- 1Department of Pharmacology, NGSM Institute of Pharmaceutical Sciences (NGSMIPS), Nitte (Deemed to be University), Mangalore, India
- 2ICMR-National Institute of Traditional Medicine, Belagavi, Karnataka, India
- 3Department of Microbiology, Shivaji University, Kolhapur, India
- 4Department of Pharmacology, KLE College of Pharmacy Belagavi, KLE Academy of Higher Education and Research (KAHER), Belagavi, India
- 5PRES’s Pravara Rural College of Pharmacy Pravaranagar, Loni, Maharashtra, India
- 6Pratiksha Institute of Pharmaceutical Sciences, Guwahati, Assam, India
- 7Royal School of Pharmacy, The Assam Royal Global University, Guwahati, Assam, India
Aim: The purpose of this study was to establish a mode of action for diosgenin against breast cancer employing a range of system biology tools and to corroborate its results with experimental facts.
Methodology: The diosgenin-regulated domains implicated in breast cancer were enriched in the Kyoto Encyclopedia of Genes and Genomes database to establish diosgenin-protein(s)-pathway(s) associations. Later, molecular docking and the lead complexes were considered for molecular dynamics simulations, MMPBSA, principal component, and dynamics cross-correlation matrix analysis using GROMACS v2021. Furthermore, survival analysis was carried out for the diosgenin-regulated proteins that were anticipated to be involved in breast cancer. For gene expression analyses, the top three targets with the highest binding affinity for diosgenin and tumor expression were examined. Furthermore, the effect of diosgenin on cell proliferation, cytotoxicity, and the partial Warburg effect was tested to validate the computational findings using functional outputs of the lead targets.
Results: The protein-protein interaction had 57 edges, an average node degree of 5.43, and a p-value of 3.83e-14. Furthermore, enrichment analysis showed 36 KEGG pathways, 12 cellular components, 27 molecular functions, and 307 biological processes. In network analysis, three hub proteins were notably modulated: IGF1R, MDM2, and SRC, diosgenin with the highest binding affinity with IGF1R (binding energy −8.6 kcal/mol). Furthermore, during the 150 ns molecular dynamics (MD) projection run, diosgenin exhibited robust intermolecular interactions and had the least free binding energy with IGF1R (−35.143 kcal/mol) compared to MDM2 (−34.619 kcal/mol), and SRC (-17.944 kcal/mol). Diosgenin exhibited the highest cytotoxicity against MCF7 cell lines (IC50 12.05 ± 1.33) µg/ml. Furthermore, in H2O2-induced oxidative stress, the inhibitory constant (IC50 7.68 ± 0.51) µg/ml of diosgenin was lowest in MCF7 cell lines. However, the reversal of the Warburg effect by diosgenin seemed to be maximum in non-cancer Vero cell lines (EC50 15.27 ± 0.95) µg/ml compared to the rest. Furthermore, diosgenin inhibited cell proliferation in SKBR3 cell lines more though.
Conclusion: The current study demonstrated that diosgenin impacts a series of signaling pathways, involved in the advancement of breast cancer, including FoxO, PI3K-Akt, p53, Ras, and MAPK signaling. Additionally, diosgenin established a persistent diosgenin-protein complex and had a significant binding affinity towards IGF1R, MDM2, and SRC. It is possible that this slowed down cell growth, countered the Warburg phenomenon, and showed the cytotoxicity towards breast cancer cells.
Introduction
Breast cancer spreads through the inner layer of the milk gland or lobules and ducts (Sariego, 2010), and it is one of the second leading causes of mortality for women between the ages of 45 and 55 (Jemal et al., 2009). It includes age, iodine deficiency (Venturi, 2001; Aceves et al., 2005; Stoddard et al., 2008), high hormone levels (Russo and Russo, 2006; Yager and Davidson, 2006), and age-related (Steiner et al., 2008) risk factors. This incident potentially results in the breast being surgically removed entirely or in requiring chemo-, radio-, or hormone-therapy (HeraviKarimovi et al., 2006). However, these practises are preoccupied with multiple side effects that are not specific to the breast tumor. Furthermore, the currently used chemotherapeutic drugs in medical care result in anemia, exhaustion, mouth soreness, vomiting, and diarrhea. This suggests the requirement to discover a novel therapeutic agent against breast cancer.
Diosgenin is a phyto steroid sapogenin obtained from the hydrolysis by strong bases, acids, or enzymes of saponin. It is commercially used as a precursor to synthesis various hormones and steroid products like pregnenolone, and cortisone including progesterone (Marker and Krueger, 1940) for the early manufacture of combined oral contraceptive pills (Djerassi, 1992). Additionally, diosgenin has the potential to inhibit activated pro-inflammatory and pro-survival signaling pathways and promote the death of a variety of cancer cells (Shishodia and Aggarwal, 2006). Additionally, diosgenin inhibits the growth of oestrogen receptor-positive MCF-7 cells by activating caspase three and upregulating the p53 tumor suppressor gene. Further, BCL2 is downregulated in estrogen receptor-negative MDA-MB-231 breast cancer cells (Srinivasan et al., 2009). Although the potential of diosgenin to treat breast cancer has been demonstrated its interaction with proteins involved in the progenesis of breast cancer has yet to be investigated.
As a result, the current study focuses on locating the potential interactions of the diosgenin-regulated proteins and attributable pathways implicated in breast cancer using a range of system biology techniques i.e., gene ontology (GO) analysis, molecular docking, and molecular dynamics (MD) simulations, and reinforcing its findings using diverse functional biomarkers using in vitro experiments in four distinct cell lines.
Materials and methods
Computational pharmacology
The detected diosgenin-regulated proteins were enriched to determine the altered pathways. Later, diosgenin was docked with proteins, and the proteins with the maximum diosgenin binding affinity were chosen for MD simulation. Furthermore, the diosgenin-regulated proteins involved in breast cancer were examined for survival and gene expression in normal and tumor cell lines, including the three hub proteins determined by molecular docking.
ADMET profile of diosgenin and its targets
Canonical SMILES of diosgenin (Figure 1) were retrieved from the PubChem database (https://pubchem.ncbi.nlm.nih.gov/) and ADME profile was predicted by SwissADME (Daina et al., 2017; http://www.swissadme.ch/) and ADMETlab 2.0 (Xiong et al., 2021; https://admetmesh.scbdd.com) and adverse effects from ADVERPred (Ivanov et al., 2018; http://www.way2drug.com/adverpred/).
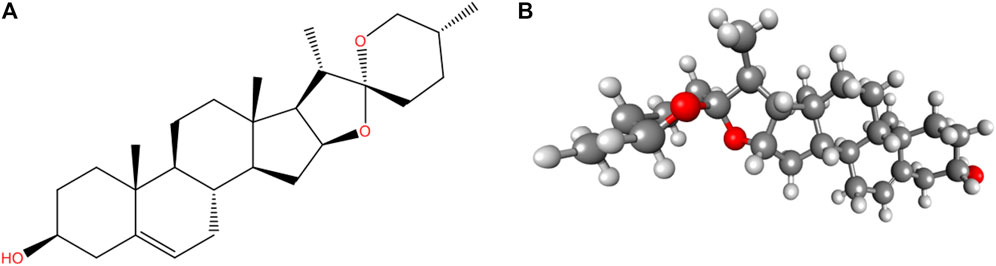
FIGURE 1. Structure of Diosgenin (A) 2D and (B) 3D (ball and stick). PubChem CID: 99474, Molecular Formula: C27H42O3, Synonyms: Nitogenin, UNII-K49P2K8WLX, (3β, 25R)-spirost-5-en-3-ol, Molecular Weight: 414.6.
Diosgenin-modulated targets were predicted using SwissTargetPrediction (Daina et al., 2019; http://www.swisstargetprediction.ch/), DIGEP-Pred (Lagunin et al., 2013; http://www.way2drug.com/ge/), and BindingDB (Liu et al., 2007; https://www.bindingdb.org/bind/index.jsp) and any duplicates were eliminated. In addition, the semantic type (neoplastic process) oestrogen receptor-positive breast cancer targets (UMLS CUI: C2938924), were retrieved from DisGeNET (https://www.disgenet.org/). Later, the diosgenin-modulated targets were compared with DisGeNET-recorded targets to trace the reported targets involved in oestrogen receptor-positive breast cancer using PivotTable (Microsoft excel 2007; https://www.microsoft.com/en-in/microsoft-365/excel).
GO and cluster analysis
The diosgenin-modulated targets involved in oestrogen receptor-positive breast cancer were queried in STRING (Szklarczyk et al., 2021; https://string-db.org/) ver 11.5 for “Homo sapiens” to trace three GO terms i.e., cellular components, molecular function, and biological processes. In addition, probable regulations of multiple pathways were also traced concerning the KEGG database (https://www.genome.jp/kegg/pathway.html) with whole genome statistical background. Also, the regulated proteins were concerned with subcellular location (COMPARTMENT), protein domains and features (InterPro), protein domain (Pfam), and tissue expression (TISSUES). Later, the protein-protein interaction (PPI) was also assessed for the cluster analysis via k means clustering to identify three lead distinct clusters.
Network construction and analysis
The network between diosgenin, its targets (involved in oestrogen receptor-positive breast cancer), and the regulated pathways were constructed using Cytoscape ver 3.5.1 (Shannon et al., 2003; https://cytoscape.org/). The constructed network was recognized as directed and inspected by translating node size and color to low values corresponding to small sizes and low values corresponding to bright colors toward edge count. In addition, the edge size and color were mapped to edge betweenness, with low values corresponding to small sizes and low values equating to bright colors.
Molecular docking
The regulation of insulin-like growth factor 1 receptor (IGF1R), E3 ubiquitin-protein ligase Mdm2 (MDM2), and proto-oncogene tyrosine-protein kinase Src (SRC) were majorly triggered in the network interaction among diosgenin-target(s)-pathway(s). As a result, these three proteins were considered for molecular docking studies.
Ligand preparation: The ligand’s 3D conformation, diosgenin, was obtained from the PubChem database (https://pubchem.ncbi.nlm.nih.gov) and converted to .pdb using Discovery Studio Visualizer (https://discover.3ds.com/discover-studio-visualizer-download) ver. 2019. The ligand’s energy was minimized using the mmff94 force field (Halgren 1996) and saved in .pdbqt format.
Preparation of macromolecule: Diosgenin was docked against IGF1R (PDB: 3I81), MDM2 (PDB: 3LBL), and SRC (PDB: 1O43); retrieved from a protein data bank maintained by the Research Collaboratory for Structural Bioinformatics (RCSB; https://www.rcsb.org). All proteins were refined and saved in .pdb format after eliminating all heteroatoms and pre-complex ligands.
Ligand–protein docking: Diosgenin was docked against the aforementioned targets using AutoDock Vina, which was run through the POAP pipeline (Trott and Olson 2010; Samdani and Vetrivel 2018; Patil et al., 2022). Nine different poses of ligand were obtained after docking. Docking results were analysed based on the binding affinity, and number of interactions as explained previously (Dwivedi et al., 2021; Badraoui et al., 2022). Further, the diosgenin pose with the lowest binding energy was chosen to visualize the ligand-protein interactions and perform MD simulation (Samdani and Vetrivel 2018).
MD simulation
To examine the structural stabilities and intermolecular interactions of diosgenin with IGF1R, MDM2, and SRC, an all-atom MD simulation in an explicit solvent was performed for 150 ns. We used the GROMACS software package, ver. 2021.3 (https://www.gromacs.org/) and Amber ff99SB-ildn force field to run MD simulations (Berendsen et al., 2005; Saeed et al., 2020; Dwivedi et al., 2022). The topological parameters of the ligands and the entire complex were calculated using the AmberTool’s xleap module (https://ambermd.org/AmberTools.php) and the partial charges of the small molecules were calculated using an antechamber with a “bcc” charge model. The built systems were solvated in a rectangular box with 10.0 Å boundary conditions from the protein’s borders in all directions using the TIP3P water model. The required amounts of counter ions were introduced to the prepared systems to neutralize the charges. The steepest descent and conjugate gradient energy reduction methods were used to discover the least energy conformations of the near-global state. To equilibrate the systems for 1 ns, “Canonical (NVT) and isobaric (NPT) ensembles” were used. A modified Berendsen thermostat approach was utilized to keep the volume and temperature consistent during NVT equilibration (300 K). During NPT equilibration, a Parrinello-Rahman barostat was used to keep the pressure constant at 1 bar. Furthermore, the Particle Mesh Ewald approximation with a cut-off value of 1 nm was used to calculate the long-range electrostatic interactions, van der Waals, and Coulomb interactions. A similar LINear Constraint Solver method was used to constrain bond length. Every complex went through a 150 ns production run with coordinates recorded every 2 fs. Other software programs, in addition to the built-in gromacs tools, were utilized to perform specific analyses on the acquired trajectories as required.
Analysis of free binding energy of complexes utilizing molecular mechanics Poisson–Boltzmann surface area (MM-PBSA)
In MD simulations and thermodynamic calculations, the relative binding energy of a ligand-protein complex was employed to investigate the binding free energies. The relative binding free energy and its contribution to individual residues were calculated using the MM-PBSA method and the “g_mmpbsa” tool (Kumari et al., 2014; Dwivedi et al., 2022). The parameters for binding free energy calculations were taken from our previous study (Khanal et al., 2022). The binding free energy (ΔG) was calculated using 50 representative snapshots taken throughout the stable trajectory observed between 100 and 150 ns. The change in entropy (ΔS) was calculated using the Schlitter formula and finally, accurate binding free energy was calculated using the formula, ΔG = ΔH–TΔS, where, ΔG = Gibbs free energy, ΔH = enthalpy change, T = temperature (Kelvin), and ΔS = entropy change.
Principal component (PCA) and dynamic cross-correlation matrix (DCCM) analysis
PCA was performed over the stable MD trajectory to examine various forms of molecular motion (Amadei et al., 1993; Amadei et al., 1996; Bhandare and Ramaswamy 2018). To accomplish this, the “least square fit” to the reference structure was used to account for the molecular translational and rotational motion. The collection of eigenvectors obtained by diagonalizing a covariance matrix was produced by a linear transform of cartesian coordinate space to reflect the direction of the molecular motion. The energy contribution of each eigenvector to the motion was presented by the eigenvalue associated with the respective eigenvector. The “time-dependent motions” that the components carry out in an atomic vibrational mode were demonstrated by projecting the trajectory onto a particular eigenvector. The atomic vibrational components’ contribution to this form of coordinated motion was shown by the projection’s time average (Amadei et al., 1993; Van Aalten et al., 1995; Amadei et al., 1996; Bhandare and Ramaswamy 2018).
DCCM evaluates the magnitude of each pairwise cross-correlation coefficient to determine whether or not atomic pair motion is correlated i.e., positive or negative (Khanal et al., 2021). We examined each DCCM component in this section, where Cij = 1 denotes the same period and phase (positive correlation), Cij = 0 indicates a lack of correlation, and Cij = −1 indicates a negative correlation between the fluctuations of i and j (Khanal et al., 2021).
Survival analysis of hub genes
To assess the prognostic values of hub genes of diosgenin-regulated oestrogen receptor-positive breast cancer targets, the Kaplan–Meier plotter breast cancer database (http://kmplot.com/analysis/) was used with APP, AR, CDK4, CRHR1, CYP17A1, CYP19A1, CYP3A4, FASN, FGFR2, GRM1, IGF1R, LYN, MDM2, MDM4, NR3C1, PDGFRB, PRCP, PTPN1, RET, SRC, and STAT3 probe ID. Patients were divided into two groups with “auto-detected best cutoff”; overall survival was analyzed against n = 2,976 samples with a follow-up of 20 years of data. Also, the subtype analysis was restricted to lymph node status (n = 2,887), ER status (n = 2,789), PGR status (n = 2,662), HER2 status (n = 2,875), KI67 status (n = 1,360), Nottingham histologic grade (n = 2,917), and PAM50 subtype (n = 2,976) and cohort restriction i.e., endocrine treated (n = 2,955) and chemo treated (n = 2,956). The difference among the groups was considered to be statistically significant if p < 0.05.
