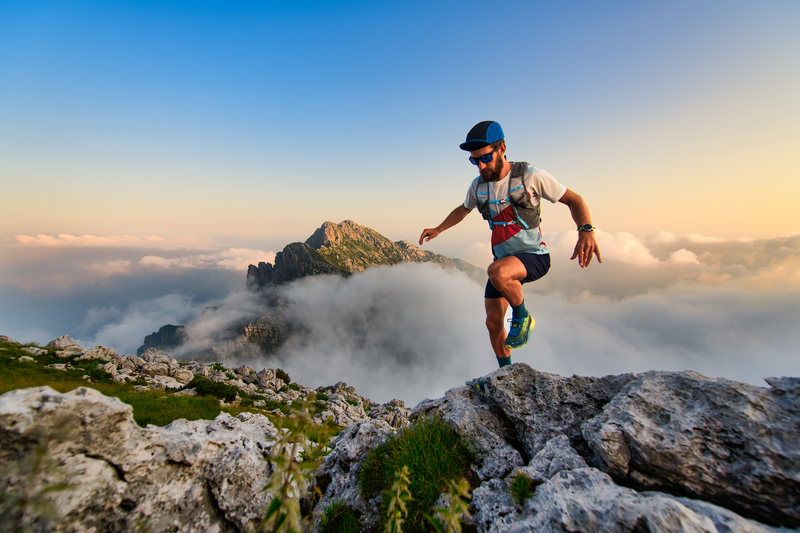
94% of researchers rate our articles as excellent or good
Learn more about the work of our research integrity team to safeguard the quality of each article we publish.
Find out more
ORIGINAL RESEARCH article
Front. Pharmacol. , 22 December 2022
Sec. Pharmacogenetics and Pharmacogenomics
Volume 13 - 2022 | https://doi.org/10.3389/fphar.2022.1078464
Background: Accumulating evidence shows that DNA methylation plays a role in antipsychotic response. However, the mechanisms by which DNA methylation changes are associated with antipsychotic responses remain largely unknown.
Methods: We performed a methylome-wide association study (MWAS) to evaluate the association between DNA methylation and the response to risperidone in schizophrenia. Genomic DNA methylation patterns were assessed using the Agilent Human DNA Methylation Microarray.
Results: We identified numerous differentially methylated positions (DMPs) and regions (DMRs) associated with antipsychotic response. CYP46A1, SPATS2, and ATP6V1E1 had the most significant DMPs, with p values of 2.50 × 10–6, 3.53 × 10–6, and 5.71 × 10–6, respectively. The top-ranked DMR was located on chromosome 7, corresponding to the PTPRN2 gene with a Šidák-corrected p-value of 9.04 × 10–13. Additionally, a significant enrichment of synaptic function and neurotransmitters was found in the differentially methylated genes after gene ontology and pathway analysis.
Conclusion: The identified DMP- and DMR-overlapping genes associated with antipsychotic response are related to synaptic function and neurotransmitters. These findings may improve understanding of the mechanisms underlying antipsychotic response and guide the choice of antipsychotic in schizophrenia.
Schizophrenia (OMIM 181500) is a severe psychiatric disorder defined by the presence of symptoms including hallucinations, delusions, disorganized speech and behavior, diminished emotional expression, social withdrawal and cognitive impairment, which has a lifetime risk of approximately .7% (McGrath et al., 2008) and a prevalence of about 1% (Greenwood et al., 2019). The onset of schizophrenia is referred to as the prodromal phase and comprises a decline in cognitive and social functions, which generally begins in the early adolescent years and precedes the onset of psychotic symptoms by more than ten years (Kahn and Keefe, 2013). Schizophrenia is highly heritable (Cardno and Gottesman, 2000) and many genetic variants involved in the risk architecture of schizophrenia have been identified across diverse populations (Weinberger, 2019). For the last 70 years, antipsychotics have been the only effective treatment for the disorder (Lisoway et al., 2021), however, only 50% of schizophrenia patients show favorable symptom improvement (Lohoff and Ferraro, 2010). Furthermore, existing therapies largely relieve primarily positive symptoms (hallucinations and delusions), and the response to existing antipsychotic medications is highly variable (Lehman et al., 2004). The lack of progress in therapeutic development is a consequence of the limited understanding of the molecular etiology of antipsychotic response (Yu H. et al., 2018).
Due to the possible role of genetic factors in antipsychotic treatment effectiveness, pharmacogenomics has become a significant consideration for improving treatment outcomes in schizophrenia (Soria-Chacartegui et al., 2021). Recently, a genome-wide association study (GWAS) and a whole-exome sequencing (WES) study have revealed a series of genetic variants associated with antipsychotic response (Wang et al., 2018; Yu H. et al., 2018). Despite advances in the pharmacogenomics of schizophrenia, the biological functions of the majority of identified variants are unclear. Moreover, none of the variants identified thus far are sufficiently informative to predict antipsychotic response (Lisoway et al., 2021). In light of this, pharmacoepigenomics is emerging as an important player in the search for the biological architecture of the response to antipsychotic treatment (Lisoway et al., 2021).
