- 1National Clinical Research Center for Hematologic Diseases, Jiangsu Institute of Hematology, The First Affiliated Hospital of Soochow University, Suzhou, China
- 2Institute of Blood and Marrow Transplantation, Collaborative Innovation Center of Hematology, Soochow University, Suzhou, China
- 3Key Laboratory of Thrombosis and Hemostasis of Ministry of Health, Suzhou, China
- 4State Key Laboratory of Radiation Medicine and Protection, Soochow University, Suzhou, China
ITP is a common autoimmune bleeding disorder with elusive pathogenesis. Our study was implemented to profile the plasma metabolic alterations of patients diagnosed with ITP, aiming at exploring the potential novel biomarkers and partial mechanism of ITP. The metabolomic analysis of plasma samples was conducted using GC-MS on 98 ITP patients and 30 healthy controls (HCs). Age and gender matched samples were selected to enter the training set or test set respectively. OPLS-DA, t-test with FDR correction and ROC analyses were employed to screen out and evaluate the differential metabolites. Possible pathways were enriched based on metabolomics pathway analysis (MetPA). A total of 85 metabolites were investigated in our study and 17 differential metabolites with diagnostic potential were identified between ITP patients and HCs. MetPA showed that the metabolic disorders of ITP patients were mainly related to phenylalanine, tyrosine and tryptophan biosynthesis, phenylalanine metabolism and glyoxylate and dicarboxylate metabolism. Additionally, we discriminated 6 differential metabolites and 5 enriched pathways in predicting the resistance to glucocorticoids in chronic ITP patients. The distinct metabolites discovered in our study could become novel biomarkers for the auxiliary diagnosis and prognosis prediction of ITP. Besides, the dysregulated pathways might contribute to the development of ITP.
Introduction
ITP is a common hematological disease, accounting for about one-third of clinical hemorrhagic disease. The diagnostic criteria of ITP are still based on clinical exclusion method, excluding thrombocytopenia secondary to other factors (such as infection, drugs and various connective tissue diseases, etc.) (Neunert et al., 2020). Treatment with glucocorticoids is recommended as the standard first-line strategy, achieving responses in 50–85% of ITP patients (Mithoowani et al., 2016; Y.; Kong et al., 2018). However, nearly 80% of the adult patients fail or become dependent on corticosteroids and require subsequent therapy like TPO receptor agonists, rituximab, splenectomy, etc (Ghanima et al., 2021). Till now, the pathogenesis of ITP remains obscure, antibody-mediated platelet destruction, T cell imbalance (Li J. et al., 2020), platelet desialylation (Li J. et al., 2015) and oxidative stress (Qi et al., 2017) have been recognized as important mechanisms of ITP.
In recent years, metabolomics obtained great development in disease diagnosis, pharmaceutical research, and other fields. The components of metabolome can be viewed as the end products of gene expression in a particular physiological period or physiological conditions and define the biochemical phenotype of a cell or tissue. These products are small molecule compounds involved in metabolism, maintenance of normal function and development of the organism, mainly including some endogenous molecules with relative molecular weight less than 1,000 daltons (Sumner et al., 2003). Metabolomics is a powerful tool for investigating phenotypic abnormalities in mutant animals and human diseases, and in modelling physiological variation in experimental animals and man (Nicholson et al., 1999). Gas chromatography tandem mass spectrometry (GC-MS) is an analytical instrument for measuring the mass to charge ratio (m/z) of ions, with the advantages of high resolution, high flux, high precision, sensitivity and high reproducibility, and can determine some heat-resistant metabolites, which is suitable for the analysis of substances with small polarity (Jonsson et al., 2005).
Benefitting from advances in omics technologies, metabolomics methods have been widely used to explore potential markers of diseases and gain novel understanding of the metabolic pathway changes in pathogenesis. At present, metabolomics methods have been applied in the study of various hematological malignancies (Chanukuppa et al., 2018), diabetes mellitus (Arneth et al., 2019), hypertension, coronary heart disease (McGarrah et al., 2018) and others. The extensive use of metabolomics methods has also attracted high attention in the study of the physiological activities of platelets, and several studies have been implemented to elucidate metabolic changes of platelets in resting or activated states. (Aibibula et al., 2018) found that platelets switched freely between glycolysis and OXPHOS, consuming either glucose or fatty acids at rest. The transition of platelets from a quiescent to an activated state promoted rapid uptake of exogenous glucose, and preferentially utilize glycolysis for ATP generation when activated. Dhenge et al. found that arachidonic acid (AA)/DHA appears to have enhanced megakaryocytes and platelets generation in cell numbers, surface marker expression, cellular ploidy and expression of cytoskeletal components (Dhenge et al., 2017). Li et al. reported that indoleamine 2, 3-dioxygenase (IDO) mediates abnormal tryptophan metabolism and plays an important role in the pathogenesis of ITP (Li Z. J. et al., 2015). Besides, branched-chain amino acids (BCAA) metabolism was reported to regulate platelet activation and was associated with arterial thrombosis risk (Y. Xu et al., 2020). To our knowledge, only few studies have investigated ITP with the implement of metabolomics so far. A study concerning gut microbiome and metabolome showed that several ITP-altered gut bacteria as well as metabolites could be diagnostic biomarkers for ITP, and were highly correlated with platelet counts, suggesting the potential role of metabolomics methods in the pathogenesis mining of ITP (Zhang X. et al., 2020).
In this study, we developed a stable and reliable GC-MS analytical method to detect human plasma samples from ITP patients, expecting to identify the plasma metabolomic profile of ITP. We screened out differential metabolites and metabolic pathways related to ITP to provide new indexes for diagnosis and prognosis of ITP. Our finding could provide a new approach to reveal possible mechanism of ITP.
Patients and Methods
Enrollment of Patients and Diagnosis Criteria
ITP patients who were hospitalized at the First Affiliated Hospital of Soochow University from August 2018 to April 2019 were enrolled in this study at the ratio of 3:1 for ITP group and HC group respectively with age and gender matched. In order to further understand the changes of metabolites in ITP patients before and after treatment, the plasma samples of patients before and till the platelet count of 100 × 109/L after treatment were collected, and the metabolites were detected by GC-MS method.
The diagnosis and staging of ITP were based on the consensus of Chinese experts on the diagnosis and treatment of adult primary immune thrombocytopenia (2020) (Thrombosis and Hemostasis Group, Chinese Society of Hematology, Chinese Medical Association, 2020). Newly diagnosed ITP (nITP) is defined as up to 3 months from diagnosis. The course of disease of persistent ITP (pITP) is 3–12 months, and chronic ITP (cITP) is defined as ITP lasting more than 12 months (Neunert et al., 2019). Sustained remission (SR) was defined as maintenance of platelet count above 50 × 109/L for over 6 months (Ahn and Harrington, 1977)and patients did not reach the standard were defined as non-SR (NSR). The good therapeutic effect is defined as a platelet count over 30 × 109/L independent of transfusion, or at least twice the baseline platelet level of the patient without any bleeding symptoms after treatment. Patients that don’t reach the criteria above are considered poor therapeutic effect and need to switch to second-line treatment (Thrombosis and Hemostasis Group, Hematology Society, Chinese Medical Association, 2016).
