- Department of Pharmacology and Therapeutics, Molecular and Integrative Biology, Institute of Systems, University of Liverpool, Liverpool, United Kingdom
The aim of the study was to apply Physiologically-Based Pharmacokinetic (PBPK) modelling to predict the effect of liver disease (LD) on the pharmacokinetics (PK) of dexamethasone (DEX) in the treatment of COVID-19. A whole-body PBPK model was created to simulate 100 adult individuals aged 18–60 years. Physiological changes (e.g., plasma protein concentration, liver size, CP450 expression, hepatic blood flow) and portal vein shunt were incorporated into the LD model. The changes were implemented by using the Child-Pugh (CP) classification system. DEX was qualified using clinical data in healthy adults for both oral (PO) and intravenous (IV) administrations and similarly propranolol (PRO) and midazolam (MDZ) were qualified with PO and IV clinical data in healthy and LD adults. The qualified model was subsequently used to simulate a 6 mg PO and 20 mg IV dose of DEX in patients with varying degrees of LD, with and without shunting. The PBPK model was successfully qualified across DEX, MDZ and PRO. In contrast to healthy adults, the simulated systemic clearance of DEX decreased (35%–60%) and the plasma concentrations increased (170%–400%) in patients with LD. Moreover, at higher doses of DEX, the AUC ratio between healthy/LD individuals remained comparable to lower doses. The exposure of DEX in different stages of LD was predicted through PBPK modelling, providing a rational framework to predict PK in complex clinical scenarios related to COVID-19. Model simulations suggest dose adjustments of DEX in LD patients are not necessary considering the low dose administered in the COVID-19 protocol.
Introduction
Chronic liver disease (LD) is prevalent in 3–8% of patients suffering with COVID-19. (Qiu et al., 2020). Chronic LD has been associated with a higher rate of mortality in COVID-19 patients and can affect the drug distribution of several treatments. (Kovalic et al., 2020). End stage chronic liver disease leads to cirrhosis which is characterised by the replacement of injured tissue with a collagenous scar and is accompanied by a loss of functional hepatocytes as well as a distortion in hepatic vasculature (Schuppan and Afdhal, 2008; Johnson et al., 2010). The severity of liver disease can be classified using the CP score A, B, and C and is based on physiological and biological parameters. (Edginton and Willmann, 2008). As the severity of liver disease increases, the distortion of the hepatic vasculature may lead to portal hypertension and in turn portacaval shunting. Shunting can significantly increase the bioavailability of a drug due to a decrease in first pass metabolism and this effect can be particularly relevant for drugs with a high first-pass extraction (Rodighiero, 1999).
DEX is a corticosteroid traditionally used in a wide range of conditions such as rheumatic or endocrine disorders for its anti-inflammatory and immunosuppressant effects (DrugBank, 2021a), and can be used as a treatment for patients with severe COVID-19 disease (Matthay and Thompson, 2020). DEX has a relatively low hepatic extraction and is metabolised by the cytochrome P450 (CYP) enzymatic system, primarily by the CYP3A4 isoform, of which it is also a weak-moderate inducer (Pilla Reddy et al., 2018; Ke and Milad, 2019; DrugBank, 2021a). A previous study showed reduced DEX clearance (CL) and prolonged half-life (t1/2) in individuals with LD compared with that in healthy subjects (Kawai et al., 1985). However, DEX plasma concentrations, different forms of administration and detailed description of the individuals included in the study (e.g., LD severity according to CP score) are lacking.
PBPK modelling is a simulation approach with multiple applications and which is accepted by regulatory agencies primarily to evaluate enzyme-based drug-drug interactions (60% of submissions between 2008 and 2017) (Grimstein et al., 2019). PBPK can account for changes in absorption, metabolism, distribution, and elimination (ADME), through the integration of in vitro data using in vitro-in vivo extrapolation (IVIVE) techniques for the prediction of PK in a cohort of virtual patients. The effect of liver disease on pharmacokinetics can be simulated considering a number of physiological changes as functional liver size, CYP450 expression, plasma protein binding and hepatic blood flow (Edginton and Willmann, 2008; Johnson et al., 2010). The aim of this study was to use PBPK modelling to predict DEX PKs for the treatment of COVID-19 in patients with liver impairment.
Materials and Methods
A whole body PBPK model constructed using Simbiology v. 5.8.2, a product of MATLAB® R2019a v. 9.6.0 (MathWorks, Natick, MA, United States 2013), was used to generate a cohort of 100 individuals aged 18–60 years (50% female and 50% male). The following assumptions were made during simulations: 1) instant and uniform drug distribution (well-stirred model) across each compartment (tissue/organ); 2) no reabsorption of the drug from the colon; and 3) drug distribution was limited by blood flow. No ethical approval was required as results for this investigation were generated virtually.
Anatomy and Physiology
The body mass index (BMI), body surface area (BSA), height and weight of the individuals were generated as described by de la Grandmaison et al., 2001 These values were used to allometrically calculate organs and tissues volumes through equations described by Bosgra et al., 2012 Density was used to calculate organs and tissues weights as described by Brown et al., 1997 Blood flows were calculated using percentage regional blood flows of the cardiac output described by the Environmental Protection Agency (EPA) (Birnbaum et al., 1994). To represent a LD population, changes to the physiological and biochemical parameters in the healthy adult model were made according to Johnson et al., 2010 The parameters and corresponding values are summarised in Table 1.
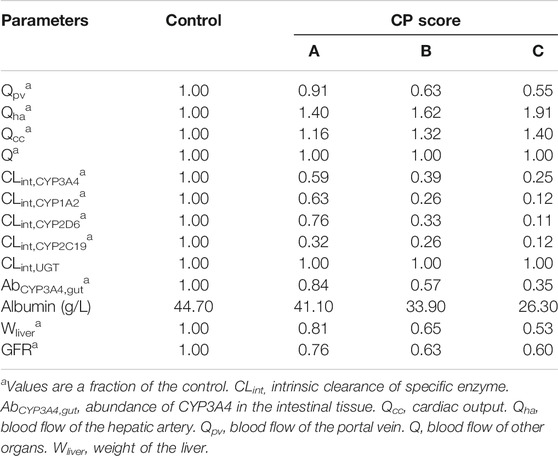
TABLE 1. Physiological and biochemical parameter changes in the liver disease model according to CP score (A, B, C). Johnson’s reported values (Johnson et al., 2010).