The investigation of epigenetic alterations and their relationship to antipsychotic response may be useful in the discovery of pertinent, non-invasive biomarkers to advance personalized psychiatric medicine. In humans, as one of the major types of epigenetic modifications, and perhaps the most well-characterized, DNA methylation refers primarily to a biological process that adds a methyl group to the cytosines of CpG dinucleotides (Greenberg and Bourc’his, 2019). Studies in antipsychotic treatment have focused on investigating the associations between antipsychotic response and DNA methylation of the promoter regions of candidate genes, such as SLC6A4 (Abdolmaleky et al., 2014), HTR1A (Tang et al., 2014), and BDNF (Dong et al., 2016). However, this strategy cannot provide a comprehensive landscape of how epigenetics affects the antipsychotic response. As genome-wide methylation scanning technology evolves, methylome-wide association studies (MWASs) have been adopted across different fields, and has successfully identified promising methylation biomarkers associated with drugs. For example, Murata.et al. used the HumanMethylation450 BeadChip (Illumina) to conduct a genome-wide assessment of human neuroblastoma cells that had received blonanserin at two different dosages (Murata et al., 2014). The authors identified 1657 hyper- and 1104 hypomethylated CpG sites in both dose groups compared to controls. Furthermore, these CpG sites were enriched outside of gene promoters, 3′-, and intergenic regions. However, there have been few studies on the association between DNA methylation and antipsychotic response at the genome-wide level. MWAS may provide additional insightful information for the traditional pharmacogenomic research.
The objective of the current study was to scan genome-wide DNA methylation data to identify differentially methylation biomarkers of antipsychotic response in blood. To analyze the association between DNA methylation and antipsychotic response, we applied both genome-wide position-based and region-based approaches (Li et al., 2021). Gene set analysis was carried out to shed light on coherent biological pathways and processes.
In total, 86 schizophrenic patients were recruited from our clinical networks in Shanghai. Diagnosis and blood sample collection were performed by skilled psychiatrists. Patients with schizophrenia were enrolled in the study if they satisfied the following requirements: 1) they satisfied the criteria for schizophrenia in the Structured Clinical Interview of the Diagnosis and Statistical Manual of Mental Disorder Fourth Edition (DSM-IV); 2) they ranged in age from 18 to 50; 3) physically fit with all laboratory results falling within acceptable ranges; and 4) took pills orally. Before participating in this study, all patients were required to designate a legal guardian to give written informed permission and assist patients in making decisions. The Bio-X Institute’s Ethical Committee at Shanghai Jiao Tong University gave its approval for this project. All of the participants were Han Chinese.
The Positive and Negative Syndrome Scale (PANSS) was used to evaluate clinical symptoms. The percentage change in PANSS to antipsychotic medication was used to assess treatment response. During an 8-week risperidone treatment, medication efficacy was assessed four times (baseline, week two, week four and week eight) using the difference in PANSS scores from baseline. The following equation was used to determine each participant’s PANSS reduction rate at week eight (Obermeier et al., 2010; Yu H. et al., 2018).
Following the instructions on the QIAamp DNA Blood Kits (Qiagen, United States of America), genomic DNA was obtained from whole blood samples. The methylation status was evaluated using the Agilent Human DNA Methylation Microarray (1 × 244 k; Agilent Technologies, Santa Clara, CA) according to the manufacturer’s recommendations. Briefly, 4 μg of DNA was broken up into 200–1000 bps. Eighty percent of the shared DNA was then immunoprecipitated using anti-5-methylcytidine monoclonal antibody, with the remaining 20% serving as a control. The DNA fragments with two sections were purified, tagged, and then hybridized onto microarray slides. An Agilent SureScan Microarray Scanner G2505C was used to create images of slides, which were then converted to digital features using Agilent Feature Extraction program (version 10.7.1.1). The Agilent Genomic Workbench CH3 application (version 7.0) was used to examine the feature data for quality control. The methylation status of all probes was determined by the Bayesian tool for methylation analysis (BATMAN) (Down et al., 2008). In the BATMAN analysis, the methylation status was categorized into three levels: “1” (high, DNA methylation >60%), “0” (moderate, DNA methylation 40–60%) and “-1” (low, DNA methylation <40%) (Down et al., 2008). Probes with poor quality (outlier flag = 1) in >10% of the subjects, associated with cellular specificity (Jaffe and Irizarry, 2014), located on sex chromosomes and BATMAN values for probes that concentrated to one level in >95% of the subjects were excluded from the remaining analyses (Huai et al., 2019; Shen et al., 2021).
To identify the association between differentially methylated positions (DMPs) and PANSS percentage change values, we used linear regression adjusting for age (Yu H. et al., 2018). The identified DMPs were annotated by Table Browser implemented in the UCSC genome browser (http://genome.ucsc.edu/cgi-bin/hgTables) (Shen et al., 2021). Differentially methylated regions (DMRs) were determined using comb-p (Pedersen et al., 2012) with a distance of 500 bp and a seeded p-value of .01. The DMR analysis was carried out for all probes, and DMRs were considered significant and reported if they were three or more and with Šidák-corrected p less than .05. Then we used Bedtools to intersect the GTF files and determine the genomic annotation of the identified DMRs. GTF files were downloaded from GENCODE (https://www.gencodegenes.org/human/release_40lift37.html). The Human Protein Atlas database (https://www.proteinatlas.org) was used to analyze the expression patterns of DMP- and DMR-overlapping genes in the human brain (Yu et al., 2015).
To further understand the function of the overlapping genes mentioned above, the STRING v11.5 database was used to perform gene ontology (GO) analysis, pathway analysis and protein‒protein interaction (PPI) network analysis (Szklarczyk et al., 2019). Go and pathway terms were restricted to those that satisfied an adjusted p-value <.05 (false discovery rate, FDR) threshold. The detailed protein‒protein interaction network graphs were drawn using Cytoscape 3.9.1 (Shannon et al., 2003).
GeneAnalytics (https://geneanalytics.genecards.org) was used to perform gene set analysis on a combined dataset of DMPs and DMRs (Ben-Ari Fuchs et al., 2016). The rationale behind combining DMPs and DMRs into a single group for gene set analysis was to address the problem that not all probes may create DMRs. DMPs and DMRs were investigated jointly to give those regions of the genome a chance to contribute to gene set analysis.
