- 1School of Design, Shanghai Jiao Tong University, Shanghai, China
- 2Analysis and Testing Centre of Shanghai Jiao Tong University, Shanghai, China
- 3Shanghai Key Laboratory of Psychiatric Disorders, Shanghai Mental Health Center, Shanghai Jiao Tong University School of Medicine, Shanghai, China
Our objective was to explore the mechanism of essential oil that was extracted from Cinnamomum camphora chvar. Borneol (Borneol essential oil) for improving learning and memory impairment in mice. Brain tissue and plasma samples of a normal group, a model group, a Borneol essential oil group and a reference group were detected using gas chromatography time-of-flight mass spectrometry (GC-TOFMS) in order to find differential metabolites and analyze metabolic pathways. Results showed that there were 11 different metabolites --including glycine and azelaic acid --in plasma samples, and that there were 26 different metabolites--including adenine and aspartic acid --in brain tissue samples. These metabolites are involved in phenylalanine, tyrosine, and tryptophan biosynthesis, phenylalanine metabolism, alanine, aspartate and glutamate metabolism, arginine biosynthesis, beta-alanine metabolism, glyoxylate acid and dicarboxylate metabolism, and aminoacyl-tRNA biosynthesis. Thus, Borneol essential oil may improve learning and memory impairment by regulating amino acid metabolism and/or neurotransmitter changes.
Introduction
Metabonomics is one of the important research methods in systems biology. By analyzing a large number of endogenous metabolites in complex biological matrices, we can examine the mechanism of biochemical disturbances caused by diseases, drugs, or toxins (Phua et al., 2013). At present, metabolomics research can be completed using a variety of biological samples, including blood, feces, saliva, tissue, and urine. Gas chromatography time-of-flight mass spectrometry (GC-TOFMS) is a mature technology with fast ion collection speed and wide mass range that is suitable for the full spectrum of metabolomics analysis of biological substances (Liu et al., 2011).
Learning and memory impairment refers to the decline in the ability to remember, maintain, re-recognize and reproduce objective things (Gupta et al., 2018). A classic learning and memory disease, Alzheimer’s disease, has received increasing social attention in recent years. According to research reports in 2020, there were approximately 9.83 million Alzheimer’s disease patients in China (Jia et al., 2020). Thus, preventing and treating Alzheimer’s disease is urgent. At present, the clinical treatment of Alzheimer’s disease uses marketed drugs including donepezil and memantine. In addition, many scholars have found that rosemary essential oil (Liu et al., 2015; Filiptsova et al., 2017), citronella essential oil (Wang and Kang, 2017), and peppermint compound essential oil (Sun et al., 2019) can improve learning and memory impairment in rats and/or humans. Previous work by our team has also determined that, after sniffing Cinnamomum camphora chvar. Borneol essential oil (Borneol essential oil), mice’s scores on the Morris water maze, a classic index of learning and memory, improved, and that their hippocampal β-amyloid proteins decreased. Thus, it appears that Borneol essential oils can improve learning and memory impairment in mice. In this study, we collected brain tissue and plasma samples from mice, and used GC-TOFMS metabonomics to explore the physiological mechanism underlying Borneol essential oil’s effects on improving learning and memory impairment in mice.
Materials
Reagents and Equipment
Borneol essential oil and rosemary essential oil were provided by the aromatherapy laboratory of Shanghai Jiao Tong University. Methanol, chloroform, methoxyamine hydrochloride, pyridine, L-2-chlorophenylalanine, and n,o-bis-trimethylsilyl-trifluoroacetamide (BSTFA) that contained 1% trimethylchlorosilane (TMCS) were provided by the Analysis and Testing Centre of Shanghai Jiao Tong University.
Agilent 6890N gas chromatograph and Pegasus 4D TOF-MS were from the Laboratory Equipment Corporation (United States); the TGL-16M high-speed freezing centrifuge was from the Hunan Xiangyi Laboratory Instrument Company Limited (China), and the LNG-T88 vacuum centrifugal concentrator was from the Taicang Huamei Biochemical Instrument Factory (China).
Animals and Groups
Six-month-old learning and memory impairment model mice induced by APP/PS1 transgenic (model mice) and normal C57BL/6J mice (normal mice) of the same genetic background were provided by the Changzhou Cavens Laboratory Animal Co., Ltd., with license number SCXK (Su) 2016-0010. We constructed a normal group which used normal mice. We constructed a model group, a Borneol essential oil group and a reference group, which used model mice. We had eight mice in each group. The mice in the Borneol essential oil group sniffed Borneol essential oil, and the mice in the reference group sniffed rosemary essential oil [efficacy was determined by animal and volunteer experiments (Liu et al., 2015; Filiptsova et al., 2017)], 2 times/day, 1 h each time, for 21 days. There was no treatment for the model group or the normal group. We uniformly collected metabonomics samples from all mice after 21 days.