Study Approval
The study protocol was approved by the Faculty Hospital Ethics Committee at the First Affiliated Hospital of Soochow University in accordance with the guidelines in the Declaration of Helsinki. All ITP patients and HCs in this study signed informed consent forms, requiring patients and HCs to have a light diet and avoid stimulation from tobacco, alcohol and spicy food before sampling, so as to reduce the fluctuation of metabolite levels caused by dietary factors. Healthy subjects matched with the age and gender of ITP patients were selected to minimize the possible interference factors.
Sample Acquisition and Preservation
All fasting peripheral blood samples were collected with EDTA anticoagulation tubes and centrifuged at 3000 rpm for 15 min. Each plasma sample was divided into equal aliquots and stored at −80°C for analysis.
With batch experiments, plasma samples were thawed at room temperature, 100 μl of which were extracted and vortexed for quality control (QC). Subsequently, another 100 μl plasma was taken and mixed with 400 μl glacial acetonitrile, 80 μl 2, 4-dichlorobenzoic acid (BOC) with concentration of 0.2 mg/ml and 80 μl tridecanoic acid with concentration of 5 μg/ml as the internal standard, and then vortexed and mixed for 2 min. Then ultrasonic extraction was carried out for 15 min (1°C), followed by centrifugation for 15 min (13000 rpm/min, 4°C), and the supernatant 450 μl was transferred to a new EP tube and placed in a refrigerator at −80°C for more than 6 h. Then the samples were made into freeze-dried powder to ensure that the sample is absolutely anhydrous. The dried samples were redissolved with methoxyamine pyridine solution (15 mg/ml, 50 μl) and vortexed. Afterwards, the sample was oximated in a metal bath thermostat at 70°C for 1 h, followed by mixture with 50 μl N-methyl trimethylsilyl trifluoroacetyl (MSTFA) [containing 1% trimethylchlorosilane (TMCS) as catalyst] in a metal bath thermostat at 70°C for 1 h and cooling for half an hour. Finally, the derivatized samples were mixed with 100 μl n-heptane and centrifuged (13000 rpm/min, 4°C, 15 min) and then the supernatant was analyzed by GC-MS. All derived samples were tested by GC-MS within 2 days after derivation to ensure the stability and reliability of the results.
Gas Chromatography-Mass Spectrometry -Based Metabolomics
Metabolic profiling of plasma samples was acquired using a TSQ 8000 EVO Triple Quadpole GMS (Thermo, United States). Each derivatized sample was injected into a DB-5 fused silica capillary column (30 m × 0.25 mm ×0.25 μM; Agilent, United States) in a split mode (ratio 10:1). Helium with high purity (99.9%) was used as the carrier gas, and operated with a constant flow rate of 1.1 ml/min. The initial column temperature of 80°C was maintained for 5 min, then increased to 170°C at 5°C/min, and finally to 300°C at 10°C per minute intervals. The temperatures of the inlet and ion source were at 280 and 230°C. The mass spectra data were obtained in a full scan with 30–600 m/z. Plasma samples were all running in a random order and the QC sample was inserted approximately every 10 experimental samples.
The plasma metabolites were analyzed by GC-MS, and the original data were imported to obtain the Total Ion Chromatogram (TIC). Automated Mass Spectral Deconvoltion and Identification System (AMDIS) and the National Institute of Standards Technology (NIST) 11.0 mass spectrometry database for mass spectrometric detection, deconvolution, identification and matching of each chromatographic peak and combined with the standard and retention time to further identify the substance.
Statistical Analysis
After the metabolites in plasma were identified, the original data were processed in the XCalibur workstation, and the chromatographic peaks were integrated according to retention time and quality. The integral results were corrected using the internal standard (2,4-dichrobenzoic acid). Finally, the corrected area ratio of each metabolite was obtained to form the metabolite matrix.
In order to find the characteristic metabolites of ITP patients, propensity score matching (PSM) was conducted to match patients on age and gender between the two groups, entering the training set and test set respectively. In the training set, orthogonal partial least squares discriminant analysis (OPLS-DA) and t-test combined with false discovery rate (FDR) correction were used to screen out the differential metabolites of ITP patients and HC. The receiver operating curve (ROC) was applied to evaluate the discriminatory capability of the differential metabolites in the training set and the test set respectively. Univariate and multivariate logistic regression analyses were performed to identify predictive factors for treatment and prognosis. Finally, MetPA was implemented to construct the metabolic pathway in MetaboAnalyst 5.0. OPLS-DA model was also carried out to compare the plasma metabolomic profiles of the subgroups of ITP patients to further explore factors concerning clinical application.
Results
Characteristics of Immune Thrombocytopenia Patients
Ninety-eight ITP patients hospitalized at the First Affiliated Hospital of Soochow University from August 2018 to April 2019 were enrolled in this study, including 29 males and 69 females, with a median age of 42.5 years (12–72 years). 30 HCs in the same period were selected as the control group, including 10 males and 20 females, with a median age of 39.5 years (14–78 years). Data from 114 plasma samples (from 57 patients on admission and after treatment) was further collected to validate the differential metabolites screened from ITP patients. Main characteristics of the study participants are summarized in Table 1.
2:1 PSM was conducted in the case group (98 patients with ITP) and the control group (30 HCs) according to gender and age, certain factors that may influence the plasma metabolomic profile (Supplementary Table S1) (Laugsand et al., 2016; Han et al., 2021). As a result, a total of 30 ITP patients and 15 HCs were successfully matched into the training set, while the remaining 68 ITP patients and 15 HCs samples entered the test set.
Comprehensive Metabolomic Analysis of Immune Thrombocytopenia Patients
The metabolomic analysis of plasma samples was conducted using GC-MS on 98 ITP patients and 30 HCs. Quality control (QC) samples were prepared in parallel according to the pretreatment method of plasma samples in the experimental group to monitor the reproducibility of experimental sample preparation. Representative GC-MS total ion chromatograms (TIC) over the QC plasma sample is shown in Figure 1. AMDIS and NIST mass spectrometry databases were used for mass spectrometric detection, deconvolution, identification and matching of each chromatographic peak, and retention time (RT) and mass charge ratio were combined to identify the chromatographic peaks. The retention time drift of each chromatographic peak was limited to 30 s. Then, 85 metabolites were finally identified, including saccharides, amino acids, fatty acids and so on.
