- 1Department of Nuclear Medicine, Xijing Hospital, Fourth Military Medical University, Xi’an, China
- 2Department of Respiratory Medicine, Xijing Hospital, Fourth Military Medical University, Xi’an, China
- 3School of Information Science and Technology, Northwest University, Xi’an, China
Purpose: To assess the significance of mutation mutual exclusion information in the optimization of radiomics algorithms for predicting gene mutation.
Methods: We retrospectively analyzed 258 non-small cell lung cancer (NSCLC) patients. Patients were randomly divided into training (n = 180) and validation (n = 78) cohorts. Based on radiomics features, radiomics score (RS) models were developed for predicting KRAS proto-oncogene mutations. Furthermore, a composite model combining mixedRS and epidermal growth factor receptor (EGFR) mutation status was developed.
Results: Compared with CT model, the PET/CT radiomics score model exhibited higher AUC for predicting KRAS mutations (0.834 vs. 0.770). By integrating EGFR mutation information into the PET/CT RS model, the AUC, sensitivity, specificity, and accuracy for predicting KRAS mutations were all elevated in the validation cohort (0.921, 0.949, 0.872, 0.910 vs. 0.834, 0.923, 0.641, 0.782). By adding EGFR exclusive mutation information, the composite model corrected 64.3% false positive cases produced by the PET/CT RS model in the validation cohort.
Conclusion: Integrating EGFR mutation status has potential utility for the optimization of radiomics models for prediction of KRAS gene mutations. This method may be used when repeated biopsies would carry unacceptable risks for the patient.
Introduction
Lung cancer is the leading cause of cancer death globally in 2022 (Sung et al., 2021; Siegel et al., 2022). Non-small cell lung cancer (NSCLC) accounts for about 85% of total lung cancer cases (Molina et al., 2008). Tyrosine kinase inhibitors (TKI) have clinical utility as the standard first-line therapy drugs for NSCLC patients with mutations in genes encoding epidermal growth factor receptor (EGFR), anaplastic lymphoma kinase (ALK) or ROS proto-oncogene 1 (ROS1) (Drusbosky et al., 2021; Nagasaka et al., 2021; Popat et al., 2021). Therefore, determination of genetic status is a prerequisite for targeting therapy and avoiding treatments with little clinical benefit (NCCN, 2022).
In NSCLC, over 80% of total gene mutations are accounted for by EGFR (50%) and KRAS proto-oncogene (30%) (Hirsch and Bunn, 2009; Skoulidis et al., 2021). EGFR-targeted TKIs (EGFR-TKI), such as gefitinib, afatinib and osimertinib have been widely used in the clinic. More recently, KRAS mutation-targeting drugs, such as sotorasib (AMG510) and Adagrasib (MRTX849), have been shown to elicit a 37.1–45% overall response rate in clinical trials (Palma et al., 2021). International guidelines have recommended testing for the conventional mutations, EGFR, B-Raf, ALK and ROS1(Kerr et al., 2021), in advanced NSCLC with KRAS only being included in June 2021 (NCCN NSCLC guidelines). Current ASCO, ESMO and CSCO guidelines do not include KRAS in the list of testing recommendations. Whereas some patients may have been tested for KRAS mutations to predict responsiveness to EGFR-TKI therapy, many will only receive mutation testing according to ESMO and ASCO guidelines. For the latter group, knowledge of the KRAS mutation status is particularly important to inform the choice of newly developed KRAS mutation-targeting agents. Repeated biopsies are invasive and next-generation sequencing (NGS) is expensive (Santos et al., 2019; Kerr et al., 2021; Mantilla et al., 2021). The non-invasive approach of radiomics may provide a solution to prediction of KRAS mutation status.
With recent advances in artificial intelligence, radiomics has been widely used in the prediction of gene mutations (Mu et al., 2020; Zhang et al., 2020), and the performance of these radiomics models has been subject to continuous optimization (Wu et al., 2021). To improve the accuracy in predicting gene mutations, previous studies integrated various clinical information, including smoking history, radiographic features, and serum tumor markers into radiomics models (Zhang et al., 2020; Chang et al., 2021; Ren et al., 2021; Weng et al., 2021). However, because of the weak theoretical connection between the above clinical information and genetic mutations, a better optimization method may be potentially achieved by integrating more directly relevant genetic information.
Previous work has shown the mutual exclusivity of multiple gene mutations, including EGFR and KRAS, a phenomenon referred to as gene mutation mutual exclusion (Shigematsu and Gazdar, 2006; Kim et al., 2015; Mok et al., 2016). Therefore, in theory, EGFR mutation status should be related to KRAS mutation status, an observation which could be exploited in optimizing the accuracy of KRAS radiomics models. The current study aims to verify whether knowledge of EGFR mutation status could be used to improve the accuracy of the radiomics model for predicting KRAS mutation status based on 18F-FDG PET/CT multimodality imaging data.
