation of virus infection, oxidative stress, inflammation, immune response and metabolic process. One of the characteristics of oxidative stress is the excessive production of reactive oxygen species (ROS) that damage lung tissues over time (Otoupalova et al., 2020). In response to lung tissues damage, lung fibroblasts proliferate and migrate to the damaged area to differentiate into myofibroblasts, causing increased fibronectin, type I and III collagen (Thannickal et al., 2004). Furthermore, oxidative stress participates in the pathogenesis of COVID-19, and SARS-CoV-2 infection induces oxidative stress through increasing the production of ROS and inhibiting antioxidant capacity mediated by the nuclear factor erythroid 2-related factor 2 in the host (Olagnier et al., 2020). Unfortunately, raised oxidative stress will induce inflammatory cascades that ultimately lead to in apoptosis, lung injury and dysregulated of immune responses (Delgado-Roche and Mesta, 2020). The GO result suggests that the effect of kaempferol against COVID-19/PF co-occurrence may be closely associated with the regulation of biological process of oxidative stress, inflammation, immune response and metabolic process.
Furthermore, it was pleasant to find that critical targets were mainly involved in oxidative stress, inflammation, cell growth process, metabolism, immunity and virus infection-related pathways. Among the KEGG pathways, IL-17 signaling pathway, TNF signaling pathway, Toll-like receptor signaling pathway, HIF-1 signaling pathway, EGFR tyrosine kinase inhibitor resistance and PI3K/Akt signaling pathway showed significant significance. IL-17 is found to be highly expressed in patients with COVID-19 and PF comorbidities (Nuovo et al., 2012; Jahaj et al., 2021). Circulating IL-17 is overexpressed in severe COVID-19 patients compared to severe non-COVID-19 patients (Jahaj, et al., 2021). IL-17 signaling pathway is closely related to T helper (Th)17 cell differentiation and exacerbates cytokine storm during SARS-CoV-2 infection (Wu and Yang, 2020). High levels of IL-17 are also found in the lung tissues of IPF patients, which demonstrates that IL-17 signaling pathway is related to IPF progress (Nuovo, et al., 2012). TLRs are pattern recognition receptors involved in the PF process by regulating inflammation and injury repair (Kim et al., 2011). Moreover, activation of Toll-like receptor signaling pathway promotes the overexpression of pro-inflammatory factors (Conti et al., 2020). And interaction between TLRs and viral particles is one of the reasons that causes death of COVID-19 patients (Patra et al., 2021). HIF-1, an important transcriptional factor in response to hypoxia, plays an important role in mammalian oxygen homeostasis and is involved in PF progress (Epstein et al., 2001; Xiong and Liu, 2017). Selective silence of HIF-1α in alveolar epithelial cells can inhibit the progression of bleomycin-induced PF (Weng et al., 2014). Dysregulation of HIF exacerbates edema and inflammation in the lung tissues of patients with ALI, which is associated with glycolysis and mitochondrial respiration (Eckle et al., 2013). Other study also shows that the viral ORF3a protein increases the expression of HIF-1α, which in turn aggravates SARS-CoV-2 infection and inflammatory response (Tian et al., 2021). Besides, EGFR has dual pro-fibrotic and anti-fibrotic effect, and cancer patients treated with EGFR tyrosine kinase inhibitors-monoclonal antibody present an elevated incidence of interstitial lung disease (Osawa et al., 2015). However, a study suggests that gefitinib can inhibit the progression of mice models of bleomycin-induced PF (Ishii et al., 2006). Spontaneous PF is observed in transgenic mice with high expression of EGFR ligands (Korfhagen et al., 1994; Perugorria et al., 2008), and EGFR ligands silencing contribute to improving PF (Madtes et al., 1999). In a word, these studies show that abnormal EGFR expression promotes the development of PF. Moreover, EGFR inhibits IFN-I production (Lupberger et al., 2013) and significantly increases during ALI (Finigan et al., 2012), indicating that EGFR is a potential targeted pathway for treating COVID-19. ALI caused by cytokine storm is the characteristic of COVID-19, and only the combination of TNF-α (an important subtype of TNF signaling pathway) and IFN-γ can induce inflammatory cell death during SARS-CoV-2 infection (Karki et al., 2021). In addition, TNF-α significantly increases in mice models of bleomycin-induced PF (Hou, et al., 2018). PI3K/AKT kinase inhibitors are confirmed to inhibit the replication of MERS (Kindrachuk, et al., 2015), and the inhibition of PI3K/AKT signaling pathway contributes to alleviating PF (Fang et al., 2020). The above researches illustrate that COVID-19 and PF share the common targeting pathways, and IL-17, TNF, HIF-1, EGFR, PI3K/AKT and Toll-like receptor signaling pathways were the critical mechanisms of kaempferol against COVID-19/PF co-occurrence.
Conclusion
This study is the first to elucidate the effect of kaempferol against COVID-19/PF co-occurrence by bioinformatics and systems pharmacology tools. The underlying mechanisms of kaempferol against COVID-19/PF co-occurrence may be related to bind to EGFR, SRC, MAPK3, MAPK1, MAPK8, AKT1, RELA and PIK3CA. Kaempferol might regulate inflammation, oxidative stress, immunity, virus infection, cell growth process and metabolism through targeting EGFR, IL-17, TNF, HIF-1, PI3K/AKT and Toll-like receptor signaling pathways to perform anti-COVID-19/PF co-occurrence effect. These findings suggest the possibility that kaempferol is a candidate compound to treat COVID-19/PF co-occurrence, but clinical, in vivo and in vitro experiments are needed to carry out to verify the predicted effect of kaempferol on COVID-19/PF co-occurrence in the future. This study contributes to providing effective strategy for exploring therapeutic approach for COVID-19/PF co-occurrence.
Data Availability Statement
The original contributions presented in the study are included in the article/Supplementary Material, further inquiries can be directed to the corresponding authors.
Author Contributions
HL, X-FH, and X-HL participated in the guidance and revision of the entire article. YJ conducted the writing and data analysis, Y-ZX and K-NY performed the literature searches and data analysis. C-WP, X-YL, S-FZ, and H-TH conducted the data analysis and revision of the article.
