- 1Nantong Hospital of Traditional Chinese Medicine, Affiliated Traditional Chinese Medicine Hospital of Nantong University, Nantong, China
- 2Department of Occupational Medicine and Environmental Toxicology, Nantong Key Laboratory of Environmental Toxicology, School of Public Health, Nantong University, Nantong, China
- 3Department of Thoracic and Cardiac Surgery, Nanjing Brain Hospital, Nanjing, China
- 4Nantong Fourth People’s Hospital, Nantong, China
- 5Jiangsu Provincial Center for Disease Control and Prevention, Nanjing, China
Background: Ferroptosis is a novel process of programmed cell death driven by excessive lipid peroxidation that is associated with the development of lung adenocarcinoma. N6-methyladenosine (m6a) modification of multiple genes is involved in regulating the ferroptosis process, while the predictive value of N6-methyladenosine- and ferroptosis-associated lncRNA (FMRlncRNA) in the prognosis of patients remains with LUAD remains unknown.
Methods: Unsupervised cluster algorithm was applied to generate subcluster in LUAD according to ferroptosis-associated lncRNA. Stepwise Cox analysis and LASSO algorithm were applied to develop a prognostic model. Cellular location was detected by single-cell analysis. Also, we conducted Gene set enrichment analysis (GSEA) enrichment, immune microenvironment and drug sensitivity analysis. In addition, the expression and function of the LINC01572 were investigated by several in vitro experiments including qRT-PCR, cell viability assays and ferroptosis assays.
Results: A novel ferroptosis-associated lncRNAs-based molecular subtype containing two subclusters were determined in LUAD. Then, we successfully created a risk model according to five ferroptosis-associated lncRNAs (LINC00472, MBNL1-AS1, LINC01572, ZFPM2-AS1, and TMPO-AS1). Our nominated model had good stability and predictive function. The expression patterns of five ferroptosis-associated lncRNAs were confirmed by polymerase chain reaction (PCR) in LUAD cell lines. Knockdown of LINC01572 significantly inhibited cell viability and induced ferroptosis in LUAD cell lines.
Conclusion: Our data provided a risk score system based on ferroptosis-associated lncRNAs with prognostic value in LUAD. Moreover, LINC01572 may serve as a novel ferroptosis suppressor in LUAD.
Introduction
Lung cancer (LC) is the leading cause of cancer-related deaths globally (Bray et al., 2018). The number of patients with LUAD accounts for about 50% of all LC cases (Coudray et al., 2018; Chen et al., 2021). Most patients re at an advanced stage at the time of diagnosis, with dismal survival outcome. (Denisenko et al., 2018). LUAD is treated by surgery, radiotherapy and targeted therapy. Targeted therapy with its precise and efficient characteristics has played central part in clinical treatment (Imielinski et al., 2012). Therefore, it is crucial to find new therapeutic targets to improve the treatment of LUAD.
Long non-coding RNAs (lncRNAs) are transcripts of more than 200 nucleotides, and according to current reports, lncRNAs have no direct transcriptional ability to encode proteins (Peng et al., 2017; Geng et al., 2022). However, essential effects of lncRNAs in regulating RNA transcription, translation and protein dynamics have now been identified, and there is increasing evidence that lncRNAs have different roles in the pathogenesis of cancer (Ma et al., 2018; Song et al., 2021a). Researchers have recently used lncRNA microarrays, lncRNA sequencing, and qRT-PCR to identify lncRNAs that are differentially expressed in tumor tissues. Long non-coding RNA MALAT1 was upregulated in gastric carcinoma and positively regulates autophagy in multiple cancers (Wang et al., 2021; Gu et al., 2022). The levels of HOTAIR in metastatic breast cancer tissues were higher than normal breast epithelium and primary breast cancer foci, and high HOTAIR expression was associated with poorer prognosis in patients and with metastasis in the course of the disease (Gupta et al., 2010; Liu et al., 2022a).
N6-methyladenosine (m6A) is the most abundant epigenetic modification in eukaryotic mRNA and non-coding RNA, and this chemical modification process is dynamic and reversible (Ma et al., 2019; Liu et al., 2022b). It is generally accepted that m6A modifications are regulated by three proteins, including “writers,” “erasers,” and “readers” (He et al., 2019). LncRNA-PACERR, a crucial regulator of TAMs in the PDAC microenvironment, could enhance the expression of KLF12 in an m6A-dependent manner, thereby promoting cell viability and metastasis (Liu et al., 2022c). FTO mediates the m6A modification of LINC00022 and boost ubiquitination-mediated degradation of p21 to promote tumor growth in ESCC in vivo (Cui et al., 2021).
Ferroptosis, a subtype of programmed cell death, can be regulated through m6A methylation to maintain cell cycle and tissue homeostasis (Song et al., 2021b; Shen et al., 2021). Ferroptosis is mainly characterized by iron accretion and lipid peroxidation (Mou et al., 2019). Ma et al. (2021) found that m6A reader YTHDC2 can inhibit LUAD tumorigenesis by SLC7A11-dependent antioxidant function (Liu et al., 2021). In gastric cancer, lncRNA CBSLR interacts with YTHDF2, which reduces the expression of CBS mRNA, contributing to iron death resistance (Yang et al., 2022). In this study, we identified a group of specific lncRNAs associated explicitly with the prognostic status of LUAD. Moreover, these lncRNAs can further evaluate the guiding value of immune efficacy, immune infiltration, drug sensitivity, and biological function for clinical treatment.
Materials and methods
Data collection
The transcriptome data of LUAD cases were obtained from TCGA (https://portal.gdc.cancer.gov/). Patients with missing survival information were excluded. The FRGs were downloaded from FerrDb (http://www.zhounan.org/ferrdb/current/). In addition, we extracted the gene set for m6A regulators from previous literature (Du et al., 2021).
Determination of ferroptosis and m6A related lncRNA (FMRlncRNA)
Pearson correlation analysis was used to screened out lncRNAs related to PRGs or m6A regulators The association was considered significant if the correlation coefficient |R2| > .4 at p < .001. Differentially expressed lncRNAs (DElncRNAs) were selected by the “limma” package (Ritchie et al., 2015).
Unsupervised gene clustering
Consensus clustering was applied with “ConsensusClusterPlus” package (Wilkerson and Hayes, 2010). To identify the favorable cluster value, the Delta area and cumulative distribution function (CDF) were estimated. Then we compared the clinical outcomes among subtypes using survival analysis.
Development of FMRlncRNA model
We randomly classified the included cases (n = 500) into training and validation cohorts at a 1:1 ratio. The model was generated by stepwise Cox regression and LASSO algorithm. The risk score of each case with LUAD was evaluated according to the following formula:
All patients were classified by the median risk score into high- and low-risk groups.
Survival analysis
The discrepancies in clinical outcome between groups were examined by Kaplan-Meier survival analysis. The reliability of model in outcome assessment was investigated by drawing ROC curves. The independent value of model in LUAD was verified via Cox relevant analyses.
Single-cell analysis
The single-cell data set GSE123904 of LUAD was collected from the GEO database. We applied “Seurat” package to conduct data quality control and integration (Mangiola et al., 2021). The PCA analysis and t-SNE algorithm were utilized to determine cell subclusters. Using “FindAllMarkers” to obtain the specific biomarker of different cell population.
Gene set enrichment analysis (GSEA)
We chose the Hallmark and KEGG as the reference gene sets. Then 1000 enrichment analyses were done with the default weighted method. Any gene set with FDR < .25 and p < .05 was regarded as significant.
Immune activity analysis
Five bioinformatics algorithms (CIBERSORT, ESTIMATE, MCPcounter, ssGSEA, and TIMER) were applied to detect immune responses between two group. Additionally, we employed ssGSEA to evaluate the immunocyte infiltrating as well as immune function between two groups.
Drug sensitivity analysis
The effect of chemotherapy was evaluated by Genomics of Drug Sensitivity in Cancer (GDSC8). The half-maximal inhibitory concentration (IC50) was estimated which represented the drug response.
Cell culture and transfection
The LUAD cell lines (A549 and NCI-H2009) and bronchial epithelioid cells (HBE) were obtained from shanghai. The LUAD cells were cultured in RPMI-1640 medium and maintained in a humidified incubator at 37°C in 5% CO2. The silencing RNA against LINC01572 (si-LINC01572) were synthesized and purchased from RIBBIO (Guangzhou, China). The sequences of si-LINC01572 were shown as Supplementary Table S1. Lipofectamine 3000 (Invitrogen) was used to transfect siRNA and its negative control.
CCK8 assay
5,000 cells per well were seeded in a 96-well plate to measure cell viability. Each well was replaced with fresh DMEM containing 10 µl of Cell Counting Kit-8 (CCK8) reagent. After 4 h of incubation at 37°C, the absorbance of each well was measured at 450 nm.
EdU assay
We utilized Ribobio’s Edu staining kit to assess cell proliferation. 5000 cells were seeded in a 96-well plate. EdU solution (25 μM) was added to the well plate for 2 h the next day. Afterward, cells were fixed in 4% paraformaldehyde for 30 min, followed by 50 μl, 2 mg/ml glycine for 5 min. After incubation with 100 μl .5% Triton X-100, cells were incubated with 100 μl 1× Apollo® 567 fluorescent staining solution for 30 min in a dark environment. The nuclei of the cells were stained with DAPI. Finally, the images were observed with an inverted fluorescence microscope.
