- 1Programa de Investigación en Salud Global, Facultad de Ciencias de la Salud, Universidad Internacional SEK, Quito, Ecuador
- 2Latin American Network for the Implementation and Validation of Clinical Pharmacogenomics Guidelines (RELIVAF-CYTED), Madrid, Spain
- 3Escuela de Medicina, Facultad de Ciencias Médicas de la Salud y de la Vida, Universidad Internacional del Ecuador, Quito, Ecuador
- 4One Health Research Group, Faculty of Medicine, Universidad de Las Américas, Quito, Ecuador
- 5Institut für Humangenetik Lübeck, Universität zu Lübeck, Lübeck, Germany
- 6Integrated Research and Treatment Center, Center for Sepsis Control and Care (CSCC), Jena University Hospital, Jena, Germany
- 7Heidelberg Institute of Global Health, Faculty of Medicine, University of Heidelberg, Heidelberg, Germany
- 8Latin American Network for Cancer Research (LAN-CANCER), Lima, Peru
- 9Centro de Investigación para la Salud en América Latina (CISeAL), Pontificia Universidad Católica del Ecuador, Quito, Ecuador
- 10Grupo de Bio-Quimioinformática, Universidad de Las Américas, Quito, Ecuador
- 11BIOscience Research Group, Quito, Ecuador
- 12Facultade de Ciencias, Universidade da Coruña, A Coruña, Spain
- 13Instituto Nacional de Investigación en Salud Pública, Quito, Ecuador
- 14Biotechnology Engineering Career, Faculty of Life Sciences, Universidad Regional Amazónica Ikiam, Tena, Ecuador
- 15Faculty of Medicine, Universidad de Las Américas, Quito, Ecuador
- 16Laboratory of Chemical Carcinogenesis and Pharmacogenetics, Department of Basic-Clinical Oncology, Faculty of Medicine, University of Chile, Santiago, Chile
Background: It is imperative to identify drugs that allow treating symptoms of severe COVID-19. Respiratory failure is the main cause of death in severe COVID-19 patients, and the host inflammatory response at the lungs remains poorly understood.
Methods: Therefore, we retrieved data from post-mortem lungs from COVID-19 patients and performed in-depth in silico analyses of single-nucleus RNA sequencing data, inflammatory protein interactome network, and shortest pathways to physiological phenotypes to reveal potential therapeutic targets and drugs in advanced-stage COVID-19 clinical trials.
Results: Herein, we analyzed transcriptomics data of 719 inflammatory response genes across 19 cell types (116,313 nuclei) from lung autopsies. The functional enrichment analysis of the 233 significantly expressed genes showed that the most relevant biological annotations were inflammatory response, innate immune response, cytokine production, interferon production, macrophage activation, blood coagulation, NLRP3 inflammasome complex, and the TLR, JAK-STAT, NF-κB, TNF, oncostatin M signaling pathways. Subsequently, we identified 34 essential inflammatory proteins with both high-confidence protein interactions and shortest pathways to inflammation, cell death, glycolysis, and angiogenesis.
Conclusion: We propose three small molecules (baricitinib, eritoran, and montelukast) that can be considered for treating severe COVID-19 symptoms after being thoroughly evaluated in COVID-19 clinical trials.
Introduction
The severe acute respiratory syndrome coronavirus 2 (SARS-CoV-2), etiological agent of the coronavirus disease 2019 (COVID-19), has led to more than 425 million cases and more than 5.9 million deaths globally (WHO, 2021). Since the World Health Organization (WHO) declared the outbreak of COVID-19 as a pandemic, the novel coronavirus has been acquiring several mutations that not only increase its transmissibility rate but also mediates evasion of the host immune response and vaccination surveillance. Positive selection maintains amino-acid variants that increase virus fitness, whereas negative selection generally removes changes that reduce virus fitness (Lo Presti et al., 2020). For instance, some of the most predominant variants are capable of escaping monoclonal antibodies, partially eluding the polyclonal immune responses induced by previous infection or even allowing re-infections. It should be noted that recent improvements in immune escape are linked to mutations that alter the N-terminal domain (NTD) rather than the receptor-binding domain (RBD) of the spike (S) protein, where early and functionally important alterations predominated (Burioni and Topol, 2021). However, improved transmissibility, rather than immunoreaction or increased lethality, are considered as the main route for the virus to become fitter and more viable (Boehm et al., 2021). The variants that are being carefully monitored include B.1.1.7 (Alpha), B.1.351 (Beta), P.1 (Gamma), B.1.617.2 (Delta), and B.1.1.529 (Omicron) as variants of concern (VOCs); and C.37 (Lambda) and B.1.621 (Mu) as variants of interest (VOIs). It is expected that more variants will emerge over time that will need to be closely monitored, since they are a potential threat to public health (Burton and Topol, 2021; Harvey et al., 2021).
SARS-CoV-2 has the ability to infect human body cells through the angiotensin-converting enzyme 2 (ACE2) protein receptor (Mccallum et al., 2021). Lung homeostasis maintains a fine balance between tolerance mechanisms against non-pathogenic agents, pro-inflammatory immune system activation to fight off respiratory tract infections, and anti-inflammatory and pro-fibrotic processes to minimize tissular lesion and promote repair functions. Heterogeneous lung cell types mediate these complex mechanisms. The pulmonary alveolar epithelium is mainly composed of alveolar type I (AT1) and type II (AT2) cells, which allow the gas-exchange function and synthesize pulmonary surfactant factors, respectively (Wang et al., 2018). Airway epithelial cells are responsible for early pathogen recognition and production of pro-inflammatory cytokines and type I interferon (Yoo et al., 2013). Pulmonary endothelial cells have pleiotropic functions that range from gas interchange to regulating vascular tone and facilitating immune cell recruitment and diapedesis upon receiving pro-inflammatory stimuli (Niethamer et al., 2020). Mast cells are innate immune cells involved in defense and surveillance (Espinosa and Valitutti, 2018). Macrophages are key sentinel cells that detect pathogen invasion or tissue damage and initiate acute inflammatory processes (Biswas and Mantovani, 2014). Conventional dendritic cells (cDCs) are the bridge between the innate and adaptive immune responses as they constantly sample antigens from the airways and/or the infected lung tissue. Thus, cDCs migrate to T-cell areas of secondary lymphoid organs and present antigens to T lymphocytes thereby activating them (Schraml and Reise Sousa, 2015). Monocytes, subsets of leukocytes mostly originated from myeloid progenitors in the bone marrow, are able to differentiate into macrophages or dendritic cells in peripheral tissues (Murray, 2018). Natural killer (NK) cells eliminate infected cells by releasing perforin and granzymes or by death receptor signaling (FasL/Fas interactions and the subsequent induction of apoptosis) (Maucourant et al., 2020; van Eeden et al., 2020). NK cells can also release IFNγ upon activation, thereby contributing to naive T helper cell activation and differentiation (Culley, 2009). The CD4+ T helper cell population induce long-term cellular and humoral antigen-specific immunity (Peng et al., 2020). The cytotoxic CD8+ T cells are activated by specific pathogen or tumor-derived antigen presented on class I major histocompatibility complex (MHC I) molecules (Zhang and Bevan, 2011). Treg cells prevent autoimmune responses by suppressing the activation of conventional T-cells (Gladstone et al., 2020; Savage et al., 2020). B cells have a key role in the humoral adaptive immune response and are responsible for the production of antigen-specific immunoglobulins (Shuwa et al., 2021). Plasma blast cells are terminally differentiated populations of effector B cells that produce antibodies during initial exposure to a pathogen and mediate the protective effects of vaccination (Nutt et al., 2015). Fibroblasts produce cytokines and chemokines (Smith et al., 1997). Smooth muscle cells provide the main support for the vessel wall structure and regulate vascular tone to maintain intravascular pressure and tissue perfusion (Wang et al., 2015). Lastly, neuronal cells release neurotransmitters and neuropeptides that allow fast communication with immune cells, maintaining homeostasis and fighting infections (Blake et al., 2019).
Single-cell biology techniques has been widely implemented to study the cellular underpinnings of COVID-19 (López-Cortés et al., 2021). Scientific evidence through single-cell RNA sequencing (scRNA-seq) analyses of bronchoalveolar lavage fluid and blood from severe COVID-19 patients has revealed the effects of SARS-CoV-2 infection on immune responses and cytokine dysregulation (Wilk et al., 2020; Xu et al., 2020). Additionally, several autopsy studies examining formalin-fixed paraffin-embedded (FFPE) tissue sections extended our knowledge on virus organotropism. However, these studies were limited in their discovery potential due to tissue type, prolonged post-mortem intervals–affecting RNA quality–, and low-plex assays (Ackermann et al., 2020b; De Michele et al., 2020; Puelles et al., 2020). Since the respiratory failure is the main cause of death in severe COVID-19 patients and the host inflammatory response at the lung tissue level remains poorly understood, Melms et al. (2021) have published the single-cell lung atlas of lethal COVID-19 cases, and motivated by this study, we performed in-depth in silico analyses of snRNA-seq data; inflammatory protein-protein interactome (iPPI) network; functional enrichment analysis; and the shortest pathways to physiological phenotypes (cell death, inflammation, glycolysis, and angiogenesis) to reveal potential therapeutic targets and drugs in advanced-stage COVID-19 clinical trials.
Methods
Demographic Information of Donor Samples
The retrieved data from Melms et al. (2021) consisted of a cohort of 19 (100%) COVID-19 patients (12 males and 7 females) who died at a median age of 72 years. Of them, 13 (68%) were Hispanic or Latino, 7 (37%) had body mass index higher than 30.0 (obese and severely obese), and all cases had lungs, heart, kidneys or liver failure at time of death. On the other hand, the control cohort comprised 7 (100%) individuals (4 males and 3 females) with a median age of 70 years. Of them, 5 (71%) were white individuals, and 5 (71%) had body mass index between 25 and 29.9 considered as overweight. All information of donor samples is fully detailed in the Supplementary Table S1.
Gene/Protein Sets
We have retrieved a total of 719 inflammatory response genes/proteins from the David Bioinformatics Resource (https://david.ncifcrf.gov/) (Huang et al., 2009) using the (GO) term: 0006954 inflammatory response. We have also retrieved the 332 human proteins physically interacting SARS-CoV-2 proteins evidenced by Gordon et al (Gordon et al., 2020). Both sets will allow us to perform multi-omics analysis to identify potential therapeutic targets and drugs to treat severe COVID-19.
Single-Nucleus RNA Sequencing Data
We performed in-depth in silico analyses comparing the transcriptomics data of 719 genes involved in the inflammatory response between 9608 alveolar type I cells, 11341 alveolar type II cells, 7332 airway epithelial cells, 1845 B cells, 7586 CD4+ T cells, 3561 CD8+ T cells, 2814 cycling NK/T cells, 1083 dendritic cells, 5386 endothelial cells, 21472 fibroblast cells, 25960 macrophages, 1438 mast cells, 3464 monocytes, 2141 NK cells, 2017 neuronal cells, 5391 plasma cells, 1437 smooth muscle cells, 649 Treg cells, and 1788 other epithelial cells. The snRNA-seq database was taken from the ‘COVID-19 Studies’ section of the Single Cell Portal (https://singlecell.broadinstitute.org/single_cell/covid19), and the transcriptomics data of 116,313 nuclei was taken from ‘Columbia University/NYP COVID-19 Lung Atlas’ study (https://singlecell.broadinstitute.org/single_cell/study/SCP1219/columbia-university-nyp-covid-19-lung-atlas?cluster=UMAP&spatialGroups=--&annotation=cell_type_intermediate--group--study&subsample=all#study-summary) (Melms et al., 2021).