Methods
Sample Collection and Pretreatment
Plasma Samples
The mice were anesthetized with ether, and blood was taken from their eyeballs. The blood was added to EDTA anticoagulant, centrifuged in the centrifuge for 15 min (4°C, 3,000 r/min), and the supernatant plasma was taken and stored in a −80°C ultra-low temperature refrigerator for later use. When in use, the plasma was thawed at room temperature. We added 100 μl plasma to centrifuge tube, and then added 300 μl methanol-chloroform (3:1, V/V) and 10 μl L-2-chlorophenylalanine (0.3 mg/ml). We then shook the centrifuge tube for 30 s, and placed it in a freezer at 20°C for 20 min. We next centrifuged the sample for 10 min (4°C, 12,000 r/min), taking 300 μl supernatant into a 1.5 ml autosampler vial, concentrated the sample in vacuum and dried it with nitrogen. We added 80 μl pyridine solution to methoxyamine hydrochloride (15 mg/ml), sealed the vial, and put the sample on a shaking bed for one night at room temperature. Then, we opened the vial, added 80 μl of BSTFA (including 1% TMCS), sealed the vial, and put the sample in a thermostatic box for 60 min at 70°C. We detected samples at room temperature (Liu et al., 2017).
Brain Tissue Samples
We decapitated the mice and removed their brain tissue. We washed the tissue with normal saline, and then added normal saline and ground it up to make a 10% volume brain tissue homogenate. After centrifuging the sample for 10 min (4°C, 3,500 rpm), we took out the supernatant and placed it in a −80°C ultra-low temperature refrigerator for later use. The following steps were basically the same as those outlined in Plasma Samples.
Parameters of GC-TOFMS
The DB-5MS capillary column (length: 30 m, diameter: 250 μm, thickness: 0.25 μm) was used. We set the starting temperature of column to 80°C for 2 min, increased it to 180°C at 10°C/min, increased it to 240°C at 5°C/min, and then increased it to 290°C at 25°C/min for 9 min. The carrier gas was helium, and the flow rate was 1 ml/min. The interface and ion source temperatures were 270 and 220°C, respectively. The ionization mode of the mass spectrometer was set to EI, and the ionization voltage was 70 eV. The machine was scanned in a full scan mode, with a scanning range of 30–550 m/z. The plasma sample solvent was delayed by 7.5 min, and the brain tissue sample solvent was delayed by 7.6 min.
Data Processing and Analysis
LECO chroma TOF software was used to process the original data, including peak area integration, baseline calibration, and baseline filtering, to obtain the mathematical matrix. We imported this matrix into the SIMCA software for multidimensional statistical analysis. Potential differential metabolites were screened according to variable importance in the projection (VIP) and t-tests. The specific standards were VIP > 1 and p < 0.05. The NIST database and databases from Shanghai Jiao Tong University analysis and the Testing Centre were used to identify substances. Finally, the MetaboAnalyst platform was used to investigate the involved metabolic pathways (Liu et al., 2017).
Results
Principal Component Analysis
The result of a principal component analysis (PCA) showed that there were obvious differences between the plasma samples from the normal group, model group, Borneol essential oil group and the reference group (Figure 1). All samples were located within the 95% confidence interval, and the cumulative interpretation rate of the model was R2X = 0.687, Q2 = 0.134. There were also certain differences between the brain tissue samples in the four groups (Figure 2). All samples were located in 95% confidence interval, and the cumulative interpretation rate of the model was R2X = 0.665, Q2 = 0.392.
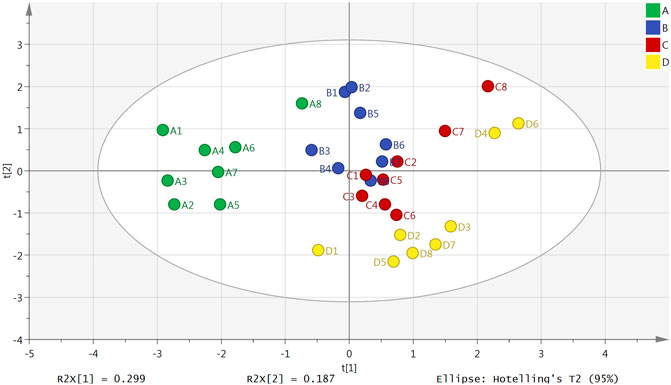
FIGURE 1. PCA scores plot of the normal group (A), model group (B), Borneol essential oil group (C), and reference group (D) in plasma samples (n = 8).