Reverse transcription-polymerase chain reaction (PCR)
Total RNA was extracted from cells using Trizol reagent according to the manufacturer’s instructions. RNA was then reverse-transcribed to cDNA with Primer-script Master Mix (Takara Bio, RR0236-1, Kusatsu, Japan). Quantitative PCR was performed with SYBR Green I Master Mix (Takara Bio, Q34-02, Kusatsu, Japan). Supplementary Table S1 displays primer sequences of all genes. The 2−ΔΔCt method was adopted for calculating relative gene expression, with GAPDH being the endogenous control.
Determination of lipid peroxidation and iron content
Lipid peroxidation detection kits (Abcam) were used to evaluate the concentrations of the lipid peroxidation products MDA and 4-HNE. To investigate the degree of iron deposit, an iron assay kit (Abcam) was used for detection in cell lysates according to the manufacturer’s instructions. The results were measured using a microplate reader.
Statistical analysis
All statistical data were analyzed using GraphPad 9.4 and the R software version 4.0.
Results
Ferroptosis and m6A-related lncRNA (FMRlncRNA) identification and unsupervised cluster analysis
The workflow of our research is shown in Figure 1.
Firstly, a total of 3219 DElncRNA were determined between LUAD specimens and normal control. Then, we screened 601 intersecting DEFMRlncRNAs for the next analysis (Figure 2A). Based on the 601 DEFMRlncRNAs, unsupervised cluster analysis suggested the ideal value of subcluster was two (Figure 2B). Survival analysis indicated that cluster1 had a notably better survival outcome than cluster2 (Figure 2C). Besides, there were also large differences in immunocytes between the two clusters (Figure 2D). We also tested whether the four immune checkpoints (PD-L1, CD276, CTLA4, LAG3), which differ in tumor and normal tissues, differed in two clusters, showing that CD276 expression was higher in cluster2 than in cluster1, while CTLA4 expression was lower than in cluster1. PD-L1 and LAG3 showed no significant difference in expression between the two clusters (Figure 2E).
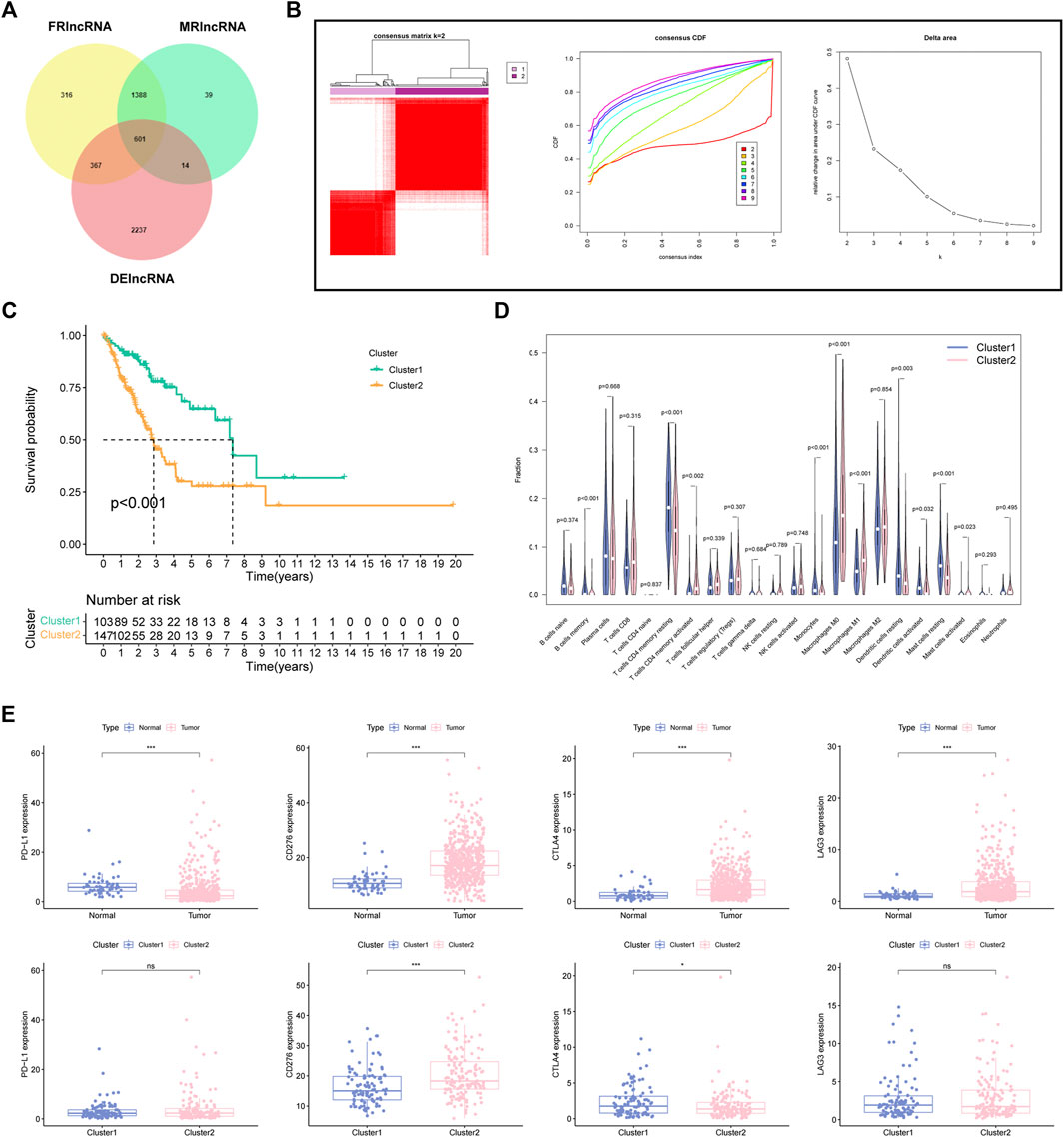
FIGURE 2. Determination of FMRlncRNA molecular subtype in LUAD (A) The Venn plot of intersection DElncRNAs. (B) Consensus clustering results. (C) Kaplan–Meier survival analysis, (D) immune cell differential analysis for patients between two subclusters. (E) Immune checkpoint analysis (ns > .05, *p < .05, ***p < .001).
Establishment of FMRlncRNA model (FMRLM)
All LUAD samples were equally divided into the training (n = 250) and validation groups (n = 250). In the training group, based on univariate Cox, we found 37 FMRlncRNAs associated with survival outcome on the basis of univariate Cox (Figure 3A). To avoid over-fitting prognostic features, we performed LASSO regression (Figure 3B). Finally, a risk score system containing (LINC00472, MBNL1-AS1, LINC01572, ZFPM2-AS1, and TMPO-AS1) was generated by multivariate analysis. The risk model equation was: (1.754 × LINC01572) + (2.131 × TMPO-AS1) + (1.142 × ZFPM2-AS1) + (−2.366 × LINC00472) + (−1.351 × MBNL1-AS1). According to GEPIA2 portal, we found that there was a remarkable difference in expression between tumor and normal samples (Figure 3C). In addition, the expression of LINC01572 and TMPO-AS1 differed significantly between tumor stages, with higher expression associated with higher tumor stage (p < .05) (Figure 3D). Survival curves illustrated the prognostic value of each signature FMRlncRNAs (Figure 3E).