Oral Absorption
Oral absorption was simulated using a compartmental absorption and transit model (Bosgra et al., 2012). The drug absorption rate constant (Ka) was calculated using the effective permeability (Peff) based on the in vivo regional jejunal permeability in humans for PRO (Gertz et al., 2010). For MDZ and DEX values observed in the literature for Ka were applied. The parameters are described in Table 2.
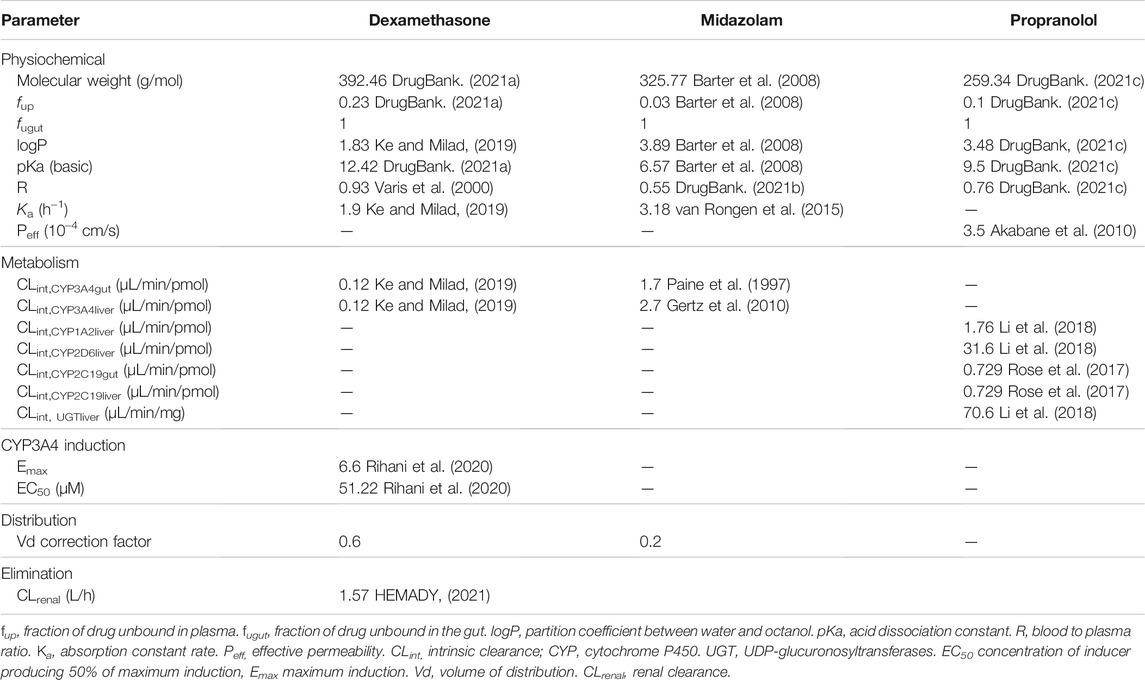
TABLE 2. Physiochemical and pharmacokinetic characteristics of dexamethasone, midazolam, and propranolol.
Intestinal Metabolism
The clearance of MDZ, PRO and DEX in the gut (CLgut) were calculated considering the intrinsic clearance (Clint) and abundance of the enzyme involved in the metabolism of each drug in the intestinal tissue (Eq. 1):
Where CLintENZYME is the Clint of CYP3A4 for MDZ and DEX, and the Clint of CYP2C19 for PRO, AbENZYME,gut is its relative abundance in the intestinal tissue [AbCYP3A4,gut = 19.2 pmol/mg, (Paine et al., 1997) AbCYP2C19,gut = 2.1 ± 0.1 pmol/mg (Paine et al., 2006)], MPPGI is the amount of microsomal protein per gram of intestine [MPPGI = 2.7 mg/g (Paine et al., 1997)], and Wintestines is the weight of the intestines. The Clint of each enzyme is described in Table 2. CYP2D6 and CYP1A2 was not considered in the gut for PRO since its contribution to total intestinal CYP is minimal (<1%). (Thelen and Dressman, 2009)
The fraction of drug escaping gut metabolism and transitioning to the liver (Fg) was computed with the following equation (Eq. 2):
Where Qgut represents the blood flow to the gut, and fu,gut is the fraction unbound of the drug in the gut, considered equal one in the model. (Yu and Amidon, 1999)
Hepatic Metabolism
Similarly, to the gut, the intrinsic clearance of each enzyme involved in the hepatic metabolism of MDZ, PRO and DEX were scaled up to the whole liver (CLint, liver) considering the equation below (Eq. 3):
Where CLintENZYME is the Clint of CYP3A4 for MDZ and DEX, and the Clint of CYP1A2, CYP2D6, CYP2C19 and UDP-glucuronosyltransferases (UGT) for PRO, AbENZYME, liver is the abundance of the enzyme in the liver [AbCYP3A4,liver = 155 pmol/mg, (Emoto et al., 2015) AbCYP1A2,liver = 29.4 ± 29.6 pmol/mg, (Achour et al., 2014) AbCYP2D6,liver = 11.9 ± 13.2 pmol/mg, (Achour et al., 2014) AbCYP2C19,liver = 17.8 ± 3.3 pmol/mg (Lasker et al., 1998)] and MPPGL is the amount of microsomal protein per gram of liver, Wliver is the weight of the liver. The Clint of CYP3A4 and CYP2C19 was considered the same in the gut and liver for DEX and PRO, respectively, since no data specific to the gut were available. The intrinsic clearance of CYP3A4 in the gut and liver for MDZ were considered different All parameters are described in Table 2. For PRO an additional CLint, liver was assumed to account for UGT metabolism (Eq. 4):
The MPPGL was calculated according to equation reported by (Eq. 5) Barter et al., 2008:
The total hepatic intrinsic clearance (∑CLint, liver) was considered as the sum of all enzymes involved in the metabolism. The hepatic systemic clearance (CLhep) was calculated considering blood flow and the total ∑CLint, liver (Eq. 6):
Where Qhv is the hepatic blood flow rate, fup is the fraction of drug unbound in plasma and R is the blood to plasma ratio. The fraction of drug that escapes hepatic metabolism and reaches the systemic circulation (Fh) is represented by the following equation (Eq. 7):
Where Qpv is the blood flow rate of the portal vein.