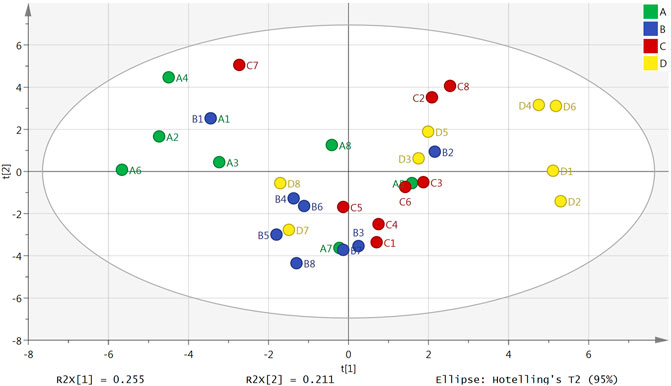
FIGURE 2. PCA scores plot of the normal group (A), model group (B), Borneol essential oil group (C), and reference group (D) in brain tissue samples (n = 8).
Orthogonal Projections to Latent Structures Discriminant Analysis
In order to maximize the separation of samples and pay attention to the substances that contributed to model clustering, we used the supervised method of orthogonal projections to latent structures discriminant analysis (OPLS-DA) to establish the model again, and carried out permutation testing (n = 200) to evaluate whether the model was overfitted. The results from the plasma samples are shown in Figure 3: the OPLS-DA scores plot diagram of the normal group and model group (R2X = 0.474, R2Y = 0.948, Q2 = 0.830) and its permutation testing diagram (R2 = 0.711, Q2 = −0.480); the OPLS-DA scores plot diagram of the model group and Borneol essential oil group (R2X = 0.375, R2Y = 0.938, Q2 = 0.654) and its permutation testing diagram (R2 = 0.732, Q2 = −0.519); the OPLS-DA scores plot diagram of the model group and reference group (R2X = 0.461, R2Y = 0.964, Q2 = 0.879) and its permutation testing diagram (R2 = 0.626, Q2 = −0.477). Parameters indicate that these models are relatively stable, have good prediction abilities, and are not overfitted.
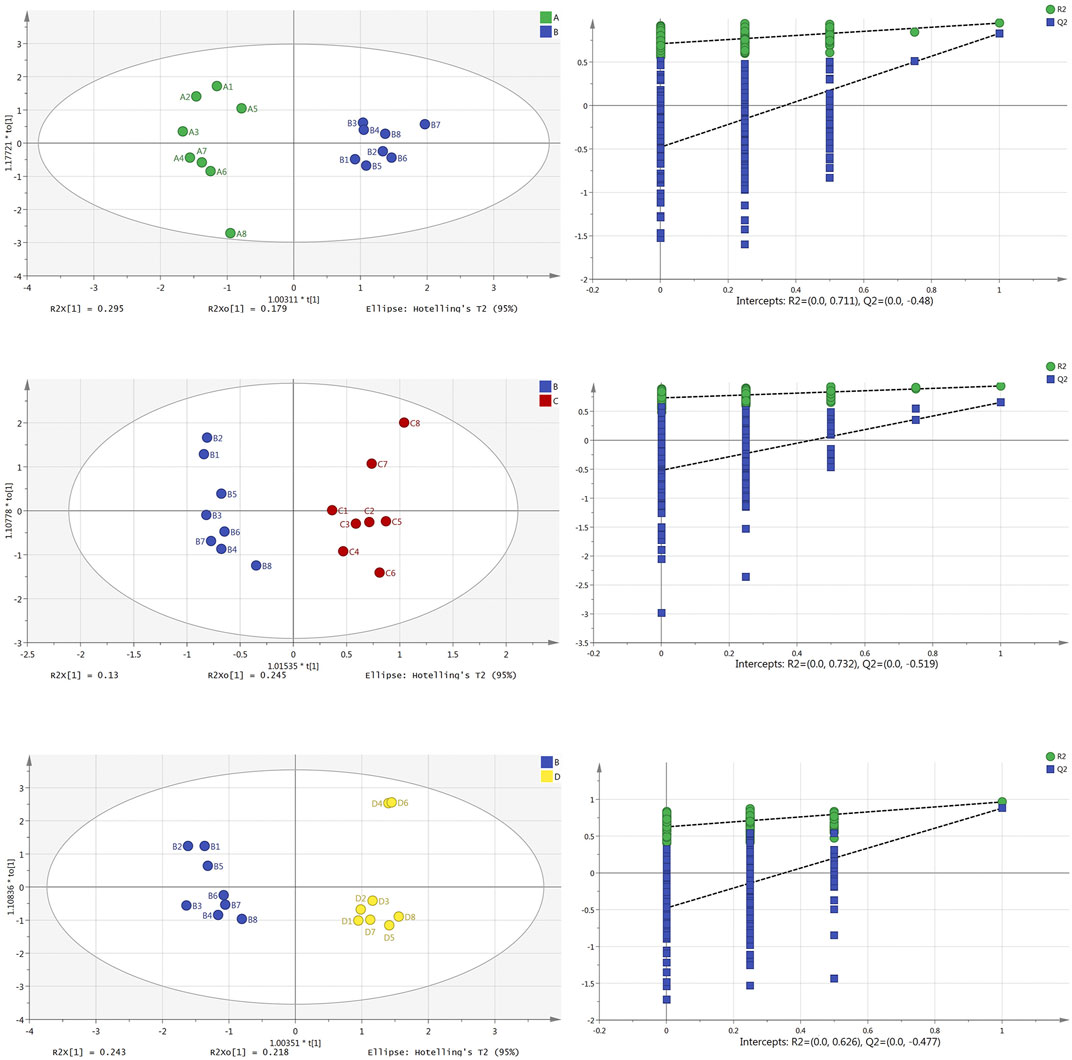
FIGURE 3. OPLS-DA scores plot and permutation test diagram of normal group (A), model group (B), Borneol essential oil group (C), and reference group (D) in plasma samples (n = 8).