Gene expression analysis in tumor, normal and metastatic tissues
Herein, we evaluated the top three genes based on the log-rank test from survival analysis, and in silico molecular docking was evaluated for the gene expression in normal, tumor, and metastatic tissues using RNA sequence data in the platform of tumor, normal and metastatic samples. These data were analyzed using Kruskal Wallis and Dunn test.
Experimental pharmacology
Through different in vitro pharmacology profiling, we investigated the influence of diosgenin on breast cancer cell lines. To begin, brine shrimp lethality (BSL) bioassay was used to assess its cytotoxicity and the effect was compared with doxorubicin. Later, the effect of diosgenin on breast cancer cell lines (MCF7, MDA-MB-231, SKBR3, and T47D) was compared to normal epithelial cell lines (Vero) by emphasizing on cell viability, proliferation, and Warburg effect.
BSL bioassay
The brine shrimp lethality bioassay was performed as explained by McLaughlin et al. (1998) with minor modifications. Here, Artemia salina Leach. eggs from Seamonk international Artemia cyst 003 were used for the assay. Briefly, 10–12 brine shrimps were incubated within the different concentrations of diosgenin and doxorubicin (prepared in seawater) for 24 h. Controls were used without the test agents. After 24 h, the survived shrimps were counted and the % cytotoxicity was calculated as
The LC50 was calculated using a linear regression curve.
In vitro MTT assay tumor and non-tumor cell lines
The cytotoxic activity of diosgenin and doxorubicin on tumor and normal cell lines was performed using an MTT assay (Mosmann, 1983) with minor modifications. Briefly, cell lines were plated onto 96-well flat-bottom plates, maintaining the cell density (20,000 cells/well), and were allowed to proliferate (24 h). After that, the cells were treated with different concentrations of diosgenin and doxorubicin maintaining the final volume of 250 µL after adding DMEM media (supplemented with 3% FBS) and incubated (37°C, 48 h, 5% CO2). Next, 20 μL of MTT reagent was added and incubated (37°C in 5% CO2, 4 h). After incubation, the wells were washed (PBS, 3X) to discard the MTT. Then, formazan crystals were dissolved in DMSO (99.5% v/v, 100 μL) by gentle shaking. The absorbance was then recorded (570 nm) using an ELISA plate reader. The cell viability was calculated as
In vitro scratch assay
In vitro scratch assay was performed as explained by Bolla et al (2019) with minor modifications. Briefly, a stock solution (200 μg/ml) of diosgenin and doxorubicin was prepared and sterilized by filtering using a sterile membrane filter (0.22 µm). Later the solution was diluted using geometric series up to 1.56 μg/ml. This series of concentrations were chosen based on the number of experiments (trial and error). All the cells were seeded (2 × 105 cells/well) in 12-well tissue culture plates to obtain the confluence of 80–90% after 24 h of culture. After 24 h of post-seeding, the cell monolayers were scraped to create scratches of (300 µm). The detached cells and debris were washed with phosphate buffer. The media containing the samples were added to each well. Suitable controls were used by adding the minimal media and the scratch coverage was recorded at 0, 12, 24, 48, and 72 h after sample addition. The percentage scratch coverage was calculated using the following formula
Effect of diosgenin on Warburg effect
The effect of diosgenin on the Warburg phenomena was evaluated by evaluating glucose uptake via the above-mentioned cancer cell lines vs. normal cell lines (Vero). Initially, the cells were grown in six well plates and incubated (37°C, 48 h) in a CO2 incubator. After the formation of the confluent monolayer, the culture was renewed (DMEM consisting of 0.2% BSA) and again incubated (37°C, 18 h) in the CO2 incubator. After incubation, the media was discarded and washed with KRP buffer. The cells were then treated with diosgenin and metformin in the presence of insulin followed by the addition of glucose (1 M) and incubated (30 min). The remaining amount of glucose was quantified from the supernatant. The percentage glucose uptake was calculated as the difference between the initial and final glucose content in the incubated medium (Gupta et al., 2009).
Statistical analysis
For the enrichment analysis, the whole-genome statistical background was used. The inhibitory constant (IC50) and effective concentration (EC50) were calculated using linear regression in GraphPad Prism (https://www.graphpad.com/) ver 5.0.
Results
Computational pharmacology
Diosgenin ADMET profile and its targets
Diosgenin has molecular weight of 414.62 g/mol with 3 H-bond acceptors and 1 H-bond donor. Diosgenin was predicted to possess the high human intestinal absorption (>30). In addition, it also showed the plasma protein binding, fraction unbound in plasma, and its volume of distribution 97.743%, 1.872%, and 1.695 L/kg, respectively. It was predicted as no inhibitory action towards CYP1A2, CYP2C19, CYP2C9, CYP2D6, and CYP3A4 and showed high clearance rate of 23.332 ml/min/kg. In addition, it was not predicted for any side effects based on the ADVERPred server projection.
Diosgenin was predicted to regulate 105 targets in SwissTargetPrediction and four in DIGEP-Pred and BindingDB. The predicted targets in BindingDB were in common with SwissTargetPrediction. Similarly, a total of 510 different targets were recorded in DisGeNET for the neoplastic process of oestrogen receptor-positive breast cancer targets (UMLS CUI: C2938924). In general, the Diosgenin targeted 21 different proteins involved in breast cancer compared to recorded targets in DisGeNET (UMLS CUI: C2938924); Supplementary Figure S1.
Gene set enrichment analysis
The interaction of diosgenin-targeted 21 proteins had a total of 57 edges, 5.43 average node degree, 0.701 average local clustering coefficient, 17 edges (expected), and 3.83e-14 enrichment p-value (Figure 2).
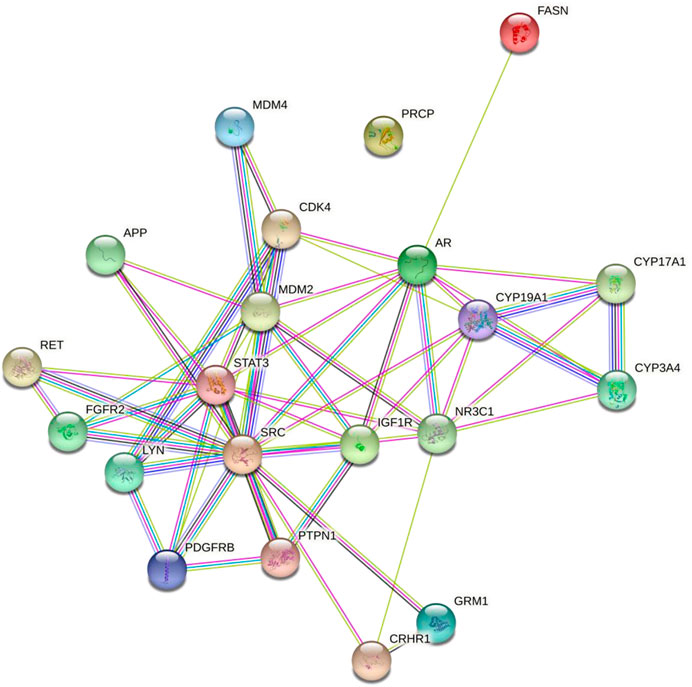
FIGURE 2. Protein-protein interaction of the diosgenin-triggered protein. Node color; colored nodes: query proteins and first shell of interactors,
white nodes: second shell of interactors, Node content;
empty nodes: proteins of unknown 3D structure,
filled nodes: some 3D structure is known or predicted, Known Interactions;
from curated databases,
experimentally determined, Predicted Interactions;
gene neighborhood,
gene fusions,
gene co-occurrence and Others;
text mining,
co-expression,
protein homology.
KEGG enrichment analysis
Concerning the KEGG record, the PPI reflected the regulation of 36 different pathways in which EGFR tyrosine kinase inhibitor resistance (hsa01521) had the lowest false discovery rate i.e., 1.06E-05 via the regulation of five genes i.e., PDGFRB, STAT3, IGF1R, SRC, and FGFR2 against 78 background genes at 1.78 strength. Additionally, eight different pathways were detected for keyword “cancer” i.e. pathways in cancer; hsa05200 (regulated eight genes i.e. CDK4, MDM2, PDGFRB, STAT3, IGF1R, RET, AR, and FGFR2 against 517 background genes at 1.16 strength and 1.06E-05 false discovery rate), prostate cancer; hsa05215 (regulated five genes i.e. MDM2, PDGFRB, IGF1R, AR, and FGFR2 against 96 background genes at 1.69 strength and 1.06E-05 false discovery rate), bladder cancer; hsa05219 (regulated three genes i.e. CDK4, MDM2, and SRC against 41 background genes at 1.83 strength and 0.00062 false discovery rate), microRNAs in cancer; hsa05206 (regulated four genes i.e. MDM2, PDGFRB, STAT3, and MDM4 against 160 background genes at 1.37 strength and 0.00099 false discovery rate), proteoglycans in cancer; hsa05205 (regulated four genes i.e. MDM2, STAT3, IGF1R, and SRC against 196 background genes at 1.28 strength and 0.0015 false discovery rate), central carbon metabolism in cancer; hsa05230 (regulated three genes i.e. PDGFRB, RET, and FGFR2 against 69 background genes at 1.61 strength and 0.0015 false discovery rate), non-small cell lung cancer; hsa05223 (regulated two genes i.e. CDK4 and STAT3 against 68 background genes at 1.44 strength and 0.0349 false discovery rate), and pancreatic cancer; hsa05212 (regulated two genes i.e. CDK4 and STAT3 against 73 background genes at 1.41 strength and 0.0387 false discovery rate). Also, a total of 129 genes were modulated in 36 different pathways in which insulin-like growth factor-1 receptor (IGF1R) was majorly triggered in 18 different pathways i.e., EGFR tyrosine kinase inhibitor resistance; hsa01521, pathways in cancer; hsa05200, prostate cancer; hsa05215, long-term depression; hsa04730, glioma; hsa05214, melanoma; hsa05218, endocrine resistance; hsa01522, ovarian steroidogenesis; hsa04913, signaling pathways regulating pluripotency of stem cells; hsa04550, adherens junction; hsa04520, proteoglycans in cancer; hsa05205, endocytosis; hsa04144, focal adhesion; hsa04510, and Ras; hsa04014, MAPK; hsa04010, FoxO; hsa04068, PI3K-Akt; hsa04151, and Rap1 signaling pathway; hsa04015 (Supplementary File S1; Supplementary Sheet S1). The modulated genes in the KEGG pathways were observed to be common with three GO terms i.e., cellular components, molecular function, and biological processes (Supplementary Figure S2); detailed below. Further, the interaction between the diosgenin-modulated targets and triggered pathways is presented in Figure 3. The associated protein-pathways interaction with respective false discovery rate and strengths is presented in Figure 4.
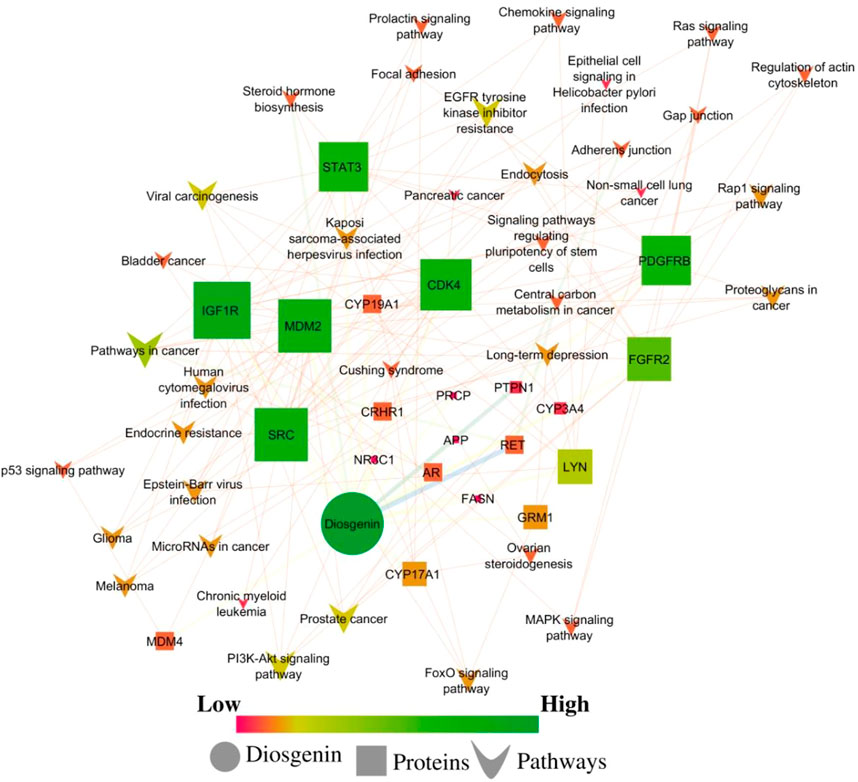
FIGURE 3. Network interaction of diosgenin-regulated proteins and respective pathways. Pink color presents a low edge count and green color presents a high edge count. A large node size presents a higher edge count and a smaller edge count presents a lower edge count.
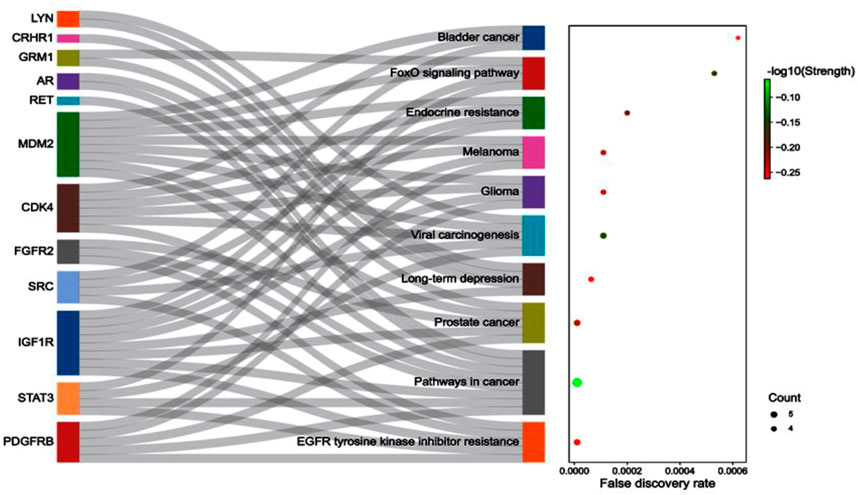
FIGURE 4. The associated protein-pathways interaction with respective false discovery rate and strength.
GO analysis
GO analysis identified 12 GO terms for cellular components in which receptor complex; GO:0043235 had the lowest false discovery rate i.e., 0.00025 regulated seven genes i.e., PDGFRB, IGF1R, APP, RET, GRM1, FGFR2, and LYN against 381 genes at 1.23 strength. Also, a total of 21 genes were triggered in multiple cellular components in which tyrosine-protein kinase Lyn (LYN) was majorly triggered in 11 cellular components except for cytoplasmic vesicles; GO:0031410 (Supplementary File S1; Supplementary Sheet S1). In addition, the Pearson p-value for cellular components was 0.795 strength vs. false discovery rate, 0.0003 strength vs. observed gene count, 0.8093 false discovery rate vs. observed gene count, 2.684e-004 observed gene count vs. strength, and 0.809 observed gene count vs. false discovery rate. In addition, the minimum observed Pearson r was −0.084 and the maximum was 1.000 (Supplementary Figure S3). The proteins modulated by the diosgenin in the different cellular compartments are presented in Figure 5.