Given DEX`s auto-induction of CYP3A4, induction of CYP in the intestine and liver were calculated from using the following equation (Eq. 8):
Where Emax is the maximum enzyme activity, Corgan,u is the average unbound drug concentration in the intestinal and liver tissues and EC50 is the DEX concentration required to reach half of the maximum enzyme activity. Then the CLgut and CLint, liver (Eq. 1 and Eq. 3) was multiplied by IndCYP3A4,organ.
Portocaval shunting was incorporated into the LD model by implementing a shunt index that considers the varying levels of shunting associated with the different severities of liver disease as well as the serum total bile acid concentrations in the peripheral vein (Ohkubo et al., 1984; Simón-Talero et al., 2018). The fraction of drug that bypasses the liver due to shunting (Fshunt) is represented by the following (Eq. 9):
Distribution
Drug distribution was calculated using first-order differential equations, with the volume of distribution (Vd) computed using the tissue to plasma ratio (TP) of each organ and the volume of each organ compartment (Poulin and Theil, 2002). A correction factor (Table 2) was applied to the Vd of MDZ and DEX via curve-fitting method to match observed Vd values in the literature (DrugBank, 2021a; DrugBank, 2021b). The physiochemical properties of the drugs used in the models are detailed in Table 2.
Elimination
Elimination of MDZ and PRO were considered as exclusively hepatic however, for DEX an additional renal clearance amounting to 10% of the systemic clearance was applied in accordance with the literature (Table 2). (HEMADY, 2021) A liver impairment scaling factor for renal function was applied as described in Table 1.
Model Qualification
The model was firstly qualified for DEX, MDZ and PRO in a healthy population followed by qualification in a LD population for MDZ and PRO. The model qualification was extended to MDZ and PRO as the availability of observed clinical data across CP scores is incomplete and not all stages of LD and shunt index have been fully described. Specifically, MDZ was chosen due to its similarity in metabolic pathway to DEX, as both drugs are predominantly metabolised by CYP3A4. However, PK data for MDZ were not available for LD individuals with different CP scores, so a mixture of patients with different liver disease degrees was used (Pentikäinen et al., 1989). Therefore, to validate CP-A, -B and -C and the shunt index incorporated into the LD model individually, PRO was chosen due to the availability of observed clinical data reflecting these scenarios (Taegtmeyer et al., 2014). A schematic representation of this workflow is shown in Figure 1.
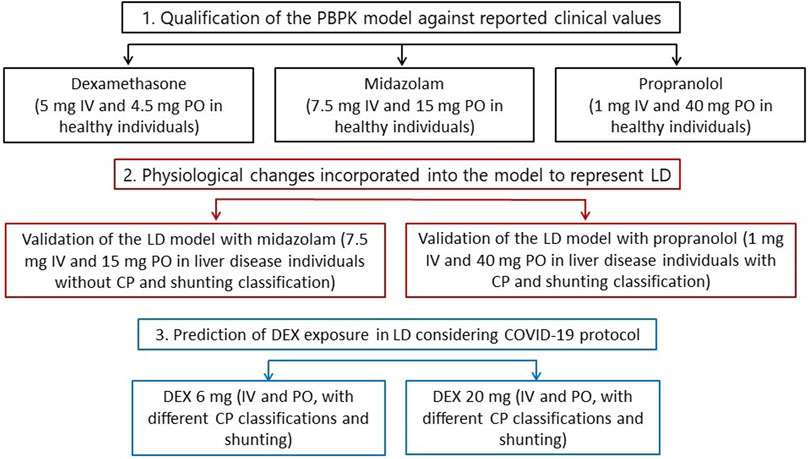
FIGURE 1. Overall step-by-step workflow representing the PBPK modelling qualification and predictions. IV, intravenous. PO, oral. LD, liver disease. CP, Child-Pugh. DEX, dexamethasone.
The model qualification was performed according to recommendations of the European Medicine Agency (EMA) (EMA, 2021b) and was considered validated when the mean of simulated PK parameters for each drug was less than two-fold of the observed clinical mean and the absolute average fold error (AFE) was below 2 (Abduljalil et al., 2014). The doses and regimens of the drugs were chosen to reflect the clinical studies used to validate the model (Pentikäinen et al., 1989; Varis et al., 2000; Taegtmeyer et al., 2014). Due to the type of clinical data available for MDZ, simulated PK parameters for MDZ in the LD population were calculated considering the mean of conditions CP-A, -B and -C in order to reflect the clinical data sets. For PRO, clinical data were reported for 15 individuals alongside their respective CP score and shunt index thus, 100 simulations were carried out for each individual with their specific age, weight, CP score and shunt index implemented in the LD model (Taegtmeyer et al., 2014). The mean PK parameters for PRO across all individuals were also calculated and compared for both simulated and observed clinical data.
Predictions
The PBPK model was used to predict the PK of DEX in virtual populations with varying degrees of liver disease, classified according to CP scores (A, B, and C). The varying levels of portacaval-shunting associated with liver disease considered in the simulations was an aleatory linearly spaced range with minimum value of 0.1 and maximum value of 0.7 as previously described (Simón-Talero et al., 2018).
The dosages selected for the simulations were in line with current COVID-19 protocols stipulated by the National Institute for Health and Care Excellence; 6 mg dose once a day PO for 7–10 days; 6 mg dose once a day IV for 7–10 days (The National Institute for Health and Care Excellence, 2021). To further evaluate high doses of DEX, simulations with 20 mg were made. The PK parameters were calculated considering steady-state plasma concentration on 10th day in accordance with the COVID-19 protocol.
Results
Model Qualification
The PBPK model was successfully qualified for all drugs in healthy (MDZ, PRO and DEX) and LD individuals (MDZ and PRO) according to the selected criteria. The simulated and observed PK parameters for each drug as well as the AFE values are presented in Table 3, as previously described. The plasma concentration-time profiles of each drug are described in the supplementary material (Supplementary Figures S1–5).