Similarly, the parameter findings from brain tissue samples (Figure 4) show that all have good prediction abilities and are not overfitted. Main parameters: the OPLS-DA scores plot diagram of the normal group and model group (R2X = 0.421, R2Y = 0.973, Q2 = 0.483) and its permutation testing diagram (R2 = 0.707, Q2 = −0.422); the OPLS-DA scores plot diagram of the model group and Borneol essential oil group (R2X = 0.543, R2Y = 0.955, Q2 = 0.720) and its permutation testing diagram (R2 = 0.869, Q2 = −0.408); the OPLS-DA scores plot diagram of the model group and reference group (R2X = 0.513, R2Y = 0.907, Q2 = 0.639) and its permutation testing diagram (R2 = 0.898, Q2 = −0.307). Parameters indicate that those models are relatively stable, have good prediction abilities, and are not overfitted.
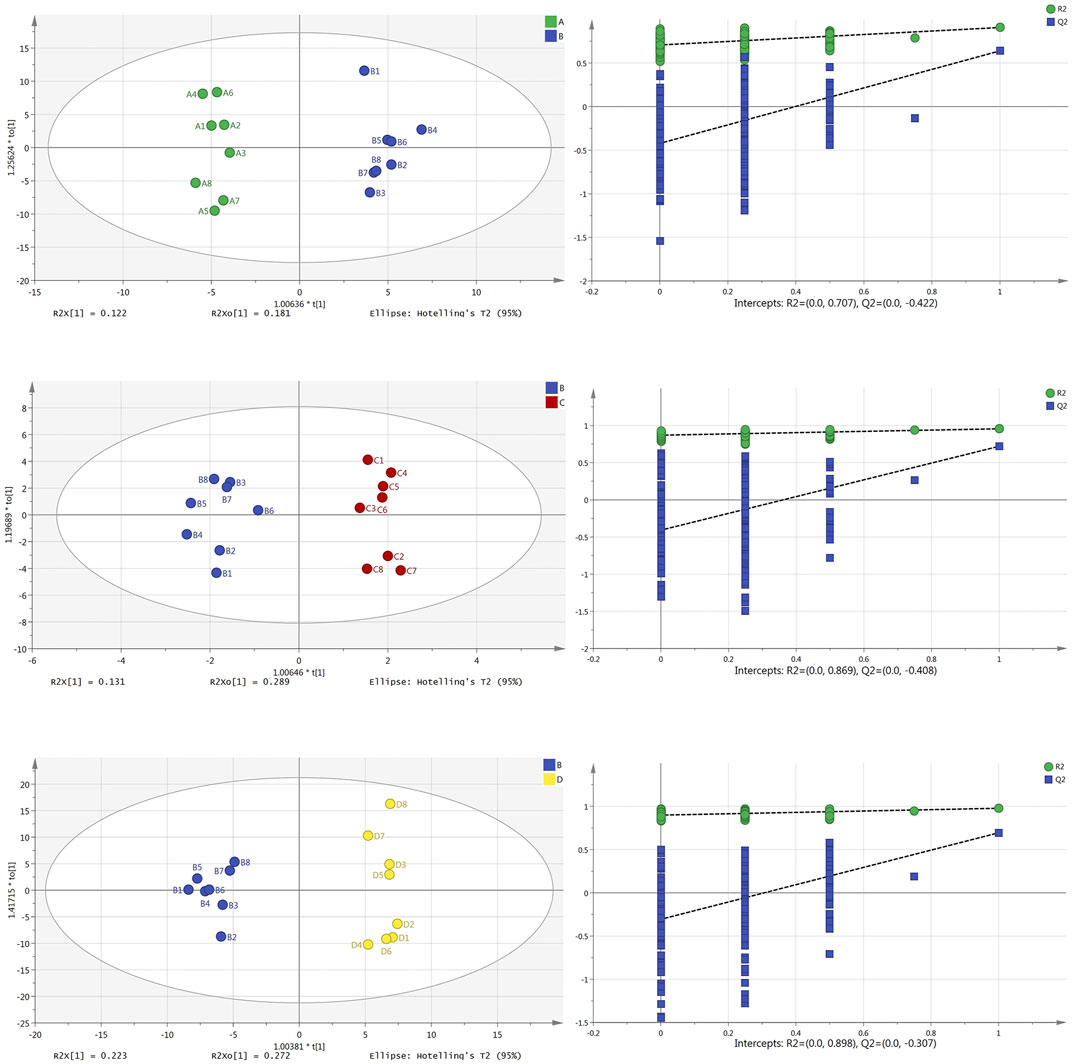
FIGURE 4. OPLS-DA scores plot and permutation test diagram of normal group (A), model group (B), Borneol essential oil group (C), and reference group (D) in brain tissue samples (n = 8).