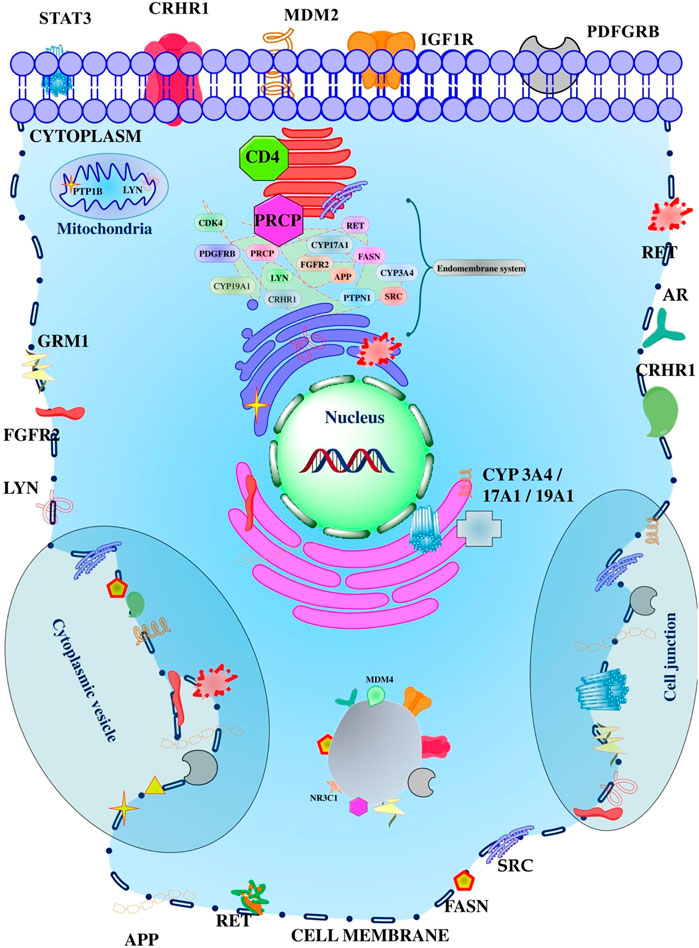
FIGURE 5. Different proteins affected by the diosgenin in the various compartment of the cell. The cellular organelles presented reflect the membrane-bounded organelle or intracellular organelle.
Likewise, 27 different molecular functions GO terms were identified via the PPI in which protein tyrosine kinase activity; GO:0004713 had the lowest false discovery rate i.e., 3.53E-05 to trigger six genes i.e., PDGFRB, IGF1R, RET, SRC, FGFR2, and LYN against 137 background genes at 1.61 strength. Herein, a total of 22 genes were triggered in 27 molecular functions in which proto-oncogene tyrosine-protein kinase Src (SRC) was involved in 18 molecular functions except hormone binding; GO:0042562, transmembrane receptor protein tyrosine kinase activity; GO:0004714, transition metal ion binding; GO:0046914, steroid hydroxylase activity; GO:0008395, oxygen binding; GO:0019825, nuclear receptor activity; GO:0004879, peptide hormone binding; GO:0017046, platelet-derived growth factor receptor binding; GO:0005161, and steroid-binding; GO:0005496 (Supplementary File S1; Supplementary Sheet S3). Herein, the correlation p values were 0.130 strength vs. false discovery rate, 2.0510e-009 strength vs. observed gene count, and 0.753 false discovery rate vs. observed gene count. Within the interaction of strength, false discovery rate, and observed gene count, −0.877 and 1.000 were minimum and maximum r values for molecular function (Supplementary Figure S3).
Similarly, a total of 307 biological processes was traced due to PPI in which cellular response to oxygen-containing compound; GO:1901701 had the lowest false discovery rate i.e., 2.72E-09 in modulating 14 genes i.e., NR3C1, CDK4, MDM2, PDGFRB, STAT3, IGF1R, APP, RET, PTPN1, SRC, AR, CRHR1, FGFR2, and LYN against 1,055 background proteins at 1.09 strength. In addition, a total of 21 genes were triggered for 307 biological processes via the 21 proteins associated with breast cancer in which SRC was involved and linked with 213 biological processes. In addition, the order of triggered genes in multiple pathways was as CYP17A1 (17) < CYP3A4 (22) < PRCP (33) < CRHR1 (35) < FASN (42) < GRM1 (52) < CYP19A1 (59) < NR3C1 (60) < MDM4 (71) < CDK4 (111) < IGF1R (113) < PTPN1 (114) < RET (130) < MDM2 (134) < AR (139) < STAT3 (140) < FGFR2 (149) < PDGFRB (160) < LYN (161) < APP (198) < SRC (213). The detailed biological processes of participation of the above genes are supplemented in Supplementary File S1; Supplementary Sheet S4. The Pearson correlation p-value was found to be 0.034 strength vs. false discovery rate, 1.0622e-063 strength vs. observed gene count, and 8.1767e-017 false discovery rate vs. observed gene count. In addition, the minimum Pearson r values for biological processes were -0.779 and 1.000 respectively (Supplementary Figure S3).
Compartments enrichment analysis
The PPI reflected the modulation of proteins in 16 different compartments in which the intrinsic component of plasma membrane; GOCC:0031226 had the lowest false discovery rate i.e., 0.00027 in regulating nine genes i.e., PDGFRB, STAT3, IGF1R, APP, RET, GRM1, CRHR1, FGFR2, and LYN against 841 background genes at 1 strength. Herein, a total 21 were triggered in 16 compartments in which LYN was traced in all; the order of genes regulation in multiple enriched compartments was MDM4 (5) < NR3C1 (6) = AR (6) < CYP17A1 (7) < CYP19A1 (8) = CYP3A4 (8) = CDK4 (8) = GRM1 (8) = MDM2 (8) < FASN (9) = PDGFRB (9) = PRCP (9) = PTPN1 (9) = SRC (9) < CRHR1 (12) = FGFR2 (12) < IGF1R (13) < RET (14) = STAT3 (14) < APP (15) < LYN (16); Supplementary File S1; Supplementary Sheet S5.
InterPro enrichment analysis
Protein-protein enrichment analysis traced seven InterPro in which tyrosine-protein kinase, catalytic domain; IPR020635 was traced with minimum false discovery rate i.e., 8.42E-06 to regulate six genes i.e., PDGFRB, IGF1R, RET, SRC, FGFR2, and LYN against 79 background genes at 1.85 strength. Herein, in the enrichment of InterPro analysis, a total of nine genes were triggered in order as MDM2 (1) = MDM4 (1) < CDK4 (3) < FGFR2 (6) = IGF1R (6) = LYN (6) = PDGFRB (6) = RET (6) = SRC (6); Supplementary File S1; Supplementary Sheet S6.
Pfam enrichment analysis
Enrichment analysis concerning to Pfam database traced the regulation of protein kinase domain; PF00069 and protein tyrosine kinase; PF07714 at the false discovery rate of 0.0068 to regulate seven genes i.e., CDK4, PDGFRB, IGF1R, RET, SRC, FGFR2, and LYN at 1.13 strength against 486 and 481 background genes respectively; Supplementary File S1; Supplementary Sheet S7.
Tissues enrichment analysis
Enrichment analysis of PPI for TISSUES traced 13 different TISSUES terms in which erythroleukemia cell; BTO:0000426 was traced with the lowest false discovery rate i.e., 0.0088 in regulating five genes i.e., MDM2, STAT3, PTPN1, SRC, and LYN at 1.32 strength against 225 background genes. Herein, there was a regulation of 21 genes i.e. APP, AR, CDK4, CRHR1, CYP17A1, CYP19A1, CYP3A4, FASN, FGFR2, GRM1, IGF1R, LYN, MDM2, MDM4, NR3C1, PDGFRB, PRCP, PTPN1, RET, SRC, and STAT3 in which nuclear receptor subfamily 3, group C, member 1 (NR3C1) was majorly triggered in 10 different TISSUES i.e. bone marrow cancer cell (BTO:0000583), organism form (BTO:0000284), leukemia cell (BTO:0001271), fibroblast (BTO:0000452), liver (BTO:0000759), whole body (BTO:0001489), reproductive system (BTO:0000081), embryonic structure (BTO:0000174), endocrine gland (BTO:0001488) and connective tissue (BTO:0000421); Supplementary File S1; Supplementary Sheet S8.
Cluster analysis
K means analysis of PPI against the whole genome of Homo sapiens traced three sets of clusters which are indicated by red (cluster 1; included AR, CYP17A1, CYP19A1, CYP3A4, FASN, NR3C1, and PRCP), green (cluster 2; included APP, CDK4, CRHR1, FGFR2, GRM1, IGF1R, LYN, PDGFRB, PTPN1, RET, SRC, and STAT3) and blue (cluster 3; included MDM2 and MDM4); Supplementary Table S1.
In cluster 1, the interaction of seven nodes corresponded to 11 edges with 3.14 average node degree, 0.8 average local clustering coefficients, 1 expected edge, and 1.07e-09 PPI enrichment p-value. Herein, a total of nine molecular functions were traced in which steroid hydroxylase activity (GO:0008395) and oxygen binding (GO:0019825) scored the lowest false discovery rate i.e., 0.0013 via the regulation of three genes i.e., CYP3A4, CYP17A1, and CYP19A1 against 36 background genes at 2.37 strength. Similarly, Steroid binding (GO:0005496) showed 0.0082 false discovery rate and regulated three genes i.e., NR3C1, CYP3A4, and AR against 104 background genes at a strength of 1.91. Similarly, two KEGG pathways i.e., steroid hormone biosynthesis (hsa00140) and ovarian steroidogenesis (hsa04913) were traced. Herein, steroid hormone biosynthesis was identified with a false discovery rate of 0.00048 to regulate three genes i.e., CYP3A4, CYP17A1, and CYP19A1 against 59 background genes at 2.15 strength. In addition, ovarian steroidogenesis regulated two genes i.e., CYP17A1 and CYP19A1 against 50 genes at 2.05 strength and 0.0327 false discovery rate. Also, two different biological processes i.e., androgen metabolic process (GO:0008209) and organic cyclic compound biosynthetic process (GO:1901362) were identified to regulate three genes i.e., CYP3A4, CYP17A1, and CYP19A1 against 27 background genes at 2.47 strength and 0.002 false discovery rate and six genes (NR3C1, FASN, CYP3A4, CYP17A1, AR, and CYP19A1I) against 1,211 background genes at 1.14 strength and 0.0033 respectively (Supplementary File S2; Supplementary Sheet S1).
In cluster 2, 12 protein interactions traced 25 edges with 4.17 average node degree, 0.843 average local clustering coefficient, seven expected edges, and 3.83e-07 PPI enrichment p-value. Herein, a total of 25 enriched cellular components were traced in which receptor complex (GO:0043235), an integral component of the plasma membrane (GO:0005887), and cell junction (GO:0030054) were majorly enriched at a false discovery rate of 2.03E-06, 0.001, and 0.0033 and strength of 1.48, 0.91, and 0.8 respectively. Herein, the receptor complex was enriched with seven genes i.e., PDGFRB, IGF1R, APP, RET, GRM1, FGFR2, and LYN, an integral component of the plasma membrane with eight genes i.e., PDGFRB, IGF1R, APP, RET, GRM1, CRHR1, FGFR2, and LYN and cell junction with eight genes i.e., CDK4, PDGFRB, STAT3, APP, GRM1, SRC, FGFR2, and LYN. Also, a total of 21 enriched molecular functions were traced for cluster 2 in which protein tyrosine kinase activity; GO:0004713 (regulated six genes i.e. PDGFRB, IGF1R, RET, SRC, FGFR2, and LYN against 137 background genes at 1.85 strength and 6.36E-07 false discovery rate), insulin receptor binding; GO:0005158 (regulated four genes i.e. IGF1R, APP, PTPN1, and SRC against 24 background genes at 2.43 strength and 4.29E-06 false discovery rate) and ephrin receptor binding; GO:0046875 (regulated four genes i.e. APP, PTPN1, SRC, and LYN against 28 background genes at 2.37 strength and 5.02E-06 false discovery rate). A total of 45 KEGG pathways were traced within the PPI of cluster 2 in which EGFR tyrosine kinase inhibitor resistance; hsa01521 (regulated five genes i.e. PDGFRB, STAT3, IGF1R, SRC, and FGFR2 against 78 background genes at 2.02 strength and 4.26E-07 false discovery rate), long-term depression; hsa04730 (regulated four genes i.e. IGF1R, GRM1, CRHR1, and LYN against 59 background genes at 2.04 strength and 1.07E-05 false discovery rate), and pathways in cancer; hsa05200 (regulated six genes i.e. CDK4, PDGFRB, STAT3, IGF1R, RET, and FGFR2 against 517 background genes at 1.28 strength and 4.31E-05 false discovery rate) were identified to be top three majorly triggered pathways. Similarly, a total of 222 biological processes were traced within the PPI of cluster 2 in which cellular response to oxygen-containing compound; GO:1901701 (regulated 11 genes i.e. CDK4, PDGFRB, STAT3, IGF1R, APP, RET, PTPN1, SRC, CRHR1, FGFR2, and LYN against 1,055 background genes at 1.23 strength and 2.37E-09 false discovery rate), regulation of protein serine/threonine kinase activity; GO:0071900 (regulated nine genes i.e. CDK4, PDGFRB, IGF1R, APP, RET, GRM1, PTPN1, SRC, and LYN against 521 genes at 1.45 strength and 1.31E-08 false discovery rate), and regulation of protein phosphorylation; GO:0001932 (regulated 11 genes i.e. CDK4, PDGFRB, STAT3, IGF1R, APP, RET, GRM1, PTPN1, SRC, FGFR2, and LYN against 1,459 background genes at 1.09 strength and 1.93E-08 false discovery rate (Supplementary File S2; Supplementary Sheet S2).
In cluster 3 the interaction of the nodes had 1 edge count, average node degree, and average local clustering coefficient with 0.0398 PPI enrichment p-values. Here, only two KEGG pathways and eight biological processes were traced. In KEGG pathways, the p53 signaling pathway; hsa04115, and microRNAs in cancer; hsa05206 were associated with two genes i.e., MDM2 and MDM4 against 72 and 160 background genes, 2.43 and 2.09 strength and 0.0064 and 0.0155 false discovery rate respectively. Further, interaction between MDM2 and MDM4 triggered eight biological processes i.e. atrioventricular valve morphogenesis; GO:0003181 (24 background genes at 2.91 strength and 0.0274 false discovery rate), endocardial cushion morphogenesis; GO:0003203 (34 background genes at 2.76 strength and 0.0274 false discovery rate), ventricular septum development; GO:0003281 (73 background genes at 2.43 strength and 0.0274 false discovery rate), atrial septum development; GO:0003283 (23 background genes at 2.93 strength and 0.0274 false discovery rate), DNA damage response, signal transduction by p53 class mediator resulting in cell cycle arrest; GO:0006977 (59 background genes, 2.52 strength and 0.0274 false discovery rate), negative regulation of cell cycle arrest; GO:0071157 (23 background genes, 2.93 strength and 0.0274 false discovery rate), regulation of signal transduction by p53 class mediator; GO:1901796 (182 background genes, 2.03 strength, and 0.0376 false discovery rate), and cellular response to hypoxia; GO:0071456 (189 background genes, 2.02 strength and 0.0395 false discovery rate); Supplementary File S2; Supplementary Sheet S3.
Diosgenin-targets-protein network analysis
The combined interaction between the diosgenin, its targets, and regulated pathways traced IGF1R, MDM2, SRC, CDK4, and PDGFRB as the top five lead hub proteins. In addition, pathways in cancer; hsa05200, EGFR tyrosine kinase inhibitor resistance; hsa01521 prostate cancer; hsa05215, viral carcinogenesis; hsa05203, and PI3K-Akt signaling pathway; hsa04151 were traced as the top five lead hub pathways modulated within diosgenin-targets-pathways interactions.