Materials and Methods
Patients
We retrospectively analyzed the PET/CT images of NSCLC patients diagnosed pathologically in Xijing Hospital from 2016 to 2020. A total of 258 NSCLC patients were screened, all of which received EGFR and KRAS genetic testing at the primary site of lung cancer. It should be noted that since KRAS is not a conventional recommended target for testing in ESMO, CAP/IASLC/AMP, and Pan-Asian guidelines, patients undergoing additional sequencing are rare in current clinical practice (Kerr et al., 2021). Inclusion criteria were: 1) confirmation of NSCLC by pathology or cytology; 2) having undergone 18F-FDG PET/CT imaging; 3) primary lesion diameter > 1cm; 4) no history of other malignant tumors. Exclusion criteria were: 1) having received radiotherapy or chemotherapy before PET/CT examination; 2) poor PET/CT image quality. This retrospective study was approved by the Medical Ethics Committee of Xijing Hospital (Approval No. KY20173008-1).
All cases were randomly assigned in a 7:3 ratio to the training cohort (n = 180) or validation cohort (n = 78). All cases in the training cohort were used to train the predictive model, while cases in the validation cohort were used to independently evaluate the model’s performance.
18F-FDG PET/CT Imaging
All patients received 18F-FDG PET/CT scans on the same equipment (Biograph 40, Siemens), following a standard clinical protocol (Delbeke et al., 2006). Briefly, patients were required to fast for >6 h before the scan and exhibit blood glucose control within 7.8 mmol/L. CT parameters were 100 kV, 110 mAs, 0.5 s rotation time, 3 mm slice thickness, 700 mm field of view, and 512 × 512 matrix. For PET scanning, 4.44–5.55 MBq/kg of 18F-FDG was injected. Scanning was initiated 60 min after tracer administration, with 3 min scans per bed position. PET and CT images were reconstructed using an ordered-subsets expectation-maximization algorithm with four iterations and eight subsets (Kang et al., 2016; Kang et al., 2019; Kang et al., 2020).
Tumor Segmentation, Feature Extraction, and Selection
All regions of interest (ROI) were defined for PET/CT images by two experienced nuclear medicine physicians using MITK (Medical Imaging Interaction Toolkit v2018.04.2) software, as described previously (Chang et al., 2021; Han et al., 2021; Wu et al., 2021; Zhou H. et al., 2021). ROIs identified by CT were manually outlined slice-by-slice by nuclear medicine physicians in the lung window (WW: 1500HU, WL: −500HU). ROIs identified from PET images were segmented by semi-automatic outlining using the “region growing 3D tools” in MITK software, referencing the 3D-ROI with a standard uptake value (SUV) threshold of 40%. For lesion boundaries close to the heart or chest wall, PET image ROIs were outlined manually with reference to the CT image.
SUVs of PET images were converted prior to feature extraction. Radiomics features (including first order features, texture features and shape features) were extracted using the Pyradiomics software package (Koyasu et al., 2020; Yu et al., 2021; Zhang et al., 2021; Zhou Y. et al., 2021). Nine image filters (wavelet, lbp2D, lbp3D, Laplacian of Gaussian, square root, square, gradient, logarithm, exponential) were used to analyze high-dimensional image features. Sigma parameters were 1.0, 2.0, 3.0, 4.0, 5.0 when using the Laplacian of Gaussian filter. A bin width of 25 (CT) and 0.1 (PET) voxel and an array shift of 1000 (CT) and 0 (PET) were used for feature extraction. All data were subjected to standardized data preprocessing.
Feature selection was performed using univariate and multivariate analyses with a stepwise selection method. To avoid overfitting of the model, Spearman analysis was used to determine the correlation between radiomics features and KRAS gene mutations. The threshold for Spearman correlation analysis was 0.3. Mann–Whitney U tests were performed to identify features with a statistical threshold of p < 0.05. Least absolute shrinkage and selection operator (LASSO) was used to select optimal features (Zhang et al., 2020).
Model Establishment, Comparison, and Evaluation
CT radiomics score (RS) and mixed PET/CT RS (mixedRS) were calculated for each patient based on the optimal feature subsets screened by LASSO. A CT RS model and PET/CT RS model were built by logistic regression. RS was then optimized by incorporating EGFR mutation information to develop the composite model by logistic regression. A nomogram was constructed based on the composite model. Calibration curves were plotted to evaluate the goodness of fit of the prediction model and the three models were compared in training and validation cohorts. Performance parameters included the AUC, accuracy, sensitivity, specificity, false positive rate (FPR), false negative rate (FNR) and Youden index (YI). AUCs of the three models were compared by Delong test. Decision curve analysis was performed to compare the clinical benefit of the three models and data balanced using the synthetic minority oversampling technique (SMOTE). SMOTE is a powerful over-sampling method that has shown a great deal of successes in class imbalanced problems (Fotouhi et al., 2019). The minority class is over-sampled by taking each sample and introducing synthetic examples along the line segments joining any/all of the nearest neighbors of the k minority class (Chawla et al., 2002).