Differential Metabolites
Under the above OPLS-DA model, according to VIP > 1 and p < 0.05, and referring to relevant research results to determine metabolites related to learning and memory. We find a total of 11 differential metabolites in plasma samples. There are five differential metabolites between the normal group and model group, five differential metabolites between the model group and Borneol essential oil group, and seven differential metabolites between the model group and the reference group. As shown in Figure 5, differential metabolites repeated amongst groups include glycine, azelaic acid, citraconic acid and adenine. Glycine and azelaic acid appear in all categories.
In brain tissue samples, 26 different metabolites in total were screened out. There were six differential metabolites between the normal group and the model group, nine differential metabolites between the model group and the Borneol essential oil group, and 22 differential metabolites between the model group and the reference group. From further analysis, we determined that differential metabolites repeated between multiple groups contained adenine, aspartic acid, D-serine, valine, glucose, ornithine, glutamic acid, lysine, and isoleucine. Adenine and aspartic acid appear in all categories, as shown in Figure 6. The specific data on differential Metabolites are shown in Supplementary Material.
Metabolic Pathway
The differential metabolites screened from plasma and brain tissue samples were put into the MetaboAnalyst 5.0 platform, and metabolic pathways related to learning and memory impairment were determined based on pathway impacts > 0.1 and p < 0.01 (−lgP > 2) (Liu et al., 2017). Results were shown in Figures 7, 8. Two metabolic pathways were found in plasma samples: phenylalanine, tyrosine and tryptophan biosynthesis, as well as phenylalanine metabolism. Six metabolic pathways are found in brain tissue samples: Phenylalanine, tyrosine and tryptophan biosynthesis; alanine, aspartate and glutamate metabolism; arginine biosynthesis; beta-alanine metabolism; glyoxylate and dicarboxylate metabolism; and aminoacyl-tRNA biosynthesis.
Discussion
In plasma and brain tissue samples, five metabolic pathways--including phenylalanine, tyrosine and tryptophan biosynthesis, phenylalanine metabolism, alanine, aspartate and glutamate metabolism, arginine biosynthesis, and beta-alanine metabolism--belong to amino acid metabolism in KEGG pathway. As the constituent unit of protein, amino acids are closely related to various physiological activities (Wu, 2009). In this study, different metabolites of amino acids such as glycine, aspartic acid and D-serine were found. Previous studies have found that the content of amino acid neurotransmitters such as glycine and aspartate would fluctuate in models of learning and memory impairment (Wu and Zhang, 2018). Madeira et al. (2015) also found that the levels of D-serine and serine racemase (the enzyme responsible for producing D-serine) in the learning and memory impairment model rats were higher than those in the control group. D-serine can act on N-methyl-D-aspartate receptors, trigger signal transduction in the central nervous system, and also plays an important role in synaptic plasticity, learning and memory (Feng et al., 2013).
Glyoxylate and dicarboxylate metabolism is a part of carbohydrate metabolism in the KEGG pathway. Sun et al. comprehensively analyzed the endogenous metabolites in the urine of Alzheimer’s disease rats treated with Shengmai-San (a compound common in traditional Chinese Medicine), and found that glyoxylate and dicarboxylate metabolism was involved (Yi et al., 2017). Some scholars carried out a study on the effects of long-term iron exposure on cognitive functioning in C57/BL6 mice. They also found that glyoxylate and dicarboxylate metabolism was one of the differential metabolic pathways in the intestinal microbial spectrum of the control group and iron treatment group (Wang et al., 2019). In this study, the differential metabolites involved in glyoxylate and dicarboxylate metabolism are glutamic acid and citric acid. The synaptic transmission process involved in glutamic acid has an important impact on learning and memory (Esposito et al., 2013; Reiner and Levitz, 2018). Studies have found that glutamic acid function fluctuates during development from mild cognitive impairment to Alzheimer’s disease, and that glutamic acid content first decreases and then increases (Zhang and Ai, 2018). Citric acid is a metabolic intermediate of mitochondrial tricarboxylic acid cycle, and many scholars believe that mitochondrial dysfunction is one of the pathogeneses of Alzheimer’s disease (Perez-Ortiz and Swerdlow, 2019). We found that, compared with the normal group, citric acid content in the model group was decreased, which may be related to the abnormal mitochondrial functioning seen in learning and memory impairment model mice.