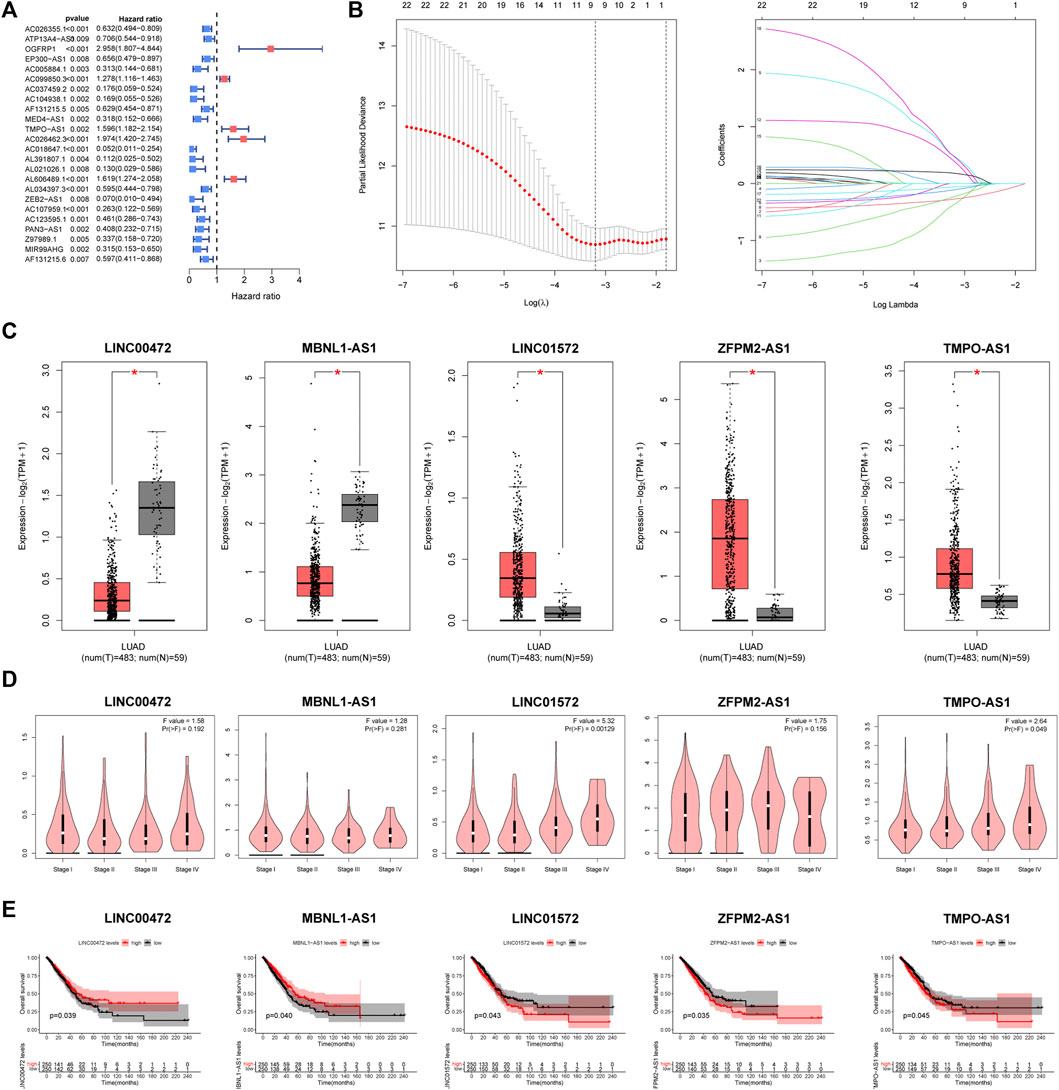
FIGURE 3. Creation process of the FMRLM (A) Univariate Cox regression analysis. (B) LASSO regression for avoiding overfit of the signature. (C) Analysis of differential expression of 5 lncRNAs in tumor and normal tissues, (D) relationship with patient tumor stage, and (E) impact on survival prognosis (*p < .05).
Single-cell RNA analysis
There were 26 FRGs and 6 MRGs associated with the 5 lncRNAs involved in the signature (Supplementary Table S2). Survival curves revealed the prognostic value of 11 genes. Among them, patients with high expression of ARNTL, IL33, TUBE1, YTHDC2 displayed a favorable outcome, while cases with high expression of AURKA, BACH1, FANCD2, HELLS, RRM2, HNRNPA2B1, RBM15 had a dismal clinical outcome (Figure 4).
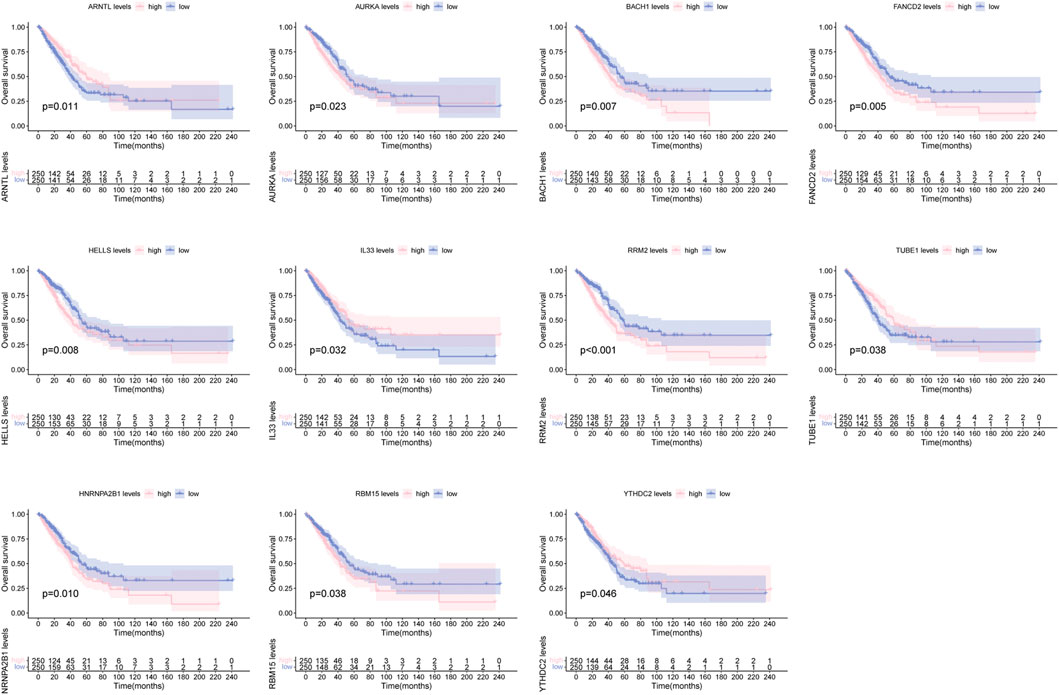
FIGURE 4. Survival curve of clinical survival for patients between groups based on 11 ferroptosis and m6A related genes.
In order to unearth the cellular location of above 11 genes, single-cell RNA analysis was applied. The GSE123904 dataset was first divided into 33 cell clusters (Figure 5A). In Figure 5B, a total of eight types of cell subpopulation were determined based on cell markers. In addition, we analyzed the malignancy of the epithelial cells (Figure 5C). The cellular location landscape of 11 genes in all cell population and epithelial cells was shown as in Figures 5D, E.
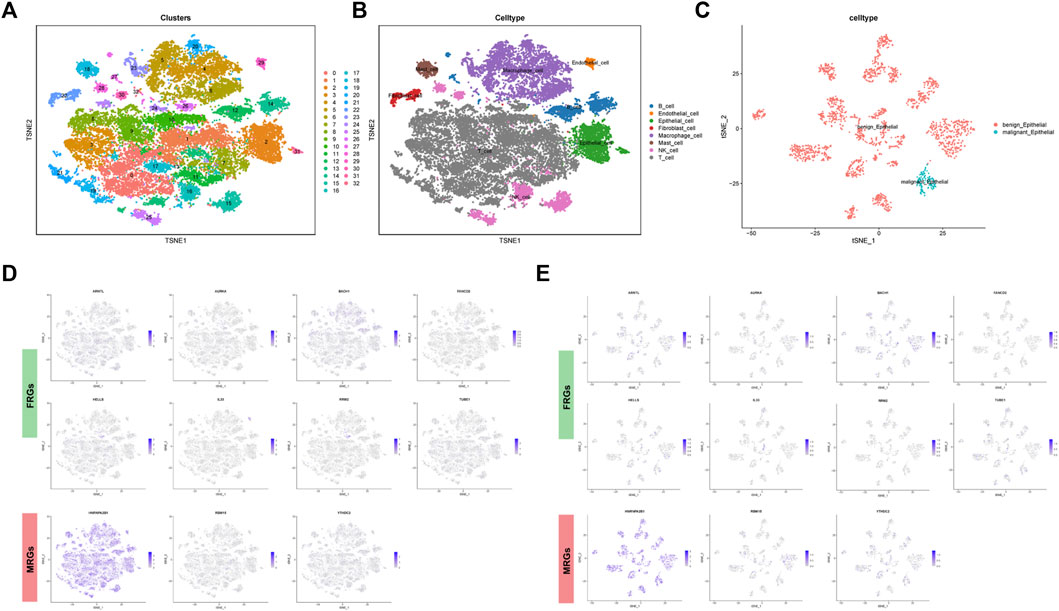
FIGURE 5. Single cell sequencing analysis. (A) Dimensionality reduction and cluster analysis. (B) Cell population annotation. (C) Classification of epithelial cells into benign and malignant epithelial cells. (D) Cellular location of FRGs and MRGs in all cells and in (E) epithelial cells.
Prognostic performance of FMRLM
In Figure 6A, the high-FMRLM group presented a dismal outcome in three LUAD cohorts (Figure 6A). In terms of AUC, the 1-, 3-, and 5-year AUCs were .781, .830, and .875 for the train set, .702, .601, and .664 for the test set, and .748, .718 and .783 for the whole group, respectively (Figure 6B), and our risk scores distinguish patients well (Figure 6C).