Immune Thrombocytopenia Exhibited a Particular Metabolomic Profile With Diagnostic Potential
In order to better visualize and denoise the multidimensional data, orthogonal partial least squares discriminant analysis (OPLS-DA) was applied to model the difference between the ITP patients and HCs in the training set and the parameters of the score plot R2Y = 0.937 and Q2 = 0.701 (Figure 2A) suggested the good explanatory and predictive ability of our model, respectively. The OPLS-DA model was further validated by the 200 random permutation tests with Q2 = -0.847 (Q2 < 0.05), indicating the model was not over-fitting (Figure 2B). Distinct separation was observed between ITP patients and HCs in the score scatter plot based on the OPLS-DA supervised model and 36 metabolites with a variable importance in projection (VIP) value > 1 (Sinclair et al., 2021) were chosen as differential biomarkers initially (Figure 2C). In combined with t-test and false discovery rate (FDR) correction, we identified 32 metabolites with p < 0.05 that may have potential to distinguish ITP patients from HCs (Supplementary Table S2). ROC analysis was performed to evaluate the differential metabolites in the training set and the test set separately. The area under curve (AUC) of each differential metabolites was calculated to determine their individual performance as ITP diagnostic biomarkers. In the training set, 23 metabolites were selected with an AUC > 0.7, followed by the ROC analysis conducted in the test set as external verification. As a result, 17 metabolites with an AUC > 0.7 (Figure 2D and summarized in Supplementary Table S3) were identified as differential metabolites with significant diagnostic potential to distinguish ITP patients from HCs: glycerol monostearate, 1-monopalmitin, glycerol, DHA, octadecadienoic acid, isovaleric acid, cis-aconitate, D-fructose, galactitol, myo-inositol, L-valine, phenylalanine, L-proline, glycine, 3-HB, creatinine, 3-hydroxypropionic acid.
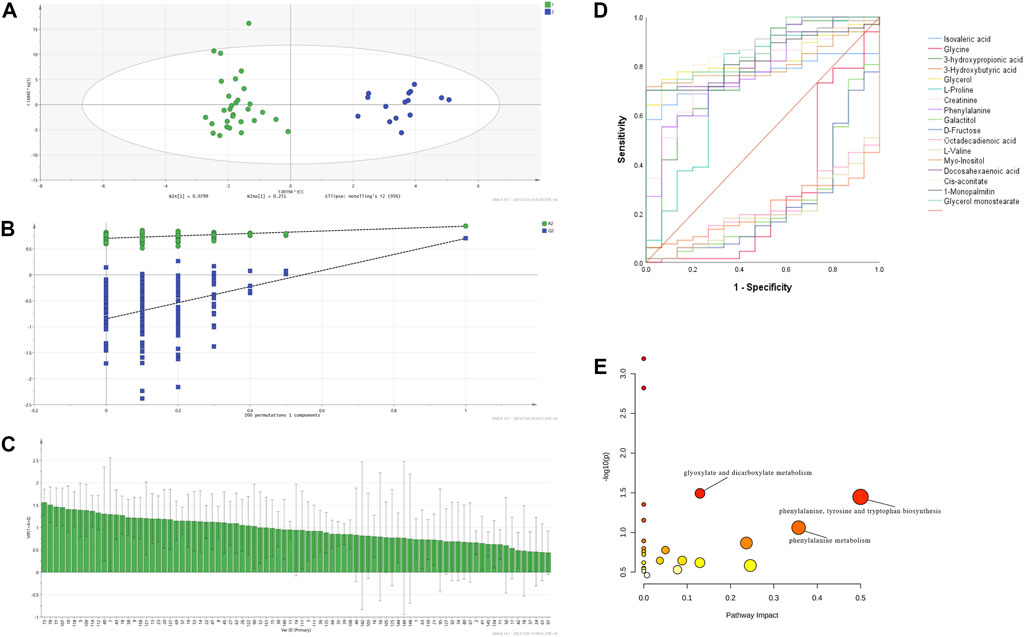
FIGURE 2. ITP exhibited a particular metabolomic profile with diagnostic potential. OPLS-DA model based on the data from the ITP patients and HCs (A) score scatter plot (B) 200 random permutation tests (C) VIP plot (D) ROC of the 17 differential metabolites with biomarker potential in ITP in the test set (E) MetPA of differential plasma metabolites in ITP patients.
The distinct variation of metabolomic profile between ITP patients and HCs prompted us to explore metabolites that could be used to predict prognosis. To determine which metabolites were of significant prognostic value in ITP patients, 17 differential metabolites and related clinical characteristics such as gender, age, clinical stage, severity, therapeutic effect of glucocorticoids, Helicobacter pylori (H. pylori) infection, reticulocyte, platelet-associated immunoglobulin (PAIgG) were compared among ITP patients between sustained remission (SR) group and non-SR (NSR) group. Univariate analysis showed that glycerol, 3-hydroxypropionic acid, galactitol, D-fructose, cis-aconitate (p < 0.05) were significantly associated with the NSR. Only metabolites with p < 0.2 in the univariate analysis were further analyzed in the multivariate logistic regression model (Kang et al., 2013). The multivariate analysis identified that glycerol (OR = 1.049, 95%CI: 1.009–1.091) was independently associated with higher risk of NSR Table 2.
Using MetaboAnalyst 5.0 online platform, pathway analysis of 17 differential metabolites was conducted and the enriched pathways were screened out by the threshold of impact value > 0.05 and -log10(p) > 1. Three deregulated pathways in ITP were identified and annotated in the bubble plot (Figure 2E): phenylalanine, tyrosine and tryptophan biosynthesis, phenylalanine metabolism, glyoxylate and dicarboxylate metabolism, with pathway impact values of 0.5, 0.36, 0.13 and the -log10(p) value of each pathway was 1.45, 1.06, 1.49, respectively.
The Metabolomic Profile did not Vary Significantly Before and After Treatment in Immune Thrombocytopenia Patients
To further explore the changes of metabolites in ITP patients before and after treatment, the principal component analysis (PCA) model was developed to compare the difference between the two groups. As was suggested in the score scatter plot below (Supplementary Figure S1), the metabolomic profile failed to show a separation tendency before and after treatment in ITP patients. Given the plasma samples were collected at the time of platelet counts just recovered and the limit of sample size, further researches are needed to determine the metabolomic profile of patients with stable remission. Besides, the metabolomic profile grouped by gender, age, clinical stage, severity, reticulocyte, PAIgG among the ITP patients in our study did not separate significantly.