Aminoacyl-tRNA biosynthesis belongs to the genetic information processing component of the KEGG pathway. Some metabonomic studies on patients with Alzheimer’s disease have shown that aminoacyl-tRNA biosynthesis is an important involved metabolic pathway for the disease (Trushina et al., 2013; Wang et al., 2020). Aminoacyl-tRNA synthase and translation factors are key elements required for protein biosynthesis (Ibba and Söll, 2004). Mutation in aminoacyl-tRNA synthase is related to a variety of diseases, including cancer and various neurodegenerative diseases (i.e., Parkinson’s disease) (Sauter et al., 2015; Kapur et al., 2017). The exact relationship between learning and memory impairment and aminoacyl-tRNA synthesis is not clear, but studies have found that aminoacyl-tRNA synthase is related to the biosynthesis of dinucleotide phosphate (Zamecnik et al., 1966). Dinucleotide phosphate can act as a neurotransmitter to stimulate the release of γ-aminobutyric acid in the central and peripheral nervous systems, and is involved in responses to oxidative stress and metabolic changes, which are closely related to the onset of learning and memory impairment (Gómez-Villafuertes et al., 2004).
This study explored the effect of Borneol essential oil on plasma and brain tissue metabolites in mice with learning and memory impairment, which was similar to some study of natural products in the treatment of Alzheimer’s disease. Xia et al. (2017) studied the effect of breviscapine on Alzheimer disease mice by using HPLC-QTOF-MS metabolomics method, and found 10 potential biomarkers in plasmas, such as indoleacrylic acid, C16 sphinganine, and sulfolithocholic acid. From metabolic pathways analysis, they thought breviscapine improved the learning and memory deficits, which might be related to adjusting phospholipids metabolism. Gong et al. (2015) used UHPLC–TOF/MS method to determine the endogenous metabolites of plasma samples, finding the content change of proline, valine, tryptophan and so on in Alzheimer disease mice, and the levels of these metabolites were recovered in different degrees after total ginsenosides administration. At present, our study found differential metabolites and involved metabolic pathways, and further work is the verification and validation of markers. In recent years, muti-omics technology has been relatively mature, and has made progress in some studies. For example, a research integrated transcriptomic, proteomic and epigenomic analyses of postmortem humanbrains to identify molecular pathways involved in Alzheimer disease. And the identification of this process highlighted potential epigenetic strategies for early-stage disease treatment (Nativio et al., 2020). In the future, we can use the muti-omics technology to explore the internal mechanism of Borneol essential oil to improve learning and memory impairment in mice.
In conclusion, we found 11 differential metabolites--including glycine, azelaic acid, citraconic acid, adenine, methionine, isoleucine, cholesterol, tyrosine, proline, phenylalanine and leucine--in plasma samples, and 26 different metabolites --including adenine, aspartic acid, D-serine, valine, glucose, ornithine, glutamic acid, lysine, isoleucine, lactic acid, citric acid, gluconic acid, α-alanine, β-alanine, cholesterol, citrulline, glycine, leucine, methionine, myo-inositol, myristic acid, oleic acid, phenylalanine, proline, tyrosine and uracil in brain tissue samples. These differential metabolites are involved in phenylalanine, tyrosine and tryptophan biosynthesis, phenylalanine metabolism, alanine, aspartate and glutamate metabolism, arginine biosynthesis, and beta-alanine metabolism, glyoxylate and dicarboxylate metabolism and aminoacyl-tRNA biosynthesis. Therefore, in concert with relevant databases and research, we deduce that Borneol essential oil may improve the learning and memory impairment in mice by regulating amino acid metabolism and changes in neurotransmitter composition.
Data Availability Statement
The raw data supporting the conclusion of this article will be made available by the authors, without undue reservation.
Ethics Statement
The animal study was reviewed and approved by Science and Technology Ethics Committee of Shanghai Jiaotong University.
Author Contributions
YT: project design, experiment. YW: programme guidance. XL: experiment. YL and DC: experiment and writing instruction.
Conflict of Interest
The authors declare that the research was conducted in the absence of any commercial or financial relationships that could be construed as a potential conflict of interest.
Publisher’s Note
All claims expressed in this article are solely those of the authors and do not necessarily represent those of their affiliated organizations, or those of the publisher, the editors and the reviewers. Any product that may be evaluated in this article, or claim that may be made by its manufacturer, is not guaranteed or endorsed by the publisher.