The criteria of the analysis of the lung transcriptomics data was the following: “all cells” as subsampling threshold, “cell type intermediate” as selected annotation, and ‘uniform manifold approximation and projection (UMAP)’ as load cluster. We adjusted the mRNA expression taking into account Z-scores ≤ -2 as underexpressed and Z-scores ≥ 2 as overexpressed. Additionally, we designed dot plots to visualize the percentage of cells expressing a certain gene, box plots to compare the mean Z-score across cell types, and scatter plots of 2D UMAPs to visualize the mean log normalized expression of a cluster of significantly expressed genes per subpopulation cell, and biological annotations across cell types.
Functional Enrichment Analysis
We performed the functional enrichment analysis to validate the correlation between significantly curated signatures of expressed genes and biological annotations related to COVID-19 (Reimand et al., 2016; Raudvere et al., 2019). The enrichment was calculated using g:GOSt version e101_eg48_p14_baf17f0 (https://biit.cs.ut.ee/gprofiler/gost) to obtain significant annotations (Benjamini-Hochberg FDR q-value < 0.001) related to GO: biological processes, Reactome signaling pathways, the Kyoto Encyclopedia of Genes and Genomes (KEGG) signaling pathways, and Wikipathways (Ogata et al., 1999; Reimand et al., 2016; Slenter et al., 2018; Raudvere et al., 2019; Jassal et al., 2020). The functional enrichment is evaluated using the well-proven cumulative hypergeometric test whose main source of information is the Ensembl database (Cunningham et al., 2018). Finally, the expression of genes involved in significant annotations was visualized in scatter plots of lung cells, and the significant terms related to lethal COVID-19 were manually curated.
Inflammatory Protein-Protein Interactome Network
The iPPI network with a highest confidence cutoff of 0.9 and zero node addition was designed between the human proteins involved in the pulmonary inflammatory response and the human proteins physically associated with SARS-CoV-2. To generate this network, we used the human proteome from the Cytoscape StringAPP (Doncheva et al., 2019), which imports protein interactions from the STRING database (Szklarczyk et al., 2015). The number of edges the node has in a network is represented by the degree centrality (López-Cortés et al., 2018; López-Cortés et al., 2020; López-Cortés et al., 2021), and it was calculated using the CytoNCA app (Tang et al., 2015). The network elements were organized through the organic layout producing a clear representation of complex networks, and the iPPI network was visualized through the Cytoscape software v.3.7.1 (Shannon et al., 2003). Finally, we ranked the inflammatory response proteins based on the highest confidence interactions with human-SARS-CoV-2 proteins. This network was validated by comparing their degree centralities with the inflammatory protein network by using the Mann-Whitney U test (p < 0.05).
Shortest Pathways to Physiological Phenotypes Related to COVID-19
CancerGenNet (https://signor.uniroma2.it/CancerGeneNet/) is a bioinformatic tool curated by SIGNOR (Perfetto et al., 2016) that based on experimental information, allows to infer likely pathways of causal interactions linking proteins to physiological phenotypes (Iannuccelli et al., 2020). The shortest distance scores or paths from proteins to cell death, inflammation, glycolysis, and angiogenesis were programmatically implemented using the shortest path function of igraph R package (Iannuccelli et al., 2020). We calculated the shortest distance scores of positive regulation from the inflammatory response proteins with the highest confidence interactions to the human-SARS-CoV-2 proteins to physiological phenotypes related to COVID-19. Lastly, once the essential inflammatory response proteins were identified, we performed a multiple comparison test by using the Bonferroni correction (p < 0.05, and a 95% confidence interval) to analyze the association of these therapeutic targets among the cell death, inflammation, glycolysis, and angiogenesis phenotypes.
Drugs Involved in Advanced-Stage COVID-19 Clinical Trials
The Open Targets Platform version 21.06 (https://www.targetvalidation.org) is a robust data integrator for visualization of potential drug targets involved in a variety of diseases including COVID-19 (Carvalho-Silva et al., 2019). This platform has developed the COVID-19 Target Prioritization Tool (https://covid19.opentargets.org/) that integrates molecular data from the ChEMBL database (Gaulton et al., 2017) to provide an evidence-based framework to support decision-making on potential drug targets for COVID-19. Lastly, this platform details phases III/IV clinical trials associated with therapeutic targets, modality, mechanism of action, phase, type of drug, and target class (Carvalho-Silva et al., 2019).
Statistical Analyses
The identification of inflammatory response genes with significant expression from 116,313 nuclei belonging to 19 different lung cell types were prioritized by using Z-scores and p-values. Therefore, genes with Z-score ≥ 2 and two-tailed p < 0.001 indicated significant over expression, and genes with Z-score ≤ -2 and two-tailed p < 0.001 indicated significant under expression. Additionally, box plots compared the mean Z-score across cell types, dot plots demonstrated the percentage of cells expressing a certain gene, and scatter plots of 2D UMAPs visualized the mean log normalized expression of significantly expressed genes per subpopulation of cells. The functional enrichment of the significantly curated signatures of expressed genes elucidated biological annotations related to lethal COVID-19. The enrichment analysis was performed by using the g:GOSt tool which determines the most significant GO: biological processes, Reactome signaling pathways, KEGG signaling pathways, and Wikipathways with Benjamini-Hochberg FDR q < 0.001. The iPPI network takes into account the highest confidence interactions (cutoff = 0.9). We validated the network of inflammatory proteins with high-confidence interactions with human proteins physically associated to SARS-CoV-2 proteins comparing the degree centrality of them with the inflammatory protein network by using the Mann-Whitney U test (p < 0.05). Lastly, we performed a multiple comparison test by using the Bonferroni correction (significant level of p < 0.05 and a 95% confidence interval) to analyze significant differences of the shortest distance scores among physiological phenotypes related to COVID-19: inflammation, cell death, glycolysis, and angiogenesis.
Results
Single-Nucleus RNA Sequencing Data
Single-nucleus biology is a powerful approach of omics medicine, needed to profile hard-to-dissociate tissues, that provides unprecented resolution to the cellular underpinnings of biological processes in order to find druggable targets for complex diseases (Slyper et al., 2020; Stephenson et al., 2021). Here, we identified 233 inflammatory response genes with significant expression in 116,313 nuclei belonging to 19 different lung cell types. Genes with the highest mean Z-score (3.26) and the most significant p-value (0.001) were identified in neural cells, followed by B cells (3.24; 0.001), mast cells (3.14; 0.002), fibroblast cells (3.0; 0.003), alveolar type II cells (2.96; 0.003), cycling NK/T cells (2.94; 0.003), endothelial cells (2.89; 0.004), macrophages (2.88; 0.004), airway epithelial cells (2.76; 0.006), alveolar type I cells (2.74; 0.006), NK cells (2.73; 0.006), dendritic cells (2.70; 0.007), smooth cells (2.68; 0.007), Treg cells (2.67; 0.008), plasma cells (2.62; 0.009), monocytes (2.47; 0.014), other epithelial cells (2.41; 0.016), CD4+ T cells (2.39; 0.017), and CD8+ T cells (2.24; 0.025) (Figure 1).
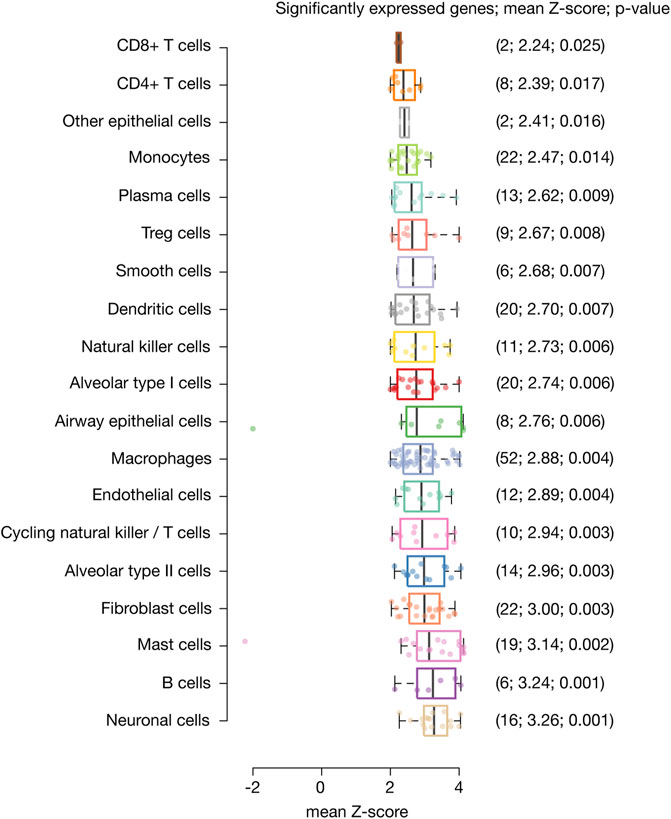
FIGURE 1. Significantly expressed genes across lung cell types. Box plots show lung cell types encompassed by significantly expressed genes, their mean Z-score and p-value. Neural cells were the cell type with the highest mean Z-score and most significant p-value, followed by B cells, mast cells, fibroblast cells, alveolar type II cells, cycling natural killer/T cells, endothelial cells, macrophages, airway epithelial cells, alveolar type I cells, natural killer cells, dendritic cells, smooth cells, Treg cells, plasma cells, monocytes, other epithelial cells, CD4+ T cells, and CD8+ T cells.
Figure 2 shows scatter plots of significant mean log normalized gene expression and dot plots of genes with the highest percentage of cells expressing per lung cell type. MECOM has the highest percentage of cells expressing in alveolar type I cells, LRRK2 in alveolar type II cells, ELF3 in airway epithelial cells, PXK in B cells, CAMK4 in CD4+ T cells, AOAH in CD8+ T cells, HMGB1 in cycling NK/T cells, CIITA in dendritic cells, RBPJ in macrophages, KIT in mast cells, SLC11A1 in monocytes cells, APP in neuronal cells, AOAH in NK cells, CALCRL in endothelial cells, RORA in fibroblasts, ASH1L in plasma cells, FN1 in smooth muscle cells, and SGMS1 in Treg cells. Lastly, the 26 inflammatory response genes significantly expressed in more that 50% of lung cells were ABR, ACER3, AOAH, APP, ASH1L, ATM, CALCRL, CAMK1D, CAMK4, CD163, CIITA, EGFR, FN1, HDAC9, IL18R1, IL1R1, KIT, LRRK2, LYN, MECOM, PRKCA, PRKCZ, RBPJ, RORA, SLC11A1, and SLIT2 (Supplementary Table S2).