Statistical Analysis
All statistical analyses were performed using R software version 3.5.1 and Python software version 3.5.6. T-tests and Mann–Whitney U tests were used to compare continuous variables, while Chi-squared tests were used to compare differences in categorical variables. A p-value <0.05 indicated statistical significance.
Results
Patient Characteristics
Clinical characteristics of the 258 patients in training and validation cohorts are shown in Table 1. Chi-square testing revealed that EGFR mutation was a significant predictor of KRAS mutation in both the training and the validation cohorts (both p < 0.001).
Feature Selection and RS Establishment
The scheme for establishment of the radiomics model is presented in Figure 1. A total of 4306 features were extracted in accordance with the image biomarker standardization initiative (IBSI) (Zwanenburg et al., 2017). After univariate analysis and LASSO screening, four features were included in the CT RS model and 12 features in the PET/CT RS model (4 CT plus 8 PET features; Figure 2; Supplementary Material). The RS was calculated using the Supplementary Formulae of RS. The CT RS and mixedRS was significantly different between patients with KRAS mutations and those with wild type KRAS in both the training and the validation cohorts (both p < 0.001). See Supplementary Table S1 for detailed information.
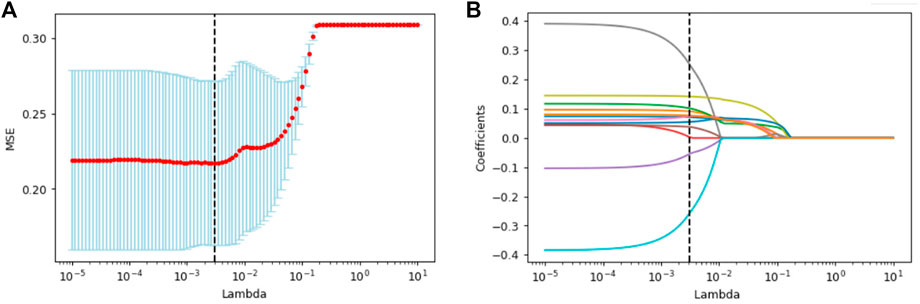
FIGURE 2. The LASSO and 10-fold cross-validation were used to select the optimal radiomics features. 12 features corresponding to the optimal lambda values were selected. (A) Mean square error path. (B) LASSO coefficient profiles of radiomics features.
Comparison of the Three Models and the Establishment of the Nomogram
In the validation cohort, the AUC, sensitivity, specificity, accuracy and YI of the PET/CT RS model were superior to the CT RS model (AUC: 0.834 vs. 0.770, sensitivity: 0.923 vs. 0.872, specificity: 0.641 vs. 0.615, accuracy: 0.782 vs. 0.744, YI: 0.564 vs. 0.487; Figure 3). Therefore, we established composite model combining mixedRS and EGFR mutation information. The composite model performed better than the PET/CT RS model in both cohorts of the study, with an AUC of 0.928 (95% CI [0.890, 0.965]) in the training cohort and 0.921 (95% CI [0.856, 0.986]) in the validation cohort. The Delong test revealed a significant difference between the AUC of the PET/CT RS model and the composite model in both the training and the validation cohorts (p < 0.001 and p = 0.012, respectively). Data used for comparison of the three models are shown in Table 2. A PET RS model was constructed in a similar manner (data shown in Table 2) for comparative purposes, although PET examination alone is rarely used in clinical practice.
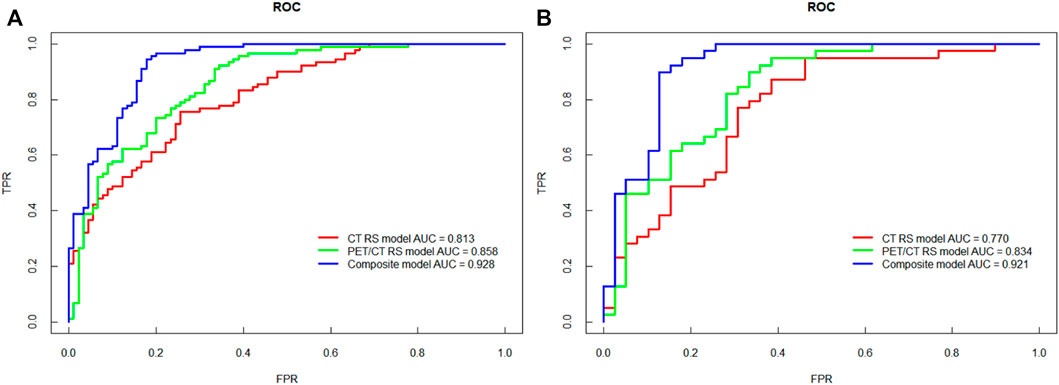
FIGURE 3. ROC curves of the three models for predicting KRAS mutations. (A) The ROC curve of the training cohort. (B) The ROC curve of the validation cohort.