In diosgenin-targets-pathways interactions, none of the nodes were single or undirected. Herein, two pathways’ nodes i.e., adherens junction and central carbon metabolism in cancer had a maximum average shortest path length i.e., 2.67. Herein, 33 nodes i.e. diosgenin, PI3K-Akt signaling pathway, EGFR tyrosine kinase inhibitor resistance, viral carcinogenesis, prostate cancer, endocrine resistance, proteoglycans in cancer, glioma, melanoma, human cytomegalovirus infection, endocytosis, Rap1 signaling pathway, FoxO signaling pathway, Kaposi sarcoma-associated herpesvirus infection, Epstein-Barr virus infection, MicroRNAs in cancer, long-term depression, focal adhesion, bladder cancer, signaling pathways regulating pluripotency of stem cells, Ras signaling pathway, MAPK signaling pathway, regulation of actin cytoskeleton, chemokine signaling pathway, p53 signaling pathway, prolactin signaling pathway, gap junction, ovarian steroidogenesis, Cushing syndrome, chronic myeloid leukemia, non-small cell lung cancer, pancreatic cancer, and epithelial cell signaling in Helicobacter pylori infection had the maximum closeness centralities i.e. 1. Further, the CYP3A4 node had the maximum clustering coefficient i.e., 0.5. Likewise, three pathways nodes i.e., adherens junction, central carbon metabolism in cancer, and pathways in cancer had maximum eccentricity i.e., 3. In addition, node diosgenin had the maximum stress i.e., 101 followed by 21°, 0.03 betweenness centrality, 21 directed nodes, 0.85 radiality, 22 edge count, and, 19 outdegrees. Further, four nodes i.e. NR3C1, PRCP, APP, and FASN had maximum neighborhood connectivity i.e., 22. In addition, the AR node had the maximum topological coefficient i.e., 0.79 and IGF1R had maximum indegree i.e., 19 (Supplementary File S3; Supplementary Sheet S1).
In a network a total of nine categories of edge betweenness were traced i.e., 1 (125 interactions), 5 (3 interactions), 6 (7 interactions), 7 (9 interactions), 19 (2 interactions), 14, 18, 38, and 51 had the 1 interaction (Figure 6). Herein, within the whole network, 51 edge betweenness was within the RET and diosgenin interaction, 38 with PTP1B and diosgenin interaction, 19 with adherens junction interaction with PTPN1, and central carbon metabolism in cancer interaction with RET, 18 with steroid hormone biosynthesis with diosgenin, and 14 with pathways in cancer with RET (Supplementary File S3; Supplementary Sheet S2).
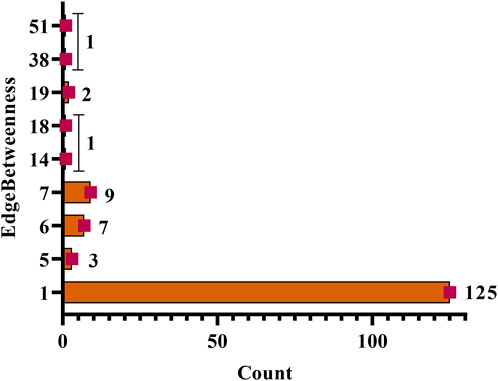
FIGURE 6. Edgebetweenness of network interaction and node interaction count. The highest edge betweenness was 51 (1 interaction) and the lowest was 1 (125 interactions).
Molecular docking
Among the three studied targets, diosgenin was predicted to possess a maximum binding affinity with IGF1R (ΔG = −8.6 kcal/mol, 11 alkyl interactions with Val983, Met1126, Val1033, Met1112, Met1049, Ala1001, and Ile1130) compared to MDM2 (ΔG = -8.5 kcal/mol, 16 alkyl interactions with Ile61, Val75, Phe86, Ile99, Leu54, Ile103, Leu57, Val93, Tyr67, and His73 and three van der Walls interactions with Phe91, Gly58, and His96) and SRC (ΔG = -7.4 kcal/mol, five alkyl interactions with Phe194, Leu200, and Ala168, four van der Waals interaction and three carbon-hydrogen bond with Arg172); Figure 7.
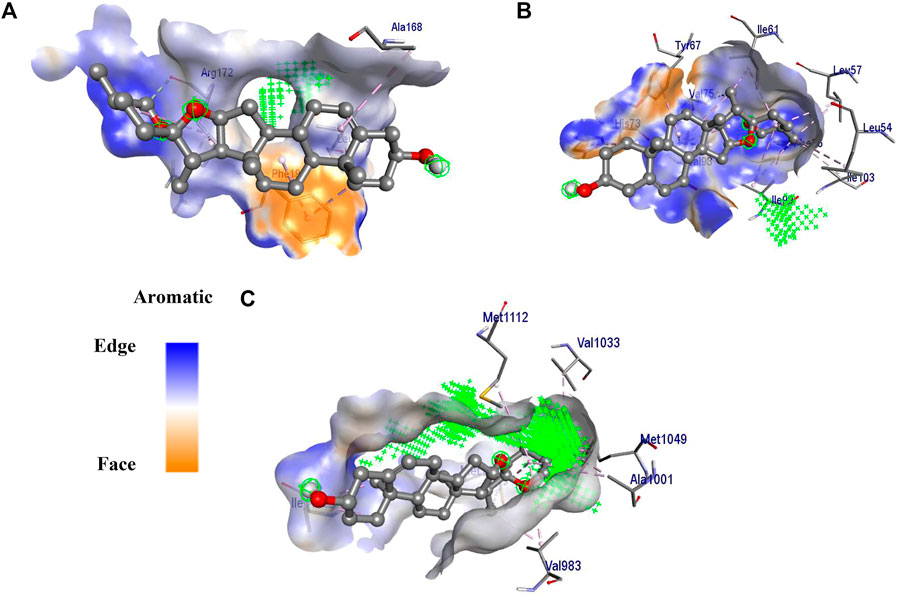
FIGURE 7. 3D interaction of diosgenin with (A) SRC, (B) MDM2 and (C) IGF1R. The surface around the ligand presents an aromatic surface. The ligand was presented in ball and stick. Amino acid residues were presented on a stick and the active site of the protein was presented with .
Molecular dynamics simulation
Stability of diosgenin–IGF1R complex
The diosgenin-IGF1R complex showed stable dynamics up to 150 ns after a 20 ns equilibration phase. Initial backbone and complex RMSD values climbed steadily increased from 1.0 Å to 3.1 Å and ∼1.4 Å to ∼3.7 Å, respectively from 0 to 20 ns After 20 ns, it was discovered that the backbone and complex RMSD (∼2.5 Å and 3.0 Å, respectively) were stabilized with lesser fluctuations (Figure 8A). The loop-forming residues Leu1064 to Pro1077 showed comparatively greater fluctuations (7.0 Å). On the other hand, residues Leu975, Val983, Ala1001, Glu1050, Asp1123, and Ile1130 that interacted with diosgenin during docking studies didn’t exhibit fluctuation because they were involved in stable non-bonded interactions. Additionally, it was discovered that residues Gly1122 to Tyr1131 forming the loop region were involved in ligand binding and showed the least RMS fluctuation (∼2.0 Å) (Figure 8B, Supplementary Movie S1). There was a formation of a compact globular shape, which was supported by a gradual drop in Rg value from 20.5 Å to 19.6 Å and was further found to be stable at 20.0 Å (Figure 8C). The initial and final surface area occupied by IGF1R and diosgenin docked complex was 163.825 nm2 and 159.1106 nm2. The complex typically occupied 159.95 nm2 (Figure 8D). The complex formed 3 H-bonds of which two were consistent during the simulation (Figure 8E). It was discovered that diosgenin and IGF1R had relative binding energy of −35.143 ± 3.03 kcal/mol. Supplementary Table S2 summarizes the free energy contribution of diosgenin with IGF1R, MDM2, and SRC. The per residue contribution energy revealed that 16 residues from the binding pocket i.e. Glu974, Leu975, Val983, Tyr984, Glu985, Ala1001, Glu1020, Val1033, Leu1051, Met1052, Asp1056, Met1112, Asp1123, Met1126, Ile1130, and Tyr1131 significantly contributed to the formation of a stable complex. These residues also scored the least per residue decomposition/contribution energy which ranged from −2.0 kJ/mol to −6.8 kJ/mol whereas the positive contribution energy of 4.0 kJ/mol was achieved by residues Lys1003, Lys1058, and Arg1109 (Figure 8F).
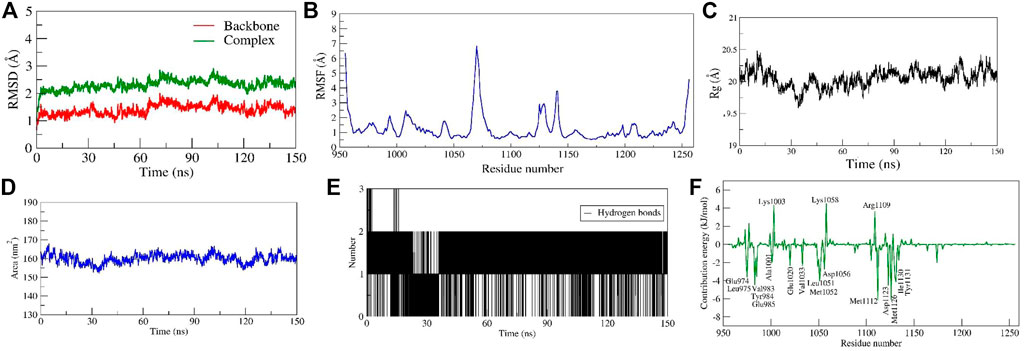
FIGURE 8. Parameters representing the structural stability of the Diosgenin-IGF1R complex. (A) Backbone and complex RMSD, (B) RMSF, (C) Rg, (D) SASA, (E) number of H-bond interactions, and (F) contribution energy plot demonstrates the significance of the ligand binding domain residues in stable complex formation.
Stability of diosgenin–MDM2 complex
Throughout a 150 ns production run, the Diosgenin-MDM2 complex exhibited stable dynamics. The initial and final backbone RMSD values were 0.62 and 1.21, respectively, and an average was 1.40 Å. Similar to this, the initial and final RMSD of the docked complex MDM2 and diosgenin were 0.92 and 2.13 respectively with an average of ∼2.30 Å (Figure 9A). The loop forming Met17 to Ser22 residues at the N-terminus had substantially greater fluctuations (∼4.2 Å). Further, residues interacting with diosgenin during docking studies (Leu57, Tyr67, Phe91, Val93, and Ile99) did not exhibit variations as they were involved in stable non-bonded interactions (Figure 9B and Supplementary Movie S2). By monitoring a stable Rg, a more compact and stable complex was formed. The complex’s initial and final Rg values were determined to be 13.2 Å and 1.31 Å. Similarly, the initial and final surface area occupied by IGF1R and diosgenin docked complex was 63.90 nm2 and 61.57 nm2. The complex had an average surface area of 62.89 nm2 (Figure 9D). Two H-bonds were established by the complex of which one was consistent during MD simulation (Figure 9E). The relative binding energy between diosgenin and IGF1R was discovered to be −34.619 ± 2.81 kcal/mol. Further, seven residues (Leu57, Gly58, Ile61, Met62, Tyr67, Val93, and Ile99) from the binding pocket scored the lowest per residue decomposition/contribution energy, ranging from −3.65 kJ/mol to −8.87 kJ/mol. These residues contributed significantly to forming the stable complex according to the per residue decomposition/contribution energy. The residue Glu69 had positive contribution energy of 2.68 kJ/mol (Figure 9F).
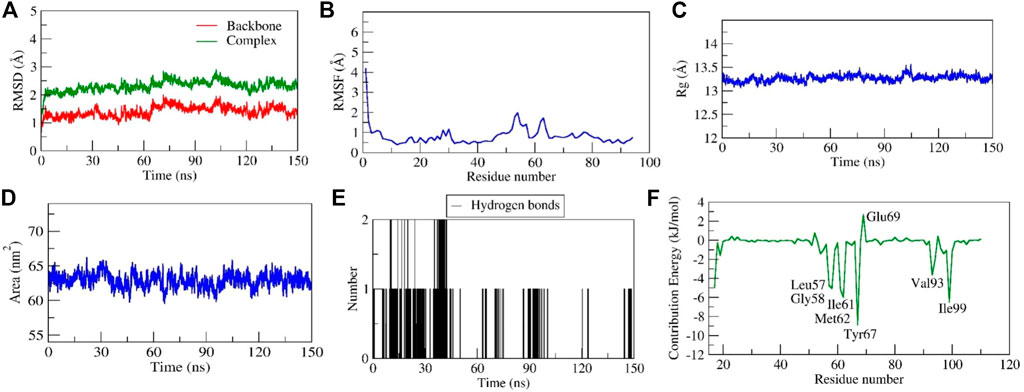
FIGURE 9. Parameters representing the structural stability of the Diosgenin-MDM2 complex. (A) Backbone and complex RMSD, (B) RMSF, (C) Rg, (D) SASA, (E) number of H-bond interactions, and (F) contribution energy plot demonstrates the significance of the ligand binding domain residues in stable complex formation.
Stability of diosgenin–SRC complex
Another diosgenin–SRC complex also showed stable dynamics with the RMSD value of < 2Å after the equilibration period of 50 ns(Figure 10A). In addition, the RMSD of the complex showed a sharp increase in its values after 40 ns to 8 Å (Figure 10A). The careful observation of the entire complex trajectory and representative snapshots extracted from the region reveal the structural transition of diosgenin from its primary binding pocket to the neighboring alternate pocket which formed a stable complex during the entire simulation period. Thus, for the first time, we report the existence of an alternate binding site other than the primary binding site on SRC. From the simulation movie (Supplementary Movie S3) it was observed that the complex undergoes major conformational changes in the secondary structure of the protein including the primary binding pocket, leading to the increased conformational flexibility of SRC, hence the diosgenin switches its original position from the primary binding pocket to the reported alternate binding pocket (formed by the residues Tyr152 to Leu164). The residual fluctuations observed show fewer C-alpha fluctuations (<2.2Å), interestingly the residues from the primary binding pocket as well as new reported alternate binding pocket show the least RMSF values ∼0.5Å (Figure 10B), mainly due to the stable non-bonded interactions shown by them. The complex diosgenin–SRC gains a compact globular shape during the simulation, thus we propose the stable complex formation was favored after the equilibration period of MD simulation (Figure 10C). The solvent-accessible surface area represents an exposure of the hydrophobic residues to the solvent, here in this complex SASA exhibited a similar trend as that of Rg values. SASA value decreased gradually till the equilibration state and further, it stabilized till the simulation end (Figure 10D), suggesting the proper folding of the hydrophobic core including both the binding sites. Two H-bonds were established by the complex of which one was consistent during MD simulation (Figure 10E). The relative binding energy between diosgenin to SRC was discovered to be −17.994 ± 5.67 kcal/mol. In addition, three residues i.e., Ile156, Thr157, and Leu164 from the binding pocket scored the lowest energy contribution per residue. These residues contributed significantly to forming the stable complex according to the per residue decomposition/contribution energy. The residue Ile156 had the least contribution energy of −8.9 kJ/mol (Figure 10F).
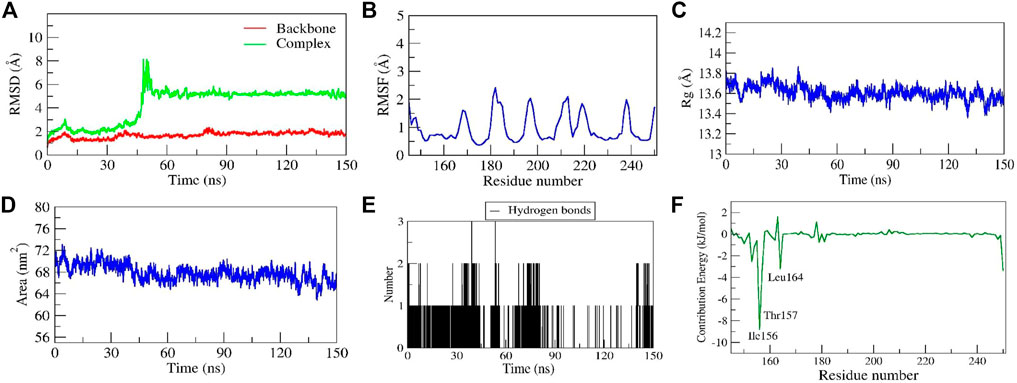
FIGURE 10. Parameters representing the structural stability of the Diosgenin-SRC complex. (A) Backbone and complex RMSD, (B) RMSF, (C) Rg, (D) SASA, (E) number of H-bond interactions, and (F) contribution energy plot demonstrates the significance of the ligand binding domain residues in stable complex formation.