Funding
This work was supported by Traditional Chinese Medicine Bureau of Guangdong Province (20211344), This work was also supported by Shenzhen Baoan District Science and Technology Bureau (2020JD555). National Natural Science Foundation of China (82004141), Bao’an Traditional Chinese Medicine Development Foundation (2020KJCX-KTYJ-5), and Science, Technology, and Innovation Commission of Shenzhen Municipality (JCYJ20190808160407500). This work was supported by the Sanming Project of Medicine in Shenzhen (Grant No. SZZYSM202106006). This work was supported by the Science and Technology Program of Guangzhou, China (Grant no. 201904010235), and the National Natural Science Foundation of Guangdong, China (Grant no. 2020A1515010589 and Grant no. 2021A1515010146). This work was also supported by the “Double First-Class” and High-level University Discipline Collaborative Innovation Team Project of Guangzhou University of Chinese Medicine (Grant no. 2021XK16), Guangdong Provincial Department of Education Innovation Team Project (Grant no. 2018KCXTD007), the Key-Area Research and Development Program of Guangdong Province (Grant no. 2020B1111100002), the National Natural Science Foundation of China (Grant nos. 81973814 and 81904132), and the Technology Research of COVID-19 Treatment and Prevention and Special Project of Traditional Chinese Medicine Application-Research on the platform construction for the prevention and treatment of viral infectious diseases with traditional Chinese medicine (Grant no. 2020KJCX-KTYJ-130).
Conflict of Interest
The authors declare that the research was conducted in the absence of any commercial or financial relationships that could be construed as a potential conflict of interest.
Publisher’s Note
All claims expressed in this article are solely those of the authors and do not necessarily represent those of their affiliated organizations, or those of the publisher, the editors and the reviewers. Any product that may be evaluated in this article, or claim that may be made by its manufacturer, is not guaranteed or endorsed by the publisher.
Acknowledgments
We thank Lingnan Medical Research Center of Guangzhou University of Chinese Medicine and the Famous Traditional Chinese Medicine inheritance physician unit of X-HL of Guangdong for their support. We thank Professor Ling Jun Wang for his support in carrying out this work. We thank X-HL for her kind help in the initiation of this research and her selfless assistance.
References
Acat, M., Yildiz Gulhan, P., Oner, S., and Turan, M. K. (2021). Comparison of Pirfenidone and Corticosteroid Treatments at the COVID‐19 Pneumonia with the Guide of Artificial Intelligence Supported Thoracic Computed Tomography. Int. J. Clin. Pract. 75, e14961. doi:10.1111/ijcp.14961
Amberger, J. S., Bocchini, C. A., Schiettecatte, F., Scott, A. F., and Hamosh, A. (2015). OMIM.org: Online Mendelian Inheritance in Man (OMIM®), an Online Catalog of Human Genes and Genetic Disorders. Nucleic Acids Res. 43 (Database issue), D789–D798. doi:10.1093/nar/gku1205
An, X., Zhang, Y., Duan, L., JinZhao, S., Zhao, S., Zhou, R., et al. (2021). The Direct Evidence and Mechanism of Traditional Chinese Medicine Treatment of COVID-19. Biomed. Pharmacother. 137, 111267. doi:10.1016/j.biopha.2021.111267
Andersson-Sjöland, A., de Alba, C. G., Nihlberg, K., Becerril, C., Ramírez, R., Pardo, A., et al. (2008). Fibrocytes Are a Potential Source of Lung Fibroblasts in Idiopathic Pulmonary Fibrosis. Int. J. Biochem. Cell. Biol. 40 (10), 2129–2140. doi:10.1016/j.biocel.2008.02.012
Antoniades, H. N., Bravo, M. A., Avila, R. E., Galanopoulos, T., Neville-Golden, J., Maxwell, M., et al. (1990). Platelet-derived Growth Factor in Idiopathic Pulmonary Fibrosis. J. Clin. Investig. 86 (4), 1055–1064. doi:10.1172/JCI114808
Antoniou, K. M., Margaritopoulos, G. A., Soufla, G., Symvoulakis, E., Vassalou, E., Lymbouridou, R., et al. (2010). Expression Analysis of Akt and MAPK Signaling Pathways in Lung Tissue of Patients with Idiopathic Pulmonary Fibrosis (IPF). J. Recept Signal Transduct. Res. 30 (4), 262–269. doi:10.3109/10799893.2010.489227
Bader, G. D., and Hogue, C. W. (2003). An Automated Method for Finding Molecular Complexes in Large Protein Interaction Networks. BMC Bioinforma. 4, 2. doi:10.1186/1471-2105-4-2
Bahri, S., Ben Ali, R., Abidi, A., and Jameleddine, S. (2017). The Efficacy of Plant Extract and Bioactive Compounds Approaches in the Treatment of Pulmonary Fibrosis: A Systematic Review. Biomed. Pharmacother. 93, 666–673. doi:10.1016/j.biopha.2017.06.052
Bellaye, P. S., Burgy, O., Causse, S., Garrido, C., and Bonniaud, P. (2014). Heat Shock Proteins in Fibrosis and Wound Healing: Good or Evil? Pharmacol. Ther. 143 (2), 119–132. doi:10.1016/j.pharmthera.2014.02.009