Six Differential Metabolites Were Identified From the cITP Patients Grouped by Therapeutic Effect of Glucocorticoids
The OPLS-DA supervised model was also used to compare plasma metabolomic profiles of cITP patients with good and poor therapeutic effect of glucocorticoids. The score scatter plot showed a clear distribution between the two groups (Figure 3A) and was validate by permutation tests (Figure 3B). The following metabolites with a VIP value > 1, p < 0.05 as well as an AUC > 0.7 were considered as metabolites with biomarker potential: lactic acid, glyceric acid, L-valine, pyruvic acid, uric acid, α-ketoglutaric acid, showing high accuracy and specificity in predicting the therapeutic effect of ITP patients with chronic phase. The AUC of the 6 differential metabolites were 0.868, 0.825, 0.816, 0.812, 0.803, 0.799, respectively (Figure 3C). These metabolites may serve as potential biomarkers to guide early switch to second-line treatment. MetPA identified 5 enriched metabolic pathways perturbed significantly between the two groups in cITP patients (Figure 3D): citrate cycle, pyruvate metabolism, glycolysis/gluconeogenesis, glyoxylate and dicarboxylate metabolism, glycerolipid metabolism (impact value > 0.05 and -log10(p) > 1) (Table 3).
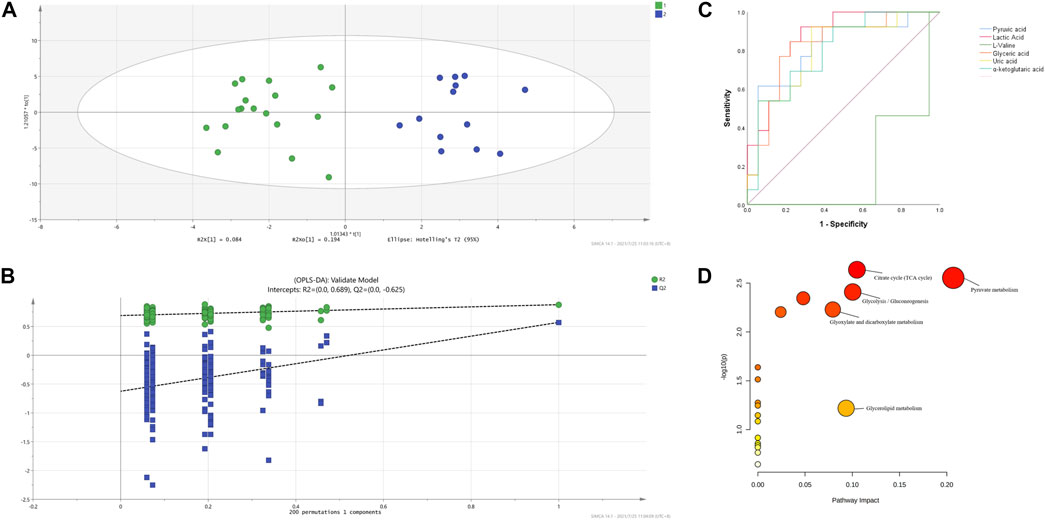
FIGURE 3. OPLS-DA model based on the data from the cITP patients grouped by therapeutic effect (A) score scatter plot (B) 200 random permutation tests (C) ROC of the six differential metabolites with biomarker potential (D) MetPA of differential plasma metabolites in cITP patients grouped by therapeutic effect.
Three Distinct Metabolites and Related Pathways Were Identified Between H. Pylori-Positive and H. Pylori-Negative Patients With cITP
Several studies have demonstrated an increased prevalence of H. pylori infection in patients with cITP (Kohda et al., 2002; Suzuki et al., 2006) and eradication of H. pylori contributed to the increase in platelet counts in cITP patients (Noonavath et al., 2014). Accordingly, we developed the OPLS-DA model based the metabolomic data between H. pylori-positive and H. pylori-negative patients with cITP and validated by permutation tests (Figures 4A,B). Combining the VIP > 1 from OPLS-DA model, p < 0.05 and AUC > 0.7 from ROC analysis (Figures 4C–E), three distinct metabolites were eventually obtained: D-fructose, galactitol, D-glucuronic acid. Followed by the MetPA, we revealed the related metabolic pathways were ascorbate and aldarate metabolism, pentose and glucuronate interconversions (Figure 4F).
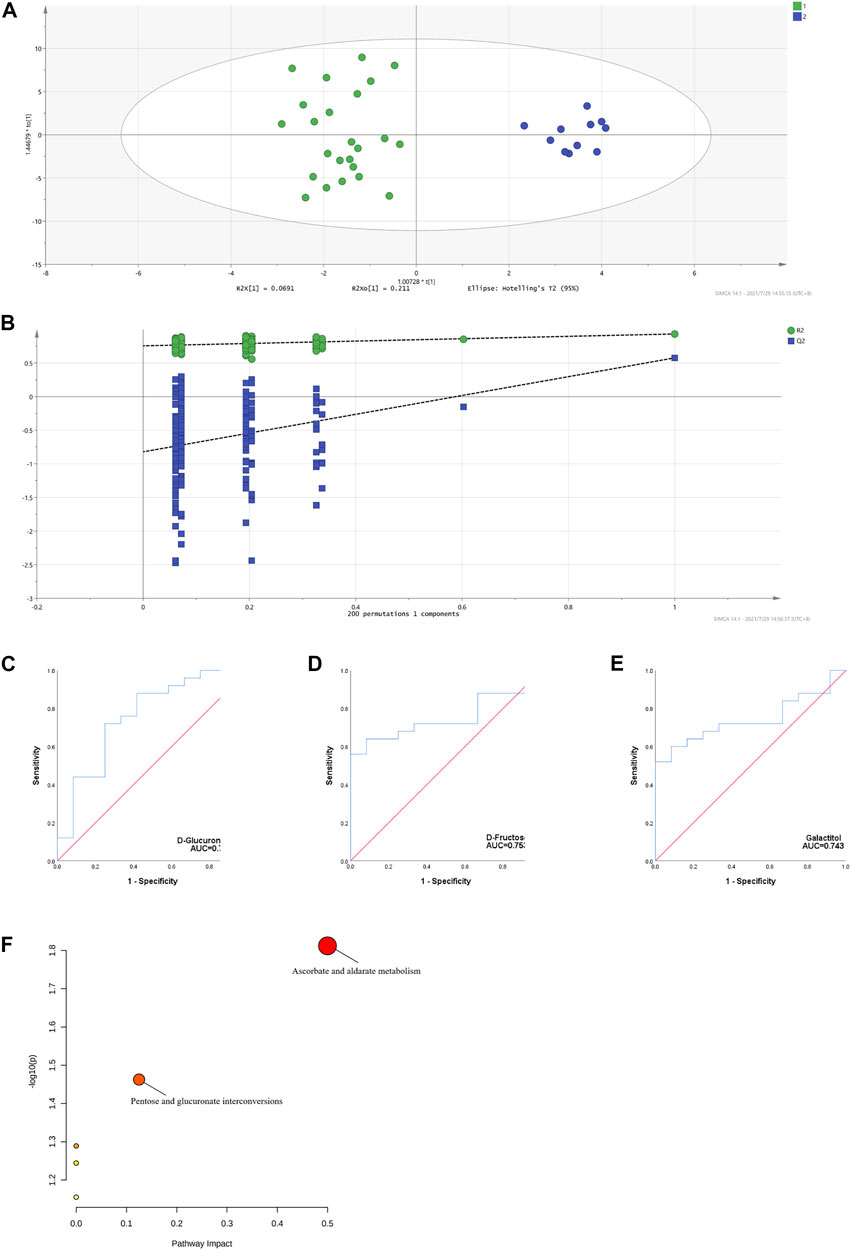
FIGURE 4. OPLS-DA model based on the data from the cITP patients grouped by (H) pylori infection (A) score scatter plot (B) 200 random permutation tests (C–E) ROC of the three distinct metabolites with biomarker potential (F) MetPA of the three distinct metabolites in cITP patients grouped by (H) pylori infection.
