- 1Department of Pharmacy, The First People’s Hospital of Chongqing Liang Jiang New Area, Chongqing, China
- 2Department of Pharmacology, Chongqing Health Center for Women and Children, Chongqing, China
Background and Objective: The public’s safety has been significantly jeopardized by the pandemic of COVID-19, which is brought on by the highly virulent and contagious SARS-CoV-2 virus. Finding novel antiviral drugs is currently of utmost importance for the treatment of patients with COVID-19. Main protease (3CLpro) of SARS-CoV-2 is involved in replication of virus, so it is considered as a promising target. Using small molecules to inhibit SARS-CoV-2-3CLpro activity may be an effective way to prevent viral replication to fight COVID-19. Despite the fact that some SARS-CoV-2-3CLpro inhibitors have been described, only few of them have high levels of inhibition at nanomolar concentrations. In this study, we aimed to screen out effective SARS-CoV-2-3CLpro inhibitors.
Methods: To identify highly effective SARS-CoV-2-3CLpro inhibitors, a pharmacophore mapping and multiple-conformation docking were efficiently applied to find novel hit compounds from a database. Then, the stability of the 3CLpro-hit complexes was validated by using molecular dynamics simulation. Finally, biological assay was used to assess the inhibition effects of hit compounds on SARS-CoV-2-3CLpro.
Results: Four hit compounds were identified by using computer-assisted strategy. Molecular dynamics simulation suggested that these hits bound stably to the 3CLpro-active pocket. Bioassay showed that all the hits had potent inhibition against SARS-CoV-2-3CLpro with IC50 values in the range of 0.017–0.83 μM. Particularly, hit one was the best 3CLpro inhibitor and its inhibition effect of SARS-CoV-2-3CLpro (IC50 = 0.017 ± 0.003 µM) was about 236 times stronger than that of ML300 (IC50 = 4.01 ± 0.66 µM).
Conclusion: These data indicate that hit one could be regarded as an anti-SARS-CoV-2 candidate worth exploring further for the treatment of COVID-19.
1 Introduction
Since December 2019, the pandemic of COVID-19 caused by SARS-CoV-2, has infected more than 166 million people and killed more than 3.5 million people worldwide (Koutsakos and Kedzierska, 2020; Nassau et al., 2022). SARS-CoV-2 usually induces respiratory symptoms, such as cough and fever (Guan et al., 2020; Narayanan et al., 2022). Although effective antiviral agents have been developed against COVID-19, the rapid mutation of the virus limits their clinical use. (Tao et al., 2021; Chakraborty et al., 2022). Some studies have shown that the mutations in the S-glycoprotein and RdRp of the genome cause therapeutic resistance of antibodies or small molecules (Tao et al., 2021; Chakraborty et al., 2022; Rockett et al., 2022; Stevens et al., 2022). Therefore, it is urgent to develop novel, highly potent antiviral candidates to treat COVID-19.
Efforts are under way to characterize molecular targets, which are essential for the development of anti-coronavirus drugs. SARS-CoV-2 main protease (3CLpro) is mainly involved in the cleavage of polyproteins (PPs) to generate non-structural proteins (NSPs), which are then compiled into replicase transcriptase complexes (RTC) (Jin et al., 2020). 3CLpro is a dimer composed of two monomers that are arranged almost perpendicular to each other (Zehra et al., 2020). Each monomer has three distinct domains: Domains I and II display an antiparallel β-barrel structure and contain the catalytic dyad (His41 and Cys145), while domain III is a large antiparallel globular structure composed of five α-helices (Zehra et al., 2020). Because 3CLpro of SARS-CoV-2 is involved in viral polyproteins processing and maturation (Xia and Kang, 2011), it is considered to be a promising target. 3CLpro cleaves the initially translated viral polyproteins, generating nonstructural proteins, and then interferes with viral replication and maturation (Ferreira et al., 2021). Thus, inhibition of 3CLpro activity would block viral replication (Vuong et al., 2020). In addition, it contains a unique recognition sequence Leu-Gln*Ser-Ala-Gly (* marks the cleavage site) during its cleavage function on viral polyprotein, which is unrecognized by any known human protease (Zehra et al., 2020). This suggests that compounds capable of inhibiting 3CLpro are unlikely to be toxic (Adegbola et al., 2021). Therefore, SARS-CoV-2-3CLpro is recognized as an effective drug target for treating COVID-19.