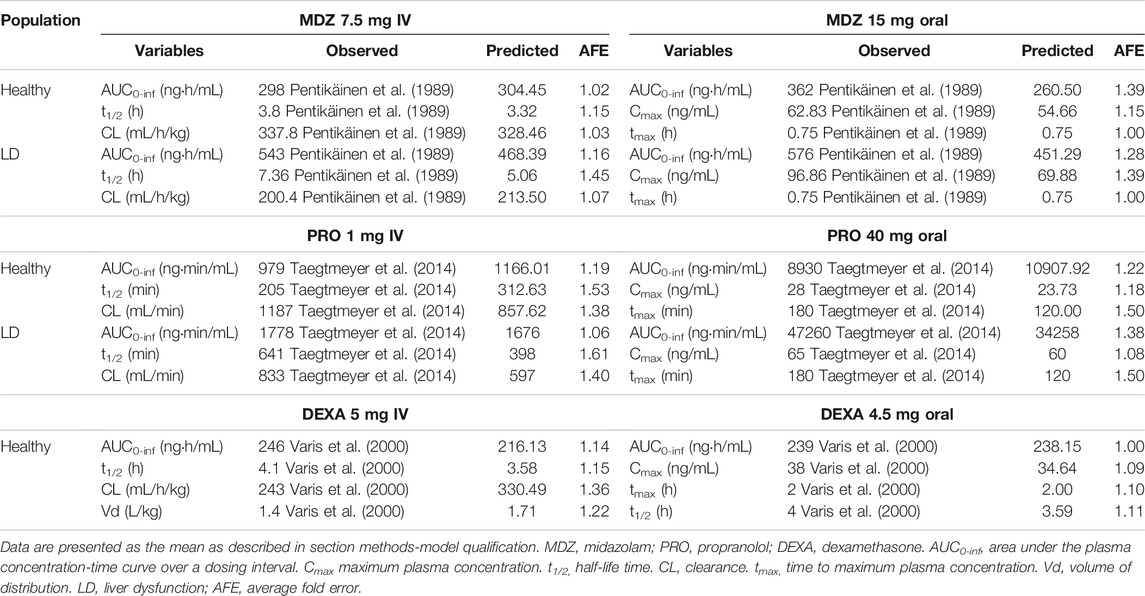
TABLE 3. Qualification of the PBPK model in healthy and liver disease individuals for midazolam, propranolol, and dexamethasone.
Predictions
The predicted PKs of DEX are shown in Table 4. The PBPK model for both the PO and IV administration of DEX predicted a decrease in the CL of DEX and increase in plasma concentration of DEX for patients with LD of all CP scores and shunt indexes. However, the exposure of DEX was found to be higher in patients with advanced CP scores, as shown in Figure 2. Furthermore, plasma concentrations of DEX were slightly higher in individuals with portal-systemic shunt compared to individuals with no shunting during PO administration. In Table 4, plasma concentrations are expressed as unbound in comparison to total plasma concentration shown in table 3. The shunting index had no effect when DEX was administered IV (Figure 2) as the portal-systemic shunt primarily affects first-pass metabolism. When exploring higher doses of DEX it was found that the AUC ratio between healthy and LD individuals remained comparable to lower doses, as described in Table 4.
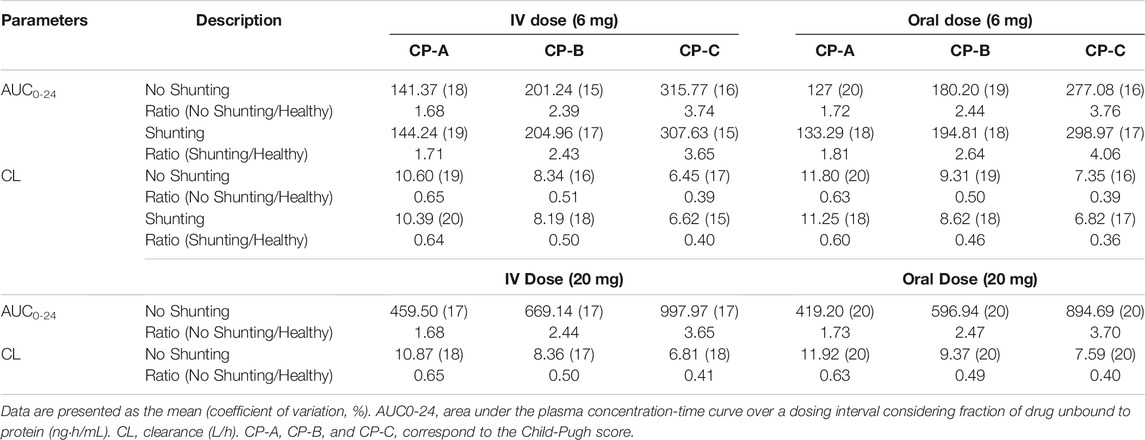
TABLE 4. Predictions of dexamethasone pharmacokinetics in virtual populations with varying degrees of liver disease.
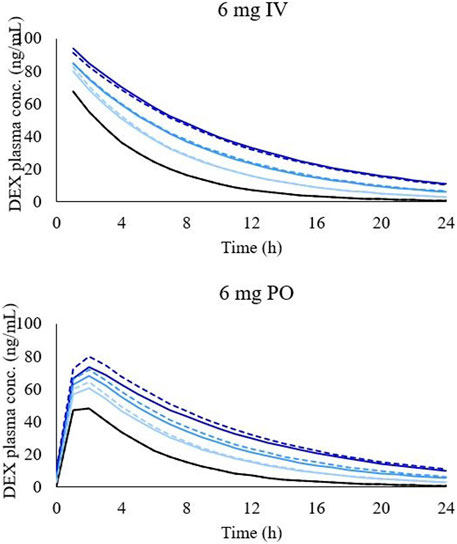
FIGURE 2. Simulated concentration-time profile of DEX in different LD conditions after 6 mg intravenous administration (graph on the top) and 6 mg oral administration (graph on the bottom). Black line, healthy individuals. Light blue line, CP-A condition, blue line, CP-B condition, and dark-blue line, CP-C condition. Dashed lines represent simulations with shunting and solid lines with no shunting.