Discussion
To the best of our knowledge, no previous research has examined metabolite the comprehensive metabolomic profile of ITP patients or identified the differential plasma metabolites of high diagnostic and prognostic accuracy. In the current study, the metabolomic data acquired by GC-MS discriminated 17 differential metabolites from ITP patients to HCs. Accordingly, the dysregulated metabolic pathways related to ITP included may be associated to phenylalanine, tyrosine and tryptophan biosynthesis, phenylalanine metabolism, glyoxylate and dicarboxylate metabolism.
The mechanism underlying ITP includes enhanced platelet clearance and impaired platelet production, which is mainly ascribe to immune dysregulation mediated by B cells and antibodies, T cell-mediated effects, tolerance checkpoint defects and more (J. Li et al., 2017). However, no study to date has elucidated the pathology of ITP from metabolic disorders. It has been reported that chronic immune stimulation correlates with reduced phenylalanine turnover (Neurauter et al., 2008). Higher serum phenylalanine levels related to immune activation were detectable in patients with Alzheimer’s disease, in the pathogenesis of which immune activation and inflammation represented critical mechanisms (Wissmann et al., 2013). Li et al. suggested that mild immune stress caused metabolic reprogramming including phenylalanine metabolism (Li C.Y. et al., 2020), implicating a potential relationship between immunomodulation and phenylalanine related metabolic pathways. The glyoxylate and dicarboxylate metabolism is closely associated with the citric acid cycle and contributes to the energy metabolism (Song et al., 2021). Previous studies have shown that the shift to glyoxylate and dicarboxylate metabolism is a marker for decreased tolerance to insult (Black et al., 2012). Higher levels of ROS were reported to be involved in the pathogenesis of corticosteroid-resistant ITP(Y. Kong et al., 2018). Cano et al. showed that dysfunctions in glyoxylate and dicarboxylate metabolism pathway indicate mitochondrial dysfunction that would result in decreased ability to detoxify reactive oxygen species (ROS), serving as predisposing factors for the development of therapy-related myelodysplasia syndrome (Cano et al., 2011). Besides, Geng et al. elucidated that glyoxylate and dicarboxylate metabolism contributed to the lipopolysaccharide (LPS)-induced systemic inflammatory response syndrome (SIRS) (Geng et al., 2020) and ITP is commonly considered as an autoimmune disease and regarded as inflammation to some extent (J. Li et al., 2017).
Among the differential metabolites, DHA is the major polyunsaturated fatty acids closely related to platelet activity. DHA has been demonstrated to diminish platelet aggregation and to lengthen bleeding time, while octadecadienoic acid was found to improve platelet aggregation (ten Hoor, 1980) and presented an inverse association with the risk for stroke (Zhang W. et al., 2020). Dietary interventions with DHA may reduce the risk of heart diseases and has been proposed as an antithrombotic therapy (Wijendran and Hayes, 2004), and were found to decrease ROS production and improve platelet redox balance in T2DM patients (McDonald et al., 2013). Increase of DHA was associated with an increased ability of platelets to respond to an inflammatory stimulus (Souza et al., 2020). Enhanced generation of megakaryocytes and platelets was observed in the presence of DHA (Siddiqui et al., 2011), possibly through upregulation of the NOTCH and AKT pathways (Dhenge et al., 2017). In our study, decreased level of DHA was observed in ITP patients compared with HCs, suggesting a profound role of DHA to improve platelets in ITP patients. In our study, glycerol was identified to be the independent risk factor of NSR in ITP patients. Glycerol is a three-carbon substance that forms the backbone of fatty acids in fats. Murgia et al. reported that glycerol was one of the potential biomarkers to distinguish systemic sclerosis from HCs by metabolomics analysis (Murgia et al., 2018). Atorvastatin is a widely used anti-lipid reagent. Emerging studies have indicated that atorvastatin may support megakaryocytopoiesis and exhibited comparable immunomodulatory function, representing a novel therapy approach for corticosteroid-resistant ITP patients. Kong’s study (Y Kong et al., 2018) demonstrated that atorvastatin restored the impaired function of endothelial progenitor cells of corticosteroid-resistant ITP patients and increased the platelet counts of them in a pilot study. More recently, Xu et al. (2021) revealed that atorvastatin ameliorated inhibited the activation of CD4+ T cells, leading to the improvement of platelet count. Glycerol monostearate and 1-monopalmitin are both monoacylglycerols, formed biochemically via release of a fatty acid. Monoacylglycerols metabolism is also related to the effects of endocannabinoids in the immune system (Chiurchiu et al., 2015). 3-HB is one of the ketone bodies serving as a biomarker to reflect fatty acid β-oxidation and ketogenic amino acid catabolism (Choi et al., 2020; L.; Guo et al., 2021). 3-HB was also found to be associate with excess glutathione demand and disrupted mitochondrial energy mechanisms, occurring during persistent oxidative stress (Volani et al., 2018). The increased 3-HB production in our study may indicate the greater oxidative stress in ITP patients. As to the involved amino acid metabolism, the glycine level was increased in ITP patients. L-valine is one of the branched-chain amino acids (BCAAs). The BCAA catabolism was reported to regulate platelet activation and was associated with arterial thrombosis risk (Y. Xu et al., 2020). L-proline is a component of collagen metabolites and increased L-proline level may be related to the activation of collagen breakdown (Y. Guo et al., 2020). In addition, glycine was reported to play a role in affecting platelet membrane potential and hence aggregation capacity (Schemmer et al., 2013).
Additionally, we distinguished six significantly altered endogenous metabolites (lactic acid, glyceric acid, L-valine, pyruvic acid, uric acid, α-ketoglutaric acid) and five enriched metabolic pathways (citrate cycle, pyruvate metabolism, glycolysis/gluconeogenesis, glyoxylate and dicarboxylate metabolism, glycerolipid metabolism) in predicting the therapeutic effect of glucocorticoids in chronic ITP patients. Three distinct metabolites (D-fructose, galactitol, D-glucuronic acid) and related pathways (ascorbate and aldarate metabolism, pentose and glucuronate interconversions) were identified between H. pylori-positive and H. pylori-negative patients with cITP. The dysregulated metabolic pathways were mainly associated with glucose metabolism, which is essential for platelet function and clearance (Fidler et al., 2017). However, the exact mechanisms between these identified metabolic profiles with ITP are far from clear. As limitations of sample qualities, more patients need to be included in the future study.