Bellaye, P. S., Shimbori, C., Yanagihara, T., Carlson, D. A., Hughes, P., Upagupta, C., et al. (2018). Synergistic Role of HSP90α and HSP90β to Promote Myofibroblast Persistence in Lung Fibrosis. Eur. Respir. J. 51 (2). doi:10.1183/13993003.00386-2017
Bian, J., Wang, K., Kong, X., Liu, H., Chen, F., Hu, M., et al. (2011). Caspase- and P38-MAPK-dependent Induction of Apoptosis in A549 Lung Cancer Cells by Newcastle Disease Virus. Arch. Virol. 156 (8), 1335–1344. doi:10.1007/s00705-011-0987-y
Care, C., Sornjai, W., Jaratsittisin, J., Hitakarun, A., Wikan, N., Triwitayakorn, K., et al. (2020). Discordant Activity of Kaempferol towards Dengue Virus and Japanese Encephalitis Virus. Molecules 25 (5). doi:10.3390/molecules25051246
Celik, I., Yadav, R., Duzgun, Z., Albogami, S., El-Shehawi, A. M., FatimawaliEmran, F., et al. (2021). Interactions of the Receptor Binding Domain of SARS-CoV-2 Variants with hACE2: Insights from Molecular Docking Analysis and Molecular Dynamic Simulation. Biology 10 (9), 880. doi:10.3390/biology10090880
Chen, D. Q., Feng, Y. L., Cao, G., and Zhao, Y. Y. (2018). Natural Products as a Source for Antifibrosis Therapy. Trends Pharmacol. Sci. 39 (11), 937–952. doi:10.1016/j.tips.2018.09.002
Consortium, G. T. (2013). The Genotype-Tissue Expression (GTEx) Project. Nat. Genet. 45 (6), 580–585. doi:10.1038/ng.2653
Conti, P., Ronconi, G., Caraffa, A., Gallenga, C. E., Ross, R., Frydas, I., et al. (2020). Induction of Pro-inflammatory Cytokines (IL-1 and IL-6) and Lung Inflammation by Coronavirus-19 (COVI-19 or SARS-CoV-2): Anti-inflammatory Strategies. J. Biol. Regul. Homeost. Agents 34 (2), 327–331. doi:10.23812/CONTI-E
Crestani, B., Cornillet, P., Dehoux, M., Rolland, C., Guenounou, M., and Aubier, M. (1994). Alveolar Type II Epithelial Cells Produce Interleukin-6 In Vitro and In Vivo. Regulation by Alveolar Macrophage Secretory Products. J. Clin. Investig. 94 (2), 731–740. doi:10.1172/JCI117392
Daina, A., Michielin, O., and Zoete, V. (2019). SwissTargetPrediction: Updated Data and New Features for Efficient Prediction of Protein Targets of Small Molecules. Nucleic Acids Res. 47 (W1), W357–W364. doi:10.1093/nar/gkz382
Davis, A. P., Grondin, C. J., Johnson, R. J., Sciaky, D., Wiegers, J., Wiegers, T. C., et al. (2021). Comparative Toxicogenomics Database (CTD): Update 2021. Nucleic Acids Res. 49 (D1), D1138–D1143. doi:10.1093/nar/gkaa891
Delgado-Roche, L., and Mesta, F. (2020). Oxidative Stress as Key Player in Severe Acute Respiratory Syndrome Coronavirus (SARS-CoV) Infection. Arch. Med. Res. 51 (5), 384–387. doi:10.1016/j.arcmed.2020.04.019
Delorey, T. M., Ziegler, C. G. K., Heimberg, G., Normand, R., Yang, Y., Segerstolpe, Å., et al. (2021). COVID-19 Tissue Atlases Reveal SARS-CoV-2 Pathology and Cellular Targets. Nature 595 (7865), 107–113. doi:10.1038/s41586-021-03570-8
Devi, K. P., Malar, D. S., Nabavi, S. F., Sureda, A., Xiao, J., Nabavi, S. M., et al. (2015). Kaempferol and Inflammation: From Chemistry to Medicine. Pharmacol. Res. 99, 1–10. doi:10.1016/j.phrs.2015.05.002
Dong, H., Luo, L., Zou, M., Huang, C., Wan, X., Hu, Y., et al. (2017). Blockade of Extracellular Heat Shock Protein 90α by 1G6-D7 Attenuates Pulmonary Fibrosis through Inhibiting ERK Signaling. Am. J. Physiol. Lung Cell. Mol. Physiol. 313 (6), L1006–L1015. doi:10.1152/ajplung.00489.2016
Eckle, T., Brodsky, K., Bonney, M., Packard, T., Han, J., Borchers, C. H., et al. (2013). HIF1A Reduces Acute Lung Injury by Optimizing Carbohydrate Metabolism in the Alveolar Epithelium. PLoS Biol. 11 (9), e1001665. doi:10.1371/journal.pbio.1001665
Epstein, A. C., Gleadle, J. M., McNeill, L. A., Hewitson, K. S., O'Rourke, J., Mole, D. R., et al. (2001). C. elegans EGL-9 and Mammalian Homologs Define a Family of Dioxygenases that Regulate HIF by Prolyl Hydroxylation. Cell. 107 (1), 43–54. doi:10.1016/s0092-8674(01)00507-4
Esfandiarei, M., Luo, H., Yanagawa, B., Suarez, A., Dabiri, D., Zhang, J., et al. (2004). Protein Kinase B/Akt Regulates Coxsackievirus B3 Replication through a Mechanism Which Is Not Caspase Dependent. J. Virol. 78 (8), 4289–4298. doi:10.1128/jvi.78.8.4289-4298.2004
Fang, L., Chen, H., Kong, R., and Que, J. (2020). Endogenous Tryptophan Metabolite 5-Methoxytryptophan Inhibits Pulmonary Fibrosis by Downregulating the TGF-Β/smad3 and PI3K/AKT Signaling Pathway. Life Sci. 260, 118399. doi:10.1016/j.lfs.2020.118399
Finigan, J. H., Downey, G. P., and Kern, J. A. (2012). Human Epidermal Growth Factor Receptor Signaling in Acute Lung Injury. Am. J. Respir. Cell. Mol. Biol. 47 (4), 395–404. doi:10.1165/rcmb.2012-0100TR
Gaur, P., Munjhal, A., and Lal, S. K. (2011). Influenza Virus and Cell Signaling Pathways. Med. Sci. Monit. 17 (6), RA148–54. doi:10.12659/msm.881801