Principal component and dynamic cross-correlation matrix
PCA is a statistical technique being used to study the dynamics of bimolecular complexes as it limits the 3 N (N = number of atoms in the protein) degrees of freedom describing functionally crucial motions of the protein. It was observed that in all the complexes maximum dynamics during the simulation have been captured by the first 10 eigenvectors, of which the first two contributed significantly to the collective motions exerted by all the simulated complexes (Figures 14A–C). Hence, we examined the collective motion sampled by the first two principal components (PCs), and 2D projections for PC1 and PC2 were plotted (Figures 11D–F). The complexes of diosgenin with MDM2 and SRC express the compact clusters in the conformational spaces those range from -1.5 to 1.5. In the MD trajectory of complexes Diosgenin-SRC and Diosgenin-MDM2, PC1 and PC2 (top two modes) showed the uniform distribution across the configurational space while the remaining complex of Diosgenin-IGF1R showed a large diversity in the conformational space and was widely clustered in the range of -4.5 to 4.5 (Figure 11D). MD trajectory sampled three states of the protein as seen by the three individual clusters in the scatterplot of PC1 v/s PC2. Herein, we propose that the Diosgenin-IGF1R complex has undergone significant conformational changes in the secondary structure during the simulation those favored in forming a stable complex. However, other complexes namely Diosgenin-SRC and Diosgenin-MDM2 were well stabilized and undergone comparatively lesser conformational changes in the secondary structure hence exhibited compact cluster in the conformations space. Further, the convergence of sampling was also analyzed by calculating the cosine content of all the trajectories obtained. The cosine content was calculated for the trajectories of the first top two principal components (PC1 and PC2) for complexes Diosgenin-SRC and Diosgenin-MDM2, and Diosgenin-IGF1R is observed as 0.024225, 0.0175249 and 0.158163 respectively. It has been reported that the value of cosine content for the first few PCs close to 1 indicated bad sampling of the trajectory pointing to all our simulation trajectories being well converged and properly sampled in the free energy landscape.
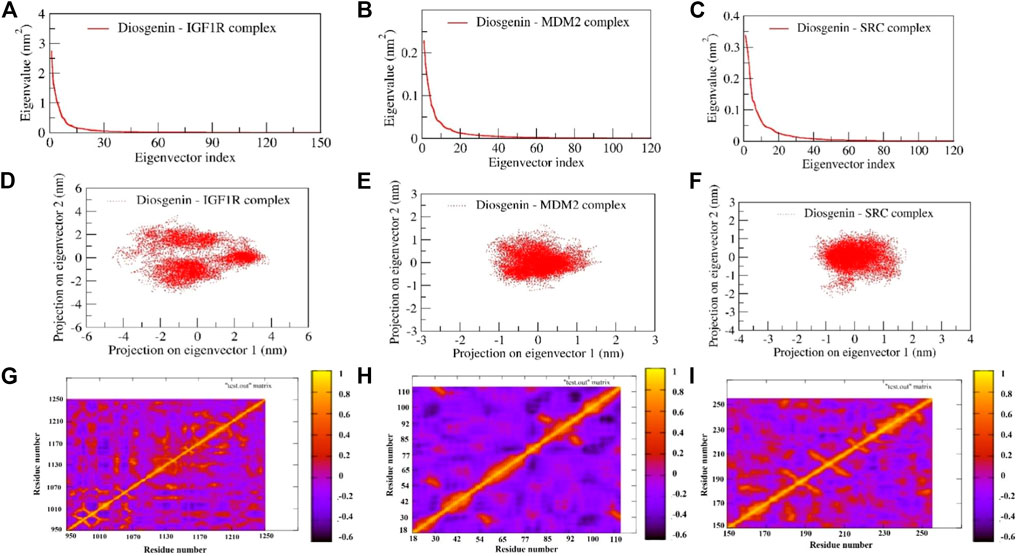
FIGURE 11. Represents the principal component analysis and dynamic cross-correlation matrix of complexes: The first 120 eigenvectors were plotted v/s eigenvalues for diosgenin with (A) IGF1R (B) MDM2, and (C) SRC. The collective motions of diosgenin with (D) IGF1R (E) MDM2, and (F) SRC using projections of MD trajectories on two eigenvectors corresponding to the first two principal components. Dynamic cross-correlation matrix of Cα atoms observed in diosgenin with (G) IGF1R (H) MDM2, and (I) SRC. The amber-colored positive regions reflect strongly correlated Cα atom movements (Cij = 1), whereas the blue-colored negative regions show anticorrelated motions (Cij = -1).
The concerted motion exerted by the three complexes during the simulation was examined using DCCM (dynamic cross-correlation matrix). The calculation of the correlation matrix is utilized to depict the dynamical information of proteins in two dimensions. To observe the correlation in the dynamics of the binding site correlation matrix over the stable trajectory of all the complexes were plotted (Figures 11F,G). The diagonal orange-red line indicates the self-correlation of the individual residues with themselves. The orange-red region in the correlation map signifies the concerted movement of the residues in the same direction whereas the dark blue region represents anti-correlated fluctuations. The N- and C-terminal region of the complex Diosgenin-MDM2 represents strong anticooperative movement with each other. Comparatively, this complex exhibited a maximum amplitude of negative correlation across all the residues. The maximum region in the complex Diosgenin-IGF1R showed positive correlation mainly at the binding pocket region (residues Glu974, Leu975, Val983, Tyr984, Glu985, Ala1001, Glu1020, Val1033, Leu1051, Met1052, Asp1056, Met1112, Asp1123, Met1126, Ile1130, and Tyr1131). This signifies the closure movement observed at the binding pocket region facilitating stable complex formation (Supplementary Movie S1). Similarly, in the Diosgenin-SRC complex, the binding pocket residues (Arg158, Glu181, Thr182, Cys188, Lys203-Lys206) showed moderately positive cooperative motion compared to other regions in the SRC structures. Interestingly, the residues from the alternate binding region show the cooperative motion results in stable non-bonded contact with the newly reported alternate binding pocket.
In general, the conformational flexibility of all the complexes i.e., Diosgenin-IGF1R, Diosgenin-MDM2, and Diosgenin-SRC varies greatly as observed in the DCCM plot and the collective dynamics nature observed in the PCA and DCCM plot favors the stable complex formation during the simulation.
Survival analysis of hub genes
To evaluate the prognostic significance of genes, we analyzed the survival curves of each gene. Among 21 genes, 17 genes were observed to had the significant effect over the Kaplan–Meier survival analysis i.e., APP (log-rank p = 0.0018), AR (log-rank p = 0.0033), CDK4 (log-rank p = 0.0062), CYP19A1 (log-rank p = 0.036), FGFR2 (log-rank p = 2.9 × 10–5), GRM1 (log-rank p = 0.027), IGF1R (log-rank p = 3.2 × 10–05), LYN (log-rank p = 0.017), MDM2 (log-rank p = 0.0012), MDM4 (log-rank p = 0.044), NR3C1 (log-rank p = 0.00038), PDGFRB (log-rank p = 1.2 × 10–06), PRCP (log-rank p = 0.015), PTPN1 (log-rank p = 0.00041), RET (log-rank p = 0.03), SRC (log-rank p = 0.0039), and STAT3 (log-rank p = 1.4 × 10–06). Similarly, four genes i.e., CRHR1 (log-rank p = 0.09), CYP17A1 (log-rank p = 0.14), CYP3A4 (log-rank p = 0.056), and FASN (log-rank p = 0.15) were insignificantly linked with disease prognosis; Table S3.
Gene expression analysis in tumor, normal and metastatic tissues
Kruskal Wallis test revealed the significant expression (p = 5.96e-04) of the PDGFRB gene in breast invasive carcinoma compared to normal and metastatic samples. In addition, the similar observations were also noted for the FGFR2 (p = 3.03e-08) and STAT3 (p = 9.51e-02) genes. In addition, Dunn test pointed the difference in PDGFRB expression in the normal vs. tumor (p = 7.74e-05), tumor vs. metastasis (p = 5.66e-02), normal vs. metastasis (p = 2.61e-01), FGFR2 in normal vs. tumor (p = 2.31e-04), tumor vs. metastasis (p = 2.98e-02), and normal vs. metastasis (p = 3.41e-01) and STAT3 normal vs. tumor (p = 1.59e-02), tumor vs. metastasis (p = 4.26e-01), and normal vs. metastasis (p = 3.57e-01); Figure 12).
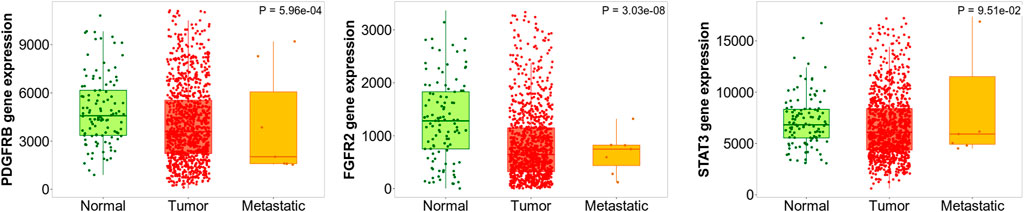
FIGURE 12. Differential gene expression analysis of PDGFRB, FBFR2, and STAT3 in tumor, normal and metastatic tissues. These genes were identified to possess the least log-rank test.
Among IGF1R, SRC, and MDM2, all the genes had a significant difference in gene expression compared to three different tissues i.e., IGF1R (p = 2.07e-01), SRC (p = 2.71e-18), and MDM2 (p = 1.91e-02) which were evaluated using Kruskal Wallis test. In addition, there was a significant difference in MDM2 expression in normal vs. tumor (p = 3.83e-03), tumor vs. metastasis (6.87e-02), and normal vs. metastasis (2.03e-01) as revealed by the Dunn test. Likewise, there was a significant difference in SRC expression in normal vs. tumor (p = 1.72e-19), tumor vs. metastasis (1.92e-03), and normal vs. metastasis (2.62e-01). Likewise, there was a significant difference in IGF1R expression in normal vs. tumor (p = 4.25e-02), tumor vs. metastasis (p = 4.91e-01), and normal vs. metastasis (p = 3.19e-01); Figure 13.
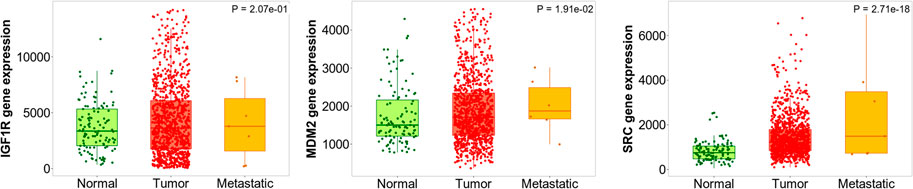
FIGURE 13. Differential gene expression analysis of IGF1R, MDM2, and RSC in tumor, normal and metastatic tissues. Diosgenin was predicted to possess the highest binding affinity with these targets.
In vitro pharmacology
BSL bioassay
Exposure to the different concentrations of diosgenin and doxorubicin showed concentration-dependent brine shrimp lethality. In addition, the LC50 was found to be 19.15 and 0.71 μg/ml respectively reflecting the doxorubicin to be 27.06 times more potent than diosgenin (Supplementary Figure S4).
In vitro MTT assay
Here, the MTT assay showed the IC50 of the diosgenin to be significantly higher than that of the doxorubicin in MCF7 (p < 0.001), MDA-MB-231 (p < 0.05), SKBR3 (p < 0.05) and Vero (p < 0.001) compared to the doxorubicin. In vitro MTT assay on MCF7 cell lines reflected the doxorubicin (IC50 3.21 ± 0.29 μg/ml) to be 3.7 times more potent than the diosgenin (IC50 12.05 ± 1.33 μg/ml). In addition, over the MDA-MB-231 cell lines, the IC50 of diosgenin was found to be (45.54 ± 23.41) µg/ml compared to doxorubicin (6.30 ± 2.67) µg/ml which suggests the doxorubicin to be 7.2 times more potent than diosgenin. In addition, the diosgenin and doxorubicin had the IC50 (15.11 ± 5.32) µg/ml and (5.47 ± 1.09) µg/ml respectively over SKBR3 reflecting the doxorubicin to be 2.76 times more potent than that of diosgenin. In addition, the IC50 of diosgenin and doxorubicin was found to be (17.78 ± 7.86) and (8.18 ± 8.18) µg/ml respectively over the T47D cell lines to point doxorubicin to be 2.17 times more potent. Further, diosgenin and doxorubicin had the IC50 of (38.59 ± 4.03) and (7.05 ± 0.69) µg/ml respectively in which the doxorubicin was found to be 7 times more cytotoxic than Vero cell lines; Supplementary Figure S5; Supplementary Table S4.
In vitro scratch assay
After the 72-h exposure to different cells, it was observed that diosgenin (200 μg/ml) had the highest effect on SKBR3 cell lines (18.22 ± 1.237%) to prevent cell migration compared to the rest. In addition, we observed a significant difference in percentage scratch closure within MCF vs. SKBR3 (p < 0.01), MDA-MB-231 vs. SKBR3 (p < 0.05), SKBR3 vs. T47D (p < 0.01) and SKBR3 vs. Vero (p < 0.001) cell lines (Supplementary Figure S6). In addition, the concentration and time-dependent effect of the diosgenin on scratch closure are presented in Supplementary Figure S7.
Effect of diosgenin and doxorubicin over H2O2-induced stress in cell lines
Upon the 24 h exposure of the H2O2, it was observed that diosgenin has an equivalent effect to ascorbic acid over MCF cell lines with an IC50 (7.68 ± 0.51) µg/ml and (7.13 ± 0.31) µg/ml respectively. In addition, diosgenin and ascorbic acid showed the IC50 of (13.58 ± 0.90) µg/ml and (10.60 ± 1.60) µg/ml respectively over the MDA-MB-231 cell lines. However, diosgenin (IC50 6.68 ± 0.67 μg/ml) had more effect over the SKBR3 compared to ascorbic acid (9.39 ± 3.09 μg/ml). Similarly, diosgenin and doxorubicin had the IC50 (8.90 ± 0.98) and (9.14 ± 0.78) µg/ml respectively over T47D cell lines. Further, the diosgenin and ascorbic acid had the IC50 (13.72 ± 1.83) µg/ml and (12.68 ± 4.53) µg/ml respectively over the Vero cell lines (Supplementary Table S5). The concentration-dependent effect of diosgenin and ascorbic acid is presented in Supplementary Figure S8.