Conclusion
In conclusion, this study, using GC-MS based metabolomic methods, has provided for the first time a comprehensive metabolomic profile in plasma of ITP patients. Our explanation of the metabolic disturbance described here and the underlying mechanisms are far from clear. The exact relationships between these metabolic alterations and ITP development need to be validated subsequently by researches with a larger sample size.
Data Availability Statement
The original contributions presented in the study are included in the article/Supplementary Material, further inquiries can be directed to the corresponding author.
Ethics Statement
The studies involving human participants were reviewed and approved by the Faculty Hospital Ethics Committee at the First Affiliated Hospital of Soochow University. Written informed consent to participate in this study was provided by the participants’ legal guardian/next of kin.
Author Contributions
ZZ performed experiments and acquired data, ZZ, MZ, and JQ analyzed data, XW and YH conceptualized and supervised the project, ZZ wrote the manuscript, MZ, RZ, and XL revised the manuscript, XW, CW, CR, and YH provided resources.
Funding
This work was supported by the National Natural Science Foundation of China (81873432 and 82070143), grants from the Jiangsu Province of China (BE2021645), and the Priority Academic Program Development of Jiangsu Higher Education Institutions (PAPD).
Conflict of Interest
The authors declare that the research was conducted in the absence of any commercial or financial relationships that could be construed as a potential conflict of interest.
Publisher’s Note
All claims expressed in this article are solely those of the authors and do not necessarily represent those of their affiliated organizations, or those of the publisher, the editors and the reviewers. Any product that may be evaluated in this article, or claim that may be made by its manufacturer, is not guaranteed or endorsed by the publisher.
Supplementary Material
The Supplementary Material for this article can be found online at: https://www.frontiersin.org/articles/10.3389/fphar.2022.845275/full#supplementary-material
References
Ahn, Y. S., and Harrington, W. J. (1977). Treatment of Idiopathic Thrombocytopenic Purpura (ITP). Annu. Rev. Med. 28, 299–309ENG. doi:10.1146/annurev.me.28.020177.001503
Aibibula, M., Naseem, K. M., and Sturmey, R. G. (2018). Glucose Metabolism and Metabolic Flexibility in Blood Platelets. J. Thromb. Haemost. 16 (11), 2300–2314. doi:10.1111/jth.14274
Arneth, B., Arneth, R., and Shams, M. (2019). Metabolomics of Type 1 and Type 2 Diabetes. Int. J. Mol. Sci. 20 (10), 2467. doi:10.3390/ijms20102467
Black, K. M., Barnett, R. J., Bhasin, M. K., Daly, C., Dillon, S. T., Libermann, T. A., et al. (2012). Microarray and Proteomic Analysis of the Cardioprotective Effects of Cold Blood Cardioplegia in the Mature and Aged Male and Female. Physiol. Genomics 44 (21), 1027–1041. doi:10.1152/physiolgenomics.00011.2012
Cano, K. E., Li, L., Bhatia, S., Bhatia, R., Forman, S. J., and Chen, Y. (2011). NMR-based Metabolomic Analysis of the Molecular Pathogenesis of Therapy-Related Myelodysplasia/acute Myeloid Leukemia. J. Proteome Res. 10 (6), 2873–2881. doi:10.1021/pr200200y
Chanukuppa, V., Taware, R., Chatterjee, T., Sharma, S., More, T. H., Taunk, K., et al. (2018). Current Understanding of the Potential of Proteomics and Metabolomics Approaches in Cancer Chemoresistance: A Focus on Multiple Myeloma. Curr. Top. Med. Chem. 18 (30), 2584–2598. doi:10.2174/1568026619666181130111202
Chiurchiù, V., Battistini, L., and Maccarrone, M. (2015). Endocannabinoid Signalling in Innate and Adaptive Immunity. Immunology 144 (3), 352–364. doi:10.1111/imm.12441
Choi, R. Y., Ji, M., Lee, M. K., and Paik, M. J. (2020). Metabolomics Study of Serum from a Chronic Alcohol-Fed Rat Model Following Administration of Defatted Tenebrio molitor Larva Fermentation Extract. Metabolites 10 (11), 436. doi:10.3390/metabo10110436
Dhenge, A., Limbkar, K., Melinkeri, S., Kale, V. P., and Limaye, L. (2017). Arachidonic Acid and Docosahexanoic Acid Enhance Platelet Formation from Human Apheresis-Derived CD34+ Cells. Cell Cycle 16 (10), 979–990. doi:10.1080/15384101.2017.1312233
Fidler, T. P., Campbell, R. A., Funari, T., Dunne, N., Balderas Angeles, E., Middleton, E. A., et al. (2017). Deletion of GLUT1 and GLUT3 Reveals Multiple Roles for Glucose Metabolism in Platelet and Megakaryocyte Function. Cell Rep. 20 (6), 1705. doi:10.1016/j.celrep.2017.10.086
Geng, C., Guo, Y., Wang, C., Cui, C., Han, W., Liao, D., et al. (2020). Comprehensive Evaluation of Lipopolysaccharide-Induced Changes in Rats Based on Metabolomics. J. Inflamm. Res. 13, 477–486. doi:10.2147/JIR.S266012
Ghanima, W., Gernsheimer, T., and Kuter, D. J. (2021). How I Treat Primary ITP in Adult Patients Who Are Unresponsive to or Dependent on Corticosteroid Treatment. Blood 137 (20), 2736–2744. doi:10.1182/blood.2021010968
Guo, L., Kuang, J., Zhuang, Y., Jiang, J., Shi, Y., Huang, C., et al. (2021). Serum Metabolomic Profiling to Reveal Potential Biomarkers for the Diagnosis of Fatty Liver Hemorrhagic Syndrome in Laying Hens. Front. Physiol. 12, 590638. doi:10.3389/fphys.2021.590638
Guo, Y., Wan, S., Han, M., Zhao, Y., Li, C., Cai, G., et al. (2020). Plasma Metabolomics Analysis Identifies Abnormal Energy, Lipid, and Amino Acid Metabolism in Abdominal Aortic Aneurysms. Med. Sci. Monit. 26, e926766. doi:10.12659/MSM.926766