George, P. M., Wells, A. U., and Jenkins, R. G. (2020). Pulmonary Fibrosis and COVID-19: the Potential Role for Antifibrotic Therapy. Lancet Respir. Med. 8 (8), 807–815. doi:10.1016/s2213-2600(20)30225-3
Giacomelli, C., Piccarducci, R., Marchetti, L., Romei, C., and Martini, C. (2021). Pulmonary Fibrosis from Molecular Mechanisms to Therapeutic Interventions: Lessons from Post-COVID-19 Patients. Biochem. Pharmacol. 193, 114812. doi:10.1016/j.bcp.2021.114812
Gong, J. H., Cho, I. H., Shin, D., Han, S. Y., Park, S. H., and Kang, Y. H. (2014). Inhibition of Airway Epithelial-To-Mesenchymal Transition and Fibrosis by Kaempferol in Endotoxin-Induced Epithelial Cells and Ovalbumin-Sensitized Mice. Lab. Investig. 94 (3), 297–308. doi:10.1038/labinvest.2013.137
Goodwin, A., and Jenkins, G. (2009). Role of Integrin-Mediated TGFbeta Activation in the Pathogenesis of Pulmonary Fibrosis. Biochem. Soc. Trans. 37 (Pt 4), 849–854. doi:10.1042/BST0370849
Hammond, J., Leister-Tebbe, H., Gardner, A., Abreu, P., Bao, W., Wisemandle, W., et al. (2022). Oral Nirmatrelvir for High-Risk, Nonhospitalized Adults with Covid-19. N. Engl. J. Med. 386, 1397–1408. doi:10.1056/NEJMoa2118542
Han, H., Cho, J. W., Lee, S., Yun, A., Kim, H., Bae, D., et al. (2018). TRRUST V2: an Expanded Reference Database of Human and Mouse Transcriptional Regulatory Interactions. Nucleic Acids Res. 46 (D1), D380–D386. doi:10.1093/nar/gkx1013
Hou, J., Ma, T., Cao, H., Chen, Y., Wang, C., Chen, X., et al. (2018). TNF-α-induced NF-Κb Activation Promotes Myofibroblast Differentiation of LR-MSCs and Exacerbates Bleomycin-Induced Pulmonary Fibrosis. J. Cell. Physiol. 233 (3), 2409–2419. doi:10.1002/jcp.26112
Huang, J. F., Chuang, Y. H., Dai, C. Y., Yu, M. L., Huang, C. F., Hsiao, P. J., et al. (2011). Hepatic Akt Expression Correlates with Advanced Fibrosis in Patients with Chronic Hepatitis C Infection. Hepatol. Res. 41 (5), 430–436. doi:10.1111/j.1872-034X.2011.00786.x
Imran, M., Salehi, B., Sharifi-Rad, J., Aslam Gondal, T., Saeed, F., Imran, A., et al. (2019). Kaempferol: A Key Emphasis to its Anticancer Potential. Molecules 24 (12). doi:10.3390/molecules24122277
Ishii, Y., Fujimoto, S., and Fukuda, T. (2006). Gefitinib Prevents Bleomycin-Induced Lung Fibrosis in Mice. Am. J. Respir. Crit. Care Med. 174 (5), 550–556. doi:10.1164/rccm.200509-1534OC
Jaffar, J., Glaspole, I., Symons, K., and Westall, G. (2021). Inhibition of NF-Κb by ACT001 Reduces Fibroblast Activity in Idiopathic Pulmonary Fibrosis. Biomed. Pharmacother. 138, 111471. doi:10.1016/j.biopha.2021.111471
Jahaj, E., Vassiliou, A. G., Keskinidou, C., Gallos, P., Vrettou, C. S., Tsipilis, S., et al. (2021). Evaluating the Role of the Interleukin-23/17 Axis in Critically Ill COVID-19 Patients. J. Pers. Med. 11 (9). doi:10.3390/jpm11090891
John, A. E., Joseph, C., Jenkins, G., and Tatler, A. L. (2021). COVID-19 and Pulmonary Fibrosis: A Potential Role for Lung Epithelial Cells and Fibroblasts. Immunol. Rev. 302 (1), 228–240. doi:10.1111/imr.12977
Karki, R., Sharma, B. R., Tuladhar, S., Williams, E. P., Zalduondo, L., Samir, P., et al. (2021). Synergism of TNF-α and IFN-γ Triggers Inflammatory Cell Death, Tissue Damage, and Mortality in SARS-CoV-2 Infection and Cytokine Shock Syndromes. Cell. 184 (1), 149–e17. doi:10.1016/j.cell.2020.11.025
Keiser, M. J., Roth, B. L., Armbruster, B. N., Ernsberger, P., Irwin, J. J., and Shoichet, B. K. (2007). Relating Protein Pharmacology by Ligand Chemistry. Nat. Biotechnol. 25 (2), 197–206. doi:10.1038/nbt1284
Khaerunnisa, S., Kurniawan, H., Awaluddin, R., Suhartati, S., and Soetjipto, S. (2020). Potential Inhibitor of COVID‐19 Main Protease (M Pro ) from Several Medicinal Plant Compounds by Molecular Docking Study Medicine & Pharmacology . doi:10.20944/preprints202003.0226.v1Potential Inhibitor of COVID-19 Main Protease (Mpro) from Several Medicinal Plant Compounds by Molecular Docking Study
Khan, A., Heng, W., Wang, Y., Qiu, J., Wei, X., Peng, S., et al. (2021). In Silico and In Vitro Evaluation of Kaempferol as a Potential Inhibitor of the SARS-CoV-2 Main Protease (3CLpro). Phytother. Res. 35 (6), 2841–2845. doi:10.1002/ptr.6998
Kim, H. S., Go, H., Akira, S., and Chung, D. H. (2011). TLR2-mediated Production of IL-27 and Chemokines by Respiratory Epithelial Cells Promotes Bleomycin-Induced Pulmonary Fibrosis in Mice. J. Immunol. 187 (8), 4007–4017. doi:10.4049/jimmunol.1101654
Kim, S., Chen, J., Cheng, T., Gindulyte, A., He, J., He, S., et al. (2021). PubChem in 2021: New Data Content and Improved Web Interfaces. Nucleic Acids Res. 49 (D1), D1388–D1395. doi:10.1093/nar/gkaa971