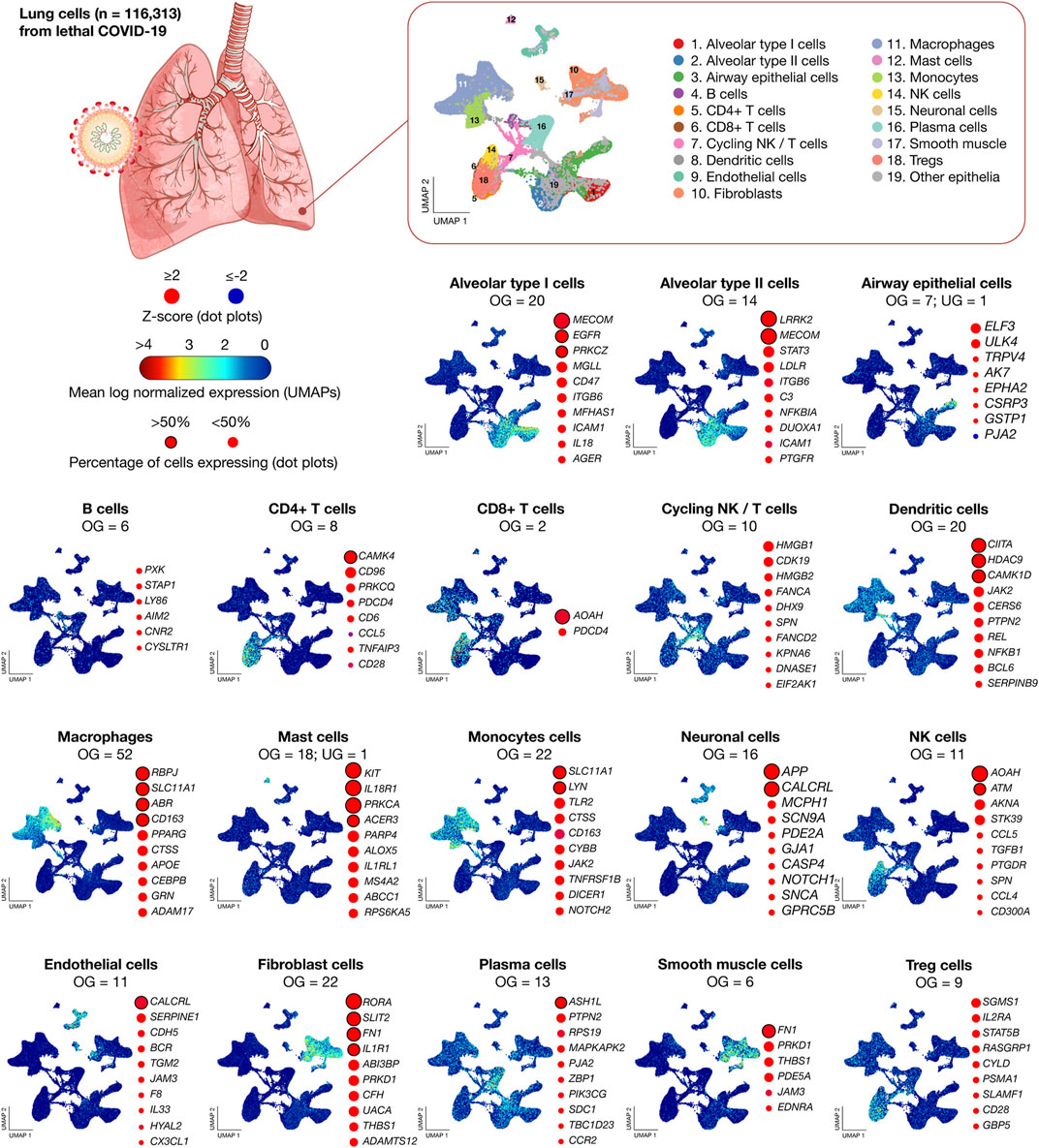
FIGURE 2. Transcriptomics data of 116,313 lung nuclei from 19 lethal COVID-19 patients. UMAPs show the mean log normalized expression of significantly expressed genes per lung cell type. Dot plots show the ranking of genes with the highest percentage of cells expressing. UMAP: uniform manifold approximation and projection for dimension reduction; NK, natural killer; OG, overexpressed genes; and UG, underexpressed genes.
Functional Enrichment Analysis
This enrichment was performed using g:GOSt to obtain significant biological processes and signaling pathways related to lethal COVID-19 (Benjamini-Hochberg FDR q < 0.001) (Reimand et al., 2016; Raudvere et al., 2019). Figure 3 shows scatter plots of significantly expressed genes (n = 233) in lung cells of lethal COVID-19 autopsies. After a manual curation of biological annotations, the most significant GO terms were inflammatory response (5.9 × 10−241), cytokine production (9.5 × 10−62), innate immune response (1.0 × 10−30), macrophage activation (1.1 × 10−29), toll-like receptor signaling pathway (3.8 × 10−15), type I and II interferon production (1.8 × 10−13), the Janus Kinase (JAK)/Signal Transducers and Activators of Transcription (STAT) signaling pathway (9.0 × 10−8), NF-κB signaling pathway (2.0 × 10−6), thymic stromal lymphopoietin (TSLP) (4.5 × 10−6), TNF signaling pathway (4.9 × 10−6), blood coagulation (5.6 × 10−6), oncostatin M signaling pathway (5.9 × 10−6), AGE-RAGE signaling pathway (5.9 × 10−6), IL-1 and megakaryocytes in obesity (6.8 × 10−6), and NLRP3 inflammasome complex (2.5 × 10−4) (Supplementary Table S3).
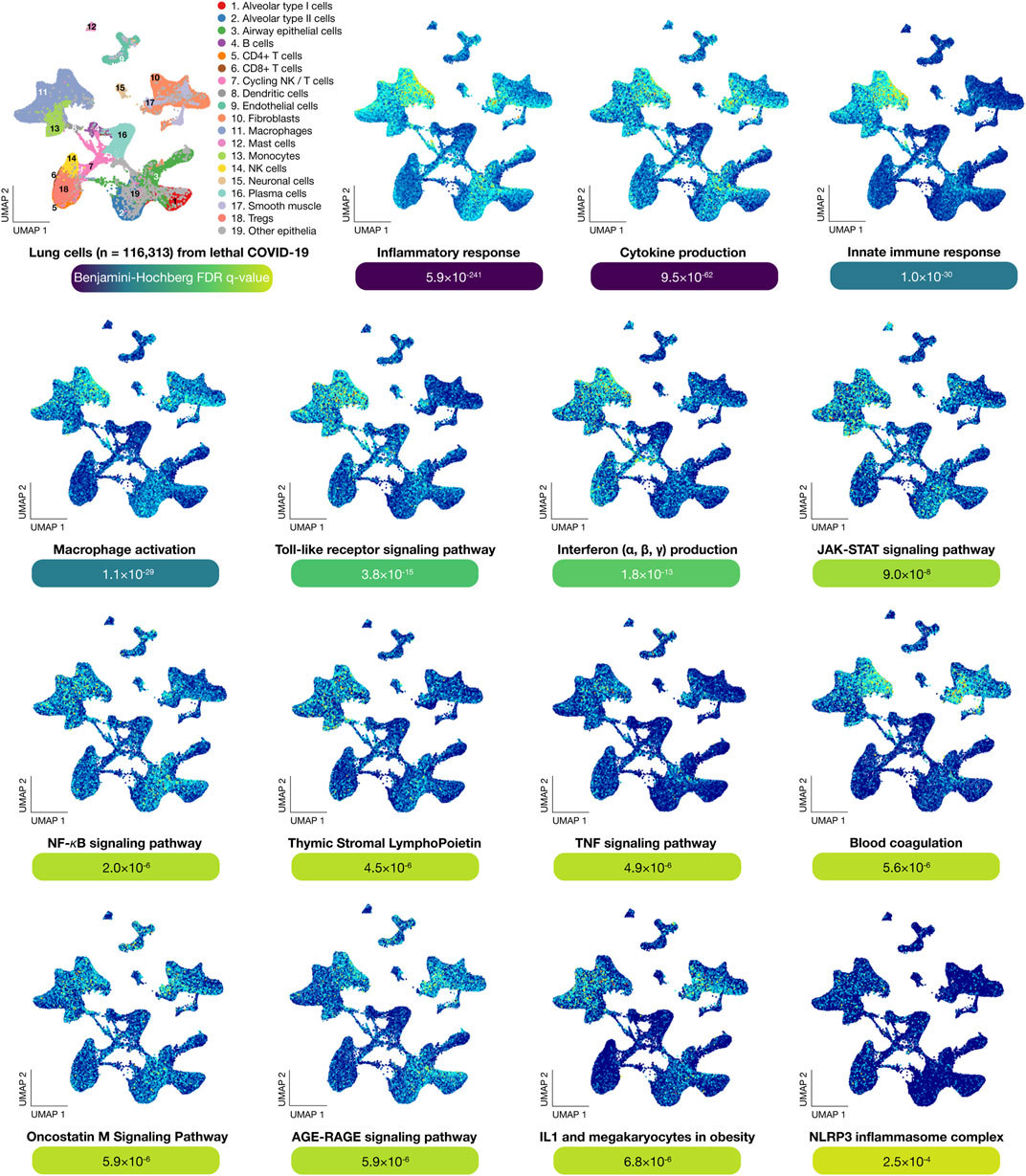
FIGURE 3. Functional enrichment analysis. UMAPs show the most significant genes per lung cell type involved in biological processes and signaling pathways. The most significant (Benjamini-Hochberg FDR q-value < 0.001) biological term was inflammatory response, followed by cytokine production, innate immune response, macrophage activation, Toll-like receptor signaling pathway, interferon production, JAK-STAT signaling pathway, NF-κB signaling pathway, thymic stromal lymphopoietin, TNF signaling pathway, blood coagulation, oncostatin M signaling pathway, AGE-RAGE signaling pathway, IL-1 and megakaryocytes in obesity, and NLRP3 inflammasome complex. UMAP: uniform manifold approximation and projection for dimension reduction.
Inflammatory Protein-Protein Interactome Network
We generated the iPPI network encompassing 265 nodes and 2052 edges (Figure 4). Of them, 159 pulmonary inflammatory response proteins had a mean of degree centrality of 8 and 108 human-SARS-CoV-2 proteins had a mean of degree centrality of 7.2. The top ten inflammatory response proteins with the highest degree centrality were APP (38), NFKB1 (36), STAT3 (34), C3 (31), ITGAM (29), FN1 (26), PTAFR (24), JAK2 (22), EGFR (20), and LYN (20). The top ten human-SARS-CoV-2 proteins with the highest degree centrality were GNB1(29), GNG5 (25), RHOA (23), ITGB1 (22), STOM (20), RAB14 (20), PRKAR2B (17), RAB8A (17), PRKACA (17), and ANO6 (16). Additionally, 111 pulmonary inflammatory response proteins had the highest confidence interactions (cutoff = 0.9) with human-SARS-CoV-2 proteins, and a mean of degree centrality of 10.1, being the top ten: C3 (11 interactions), FN1 (11), NFKB1 (10), RPS19 (10), CTSC (9), HSPD1 (9), APP (8), ITGAM (8), SNAP23 (8), and MAPK14 (7) (Supplementary Table S4). Lastly, the network of inflammatory proteins linked to human-SARS-CoV-2 proteins showed significantly higher degree centralities in comparison to the inflammatory protein network (Mann-Whitney U test, p < 0.05).
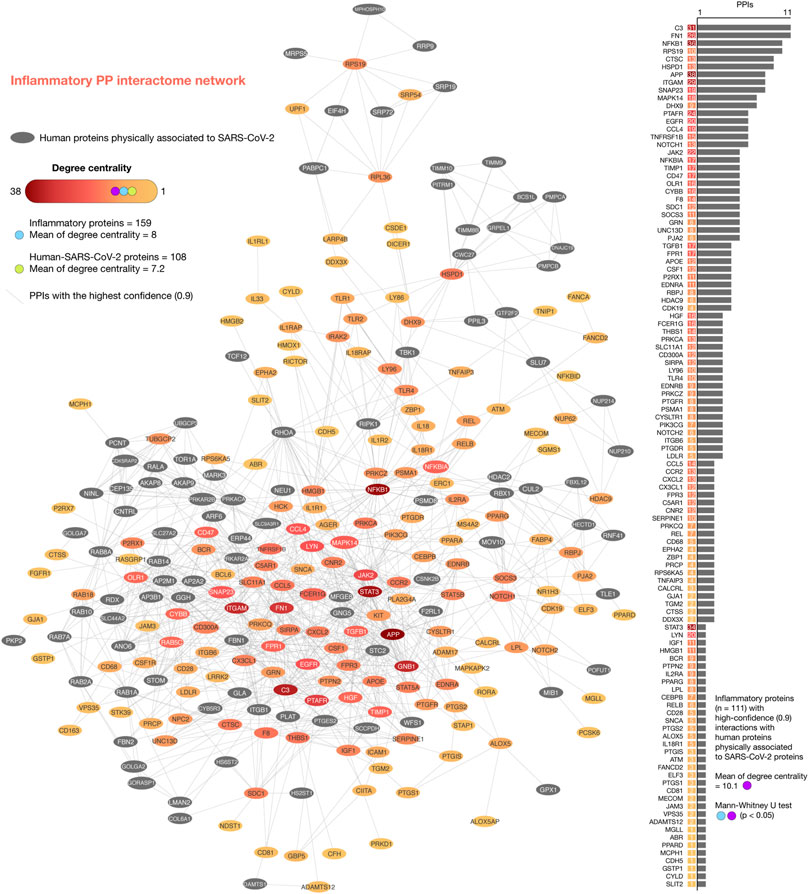
FIGURE 4. Inflammatory protein-protein interactome network. iPPI network was made up of 265 nodes and 2052 edges. Of them, 159 inflammatory response proteins had a mean of degree centrality of 8, and 108 human-SARS-CoV-2 proteins had a mean of degree centrality of 7.2. The top ten inflammatory response proteins with the highest degree centralities were APP, NFKB1, STAT3, C3, ITGAM, FN1, PTAFR, JAK2, EGFR, and LYN. The top ten human-SARS-CoV-2 proteins with the highest degree centralities were GNB1, GNG5, RHOA, ITGB1, STOM, RAB14, PRKAR2B, RAB8A, PRKACA, and ANO6. Additionally, the network of 111 inflammatory response proteins linked to human-SARS-CoV-2 proteins showed high-confidence interactions (cutoff = 0.9) and significantly higher degree centralities (Mann-Whitney U test, p < 0.05) in comparison to the complete iPPI network. The top ten proteins with the highest degree centralities were C3, FN1, NFKB1, RPS19, CTSC, HSPD1, APP, ITGAM, SNAP23, and MAPK14.