Han, Q., Li, H., Jia, M., Wang, L., Zhao, Y., Zhang, M., et al. (2021). Age‐related Changes in Metabolites in Young Donor Livers and Old Recipient Sera after Liver Transplantation from Young to Old Rats. Aging Cell 20, e13425. doi:10.1111/acel.13425
Jonsson, P., Bruce, S. J., Moritz, T., Trygg, J., Sjöström, M., Plumb, R., et al. (2005). Extraction, Interpretation and Validation of Information for Comparing Samples in Metabolic LC/MS Data Sets. Analyst 130 (5), 701–707. doi:10.1039/b501890k
Kang, S. J., Cho, Y. R., Park, G. M., Ahn, J. M., Han, S. B., Lee, J. Y., et al. (2013). Predictors for Functionally Significant In-Stent Restenosis: an Integrated Analysis Using Coronary Angiography, IVUS, and Myocardial Perfusion Imaging. JACC Cardiovasc Imaging 6 (11), 1183–1190. doi:10.1016/j.jcmg.2013.09.006
Kohda, K., Kuga, T., Kogawa, K., Kanisawa, Y., Koike, K., Kuroiwa, G., et al. (2002). Effect of Helicobacter pylori Eradication on Platelet Recovery in Japanese Patients with Chronic Idiopathic Thrombocytopenic Purpura and Secondary Autoimmune Thrombocytopenic Purpura. Br. J. Haematol. 118 (2), 584–588. doi:10.1046/j.1365-2141.2002.03612.x
Kong, Y., Cao, X. N., Zhang, X. H., Shi, M. M., Lai, Y. Y., Wang, Y., et al. (2018). Atorvastatin Enhances Bone Marrow Endothelial Cell Function in Corticosteroid-Resistant Immune Thrombocytopenia Patients. Blood 131 (11), 1219–1233. doi:10.1182/blood-2017-09-807248
Laugsand, L. E., Janszky, I., Vatten, L. J., Dalen, H., Midthjell, K., Grill, V., et al. (2016). Autoimmune Diabetes in Adults and Risk of Myocardial Infarction: the HUNT Study in Norway. J. Intern Med. 280 (5), 518–531. doi:10.1111/joim.12530
Li, C. Y., Niu, M., Liu, Y. L., Tang, J. F., Chen, W., Qian, G., et al. (2020). Screening for Susceptibility-Related Factors and Biomarkers of Xianling Gubao Capsule-Induced Liver Injury. Front. Pharmacol. 11, 810. doi:10.3389/fphar.2020.00810
Li, J., Hua, M., Hu, X., Zhang, Y., Feng, Q., Qiu, J., et al. (2020). Dexamethasone Suppresses the Th17/1 Cell Polarization in the CD4+ T Cells from Patients with Primary Immune Thrombocytopenia. Thromb. Res. 190, 26–34. doi:10.1016/j.thromres.2020.04.004
Li, J., Ma, S., Shao, L., Ma, C., Gao, C., Zhang, X. H., et al. (2017). Inflammation-Related Gene Polymorphisms Associated with Primary Immune Thrombocytopenia. Front. Immunol. 8, 744. doi:10.3389/fimmu.2017.00744
Li, J., van der Wal, D. E., Zhu, G., Xu, M., Yougbare, I., Ma, L., et al. (2015). Desialylation Is a Mechanism of Fc-independent Platelet Clearance and a Therapeutic Target in Immune Thrombocytopenia. Nat. Commun. 6, 7737. doi:10.1038/ncomms8737
Li, Z. J., Liu, X. Q., Xu, J. Q., and Chu, X. X. (2015). Research Progress on Role of Abnormal Tryptophan Metabolism in Immune Thrombocytopenia. Zhongguo shi yan xue ye xue za zhi 23 (6), 1813–1816. doi:10.7534/j.issn.1009-2137.2015.06.051
McDonald, D. M., O'Kane, F., McConville, M., Devine, A. B., and McVeigh, G. E. (2013). Platelet Redox Balance in Diabetic Patients with Hypertension Improved by N-3 Fatty Acids. Diabetes Care 36 (4), 998–1005. doi:10.2337/dc12-0304
McGarrah, R. W., Crown, S. B., Zhang, G. F., Shah, S. H., and Newgard, C. B. (2018). Cardiovascular Metabolomics. Circ. Res. 122 (9), 1238–1258. doi:10.1161/CIRCRESAHA.117.311002
Mithoowani, S., Gregory-Miller, K., Goy, J., Miller, M. C., Wang, G., Noroozi, N., et al. (2016). High-dose Dexamethasone Compared with Prednisone for Previously Untreated Primary Immune Thrombocytopenia: a Systematic Review and Meta-Analysis. Lancet Haematol. 3 (10), e489–e496. doi:10.1016/s2352-3026(16)30109-0
Murgia, F., Svegliati, S., Poddighe, S., Lussu, M., Manzin, A., Spadoni, T., et al. (2018). Metabolomic Profile of Systemic Sclerosis Patients. Sci. Rep. 8 (1), 7626. doi:10.1038/s41598-018-25992-7
Neunert, C., Terrell, D. R., Arnold, D. M., Buchanan, G., Cines, D. B., Cooper, N., et al. (2019). American Society of Hematology 2019 Guidelines for Immune Thrombocytopenia. Blood Adv. 3 (23), 3829–3866. doi:10.1182/bloodadvances.2019000966
Neunert, C., Terrell, D. R., Arnold, D. M., Buchanan, G., Cines, D. B., Cooper, N., et al. (2020). Neunert C, Terrell DR, Arnold DM, Buchanan G, Cines DB, Cooper N, Cuker A, Despotovic JM, George JN, Grace RF, Kühne T, Kuter DJ, Lim W, McCrae KR, Pruitt B, Shimanek H, Vesely SK American Society of Hematology 2019 Guidelines for Immune Thrombocytopenia. Blood Adv. 2019;3(23):3829-3866. Blood Adv. 4 (2), 252. doi:10.1182/bloodadvances.2019001380
Neurauter, G., Schröcksnadel, K., Scholl-Bürgi, S., Sperner-Unterweger, B., Schubert, C., Ledochowski, M., et al. (2008). Chronic Immune Stimulation Correlates with Reduced Phenylalanine Turnover. Curr. Drug Metab. 9 (7), 622–627. doi:10.2174/138920008785821738
Nicholson, J. K., Lindon, J. C., and Holmes, E. (1999). 'Metabonomics': Understanding the Metabolic Responses of Living Systems to Pathophysiological Stimuli via Multivariate Statistical Analysis of Biological NMR Spectroscopic Data. Xenobiotica 29 (11), 1181–1189. doi:10.1080/004982599238047
Noonavath, R. N., Lakshmi, C. P., Dutta, T. K., and Kate, V. (2014). Helicobacter pylori Eradication in Patients with Chronic Immune Thrombocytopenic Purpura. World J. Gastroenterol. 20 (22), 6918–6923. doi:10.3748/wjg.v20.i22.6918