Effect of diosgenin on Warburg effect
It was observed that diosgenin had efficacy in dealing glucose uptake in tumor cells. The EC50 of the diosgenin was observed to be significantly higher in glucose uptake in MCF7 (p < 0.001), SKBR3 (p < 0.05), T47D (p < 0.001), and Vero (p < 0.01) cell lines compared to metformin in the presence of insulin. It was observed that diosgenin had a comparatively higher EC50 (26.19 ± 2.77) µg/ml compared to metformin (3.10 ± 0.99) µg/ml which showed the diosgenin to possess a comparatively lower glucose uptake efficacy than metformin in MCF7 cell lines. In addition, in MDA-MB-231 cell lines, diosgenin and metformin had an equivalent EC50 in promoting glucose uptake i.e., EC50 (17.92 ± 1.19) and (17.03 ± 2.59) µg/ml respectively. Furthermore, it was observed that diosgenin had comparatively higher the EC50 in promoting the glucose uptake in SKBR3 (EC50 19.99 ± 2.91 μg/ml), T47D (EC50 37.47 ± 1.75 μg/ml), and Vero (EC50 15.27 ± 0.95 μg/ml) compared to metformin (EC50 SKBR3; 11.74 ± 3.19 μg/ml, T47D; 12.50 ± 1.42 μg/ml, and Vero; 11.79 ± 1.65 μg/ml); Supplementary Table S6; Supplementary Figure S9
Discussion
The present study focused on tracing the targets of diosgenin and evaluates the probably triggered pathways via the PPI in breast cancer. In this regard initially, we predicted the targets using three open-source platforms i.e. SwissTargetPrediction, DIGEP-Pred, and BindingDB; matched with estrogen receptor-positive breast cancer targets (UMLS CUI: C2938924); retrieved from DisGeNET in which diosgenin was predicted to target 21 different proteins i.e. APP, CDK4, CRHR1, CYP17A1, CYP19A1, CYP3A4, FASN, FGFR2, GRM1, IGF1R, LYN, MDM2, MDM4, PDGFRB, PRCP, PTPN1, RET, and SRC, (predicted in SwissTargetPrediction) AR and NR3C1 (predicted in DIGEP-Pred), and STAT3 (predicted in SwissTargetPrediction and BindingDB) in the breast cancer. Herein concerning the KEGG database, it was observed that 36 different pathways were triggered in which 12 pathways i.e. pathways in cancer; hsa05200, prostate cancer; hsa05215, viral carcinogenesis; hsa05203, glioma; hsa05214, melanoma; hsa05218, bladder cancer; hsa05219, MicroRNAs in cancer; hsa05206, Kaposi sarcoma-associated herpesvirus infection; hsa05167, proteoglycans in cancer; hsa05205, central carbon metabolism in cancer; hsa05230, non-small cell lung cancer; hsa05223, and pancreatic cancer; hsa05212 were identified which explores the anti-cancer pharmacological spectra of the diosgenin.
Breast cancer is one of the main causes of death among women. Chiefly, the cells lining the milk-forming duct of the mammary glands are the origination of breast cancer (Herbein and Kumar, 2014) which can be further subdivided based on the presence or absence of the hormone receptors i.e., estrogen and progesterone subtypes and human epidermal growth factor receptor-2 (HER2). In addition, the estrogen receptor pathway triggers hormone receptor-positive breast cancer (Rani et al., 2019). Similarly, in HER2-positive breast cancer, HER2 triggers RAS/RAF/MAPK and PI3K/AKT signaling pathways that stimulate cell growth, survival, and differentiation (Dittrich et al., 2014). We discovered 21 diosgenin-regulated proteins that are involved with oestrogen receptor-positive breast cancer (UMLS CUI: C2938924) targets in this investigation. In addition, three pathways i.e., PI3K-Akt (modulated five genes i.e., CDK4, MDM2, PDGFRB, IGF1R, and FGFR2), Ras (modulated three genes i.e., PDGFRB, IGF1R, and FGFR2), and MAPK (modulated three genes i.e., PDGFRB, IGF1R, and FGFR2) signaling pathways associated to HER2 positive breast cancer were modulated. Since diosgenin modulated three pathways closely associated with HER2-linked pathogenesis, it can be speculated that diosgenin could act via the manipulation of HER2.
It has previously been proposed that Akt activation influences endocrine resistance in metastatic breast cancer. In addition, Akt activation in the downstream pathway of HER2 could resist the endocrine therapy of breast cancer (Tokunaga et al., 2006). Furthermore, Ras proteins activate the cytoplasm and extracellular signaling networks via receptor tyrosine kinase and are involved in cell proliferation, survival, growth, metabolism, motility, and apoptosis, and their hyperactivation promotes the growth and progression of breast cancer. In addition, Ras’s intracellular localization, activation, and signaling have been used in developing therapeutic candidates against breast cancer via the enzymes involved in posttranslational modification of Ras e.g., farnesyltransferase and geranylgeranyltransferase 1 (Moon, 2021). Further, MAPK links the extracellular mitogenic signals to cell proliferation which may be concerned with or act independently towards estrogen-mediated events in breast cancer cells (Yue et al., 2002). In the present study, we identified the modulation of the above-modulated pathways i.e., PI3K-Akt signaling pathway (false discovery rate: 0.0011, strength: 1.12), Ras signaling pathway (false discovery rate: 0.0263, strength: 1.09), and MAPK signaling pathway (false discovery rate: 0.0446, strength: 0.99). This suggests the probability of involvement of these pathways linked to HER2 function which could be modulated by diosgenin in breast cancer.
The role of EGFR dysregulation or mutation in cancer etiology, particularly breast cancer, has been proposed previously. However, resistance towards EGFR tyrosine kinase inhibitors may occur due to secondary mutations (T790M), activation of secondary pathways (AXL, c-Met, HGF), aberrant downstream pathways (K-RAS mutations, loss of PTEN), deregulation of the EGFR tyrosine kinase-mediated apoptosis, histological transformation, and ATP binding cassette transporter effusion, etc (Huang and Fu 2015).
The FoxO signaling system interacts with the PI3K-Akt signaling pathway and relates to cancer progression, particularly breast cancer advancement (Farhan et al., 2017). In addition, FoxO negatively regulates activated EGFR signaling which was demonstrated via the in vitro cell line culture method and in vivo models (Sangodkar et al., 2012). Here, in the present study, we identified the regulation of the FoxO signaling pathway via the modulation of four genes i.e. MDM2, STAT3, IGF1R, and GRM1 which could support the functioning of the PI3K-Akt and EGFR signal against breast cancer pathogenesis.
Furthermore, the Rap1 signal has been linked to tumor cell proliferation, invasion, and metastasis through regulating integrin- or cadherin-mediated cell function, cytoskeletal alterations, protease (metal metalloprotease) production, and cell adhesion (Zhang et al., 2017). In addition, Rap1 has been traced to attenuate metastasis and EGFR-triggered carcinoma (Huang et al., 2012). Since breast cancer progression is closely linked to EGFR tyrosine kinase signal; it can be speculated that diosgenin-mediated Rap1 signal could attenuate tumor invasion and metastasis; was observed to be modulated via the modulation of three genes i.e., PDGFRB, IGF1R, and FGFR2.
The p53 acts as a transcription factor for p21 via cyclin-CDK interactions which is important for the transition of the G2 phase to the mitosis phase (Alam et al., 2015). In addition, p21 protects cells from apoptosis, regulates the cell cycle, causes apoptosis, and decreases cell proliferation in tumor cells (Wang et al., 2021). Mutant p53 has been pointed to as the guardian of the cancer cells (Mantovani et al., 2019) and is also associated with worsening breast cancer affecting overall survival (Gasco et al., 2002). In the present study, we identified the regulation of the three genes i.e., CDK4, MDM2, and MDM4 which could activate the p53 signal against breast cancer. Furthermore, excessive plasma prolactin levels have been associated with an increased risk of breast cancer in both premenopausal and postmenopausal women; is more prominent in estrogen or progestogen receptor cancer type (Tworoger et al., 2004; Tworoger et al., 2006). Here, in the present study, we identified the diosgenin to regulate the prolactin signaling pathway via the regulation of three genes i.e., STAT3, CYP17A1, and SRC which could avoid estrogen or progestogen receptor-mediated breast cancer progression. In addition, chemokine signals are not limited to tissue differentiation, hematopoiesis, inflammation, and immune regulation but also process tumor development by triggering angiogenesis, metastasis, drug resistance, and immunity of breast cancer (Liu et al., 2020). In the present study, we identified the diosgenin to trigger the chemokine signaling pathway, and regulated three genes i.e., STAT3, SRC, and LYN at 1.18 strength and 0.0168 false discovery rate). In addition, in enrichment analysis i.e., tissues, Pfam, InterPro, and compartments enrichment analysis we observed the multiple proteins that are concerned with the breast cancer prognosis.
Also, in the present study, we identified three proteins i.e., IGF1R, MDM2, and SRC in diosgenin-protein(s)-pathway(s) interaction. Hence, these were further considered for post-network analysis i.e., molecular docking and molecular dynamics simulation. Apart from handling the transcription, IGF1R can trigger the growth and metastasis of malignant melanoma cells through the PI3K-Akt signaling pathway (Ekyalongo and Yee, 2017). Diosgenin was anticipated to interact with IGF1R in the current investigation, potentially preventing breast cancer metastasis and tumor invasion, which could be PI3K-Akt driven, as previously discussed.
The IGF1R is a transcription factor that binds to DNA and influence transcription. Both ERK1/2 and AKT are involved in the transcriptional control of the IGF1R gene. MicroRNA-139-5p modulates the growth and metastasis of malignant melanoma cells via the PI3K/AKT signaling pathway by binding to IGF1R (binding energy -8.6 kcal/mol). Previously, MDM2 amplification has been reported to relate to estrogen receptor status and its presence has been indicated in human breast cancer cell (Quesnel et al., 1994); was observed to be manipulated with diosgenin in the third cluster of the PPI binding (binding energy −8.5 kcal/mol). Likewise, another modulated protein i.e., SRC has been reported to increase its expression in human breast cancer by 4–30 fold which was evidenced via both immunohistochemistry and immunoblotting (Verbeek et al., 1996); was also predicted to be modulated by diosgenin interaction (binding energy -7.4 kcal/mol). In addition, since 17 genes i.e. APP, AR, CDK4, CYP19A1, FGFR2, GRM1, IGF1R, LYN, MDM2, MDM4, NR3C1, PDGFRB, PRCP, PTPN1, RET, SRC, and STAT3 were observed to have a significant role in disease prognosis, it can be speculated that the above-modulated proteins are primarily concerned with breast cancer management with diosgenin treatment.
The docking study revealed diosgenin to interact with active site residues of three potential targets involved in breast cancer via IGF1R, MDM2, and SRC. Diosgenin formed stable intermolecular interactions throughout 150 ns MD simulation revealing them as the best lead. Among the interactions of diosgenin with IGF1R, diosgenin interactions with Leu975, Val983, Met1112, Met1126, and Ile1130 were consistent in both docking and MD simulation. Multiple studies have demonstrated these residues involve the pocket as a primary binding site (also validated by PrankWeb server; https://prankweb.cz/) for inhibition of IGF1R (Munshi et al., 2002; Li et al., 2009; Guo et al., 2015). This indicates, that diosgenin as a potent lead hit against IGF1R. Similarly, diosgenin scored the lowest binding energy of -8.5 kcal/mol and binding free energy of -34.619 kcal/mol and formed stable interactions Leu57, Gly58, Ile61, Met62, Tyr67, Val93, and Ile99 throughout the 150 ns MD production run. Both docking and MD simulation revealed diosgenin as a potent lead hit for targeting MDM2. Interestingly, a study by Li et al. (2021) identified AG-690/37072075 and AO-022/43452814 as potent anticancer lead hits against MDM2. These molecules were predicted to interact with the residues “Leu57, Gly58, Ile61, Met62, Tyr67, Val93, and Ile99” and were also found to inhibit p53-MDM2 interaction in wild-type p53 cells. Hence, we believe that diosgenin may interfere with the p53-MDM2 interaction (MDM2 inhibits the transcriptional activity of p53 by attaching to its transactivation domain), and further experimental studies are required to validate our findings. Further, diosgenin scored -7.4 kcal/mol binding energy against SRC and formed interaction with Ala168, Arg172, Phe194, and Leu200. The structural shift of diosgenin from its initial binding pocket to the neighboring alternative pocket was revealed through MD simulation, and the complex remained stable throughout the entire simulation. As a result, we are the first to disclose the discovery of a secondary binding site in the SRC. The simulation movie (Supplementary Movie S3) shows that the complex undergoes significant conformational changes in the protein’s secondary structure, including the primary binding pocket, increasing the conformational flexibility of SRC and causing diosgenin to switch positions from the primary binding pocket to the alternate binding pocket that has been reported. The PCA and DCCM revealed that diosgenin with IGF1R, MDM2, and SRC exhibited significant differences in conformational flexibility and also support the stable complex formation during the simulation.
During the differential gene expression analysis of diosgenin-regulated genes, we observed that PDGFRB, FBFR2, and STAT3 possess maximum gene expression in tumor and metastatic vs. normal tissues. In addition, molecular docking and simulation also identified IGF1R, MDM2, and SRC could be the prime diosgenin-modulated targets against breast cancer. Hence, to confirm this we further assessed the functional role of each target using cell line studies. The PDGF family (PDFGRB) has been reported to use PDGF ligands released by cancer stromal cells from breast cancer cells to drive cell proliferation (Farooqi and Siddik, 2015). Similarly, FBFR2 has been reported to promoting cell self-renewal by interacting with NF-kB signals (Kim et al., 2013). Likewise in previous studies, STAT3 inhibition has been reported to decrease cell proliferation and apoptosis promotion in various cancers including breast cancer (Kanai et al., 2003; Pancotti et al., 2012; Chen et al., 2013). In this regard, because these targets were directly triggered by diosgenin and were also significantly higher in the tumor than in the normal, diosgenin may limit cell proliferation and promote apoptosis by interfering with the self-renewal process. This hypothesis was further supported by the MTT and scratch assays. Previously, an increase in glucose uptake has been reported in the cancer cell which supports ATP production and acts as a fuel (Adekola et al., 2012). This glucose uptake can be reduced by blocking insulin’s impact on cancer cells. In comparison to metformin, the current investigation found a considerably greater effective concentration to limit glucose uptake. As a result, diosgenin may inhibit glucose uptake in cancer cells. In the current investigation, however, the glucose absorption assay was done in the presence of insulin. As a consequence, more research is needed to determine its role in glucose uptake in the absence of insulin. Similarly, MDM2 helps cancer cells to escape p53 surveillance and avoid cellular apoptosis (Momand et al., 1998). In the present study, since, diosgenin had a significant binding affinity with MDM2, it could probably promote the apoptosis that needs to be further confirmed. In addition, SRC has been indicated for cancer metastasis and also helps in cancer progression and development which is an indicator of cell proliferation (Wheeler et al., 2009). In the current investigation, we discovered that diosgenin inhibited cell proliferation in a variety of cell lines. This may be due to the blockage of the SRC generated by diosgenin by binding to it. This could have prevented cell multiplication, as revealed by the scratch assay. Based on the information presented above, it is reasonable to believe that diosgenin may act against breast cancer by reducing glucose utilization, invasion, and metastasis via the surface protein IGF1R. Furthermore, it may limit cancer cell proliferation by acting on another surface protein, PDGFRB. In addition, the diosgenin may also act over the MDM2 and prevent the cancer cell to escape the p53 surveillance in the cytoplasm. Also, diosgenin may primarily act over two cytoplasmic targets FGFR2 and SRC to prevent the interaction with NF-kB (prevents cell self-renewal) and metastatic spread respectively (Figure 14) and may also modulate other pathways within the breast cancer pathogenesis (Figure 15) which was evidenced during KEGG pathway analysis.