The FPR of the composite model (12.8%) was significantly decreased compared to the PET/CT RS model (35.9%) in validation cohort, and no additional false negative error was generated. In the validation cohort, the composite model corrected 64.3% false positive errors generated by the PET/CT RS model. The nomogram that integrates mixedRS and EGFR was created for further clinical use in predicting KRAS mutation status (Figure 4A). The nomogram calibration curves are presented in Figures 4B,C, which indicated good consistency between the predicted and actual values.
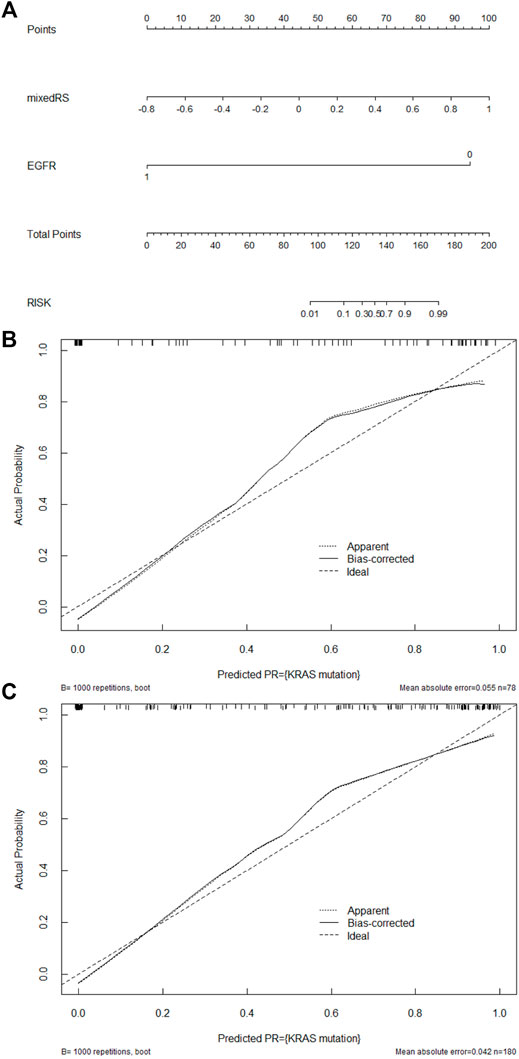
FIGURE 4. Development and performance of a nomogram. (A) Nomogram establishment by integrating mixedRS and EGFR. Nomogram calibration curves in the training (B) and validation (C) cohorts. The diagonal dashed line represents a predicted value equal to the true value, and the solid line is the model’s prediction of KRAS mutation. The closer the two lines are, the better the performance.
Decision Curve Analysis
To evaluate the clinical usefulness of the established radiomics models, decision curves were drawn (Figure 5). The composite model had higher net benefit than CT RS model and PET/CT RS model when the threshold probability reached <90%.
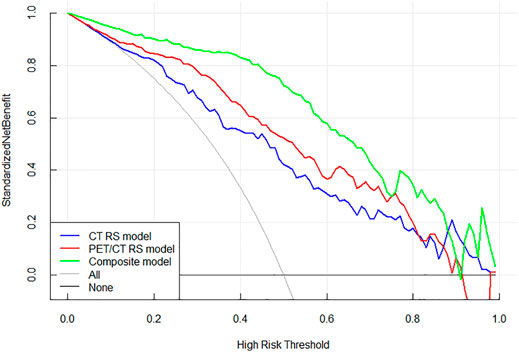
FIGURE 5. Decision curves of the three models. The green line represents the composite model incorporating mixedRS and EGFR. The blue and red lines represent CT RS model and PET/CT RS model, respectively. The grey line indicates the assumption that all patients possess the gene mutation, while the black line indicates the assumption that all patients possess the wild type gene.
Discussion
Two findings of the current study may be highlighted. Firstly, three radiomics prediction models for KRAS mutations were established: CT RS model, PET/CT RS model and composite model combining mixedRS and EGFR. Addition of PET information improved the accuracy of KRAS prediction. Secondly, EGFR information was found to be of benefit in establishing a radiomics model to predict KRAS mutation status, a finding that has clinical significance for patients who would otherwise have to suffer repeated biopsies. Relative to the PET/CT RS model, the composite mixedRS and EGFR gene status model showed improved accuracy and AUC for predicting KRAS mutations without a significant effect on sensitivity.
Analysis of the RS formulae developed during the current study revealed a link between radiomics features and gene mutations. CT_wavelet-HHL_firstorder_Skewness, associated with the asymmetry of value distribution about the mean, and CT_wavelet-LHH_gldm_DNUN, associated with the similarity of dependence throughout the image, were both positively correlated with KRAS mutations in both the CT RS model and the PET/CT RS model. Furthermore, PET_lbp-2D_gldm_GLV, associated with the variance in grey level, and PET_lbp-2D_firstorder_Variance, associated with the squared distances of each intensity value from the mean value, were both negatively correlated with KRAS mutations. Interpretation of the features of radiomics remains difficult which limits the clinical applications. More research is required to verify the theoretical link between radiomic features and clinical outcome.