Qi, J., You, T., Pan, T., Wang, Q., Zhu, L., and Han, Y. (2017). Downregulation of Hypoxia-Inducible Factor-1α Contributes to Impaired Megakaryopoiesis in Immune Thrombocytopenia. Thromb. Haemost. 117 (10), 1875–1886. doi:10.1160/th17-03-0155
Schemmer, P., Zhong, Z., Galli, U., Wheeler, M. D., Xiangli, L., Bradford, B. U., et al. (2013). Glycine Reduces Platelet Aggregation. Amino Acids 44 (3), 925–931. doi:10.1007/s00726-012-1422-8
Siddiqui, N. F., Shabrani, N. C., Kale, V. P., and Limaye, L. S. (2011). Enhanced Generation of Megakaryocytes from Umbilical Cord Blood-Derived CD34(+) Cells Expanded in the Presence of Two Nutraceuticals, Docosahexanoic Acid and Arachidonic Acid, as Supplements to the Cytokine-Containing Medium. Cytotherapy 13 (1), 114–128. doi:10.3109/14653241003588858
Sinclair, E., Walton-Doyle, C., Sarkar, D., Hollywood, K. A., Milne, J., Lim, S. H., et al. (2021). Validating Differential Volatilome Profiles in Parkinson's Disease. ACS Cent. Sci. 7 (2), 300–306. doi:10.1021/acscentsci.0c01028
Song, Y., Hu, T., Gao, H., Zhai, J., Gong, J., Zhang, Y., et al. (2021). Altered Metabolic Profiles and Biomarkers Associated with Astragaloside IV-Mediated Protection against Cisplatin-Induced Acute Kidney Injury in Rats: An HPLC-TOF/MS-based Untargeted Metabolomics Study. Biochem. Pharmacol. 183, 114299. doi:10.1016/j.bcp.2020.114299
Souza, P. R., Marques, R. M., Gomez, E. A., Colas, R. A., De Matteis, R., Zak, A., et al. (2020). Enriched Marine Oil Supplements Increase Peripheral Blood Specialized Pro-resolving Mediators Concentrations and Reprogram Host Immune Responses: A Randomized Double-Blind Placebo-Controlled Study. Circ. Res. 126 (1), 75–90. doi:10.1161/CIRCRESAHA.119.315506
Sumner, L. W., Mendes, P., and Dixon, R. A. (2003). Plant Metabolomics: Large-Scale Phytochemistry in the Functional Genomics Era. Phytochemistry 62 (6), 817–836. doi:10.1016/s0031-9422(02)00708-2
Suzuki, H., Marshall, B. J., and Hibi, T. (2006). Overview: Helicobacter pylori and Extragastric Disease. Int. J. Hematol. 84 (4), 291–300. doi:10.1532/IJH97.06180
ten Hoor, F. (1980). Cardiovascular Effects of Dietary Linoleic Acid. Nutr. Metab. 24 (Suppl. 1), 162–180. doi:10.1159/000176379
Thrombosis and Hemostasis Group, Chinese Society of Hematology, Chinese Medical Association (2020). Chinese Guideline on the Diagnosis and Management of Adult Primary Immune Thrombocytopenia (Version 2020). Zhonghua Xue Ye Xue Za Zhi 41 (8), 617–623. doi:10.3760/cma.j.issn.0253-2727.2020.08.001
Thrombosis and Hemostasis Group, Hematology Society, Chinese Medical Association (2016). Consensus of Chinese Experts on Diagnosis and Treatment of Adult Primary Immune Thrombocytopenia (Version 2016). Zhonghua Xue Ye Xue Za Zhi 37 (2), 89–93. doi:10.3760/cma.j.issn.0253-2727.2016.02.001
Volani, C., Paglia, G., Smarason, S. V., Pramstaller, P. P., Demetz, E., Pfeifhofer-Obermair, C., et al. (2018). Metabolic Signature of Dietary Iron Overload in a Mouse Model. Cells 7 (12). doi:10.3390/cells7120264
Wijendran, V., and Hayes, K. C. (2004). Dietary N-6 and N-3 Fatty Acid Balance and Cardiovascular Health. Annu. Rev. Nutr. 24, 597–615. doi:10.1146/annurev.nutr.24.012003.132106
Wissmann, P., Geisler, S., Leblhuber, F., and Fuchs, D. (2013). Immune Activation in Patients with Alzheimer's Disease Is Associated with High Serum Phenylalanine Concentrations. J. Neurol. Sci. 329, 29–33. doi:10.1016/j.jns.2013.03.007
Xu, P., Zhao, Y., Yu, T., Yu, Y., Ni, X., Wang, H., et al. (2021). Atorvastatin Restores Imbalance of Cluster of Differentiation 4 (CD4) + T Cells in Immune Thrombocytopenia In Vivo and In Vitro. Br. J. Haematol. [Online ahead of print]. doi:10.1111/bjh.17938
Xu, Y., Jiang, H., Li, L., Chen, F., Liu, Y., Zhou, M., et al. (2020). Branched-Chain Amino Acid Catabolism Promotes Thrombosis Risk by Enhancing Tropomodulin-3 Propionylation in Platelets. Circulation 142 (1), 49–64. doi:10.1161/CIRCULATIONAHA.119.043581
Zhang, W., Zhou, F., Huang, H., Mao, Y., and Ye, D. (2020). Biomarker of Dietary Linoleic Acid and Risk for Stroke: A Systematic Review and Meta-Analysis. Nutrition 79-80, 110953. doi:10.1016/j.nut.2020.110953
Keywords: primary immune thrombocytopenia, gas chromatography tandem mass spectrometry, metabolomics, OPLS -DA, metabolomics pathway analysis
Citation: Zhang Z, Wu X, Zhou M, Qi J, Zhang R, Li X, Wang C, Ruan C and Han Y (2022) Plasma Metabolomics Identifies the Dysregulated Metabolic Profile of Primary Immune Thrombocytopenia (ITP) Based on GC-MS. Front. Pharmacol. 13:845275. doi: 10.3389/fphar.2022.845275
Received: 29 December 2021; Accepted: 06 May 2022;
Published: 24 May 2022.
Edited by:
Alastair George Stewart, The University of Melbourne, AustraliaReviewed by:
Meng L.V., Peking University People’s Hospital, ChinaYunfeng Cheng, Fudan University, China
Copyright © 2022 Zhang, Wu, Zhou, Qi, Zhang, Li, Wang, Ruan and Han. This is an open-access article distributed under the terms of the Creative Commons Attribution License (CC BY). The use, distribution or reproduction in other forums is permitted, provided the original author(s) and the copyright owner(s) are credited and that the original publication in this journal is cited, in accordance with accepted academic practice. No use, distribution or reproduction is permitted which does not comply with these terms.
*Correspondence: Yue Han, aGFueXVlQHN1ZGEuZWR1LmNu
†These authors have contributed equally to this work