Discussion
The clinical management of individuals with LD is challenging. The PK of DEX in LD patients has been partially described through a clinical study, showing a reduced clearance and increased half-life, but no information of total exposure (AUC0-inf) and PK profiles were available (Kawai et al., 1985). The PBPK model described herein simulated DEX PK in different stages of LD with various grades of shunting, providing evidence-based guidance towards the clinical management of COVID-19 in LD patients.
Overall, the plasma concentration of DEX is expected to increase with liver impairment. The PBPK model was successfully validated and predicted an increase in AUC0-24 of 172% (181% with shunt), 244% (264% with shunt), and 376% (406% with shunt) compared to healthy individuals for CP-A, CP-B, and CP-C, respectively when DEX was administered orally. Furthermore, the corresponding clearance values were predicted to decrease approximately 35%, 50% and 60% in comparison to healthy individuals for CP-A, CP-B, and CP-C, respectively. The trend for CL to decrease was comparable to that previously found in the DEX clinical trial in LD patients (Kawai et al., 1985). Furthermore, although first-pass metabolism can represent a relevant process in DEX PK, the predicted difference in AUC0-24 and CL between IV and PO administrations in LD individuals was minimal and is likely due to the high bioavailability (70–78%) of DEX (DrugBank, 2021a). Additionally, first-pass metabolism is thought to be impacted by the shunt effect, increasing a drug’s bioavailability, yet the impact of shunting on the AUC0-24 and CL of DEX remained minimal. Simulations of DEX 20 mg IV and PO once a day were made since higher doses are being investigated to present clinical improvement and decrease in inflammatory biomarkers in patients hospitalized with COVID-19, but remain unproven (Vecchié et al., 2021). However, the AUC ratio between healthy and individuals with LD remained comparable to the lower dose (6 mg once a day), showing linear PK. For this reason, no simulation with shunt effect was performed with the higher dose since the same behaviour of lower dose is expected.
According to the FDA and the EMA guidance for industry, PK studies should be conducted in patients with impaired hepatic function to evaluate whether a dose adjustment is necessary (FDA, 2021; EMA, 2021a), yet the number of drugs that provide this specific recommendation for dosage adjustment based on different hepatic functions is very limited (Verbeeck, 2008). This LD PBPK model could be applied to evaluate other drugs for use in COVID-19, such as anticoagulants, other corticosteroids, antiviral agents, antibiotics, anti-inflammatory drugs (Wu et al., 2020).
According to the FDA guidelines, dosage adjustments should be recommended when a two-fold or greater increase in the AUC is observed (FDA, 2021). However, dose adjustment in COVID-19 patients is complex, defining a multifactorial scenario for which polypharmacy and pre-existing conditions should be considered alongside the risk of potential drug-drug interactions (DDIs) which may lead to altered PK. Moreover, the use of support resources in the care of COVID-19 patients, such as renal replacement, ventilation, volume replacement, can affect drug ADME generating further complexities in the assessment of dosing strategies (Venisse et al., 2020).
There are some aspects in the application of DEX for the treatment of COVID-19 that should be considered when analysing the data from this study. Firstly, severe COVID-19 is associated with a systemic hyper-inflammation state with increased cytokine levels and highly elevated C-reactive protein (CRP) all of which are known to impact drug PK through the downregulation of CYP isoenzymes (Dickmann et al., 2011; Simon et al., 2019; Simon et al., 2021). The current PBPK model does not incorporate these mechanisms due to limited data/ability to verify the model. Secondly, DEX has been co-administered with tocilizumab for the treatment of COVID-19, however, the DDI between these drugs has not been studied in LD individuals. Tocilizumab has been reported to inhibit interleukin 6 (IL-6), increasing the activity of CYP450 enzymes and therefore producing increased metabolism of drugs that are CYP450 substrates (ACTEMRA, 2021). This pharmacodynamic DDI may compensate for the decreased CL observed in LD condition. Finally, corticosteroids such as DEX can present side effects relating to the central nervous system. In most cases the side effects occur within the five first days of treatment, however, the psychiatric symptoms tend to begin after 11 days and become more pronounced with extended periods of treatment. The psychiatric side effect also appears to be dose-dependent, occurring more often for doses up to 80 mg daily (Ciriaco et al., 2013). Whilst side effects need to be monitored during the administration of DEX, no dose adjustments seem necessary in healthy or LD patients.
Though the PK of DEX in LD was successfully predicted, the model is characterized by some limitations. Although a direct and proportional relationship between CP score and increase in the plasma drug exposure was assume in the model, as demonstrated before by Johnson et al., 2010 and also a linear correlation between portal vein shunt index and serum total bile acid concentrations in the peripheral vein (Ohkubo et al., 1984), outliers individuals will not be represented by this assumption as demonstrated in Supplementary Table S2. Furthermore, the prevalence of spontaneous portosystemic shunt (SPS) increases as liver function injures, probably as an effect of damaging portal hypertension (Simón-Talero et al., 2018). However, large-SPS can be present in CP-A individuals as no SPS or small-SPS can be present in CP-C individuals (Simón-Talero et al., 2018). For this reason, the varying levels of portacaval-shunting associated with LD considered in the simulations was an aleatory linearly spaced range between 0.1 and 0.7. Other factors such as inter-individual variability, polymorphism, age (e.g., propranolol showed greater plasma level in elderly compared to young individuals) (Castleden et al., 1975), and unknown LD physiopathology mechanisms not represented in the model corroborate the challenge of qualifying the LD PBPK model against specific CP classifications.
Conclusion
An increased exposure of DEX across varying stages of LD was predicted using PBPK modelling. Although DEX exposure was predicted to be more than 3 times higher in CP-C individuals, no dose adjustments seem necessary in patients with LD considering DEX’s low hepatic extraction, the low dose administered in the COVID-19 protocol and short period of treatment (10 days), and the therapeutic index of DEX. This study provides in silico evidence-based guidance towards the management of complex clinical scenarios related to COVID-19 and provides a rational framework for future PBPK modelling applications in LD patients. Further PBPK modelling initiatives would be necessary to evaluate the net effect of both LD and inflammatory physiological alterations on the PK of drugs used in the treatment of COVID-19.
Study Highlights
- To propose a PBPK model capable of simulating the PK of drugs in LD patients classified according to the Child-Pugh system.
- To integrate portacaval-shunting associated with LD in a PBPK model.
- To predict DEX exposure in LD patients considering the dose administered in the COVID-19 protocol.