Kindrachuk, J., Ork, B., Hart, B. J., Mazur, S., Holbrook, M. R., Frieman, M. B., et al. (2015). Antiviral Potential of ERK/MAPK and PI3K/AKT/mTOR Signaling Modulation for Middle East Respiratory Syndrome Coronavirus Infection as Identified by Temporal Kinome Analysis. Antimicrob. Agents Chemother. 59 (2), 1088–1099. doi:10.1128/AAC.03659-14
Korfhagen, T. R., Swantz, R. J., Wert, S. E., McCarty, J. M., Kerlakian, C. B., Glasser, S. W., et al. (1994). Respiratory Epithelial Cell Expression of Human Transforming Growth Factor-Alpha Induces Lung Fibrosis in Transgenic Mice. J. Clin. Investig. 93 (4), 1691–1699. doi:10.1172/JCI117152
Lancaster, L., Crestani, B., Hernandez, P., Inoue, Y., Wachtlin, D., Loaiza, L., et al. (2019). Safety and Survival Data in Patients with Idiopathic Pulmonary Fibrosis Treated with Nintedanib: Pooled Data from Six Clinical Trials. BMJ Open Respir. Res. 6 (1), e000397. doi:10.1136/bmjresp-2018-000397
Le Cras, T. D., Korfhagen, T. R., Davidson, C., Schmidt, S., Fenchel, M., Ikegami, M., et al. (2010). Inhibition of PI3K by PX-866 Prevents Transforming Growth Factor-Alpha-Induced Pulmonary Fibrosis. Am. J. Pathol. 176 (2), 679–686. doi:10.2353/ajpath.2010.090123
Lechowicz, K., Drożdżal, S., Machaj, F., Rosik, J., Szostak, B., Zegan-Barańska, M., et al. (2020). COVID-19: The Potential Treatment of Pulmonary Fibrosis Associated with SARS-CoV-2 Infection. J. Clin. Med. 9 (6). doi:10.3390/jcm9061917
Li, C., Chu, H., Liu, X., Chiu, M. C., Zhao, X., Wang, D., et al. (2020). Human Coronavirus Dependency on Host Heat Shock Protein 90 Reveals an Antiviral Target. Emerg. Microbes Infect. 9 (1), 1–27. doi:10.1080/22221751.2020.1850183
Li, L., Wang, R., Hu, H., Chen, X., Yin, Z., Liang, X., et al. (2021). The Antiviral Activity of Kaempferol against Pseudorabies Virus in Mice. BMC Vet. Res. 17 (1), 247. doi:10.1186/s12917-021-02953-3
Liao, Q. J., Ye, L. B., Timani, K. A., Zeng, Y. C., She, Y. L., Ye, L., et al. (2005). Activation of NF-kappaB by the Full-Length Nucleocapsid Protein of the SARS Coronavirus. Acta Biochim. Biophys. Sin. (Shanghai) 37 (9), 607–612. doi:10.1111/j.1745-7270.2005.00082.x
Liu, G., Cooley, M. A., Jarnicki, A. G., Borghuis, T., Nair, P. M., Tjin, G., et al. (2019). Fibulin-1c Regulates Transforming Growth Factor-β Activation in Pulmonary Tissue Fibrosis. JCI Insight 5. doi:10.1172/jci.insight.124529
Liu, G., Cooley, M. A., Nair, P. M., Donovan, C., Hsu, A. C., Jarnicki, A. G., et al. (2017). Airway Remodelling and Inflammation in Asthma Are Dependent on the Extracellular Matrix Protein Fibulin-1c. J. Pathol. 243 (4), 510–523. doi:10.1002/path.4979
Liu, H., Yu, H., Cao, Z., Gu, J., Pei, L., Jia, M., et al. (2019). Kaempferol Modulates Autophagy and Alleviates Silica-Induced Pulmonary Fibrosis. DNA Cell. Biol. 38 (12), 1418–1426. doi:10.1089/dna.2019.4941
Lu, Y., Azad, N., Wang, L., Iyer, A. K., Castranova, V., Jiang, B. H., et al. (2010). Phosphatidylinositol-3-kinase/akt Regulates Bleomycin-Induced Fibroblast Proliferation and Collagen Production. Am. J. Respir. Cell. Mol. Biol. 42 (4), 432–441. doi:10.1165/rcmb.2009-0002OC
Lupberger, J., Duong, F. H., Fofana, I., Zona, L., Xiao, F., Thumann, C., et al. (2013). Epidermal Growth Factor Receptor Signaling Impairs the Antiviral Activity of Interferon-Alpha. Hepatology 58 (4), 1225–1235. doi:10.1002/hep.26404
Madtes, D. K., Elston, A. L., Hackman, R. C., Dunn, A. R., and Clark, J. G. (1999). Transforming Growth Factor-Alpha Deficiency Reduces Pulmonary Fibrosis in Transgenic Mice. Am. J. Respir. Cell. Mol. Biol. 20 (5), 924–934. doi:10.1165/ajrcmb.20.5.3526
Maheshwari, S., Miller, M. S., O'Meally, R., Cole, R. N., Amzel, L. M., and Gabelli, S. B. (2017). Kinetic and Structural Analyses Reveal Residues in Phosphoinositide 3-kinase α that Are Critical for Catalysis and Substrate Recognition. J. Biol. Chem. 292 (33), 13541–13550. doi:10.1074/jbc.M116.772426
Mahmud, S., Mita, M. A., Biswas, S., Paul, G. K., Promi, M. M., Afrose, S., et al. (2021). Molecular Docking and Dynamics Study to Explore Phytochemical Ligand Molecules against the Main Protease of SARS-CoV-2 from Extensive Phytochemical Datasets. Expert Rev. Clin. Pharmacol. 14 (10), 1305–1315. doi:10.1080/17512433.2021.1959318
Meyer, B., Chiaravalli, J., Gellenoncourt, S., Brownridge, P., Bryne, D. P., Daly, L. A., et al. (2021). Characterising Proteolysis during SARS-CoV-2 Infection Identifies Viral Cleavage Sites and Cellular Targets with Therapeutic Potential. Nat. Commun. 12 (1), 5553. doi:10.1038/s41467-021-25796-w
Mizutani, T., Fukushi, S., Saijo, M., Kurane, I., and Morikawa, S. (2004). Phosphorylation of P38 MAPK and its Downstream Targets in SARS Coronavirus-Infected Cells. Biochem. Biophys. Res. Commun. 319 (4), 1228–1234. doi:10.1016/j.bbrc.2004.05.107