A total of 83 schizophrenic patients (37.7 ± 11.2 years; 45 males and 38 females) met the inclusion criteria (Figure 1). The DNA methylation status of the peripheral blood cells was detected using the Agilent Human 1 × 244 k DNA Methylation Microarray. After quality control, a total of 229,786 methylation probes were retained to perform whole-genome methylation analysis.
The results of linear regression analysis demonstrated that 17 DMPs exhibited strong association with PANSS percentage change values (p < 5 × 10–5; Figure 2 and Table 1). These DMPs were mapped to 11 unique genes. The most significant DMPs were annotated to genes CYP46A1, SPATS2, and ATP6V1E1 with p values of 2.50 × 10–6, 3.53 × 10–6, and 5.71 × 10–6, respectively. There were two other DMPs (probe 176,224: Chr16:32096066–32096110 and probe 176,445: Chr16:33039318–33039362) located in the chromosome 16p11.2 region which has been consistently highlighted in genetic studies of schizophrenia and antipsychotic response (McCarthy et al., 2009; Lit et al., 2012; Chang et al., 2017; Marshall et al., 2017) (Table 1). More importantly, five genes (NME9, CPLX1, CYP46A1, PTPRN2, and S1PR5) were highly expressed in brain tissue (Supplementary Figure S1).
FIGURE 2. Manhattan plots of p values against their respective chromosomal positions for methylome-wide association study. The red line represents the genome-wide significance level (p < 5 × 10–5).
The DMR analysis, which enabled us to identify regions of the genome with ≥3 probes, revealed a total of 21 DMRs significantly associated with antipsychotic response (Šidák-corrected p-value <.05; Table 2), overlapping with 21 unique genes. The two most significant DMRs were located on Chr7:157524168–157526728 (p Šidák = 9.04 × 10–13) and Chr 9: 140735579–140737213 (p Šidák = 2.69 × 10–11), corresponding to the PTPRN2 gene and EHMT1gene, respectively. Of the 21 genes, six genes (ATP9B, CFAP46, GRIN1, PLEKHG4B, PTPRN2, and TUBB3) were highly expressed in brain tissue (Supplementary Figure S2).
To describe common characteristics of the DMPs, we performed GO and pathway enrichment analyses. The top 20 GO terms were listed in the categories of biological processes, cellular components and molecular functions according to the FDR values (Figure 3A). The functions of the differentially methylated genes were mainly involved in synaptic functions and neurotransmitter processes, such as synaptic vesicle exocytosis, presynapse, syntaxin binding, neurotransmitter secretion, and neurotransmitter transmembrane transporter activity. Pathway analysis was performed utilizing the Kyoto Encyclopedia of Genes and Genomes (KEGG) database, Reactome Pathway database, and WikiPathways database. The top-ranked enriched terms were involved in synapse- and neurotransmitters-related pathways, such as the synaptic vesicle pathway, dopamine neurotransmitter release cycle, and serotonin neurotransmitter release cycle (Figure 3B). Additionally, the Wnt signaling pathway, known to be crucial for neurodevelopment and nervous system regulation (Meffre et al., 2014; Yu Z. et al., 2018), was also significantly enriched (Figure 3B).
FIGURE 3. Significantly enriched GO and pathway terms of DMP-related genes. (A) The Y-axis represents the GO terms and the X-axis represents the p values and gene count of enrichment; (B) The Y-axis represents the pathway terms and the X-axis represents the p values and gene count of enrichment. Synaptic function and neurotransmitters-related terms are labeled with grey boxes.
Consistent with the DMP results, DMR-related genes were also linked to synaptic functions (e.g., excitatory postsynaptic potential, synaptic transmission, postsynaptic density, and postsynaptic density membrane) (Figure 4A). In addition, we identified several GO terms related to glutamate functions, such as ionotropic glutamate receptor activity, NMDA glutamate receptor activity, glutamate-gated calcium ion channel activity, and NMDA selective glutamate receptor complex (Figure 4A). Consistent with the GO results, the top-ranked enriched terms from the three pathway databases were centered on glutamate related pathways, especially NMDA related pathways, such as activation of AMPK downstream of NMDARs, unblocking of NMDA receptors, glutamate binding and activation, assembly and cell surface presentation of NMDA receptors (Figure 4B).
FIGURE 4. Significantly enriched GO and pathway terms of DMR-related genes. (A) The Y-axis represents the GO terms and the X-axis represents the p values and gene count of enrichment; (B) The Y-axis represents the pathway terms and the X-axis represents the p values and gene count of enrichment. Synaptic and glutamate function-related terms are labeled with grey boxes.
Two PPI networks were obtained by investigating PPI via the STRING database using DMP- and DMR-overlapping genes and previously reported candidate genes that showed a positive association with antipsychotic response (Supplementary Figure S3) (Yu H. et al., 2018). As shown in Supplementary Figure S3a, all DMP-overlapping genes can construct a PPI network with half of the DMR-overlapping genes. Furthermore, we found a highly interconnected PPI network constructed by the DMP-overlapping genes, DMR-overlapping genes, and candidate genes. (Supplementary Figure S3b).
Detailed outcomes for combined gene set enrichment of DMPs and DMRs can be found in Table 3. In short, the top-two enriched tissues were brain and neurons; the top-two enriched systems were nervous system and sensory organs; the top-two enriched diseases were schizophrenia and autosomal dominant non-syndromic intellectual disability; and the top-two enriched human phenotype ontologies (HPO) were abnormal hippocampal morphology and abnormal morphology of the limbic system. For the GO and pathway enrichment analyses, the top-ranked enriched terms were also concentrated on synaptic functions.