Shortest Pathways to Physiological Phenotypes Related to COVID-19
We analyzed the 111 pulmonary inflammatory response proteins with the highest confidence interactions (cutoff = 0.9) to human-SARS-CoV-2 proteins in order to find the shortest pathways to inflammation, cell death, angiogenesis, and glycolysis (Iannuccelli et al., 2020). Figure 5A shows box plots encompassing proteins with the shortest distance scores to physiological phenotypes related to COVID-19. Cell death was the phenotype with the shortest mean of distance score (2.82), followed by inflammation (3.06), glycolysis (3.12), and angiogenesis (3.79). Figure 5B shows a Venn diagram integrating inflammatory proteins with shortest pathways to biological phenotypes related to COVID-19. We found 34 essential inflammatory response proteins with shortest pathways simultaneously to inflammation, glycolysis, cell death, and angiogenesis (Supplementary Table S5). Figure 5C shows the ranking of inflammatory response proteins with the shortest distance score to cell death, inflammation, glycolysis, and angiogenesis. Interestingly, the Bonferroni correction demonstrated that these 34 essential proteins do not have significant distance scores between physiological phenotypes (p > 0.1). The top ten essential proteins with shortest pathways of positive regulation to cell death were ATM (1.20), NFKBIA (1.42), TNFRSF1B (1.64), APP (1.73), MAPK14 (1.73), PRKCZ (1.93), TLR4 (1.97), JAK2 (2.26), TGFB1 (2.35), and MECOM (2.36). The top ten essential proteins with shortest pathways of positive regulation to inflammation were PTGS2 (0.53), PRKCZ (1.39), NFKB1A (1.71), MAPK14 (1.92), TNFRSF1B (2.40), TLR4 (2.44), ATM (2.45), MECOM (2.57), PIK3CG (2.63), and EGFR (2.64). The top ten essential proteins with shortest pathways of positive regulation to glycolysis were ATM (1.67), CD28 (1.84), EGFR (1.98), TNFAIP3 (2.00), HGF (2.03), CYLD (2.09), PRKCZ (2.21), EPHA2 (2.25), JAK2 (2.27), and PIK3CG (2.34). The top ten essential proteins with shortest pathways of positive regulation to angiogenesis were TGFB1 (0.86), STAT3 (1.97), MAPK14 (1.98), EGFR (2.48), JAK2 (2.58), ATM (2.91), NFKBIA (2.96), PRKCZ (3.13), TLR4 (3.35), and PTAFR (3.46) (Supplementary Table S6). Lastly, Figure 6 details all shortest pathways and distance scores of positive regulation from the 34 essential proteins to the inflammation phenotype.
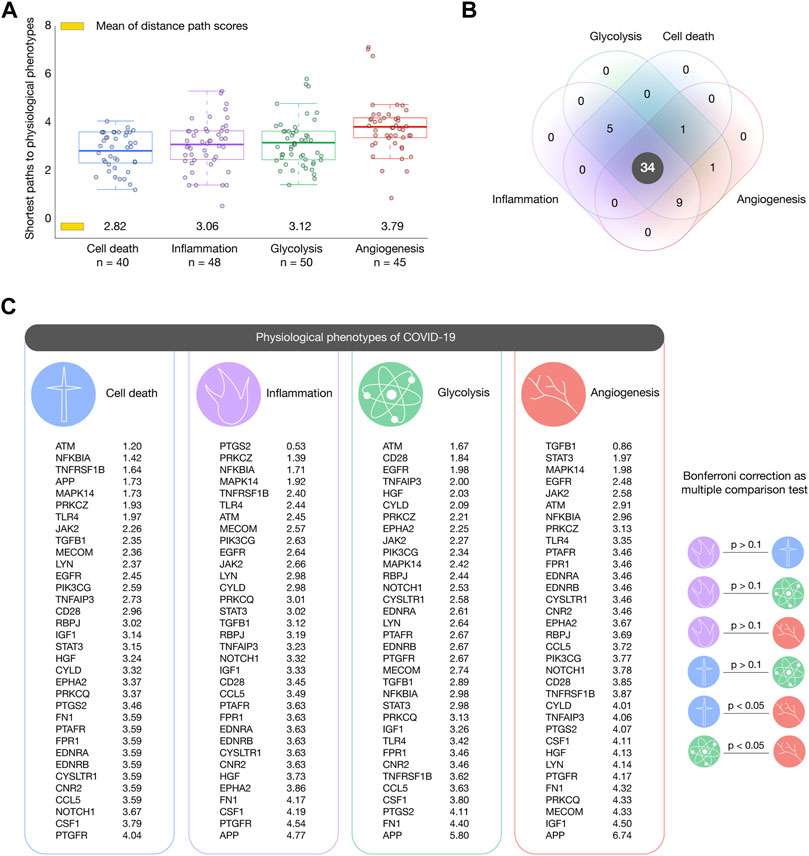
FIGURE 5. Shortest paths to cancer hallmark phenotypes. (A) Box plots encompassing inflammatory response proteins with the shortest mean of distance score per phenotype. Cell death was the phenotype with the shortest paths, followed by inflammation, glycolysis, and angiogenesis. (B) Venn diagram of inflammatory response proteins with shortest paths to hallmarks of cancer related to COVID-19. (C) Ranking of the most essential proteins with shortest paths to cell death, inflammation, glycolysis, and angiogenesis.
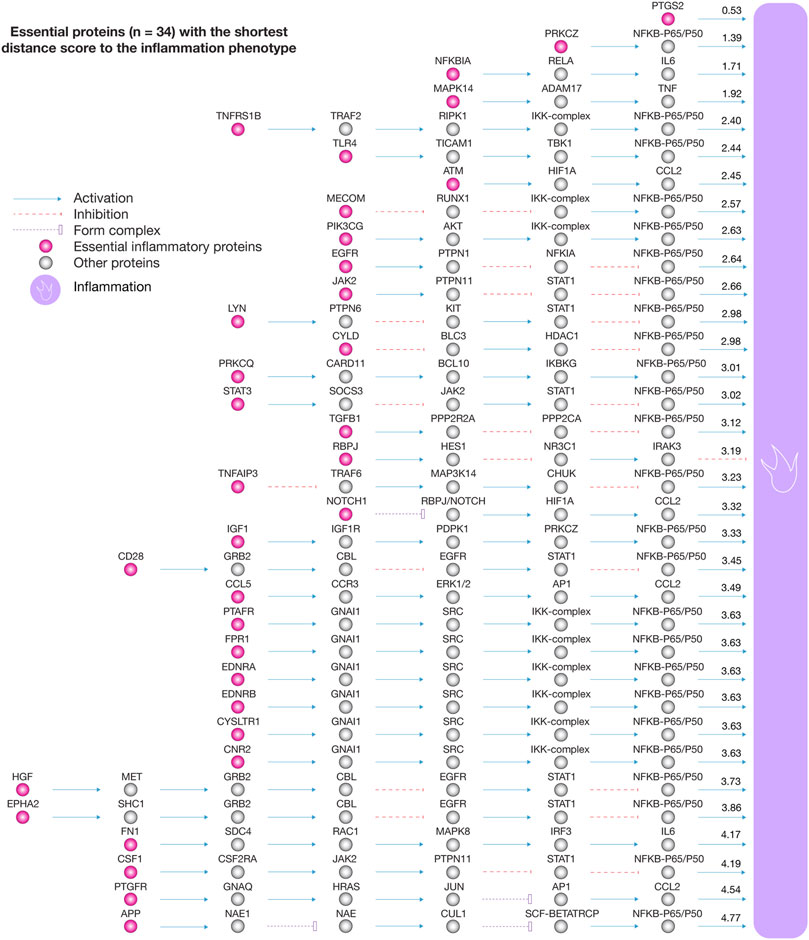
FIGURE 6. Essential proteins with the shortest distance score to the inflammation phenotype. The essential proteins with positive regulation to inflammation were PTGS2, PRKCZ, NFKBIA, MAPK14, TNFRSF1B, TLR4, ATM, MECOM, PIK3CG, EGFR, JAK2, LYN, CYLD, PRKCQ, STAT3, TGFB1, RBPJ, TNFAIP3, NOTCH1, IGF1, CD28, CCL5, PTAFR, FPR1, EDNRA, EDNRB, CYSLTR1, CNR2, HGF, EPHA2, FN1, CSF1, PTGFR, and APP.
Drugs Involved in Advanced-Stage COVID-19 Clinical Trials
According to the Open Targets Platform, Figure 7 details the current status of COVID-19 clinical trials regarding to our essential inflammatory proteins (Carvalho-Silva et al., 2019). There are 3 drugs (small molecules) that are being analyzed in advanced-stage clinical trials (phases III and IV) and act on 3 essential inflammatory proteins. Baricitinib is a tyrosine-protein kinase JAK2 inhibitor that acts on the JAK2 protein and it is being studied in 7 phase III clinical trials (NCT04970719, NCT04401579, NCT04640168, NCT04693026, NCT04421027, NCT04832880, and NCT04890626). Eritoran is a Toll-like receptor 4/MD-2 antagonist that acts on the TLR4 protein and it is being studied in one phase IV clinical trial (NCT02735707). Lastly, montelukast is a cysteinyl leukotriene receptor 1 antagonist that acts on the CYSLTR1 protein and it is being studied in 2 phase IV clinical trials (NCT04389411 and NCT04695704) (Supplementary Table S7).