Nagy, P. D., Wang, R. Y., Pogany, J., Hafren, A., and Makinen, K. (2011). Emerging Picture of Host Chaperone and Cyclophilin Roles in RNA Virus Replication. Virology 411 (2), 374–382. doi:10.1016/j.virol.2010.12.061
Noble, P. W., Albera, C., Bradford, W. Z., Costabel, U., Glassberg, M. K., Kardatzke, D., et al. (2011). Pirfenidone in Patients with Idiopathic Pulmonary Fibrosis (CAPACITY): Two Randomised Trials. Lancet 377 (9779), 1760–1769. doi:10.1016/S0140-6736(11)60405-4
Nuovo, G. J., Hagood, J. S., Magro, C. M., Chin, N., Kapil, R., Davis, L., et al. (2012). The Distribution of Immunomodulatory Cells in the Lungs of Patients with Idiopathic Pulmonary Fibrosis. Mod. Pathol. 25 (3), 416–433. doi:10.1038/modpathol.2011.166
Olagnier, D., Farahani, E., Thyrsted, J., Blay-Cadanet, J., Herengt, A., Idorn, M., et al. (2020). SARS-CoV2-mediated Suppression of NRF2-Signaling Reveals Potent Antiviral and Anti-inflammatory Activity of 4-Octyl-Itaconate and Dimethyl Fumarate. Nat. Commun. 11 (1), 4938. doi:10.1038/s41467-020-18764-3
Osawa, M., Kudoh, S., Sakai, F., Endo, M., Hamaguchi, T., Ogino, Y., et al. (2015). Clinical Features and Risk Factors of Panitumumab-Induced Interstitial Lung Disease: a Postmarketing All-Case Surveillance Study. Int. J. Clin. Oncol. 20 (6), 1063–1071. doi:10.1007/s10147-015-0834-3
Otasek, D., Morris, J. H., Bouças, J., Pico, A. R., and Demchak, B. (2019). Cytoscape Automation: Empowering Workflow-Based Network Analysis. Genome Biol. 20 (1), 185. doi:10.1186/s13059-019-1758-4
Otoupalova, E., Smith, S., Cheng, G., and Thannickal, V. J. (2020). Oxidative Stress in Pulmonary Fibrosis. Compr. Physiol. 10 (2), 509–547. doi:10.1002/cphy.c190017
Pan, L. H., Yamauchi, K., Uzuki, M., Nakanishi, T., Takigawa, M., Inoue, H., et al. (2001). Type II Alveolar Epithelial Cells and Interstitial Fibroblasts Express Connective Tissue Growth Factor in IPF. Eur. Respir. J. 17 (6), 1220–1227. doi:10.1183/09031936.01.00074101
Pan, Y., Guan, H., Zhou, S., Wang, Y., Li, Q., Zhu, T., et al. (2020). Initial CT Findings and Temporal Changes in Patients with the Novel Coronavirus Pneumonia (2019-nCoV): a Study of 63 Patients in Wuhan, China. Eur. Radiol. 30 (6), 3306–3309. doi:10.1007/s00330-020-06731-x
Patra, R., Chandra Das, N., and Mukherjee, S. (2021). Targeting Human TLRs to Combat COVID-19: A Solution? J. Med. Virol. 93 (2), 615–617. doi:10.1002/jmv.26387
Perugorria, M. J., Latasa, M. U., Nicou, A., Cartagena-Lirola, H., Castillo, J., Goñi, S., et al. (2008). The Epidermal Growth Factor Receptor Ligand Amphiregulin Participates in the Development of Mouse Liver Fibrosis. Hepatology 48 (4), 1251–1261. doi:10.1002/hep.22437
Piñero, J., Bravo, À., Queralt-Rosinach, N., Gutiérrez-Sacristán, A., Deu-Pons, J., Centeno, E., et al. (2017). DisGeNET: a Comprehensive Platform Integrating Information on Human Disease-Associated Genes and Variants. Nucleic Acids Res. 45 (D1), D833–D839. doi:10.1093/nar/gkw943
Rameshwar, P., Narayanan, R., Qian, J., Denny, T. N., Colon, C., and Gascon, P. (2000). NF-kappa B as a Central Mediator in the Induction of TGF-Beta in Monocytes from Patients with Idiopathic Myelofibrosis: an Inflammatory Response beyond the Realm of Homeostasis. J. Immunol. 165 (4), 2271–2277. doi:10.4049/jimmunol.165.4.2271
Rebhan, M., Chalifa-Caspi, V., Prilusky, J., and Lancet, D. (1997). GeneCards: Integrating Information about Genes, Proteins and Diseases. Trends Genet. 13 (4), 163. doi:10.1016/s0168-9525(97)01103-7
Ren, J., Lu, Y., Qian, Y., Chen, B., Wu, T., and Ji, G. (2019). Recent Progress Regarding Kaempferol for the Treatment of Various Diseases. Exp. Ther. Med. 18 (4), 2759–2776. doi:10.3892/etm.2019.7886
Roschewski, M., Lionakis, M. S., Sharman, J. P., Roswarski, J., Goy, A., Monticelli, M. A., et al. (2020). Inhibition of Bruton Tyrosine Kinase in Patients with Severe COVID-19. Sci. Immunol. 5 (48). doi:10.1126/sciimmunol.abd0110
Rose, Y., Duarte, J. M., Lowe, R., Segura, J., Bi, C., Bhikadiya, C., et al. (2021). RCSB Protein Data Bank: Architectural Advances towards Integrated Searching and Efficient Access to Macromolecular Structure Data from the PDB Archive. J. Mol. Biol. 433 (11), 166704. doi:10.1016/j.jmb.2020.11.003
Ru, J., Li, P., Wang, J., Zhou, W., Li, B., Huang, C., et al. (2014). TCMSP: a Database of Systems Pharmacology for Drug Discovery from Herbal Medicines. J. Cheminform 6, 13. doi:10.1186/1758-2946-6-13
Schaller, T., Hirschbühl, K., Burkhardt, K., Braun, G., Trepel, M., Märkl, B., et al. (2020). Postmortem Examination of Patients with COVID-19. JAMA 323 (24), 2518–2520. doi:10.1001/jama.2020.8907
Shaldam, M. A., Yahya, G., Mohamed, N. H., Abdel-Daim, M. M., and Al Naggar, Y. (2021). In Silico screening of Potent Bioactive Compounds from Honeybee Products against COVID-19 Target Enzymes. Environ. Sci. Pollut. Res. Int. 28 (30), 40507–40514. doi:10.1007/s11356-021-14195-9