We conducted a genome-wide methylation study in peripheral blood cells to investigate the association between DNA methylation and antipsychotic response. This study identified 17 DMPs and 21 DMRs, despite the use of peripheral blood cells, most of which were linked to genes involved in synaptic function and neurotransmitters. The combination strategy of position- and region-based analysis yielded notable findings that overlap a gene set enriched for biological processes highly relevant to antipsychotic response etiology.
Our results support the hypothesis that the mechanism of action of antipsychotic drugs might be mediated mainly by the neurotransmitter systems (Brandl et al., 2014). Additionally, the majority of the genes discovered were related to synaptic function. One of our top DMP findings was in the CYP46A1 gene (Table 1), which encodes a member of the cytochrome P450 superfamily of enzymes. CYP46A1 may promote steady-state cholesterol in neurons, activate the mevalonate pathway, and coordinate the synthesis of new cholesterol and non-sterol involved in synaptic activity and learning (Mast et al., 2003; Mast et al., 2017). In addition, studies have shown that CYP46A1 may be controlled epigenetically (Shafaati et al., 2009; Milagre et al., 2010; Nunes et al., 2010) and by specific transcription factors at the basal expression level (Milagre et al., 2008; Milagre et al., 2012). Taken together, our findings indicated that aberrant DNA methylation of CYP46A1 may have an impact on its expression, thereby contributing to antipsychotic response etiology. We also identified STAG1 as a gene potentially involved in antipsychotic response etiology. Singh et al. (Singh et al., 2022) demonstrated that several common and rare variants showed a convergence in STAG1that was significantly associated with schizophrenia. In addition to DMP-overlapping genes, we also found that two DMPs were located at the chromosome 16p11.2 region. Accumulating evidence suggests that the chromosome 16p11.2 region is implicated in schizophrenia (McCarthy et al., 2009; Chang et al., 2017; Marshall et al., 2017). Importantly, several studies reported regulatory effects of 16p11.2 copy number variations on dendritic spine development and synaptic function (Escamilla et al., 2017; Yadav et al., 2017; Richter et al., 2019). In addition, RNF40 located at the 16p11.2 region was associated with the risperidone response in children with autism spectrum disorders (Lit et al., 2012).
Comparing these results within the DMP-antipsychotic response literature is difficult because a majority of the studies used candidate gene approaches. The only genome-wide study used the Agilent Human DNA Methylation Microarray platform to compare the methylation profiles of peripheral blood in the same subjects before and after antipsychotic treatment to controls (Rukova et al., 2014). No methylation alterations were significantly linked with treatment response, likely due to a small sample size (n = 20 blood samples) (Rukova et al., 2014). Small sample sizes are common in pharmacoepigenetic studies because of difficulties of sample recruitment and technology costs, but DMR analysis can mitigate power issues caused by small sample sizes by lessening the burden of multiple tests. We discovered that regional DNA methylation changes are more likely to contribute to the antipsychotic response in the DMR analysis. Furthermore, some DMR-overlapping genes identified were related to synaptic function. The most significant DMR corresponded to the PTPRN2, which is required for normal accumulation of the neurotransmitters norepinephrine, dopamine and serotonin in the brain (Rukova et al., 2014). EHMT1 is a histone methyltransferase that can mark the genomic region packaged with methylated histones (such as histone H3) for transcriptional repression. Defects in EHMT1 are a cause of Kleefstra Syndrome, a genetic disorder linked with schizophrenia (Akbarian and Huang, 2009). In addition, Drosophila EHMT1 mutants exhibited decreased dendrite branching of sensory neurons and impaired short and long-term memory that were reversed by restoring EHMT expression (Kramer et al., 2011). We also identified another target gene, GRIN1, which encodes a member of the glutamate receptor channel superfamily. GRIN1 is involved in synaptic plasticity, which is thought to underpin memory and learning. The glutamate receptor subunit GRIN2A was recently found to be associated with schizophrenia in the largest GWAS of schizophrenia so far (Trubetskoy et al., 2022). DLGAP2 is a membrane-associated protein that may play a role in synapse organization and signaling in neuronal cells. Li et al. found that DNA methylation of DLGAP2 was associated with tardive dyskinesia caused by antipsychotic medications (Li et al., 2018). The results of enrichment analysis of annotated genes within DMRs were consistent with that of the DMP-related gene analysis, indicating that a network of neuronal-functioning genes contributes to the antipsychotic response.
Our gene set analysis revealed multiple lines of evidence linking differentially methylated genes to the antipsychotic response. Additionally, various biological processes, such as MTHFR deficiency, were also enriched for genes with aberrant methylation, warranting further investigation. The thoroughly characterized databases have provided vital data for constructing highly interconnected PPI networks that could reveal the underlying biological processes. Therefore, we investigated the interaction between differentially methylated genes and various known antipsychotic response risk genes. We found that the top susceptibility genes of antipsychotic response identified by GWAS (Yu H. et al., 2018) encoded a densely interconnected PPI network. Intriguingly, the DMP- and DMR-overlapping genes were also involved in this network, indicating their potential involvement in the common molecular network modulating the mechanism of antipsychotic response.
A crucial consideration for interpreting DNA methylation results is the tissue source (Michels et al., 2013; Lappalainen and Greally, 2017). It is suggested that CpGs from specific genomic regions had a statistically significant correlation between brain and peripheral blood samples (Walton et al., 2016). Notably, we found significantly enriched GO terms and pathways for the DMP- and DMR-overlapping genes, suggesting that the development of certain brain regions may be reflected in the DNA methylome of blood samples. According to the findings of gene set analysis, certain epigenetic markers in brain tissue can be mirrored by the corresponding sites in peripheral blood samples.