Data Availability Statement
The original contributions presented in the study are included in the article/Supplementary Material, further inquiries can be directed to the corresponding author.
Author Contributions
All authors contributed to the overall concept of the model. MM, NC, and MB performed the modelling design, qualification, and application of the model. MM, MB, and MS wrote the manuscript with support from all the other authors. All authors reviewed and contributed to the final manuscript.
Funding
This project is funded by a research grant awarded by the UKRI (MRC) and the DHSC (NIHR), grant reference: MR/V020498/1.
Conflict of Interest
The authors declare that the research was conducted in the absence of any commercial or financial relationships that could be construed as a potential conflict of interest.
Publisher’s Note
All claims expressed in this article are solely those of the authors and do not necessarily represent those of their affiliated organizations, or those of the publisher, the editors and the reviewers. Any product that may be evaluated in this article, or claim that may be made by its manufacturer, is not guaranteed or endorsed by the publisher.
Supplementary Material
The Supplementary Material for this article can be found online at: https://www.frontiersin.org/articles/10.3389/fphar.2022.814134/full#supplementary-material
References
Abduljalil, K., Cain, T., Humphries, H., and Rostami-Hodjegan, A. (2014). Deciding on success Criteria for Predictability of Pharmacokinetic Parameters from In Vitro Studies: an Analysis Based on In Vivo Observations. Drug Metab. Dispos 42 (9), 1478–1484. doi:10.1124/dmd.114.058099
Achour, B., Russell, M. R., Barber, J., and Rostami-Hodjegan, A. (2014). Simultaneous Quantification of the Abundance of Several Cytochrome P450 and Uridine 5'-Diphospho-Glucuronosyltransferase Enzymes in Human Liver Microsomes Using Multiplexed Targeted Proteomics. Drug Metab. Dispos 42 (4), 500–510. doi:10.1124/dmd.113.055632
Akabane, T., Tabata, K., Kadono, K., Sakuda, S., Terashita, S., and Teramura, T. (2010). A Comparison of Pharmacokinetics between Humans and Monkeys. Drug Metab. Dispos 38 (2), 308–316. doi:10.1124/dmd.109.028829
Barter, Z. E., Chowdry, J. E., Harlow, J. R., Snawder, J. E., Lipscomb, J. C., and Rostami-Hodjegan, A. (2008). Covariation of Human Microsomal Protein Per Gram of Liver with Age: Absence of Influence of Operator and Sample Storage May Justify Interlaboratory Data Pooling. Drug Metab. Dispos 36 (12), 2405–2409. doi:10.1124/dmd.108.021311
Birnbaum, L., Brown, R., Bischoff, K., Foran, J., Blancato, J., Clewell, H., et al. (1994). Physiological Parameter Values for PBPK Models. Washington, DC: International Life Sciences Institute, Risk Science Institute.
Bosgra, S., van Eijkeren, J., Bos, P., Zeilmaker, M., and Slob, W. (2012). An Improved Model to Predict Physiologically Based Model Parameters and Their Inter-individual Variability from Anthropometry. Crit. Rev. Toxicol. 42 (9), 751–767. doi:10.3109/10408444.2012.709225
Brown, R. P., Delp, M. D., Lindstedt, S. L., Rhomberg, L. R., and Beliles, R. P. (1997). Physiological Parameter Values for Physiologically Based Pharmacokinetic Models. Toxicol. Ind. Health 13 (4), 407–484. doi:10.1177/074823379701300401
Castleden, C. M., Kaye, C. M., and Parsons, R. L. (1975). The Effect of Age on Plasma Levels of Propranolol and Practolol in Man. Br. J. Clin. Pharmacol. 2 (4), 303–306. doi:10.1111/j.1365-2125.1975.tb02774.x
Ciriaco, M., Ventrice, P., Russo, G., Scicchitano, M., Mazzitello, G., Scicchitano, F., et al. (2013). Corticosteroid-related central Nervous System Side Effects. J. Pharmacol. Pharmacother. 4 (Suppl. 1), S94–S98. doi:10.4103/0976-500X.120975
de la Grandmaison, G. L., Clairand, I., and Durigon, M. (2001). Organ Weight in 684 Adult Autopsies: New Tables for a Caucasoid Population. Forensic Sci. Int. 119 (2), 149–154. doi:10.1016/s0379-0738(00)00401-1
Dickmann, L. J., Patel, S. K., Rock, D. A., Wienkers, L. C., and Slatter, J. G. (2011). Effects of Interleukin-6 (IL-6) and an anti-IL-6 Monoclonal Antibody on Drug-Metabolizing Enzymes in Human Hepatocyte Culture. Drug Metab. Dispos 39 (8), 1415–1422. doi:10.1124/dmd.111.038679
DrugBank (2021a). Dexamethasone. Available at: https://go.drugbank.com/drugs/DB01234 (Accessed 27 October 2021).
DrugBank (2021b). Midazolam. Available at: https://go.drugbank.com/drugs/DB00683 (Accessed 27 October 2021).
DrugBank (2021c). Propranolol. Available at: https://go.drugbank.com/drugs/DB00571 (Accessed 27 October 2021).
Edginton, A. N., and Willmann, S. (2008). Physiology-based Simulations of a Pathological Condition: Prediction of Pharmacokinetics in Patients with Liver Cirrhosis. Clin. Pharmacokinet. 47 (11), 743–752. doi:10.2165/00003088-200847110-00005
EMA. (2021a). Guideline on the Evaluation of the Pharmacokinetics of Medicinal Products in Patients with Impaired Hepatic Function. Available at: https://www.ema.europa.eu/en/evaluation-pharmacokinetics-medicinal-products-patients-impaired-hepatic-function (Accessed 27 October 2021).
EMA (2021b). Guideline on the Qualification and Reporting of Physiologically Based Pharmacokinetic (PBPK) Modelling and Simulation. Available at: https://www.ema.europa.eu/en/documents/scientific-guideline/draft-guideline-qualification-reporting-physiologically-based-pharmacokinetic-pbpk-modelling_en.pdf (Accessed 10 June 2020).