Shen, L., Liu, F., Huang, L., Liu, G., Zhou, L., and Peng, L. (2021). VDA-RWLRLS: An Anti-SARS-CoV-2 Drug Prioritizing Framework Combining an Unbalanced Bi-random Walk and Laplacian Regularized Least Squares. Comput. Biol. Med. 140, 105119. doi:10.1016/j.compbiomed.2021.105119
Sibinska, Z., Tian, X., Korfei, M., Kojonazarov, B., Kolb, J. S., Klepetko, W., et al. (2017). Amplified Canonical Transforming Growth Factor-β Signalling via Heat Shock Protein 90 in Pulmonary Fibrosis. Eur. Respir. J. 49 (2). doi:10.1183/13993003.01941-2015
Sisson, T. H., Mendez, M., Choi, K., Subbotina, N., Courey, A., Cunningham, A., et al. (2010). Targeted Injury of Type II Alveolar Epithelial Cells Induces Pulmonary Fibrosis. Am. J. Respir. Crit. Care Med. 181 (3), 254–263. doi:10.1164/rccm.200810-1615OC
Soleimanpour, S., and Yaghoubi, A. (2021). COVID-19 Vaccine: where Are We Now and where Should We Go? Expert Rev. Vaccines 20 (1), 23–44. doi:10.1080/14760584.2021.1875824
Song, P., Li, W., Xie, J., Hou, Y., and You, C. (2020). Cytokine Storm Induced by SARS-CoV-2. Clin. Chim. Acta 509, 280–287. doi:10.1016/j.cca.2020.06.017
Sontake, V., Wang, Y., Kasam, R. K., Sinner, D., Reddy, G. B., Naren, A. P., et al. (2017). Hsp90 Regulation of Fibroblast Activation in Pulmonary Fibrosis. JCI Insight 2 (4), e91454. doi:10.1172/jci.insight.91454
Szklarczyk, D., Gable, A. L., Nastou, K. C., Lyon, D., Kirsch, R., Pyysalo, S., et al. (2021). The STRING Database in 2021: Customizable Protein-Protein Networks, and Functional Characterization of User-Uploaded Gene/measurement Sets. Nucleic Acids Res. 49 (D1), D605–D612. doi:10.1093/nar/gkaa1074
Tang, Y., Li, M., Wang, J., Pan, Y., and Wu, F. X. (2015). CytoNCA: a Cytoscape Plugin for Centrality Analysis and Evaluation of Protein Interaction Networks. Biosystems 127, 67–72. doi:10.1016/j.biosystems.2014.11.005
Tatler, A. L., and Jenkins, G. (2012). TGF-β Activation and Lung Fibrosis. Proc. Am. Thorac. Soc. 9 (3), 130–136. doi:10.1513/pats.201201-003AW
Thannickal, V. J., Toews, G. B., White, E. S., Lynch, J. P., and Martinez, F. J. (2004). Mechanisms of Pulmonary Fibrosis. Annu. Rev. Med. 55, 395–417. doi:10.1146/annurev.med.55.091902.103810
Tian, M., Liu, W., Li, X., Zhao, P., Shereen, M. A., Zhu, C., et al. (2021). HIF-1α Promotes SARS-CoV-2 Infection and Aggravates Inflammatory Responses to COVID-19. Signal Transduct. Target Ther. 6 (1), 308. doi:10.1038/s41392-021-00726-w
Trott, O., and Olson, A. J. (2010). AutoDock Vina: Improving the Speed and Accuracy of Docking with a New Scoring Function, Efficient Optimization, and Multithreading. J. Comput. Chem. 31 (2), 455–461. doi:10.1002/jcc.21334
UniProt, C. (2015). UniProt: a Hub for Protein Information. Nucleic Acids Res. 43, D204–D212. doi:10.1093/nar/gku989
Vagapova, E. R., Lebedev, T. D., and Prassolov, V. S. (2021). Viral Fibrotic Scoring and Drug Screen Based on MAPK Activity Uncovers EGFR as a Key Regulator of COVID-19 Fibrosis. Sci. Rep. 11 (1), 11234. doi:10.1038/s41598-021-90701-w
Vittal, R., Horowitz, J. C., Moore, B. B., Zhang, H., Martinez, F. J., Toews, G. B., et al. (2005). Modulation of Prosurvival Signaling in Fibroblasts by a Protein Kinase Inhibitor Protects against Fibrotic Tissue Injury. Am. J. Pathol. 166 (2), 367–375. doi:10.1016/S0002-9440(10)62260-2
Wang, B., Li, R., Lu, Z., and Huang, Y. (2020). Does Comorbidity Increase the Risk of Patients with COVID-19: Evidence from Meta-Analysis. Aging (Albany NY) 12 (7), 6049–6057. doi:10.18632/aging.103000
Wang, W., Ye, L., Ye, L., Li, B., Gao, B., Zeng, Y., et al. (2007). Up-regulation of IL-6 and TNF-Alpha Induced by SARS-Coronavirus Spike Protein in Murine Macrophages via NF-kappaB Pathway. Virus Res. 128 (1-2), 1–8. doi:10.1016/j.virusres.2007.02.007
Wang, Y., Zhang, S., Li, F., Zhou, Y., Zhang, Y., Wang, Z., et al. (2020). Therapeutic Target Database 2020: Enriched Resource for Facilitating Research and Early Development of Targeted Therapeutics. Nucleic Acids Res. 48 (D1), D1031–D1041. doi:10.1093/nar/gkz981
Weng, T., Poth, J. M., Karmouty-Quintana, H., Garcia-Morales, L. J., Melicoff, E., Luo, F., et al. (2014). Hypoxia-induced Deoxycytidine Kinase Contributes to Epithelial Proliferation in Pulmonary Fibrosis. Am. J. Respir. Crit. Care Med. 190 (12), 1402–1412. doi:10.1164/rccm.201404-0744OC
Wishart, D. S., Feunang, Y. D., Guo, A. C., Lo, E. J., Marcu, A., Grant, J. R., et al. (2018). DrugBank 5.0: a Major Update to the DrugBank Database for 2018. Nucleic Acids Res. 46 (D1), D1074–D1082. doi:10.1093/nar/gkx1037
World Health Organization (2022). WHO Coronavirus (COVID-19) Dashboard. from Avaible at: https://covid19.who.int/.