Validation analysis is critical for DNA methylation association studies to confirm preliminary findings. While no mass spectrometry-based bisulfite sequencing was completed, the literature was searched extensively for associations between antipsychotic response and biological signatures. As previously mentioned, a DMP-overlapping gene (STAG1) identified in this study was also found in the largest exome sequencing study of schizophrenia thus far (Rukova et al., 2014); a DMR-overlapping gene (GRIN1) in this study and GRIN2A identified in the largest GWAS of schizophrenia thus far (Trubetskoy et al., 2022) all belong to the glutamate receptor subunit. The findings from a previous pharmacogenomic study (Yu H. et al., 2018) and MWAS (Li et al., 2021) in schizophrenia implicated biological processes associated with synaptic functions, neurotransmitter receptors, and schizophrenia susceptibility. Similarly, this study identified DMP- and DMR-overlapping genes integral to synaptic vesicle exocytosis, presynapse, excitatory postsynaptic potential, regulation of neurotransmitter secretion, neurotransmitter transmembrane transporter activity (e.g., GRIN1, TUBB3, CPLX1, CYP46A1) and risk for schizophrenia (e.g., DLGAP2, EHMT1, CPLX1, GRIN1). While these similarities do not provide direct replication, they do lend credence to the DNA methylation findings.
While this study identified interesting genes and pathways, there are limitations that are worth noting. First, the sample size was modest, which may limit the statistical power to conduct robust single-site analysis. However, several pharmacoepigenomic studies have identified a series of reliable findings with small sample sizes (Maltby et al., 2015; Maltby et al., 2018; Henderson-Smith et al., 2019). Second, some environmental factors known to influence epigenetic modifications (such as smoking, stress, and circadian rhythm) were not controlled in the analysis. Third, because not all probes have the potential to form regions, regional analysis is inherently limited (Ong and Holbrook, 2014). Finally, this study was limited in its evaluation of functional importance.
In summary, we discovered a set of DMPs and DMRs that were linked to antipsychotic response. To our knowledge, the present study is the first MWAS of the risperidone response in patients with schizophrenia using a technology that provides good coverage of methylation sites across the genome. Our findings show how DNA methylation research can offer fresh perspectives to increase our understanding of antipsychotic response and produce biomarkers with the potential to enhance clinical efficacy.
The original contributions presented in the study are included in the article/Supplementary Materials, further inquiries can be directed to the corresponding authors.
The studies involving human participants were reviewed and approved by the Ethical Committee of Bio-X institute of Shanghai Jiao Tong University. The patients/participants provided their written informed consent to participate in this study.
MZ, XW, and SQ designed this study. HD and JM wrote the manuscript. WZ, ML, LS, and CH analyzed data. HW, XZ, and NZ performed experiments. SG and QW assisted with the analysis and creation of the tables and figures. LH supervised the overall project and revised the manuscript.
This work was supported by grants from the National Nature Science Foundation of China (82274025, 81773818, 81273596, 81503051), Shanghai science and Technology Innovation Fund (21002411100, 20DZ2202000), Major projects of scientific and technological innovation 2030 (2021ZD0200801, 2021ZD0200800), Shanghai Municipal Science and Technology Major Project (2017SHZDZX01), National key research and development program (2016YFC0905000, 2016YFC1200200), Shanghai Key Laboratory of Psychotic Disorders (13dz2260500).
We are greatly indebted to all the patients who took part in this research, as well as the clinical staff who facilitated their involvement.
The authors declare that the research was conducted in the absence of any commercial or financial relationships that could be construed as a potential conflict of interest.
All claims expressed in this article are solely those of the authors and do not necessarily represent those of their affiliated organizations, or those of the publisher, the editors and the reviewers. Any product that may be evaluated in this article, or claim that may be made by its manufacturer, is not guaranteed or endorsed by the publisher.
The Supplementary Material for this article can be found online at: https://www.frontiersin.org/articles/10.3389/fphar.2022.1078464/full#supplementary-material
Abdolmaleky, H. M., Nohesara, S., Ghadirivasfi, M., Lambert, A. W., Ahmadkhaniha, H., Ozturk, S., et al. (2014). DNA hypermethylation of serotonin transporter gene promoter in drug naïve patients with schizophrenia. Schizophr. Res. 152 (2-3), 373–380. doi:10.1016/j.schres.2013.12.007
Akbarian, S., and Huang, H. S. (2009). Epigenetic regulation in human brain-focus on histone lysine methylation. Biol. Psychiatry 65 (3), 198–203. doi:10.1016/j.biopsych.2008.08.015