Emoto, C., Fukuda, T., Venkatasubramanian, R., and Vinks, A. A. (2015). The Impact of CYP3A5*3 Polymorphism on Sirolimus Pharmacokinetics: Insights from Predictions with a Physiologically-Based Pharmacokinetic Model. Br. J. Clin. Pharmacol. 80 (6), 1438–1446. doi:10.1111/bcp.12743
FDA (2021). Guidance for Industry: Pharmacokinetics in Patients with Impaired Hepatic Function: Study Design, Data Analysis, and Impact on Dosing and Labelling. Available at: https://www.fda.gov/media/71311/download (Accessed 27 October 2021).
Gertz, M., Harrison, A., Houston, J. B., and Galetin, A. (2010). Prediction of Human Intestinal First-Pass Metabolism of 25 CYP3A Substrates from In Vitro Clearance and Permeability Data. Drug Metab. Dispos 38 (7), 1147–1158. doi:10.1124/dmd.110.032649
Grimstein, M., Yang, Y., Zhang, X., Grillo, J., Huang, S. M., Zineh, I., et al. (2019). Physiologically Based Pharmacokinetic Modeling in Regulatory Science: An Update from the U.S. Food and Drug Administration's Office of Clinical Pharmacology. J. Pharm. Sci. 108 (1), 21–25. doi:10.1016/j.xphs.2018.10.033
HEMADY (dexamethasone Tablets) (2021). US Prescribing Information. East Windsor, NJ: Acrotech Biopharma LLC.
Johnson, T. N., Boussery, K., Rowland-Yeo, K., Tucker, G. T., and Rostami-Hodjegan, A. (2010). A Semi-mechanistic Model to Predict the Effects of Liver Cirrhosis on Drug Clearance. Clin. Pharmacokinet. 49 (3), 189–206. doi:10.2165/11318160-000000000-00000
Kawai, S., Ichikawa, Y., and Homma, M. (1985). Differences in Metabolic Properties Among Cortisol, Prednisolone, and Dexamethasone in Liver and Renal Diseases: Accelerated Metabolism of Dexamethasone in Renal Failure. J. Clin. Endocrinol. Metab. 60 (5), 848–854. doi:10.1210/jcem-60-5-848
Ke, A. B., and Milad, M. A. (2019). Evaluation of Maternal Drug Exposure Following the Administration of Antenatal Corticosteroids during Late Pregnancy Using Physiologically-Based Pharmacokinetic Modeling. Clin. Pharmacol. Ther. 106 (1), 164–173. doi:10.1002/cpt.1438
Kovalic, A. J., Satapathy, S. K., and Thuluvath, P. J. (2020). Prevalence of Chronic Liver Disease in Patients with COVID-19 and Their Clinical Outcomes: a Systematic Review and Meta-Analysis. Hepatol. Int. 14 (5), 612–620. doi:10.1007/s12072-020-10078-2
Lasker, J. M., Wester, M. R., Aramsombatdee, E., and Raucy, J. L. (1998). Characterization of CYP2C19 and CYP2C9 from Human Liver: Respective Roles in Microsomal Tolbutamide, S-Mephenytoin, and Omeprazole Hydroxylations. Arch. Biochem. Biophys. 353 (1), 16–28. doi:10.1006/abbi.1998.0615
Li, M., Zhu, L., Chen, L., Li, N., and Qi, F. (2018). Assessment of Drug-Drug Interactions between Voriconazole and Glucocorticoids. J. Chemother. 30 (5), 296–303. doi:10.1080/1120009X.2018.1506693
Matthay, M. A., and Thompson, B. T. (2020). Dexamethasone in Hospitalised Patients with COVID-19: Addressing Uncertainties. Lancet Respir. Med. 8 (12), 1170–1172. doi:10.1016/S2213-2600(20)30503-8
Ohkubo, H., Okuda, K., Iida, S., Ohnishi, K., Ikawa, S., and Makino, I. (1984). Role of portal and Splenic Vein Shunts and Impaired Hepatic Extraction in the Elevated Serum Bile Acids in Liver Cirrhosis. Gastroenterology 86 (3), 514–520. doi:10.1016/s0016-5085(84)80022-0
Paine, M. F., Khalighi, M., Fisher, J. M., Shen, D. D., Kunze, K. L., Marsh, C. L., et al. (1997). Characterization of Interintestinal and Intraintestinal Variations in Human CYP3A-dependent Metabolism. J. Pharmacol. Exp. Ther. 283 (3), 1552–1562.
Paine, M. F., Hart, H. L., Ludington, S. S., Haining, R. L., Rettie, A. E., and Zeldin, D. C. (2006). The Human Intestinal Cytochrome P450 "pie". Drug Metab. Dispos 34 (5), 880–886. doi:10.1124/dmd.105.008672
Pentikäinen, P. J., Välisalmi, L., Himberg, J. J., and Crevoisier, C. (1989). Pharmacokinetics of Midazolam Following Intravenous and Oral Administration in Patients with Chronic Liver Disease and in Healthy Subjects. J. Clin. Pharmacol. 29 (3), 272–277.