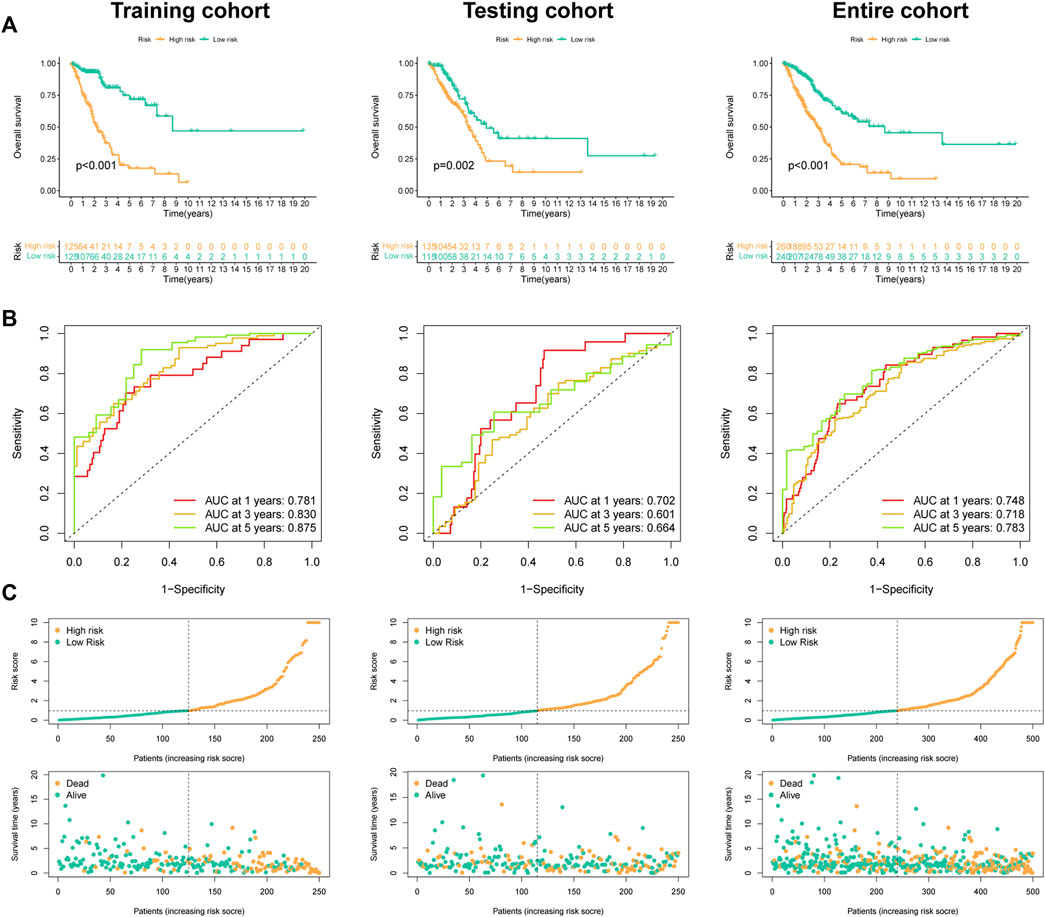
FIGURE 6. Predictive ability of the FMRLM. (A) Survival analysis between two risk groups in the train, test, and all sets, respectively. (B) ROC curves analysis. (C) Exhibition of FMRLM on risk score and survival status between two groups in three cohorts.
Independent prognosis analysis of FMRLM
Univariate Cox regression disclosed that FMRLM was greatly meaningful in three cohorts (Figure 7A). After employing multivariate regression, the FMRLM was also an independent prognostic index of LUAD (Figure 7B). ROC curves illustrated the FMRLM had better predictive ability than other clinical variables (Figure 7C).
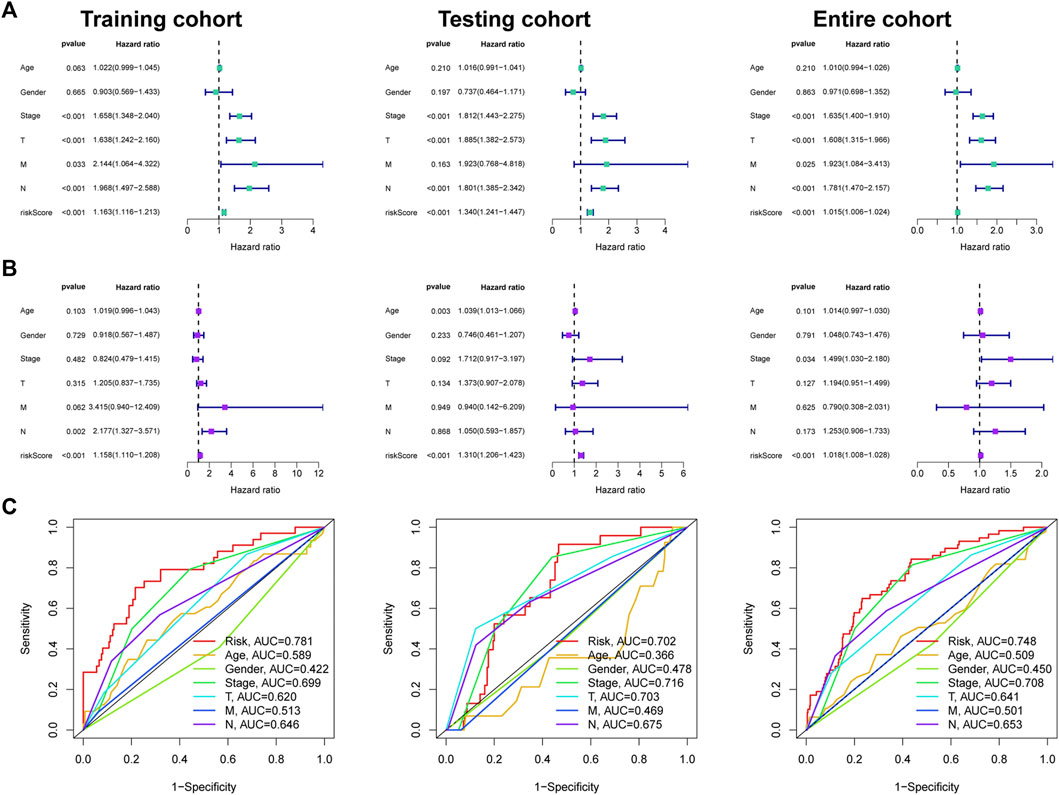
FIGURE 7. Independent prognosis analysis. (A) Univariate and (B) multivariate Cox regression analysis of clinical factors and risk score with survival outcome. (C) ROC curves analysis.
Immune microenvironment analysis
We analyzed the infiltration level of immunocytes of two groups using data from several platforms (Figure 8A). The results showed that B cells memory and Macrophages M0 were enriched in low-FMRLM group, while dendritic cells resting, Macrophages M2, Monocytes, and NK cells activated were enriched in high-FMRLM group (Figure 8B). Also, we observed that the low-FMRLM group had more prosperous immune functions such as cytolytic activity, HLA, T cell co-stimulation, and type II IFN response (Figure 8C), and several genes associated with sensitivity to radiotherapies such as FLT3, EZH2, TBX5, MET, and KIT had different expression between two subgroups (Figure 8D). CD160 and CTLA4 are highly expressed in the low-FMRLM group, while CD276 and TNFSF9 are more highly expressed in the high-FMRLM group (Figure 8E), and the expression of several m6A regulators (YTHDF2, FTO, HNRNPC, YTHDC2, and METTL3) differed between two subgroups (Figure 8F).
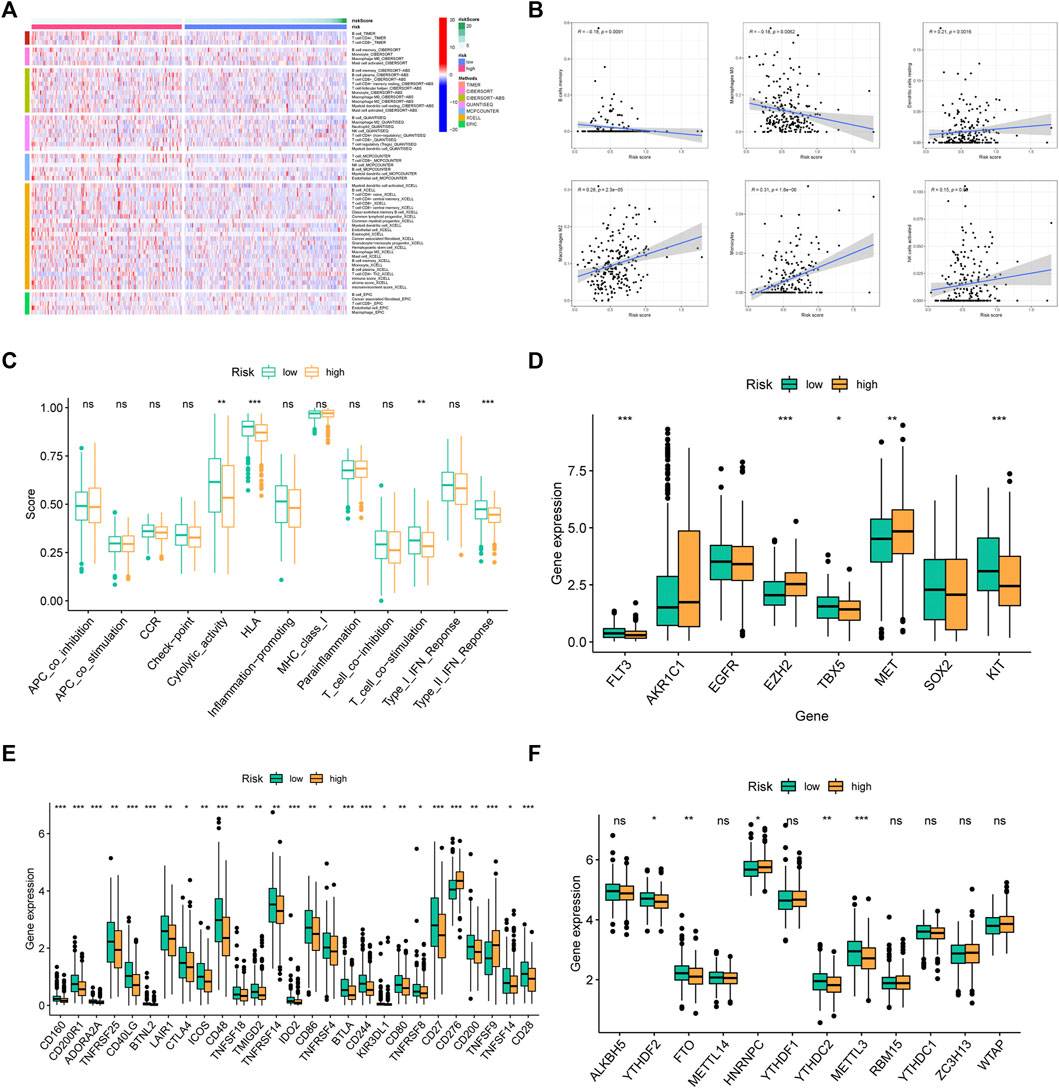
FIGURE 8. Immune microenvironment analysis. (A) The immune cell differential expression analysis of risk groups. (B) The correlation between risk score and immune cells. (C) Analysis of differences in immune functions, (D) chemosensitivity-related genes, (E) immune checkpoints, and (F) m6A regulators between risk groups (ns > .05, *p < .05, **p < .01, ***p < .001).