Radiomics has been widely used in the prediction of genetic mutations since 2012 with much effort being expended in optimization of the approach. Zhang et al. combined clinical information, such as smoking and gender, with a radiomics model to improve the prediction of EGFR mutations in lung cancer compared with a radiomics model alone (Zhang et al., 2020). Although non-smoking women have been shown to be more likely to have EGFR mutations (Lynch et al., 2004; Dogan et al., 2012), there is still no direct correlation between this clinical information and gene mutation status.
Expert consensus, represented by the latest NCCN guidelines, affirms the existence of the mutually exclusive phenomenon among genetic mutations, especially between EGFR and KRAS genes (Marchetti et al., 2005; Amado et al., 2008; Roosan et al., 2021; NCCN, 2022). Although the mechanism is not fully understood, it may be that tumors with KRAS mutations have already activated further downstream effectors and removing the requirement for EGFR mutations (NCCN, 2022). Therefore, the mutually exclusive genetic phenomenon is more directly correlated to gene mutation status than other clinical information. The current study confirms that clinical information regarding EGFR can be used to optimize radiomics algorithms for prediction of KRAS gene mutations.
Hitherto, most patients have only been tested for mutations in a limited number of genes, such as EGFR. With the advent of KRAS-targeted drugs, including sotorasib, and RET-targeted, pralsetinib (Thein et al., 2021), version 5.2021 of the NCCN guidelines for NSCLC has been amended to indicate the need for KRAS genetic testing. Puncture biopsy is risky with potential outcomes such as pneumothorax, hemoptysis and even death. However, the current findings demonstrate a non-invasive method to predict KRAS status based on pre-existing results of mutation testing. This method not only has the potential to avoid secondary biopsies for patients at risk but also to guide patient selection for new KRAS-targeted drugs.
We acknowledge some limitations to the current study. Firstly, as a single center retrospective study, few KRAS mutation cases were included since KRAS was not routinely tested. This limitation could be overcome by further validation in multi-center studies with expanded sample sizes. Secondly, only EGFR and KRAS mutations were investigated with other mutations, such as ALK and ROS1, not included. The prevalence of ALK and ROS1 mutations in NSCLC patients is 2–7% and 1–2%, respectively, with the mutations only occurring in 1.2% of patients enrolled in the current study. More research is needed to confirm the value of ALK and ROS1 mutations in optimizing the radiomics model. Thirdly, the current results were obtained from imaging data and samples of primary lesions. Tumors are highly heterogeneous and mutation status may differ between the primary and metastatic lesions or even within a single lesion. Further studies are warranted to address more complex issues related to the heterogeneity of mutations. In conclusion, although radiomics features are difficult to interpret, the current approach may have utility as a complementary method in the clinic, particularly among patients for whom repeated biopsies carry unacceptable risks.
Data Availability Statement
The original contributions presented in the study are included in the article/Supplementary Material, further inquiries can be directed to the corresponding authors.
Ethics Statement
The studies involving human participants were reviewed and approved by the Ethics Committee of Xijing Hospital. The ethics committee waived the requirement of written informed consent for participation.
Author Contributions
JyW drafted the manuscript. JyW, XL, WH and SW collected the data. ZQ, GL, YW, ZX, YY, XL and WM participated in the design and coordination of the study. JyW and WH assisted with data statistics and interpretation. WY, XC, FK and JW conceived the presented idea. FK proofed the paper. All authors have read and approved the final version of the manuscript.
Funding
This study was supported by the National Natural Science Foundation of China (Grant Nos 91959208, 82122033, 81971646, 81871379) and the Natural Science Basic Research Program of Shaanxi Province (No. 2021JQ-352).
Conflict of Interest
The authors declare that the research was conducted in the absence of any commercial or financial relationships that could be construed as a potential conflict of interest.
Publisher’s Note
All claims expressed in this article are solely those of the authors and do not necessarily represent those of their affiliated organizations, or those of the publisher, the editors and the reviewers. Any product that may be evaluated in this article, or claim that may be made by its manufacturer, is not guaranteed or endorsed by the publisher.
Acknowledgments
The authors would like to thank Zhoushe Zhao for his technical assistance.