Pilla Reddy, V., Walker, M., Sharma, P., Ballard, P., and Vishwanathan, K. (2018). Development, Verification, and Prediction of Osimertinib Drug-Drug Interactions Using PBPK Modeling Approach to Inform Drug Label. CPT Pharmacometrics Syst. Pharmacol. 7 (5), 321–330. doi:10.1002/psp4.12289
Poulin, P., and Theil, F. P. (2002). Prediction of Pharmacokinetics Prior to In Vivo Studies. 1. Mechanism-Based Prediction of Volume of Distribution. J. Pharm. Sci. 91 (1), 129–156. doi:10.1002/jps.10005
Qiu, P., Zhou, Y., Wang, F., Wang, H., Zhang, M., Pan, X., et al. (2020). Clinical Characteristics, Laboratory Outcome Characteristics, Comorbidities, and Complications of Related COVID-19 Deceased: a Systematic Review and Meta-Analysis. Aging Clin. Exp. Res. 32 (9), 1869–1878. doi:10.1007/s40520-020-01664-3
Rihani, B. A. S., Deodhar, M., Dow, P., Turgeon, J., and Michaud, V. (2020). Is Dexamethasone a Substrate, an Inducer, or a Substrate-Inducer of CYP3As. Arch. Pharm. Pharmacol. Res. 2. doi:10.33552/APPR.2020.02.000546
Rodighiero, V. (1999). Effects of Liver Disease on Pharmacokinetics. An Update. Clin. Pharmacokinet. 37 (5), 399–431. doi:10.2165/00003088-199937050-00004
Rose, R. H., Turner, D. B., Neuhoff, S., and Jamei, M. (2017). Incorporation of the Time-Varying Postprandial Increase in Splanchnic Blood Flow into a PBPK Model to Predict the Effect of Food on the Pharmacokinetics of Orally Administered High-Extraction Drugs. AAPS J. 19 (4), 1205–1217. doi:10.1208/s12248-017-0099-z
Schuppan, D., and Afdhal, N. H. (2008). Liver Cirrhosis. Lancet 371 (9615), 838–851. doi:10.1016/S0140-6736(08)60383-9
Simon, F., Garcia, J., Guyot, L., Guitton, J., Vilchez, G., Bardel, C., et al. (2019). Impact of Interleukin-6 on Drug-Metabolizing Enzymes and Transporters in Intestinal Cells. AAPS J. 22 (1), 16. doi:10.1208/s12248-019-0395-x
Simon, F., Gautier-Veyret, E., Truffot, A., Chenel, M., Payen, L., Stanke-Labesque, F., et al. (2021). Modeling Approach to Predict the Impact of Inflammation on the Pharmacokinetics of CYP2C19 and CYP3A4 Substrates. Pharm. Res. 38 (3), 415–428. doi:10.1007/s11095-021-03019-7
Simón-Talero, M., Roccarina, D., Martínez, J., Lampichler, K., Baiges, A., Low, G., et al. (2018). Association between Portosystemic Shunts and Increased Complications and Mortality in Patients with Cirrhosis. Gastroenterology 154 (6), 1694–1705. e1694. doi:10.1053/j.gastro.2018.01.028
Taegtmeyer, A. B., Haschke, M., Tchambaz, L., Buylaert, M., Tschöpl, M., Beuers, U., et al. (2014). A Study of the Relationship between Serum Bile Acids and Propranolol Pharmacokinetics and Pharmacodynamics in Patients with Liver Cirrhosis and in Healthy Controls. PLoS One 9 (6), e97885. doi:10.1371/journal.pone.0097885
The National Institute for Health and Care Excellence (NICE) (2021). COVID-19 Rapid Guideline: Managing COVID-19. Available at: https://www.nice.org.uk/guidance/ng191/resources/covid19-rapid-guideline-managing-covid19-pdf-51035553326 (Accessed 27 October 2021).
Thelen, K., and Dressman, J. B. (2009). Cytochrome P450-Mediated Metabolism in the Human Gut wall. J. Pharm. Pharmacol. 61 (5), 541–558. doi:10.1211/jpp/61.05.0002
van Rongen, A., Kervezee, L., Brill, M., van Meir, H., den Hartigh, J., Guchelaar, H. J., et al. (2015). Population Pharmacokinetic Model Characterizing 24-Hour Variation in the Pharmacokinetics of Oral and Intravenous Midazolam in Healthy Volunteers. CPT Pharmacometrics Syst. Pharmacol. 4 (8), 454–464. doi:10.1002/psp4.12007
Varis, T., Kivistö, K. T., Backman, J. T., and Neuvonen, P. J. (2000). The Cytochrome P450 3A4 Inhibitor Itraconazole Markedly Increases the Plasma Concentrations of Dexamethasone and Enhances its Adrenal-Suppressant Effect. Clin. Pharmacol. Ther. 68 (5), 487–494. doi:10.1067/mcp.2000.110772
Vecchié, A., Batticciotto, A., Tangianu, F., Bonaventura, A., Pennella, B., Abenante, A., et al. (2021). High-dose Dexamethasone Treatment for COVID-19 Severe Acute Respiratory Distress Syndrome: a Retrospective Study. Intern. Emerg. Med. 16 (7), 1913–1919. doi:10.1007/s11739-021-02800-1
Venisse, N., Peytavin, G., Bouchet, S., Gagnieu, M. C., Garraffo, R., Guilhaumou, R., et al. (2020). Concerns about Pharmacokinetic (PK) and Pharmacokinetic-Pharmacodynamic (PK-PD) Studies in the New Therapeutic Area of COVID-19 Infection. Antivir. Res 181, 104866. doi:10.1016/j.antiviral.2020.104866
Verbeeck, R. K. (2008). Pharmacokinetics and Dosage Adjustment in Patients with Hepatic Dysfunction Eur. J. Clin. Pharmacol. 64 (12), 1147–1161. doi:10.1007/s00228-008-0553-z
Wu, R., Wang, L., Kuo, H. D., Shannar, A., Peter, R., Chou, P. J., et al. (2020). An Update on Current Therapeutic Drugs Treating COVID-19. Curr. Pharmacol. Rep. 56, 70–15. doi:10.1007/s40495-020-00216-7
Keywords: dexamethasone, liver disease, COVID-19, PBPK modelling, pharmacokinetic (PK)
Citation: Montanha MC, Cottura N, Booth M, Hodge D, Bunglawala F, Kinvig H, Grañana-Castillo S, Lloyd A, Khoo S and Siccardi M (2022) PBPK Modelling of Dexamethasone in Patients With COVID-19 and Liver Disease. Front. Pharmacol. 13:814134. doi: 10.3389/fphar.2022.814134
Received: 15 November 2021; Accepted: 11 January 2022;
Published: 28 January 2022.
Edited by:
Shuiying Hu, The Ohio State University, United StatesReviewed by:
Qiang Fu, Black Diamond Therapeutics Inc., United StatesBart Hens, Pfizer, United Kingdom
Copyright © 2022 Montanha, Cottura, Booth, Hodge, Bunglawala, Kinvig, Grañana-Castillo, Lloyd, Khoo and Siccardi. This is an open-access article distributed under the terms of the Creative Commons Attribution License (CC BY). The use, distribution or reproduction in other forums is permitted, provided the original author(s) and the copyright owner(s) are credited and that the original publication in this journal is cited, in accordance with accepted academic practice. No use, distribution or reproduction is permitted which does not comply with these terms.
*Correspondence: Maiara Camotti Montanha, TS5jYW1vdHRpLW1vbnRhbmhhQGxpdmVycG9vbC5hYy51aw==