Drug sensitivity analysis
As revealed in Figure 9, Cisplatin, Docetaxel, Gemcitabine, Lapatinib, and Paclitaxel had a higher IC50 in low-FMRLM group, and their IC50 values were negatively correlated with the risk score, suggesting that patients with high risk were more sensitive to them. Rapamycin, on the other hand, had a higher IC50 in high-FMRLM group (Figures 9A, B).
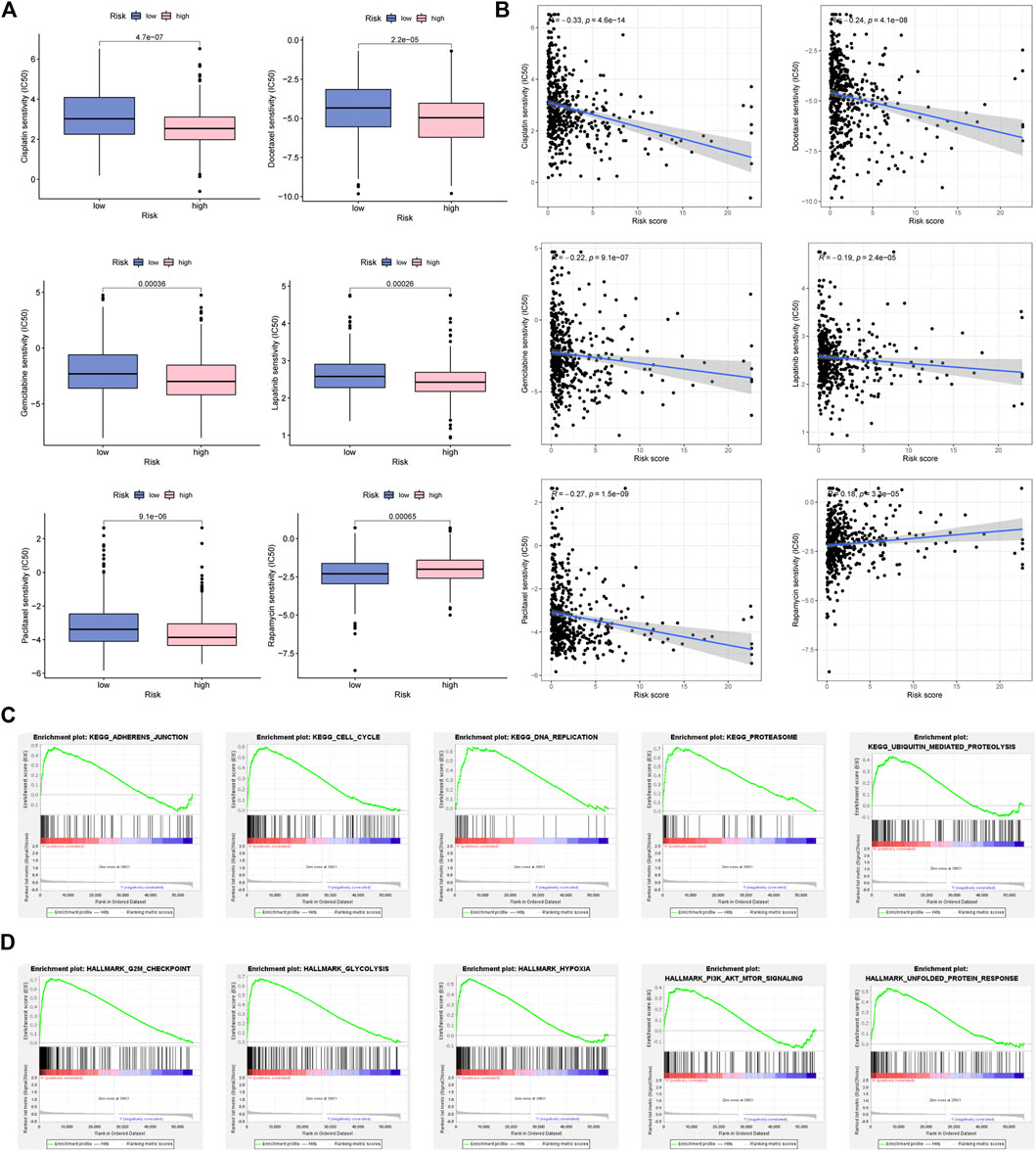
FIGURE 9. Drug sensitivity analysis and GSEA enrichment. (A) Differences and (B) correlation analysis between risk score and drug IC50. (C) KEGG and (D) Hallmark enrichment.
GSEA of FMRLM
To unearth the underlying function and pathways of signature, GSEA enrichment was conducted. In Figure 9C, we observed that cell cycle, DNA repair and ubiquitination were greatly enriched in high-risk cohort. In terms of Hallmark in tumor, glycolysis, hypoxia and PI3K/AKT/MTOR were activated in high-risk cohort (Figure 9D).
Downregulation of LINC01572 induced cell ferroptosis
PCR results suggested that LINC00472 and MBNL1-AS1 presented lower expression in LUAD cell lines, whereas LINC01572, ZFPM2-AS1, and TMPO-AS1 were highly expressed in A549 and H2009 cell lines (Figure 10A). Figure 10B illustrated favorable knock-down efficiency of LINC01572 in two cell lines. CCK8 assay indicated a remarkable decline in the cell viability with si-LINC01572 compared to the NC group (Figure 10C), with the same similar results for EdU assay (Figure 10D). Based on the cellular MDA, 4HNE and iron assays, we observed that silencing LINC01572 could promote cell ferroptosis of A549 and H2009 cell lines (Figures 10E–H).
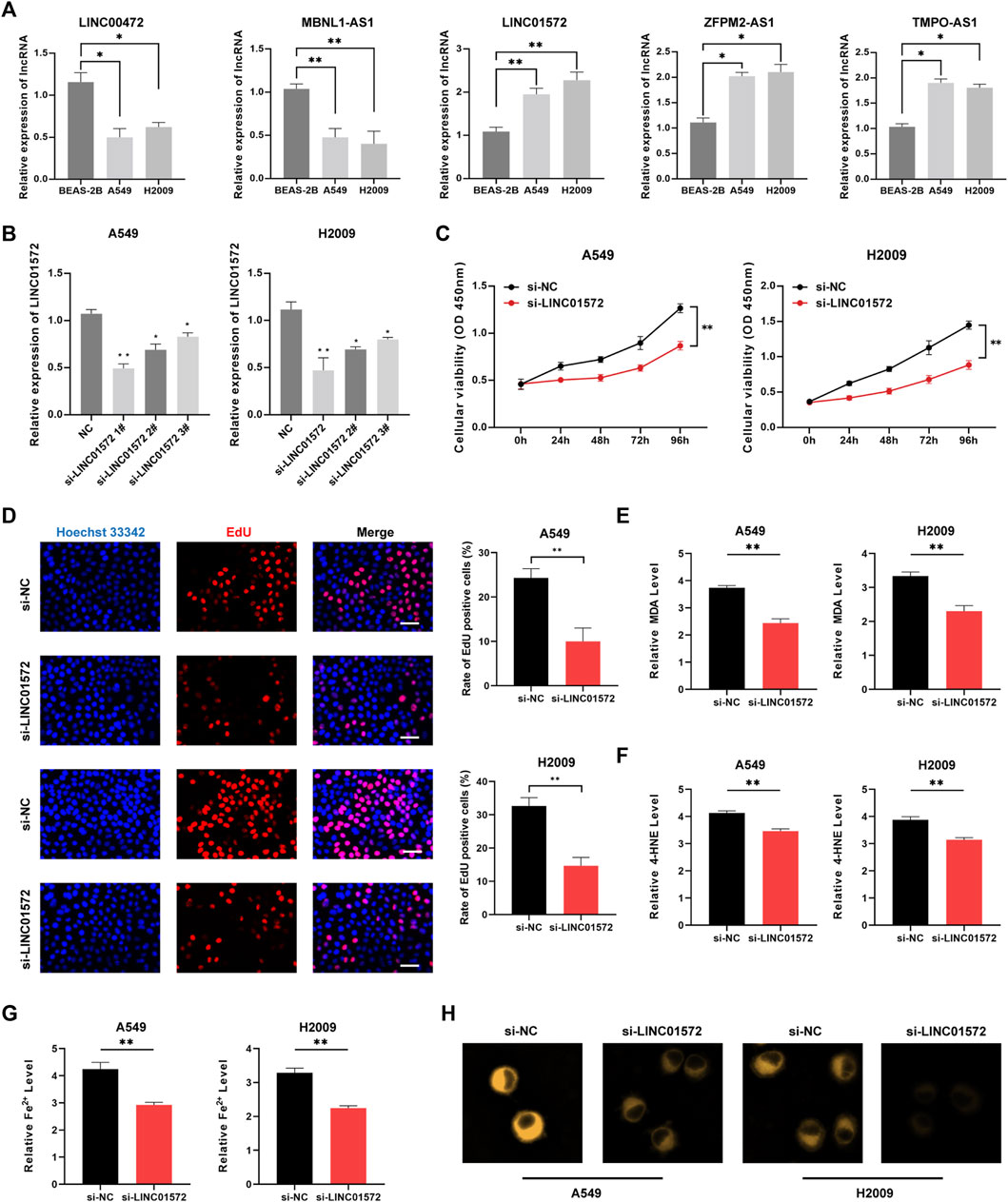
FIGURE 10. Downregulation of LINC01572 induced cell ferroptosis. (A) Expression patterns of five lncRNAs in different cell lines by qRT-PCR. (B) LINC01572 A was successfully knocked down in LUAD cell lines (C) CCK8 assay, (D) EdU assay, (E) MDA assay, (F) 4HNE assay and (G,H) cellular iron assay in different treatment groups (*p < .05, ***p < .001).