Supplementary Material
The Supplementary Material for this article can be found online at: https://www.frontiersin.org/articles/10.3389/fphar.2022.770411/full#supplementary-material
References
Esposito, Z., Belli, L., Toniolo, S., Sancesario, G., Bianconi, C., and Martorana, A. (2013). Amyloid β, Glutamate, Excitotoxicity in Alzheimer's Disease: Are We on the Right Track? CNS Neurosci. Ther. 19 (8), 549–555. doi:10.1111/cns.12095
Feng, Y. Q., Xiao, H., and Shi, Y. W. (2013). D-serine and Serine Racemases. Hans J. Biomed. 3 (2), 12–16. doi:10.12677/HJBM.2013.32003
Filiptsova, O. V., Gazzavi-Rogozina, L. V., Timoshyna, I. A., Naboka, O. I., Dyomina, Y. V., and Ochkur, A. V. (2017). The Essential Oil of Rosemary and its Effect on the Human Image and Numerical Short-Term Memory. Egypt. J. Basic Appl. Sci. 4 (2), 107–111. doi:10.1016/j.ejbas.2017.04.002
Gómez-Villafuertes, R., Pintor, J., Gualix, J., and Miras-Portugal, M. T. (2004). Gaba Modulates Presynaptic Signalling Mediated by Dinucleotides on Rat Synaptic Terminals. J. Pharmacol. Exp. Ther. 308 (3), 1148–1157. doi:10.1124/jpet.103.061564
Gong, Y., Liu, Y., Zhou, L., Di, X., Li, W., Li, Q., et al. (2015). A UHPLC-TOF/MS Method Based Metabonomic Study of Total Ginsenosides Effects on Alzheimer Disease Mouse Model. J. Pharm. Biomed. Anal. 115, 174–182. doi:10.1016/j.jpba.2015.07.007
Gupta, A., Singh, M. P., and Sisodia, S. S. (2018). A Review on Learning and Memory. J. Drug Deliv. Therap. 8 (2), 153–157. doi:10.22270/jddt.v8i2.1671
Ibba, M., and Söll, D. (2004). Aminoacyl-tRNAs: Setting the Limits of the Genetic Code. Genes Dev. 18 (7), 731–738. doi:10.1101/gad.1187404
Jia, L., Du, Y., Chu, L., Zhang, Z., Li, F., Lyu, D., et al. (2020). Prevalence, Risk Factors, and Management of Dementia and Mild Cognitive Impairment in Adults Aged 60 Years or Older in China: a Cross-Sectional Study. Lancet Public Health 5 (12), e661–671. doi:10.1016/S2468-2667(20)30185-7
Kapur, M., Monaghan, C. E., and Ackerman, S. L. (2017). Regulation of mRNA Translation in Neurons-A Matter of Life and Death. Neuron 96 (3), 616–637. doi:10.1016/j.neuron.2017.09.057
Liu, C. S., Cao, L. Q., Li, G. W., and Fu, J. (2015). Effects of Rosemary Essential Oil on Learning and Memory and Expression of Acetylcholinesterase in Hippocampal CA1 Region of C57BL/6 Mice. Chin. J. Gerontol. 35 (3), 736–738. doi:10.3969/j.issn.1005-9202.2015.03.077
Liu, Y., Chen, T., Qiu, Y., Cheng, Y., Cao, Y., Zhao, A., et al. (2011). An Ultrasonication-Assisted Extraction and Derivatization Protocol for GC/TOFMS-based Metabolite Profiling. Anal. Bioanal. Chem. 400 (5), 1405–1417. doi:10.1007/s00216-011-4880-z
Liu, Y. M., Wei, Z. Y., Chen, X., Wang, D. Y., and Wu, D. H. (2017). Metabonomic Study of Ischemic Stroke Based on GC-TOFMS Approach. J. Shanghai Jiaotong Univ. (Med. Sci.) 37 (2), 207–211. doi:10.3969/j.issn.1674-8115.2017.02.015
Madeira, C., Lourenco, M. V., Vargas-Lopes, C., Suemoto, C. K., Brandão, C. O., Reis, T., et al. (2015). D-Serine Levels in Alzheimer's Disease: Implications for Novel Biomarker Development. Transl Psychiatry 5 (5), e561. doi:10.1038/tp.2015.52
Nativio, R., Lan, Y., Donahue, G., Sidoli, S., Berson, A., Srinivasan, A. R., et al. (2020). An Integrated Multi-Omics Approach Identifies Epigenetic Alterations Associated with Alzheimer's Disease. Nat. Genet. 52 (10), 1024–1035. doi:10.1038/s41588-020-0696-0
Perez Ortiz, J. M., and Swerdlow, R. H. (2019). Mitochondrial Dysfunction in Alzheimer's Disease: Role in Pathogenesis and Novel Therapeutic Opportunities. Br. J. Pharmacol. 176 (18), 3489–3507. doi:10.1111/bph.14585
Phua, L. C., Koh, P. K., Cheah, P. Y., Ho, H. K., and Chan, E. C. (2013). Global Gas Chromatography/time-Of-Flight Mass Spectrometry (GC/TOFMS)-based Metabonomic Profiling of Lyophilized Human Feces. J. Chromatogr. B Analyt Technol. Biomed. Life Sci. 937, 103–113. doi:10.1016/j.jchromb.2013.08.025
Reiner, A., and Levitz, J. (2018). Glutamatergic Signaling in the central Nervous System: Ionotropic and Metabotropic Receptors in Concert. Neuron 98 (6), 1080–1098. doi:10.1016/j.neuron.2018.05.018