Supplementary Material
The Supplementary Material for this article can be found online at: https://www.frontiersin.org/articles/10.3389/fphar.2022.862581/full#supplementary-material
References
Amado, R. G., Wolf, M., Peeters, M., Van Cutsem, E., Siena, S., Freeman, D. J., et al. (2008). Wild-type KRAS Is Required for Panitumumab Efficacy in Patients with Metastatic Colorectal Cancer. J. Clin. Oncol. 26 (10), 1626–1634. doi:10.1200/jco.2007.14.7116
Chang, C., Zhou, S., Yu, H., Zhao, W., Ge, Y., Duan, S., et al. (2021). A Clinically Practical Radiomics-Clinical Combined Model Based on PET/CT Data and Nomogram Predicts EGFR Mutation in Lung Adenocarcinoma. Eur. Radiol. 31 (8), 6259–6268. doi:10.1007/s00330-020-07676-x
Chawla, N. V., Bowyer, K. W., Hall, L. O., and Kegelmeyer, W. P. (2002). SMOTE: Synthetic Minority Over-sampling Technique. jair 16 (0), 321–357. doi:10.1613/jair.953
Delbeke, D., Coleman, R. E., Guiberteau, M. J., Brown, M. L., Royal, H. D., Siegel, B. A., et al. (2006). Procedure Guideline for Tumor Imaging with 18F-FDG PET/CT 1.0. J. Nucl. Med. 47 (5), 885–895.
Dogan, S., Shen, R., Ang, D. C., Johnson, M. L., D'Angelo, S. P., Paik, P. K., et al. (2012). Molecular Epidemiology of EGFR and KRAS Mutations in 3,026 Lung Adenocarcinomas: Higher Susceptibility of Women to Smoking-Related KRAS-Mutant Cancers. Clin. Cancer Res. 18 (22), 6169–6177. doi:10.1158/1078-0432.Ccr-11-3265
Drusbosky, L. M., Dawar, R., Rodriguez, E., and Ikpeazu, C. V. (2021). Therapeutic Strategies in METex14 Skipping Mutated Non-small Cell Lung Cancer. J. Hematol. Oncol. 14 (1), 129. doi:10.1186/s13045-021-01138-7
Fotouhi, S., Asadi, S., and Kattan, M. W. (2019). A Comprehensive Data Level Analysis for Cancer Diagnosis on Imbalanced Data. J. Biomed. Inform. 90, 103089. doi:10.1016/j.jbi.2018.12.003
Han, Y., Ma, Y., Wu, Z., Zhang, F., Zheng, D., Liu, X., et al. (2021). Histologic Subtype Classification of Non-small Cell Lung Cancer Using PET/CT Images. Eur. J. Nucl. Med. Mol. Imaging 48 (2), 350–360. doi:10.1007/s00259-020-04771-5
Hirsch, F. R., and Bunn, P. A. (2009). EGFR Testing in Lung Cancer Is Ready for Prime Time. Lancet Oncol. 10 (5), 432–433. doi:10.1016/s1470-2045(09)70110-x
Kang, F., Han, Q., Zhou, X., Zheng, Z., Wang, S., Ma, W., et al. (2020). Performance of the PET Vascular Activity Score (PETVAS) for Qualitative and Quantitative Assessment of Inflammatory Activity in Takayasu's Arteritis Patients. Eur. J. Nucl. Med. Mol. Imaging 47 (13), 3107–3117. doi:10.1007/s00259-020-04871-2
Kang, F., Mu, W., Gong, J., Wang, S., Li, G., Li, G., et al. (2019). Integrating Manual Diagnosis into Radiomics for Reducing the False Positive Rate of 18F-FDG PET/CT Diagnosis in Patients with Suspected Lung Cancer. Eur. J. Nucl. Med. Mol. Imaging 46 (13), 2770–2779. doi:10.1007/s00259-019-04418-0
Kang, F., Wang, S., Tian, F., Zhao, M., Zhang, M., Wang, Z., et al. (2016). Comparing the Diagnostic Potential of 68Ga-Alfatide II and 18F-FDG in Differentiating between Non-small Cell Lung Cancer and Tuberculosis. J. Nucl. Med. 57 (5), 672–677. doi:10.2967/jnumed.115.167924
Kerr, K. M., Bibeau, F., Thunnissen, E., Botling, J., Ryška, A., Wolf, J., et al. (2021). The Evolving Landscape of Biomarker Testing for Non-small Cell Lung Cancer in Europe. Lung Cancer 154, 161–175. doi:10.1016/j.lungcan.2021.02.026
Kim, J. O., Lee, J., Shin, J. Y., Oh, J. E., Jung, C. K., Park, J. K., et al. (2015). KIF5B-RET Fusion Gene May Coincide Oncogenic Mutations of EGFR or KRAS Gene in Lung Adenocarcinomas. Diagn. Pathol. 10, 143. doi:10.1186/s13000-015-0368-z
Koyasu, S., Nishio, M., Isoda, H., Nakamoto, Y., and Togashi, K. (2020). Usefulness of Gradient Tree Boosting for Predicting Histological Subtype and EGFR Mutation Status of Non-small Cell Lung Cancer on 18F FDG-PET/CT. Ann. Nucl. Med. 34 (1), 49–57. doi:10.1007/s12149-019-01414-0