Discussion
Iron ions play a central part in the facilitation of the process of ferroptosis as the most essential nutrient for tumor cell survival. Consequently, anti-tumor by inducing cellular ferroptosis has become a hot research topic in recent years. RNA methylation has recently been reported to regulate ferroptosis in gastric cancer, suggesting combination of methylation modification and ferroptosis might be therapy target in tumor management (Yang et al., 2022).
The risk signature established in this study consists of 5 lncRNAs, among which LINC01572, TMPO-AS1 and ZFPM2-AS1 are risk lncRNAs, and LINC00472 and MBNL1-AS1 are protective lncRNAs. There is a lack of systematic studies of LINC01572 in LUAD. As suggested by Chen et al. (2017), LINC01572 is upregulation in LC and its expression level can distinguish between early and advanced stage. The expression of LINC01572 in the blood of cisplatin resistant gastric cancer patients is significantly increased, and it may produce chemotherapy resistance through the mechanism of inducing autophagy (Song et al., 2020). Numerous reports demonstrated that TMPO-AS1 has been revealed to be a key biomarker for evaluating the prognosis of LUAD (Li et al., 2016; Wang et al., 2019). ZFPM2-AS1 is an oncogene in gastric cancer (Sasa et al., 2022), but it has also been shown to be involved in the regulation of LUAD cell growth, which can be used as a new potential target for LUAD treatment (Han et al., 2020). As a potential lncRNA in human LUAD, LINC00472 has been proved to be a tumor suppressor, which could suppress LUAD cells viability (Sui et al., 2016). MBNL1-AS1 is a crucial tumor regulator and plays a negative regulatory role in a variety of tumors including lung cancer (Cao et al., 2020).
Immunosuppressive cells such as tumor-associated macrophages (TAM), cancer-associated fibroblasts (CAF), and neutrophils can be tumor-modified to produce a tumor-supportive microenvironment (Quail and Joyce, 2013; Chu et al., 2021). In our research, patients with high risk had significantly higher proportions of immunosuppressive cells. Macrophages act as scavengers, regulating the immune response to pathogens and maintaining tissue homeostasis. Immunotherapies and therapeutic strategies aimed at reducing the proportion of M2 macrophages or converting M2 macrophages to M1 macrophages have been proposed to suppress tumor survival (Sica et al., 2006). Several studies have shown that CAFs can promote tumor growth in several ways: secreting ECM proteins, inducing inflammation and angiogenesis, altering the metabolism and epigenome of cancer cells, establishing immunosuppression, conferring therapeutic resistance, and radiation protection (Mhaidly and Mechta-Grigoriou, 2021). Neutrophils are important intrinsic immune cells for the body’s antibacterial defense. In recent years, an elevated neutrophil-to-lymphocyte ratio has been recognized as a poor prognostic indicator of overall survival in cancer patients. Neutrophils form a sticky reticulum called neutrophil extracellular trap (NET) that has been shown to be involved in tumor metastasis (Erpenbeck and Schön, 2017).
One extremely promising approach to achieving tumor immunotherapy is to block the immune checkpoint by which tumor disguise themselves as normal cells. To date, immune checkpoint blocking drugs targeting CTLA-4 and PD-L1 have been used in the clinic and represent a milestone in antitumor therapy (Topalian et al., 2016). In the present study, CD27 was significantly less expressed in the low-FMRLM group. CD27 is a member of the tumor necrosis factor receptor superfamily and, in combination with its natural ligand CD70, activates the differentiation of T cells into effector and memory T cells and thus has potential as an immunomodulatory target in cancer therapy (Starzer and Berghoff, 2020). Moreover, among the immune checkpoints we examined, CD276 and CD28 belong to the B7 and CD28 families, representing immune signaling of tumors and immune cells, respectively.
The human leukocyte antigen (HLA) is a highly genetically polymorphic group of closely linked genes that control intercellular recognition and regulate the immune response. As an independent factor in tumor-associated antigen presentation, HLA-I plays an important role in antitumor immune response and neoplastic tumor progression. CD8+T cell-dependent killing of cancer cells requires HLA-I molecules for efficient tumor antigen presentation (Chowell et al., 2018). The absence of HLA class I molecules on the tumor cell surface is a major obstacle to the success of T cell-mediated immunotherapy (Garrido, 2019). The interferon-stimulated response element (ISRE) of all classical HLA-I genes mediates IFN-γ-induced transactivation, and of the non-classical HLA-I molecules, only the ISRE of HLA-F mediates IFN-γ induction (Gobin et al., 1999).
As is known, m6A modification is one of the emerging frontiers of research, and its modifying function has been linked to the development and progression of many human diseases, including lung cancer (Wang et al., 2020). In our m6A regulator expression analysis, METTL3, FTO, and YTHDC2 were significantly differentially expressed among two groups. It has also been shown that these three m6A regulators are associated with LUAD growth and prognosis. METTL3, YTHDC2 are upregulated in LUAD and promote LUAD growth (Zhang et al., 2020; Zhang et al., 2022a; Xu et al., 2022). Downregulation of YTHDC2 is associated with poor clinical outcome (Ma et al., 2021). As revealed by Ning et al. (2022), FTO is lowly expressed in poor prognosis LUAD samples and has predominantly antitumor activity.
To further test the speculation, we analyzed the pattern of Chemoradiotherapy sensitivity genes. Our data suggested FLT3 and KIT were upregulated in low-FMRLM group. It has been shown that Imatinib mesylate treatment of advanced melanoma yielded significant clinical responses in patients with KIT gene mutations (Carvajal et al., 2011). SNHG17 epigenetically represses LATS2 expression by recruiting EZH2 to the promoter region of LATS2, exacerbating the malignant phenotype of gefitinib-resistant LUAD cells (Zhang et al., 2022b). CAPN1 could inhibit the stability of c-Met, which in turn confer chemotherapy resistance to LUAD cells (Chen et al., 2020).
In GSEA enrichment studies between two groups were enriched for the characteristics of malignancy: Glycolysis, Hypoxia, PI3K/AKT/MTOR signaling. PI3K signaling pathway is essential for cell growth Overactivated in many cancer types, possible mechanisms by which the PI3K/AKT/mTOR axis promotes oncogenic transformation include stimulation of proliferation, survival, metabolic reprogramming, invasion, metastasis, inhibition of autophagy and senescence (Liu et al., 2022d). Glycolysis is increasingly being revealed as a marker of tumor progression. The possible pro-cancer mechanism is that induced glycolysis and increased glucose uptake promote lipid, protein, and nucleotide production, thereby promoting tumor cell proliferation and division. Multiple genes associated with glycolysis have been reported to be involved in cancer progression and LUAD is no exception (Zhou et al., 2019). Similarly, hypoxia has been identified as a factor in tumor progression and poor prognosis. Interestingly, hypoxia induces a metabolic shift from oxidative phosphorylation to glycolysis and increases glycogen synthesis, and this metabolic reprogramming favors tumor growth (Li et al., 2020).
Nevertheless, there are still several issues to be addressed. First, the signature was created according to patient information downloaded from public databases, which has the disadvantage of being limited and incomplete, and the restriction of selection bias. Second, our study lacks further validation with wet lab experiments.
Conclusion
In summary, we successfully created a robust risk score system based on FMRncRNAs. Our data highlights the prognostic value and possible clinical potency of FMRLM, which may serve as the therapeutic target for LUAD.
Data availability statement
The original contributions presented in the study are included in the article/Supplementary Material, further inquiries can be directed to the corresponding authors.
Author contributions
LH and XZ visualized the study and took part in the study design, and performance. XW, FW, and WS performed bioinformatics analysis. WC carried out the wet experiments. YL and LZ conducted the manuscript writing and bioinformatics analysis. All authors read and approved the final manuscript.