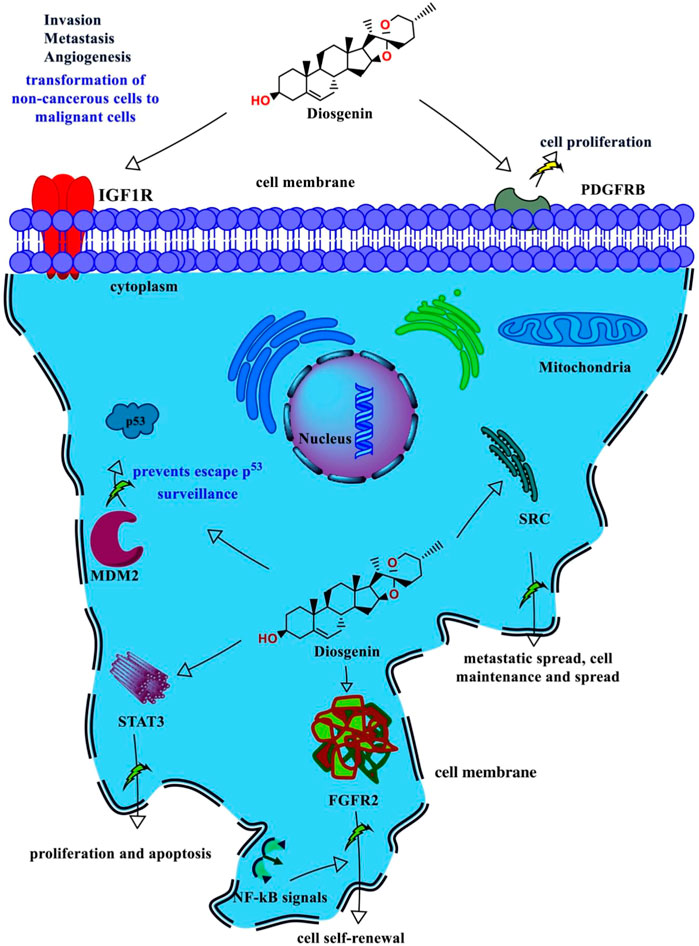
FIGURE 14. Mechanism of action of diosgenin against oestrogen receptor-positive breast cancer. Diosgenin acts on the two cell surface proteins IGF1R and PDGFRB and inhibits invasion, metastasis, angiogenesis, and cell proliferation. In addition, it acts on the four cytoplasmic proteins i.e., MDM2, STAT3, FGFR2, and SRC. Diosgenin may inhibit the cell to escape p53 surveillance by binding with MDM2, inhibit cell proliferation and promote apoptosis by binding to STAT3, interfere with NF-kB signals check the cancer cell self-renewal, and inhibit the metastatic spread by inhibiting the SRC action.
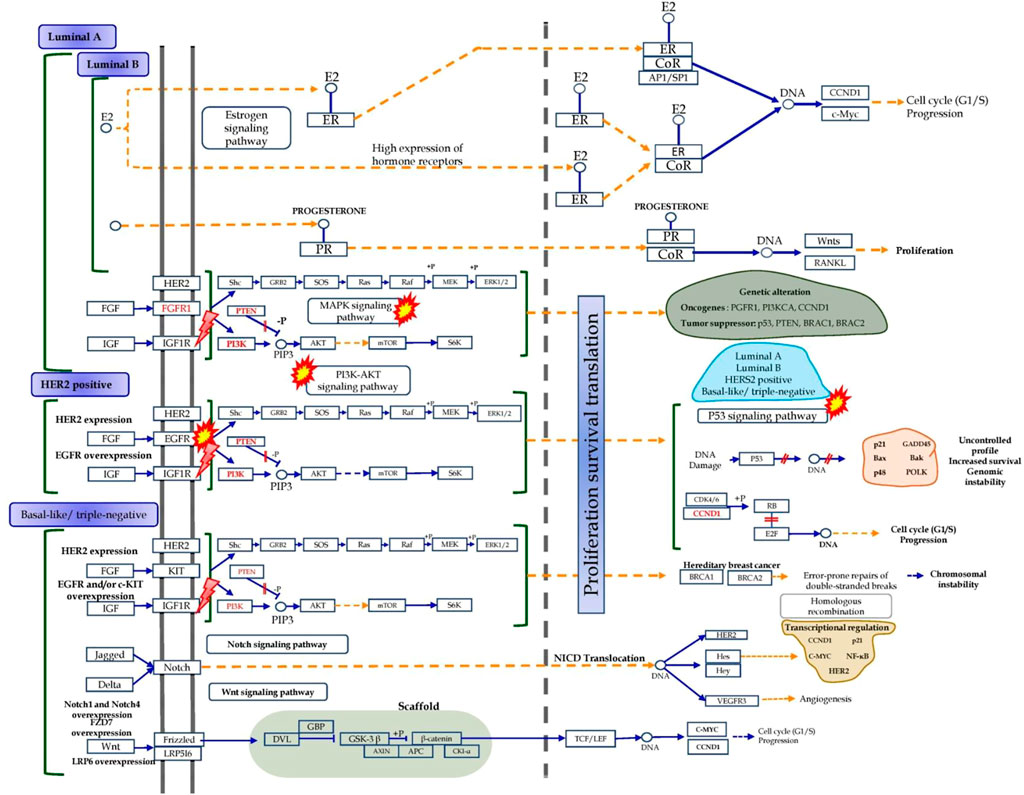
FIGURE 15. Probable checkpoints affected by the diosgenin in the pathogenesis of breast cancer (Homo sapiens (human); hsa05224) targets/pathways. In the KEGG pathway analysis, it was diosgenin-regulated targets involved in breast cancer were also identified to trigger EGFR, PI3K-Akt, MAPK, and p53 signaling pathways.
Although diosgenin inhibited cell growth, was cytotoxic, and had an effect on the various cellular compartments of the tumor cell, the inhibitory constant efficacy was found to be greater than that of doxorubicin. In addition, the previous drug discovery process utilized the concept of the “lock and key” approach in which a designed drug is specific to a single protein e.g. doxorubicin complexes with DNA by intercalation and inhibits topoisomerase II. However, this approach has often failed many times and molecules were potent cytotoxic to normal cells which was also observed in the present study. However, a single compound preferably from a natural source tends to act through a polypharmacology approach in which a single molecule can target multiple proteins based on the concept “master key can unlock multiple locks” to target multiple proteins and pathways (Chandran et al., 2017). If it happens, the amount of concentration required is a bit high; however, approaches should be made through the targeted drug delivery to breast cancer which is yet to be studied. This issue could be remedied by increasing diosgenin’s cellular permeability and promoting anti-cancer action against breast tumors via a novel drug delivery mechanism, as established in previous studies (Dhamecha et al., 2015; Jagwani et al., 2020; Dhamecha et al., 2021; Ramasamy et al., 2021). In addition, the effect of diosgenin on the hub genes (SRC, MDM2, and IGF1R) expression is based on the computational models which need to be further evaluated using real-time or reverse transcriptase polymerase chain reaction even though the present study pointed their functional effect via the glucose uptake, cell proliferation, and apoptosis which is the perspective of the present findings.
Conclusion
The present study utilized a series of system biology tools to trace the potential action of diosgenin against breast cancer. Herein, we identified the probable action of the diosgenin against breast cancer via FoxO, PI3K-Akt, p53, Ras, and MAPK signaling pathways. In addition, we traced the selectivity of the diosgenin to manipulate the action of three hub genes i.e., IGF1R, MDM2, and SRC. Our molecular modeling study reveals that the stable complex formation is primarily facilitated by the cooperative closure motion exerted by the primary binding pocket residues in diosgenin-MDM2, and diosgenin-IGF1R complex while, in Diosgenin-SRC similar compact closure dynamics are also observed at the alternate binding pocket. Conformational flexibility and convergence of the trajectories during MD have been investigated using PCA. Diosgenin showed stable non-bonded interactions forming stable binary complexes with all three screened targets namely SRC, MDM2, and IGF1R. Thus, we proposed that these stable interactions of Diosgenin would trigger the successful inhibition of SRC, MDM2, and IGF1R; the newly identified targets in breast cancer. The results of our computer modeling investigations agree well with the results of the experimental cell line tests. As a result, we hope that this work will open the way for the development of novel therapeutic techniques and/or medication candidates for breast cancer.
Data availability statement
The original contributions presented in the study are included in the article/Supplementary Material, further inquiries can be directed to the corresponding authors.
Author contributions
PK: Conceptualized, experimented, drafted, edited, reviewed, and supervised the entire work. VP: Equal contribution in experimentation, drafting, editing, and reviewing the draft. VVB, PPP, PSRD, KB: Assisted in experimentation and editing. BMP, DRH, and SR: Reviewed, edited, and approved the entire work.
Funding
The work was partially supported by the Indian Council of Medical Research-National Institute of Traditional Medicine (ICMR-NITM), Belagavi, Karnataka, India (Intramural funds).
Acknowledgments
The authors gratefully acknowledge the NGSMIPS-Cell culture lab and Bioinformatics center of ICMR-NITM for providing resources.
Conflict of interest
The authors declare that the research was conducted in the absence of any commercial or financial relationships that could be construed as a potential conflict of interest.
Publisher’s note
All claims expressed in this article are solely those of the authors and do not necessarily represent those of their affiliated organizations, or those of the publisher, the editors and the reviewers. Any product that may be evaluated in this article, or claim that may be made by its manufacturer, is not guaranteed or endorsed by the publisher.
Supplementary material
The Supplementary Material for this article can be found online at: https://www.frontiersin.org/articles/10.3389/fphar.2022.1052849/full#supplementary-material
References
Aceves, C., Anguiano, B., and Delgado, G. (2005). Is iodine a gatekeeper of the integrity of the mammary gland? J. Mammary Gland. Biol. Neoplasia 10 (2), 189–196. doi:10.1007/s10911-005-5401-5
Adekola, K., Rosen, S. T., and Shanmugam, M. (2012). Glucose transporters in cancer metabolism. Curr. Opin. Oncol. 24 (6), 650–654. doi:10.1097/CCO.0b013e328356da72
Alam, M. J., Kumar, S., Singh, V., and Singh, R. K. B. (2015). Bifurcation in cell cycle dynamics regulated by p53. PLoS ONE 10 (6), e0129620. doi:10.1371/journal.pone.0129620
Amadei, A., Linssen, A. B., and Berendsen, H. J. (1993). Essential dynamics of proteins. Proteins 17 (4), 412–425. doi:10.1002/prot.340170408
Amadei, A., Linssen, A. B., De Groot, B. L., Van Aalten, D. M., and Berendsen, H. J. (1996). An efficient method for sampling the essential subspace of proteins. J. Biomol. Struct. Dyn. 13 (4), 615–625. doi:10.1080/07391102.1996.10508874
Badraoui, R., Saoudi, M., Hamadou, W. S., Elkahoui, S., Siddiqui, A. J., Alam, J. M., et al. (2022). Antiviral effects of artemisinin and its derivatives against SARS-CoV-2 main protease: Computational evidences and interactions with ACE2 allelic variants. Pharmaceuticals 15 (2), 129. doi:10.3390/ph15020129
Berendsen, H. J., Hess, B., Lindahl, E., Van Der Spoel, D., Mark, A. E., and Groenhof, G. (2005). GROMACS: Fast, flexible, and free. J. Comput. Chem. 26 (16), 1701–1718. doi:10.1002/jcc.20291
Bhandare, V. V., and Ramaswamy, A. (2018). The proteinopathy of D169G and K263E mutants at the RNA recognition motif (RRM) domain of tar DNA-binding protein (tdp43) causing neurological disorders: A computational study. J. Biomol. Struct. Dyn. 36 (4), 1075–1093. doi:10.1080/07391102.2017.1310670
Bolla, S. R., Mohammed Al-Subaie, A., Yousuf Al-Jindan, R., Papayya, B. J., Kanchi, R. P., Veeraraghavan, V. P., et al. (2019). In vitro wound healing potency of methanolic leaf extract of Aristolochiasaccata is possibly mediated by its stimulatory effect on collagen-1 expression. Heliyon 5 (5), e01648. doi:10.1016/j.heliyon.2019.e01648
Chandran, U., Mehendale, N., Patil, S., Chaguturu, R., and Patwardhan, B. (2017). Network pharmacology. Innovative Approaches Drug Discov. 2017, 127–164. doi:10.1016/B978-0-12-801814-9.00005-2
Chen, H., Yang, Z., Ding, C., Chu, L., Zhang, Y., Terry, K., et al. (2013). Discovery of O-alkylamino tethered niclosamide derivatives as potent and orally bioavailable anticancer agents. ACS Med. Chem. Lett. 4 (2), 180–185. doi:10.1021/ml3003082
Daina, A., Michielin, O., and Zoete, V. (2017). SwissADME: a free web tool to evaluate pharmacokinetics, drug-likeness and medicinal chemistry friendliness of small molecules. Sci. Rep. 7 (1), 42717–42723. doi:10.1038/srep42717
Daina, A., Michielin, O., and Zoete, V. (2019). SwissTargetPrediction: updated data and new features for efficient prediction of protein targets of small molecules. Nucleic Acids Res. 47 (W1), W357–W364. doi:10.1093/nar/gkz382
Dhamecha, D., Jalalpure, S., and Jadhav, K. (2015). Doxorubicin functionalized gold nanoparticles: Characterization and activity against human cancer cell lines. Process Biochem. 50 (12), 2298–2306. doi:10.1016/j.procbio.2015.10.007
Dhamecha, D., Le, D., Chakravarty, T., Perera, K., Dutta, A., and Menon, J. U. (2021). Fabrication of PNIPAm-based thermoresponsive hydrogel microwell arrays for tumor spheroid formation. Mater. Sci. Eng. C Mat. Biol. Appl. 125, 112100. doi:10.1016/j.msec.2021.112100
Dittrich, A., Gautrey, H., Browell, D., Tyson-Capper, A., and Tyson-Capper, A. (2014). The HER2 signaling network in breast cancer-like a spider in its web. J. Mammary Gland. Biol. Neoplasia 19 (3-4), 253–270. doi:10.1007/s10911-014-9329-5
Djerassi, C. (1992). Steroid research at syntex: "the pill" and cortisone. Steroids 57 (12), 631–641. doi:10.1016/0039-128x(92)90016-3
Dwivedi, P. S. R., Patil, R., Khanal, P., Gurav, N. S., Murade, V. D., Hase, D. P., et al. (2021). Exploring the therapeutic mechanisms of Cassia glauca in diabetes mellitus through network pharmacology, molecular docking and molecular dynamics. RSC Adv. 11 (62), 39362–39375. doi:10.1039/d1ra07661b
Dwivedi, P. S. R., Patil, V. S., Khanal, P., Bhandare, V. V., Gurav, S., Harish, D. R., et al. (2022). System biology-based investigation of Silymarin to trace hepatoprotective effect. Comput. Biol. Med. 142, 105223. doi:10.1016/j.compbiomed.2022.105223
Ekyalongo, R. C., and Yee, D. (2017). Revisiting the IGF-1R as a breast cancer target. NPJ Precis. Oncol. 1, 14. doi:10.1038/s41698-017-0017-y
Farhan, M., Wang, H., Gaur, U., Little, P. J., Xu, J., and Zheng, W. (2017). FOXO signaling pathways as therapeutic targets in cancer. Int. J. Biol. Sci. 13 (7), 815–827. doi:10.7150/ijbs.20052
Farooqi, A. A., and Siddik, Z. H. (2015). Platelet-derived growth factor (PDGF) signalling in cancer: rapidly emerging signalling landscape. Cell biochem. Funct. 33 (5), 257–265. doi:10.1002/cbf.3120
Gasco, M., Shami, S., and Crook, T. (2002). The p53 pathway in breast cancer. Breast Cancer Res. 4 (2), 70–76. doi:10.1186/bcr426
Guo, F. J., Sun, J., Gao, L. L., Wang, X. Y., Zhang, Y., Qian, S. S., et al. (2015). Discovery of phenylpiperazine derivatives as IGF-1R inhibitor with potent antiproliferative properties in vitro. Bioorg. Med. Chem. Lett. 25 (5), 1067–1071. doi:10.1016/j.bmcl.2015.01.011
Gupta, R. N., Pareek, A., Suthar, M., Rathore, G. S., Basniwal, P. K., and Jain, D. (2009). Study of glucose uptake activity of Helicteresisora Linn. fruits in L-6 cell lines. Int. J. Diabetes Dev. Ctries. 29 (4), 170–173. doi:10.4103/0973-3930.57349
Halgren, T. A. (1996). Merck molecular force field. V. Extension of MMFF94 using experimental data, additional computational data, and empirical rules. J. Comput. Chem. 17, 616–641. doi:10.1002/(sici)1096-987x(199604)17:5/6<616::aid-jcc5>3.0.co;2-x
HeraviKarimovi, M., Pourdehqan, M., JadidMilani, M., Foroutan, S. K., and Aieen, F. (2006). Study of the effects of group counseling on quality of sexual life of patients with breast cancer under chemotherapy at Imam Khomeini Hospital. J. MazandaranUniv Med. Sci. 16 (54), 43–51.