Ben-Ari Fuchs, S., Lieder, I., Stelzer, G., Mazor, Y., Buzhor, E., Kaplan, S., et al. (2016). GeneAnalytics: An integrative gene set analysis tool for next generation sequencing, RNAseq and microarray data. Omics 20 (3), 139–151. doi:10.1089/omi.2015.0168
Brandl, E. J., Kennedy, J. L., and Müller, D. J. (2014). Pharmacogenetics of antipsychotics. Can. J. Psychiatry 59 (2), 76–88. doi:10.1177/070674371405900203
Cardno, A. G., and Gottesman, (2000). Twin studies of schizophrenia: From bow-and-arrow concordances to star wars mx and functional genomics. Am. J. Med. Genet. 97 (1), 12–17. doi:10.1002/(sici)1096-8628(200021)97:1<12::aid-ajmg3>3.0.co;2-u
Chang, H., Li, L., Li, M., and Xiao, X. (2017). Rare and common variants at 16p11.2 are associated with schizophrenia. Schizophr. Res. 184, 105–108. doi:10.1016/j.schres.2016.11.031
Dong, E., Tueting, P., Matrisciano, F., Grayson, D. R., and Guidotti, A. (2016). Behavioral and molecular neuroepigenetic alterations in prenatally stressed mice: Relevance for the study of chromatin remodeling properties of antipsychotic drugs. Transl. Psychiatry 6 (1), e711. doi:10.1038/tp.2015.191
Down, T. A., Rakyan, V. K., Turner, D. J., Flicek, P., Li, H., Kulesha, E., et al. (2008). A Bayesian deconvolution strategy for immunoprecipitation-based DNA methylome analysis. Nat. Biotechnol. 26 (7), 779–785. doi:10.1038/nbt1414
Escamilla, C. O., Filonova, I., Walker, A. K., Xuan, Z. X., Holehonnur, R., Espinosa, F., et al. (2017). Kctd13 deletion reduces synaptic transmission via increased RhoA. Nature 551 (7679), 227–231. doi:10.1038/nature24470
Greenberg, M. V. C., and Bourc'his, D. (2019). The diverse roles of DNA methylation in mammalian development and disease. Nat. Rev. Mol. Cell Biol. 20 (10), 590–607. doi:10.1038/s41580-019-0159-6
Greenwood, T. A., Lazzeroni, L. C., Maihofer, A. X., Swerdlow, N. R., Calkins, M. E., Freedman, R., et al. (2019). Genome-wide association of endophenotypes for schizophrenia from the consortium on the genetics of schizophrenia (COGS) study. JAMA Psychiatry 76 (12), 1274–1284. doi:10.1001/jamapsychiatry.2019.2850
Henderson-Smith, A., Fisch, K. M., Hua, J., Liu, G., Ricciardelli, E., Jepsen, K., et al. (2019). DNA methylation changes associated with Parkinson's disease progression: Outcomes from the first longitudinal genome-wide methylation analysis in blood. Epigenetics 14 (4), 365–382. doi:10.1080/15592294.2019.1588682
Huai, C., Wei, Y., Li, M., Zhang, X., Wu, H., Qiu, X., et al. (2019). Genome-wide analysis of DNA methylation and antituberculosis drug-induced liver injury in the han Chinese population. Clin. Pharmacol. Ther. 106 (6), 1389–1397. doi:10.1002/cpt.1563
Jaffe, A. E., and Irizarry, R. A. (2014). Accounting for cellular heterogeneity is critical in epigenome-wide association studies. Genome Biol. 15 (2), R31. doi:10.1186/gb-2014-15-2-r31
Kahn, R. S., and Keefe, R. S. (2013). Schizophrenia is a cognitive illness: Time for a change in focus. JAMA Psychiatry 70 (10), 1107–1112. doi:10.1001/jamapsychiatry.2013.155
Kramer, J. M., Kochinke, K., Oortveld, M. A., Marks, H., Kramer, D., de Jong, E. K., et al. (2011). Epigenetic regulation of learning and memory by Drosophila EHMT/G9a. PLoS Biol. 9 (1), e1000569. doi:10.1371/journal.pbio.1000569
Lappalainen, T., and Greally, J. M. (2017). Associating cellular epigenetic models with human phenotypes. Nat. Rev. Genet. 18 (7), 441–451. doi:10.1038/nrg.2017.32
Lehman, A. F., Lieberman, J. A., Dixon, L. B., McGlashan, T. H., Miller, A. L., Perkins, D. O., et al. (2004). Practice guideline for the treatment of patients with schizophrenia, second edition. Am. J. Psychiatry 161 (2), 1–56.
Li, M., Li, Y., Qin, H., Tubbs, J. D., Li, M., Qiao, C., et al. (2021). Genome-wide DNA methylation analysis of peripheral blood cells derived from patients with first-episode schizophrenia in the Chinese Han population. Mol. Psychiatry 26 (8), 4475–4485. doi:10.1038/s41380-020-00968-0
Li, Y., Wang, K., Zhang, P., Huang, J., An, H., Wang, N., et al. (2018). Quantitative DNA methylation analysis of DLGAP2 gene using pyrosequencing in schizophrenia with tardive dyskinesia: A linear mixed model approach. Sci. Rep. 8 (1), 17466. doi:10.1038/s41598-018-35718-4
Lisoway, A. J., Chen, C. C., Zai, C. C., Tiwari, A. K., and Kennedy, J. L. (2021). Toward personalized medicine in schizophrenia: Genetics and epigenetics of antipsychotic treatment. Schizophr. Res. 232, 112–124. doi:10.1016/j.schres.2021.05.010
Lit, L., Sharp, F. R., Bertoglio, K., Stamova, B., Ander, B. P., Sossong, A. D., et al. (2012). Gene expression in blood is associated with risperidone response in children with autism spectrum disorders. Pharmacogenomics J. 12 (5), 368–371. doi:10.1038/tpj.2011.23
Lohoff, F. W., and Ferraro, T. N. (2010). Pharmacogenetic considerations in the treatment of psychiatric disorders. Expert Opin. Pharmacother. 11 (3), 423–439. doi:10.1517/14656560903508762