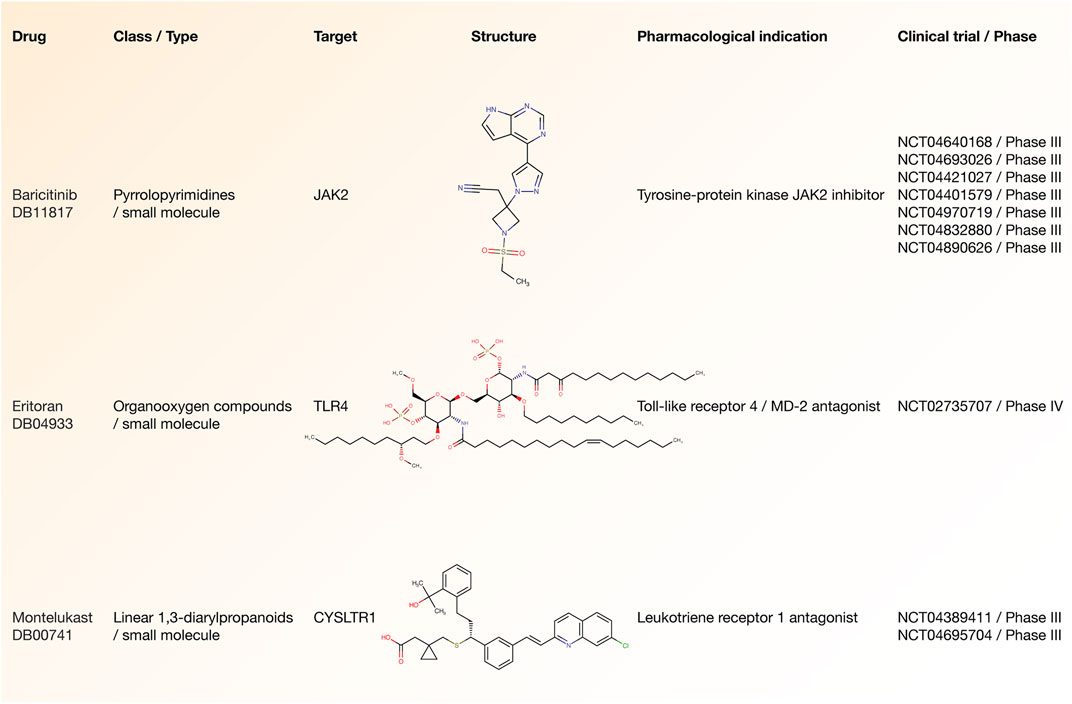
FIGURE 7. Drugs involved in advanced-stage COVID-19 clinical trials. Drug name, class type, druggable target, structure, pharmacological indication, and clinical trial number related to small molecules involved in phase III/IV clinical trials.
Discussion
A wide spectrum of clinical features has been discovered in severe COVID-19. For instance, dyspnea, acute respiratory distress syndrome (ARDS) (Montenegro et al., 2020), respiratory failure, lung edema, severe hypoxemia, cardiac arrhythmias, lymphopenia (Terpos et al., 2020), hyperferritinemia, rhabdomyolysis, intravascular coagulopathy (Fogarty et al., 2020), and pulmonary thromboembolism (Rotzinger et al., 2020). Nowadays, it is known that SARS-CoV-2 not only causes respiratory tract infection, but also skin, kidneys, blood, and central neural system pathologies (Delorey et al., 2021). Therefore, it is imperative to continuously review the physiopathological mechanisms of the SARS-CoV-2 infection and the clinical manifestations, especially with the appearance of new genomic variants (Ortiz-Prado et al., 2020).
Single-cell biology provides a high quality resolution to the cellular underpinnings of biological processes in order to find therapeutically actionable targets (Gawel et al., 2019; Stephenson et al., 2021). Melms et al have previously published the single-cell lung atlas of lethal COVID-19 (Melms et al., 2021). Motivated by this study, we performed in-depth in silico analyses comparing the transcriptional data of 719 inflammatory response genes across 19 lung cell types belonging to COVID-19 autopsies. Regarding lung cell types with significantly expressed inflammatory genes, neuronal cells had the highest mean Z-score and significant p-value (3.26; 0.001), followed by B-cells (3.24; 0.001), mast cells (3.14; 0.002), fibroblast cells (3.00; 0.003), alveolar type II cells (2.96; 0.003), cycling natural killer/T cells (2.94; 0.003), endothelial cells (2.89; 0.004), macrophages (2.88; 0.004), airway epithelial cells (2.76; 0.006), alveolar type I cells (2.74; 0.006), natural killer cells (2.73; 0.006), dendritic cells (2.70; 0.007), smooth cells (2.68; 0.007), Treg cells (2.67; 0.008), plasma cells (2.62; 0.009), monocytes (2.47; 0.014), CD4+ T cells (2.39; 0.017), and CD8+ T cells (2.24; 0.025). Subsequently, the functional enrichment analysis of the 233 significantly expressed inflammatory genes showed that the most significant biological annotations were inflammatory response, cytokine production, innate immune response, macrophage activation, TLR signaling pathway, type I and II interferon production, JAK-STAT signaling pathway, NF-κB signaling pathway, TNF signaling pathway, blood coagulation, oncostatin M signaling pathway, IL-1 and megakaryocytes in obesity, and the NLRP3 inflammasome complex (Table 1).
It is important to mention that mRNAs and proteins do not necessarily follow the same expression patterns due to post-transcriptional modifications. However, according to Buccitelli and Selbach, both types of data show a reasonable correlation to reveal exciting biology (Buccitelli and Selbach, 2020). In light of this measured and controlled correlation, we performed transcriptomics and proteomics data integration to reveal therapeutic targets and potential drugs to treat severe COVID-19. The novel coronavirus employs a suite of virulent proteins that interacts with human proteins to extensively rewire the flow of information causing COVID-19 (Kumar et al., 2020; López-Cortés et al., 2021). The human proteins physically associated with SARS-CoV-2 are the first line of host proteins (Gordon et al., 2020), which also interact with proteins involved in a wide spectrum of signaling pathways and biological processes within lung cells. In our study, the network of 111 inflammatory proteins with high-confidence interactions with human proteins physically associated to SARS-CoV-2 proteins showed significantly higher degree centralities in comparison to the inflammatory protein network (Mann-Whitney U test, p < 0.05). The top ten proteins with the highest degree centralities were C3, FN1, NFKB1, RPS19, CTSC, HSPD1, APP, ITGAM, SNAP23, and MAPK14.
Subsequently, we analyzed these 111 inflammatory response proteins to identify those with the shortest pathways to physiological phenotypes related to COVID-19. Inflammation is observed in patients with SARS-CoV-2 infection (Saini et al., 2020). The chronic inflammatory process causes cell death (Lee et al., 2020; Li et al., 2020), angiogenesis (Ackermann et al., 2020a), and during the peak of inflammation, immune cells preferentially use glycolysis as a source of energy (Ardestani and Azizi, 2021). These facts provide a biological rationale to analyze and prioritize the inflammatory response proteins with the shortest distance scores to these biological phenotypes. Interestingly, we identified 34 essential inflammatory response proteins highly associated with cell death, glycolysis, and angiogenesis showing not significant difference of distance scores among them (Bonferroni correction test, p > 0.1). These proteins were: PTGS2, PRKCZ, NFKBIA, MAPK14, TNFRSF1B, TLR4, ATM, MECOM, PIK3CG, EGFR, JAK2, LYN, CYLD, PRKCQ, STAT3, TGFB1, RBPJ, TNFAIP3, NOTCH1, IGF1, CD28, CCL5, PTAFR, FPR1, EDNRA, EDNRB, CYSLTR1, CNR2, HGF, EPHA2, FN1, CSF1, PTGFR, and APP.
According to Hanahan, inflammation, cell death, glycolysis, and angiogenesis are considered hallmarks of cancer (Hanahan, 2022). However, these biological phenotypes are also observed in COVID-19 patients. Post-mortem lung sections has revealed that pulmonary inflammatory responses may induce infiltration of inflammatory cells that trigger strong immune pathogenesis (Li et al., 2020). Mechanisms of inflammatory response and cell death are strongly linked during SARS-CoV-2 infection (Amaral and Bortoluci, 2020). The infection of lung cells activates caspase-8 to trigger cell death pathways, where apoptosis, pyroptosis, and necroptosis are involved. Interestingly, Vijayakumar et al. (2022) found that post-COVID-19 patients showed abnormal airway proteomes, with elevated concentration of proteins associated with apoptosis. Regarding glycolysis, scientific evidence showed that high glucose concentration and glycolysis are essential for SARS-CoV-2 replication, inflammatory response, and upregulation of ACE2 (Codo et al., 2020). Lastly, vasoconstriction and subsequent hypoxia, stimulate the formation of new blood vessels by promoting branching of pre-existing blood vessels and de novo angiogenesis that contributes to the already established systemic hypoxia (Huertas et al., 2020). This process together with the systemic hypoxia observed in severe COVID-19 patients cause a structural and functional reorganization of the pulmonary tissue. Interestingly, Ackermann et al. (2020b) found that in lungs from COVID-19 patients, the amount of new vessel growth was 2.7 times as high as that in lungs from patients with influenza.
Effective therapies are urgently required to treat symptoms and to reduce the mortality caused by SARS-CoV-2 infection. The drug repurposing strategy is the best option to rapidly identify a therapeutic that is effective at improving the clinical outcomes of severe COVID-19 disease (Tejera et al., 2020; López-Cortés et al., 2021). Hence, once we proposed the 34 essential inflammatory response proteins strongly linked to physiological phenotypes of COVID-19, we used the COVID-19 Target Prioritization Tool from the Open Target Platform (Carvalho-Silva et al., 2019) to identify 3 small molecules (baricitinib, eritoran, and montelukast) involved in phases III/IV clinical trials that acts on 3 therapeutic targets (JAK2, TLR4, and CYSLTR1).
Walz et al. (2021) concluded that Janus kinase-inhibitor treatment is significantly associated with positive clinical outcomes in terms of mortality, intensive care unit admission, and discharge. Baricitinib is a tyrosine-protein kinase JAK2 inhibitor mainly used for rheumatoid arthritis, and among its pharmacological properties it has an antiviral effect on the entry of a virus (Cantini et al., 2020). The WHO’s Guideline Development Group found moderate certainty evidence that baricitinib improved survival and reduced the need for ventilation, with no observed increase in adverse effects (Kmietowicz, 2022). Additionally, the WHO strongly recommends baricitinib for patients with severe COVID-19 in combination with corticosteroids (Rochwerg et al., 2020). At the moment, baricitinib is being analyzed in 7 phase III clinical trials with positive clinical outcomes, and has been approved by the WHO, the Food and Drug Administration (FDA) of the United States, and the National Institutes of Health (NIH) for emergent use in severe COVID-19 (COVID-19 Treatment Guidelines Panel, 2020; Kmietowicz, 2022). On the other hand, eritoran is a Toll-like receptor 4/MD-2 antagonist that downregulates the intracellular generation of pro-inflammatory cytokines IL-6 and TNF-alpha in human monocytes. Shirey et al. (2021) examined how antagonizing TLR4 signaling has been effective experimentally in ameliorating acute lung injury and lethal infection in challenge models triggered by acute lung injury-inducing viruses. At the moment, eritoran is being analyzed in 1 phase IV clinical trial with positive clinical outcomes. Lastly, montelukast is a cysteinyl leukotriene receptor 1 antagonist used as part of an asthma therapy regimen whose mechanism blocks the action of leukotriene D4 resulting in decreased inflammation of lung smooth muscle (Wishart et al., 2018). This leukotriene receptor antagonist is being analyzed in 2 phase III clinical trials with positive clinical outcomes.
Considering the enormous pressure that health systems are facing due to the COVID-19 pandemic, it is imperative to recognize the urgent need to diminish the gaps between research and the implementation of public health measures (Park et al., 2021). This is of particular interest to our research given that we acknowledge that clinical trials are essential in evidence-based medicine, and consequently, in the decision making process of public health policies and strategies. Clinical trial networks are essential to coordinate actions between clinical researchers and health practitioners, also promoting knowledge sharing, leadership, and cost-time reductions. In addition, it is critical to decentralize, improve, and increase clinical trials in low and middle-income countries.
The role of health research is fundamental in the response to COVID-19. Analyzing potential drug targets for COVID-19, especially the ones that can serve for severe cases, need an urgent and efficient development of well designed and managed clinical trials. Those which can provide potential interventions that help people to live longer, diminish long-term effects, manage pain and/or possible disabilities. In addition, the possible positive effects on the reduction of hospitalization cost, both at the individual level and in terms of possible savings for the national health system. As another study also mentioned, the potential and benefits of repositioning already approved drugs in COVID-19 clinical trials represent a “potential, prompt, cost-effective, and safe solutions for the public and global health problems, with a human-centered approach” (López-Cortés et al., 2021).