Wu, D., and Yang, X. O. (2020). TH17 Responses in Cytokine Storm of COVID-19: An Emerging Target of JAK2 Inhibitor Fedratinib. J. Microbiol. Immunol. Infect. 53 (3), 368–370. doi:10.1016/j.jmii.2020.03.005
Wu, T., Hu, E., Xu, S., Chen, M., Guo, P., Dai, Z., et al. (2021). clusterProfiler 4.0: A Universal Enrichment Tool for Interpreting Omics Data. Innovation 2 (3), 100141. doi:10.1016/j.xinn.2021.100141
Wu, Y., Zhang, F., Yang, K., Fang, S., Bu, D., Li, H., et al. (2019). SymMap: an Integrative Database of Traditional Chinese Medicine Enhanced by Symptom Mapping. Nucleic Acids Res. 47 (D1), D1110–D1117. doi:10.1093/nar/gky1021
Wu, Z., and McGoogan, J. M. (2020). Characteristics of and Important Lessons from the Coronavirus Disease 2019 (COVID-19) Outbreak in China: Summary of a Report of 72 314 Cases from the Chinese Center for Disease Control and Prevention. JAMA 323 (13), 1239–1242. doi:10.1001/jama.2020.2648
Xia, H., Diebold, D., Nho, R., Perlman, D., Kleidon, J., Kahm, J., et al. (2008). Pathological Integrin Signaling Enhances Proliferation of Primary Lung Fibroblasts from Patients with Idiopathic Pulmonary Fibrosis. J. Exp. Med. 205 (7), 1659–1672. doi:10.1084/jem.20080001
Xiong, A., and Liu, Y. (2017). Targeting Hypoxia Inducible Factors-1α as a Novel Therapy in Fibrosis. Front. Pharmacol. 8, 326. doi:10.3389/fphar.2017.00326
Xu, J., Xu, X., Jiang, L., Dua, K., Hansbro, P. M., and Liu, G. (2020). SARS-CoV-2 Induces Transcriptional Signatures in Human Lung Epithelial Cells that Promote Lung Fibrosis. Respir. Res. 21 (1), 182. doi:10.1186/s12931-020-01445-6
Xu, X. W., Wu, X. X., Jiang, X. G., Xu, K. J., Ying, L. J., Ma, C. L., et al. (2020). Clinical Findings in a Group of Patients Infected with the 2019 Novel Coronavirus (SARS-Cov-2) outside of Wuhan, China: Retrospective Case Series. BMJ 368, m606. doi:10.1136/bmj.m606
Xu, Z., Shi, L., Wang, Y., Zhang, J., Huang, L., Zhang, C., et al. (2020). Pathological Findings of COVID-19 Associated with Acute Respiratory Distress Syndrome. Lancet Respir. Med. 8 (4), 420–422. doi:10.1016/S2213-2600(20)30076-X
Yao, Z. J., Dong, J., Che, Y. J., Zhu, M. F., Wen, M., Wang, N. N., et al. (2016). TargetNet: a Web Service for Predicting Potential Drug-Target Interaction Profiling via Multi-Target SAR Models. J. Comput. Aided Mol. Des. 30 (5), 413–424. doi:10.1007/s10822-016-9915-2
Yin, X., Riva, L., Pu, Y., Martin-Sancho, L., Kanamune, J., Yamamoto, Y., et al. (2021). MDA5 Governs the Innate Immune Response to SARS-CoV-2 in Lung Epithelial Cells. Cell. Rep. 34 (2), 108628. doi:10.1016/j.celrep.2020.108628
Yu, W., Clyne, M., Khoury, M. J., and Gwinn, M. (2010). Phenopedia and Genopedia: Disease-Centered and Gene-Centered Views of the Evolving Knowledge of Human Genetic Associations. Bioinformatics 26 (1), 145–146. doi:10.1093/bioinformatics/btp618
Zhang, R., Ai, X., Duan, Y., Xue, M., He, W., Wang, C., et al. (2017). Kaempferol Ameliorates H9N2 Swine Influenza Virus-Induced Acute Lung Injury by Inactivation of TLR4/MyD88-Mediated NF-Κb and MAPK Signaling Pathways. Biomed. Pharmacother. 89, 660–672. doi:10.1016/j.biopha.2017.02.081
Zhang, X., Gao, R., Zhou, Z., Tang, X., Lin, J., Wang, L., et al. (2021). A Network Pharmacology Based Approach for Predicting Active Ingredients and Potential Mechanism of Lianhuaqingwen Capsule in Treating COVID-19. Int. J. Med. Sci. 18 (8), 1866–1876. doi:10.7150/ijms.53685
Zhou, S., Wang, Y., Zhu, T., and Xia, L. (2020). CT Features of Coronavirus Disease 2019 (COVID-19) Pneumonia in 62 Patients in Wuhan, China. AJR Am. J. Roentgenol. 214 (6), 1287–1294. doi:10.2214/AJR.20.22975
Zhou, Y., Zhou, B., Pache, L., Chang, M., Khodabakhshi, A. H., Tanaseichuk, O., et al. (2019). Metascape Provides a Biologist-Oriented Resource for the Analysis of Systems-Level Datasets. Nat. Commun. 10 (1), 1523. doi:10.1038/s41467-019-09234-6
Zhu, N., Zhang, D., Wang, W., Li, X., Yang, B., Song, J., et al. (2020). A Novel Coronavirus from Patients with Pneumonia in China, 2019. N. Engl. J. Med. 382 (8), 727–733. doi:10.1056/NEJMoa2001017
Ziegler, C. G. K., Allon, S. J., Nyquist, S. K., Mbano, I. M., Miao, V. N., Tzouanas, C. N., et al. (2020). SARS-CoV-2 Receptor ACE2 Is an Interferon-Stimulated Gene in Human Airway Epithelial Cells and Is Detected in Specific Cell Subsets across Tissues. Cell. 181 (5), 1016–e19. doi:10.1016/j.cell.2020.04.035
Keywords: kaempferol, pulmonary fibrosis, COVID-19, co-occurrence, bioinformatic analysis, system pharmacology
Citation: Jiang Y, Xie Y-Z, Peng C-W, Yao K-N, Lin X-Y, Zhan S-F, Zhuang H-F, Huang H-T, Liu X-H, Huang X-F and Li H (2022) Modeling Kaempferol as a Potential Pharmacological Agent for COVID-19/PF Co-Occurrence Based on Bioinformatics and System Pharmacological Tools. Front. Pharmacol. 13:865097. doi: 10.3389/fphar.2022.865097
Received: 29 January 2022; Accepted: 25 April 2022;
Published: 08 June 2022.
Edited by:
Joan Villena García, Universidad de Valparaíso, ChileReviewed by:
Lihong Peng, Hunan University of Technology, ChinaTalha Bin Emran, Begum Gulchemonara Trust University, Bangladesh
Copyright © 2022 Jiang, Xie, Peng, Yao, Lin, Zhan, Zhuang, Huang, Liu, Huang and Li. This is an open-access article distributed under the terms of the Creative Commons Attribution License (CC BY). The use, distribution or reproduction in other forums is permitted, provided the original author(s) and the copyright owner(s) are credited and that the original publication in this journal is cited, in accordance with accepted academic practice. No use, distribution or reproduction is permitted which does not comply with these terms.
*Correspondence: Xiao-Hong Liu, rsclxh@gzucm.edu.cn; Xiu-Fang Huang, 879172531@qq.com; Hang Li, drlihang@foxmail.com
† These authors have contributed equally to this work and share first authorship