Maltby, V. E., Graves, M. C., Lea, R. A., Benton, M. C., Sanders, K. A., Tajouri, L., et al. (2015). Genome-wide DNA methylation profiling of CD8+ T cells shows a distinct epigenetic signature to CD4+ T cells in multiple sclerosis patients. Clin. Epigenetics 7, 118. doi:10.1186/s13148-015-0152-7
Maltby, V. E., Lea, R. A., Graves, M. C., Sanders, K. A., Benton, M. C., Tajouri, L., et al. (2018). Genome-wide DNA methylation changes in CD19(+) B cells from relapsing-remitting multiple sclerosis patients. Sci. Rep. 8 (1), 17418. doi:10.1038/s41598-018-35603-0
Marshall, C. R., Howrigan, D. P., Merico, D., Thiruvahindrapuram, B., Wu, W., Greer, D. S., et al. (2017). Contribution of copy number variants to schizophrenia from a genome-wide study of 41, 321 subjects. Nat. Genet. 49 (1), 27–35. doi:10.1038/ng.3725
Mast, N., Anderson, K. W., Lin, J. B., Li, Y., Turko, I. V., Tatsuoka, C., et al. (2017). Cytochrome P450 27A1 deficiency and regional differences in brain sterol metabolism cause preferential cholestanol accumulation in the cerebellum. J. Biol. Chem. 292 (12), 4913–4924. doi:10.1074/jbc.M116.774760
Mast, N., Norcross, R., Andersson, U., Shou, M., Nakayama, K., Bjorkhem, I., et al. (2003). Broad substrate specificity of human cytochrome P450 46A1 which initiates cholesterol degradation in the brain. Biochemistry 42 (48), 14284–14292. doi:10.1021/bi035512f
McCarthy, S. E., Makarov, V., Kirov, G., Addington, A. M., McClellan, J., Yoon, S., et al. (2009). Microduplications of 16p11.2 are associated with schizophrenia. Nat. Genet. 41 (11), 1223–1227. doi:10.1038/ng.474
McGrath, J., Saha, S., Chant, D., and Welham, J. (2008). Schizophrenia: A concise overview of incidence, prevalence, and mortality. Epidemiol. Rev. 30, 67–76. doi:10.1093/epirev/mxn001
Meffre, D., Grenier, J., Bernard, S., Courtin, F., Dudev, T., Shackleford, G., et al. (2014). Wnt and lithium: A common destiny in the therapy of nervous system pathologies? Cell Mol. Life Sci. 71 (7), 1123–1148. doi:10.1007/s00018-013-1378-1
Michels, K. B., Binder, A. M., Dedeurwaerder, S., Epstein, C. B., Greally, J. M., Gut, I., et al. (2013). Recommendations for the design and analysis of epigenome-wide association studies. Nat. Methods 10 (10), 949–955. doi:10.1038/nmeth.2632
Milagre, I., Nunes, M. J., Castro-Caldas, M., Moutinho, M., Gama, M. J., and Rodrigues, E. (2012). Neuronal differentiation alters the ratio of Sp transcription factors recruited to the CYP46A1 promoter. J. Neurochem. 120 (2), 220–229. doi:10.1111/j.1471-4159.2011.07577.x
Milagre, I., Nunes, M. J., Gama, M. J., Silva, R. F., Pascussi, J. M., Lechner, M. C., et al. (2008). Transcriptional regulation of the human CYP46A1 brain-specific expression by Sp transcription factors. J. Neurochem. 106 (2), 835–849. doi:10.1111/j.1471-4159.2008.05442.x
Milagre, I., Nunes, M. J., Moutinho, M., Rivera, I., Fuso, A., Scarpa, S., et al. (2010). Chromatin-modifying agents increase transcription of CYP46A1, a key player in brain cholesterol elimination. J. Alzheimers Dis. 22 (4), 1209–1221. doi:10.3233/jad-2010-100651
Murata, Y., Nishioka, M., Bundo, M., Sunaga, F., Kasai, K., and Iwamoto, K. (2014). Comprehensive DNA methylation analysis of human neuroblastoma cells treated with blonanserin. Neurosci. Lett. 563, 123–128. doi:10.1016/j.neulet.2014.01.038
Nunes, M. J., Milagre, I., Schnekenburger, M., Gama, M. J., Diederich, M., and Rodrigues, E. (2010). Sp proteins play a critical role in histone deacetylase inhibitor-mediated derepression of CYP46A1 gene transcription. J. Neurochem. 113 (2), 418–431. doi:10.1111/j.1471-4159.2010.06612.x
Obermeier, M., Mayr, A., Schennach-Wolff, R., Seemüller, F., Möller, H. J., and Riedel, M. (2010). Should the PANSS be rescaled? Schizophr. Bull. 36 (3), 455–460. doi:10.1093/schbul/sbp124
Ong, M. L., and Holbrook, J. D. (2014). Novel region discovery method for Infinium 450K DNA methylation data reveals changes associated with aging in muscle and neuronal pathways. Aging Cell 13 (1), 142–155. doi:10.1111/acel.12159
Pedersen, B. S., Schwartz, D. A., Yang, I. V., and Kechris, K. J. (2012). Comb-p: Software for combining, analyzing, grouping and correcting spatially correlated P-values. Bioinformatics 28 (22), 2986–2988. doi:10.1093/bioinformatics/bts545
Richter, M., Murtaza, N., Scharrenberg, R., White, S. H., Johanns, O., Walker, S., et al. (2019). Altered TAOK2 activity causes autism-related neurodevelopmental and cognitive abnormalities through RhoA signaling. Mol. Psychiatry 24 (9), 1329–1350. doi:10.1038/s41380-018-0025-5
Rukova, B., Staneva, R., Hadjidekova, S., Stamenov, G., Milanova, V., and Toncheva, D. (2014). Whole genome methylation analyses of schizophrenia patients before and after treatment. Biotechnol. Biotechnol. Equip. 28 (3), 518–524. doi:10.1080/13102818.2014.933501
Shafaati, M., O'Driscoll, R., Björkhem, I., and Meaney, S. (2009). Transcriptional regulation of cholesterol 24-hydroxylase by histone deacetylase inhibitors. Biochem. Biophys. Res. Commun. 378 (4), 689–694. doi:10.1016/j.bbrc.2008.11.103
Shannon, P., Markiel, A., Ozier, O., Baliga, N. S., Wang, J. T., Ramage, D., et al. (2003). Cytoscape: A software environment for integrated models of biomolecular interaction networks. Genome Res. 13 (11), 2498–2504. doi:10.1101/gr.1239303