In conclusion, respiratory failure is the leading cause of death in severe COVID-19 and understanding the host inflammatory response at the lungs is imperative to identify actionable targets where specific drugs can work effectively. In our study we identified 34 essential inflammatory proteins with both high-confidence protein interactions and shortest pathways to physiological phenotypes related to COVID-19. Subsequently, we proposed 3 small molecules: baricitinib is a tyrosine-protein kinase JAK2 inhibitor that acts on the JAK2 protein; eritoran is a Toll-like receptor 4/MD-2 antagonist that acts on the TLR4 protein; and montelukast is a cysteinyl leukotriene receptor 1 antagonist that acts on the CYSLTR1 protein. These drugs can be considered for treating severe COVID-19 symptoms after being thoroughly evaluated in COVID-19 clinical trials.
Data Availability Statement
The datasets presented in this study can be found in online repositories. The names of the repository/repositories and accession number(s) can be found in the article/Supplementary Material.
Author Contributions
AL-C conceived the subject and the conceptualization of the study. AL-C and NK wrote the manuscript. SG, EO-P, VY, AV-G, AC, KS-R, AG-J, JB, LQ, and NK edited the manuscript and gave valuable scientific input. GE-G, JG-C, PG-R, AC-A, LP, DC-R, JB, IA-C, AP-V, AA-S, MR-M, AL-S, EA, AP-M, KN-J, AJ, AM, FA-E, and LF-G were involved in methodology and data curation. AL-C and NK supervised and did funding acquisition. Lastly, all authors have read and agreed to the published version of the manuscript.
Funding
Publication of this article was funded by Universidad de Las Américas (Quito-Ecuador), and partly funded by ANID grant COVID0789-Chile. Additionally, this work was supported by the Latin American Society of Pharmacogenomics and Personalized Medicine (SOLFAGEM).
Conflict of Interest
The authors declare that the research was conducted in the absence of any commercial or financial relationships that could be construed as a potential conflict of interest.
Publisher’s Note
All claims expressed in this article are solely those of the authors and do not necessarily represent those of their affiliated organizations, or those of the publisher, the editors and the reviewers. Any product that may be evaluated in this article, or claim that may be made by its manufacturer, is not guaranteed or endorsed by the publisher.
Supplementary Material
The Supplementary Material for this article can be found online at: https://www.frontiersin.org/articles/10.3389/fphar.2022.833174/full#supplementary-material
References
Ackermann, M., Mentzer, S. J., Kolb, M., and Jonigk, D. (2020a). Inflammation and Intussusceptive Angiogenesis in COVID-19: Everything in and Out of Flow. Eur. Respir. J. 56. doi:10.1183/13993003.03147-2020
Ackermann, M., Verleden, S. E., Kuehnel, M., Haverich, A., Welte, T., Laenger, F., et al. (2020b). Pulmonary Vascular Endothelialitis, Thrombosis, and Angiogenesis in Covid-19. N. Engl. J. Med. 383, 120–128. doi:10.1056/nejmoa2015432
Amaral, M. P., and Bortoluci, K. R. (2020). Caspase-8 and FADD: Where Cell Death and Inflammation Collide. Immunity 52, 890–892. doi:10.1016/j.immuni.2020.05.008
Ardestani, A., and Azizi, Z. (2021). Targeting Glucose Metabolism for Treatment of COVID-19. Signal. Transduct Target. Ther. 6, 112–2. doi:10.1038/s41392-021-00532-4
Bastard, P., Rosen, L. B., Zhang, Q., Michailidis, E., Hoffmann, H. H., Zhang, Y., et al. (2020). Autoantibodies against Type I IFNs in Patients with Life-Threatening COVID-19. Science 370. doi:10.1126/science.abd4585
Belančić, A., Kresović, A., and Rački, V. (2020). Potential Pathophysiological Mechanisms Leading to Increased COVID-19 Susceptibility and Severity in Obesity. Obes. Med. 19, 100259. doi:10.1016/j.obmed.2020.100259
Biswas, S., Thakur, V., Kaur, P., Khan, A., Kulshrestha, S., and Kumar, P. (2021). Blood Clots in COVID-19 Patients: Simplifying the Curious Mystery. Med. Hypotheses 146, 110371. doi:10.1016/j.mehy.2020.110371
Biswas, S. K., and Mantovani, A. (2014). Macrophages: Biology and Role in the Pathology of Diseases. 2014th Edn. New York: Springer. doi:10.1007/978-1-4939-1311-4
Blake, K. J., Jiang, X. R., and Chiu, I. M. (2019). Neuronal Regulation of Immunity in the Skin and Lungs. Trends Neurosci. 42, 537–551. doi:10.1016/j.tins.2019.05.005
Blasco, A., Coronado, M.-J., Hernández-Terciado, F., Martín, P., Royuela, A., Ramil, E., et al. (2021). Assessment of Neutrophil Extracellular Traps in Coronary Thrombus of a Case Series of Patients with COVID-19 and Myocardial Infarction. JAMA Cardiol. 6, 469. doi:10.1001/jamacardio.2020.7308
Boechat, J. L., Chora, I., Morais, A., and Delgado, L. (2021). The Immune Response to SARS-CoV-2 and COVID-19 Immunopathology - Current Perspectives. Pulmonology 27, 423–437. doi:10.1016/j.pulmoe.2021.03.008
Boehm, E., Kronig, I., Neher, R. A., Eckerle, I., Vetter, P., Kaiser, L., et al. (2021). Novel SARS-CoV-2 Variants: the Pandemics within the Pandemic. Clin. Microbiol. Infect. 27, 1109–1117. doi:10.1016/j.cmi.2021.05.022
Buccitelli, C., and Selbach, M. (2020). mRNAs, Proteins and the Emerging Principles of Gene Expression Control. Nat. Rev. Genet. 21, 630–644. doi:10.1038/s41576-020-0258-4
Burioni, R., and Topol, E. J. (2021). Has SARS-CoV-2 Reached Peak Fitness? Nat. Med. 27, 1323–1324. doi:10.1038/s41591-021-01421-7
Burton, D. R., and Topol, E. J. (2021). Toward Superhuman SARS-CoV-2 Immunity? Nat. Med. 27, 5–6. doi:10.1038/s41591-020-01180-x
Campbell, R. A., Boilard, E., and Rondina, M. T. (2021). Is There a Role for the ACE2 Receptor in SARS‐CoV‐2 Interactions with Platelets? J. Thromb. Haemost. 19, 46–50. doi:10.1111/jth.15156
Cantini, F., Goletti, D., Petrone, L., Najafi Fard, S., Niccoli, L., and Foti, R. (2020). Immune Therapy, or Antiviral Therapy, or Both for COVID-19: A Systematic Review. Drugs 80, 1929–1946. doi:10.1007/s40265-020-01421-w
Carvalho-Silva, D., Pierleoni, A., Pignatelli, M., Ong, C., Fumis, L., Karamanis, N., et al. (2019). Open Targets Platform: New Developments and Updates Two Years on. Nucleic Acids Res. 47, D1056–D1065. doi:10.1093/nar/gky1133
Catanzaro, M., Fagiani, F., Racchi, M., Corsini, E., Govoni, S., and Lanni, C. (2020). Immune Response in COVID-19: Addressing a Pharmacological challenge by Targeting Pathways Triggered by SARS-CoV-2. Signal. Transduct Target. Ther. 5, 84. doi:10.1038/S41392-020-0191-1
Channappanavar, R., Fehr, A. R., Zheng, J., Wohlford-Lenane, C., Abrahante, J. E., Mack, M., et al. (2019). IFN-I Response Timing Relative to Virus Replication Determines MERS Coronavirus Infection Outcomes. J. Clin. Invest. 129, 3625–3639. doi:10.1172/JCI126363
Codo, A. C., Davanzo, G. G., Monteiro, L. B., de Souza, G. F., Muraro, S. P., Virgilio-da-Silva, J. V., et al. (2020). Elevated Glucose Levels Favor SARS-CoV-2 Infection and Monocyte Response through a HIF-1α/Glycolysis-dependent Axis. Cell Metab 32, 498–499. doi:10.1016/j.cmet.2020.07.00710.1016/j.cmet.2020.07.015
COVID-19 Treatment Guidelines Panel (2020). Coronavirus Disease 2019 (COVID-19) Treatment Guidelines. Washington, DC: National Institute of Health. Available at: https://covid19treatmentguidelines.nih.gov/.