Sauter, C., Lorber, B., Gaudry, A., Karim, L., Schwenzer, H., Wien, F., et al. (2015). Neurodegenerative Disease-Associated Mutants of a Human Mitochondrial Aminoacyl-tRNA Synthetase Present Individual Molecular Signatures. Sci. Rep. 5, 17332. doi:10.1038/srep17332
Sun, F., Shi, M., Xu, S. S., Liang, K. K., and Xiang, W. H. (2019). Study on the Effect of Mint Compound Essential Oil Sniffing on Acetylcholinesterase in Patients with Mild Cognitive Impairment. China Pract. Med. 14 (10), 19–21. doi:10.14163/j.cnki.11-5547/r.2019.10.008
Trushina, E., Dutta, T., Persson, X. M., Mielke, M. M., and Petersen, R. C. (2013). Identification of Altered Metabolic Pathways in Plasma and CSF in Mild Cognitive Impairment and Alzheimer's Disease Using Metabolomics. PLoS One 8 (5), e63644. doi:10.1371/journal.pone.0063644
Wang, R., Satizabal, C. L., Beiser, A. S., Vasan, R. S., Decarli, C., Gerszten, R. E., et al. (2020). Circulating Metabolites Associated with Brain MRI Markers of Alzheimer's Disease. Alzheimer's Demen. 16 (S4). doi:10.1002/alz.044283
Wang, X., Zhang, J., Zhou, L., Xu, B., Ren, X., He, K., et al. (2019). Long-term Iron Exposure Causes Widespread Molecular Alterations Associated with Memory Impairment in Mice. Food Chem. Toxicol. 130, 242–252. doi:10.1016/j.fct.2019.05.038
Wang, X. F., and Kang, J. S. (2017). Protective Effect of Citronella Essential Oil on Senile Dementia Mice Induced by D-Galactose Combined with Aluminum Trichloride. Acta Neuropharmacologica 7 (2), 38–39. doi:10.3969/j.issn.2095-1396.2017.02.045
Wu, C. Y., and Zhang, D. S. (2018). Research Progress of Amino Acid Neurotransmitters, Nervous System Diseases and Their Therapeutic Drugs. Acta Neuropharmacologica 8 (6), 60.
Wu, G. (2009). Amino Acids: Metabolism, Functions, and Nutrition. Amino Acids 37 (1), 1–17. doi:10.1007/s00726-009-0269-0
Xia, H., Wu, L., Chu, M., Feng, H., Lu, C., Wang, Q., et al. (2017). Effects of Breviscapine on Amyloid Beta 1-42 Induced Alzheimer's Disease Mice: A HPLC-QTOF-MS Based Plasma Metabonomics Study. J. Chromatogr. B Analyt Technol. Biomed. Life Sci. 1057, 92–100. doi:10.1016/j.jchromb.2017.05.003
Yi, L., Liu, W., Wang, Z., Ren, D., and Peng, W. (2017). Characterizing Alzheimer's Disease through Metabolomics and Investigating Anti-alzheimer's Disease Effects of Natural Products. Ann. N. Y. Acad. Sci. 1398, 130–141. doi:10.1111/nyas.13385
Zamecnik, P. C., Stephenson, M. L., Janeway, C. M., and Randerath, K. (1966). Enzymatic Synthesis of Diadenosine Tetraphosphate and Diadenosine Triphosphate with a Purified Lysyl-sRNA Synthetase. Biochem. Biophys. Res. Commun. 24 (1), 91–97. doi:10.1016/0006-291x(66)90415-3
Keywords: essential oil of Cinnamomum camphora chvar. Borneol, learning and memory, metabonomics, differential metabolites, metabolic pathway
Citation: Tang Y, Lv X, Liu Y, Cui D and Wu Y (2022) Metabonomics Study in Mice With Learning and Memory Impairment on the Intervention of Essential Oil Extracted From Cinnamomum camphora Chvar. Borneol. Front. Pharmacol. 13:770411. doi: 10.3389/fphar.2022.770411
Received: 03 September 2021; Accepted: 23 February 2022;
Published: 10 March 2022.
Edited by:
Jia-Xu Chen, Beijing University of Chinese Medicine, ChinaReviewed by:
Wieslawa Agnieszka Fogel, Medical University of Lodz, PolandJiayu Zhang, Binzhou Medical University, China
Dehui Xu, Xi’an Jiaotong University, China
Copyright © 2022 Tang, Lv, Liu, Cui and Wu. This is an open-access article distributed under the terms of the Creative Commons Attribution License (CC BY). The use, distribution or reproduction in other forums is permitted, provided the original author(s) and the copyright owner(s) are credited and that the original publication in this journal is cited, in accordance with accepted academic practice. No use, distribution or reproduction is permitted which does not comply with these terms.
*Correspondence: Yani Wu, eW53dUBzanR1LmVkdS5jbg==