Funding
This work was supported by the Youth Project of Health Commission of Nantong (WQ2016051); the Youth Project of Health Commission of Nantong (QN2022026).
Conflict of interest
The authors declare that the research was conducted in the absence of any commercial or financial relationships that could be construed as a potential conflict of interest.
Publisher’s note
All claims expressed in this article are solely those of the authors and do not necessarily represent those of their affiliated organizations, or those of the publisher, the editors and the reviewers. Any product that may be evaluated in this article, or claim that may be made by its manufacturer, is not guaranteed or endorsed by the publisher.
Supplementary material
The Supplementary Material for this article can be found online at: https://www.frontiersin.org/articles/10.3389/fphar.2022.1098136/full#supplementary-material
References
Bray, F., Ferlay, J., Soerjomataram, I., Siegel, R. L., Torre, L. A., and Jemal, A. (2018). Global cancer statistics 2018: GLOBOCAN estimates of incidence and mortality worldwide for 36 cancers in 185 countries. CA Cancer J. Clin. 68, 394–424. doi:10.3322/caac.21492
Cao, G., Tan, B., Wei, S., Shen, W., Wang, X., Chu, Y., et al. (2020). Down-regulation of MBNL1-AS1 contributes to tumorigenesis of NSCLC via sponging miR-135a-5p. Biomed. Pharmacother. 125, 109856. doi:10.1016/j.biopha.2020.109856
Carvajal, R. D., Antonescu, C. R., Wolchok, J. D., Chapman, P. B., Roman, R-A., Teitcher, J., et al. (2011). KIT as a therapeutic target in metastatic melanoma. JAMA 305, 2327–2334. doi:10.1001/jama.2011.746
Chen, F., Song, J., Ye, Z., Xu, B., Cheng, H., Zhang, S., et al. (2021). Integrated analysis of cell cycle-related and immunity-related biomarker signatures to improve the prognosis prediction of lung adenocarcinoma. Front. Oncol. 11, 666826. doi:10.3389/fonc.2021.666826
Chen, W-J., Tang, R-X., He, R-Q., Li, D-Y., Liang, L., Zeng, J-H., et al. (2017). Clinical roles of the aberrantly expressed lncRNAs in lung squamous cell carcinoma: A study based on RNA-sequencing and microarray data mining. Oncotarget 8, 61282–61304. doi:10.18632/oncotarget.18058
Chen, Y., Tang, J., Lu, T., and Liu, F. (2020). CAPN1 promotes malignant behavior and erlotinib resistance mediated by phosphorylation of c-Met and PIK3R2 via degrading PTPN1 in lung adenocarcinoma. Thorac. Cancer 11, 1848–1860. doi:10.1111/1759-7714.13465
Chowell, D., Morris, L. G. T., Grigg, C. M., Weber, J. K., Samstein, R. M., Makarov, V., et al. (2018). Patient HLA class I genotype influences cancer response to checkpoint blockade immunotherapy. Science 359, 582–587. doi:10.1126/science.aao4572
Chu, Z-Q., Zhang, K-C., and Chen, L. (2021). Neutrophil extracellular traps in gastrointestinal cancer. World J. Gastroenterol. 27, 5474–5487. doi:10.3748/wjg.v27.i33.5474
Coudray, N., Ocampo, P. S., Sakellaropoulos, T., Narula, N., Snuderl, M., Fenyö, D., et al. (2018). Classification and mutation prediction from non-small cell lung cancer histopathology images using deep learning. Nat. Med. 24, 1559–1567. doi:10.1038/s41591-018-0177-5
Cui, Y., Zhang, C., Ma, S., Li, Z., Wang, W., Li, Y., et al. (2021). RNA m6A demethylase FTO-mediated epigenetic up-regulation of LINC00022 promotes tumorigenesis in esophageal squamous cell carcinoma. J. Exp. Clin. Cancer Res. 40, 294. doi:10.1186/s13046-021-02096-1
Denisenko, T. V., Budkevich, I. N., and Zhivotovsky, B. (2018). Cell death-based treatment of lung adenocarcinoma. Cell Death Dis. 9, 117. doi:10.1038/s41419-017-0063-y
Du, J., Ji, H., Ma, S., Jin, J., Mi, S., Hou, K., et al. (2021). m6A regulator-mediated methylation modification patterns and characteristics of immunity and stemness in low-grade glioma. Brief. Bioinform 22, bbab013. doi:10.1093/bib/bbab013
Erpenbeck, L., and Schön, M. P. (2017). Neutrophil extracellular traps: Protagonists of cancer progression? Oncogene 36, 2483–2490. doi:10.1038/onc.2016.406
Garrido, F. (2019). HLA class-I expression and cancer immunotherapy. Adv. Exp. Med. Biol. 1151, 79–90. doi:10.1007/978-3-030-17864-2_3
Geng, R., Song, J., Zhong, Z., Ni, S., Liu, W., He, Z., et al. (2022). Crosstalk of redox-related subtypes, establishment of a prognostic model and immune responses in endometrial carcinoma. Cancers (Basel) 14, 3383. doi:10.3390/cancers14143383
Gobin, S. J., van Zutphen, M., Woltman, A. M., and van den Elsen, P. J. (1999). Transactivation of classical and nonclassical HLA class I genes through the IFN-stimulated response element. J. Immunol. 163, 1428–1434.
Gu, H., Song, J., Chen, Y., Wang, Y., Tan, X., and Zhao, H. (2022). Inflammation-related LncRNAs signature for prognosis and immune response evaluation in uterine corpus endometrial carcinoma. Front. Oncol. 12, 923641. doi:10.3389/fonc.2022.923641
Gupta, R. A., Shah, N., Wang, K. C., Kim, J., Horlings, H. M., Wong, D. J., et al. (2010). Long non-coding RNA HOTAIR reprograms chromatin state to promote cancer metastasis. Nature 464, 1071–1076. doi:10.1038/nature08975
Han, S., Cao, D., Sha, J., Zhu, X., and Chen, D. (2020). LncRNA ZFPM2-AS1 promotes lung adenocarcinoma progression by interacting with UPF1 to destabilize ZFPM2. Mol. Oncol. 14, 1074–1088. doi:10.1002/1878-0261.12631
He, L., Li, H., Wu, A., Peng, Y., Shu, G., and Yin, G. (2019). Functions of N6-methyladenosine and its role in cancer. Mol. Cancer 18, 176. doi:10.1186/s12943-019-1109-9
Imielinski, M., Berger, A. H., Hammerman, P. S., Hernandez, B., Pugh, T. J., Hodis, E., et al. (2012). Mapping the hallmarks of lung adenocarcinoma with massively parallel sequencing. Cell 150, 1107–1120. doi:10.1016/j.cell.2012.08.029
Li, D-S., Ainiwaer, J-L., Sheyhiding, I., Zhang, Z., and Zhang, L-W. (2016). Identification of key long non-coding RNAs as competing endogenous RNAs for miRNA-mRNA in lung adenocarcinoma. Eur. Rev. Med. Pharmacol. Sci. 20, 2285–2295.