Culley, F. J. (2009). Natural Killer Cells in Infection and Inflammation of the Lung. Immunology 128, 151–163. doi:10.1111/j.1365-2567.2009.03167.x
Cunningham, F., Achuthan, P., Akanni, W., Allen, J., Amode, M. R., Armean, I. M., et al. (2018). Ensembl 2019. Nucleic Acids Res. 47, D745–D751. doi:10.1093/nar/gky1113
De Michele, S., Sun, Y., Yilmaz, M. M., Katsyv, I., Salvatore, M., Dzierba, A. L., et al. (2020). Forty Postmortem Examinations in COVID-19 Patients. Am. J. Clin. Pathol. 154, 748–760. doi:10.1093/ajcp/aqaa156
Delorey, T. M., Ziegler, C. G. K., Heimberg, G., Normand, R., Yang, Y., Segerstolpe, Å., et al. (2021). COVID-19 Tissue Atlases Reveal SARS-CoV-2 Pathology and Cellular Targets. Nature 595, 107–113. doi:10.1038/s41586-021-03570-8
Diamond, M. S., and Kanneganti, T. D. (2022). Innate Immunity: the First Line of Defense against SARS-CoV-2. Nat. Immunol. 23, 165–176. doi:10.1038/s41590-021-01091-0
Doncheva, N. T., Morris, J. H., Gorodkin, J., and Jensen, L. J. (2019). Cytoscape StringApp: Network Analysis and Visualization of Proteomics Data. J. Proteome Res. 18, 623–632. doi:10.1021/acs.jproteome.8b00702
Espinosa, E., and Valitutti, S. (2018). New Roles and Controls of Mast Cells. Curr. Opin. Immunol. 50, 39–47. doi:10.1016/j.coi.2017.10.012
Fogarty, H., Townsend, L., Ni Cheallaigh, C., Bergin, C., Martin‐Loeches, I., Browne, P., et al. (2020). COVID19 Coagulopathy in Caucasian Patients. Br. J. Haematol. 189, 1044–1049. doi:10.1111/bjh.16749
Gaulton, A., Hersey, A., Nowotka, M., Bento, A. P., Chambers, J., Mendez, D., et al. (2017). The ChEMBL Database in 2017. Nucleic Acids Res. 45, D945–D954. doi:10.1093/nar/gkw1074
Gawel, D. R., Serra-Musach, J., Lilja, S., Aagesen, J., Arenas, A., Asking, B., et al. (2019). A Validated Single-Cell-Based Strategy to Identify Diagnostic and Therapeutic Targets in Complex Diseases. Genome Med. 11, 47. doi:10.1186/s13073-019-0657-3
Gladstone, D. E., Kim, B. S., Mooney, K., Karaba, A. H., and D'Alessio, F. R. (2020). Regulatory T Cells for Treating Patients with COVID-19 and Acute Respiratory Distress Syndrome: Two Case Reports. Ann. Intern. Med. 173, 852–853. doi:10.7326/L20-0681
Gordon, D. E., Jang, G. M., Bouhaddou, M., Xu, J., Obernier, K., White, K. M., et al. (2020). A SARS-CoV-2 Protein Interaction Map Reveals Targets for Drug Repurposing. Nature 583, 459. doi:10.1038/s41586-020-2286-9
Hanahan, D. (2022). Hallmarks of Cancer: New Dimensions. Cancer Discov. 12, 31–46. doi:10.1158/2159-8290.CD-21-1059
Hariharan, A., Hakeem, A. R., Radhakrishnan, S., Reddy, M. S., and Rela, M. (2021). The Role and Therapeutic Potential of NF-Kappa-B Pathway in Severe COVID-19 Patients. Inflammopharmacology 29, 91–100. doi:10.1007/s10787-020-00773-9
Harvey, W. T., Carabelli, A. M., Jackson, B., Gupta, R. K., Thomson, E. C., Harrison, E. M., et al. (2021). SARS-CoV-2 Variants, Spike Mutations and Immune Escape. Nat. Rev. Microbiol. 19, 409–424. doi:10.1038/s41579-021-00573-0
Hintzen, C., Haan, C., Tuckermann, J. P., Heinrich, P. C., and Hermanns, H. M. (2008). Oncostatin M-Induced and Constitutive Activation of the JAK2/STAT5/CIS Pathway Suppresses CCL1, but Not CCL7 and CCL8, Chemokine Expression. J. Immunol. 181, 7341–7349. doi:10.4049/jimmunol.181.10.7341
Huang, da. W., Sherman, B. T., and Lempicki, R. A. (2009). Systematic and Integrative Analysis of Large Gene Lists Using DAVID Bioinformatics Resources. Nat. Protoc. 4, 44–57. doi:10.1038/nprot.2008.211
Huertas, A., Montani, D., Savale, L., Pichon, J., Tu, L., Parent, F., et al. (2020). Endothelial Cell Dysfunction: A Major Player in SARS-CoV-2 Infection (COVID-19)? Eur. Respir. J. 56. doi:10.1183/13993003.01634-2020
Iannuccelli, M., Micarelli, E., Surdo, P. L., Palma, A., Perfetto, L., Rozzo, I., et al. (2020). CancerGeneNet: Linking Driver Genes to Cancer Hallmarks. Nucleic Acids Res. 48, D416–D421. doi:10.1093/nar/gkz871
Jassal, B., Matthews, L., Viteri, G., Gong, C., Lorente, P., Fabregat, A., et al. (2020). The Reactome Pathway Knowledgebase. Nucleic Acids Res. 48, D498. doi:10.1093/nar/gkz1031
Kmietowicz, Z. (2022). Covid-19: WHO Recommends Baricitinib and Sotrovimab to Treat Patients. Bmj 376, o97. doi:10.1136/bmj.o97
Kumar, N., Mishra, B., Mehmood, A., Athar, M., and Shahid, M. (2020). Integrative Network Biology Framework Elucidates Molecular Mechanisms of SARS-CoV-2 Pathogenesis. iScience 23, 101526. doi:10.1016/j.isci.2020.101526
Lamontagne, F., Agoritsas, T., Macdonald, H., Leo, Y. S., Diaz, J., Agarwal, A., et al. (2020). A Living WHO Guideline on Drugs for Covid-19. BMJ 370, m3379. doi:10.1136/bmj.m3379
Lee, S., Channappanavar, R., and Kanneganti, T. D. (2020). Coronaviruses: Innate Immunity, Inflammasome Activation, Inflammatory Cell Death, and Cytokines. Trends Immunol. 41, 1083–1099. doi:10.1016/j.it.2020.10.005
Li, S., Zhang, Y., Guan, Z., Li, H., Ye, M., Chen, X., et al. (2020). SARS-CoV-2 Triggers Inflammatory Responses and Cell Death through Caspase-8 Activation. Signal. Transduct Target. Ther. 5, 235. doi:10.1038/s41392-020-00334-0
Lo Presti, A., Rezza, G., and Stefanelli, P. (2020). Selective Pressure on SARS-CoV-2 Protein Coding Genes and Glycosylation Site Prediction. Heliyon 6, e05001. doi:10.1016/j.heliyon.2020.e05001
Lopez, L., Sang, P. C., Tian, Y., and Sang, Y. (2020). Dysregulated Interferon Response Underlying Severe Covid-19. Viruses 12, 1433. doi:10.3390/v12121433
López-Cortés, A., Guevara-Ramírez, P., Kyriakidis, N. C., Barba-Ostria, C., León Cáceres, Á., Guerrero, S., et al. (2021). In Silico Analyses of Immune System Protein Interactome Network, Single-Cell RNA Sequencing of Human Tissues, and Artificial Neural Networks Reveal Potential Therapeutic Targets for Drug Repurposing against COVID-19. Front. Pharmacol. 12, 1–24. doi:10.3389/fphar.2021.598925
López-Cortés, A., Paz-y-Miño, C., Cabrera-Andrade, A., Barigye, S. J., Munteanu, C. R., González-Díaz, H., et al. (2018). Gene Prioritization, Communality Analysis, Networking and Metabolic Integrated Pathway to Better Understand Breast Cancer Pathogenesis. Sci. Rep. 8, 16679. doi:10.1038/s41598-018-35149-1
López-Cortés, A., Paz-y-Miño, C., Guerrero, S., Cabrera-Andrade, A., Barigye, S. J., Munteanu, C. R., et al. (2020). OncoOmics Approaches to Reveal Essential Genes in Breast Cancer: a Panoramic View from Pathogenesis to Precision Medicine. Sci. Rep. 10, 5285. doi:10.1038/s41598-020-62279-2
Luo, W., Li, Y. X., Jiang, L. J., Chen, Q., Wang, T., and Ye, D. W. (2020). Targeting JAK-STAT Signaling to Control Cytokine Release Syndrome in COVID-19. Trends Pharmacol. Sci. 41, 531–543. doi:10.1016/J.TIPS.2020.06.007
Maucourant, C., Filipovic, I., Ponzetta, A., Aleman, S., Cornillet, M., Hertwig, L., et al. (2020). Natural Killer Cell Immunotypes Related to COVID-19 Disease Severity. Sci. Immunol. 5, eabd6832. doi:10.1126/SCIIMMUNOL.ABD6832
Mccallum, M., Mccallum, M., Czudnochowski, N., Rosen, L. E., Zepeda, S. K., Bowen, J. E., et al. (2021). Structural Basis of SARS-CoV-2 Omicron Immune Evasion and Receptor Engagement. Science 8652, 1–14. doi:10.1126/science.abn8652
Melms, J. C., Biermann, J., Huang, H., Wang, Y., Nair, A., Tagore, S., et al. (2021). A Molecular Single-Cell Lung Atlas of Lethal COVID-19. Nature 595, 114–119. doi:10.1038/s41586-021-03569-1
Michalakis, K., and Ilias, I. (2020). SARS-CoV-2 Infection and Obesity: Common Inflammatory and Metabolic Aspects. Diabetes Metab. Syndr. 14, 469–471. doi:10.1016/j.dsx.2020.04.033
Montenegro, F., Unigarro, L., Paredes, G., Moya, T., Romero, A., Torres, L., et al. (2020). Acute Respiratory Distress Syndrome (ARDS) Caused by the Novel Coronavirus Disease (COVID-19): a Practical Comprehensive Literature Review. Expert Rev. Respir. Med. 15, 183–195. doi:10.1080/17476348.2020.1820329
Murray, P. J. (2018). Immune Regulation by Monocytes. Semin. Immunol. 35, 12–18. doi:10.1016/j.smim.2017.12.005
Mustafa, M. I., Abdelmoneim, A. H., Mahmoud, E. M., and Makhawi, A. M. (2020). Cytokine Storm in COVID-19 Patients, its Impact on Organs and Potential Treatment by QTY Code-Designed Detergent-free Chemokine Receptors. Mediators Inflamm. 2020, 1–7. doi:10.1155/2020/8198963
Niethamer, T. K., Stabler, C. T., Leach, J. P., Zepp, J. A., Morley, M. P., Babu, A., et al. (2020). Defining the Role of Pulmonary Endothelial Cell Heterogeneity in the Response to Acute Lung Injury. eLife 9, e53072. doi:10.7554/eLife.53072
Nutt, S. L., Hodgkin, P. D., Tarlinton, D. M., and Corcoran, L. M. (2015). The Generation of Antibody-Secreting Plasma Cells. Nat. Rev. Immunol. 15, 160–171. doi:10.1038/nri3795
Ogata, H., Goto, S., Sato, K., Fujibuchi, W., Bono, H., and Kanehisa, M. (1999). KEGG: Kyoto Encyclopedia of Genes and Genomes. Nucleic Acids Res. 27, 29–34. doi:10.1093/nar/27.1.29
Ortiz-Prado, E., Simbaña-Rivera, K., Gómez- Barreno, L., Rubio-Neira, M., Guaman, L. P., Kyriakidis, N. C., et al. (2020). Clinical, Molecular, and Epidemiological Characterization of the SARS-CoV-2 Virus and the Coronavirus Disease 2019 (COVID-19), a Comprehensive Literature Review. Diagn. Microbiol. Infect. Dis. 98, 115094. doi:10.1016/j.diagmicrobio.2020.115094
Otsuka, R., and Seino, K.-i. (2020). Macrophage Activation Syndrome and COVID-19. Inflamm. Regener 40. doi:10.1186/s41232-020-00131-w
Park, J. J. H., Mogg, R., Smith, G. E., Nakimuli-Mpungu, E., Jehan, F., Rayner, C. R., et al. (2021). How COVID-19 Has Fundamentally Changed Clinical Research in Global Health. Lancet Glob. Health 9. doi:10.1016/S2214-109X(20)30542-8
Peng, X., Ouyang, J., Isnard, S., Lin, J., Fombuena, B., Zhu, B., et al. (2020). Sharing CD4+ T Cell Loss: When COVID-19 and HIV Collide on Immune System. Front. Immunol. 11, 596631. doi:10.3389/fimmu.2020.596631
Perfetto, L., Briganti, L., Calderone, A., Cerquone Perpetuini, A., Iannuccelli, M., Langone, F., et al. (2016). SIGNOR: A Database of Causal Relationships between Biological Entities. Nucleic Acids Res. 44, D548–D554. doi:10.1093/nar/gkv1048
Puelles, V. G., Lütgehetmann, M., Lindenmeyer, M. T., Sperhake, J. P., Wong, M. N., Allweiss, L., et al. (2020). Multiorgan and Renal Tropism of SARS-CoV-2. N. Engl. J. Med. 383, 590–592. doi:10.1056/nejmc2011400