Lynch, T. J., Bell, D. W., Sordella, R., Gurubhagavatula, S., Okimoto, R. A., Brannigan, B. W., et al. (2004). Activating Mutations in the Epidermal Growth Factor Receptor Underlying Responsiveness of Non-small-cell Lung Cancer to Gefitinib. N. Engl. J. Med. 350 (21), 2129–2139. doi:10.1056/NEJMoa040938
Mantilla, W. A., Sanabria-Salas, M. C., Baldion, A. M., Sua, L. F., Gonzalez, D. M., and Lema, M. (2021). NGS in Lung, Breast, and Unknown Primary Cancer in Colombia: A Multidisciplinary Consensus on Challenges and Opportunities. JCO Glob. Oncol. 7, 1012–1023. doi:10.1200/go.21.00046
Marchetti, A., Martella, C., Felicioni, L., Barassi, F., Salvatore, S., Chella, A., et al. (2005). EGFR Mutations in Non-small-cell Lung Cancer: Analysis of a Large Series of Cases and Development of a Rapid and Sensitive Method for Diagnostic Screening with Potential Implications on Pharmacologic Treatment. J. Clin. Oncol. 23 (4), 857–865. doi:10.1200/jco.2005.08.043
Mok, T., Ladrera, G., Srimuninnimit, V., Sriuranpong, V., Yu, C. J., Thongprasert, S., et al. (2016). Tumor Marker Analyses from the Phase III, Placebo-Controlled, FASTACT-2 Study of Intercalated Erlotinib with Gemcitabine/platinum in the First-Line Treatment of Advanced Non-small-cell Lung Cancer. Lung Cancer 98, 1–8. doi:10.1016/j.lungcan.2016.04.023
Molina, J. R., Yang, P., Cassivi, S. D., Schild, S. E., and Adjei, A. A. (2008). Non-small Cell Lung Cancer: Epidemiology, Risk Factors, Treatment, and Survivorship. Mayo Clin. Proc. 83 (5), 584–594. doi:10.4065/83.5.584
Mu, W., Jiang, L., Zhang, J., Shi, Y., Gray, J. E., Tunali, I., et al. (2020). Non-Invasive Decision Support for NSCLC Treatment Using PET/CT Radiomics. Nat. Commun. 11 (1), 5228. doi:10.1038/s41467-020-19116-x
Nagasaka, M., Zhu, V. W., Lim, S. M., Greco, M., Wu, F., and Ou, S. I. (2021). Beyond Osimertinib: The Development of Third-Generation EGFR Tyrosine Kinase Inhibitors for Advanced EGFR+ NSCLC. J. Thorac. Oncol. 16 (5), 740–763. doi:10.1016/j.jtho.2020.11.028
NCCN (2022). Login. Available at: https://www.nccn.org/professionals/physician_gls/pdf/nscl.pdf (Accessed January 25, 2022).
Palma, G., Khurshid, F., Lu, K., Woodward, B., and Husain, H. (2021). Selective KRAS G12C Inhibitors in Non-small Cell Lung Cancer: Chemistry, Concurrent Pathway Alterations, and Clinical Outcomes. NPJ Precis Oncol. 5 (1), 98. doi:10.1038/s41698-021-00237-5
Popat, S., Jung, H. A., Lee, S. Y., Hochmair, M. J., Lee, S. H., Escriu, C., et al. (2021). Sequential Afatinib and Osimertinib in Patients with EGFR Mutation-Positive NSCLC and Acquired T790M: A Global Non-interventional Study (UpSwinG). Lung Cancer 162, 9–15. doi:10.1016/j.lungcan.2021.09.009
Ren, M., Yang, H., Lai, Q., Shi, D., Liu, G., Shuang, X., et al. (2021). MRI‐based Radiomics Analysis for Predicting the EGFR Mutation Based on Thoracic Spinal Metastases in Lung Adenocarcinoma Patients. Med. Phys. 48, 5142–5151. doi:10.1002/mp.15137
Roosan, M. R., Mambetsariev, I., Pharaon, R., Fricke, J., Husain, H., Reckamp, K. L., et al. (2021). Usefulness of Circulating Tumor DNA in Identifying Somatic Mutations and Tracking Tumor Evolution in Patients with Non-small Cell Lung Cancer. Chest 160, 1095–1107. doi:10.1016/j.chest.2021.04.016
Santos, M., Coudry, R. A., Ferreira, C. G., Stefani, S., Cunha, I. W., Zalis, M. G., et al. (2019). Increasing Access to Next-Generation Sequencing in Oncology for Brazil. Lancet Oncol. 20 (1), 20–23. doi:10.1016/s1470-2045(18)30822-2
Shigematsu, H., and Gazdar, A. F. (2006). Somatic Mutations of Epidermal Growth Factor Receptor Signaling Pathway in Lung Cancers. Int. J. Cancer 118 (2), 257–262. doi:10.1002/ijc.21496
Siegel, R. L., Miller, K. D., Fuchs, H. E., and Jemal, A. (2022). Cancer Statistics, 2022. CA Cancer J. Clin. 72 (1), 7–33. doi:10.3322/caac.21708