Li, L., Yang, L., Fan, Z., Xue, W., Shen, Z., Yuan, Y., et al. (2020). Hypoxia-induced GBE1 expression promotes tumor progression through metabolic reprogramming in lung adenocarcinoma. Signal Transduct. Target Ther. 5, 54. doi:10.1038/s41392-020-0152-8
Liu, C., Wang, Y., Dao, Y., Wang, S., Hou, F., Yang, Z., et al. (2022). Upregulation of CENPM facilitates lung adenocarcinoma progression via PI3K/AKT/mTOR signaling pathway. Acta Biochim. Biophys. Sin. (Shanghai) 54, 99–112. doi:10.3724/abbs.2021013
Liu, J., Geng, R., Ni, S., Cai, L., Yang, S., Shao, F., et al. (2022). Pyroptosis-related lncRNAs are potential biomarkers for predicting prognoses and immune responses in patients with UCEC. Mol. Ther. Nucleic Acids 27, 1036–1055. doi:10.1016/j.omtn.2022.01.018
Liu, J., Geng, R., Zhong, Z., Zhang, Y., Ni, S., Liu, W., et al. (2022). N1-Methyladenosine-Related lncRNAs are potential biomarkers for predicting prognosis and immune response in uterine corpus endometrial carcinoma. Oxid. Med. Cell Longev. 2022, 2754836. doi:10.1155/2022/2754836
Liu, J., Mei, J., Wang, Y., Chen, X., Pan, J., Tong, L., et al. (2021). Development of a novel immune-related lncRNA signature as a prognostic classifier for endometrial carcinoma. Int. J. Biol. Sci. 17, 448–459. doi:10.7150/ijbs.51207
Liu, Y., Shi, M., He, X., Cao, Y., Liu, P., Li, F., et al. (2022). LncRNA-PACERR induces pro-tumour macrophages via interacting with miR-671-3p and m6A-reader IGF2BP2 in pancreatic ductal adenocarcinoma. J. Hematol. Oncol. 15, 52. doi:10.1186/s13045-022-01272-w
Ma, L., Zhang, X., Yu, K., Xu, X., Chen, T., Shi, Y., et al. (2021). Targeting SLC3A2 subunit of system XC- is essential for m6A reader YTHDC2 to be an endogenous ferroptosis inducer in lung adenocarcinoma. Free Radic. Biol. Med. 168, 25–43. doi:10.1016/j.freeradbiomed.2021.03.023
Ma, S., Chen, C., Ji, X., Liu, J., Zhou, Q., Wang, G., et al. (2019). The interplay between m6A RNA methylation and noncoding RNA in cancer. J. Hematol. Oncol. 12, 121. doi:10.1186/s13045-019-0805-7
Ma, Y., Zhang, J., Wen, L., and Lin, A. (2018). Membrane-lipid associated lncRNA: A new regulator in cancer signaling. Cancer Lett. 419, 27–29. doi:10.1016/j.canlet.2018.01.008
Mangiola, S., Doyle, M. A., and Papenfuss, A. T. (2021). Interfacing Seurat with the R tidy universe. Bioinformatics 37, 4100–4107. doi:10.1093/bioinformatics/btab404
Mhaidly, R., and Mechta-Grigoriou, F. (2021). Role of cancer-associated fibroblast subpopulations in immune infiltration, as a new means of treatment in cancer. Immunol. Rev. 302, 259–272. doi:10.1111/imr.12978
Mou, Y., Wang, J., Wu, J., He, D., Zhang, C., Duan, C., et al. (2019). Ferroptosis, a new form of cell death: Opportunities and challenges in cancer. J. Hematol. Oncol. 12, 34. doi:10.1186/s13045-019-0720-y
Ning, J., Wang, F., Bu, J., Zhu, K., and Liu, W. (2022). Down-regulated m6A reader FTO destabilizes PHF1 that triggers enhanced stemness capacity and tumor progression in lung adenocarcinoma. Cell Death Discov. 8, 354. doi:10.1038/s41420-022-01125-y
Peng, W-X., Koirala, P., and Mo, Y-Y. (2017). LncRNA-mediated regulation of cell signaling in cancer. Oncogene 36, 5661–5667. doi:10.1038/onc.2017.184
Quail, D. F., and Joyce, J. A. (2013). Microenvironmental regulation of tumor progression and metastasis. Nat. Med. 19, 1423–1437. doi:10.1038/nm.3394
Ritchie, M. E., Phipson, B., Wu, D., Hu, Y., Law, C. W., Shi, W., et al. (2015). Limma powers differential expression analyses for RNA-sequencing and microarray studies. Nucleic Acids Res. 43, e47. doi:10.1093/nar/gkv007
Sasa, G. B. K., Xuan, C., Lyu, G., Ding, X., and Meiyu, F. (2022). Long non-coding RNA ZFPM2-AS1: A novel biomarker in the pathogenesis of human cancers. Mol. Biotechnol. 64, 725–742. doi:10.1007/s12033-021-00443-3
Shen, M., Li, Y., Wang, Y., Shao, J., Zhang, F., Yin, G., et al. (2021). N6-methyladenosine modification regulates ferroptosis through autophagy signaling pathway in hepatic stellate cells. Redox Biol. 47, 102151. doi:10.1016/j.redox.2021.102151
Sica, A., Schioppa, T., Mantovani, A., and Allavena, P. (2006). Tumour-associated macrophages are a distinct M2 polarised population promoting tumour progression: Potential targets of anti-cancer therapy. Eur. J. Cancer 42, 717–727. doi:10.1016/j.ejca.2006.01.003
Song, J., Liu, Y., Guan, X., Zhang, X., Yu, W., and Li, Q. (2021). A novel ferroptosis-related biomarker signature to predict overall survival of esophageal squamous cell carcinoma. Front. Mol. Biosci. 8, 675193. doi:10.3389/fmolb.2021.675193
Song, J., Sun, Y., Cao, H., Liu, Z., Xi, L., Dong, C., et al. (2021). A novel pyroptosis-related lncRNA signature for prognostic prediction in patients with lung adenocarcinoma. Bioengineered 12, 5932–5949. doi:10.1080/21655979.2021.1972078
Song, Z., Jia, N., Li, W., and Zhang, X-Y. (2020). LINC01572 regulates cisplatin resistance in gastric cancer cells by mediating miR-497-5p. Onco Targets Ther. 13, 10877–10887. doi:10.2147/OTT.S267915
Starzer, A. M., and Berghoff, A. S. (2020). New emerging targets in cancer immunotherapy: CD27 (TNFRSF7). ESMO Open 4, e000629. doi:10.1136/esmoopen-2019-000629
Sui, J., Li, Y-H., Zhang, Y-Q., Li, C-Y., Shen, X., Yao, W-Z., et al. (2016). Integrated analysis of long non-coding RNA-associated ceRNA network reveals potential lncRNA biomarkers in human lung adenocarcinoma. Int. J. Oncol. 49, 2023–2036. doi:10.3892/ijo.2016.3716
Topalian, S. L., Taube, J. M., Anders, R. A., and Pardoll, D. M. (2016). Mechanism-driven biomarkers to guide immune checkpoint blockade in cancer therapy. Nat. Rev. Cancer 16, 275–287. doi:10.1038/nrc.2016.36
Wang, T., Kong, S., Tao, M., and Ju, S. (2020). The potential role of RNA N6-methyladenosine in Cancer progression. Mol. Cancer 19, 88. doi:10.1186/s12943-020-01204-7
Wang, Y., Fu, J., Wang, Z., Lv, Z., Fan, Z., and Lei, T. (2019). Screening key lncRNAs for human lung adenocarcinoma based on machine learning and weighted gene co-expression network analysis. Cancer Biomark. 25, 313–324. doi:10.3233/CBM-190225
Wang, Z., Wang, X., Zhang, T., Su, L., Liu, B., Zhu, Z., et al. (2021). LncRNA MALAT1 promotes gastric cancer progression via inhibiting autophagic flux and inducing fibroblast activation. Cell Death Dis. 12, 368. doi:10.1038/s41419-021-03645-4
Wilkerson, M. D., and Hayes, D. N. (2010). ConsensusClusterPlus: A class discovery tool with confidence assessments and item tracking. Bioinformatics 26, 1572–1573. doi:10.1093/bioinformatics/btq170
Xu, Y., Lv, D., Yan, C., Su, H., Zhang, X., Shi, Y., et al. (2022). METTL3 promotes lung adenocarcinoma tumor growth and inhibits ferroptosis by stabilizing SLC7A11 m6A modification. Cancer Cell Int. 22, 11. doi:10.1186/s12935-021-02433-6
Yang, H., Hu, Y., Weng, M., Liu, X., Wan, P., Hu, Y., et al. (2022). Hypoxia inducible lncRNA-CBSLR modulates ferroptosis through m6A-YTHDF2-dependent modulation of CBS in gastric cancer. J. Adv. Res. 37, 91–106. doi:10.1016/j.jare.2021.10.001
Zhang, D., Zhang, D., Wang, C., Yang, X., Zhang, R., Li, Q., et al. (2022). Gene and prognostic value of N6-methyladenosine (m6A) modification regulatory factors in lung adenocarcinoma. Eur. J. Cancer Prev. 31, 354–362. doi:10.1097/CEJ.0000000000000717
Zhang, H., Wang, S-Q., Wang, L., Lin, H., Zhu, J-B., Chen, R., et al. (2022). m6A methyltransferase METTL3-induced lncRNA SNHG17 promotes lung adenocarcinoma gefitinib resistance by epigenetically repressing LATS2 expression. Cell Death Dis. 13, 657. doi:10.1038/s41419-022-05050-x
Zhang, Y., Liu, X., Liu, L., Li, J., Hu, Q., and Sun, R. (2020). Expression and prognostic significance of m6A-related genes in lung adenocarcinoma. Med. Sci. Monit. 26, e919644. doi:10.12659/MSM.919644
Keywords: lung adenocarcinoma, long non-coding RNA, ferroptosis, N6-methyladenosine (m6A) methylation, signature, LINC01572
Citation: Hong L, Wang X, Cui W, Wang F, Shi W, Yu S, Luo Y, Zhong L and Zhao X (2023) Construction of a ferroptosis scoring system and identification of LINC01572 as a novel ferroptosis suppressor in lung adenocarcinoma. Front. Pharmacol. 13:1098136. doi: 10.3389/fphar.2022.1098136
Received: 14 November 2022; Accepted: 12 December 2022;
Published: 04 January 2023.
Edited by:
Zhijie Xu, Xiangya Hospital, Central South University, ChinaReviewed by:
Yingkun Xu, Chongqing Medical University, ChinaNi Zeng, Affiliated Hospital of Zunyi Medical University, China
Copyright © 2023 Hong, Wang, Cui, Wang, Shi, Yu, Luo, Zhong and Zhao. This is an open-access article distributed under the terms of the Creative Commons Attribution License (CC BY). The use, distribution or reproduction in other forums is permitted, provided the original author(s) and the copyright owner(s) are credited and that the original publication in this journal is cited, in accordance with accepted academic practice. No use, distribution or reproduction is permitted which does not comply with these terms.
*Correspondence: Yonghua Luo, bnRsdW95b25naHVhQDE2My5jb20=; Lixin Zhong, emx4enkwNjY2QHNpbmEuY29t; Xinyuan Zhao, emhhb3hpbnl1YW5AbnR1LmVkdS5jbg==
†These authors have contributed equally to this work