Qian, Z., Paul, B., Effort, C. H. G., Aurélie, C., and Jean-Laurent, C. (2022). Human Genetic and Immunological Determinants of Critical COVID-19 Pneumonia. Nature. doi:10.1038/s41586-022-04447-0
Raudvere, U., Kolberg, L., Kuzmin, I., Arak, T., Adler, P., Peterson, H., et al. (2019). g:Profiler: a Web Server for Functional Enrichment Analysis and Conversions of Gene Lists (2019 Update). Nucleic Acids Res. 47, W191–W198. doi:10.1093/nar/gkz369
Reimand, J., Arak, T., Adler, P., Kolberg, L., Reisberg, S., Peterson, H., et al. (2016). g:Profiler-a Web Server for Functional Interpretation of Gene Lists (2016 Update). Nucleic Acids Res. 44, W83–W89. doi:10.1093/nar/gkw199
Rodrigues, T. S., Sá, K. S. G. de., Ishimoto, A. Y., Becerra, A., Oliveira, S., Almeida, L., et al. (2020). Inflammasome Activation in COVID-19 Patients. medRxiv. doi:10.1101/2020.08.05.20168872
Rojas, P., and Sarmiento, M. (2021). JAK/STAT Pathway Inhibition May Be a Promising Therapy for COVID-19-Related Hyperinflammation in Hematologic Patients. Acta Haematol. 144, 1–5. doi:10.1159/000510179
Rotzinger, D. C., Beigelman-Aubry, C., von Garnier, C., and Qanadli, S. D. (2020). Pulmonary Embolism in Patients with COVID-19: Time to Change the Paradigm of Computed Tomography. Thromb. Res. 190, 58–59. doi:10.1016/j.thromres.2020.04.011
Rowaiye, A. B., Okpalefe, O. A., Onuh Adejoke, O., Ogidigo, J. O., Hannah Oladipo, O., Ogu, A. C., et al. (2021). Attenuating the Effects of Novel COVID-19 (SARS-CoV-2) Infection-Induced Cytokine Storm and the Implications. J. Inflamm. Res. 14, 1487–1510. doi:10.2147/JIR.S301784
Saini, K. S., Lanza, C., Romano, M., de Azambuja, E., Cortes, J., de las Heras, B., et al. (2020). Repurposing Anticancer Drugs for COVID-19-Induced Inflammation, Immune Dysfunction, and Coagulopathy. Br. J. Cancer 123, 694–697. doi:10.1038/s41416-020-0948-x
Sanchez-Infantes, D., and Stephens, J. M. (2020). Adipocyte Oncostatin Receptor Regulates Adipose Tissue Homeostasis and Inflammation. Front. Immunol. 11, 612013. doi:10.3389/fimmu.2020.612013
Satarker, S., Tom, A. A., Shaji, R. A., Alosious, A., Luvis, M., and Nampoothiri, M. (2020). JAK-STAT Pathway Inhibition and Their Implications in COVID-19 Therapy. Postgrad. Med. 133, 1. doi:10.1080/00325481.2020.1855921
Savage, P. A., Klawon, D. E. J., and Miller, C. H. (2020). Regulatory T Cell Development. Annu. Rev. Immunol. 38, 421–453. doi:10.1146/annurev-immunol-100219-020937
Schraml, B. U., and Reise Sousa, C. (2015). Defining Dendritic Cells. Curr. Opin. Immunol. 32, 13–20. doi:10.1016/j.coi.2014.11.001
Setiadi, H., El Banayosy, A. M., Koerner, M. M., Maybauer, M. O., Harper, M. D., Horstmanshof, D. A., et al. (2020). Oncostatin M as a Biomarker to Predict the Outcome of V-V ECMO Supported Patients with Acute Pulmonary Failure. J. Heart Lung Transplant. 39, S390. doi:10.1016/j.healun.2020.01.513
Shannon, P., Markiel, A., Ozier, O., Baliga, N. S., Wang, J. T., Ramage, D., et al. (2003). Cytoscape: a Software Environment for Integrated Models of Biomolecular Interaction Networks. Genome Res. 13, 2498–2504. doi:10.1101/gr.1239303
Shi, C. S., Nabar, N. R., Huang, N. N., and Kehrl, J. H. (20192019). SARS-coronavirus Open Reading Frame-8b Triggers Intracellular Stress Pathways and Activates NLRP3 Inflammasomes. Cell Death Discov 5, 101–112. doi:10.1038/s41420-019-0181-7
Shirey, K. A., Blanco, J. C. G., and Vogel, S. N. (2021). Targeting TLR4 Signaling to Blunt Viral-Mediated Acute Lung Injury. Front. Immunol. 12, 705080. doi:10.3389/fimmu.2021.705080
Shuwa, H. A., Shaw, T. N., Knight, S. B., Wemyss, K., McClure, F. A., Pearmain, L., et al. (2021). Alterations in T and B Cell Function Persist in Convalescent COVID-19 Patients. Med (N Y) 2, 720–e4. doi:10.1016/j.medj.2021.03.013
Slenter, D. N., Kutmon, M., Hanspers, K., Riutta, A., Windsor, J., Nunes, N., et al. (2018). WikiPathways: A Multifaceted Pathway Database Bridging Metabolomics to Other Omics Research. Nucleic Acids Res. 46, D661–D667. doi:10.1093/nar/gkx1064
Slyper, M., Porter, C. B. M., Ashenberg, O., Waldman, J., Drokhlyansky, E., Wakiro, I., et al. (2020). A Single-Cell and Single-Nucleus RNA-Seq Toolbox for Fresh and Frozen Human Tumors. Nat. Med. 26, 792–802. doi:10.1038/s41591-020-0844-1
Smith, R. S., Smith, T. J., Blieden, T. M., and Phipps, R. P. (1997). Fibroblasts as sentinel Cells. Synthesis of Chemokines and Regulation of Inflammation. Am. J. Pathol. 151, 317–322.
Stephenson, E., Reynolds, G., Botting, R. A., Calero-Nieto, F. J., Morgan, M. D., Tuong, Z. K., et al. (2021). Single-cell Multi-Omics Analysis of the Immune Response in COVID-19. Nat. Med. 27, 904–916. doi:10.1038/s41591-021-01329-2
Szklarczyk, D., Franceschini, A., Wyder, S., Forslund, K., Heller, D., Huerta-Cepas, J., et al. (2015). STRING V10: Protein-Protein Interaction Networks, Integrated over the Tree of Life. Nucleic Acids Res. 43, D447–D452. doi:10.1093/nar/gku1003
Tang, N., Li, D., Wang, X., and Sun, Z. (2020). Abnormal Coagulation Parameters Are Associated with Poor Prognosis in Patients with Novel Coronavirus Pneumonia. J. Thromb. Haemost. 18, 844–847. doi:10.1111/jth.14768
Tang, Y., Li, M., Wang, J., Pan, Y., and Wu, F.-X. (2015). CytoNCA: A Cytoscape Plugin for Centrality Analysis and Evaluation of Protein Interaction Networks. BioSystems 127, 67–72. doi:10.1016/j.biosystems.2014.11.005
Tejera, E., Munteanu, C. R., López-Cortés, A., Cabrera-Andrade, A., and Pérez-Castillo, Y. (2020). Drugs Repurposing Using QSAR, Docking and Molecular Dynamics for Possible Inhibitors of the SARS-CoV-2 Mpro Protease. Molecules 25, 5172. doi:10.3390/molecules25215172
Terpos, E., Ntanasis‐Stathopoulos, I., Elalamy, I., Kastritis, E., Sergentanis, T. N., Politou, M., et al. (2020). Hematological Findings and Complications of COVID ‐19. Am. J. Hematol. 95, 834–847. doi:10.1002/ajh.25829
van Eeden, C., Khan, L., Osman, M. S., and Cohen Tervaert, J. W. (2020). Natural Killer Cell Dysfunction and its Role in Covid-19. Int. J. Mol. Sci. 21, 6351. doi:10.3390/ijms21176351
Vijayakumar, B., Boustani, K., Ogger, P. P., Papadaki, A., Tonkin, J., Orton, C. M., et al. (2022). Immuno-proteomic Profiling Reveals Aberrant Immune Cell Regulation in the Airways of Individuals with Ongoing post-COVID-19 Respiratory Disease. Immunity 55, 542. doi:10.1016/j.immuni.2022.01.017
Vora, S. M., Lieberman, J., and Wu, H. (2021). Inflammasome Activation at the Crux of Severe COVID-19. Nat. Rev. Immunol. 21, 694–703. doi:10.1038/s41577-021-00588-x
Walz, L., Cohen, A. J., Rebaza, A. P., Vanchieri, J., Slade, M. D., Dela Cruz, C. S., et al. (2021). JAK-inhibitor and Type I Interferon Ability to Produce Favorable Clinical Outcomes in COVID-19 Patients: a Systematic Review and Meta-Analysis. BMC Infect. Dis. 21, 47. doi:10.1186/s12879-020-05730-z
Wang, G., Jacquet, L., Karamariti, E., and Xu, Q. (2015). Origin and Differentiation of Vascular Smooth Muscle Cells. J. Physiol. 593, 3013–3030. doi:10.1113/JP270033
Wang, Y., Tang, Z., Huang, H., Li, J., Wang, Z., Yu, Y., et al. (2018). Pulmonary Alveolar Type I Cell Population Consists of Two Distinct Subtypes that Differ in Cell Fate. Proc. Natl. Acad. Sci. U S A. 115, 2407–2412. doi:10.1073/pnas.1719474115
Wilk, A. J., Rustagi, A., Zhao, N. Q., Roque, J., Martínez-Colón, G. J., McKechnie, J. L., et al. (2020). A Single-Cell Atlas of the Peripheral Immune Response in Patients with Severe COVID-19. Nat. Med. 26, 1070–1076. doi:10.1038/s41591-020-0944-y
Wishart, D. S., Feunang, Y. D., Guo, A. C., Lo, E. J., Marcu, A., Grant, J. R., et al. (2018). DrugBank 5.0: a Major Update to the DrugBank Database for 2018. Nucleic Acids Res. 46, D1074–D1082. doi:10.1093/nar/gkx1037
Xu, G., Qi, F., Li, H., Yang, Q., Wang, H., Wang, X., et al. (2020). The Differential Immune Responses to COVID-19 in Peripheral and Lung Revealed by Single-Cell RNA Sequencing. Cell Discov 6, 73. doi:10.1038/s41421-020-00225-2
Yoo, J. K., Kim, T. S., Hufford, M. M., and Braciale, T. J. (2013). Viral Infection of the Lung: Host Response and Sequelae. J. Allergy Clin. Immunol. 132, 1263–1277. doi:10.1016/j.jaci.2013.06.006
Zhang, N., and Bevan, M. J. (2011). CD8(+) T Cells: Foot Soldiers of the Immune System. Immunity 35, 161–168. doi:10.1016/j.immuni.2011.07.010
Keywords: pulmonary inflammatory response, clinical trials, drugs, lethal COVID-19, single nucleus RNA sequencing
Citation: López-Cortés A, Guerrero S, Ortiz-Prado E, Yumiceba V, Vera-Guapi A, León Cáceres Á, Simbaña-Rivera K, Gómez-Jaramillo AM, Echeverría-Garcés G, García-Cárdenas JM, Guevara-Ramírez P, Cabrera-Andrade A, Puig San Andrés L, Cevallos-Robalino D, Bautista J, Armendáriz-Castillo I, Pérez-Villa A, Abad-Sojos A, Ramos-Medina MJ, León-Sosa A, Abarca E, Pérez-Meza ÁA, Nieto-Jaramillo K, Jácome AV, Morillo A, Arias-Erazo F, Fuenmayor-González L, Quiñones LA and Kyriakidis NC (2022) Pulmonary Inflammatory Response in Lethal COVID-19 Reveals Potential Therapeutic Targets and Drugs in Phases III/IV Clinical Trials. Front. Pharmacol. 13:833174. doi: 10.3389/fphar.2022.833174
Received: 10 December 2021; Accepted: 07 March 2022;
Published: 29 March 2022.
Edited by:
Siddappa N. Byrareddy, University of Nebraska Medical Center, United StatesReviewed by:
Ravi Misra, University of Rochester, United StatesSameh S. M. Soliman, University of Sharjah, United Arab Emirates
Copyright © 2022 López-Cortés, Guerrero, Ortiz-Prado, Yumiceba, Vera-Guapi, León Cáceres, Simbaña-Rivera, Gómez-Jaramillo, Echeverría-Garcés, García-Cárdenas, Guevara-Ramírez, Cabrera-Andrade, Puig San Andrés, Cevallos-Robalino, Bautista, Armendáriz-Castillo, Pérez-Villa, Abad-Sojos, Ramos-Medina, León-Sosa, Abarca, Pérez-Meza, Nieto-Jaramillo, Jácome, Morillo, Arias-Erazo, Fuenmayor-González, Quiñones and Kyriakidis. This is an open-access article distributed under the terms of the Creative Commons Attribution License (CC BY). The use, distribution or reproduction in other forums is permitted, provided the original author(s) and the copyright owner(s) are credited and that the original publication in this journal is cited, in accordance with accepted academic practice. No use, distribution or reproduction is permitted which does not comply with these terms.
*Correspondence: Andrés López-Cortés, aalc84@gmail.com; Nikolaos C. Kyriakidis , nickyriakidis82@yahoo.gr