Herbein, G., and Kumar, A. (2014). The oncogenic potential of human cytomegalovirus and breast cancer. Front. Oncol. 4, 230. doi:10.3389/fonc.2014.00230
Huang, L., and Fu, L. (2015). Mechanisms of resistance to EGFR tyrosine kinase inhibitors. Acta Pharm. Sin. B 5 (5), 390–401. doi:10.1016/j.apsb.2015.07.001
Huang, M., Anand, S., Murphy, E. A., Desgrosellier, J. S., Stupack, D. G., Shattil, S. J., et al. (2012). EGFR-dependent pancreatic carcinoma cell metastasis through Rap1 activation. Oncogene 31 (22), 2783–2793. doi:10.1038/onc.2011.450
Ivanov, S. M., Lagunin, A. A., Rudik, A. V., Filimonov, D. A., and Poroikov, V. V. (2018). ADVERPred–Web service for prediction of adverse effects of drugs. J. Chem. Inf. Model. 58 (1), 8–11. doi:10.1021/acs.jcim.7b00568
Jagwani, S., Jalalpure, S., Dhamecha, D., Jadhav, K., and Bohara, R. (2020). Pharmacokinetic and pharmacodynamic evaluation of resveratrol loaded cationic liposomes for targeting hepatocellular carcinoma. ACS Biomater. Sci. Eng. 6 (9), 4969–4984. doi:10.1021/acsbiomaterials.0c00429
Jemal, A., Siegel, R., Ward, E., Hao, Y., Xu, J., and Thun, M. J. (2009). Cancer statistics, 2009. CA. Cancer J. Clin. 59 (4), 225–249. doi:10.3322/caac.20006
Kanai, M., Konda, Y., Nakajima, T., Izumi, Y., Kanda, N., Nanakin, A., et al. (2003). Differentiation-inducing factor-1 (DIF-1) inhibits STAT3 activity involved in gastric cancer cell proliferation via MEK-ERK-dependent pathway. Oncogene 22 (4), 548–554. doi:10.1038/sj.onc.1206109
Khanal, P., Zargari, F., Far, B. F., Kumar, D., Mogana, R., Mahdi, Y. K., et al. (2021). Integration of system biology tools to investigate huperzine A as an anti-Alzheimer agent. Front. Pharmacol. 12, 785964. doi:10.3389/fphar.2021.785964
Khanal, P., Patil, V. S., Bhandare, V. V., Dwivedi, P. S., Shastry, C. S., Patil, B. M., et al. (2022). Computational investigation of benzalacetophenone derivatives against SARS-CoV-2 as potential multi-target bioactive compounds. Comput. Biol. Med. 146, 105668. doi:10.1016/j.compbiomed.2022.105668
Kim, S., Dubro ka, A., Salamone, R. J., Walker, J. R., Grandinetti, K. B., Bonamy, G. M., et al. (2013). FGFR2 promotes breast tumorigenicity through maintenance of breast tumor-initiating cells. PLoS One 8 (1), e51671. doi:10.1371/journal.pone.0051671
Kumari, R., Kumar, R., and Lynn, A.Open Source Drug Discovery Consortium (2014). g_mmpbsa-a GROMACS tool for high-throughput MM-PBSA calculations. J. Chem. Inf. Model. 54 (7), 1951–1962. doi:10.1021/ci500020m
Lagunin, A., Ivanov, S., Rudik, A., Filimonov, D., and Poroikov, V. (2013). DIGEP-pred: Web service for in silico prediction of drug-induced gene expression profiles based on structural formula. Bioinformatics 29 (16), 2062–2063. doi:10.1093/bioinformatics/btt322
Li, R., Pourpak, A., and Morris, S. W. (2009). Inhibition of the insulin-like growth factor-1 receptor (IGF1R) tyrosine kinase as a novel cancer therapy approach. J. Med. Chem. 52 (16), 4981–5004. doi:10.1021/jm9002395
Li, B. H., Ge, J. Q., Wang, Y. L., Wang, L. J., Zhang, Q., and Bian, C. (2021). Ligand-based and docking-based virtual screening of MDM2 inhibitors as potent anticancer agents. Comput. Math. Methods Med. 2021, 3195957. doi:10.1155/2021/3195957
Liu, T., Lin, Y., Wen, X., Jorissen, R. N., and Gilson, M. K. (2007). BindingDB: a web-accessible database of experimentally determined protein-ligand binding affinities. Nucleic Acids Res. 35, D198–D201. doi:10.1093/nar/gkl999
Liu, H., Yang, Z., Lu, W., Chen, Z., Chen, L., Han, S., et al. (2020). Chemokines and chemokine receptors: A new strategy for breast cancer therapy. Cancer Med. 9 (11), 3786–3799. doi:10.1002/cam4.3014
Mantovani, F., Collavin, L., and Del Sal, G. (2019). Mutant p53 as a guardian of the cancer cell. Cell Death Differ. 26 (2), 199–212. doi:10.1038/s41418-018-0246-9
Marker, R. E., and Krueger, J. (1940). Sterols. CXII. Sapogenins. XLI. The preparation of Trillin and its conversion to progesterone. J. Am. Chem. Soc. 62 (12), 3349–3350. doi:10.1021/ja01869a023
McLaughlin, J., Rogers, L. L., and Anderson, J. E. (1998). The use of biological assays to evaluate botanicals. Drug Inf. J. 32 (2), 513–524. doi:10.1177/009286159803200223
Momand, J., Jung, D., Wilczynski, S., and Niland, J. (1998). The MDM2 gene amplification database. Nucleic Acids Res. 26 (15), 3453–3459. doi:10.1093/nar/26.15.3453
Moon, A. (2021). Ras signaling in breast cancer. Adv. Exp. Med. Biol. 1187, 81–101. doi:10.1007/978-981-32-9620-6_4
Mosmann, T. (1983). Rapid colorimetric assay for cellular growth and survival: Application to proliferation and cytotoxicity assays. J. Immunol. Methods 65 (1-2), 55–63. doi:10.1016/0022-1759(83)90303-4
Munshi, S., Kornienko, M., Hall, D. L., Reid, J. C., Waxman, L., Stirdivant, S. M., et al. (2002). Crystal structure of the apo, unactivated insulin-like growth factor-1 receptor kinase: implication for inhibitor specificity. J. Biol. Chem. 277 (41), 38797–38802. doi:10.1074/jbc.M205580200
Pancotti, F., Roncuzzi, L., Maggiolini, M., and Gasperi-Campani, A. (2012). Caveolin-1 silencing arrests the proliferation of metastatic lung cancer cells through the inhibition of STAT3 signaling. Cell. Signal. 24 (7), 1390–1397. doi:10.1016/j.cellsig.2012.02.015
Patil, V. S., Harish, D. R., Vetrivel, U., Deshpande, S. H., Khanal, P., Hegde, H. V., et al. (2022). Pharmacoinformatics analysis reveals flavonoids and diterpenoids from Andrographis paniculata and Thespesia populnea to target hepatocellular carcinoma induced by hepatitis B virus. Appl. Sci. 12 (21), 10691. doi:10.3390/app122110691
Quesnel, B., Preudhomme, C., Fournier, J., Fenaux, P., and Peyrat, J. P. (1994). MDM2 gene amplification in human breast cancer. Eur. J. Cancer 30A (7), 982–984. doi:10.1016/0959-8049(94)90128-7
Ramasamy, S., Dhamecha, D., Kaliyamoorthi, K., Pillai, A. S., Alexander, A., Dhanaraj, P., et al. (2021). Magnetic hydroxyapatite nanomaterial–cyclodextrin tethered polymer hybrids as anticancer drug carriers. Mater. Adv. 2 (10), 3315–3327. doi:10.1039/d1ma00142f
Rani, A., Stebbing, J., Giamas, G., and Murphy, J. (2019). Endocrine resistance in hormone receptor positive breast cancer-from mechanism to therapy. Front. Endocrinol. 10, 245. doi:10.3389/fendo.2019.00245
Russo, J., and Russo, I. H. (2006). The role of estrogen in the initiation of breast cancer. J. Steroid Biochem. Mol. Biol. 102 (1-5), 89–96. doi:10.1016/j.jsbmb.2006.09.004
Saeed, M., Saeed, A., Alam, M. J., and Alreshidi, M. (2020). Identification of persuasive antiviral natural compounds for COVID-19 by targeting endoribonuclease NSP15: A structural-bioinformatics approach. Molecules 25 (23), 5657. doi:10.3390/molecules25235657
Samdani, A., and Vetrivel, U. (2018). POAP: A GNU parallel based multithreaded pipeline of open babel and AutoDock suite for boosted high throughput virtual screening. Comput. Biol. Chem. 74, 39–48. doi:10.1016/j.compbiolchem.2018.02.012
Sangodkar, J., Dhawan, N. S., Melville, H., Singh, V. J., Yuan, E., Rana, H., et al. (2012). Targeting the FOXO1/KLF6 axis regulates EGFR signaling and treatment response. J. Clin. Invest. 122 (7), 2637–2651. doi:10.1172/JCI62058
Sariego, J. (2010). Breast cancer in the young patient. Am. Surg. 76 (12), 1397–1400. doi:10.1177/000313481007601226
Shannon, P., Markiel, A., Ozier, O., Baliga, N. S., Wang, J. T., Ramage, D., et al. (2003). Cytoscape: a software environment for integrated models of biomolecular interaction networks. Genome Res. 13 (11), 2498–2504. doi:10.1101/gr.1239303
Shishodia, S., and Aggarwal, B. B. (2006). Diosgenin inhibits osteoclastogenesis, invasion, and proliferation through the downregulation of Akt, I kappa B kinase activation and NF-kappa B-regulated gene expression. Oncogene 25 (10), 1463–1473. doi:10.1038/sj.onc.1209194
Srinivasan, S., Koduru, S., Kumar, R., Venguswamy, G., Kyprianou, N., and Damodaran, C. (2009). Diosgenin targets Akt-mediated prosurvival signaling in human breast cancer cells. Int. J. Cancer 125 (4), 961–967. doi:10.1002/ijc.24419
Steiner, E., Klubert, D., and Knutson, D. (2008). Assessing breast cancer risk in women. Am. Fam. Physician 78 (12), 1361–1366.
Stoddard, F.R., Brooks, A. D., Eskin, B. A., and Johannes, G. J. (2008). Iodine alters gene expression in the MCF7 breast cancer cell line: evidence for an anti-estrogen effect of iodine. Int. J. Med. Sci. 5 (4), 189–196. doi:10.7150/ijms.5.189
Szklarczyk, D., Gable, A. L., Nastou, K. C., Lyon, D., Kirsch, R., Pyysalo, S., et al. (2021). The STRING database in 2021: customizable protein-protein networks, and functional characterization of user-uploaded gene/measurement sets. Nucleic Acids Res. 49 (D1), D605–D612. doi:10.1093/nar/gkaa1074
Tokunaga, E., Kimura, Y., Mashino, K., Oki, E., Kataoka, A., Ohno, S., et al. (2006). Activation of PI3K/Akt signaling and hormone resistance in breast cancer. Breast Cancer 13 (2), 137–144. doi:10.2325/jbcs.13.137
Trott, O., and Olson, A. J. (2010). AutoDock Vina: improving the speed and accuracy of docking with a new scoring function, efficient optimization, and multithreading. J. Comput. Chem. 31 (2), 455–461. doi:10.1002/jcc.21334
Tworoger, S. S., Eliassen, A. H., Rosner, B., Sluss, P., and Hankinson, S. E. (2004). Plasma prolactin concentrations and risk of postmenopausal breast cancer. Cancer Res. 64 (18), 6814–6819. doi:10.1158/0008-5472.CAN-04-1870
Tworoger, S. S., Sluss, P., and Hankinson, S. E. (2006). Association between plasma prolactin concentrations and risk of breast cancer among predominately premenopausal women. Cancer Res. 66 (4), 2476–2482. doi:10.1158/0008-5472.CAN-05-3369
Van Aalten, D. M., Findlay, J. B., Amadei, A., and Berendsen, H. J. (1995). Essential dynamics of the cellular retinol-binding protein evidence for ligand-induced conformational changes. Protein Eng. 8 (11), 1129–1135. doi:10.1093/protein/8.11.1129
Venturi, S. (2001). Is there a role for iodine in breast diseases? Breast 10 (5), 379–382. doi:10.1054/brst.2000.0267
Verbeek, B. S., Vroom, T. M., Adriaansen-Slot, S. S., Ottenhoff-Kalff, A. E., Geertzema, J. G., Hennipman, A., et al. (1996). c-Src protein expression is increased in human breast cancer. An immunohistochemical and biochemical analysis. J. Pathol. 180 (4), 383–388. doi:10.1002/(SICI)1096-9896(199612)180:4<383::AID-PATH686>3.0.CO;2-N
Wang, L., Han, H., Dong, L., Wang, Z., and Qin, Y. (2021). Function of p21 and its therapeutic effects in esophageal cancer. Oncol. Lett. 21 (2), 136–137. doi:10.3892/ol.2020.12397
Wheeler, D. L., Lida, M., and Dunn, E. F. (2009). The role of Src in solid tumors. Oncologist 14 (7), 667–678. doi:10.1634/theoncologist.2009-0009
Xiong, G., Wu, Z., Yi, J., Fu, L., Yang, Z., Hsieh, C., et al. (2021). ADMETlab 2.0: an integrated online platform for accurate and comprehensive predictions of ADMET properties. Nucleic Acids Res. 49 (W1), W5–W14. doi:10.1093/nar/gkab255
Yager, J. D., and Davidson, N. E. (2006). Estrogen carcinogenesis in breast cancer. N. Engl. J. Med. 354 (3), 270–282. doi:10.1056/NEJMra050776
Yue, W., Wang, J. P., Conaway, M., Masamura, S., Li, Y., and Santen, R. J. (2002). Activation of the MAPK pathway enhances sensitivity of MCF-7 breast cancer cells to the mitogenic effect of estradiol. Endocrinology 143 (9), 3221–3229. doi:10.1210/en.2002-220186
Keywords: breast cancer, computational pharmacology, diosgenin, gene ontology analysis, system biology
Citation: Khanal P, Patil VS, Bhandare VV, Patil PP, Patil BM, Dwivedi PSR, Bhattacharya K, Harish DR and Roy S (2023) Systems and in vitro pharmacology profiling of diosgenin against breast cancer. Front. Pharmacol. 13:1052849. doi: 10.3389/fphar.2022.1052849
Received: 24 September 2022; Accepted: 05 December 2022;
Published: 04 January 2023.
Edited by:
Mitesh Patel, Parul University, IndiaReviewed by:
Mohammad Jahoor Alam, University of Hail, Saudi ArabiaMejdi Snoussi, University of Hail, Saudi Arabia
Copyright © 2023 Khanal, Patil, Bhandare, Patil, Patil, Dwivedi, Bhattacharya, Harish and Roy. This is an open-access article distributed under the terms of the Creative Commons Attribution License (CC BY). The use, distribution or reproduction in other forums is permitted, provided the original author(s) and the copyright owner(s) are credited and that the original publication in this journal is cited, in accordance with accepted academic practice. No use, distribution or reproduction is permitted which does not comply with these terms.
*Correspondence: Pukar Khanal, cHVrYXJraGFuYWw1OEBnbWFpbC5jb20=; Darasaguppe R. Harish, ZHJoYXJpc2g0OUBnbWFpbC5jb20=
†These authors have contributed equally to this work and share first authorship