Shen, L., Lv, X., Huang, H., Li, M., Huai, C., Wu, X., et al. (2021). Genome-wide analysis of DNA methylation in 106 schizophrenia family trios in Han Chinese. EBioMedicine 72, 103609. doi:10.1016/j.ebiom.2021.103609
Singh, T., Poterba, T., Curtis, D., Akil, H., Al Eissa, M., Barchas, J. D., et al. (2022). Rare coding variants in ten genes confer substantial risk for schizophrenia. Nature 604 (7906), 509–516. doi:10.1038/s41586-022-04556-w
Soria-Chacartegui, P., Villapalos-García, G., Zubiaur, P., Abad-Santos, F., and Koller, D. (2021). Genetic polymorphisms associated with the pharmacokinetics, pharmacodynamics and adverse effects of olanzapine, aripiprazole and risperidone. Front. Pharmacol. 12, 711940. doi:10.3389/fphar.2021.711940
Szklarczyk, D., Gable, A. L., Lyon, D., Junge, A., Wyder, S., Huerta-Cepas, J., et al. (2019). STRING v11: Protein-protein association networks with increased coverage, supporting functional discovery in genome-wide experimental datasets. Nucleic Acids Res. 47 (D1), D607–D613. doi:10.1093/nar/gky1131
Tang, H., Dalton, C. F., Srisawat, U., Zhang, Z. J., and Reynolds, G. P. (2014). Methylation at a transcription factor-binding site on the 5-HT1A receptor gene correlates with negative symptom treatment response in first episode schizophrenia. Int. J. Neuropsychopharmacol. 17 (4), 645–649. doi:10.1017/s1461145713001442
Trubetskoy, V., Pardiñas, A. F., Qi, T., Panagiotaropoulou, G., Awasthi, S., Bigdeli, T. B., et al. (2022). Mapping genomic loci implicates genes and synaptic biology in schizophrenia. Nature 604 (7906), 502–508. doi:10.1038/s41586-022-04434-5
Walton, E., Hass, J., Liu, J., Roffman, J. L., Bernardoni, F., Roessner, V., et al. (2016). Correspondence of DNA methylation between blood and brain tissue and its application to schizophrenia research. Schizophr. Bull. 42 (2), 406–414. doi:10.1093/schbul/sbv074
Wang, Q., Man Wu, H., Yue, W., Yan, H., Zhang, Y., Tan, L., et al. (2018). Effect of damaging rare mutations in synapse-related gene sets on response to short-term antipsychotic medication in Chinese patients with schizophrenia: A randomized clinical trial. JAMA Psychiatry 75 (12), 1261–1269. doi:10.1001/jamapsychiatry.2018.3039
Weinberger, D. R. (2019). Thinking about schizophrenia in an era of genomic medicine. Am. J. Psychiatry 176 (1), 12–20. doi:10.1176/appi.ajp.2018.18111275
Yadav, S., Oses-Prieto, J. A., Peters, C. J., Zhou, J., Pleasure, S. J., Burlingame, A. L., et al. (2017). TAOK2 kinase mediates PSD95 stability and dendritic spine maturation through Septin7 phosphorylation. Neuron 93 (2), 379–393. doi:10.1016/j.neuron.2016.12.006
Yu, H., Yan, H., Wang, L., Li, J., Tan, L., Deng, W., et al. (2018). Five novel loci associated with antipsychotic treatment response in patients with schizophrenia: A genome-wide association study. Lancet Psychiatry 5 (4), 327–338. doi:10.1016/s2215-0366(18)30049-x
Yu, N. Y., Hallström, B. M., Fagerberg, L., Ponten, F., Kawaji, H., Carninci, P., et al. (2015). Complementing tissue characterization by integrating transcriptome profiling from the Human Protein Atlas and from the FANTOM5 consortium. Nucleic Acids Res. 43 (14), 6787–6798. doi:10.1093/nar/gkv608
Keywords: pharmacoepigenomics, antipsychotic response, MWAS, DMP, DMR
Citation: Du H, Ma J, Zhou W, Li M, Huai C, Shen L, Wu H, Zhao X, Zhang N, Gao S, Wang Q, He L, Wu X, Qin S and Zhao M (2022) Methylome-wide association study of different responses to risperidone in schizophrenia. Front. Pharmacol. 13:1078464. doi: 10.3389/fphar.2022.1078464
Received: 24 October 2022; Accepted: 12 December 2022;
Published: 22 December 2022.
Edited by:
Peng Chen, Jilin University, ChinaReviewed by:
Santasree Banerjee, Beijing Genomics Institute (BGI), ChinaCopyright © 2022 Du, Ma, Zhou, Li, Huai, Shen, Wu, Zhao, Zhang, Gao, Wang, He, Wu, Qin and Zhao. This is an open-access article distributed under the terms of the Creative Commons Attribution License (CC BY). The use, distribution or reproduction in other forums is permitted, provided the original author(s) and the copyright owner(s) are credited and that the original publication in this journal is cited, in accordance with accepted academic practice. No use, distribution or reproduction is permitted which does not comply with these terms.
*Correspondence: Xuming Wu, eHVtaW5nd3VAbnR1LmVkdS5jbg==; Shengying Qin, Y2hpbnNpckBzanR1LmVkdS5jbg==; Mingzhe Zhao, emhhb21pbmd6aGVAc2p0dS5lZHUuY24=
†These authors have contributed equally to this work and share first authorship
Disclaimer: All claims expressed in this article are solely those of the authors and do not necessarily represent those of their affiliated organizations, or those of the publisher, the editors and the reviewers. Any product that may be evaluated in this article or claim that may be made by its manufacturer is not guaranteed or endorsed by the publisher.
Research integrity at Frontiers
Learn more about the work of our research integrity team to safeguard the quality of each article we publish.