Skoulidis, F., Li, B. T., Dy, G. K., Price, T. J., Falchook, G. S., Wolf, J., et al. (2021). Sotorasib for Lung Cancers with KRAS p.G12C Mutation. N. Engl. J. Med. 384 (25), 2371–2381. doi:10.1056/NEJMoa2103695
Sung, H., Ferlay, J., Siegel, R. L., Laversanne, M., Soerjomataram, I., Jemal, A., et al. (2021). Global Cancer Statistics 2020: GLOBOCAN Estimates of Incidence and Mortality Worldwide for 36 Cancers in 185 Countries. CA Cancer J. Clin. 71 (3), 209–249. doi:10.3322/caac.21660
Thein, K. Z., Velcheti, V., Mooers, B. H. M., Wu, J., and Subbiah, V. (2021). Precision Therapy for RET-Altered Cancers with RET Inhibitors. Trends Cancer 7, 1074–1088. doi:10.1016/j.trecan.2021.07.003
Weng, Q., Hui, J., Wang, H., Lan, C., Huang, J., Zhao, C., et al. (2021). Radiomic Feature-Based Nomogram: A Novel Technique to Predict EGFR-Activating Mutations for EGFR Tyrosin Kinase Inhibitor Therapy. Front. Oncol. 11, 590937. doi:10.3389/fonc.2021.590937
Wu, G., Jochems, A., Refaee, T., Ibrahim, A., Yan, C., Sanduleanu, S., et al. (2021). Structural and Functional Radiomics for Lung Cancer. Eur. J. Nucl. Med. Mol. Imaging 48, 3961–3974. doi:10.1007/s00259-021-05242-1
Yu, Z., Ji, H., Xiao, J., Wei, P., Song, L., Tang, T., et al. (2021). Predicting Adverse Drug Events in Chinese Pediatric Inpatients with the Associated Risk Factors: A Machine Learning Study. Front. Pharmacol. 12, 659099. doi:10.3389/fphar.2021.659099
Zhang, J., Zhao, X., Zhao, Y., Zhang, J., Zhang, Z., Wang, J., et al. (2020). Value of Pre-therapy 18F-FDG PET/CT Radiomics in Predicting EGFR Mutation Status in Patients with Non-small Cell Lung Cancer. Eur. J. Nucl. Med. Mol. Imaging 47 (5), 1137–1146. doi:10.1007/s00259-019-04592-1
Zhang, M., Tong, E., Wong, S., Hamrick, F., Mohammadzadeh, M., Rao, V., et al. (2021). Machine Learning Approach to Differentiation of Peripheral Schwannomas and Neurofibromas: A Multi-Center Study. Neuro Oncol. noab211. doi:10.1093/neuonc/noab211
Zhou, H., Hu, Y., Luo, R., Zhao, Y., Pan, H., Ji, L., et al. (2021). Multi-region Exome Sequencing Reveals the Intratumoral Heterogeneity of Surgically Resected Small Cell Lung Cancer. Nat. Commun. 12 (1), 5431. doi:10.1038/s41467-021-25787-x
Zhou, Y., Ma, X. L., Zhang, T., Wang, J., Zhang, T., and Tian, R. (2021). Use of Radiomics Based on 18F-FDG PET/CT and Machine Learning Methods to Aid Clinical Decision-Making in the Classification of Solitary Pulmonary Lesions: an Innovative Approach. Eur. J. Nucl. Med. Mol. Imaging 48 (9), 2904–2913. doi:10.1007/s00259-021-05220-7
Zwanenburg, A., Leger, S., Vallières, M., and Lock, S. (2017). Image Biomarker Standardisation Initiative. Available at: https://arxiv.org/abs/1612.07003v11 (Accessed January 25, 2022).
Keywords: non-small cell lung cancer, EGFR, KRAS, PET/CT, radiomics
Citation: Wang J, Lv X, Huang W, Quan Z, Li G, Wu S, Wang Y, Xie Z, Yan Y, Li X, Ma W, Yang W, Cao X, Kang F and Wang J (2022) Establishment and Optimization of Radiomics Algorithms for Prediction of KRAS Gene Mutation by Integration of NSCLC Gene Mutation Mutual Exclusion Information. Front. Pharmacol. 13:862581. doi: 10.3389/fphar.2022.862581
Received: 26 January 2022; Accepted: 14 March 2022;
Published: 01 April 2022.
Edited by:
Qi Zeng, Xidian University, ChinaCopyright © 2022 Wang, Lv, Huang, Quan, Li, Wu, Wang, Xie, Yan, Li, Ma, Yang, Cao, Kang and Wang. This is an open-access article distributed under the terms of the Creative Commons Attribution License (CC BY). The use, distribution or reproduction in other forums is permitted, provided the original author(s) and the copyright owner(s) are credited and that the original publication in this journal is cited, in accordance with accepted academic practice. No use, distribution or reproduction is permitted which does not comply with these terms.
*Correspondence: Xin Cao, xin_cao@163.com; Fei Kang, fmmukf@qq.com; Jing Wang, 13909245902@163.com
†These authors have contributed equally to this work