In view of the continuous variation of SARS-CoV-2, the clinical researches of some antibody drugs have stalled. However, the vast majority of molecules on 3CLpro inhibitors have great potential in fighting against new coronavirus variants. The following are some advances in the development of drugs targeting SARS-CoV-2-3CLpro (Supplementary Table S1). For example, paxlovir (PF-07321332), a first protease inhibitor against the SARS-CoV-2 protease 3CLpro, has recently been approved as an antiviral for SARS-CoV-2 by the United States Food and Drug Administration (FDA) (Abdelnabi et al., 2022). Tyndall et al. discovered that S-217622 is a noncovalent nonpeptide oral inhibitor against SARS-CoV-2-3CLpro and the latest clinical results show that S-217722 has good antiviral activity and oral bioavailability (Tyndall, 2022). Boras et al. reported that PF-07304814 can act as a broad-spectrum coronavirus 3CLpro inhibitor and showed potent antiviral activity in vivo (Boras et al., 2021). In addition, EDP-235 is a novel and highly selective 3CLpro inhibitor and was evaluated in the first in-human phase I study in healthy volunteers for safety, tolerability, and pharmacokinetics (Hu et al., 2022).
Computer-aided drug design (CADD) including pharmacophore modeling and molecular docking has established itself as a valuable in silico technique for the identification of the probable inhibitors that could prevent the activity of an enzyme (Kitchen et al., 2004; Maga et al., 2008). Compared with the traditional drug discovery strategies, this method significantly decreases the time and cost to develop a new drug (Kitchen et al., 2004; Wang et al., 2005; Maga et al., 2008; Gao et al., 2010; Wieder et al., 2017). By the CACS strategy, researchers successfully identified a highly potent peptide, NKTP-3, with dual inhibitory effects on both NRP1 and KRASG12D (Zhou et al., 2022). Zheng et al. also discovered an anticancer agent TP-3 targeting tubulin and PARP-1 using a CACS approach (Zheng et al., 2021). Furthermore, Zhou et al. used a combination of pharmacophore and docking approaches to identify a first candidate peptide with dual-targeting both NRP1 and MDM2 (Zhou et al., 2021). These studies suggested that the combined screening is an attractive strategy in drug lead exploration.
In this study, with the recently resolved inhibitor-bound SARS-CoV-2-3CLpro crystal structure available (Han et al., 2022), we constructed a structure-based pharmacophore model of 3CLpro. An efficient database screening strategy was used for the virtual screening of 3CLpro inhibitors from a virtual database. Four hit compounds were finally identified based on low root-mean-square distance (RMSDx) values as well as better docking scores and subjected to molecular dynamics simulation. Biological assay showed that these four hit compounds had an inhibition effect on SARS-CoV-2-3CLpro activity. These results indicate that the structure-based approach is also suitable for the discovery of SARS-CoV-2-3CLpro inhibitors.
2 Materials and methods
2.1 Structure-based pharmacophore modeling
In this modeling study, we used the 3CLpro (PDB code: 7LME) from SARS-CoV-2 in complex with ML300 as the crystal structure. We selected this crystal structure according to the following criteria (Tian et al., 2022): 1) The organism of the selected crystal structure should be SARS-CoV-2 rather than other species. The organism of the 3CLpro structure is SARS-CoV-2. 2) One of the quality indexes of protein crystal structure is resolution, which represents the uncertainty of atomic position in crystal structure model. When there are many crystal structures available, we choose the one with high resolution (that is, the one with small resolution value). Generally, structures with a resolution less than 3 Å are sufficient for pharmacophore modeling. The 3CLpro crystal structure has a high resolution of 2.10 Å 3) The selected crystal structure should include an active pocket; the crystal structure of 3CLpro contains the active-binding pocket. This structure file was prepared by the MOE program (Zhou et al., 2019a), with the following protocol: hydrogens were added, water molecules were removed, partial charges were computed and energy minimization was carried out using the Amber10:EHT forcefield. In the Pharmacophore Query Editor, PCH pharmacophore scheme including hydrogen-bond acceptor, aromatic, and hydrophobic feature was selected. Amber10:EHT forcefield was assigned to the system. Pharmacophore Query Editor was used to manually construct a visualized 3D-pharmacophore model by analyzing protein-ligand interaction in the 3CLpro complex (Zhou et al., 2019a; Zhou et al., 2019b). In our study, the generated pharmacophore model was exported and translated into a pharmacophore file by a script.
2.2 Güner–Henry (GH) scoring method
According to a previously reported method (Zhou et al., 2019a), the GH method was used to evaluate precision of model selectivity. In the study, we used the generated pharmacophore model to successfully screen actives from a 1,090 molecular database. This database consists of the decoy set containing 1,080 molecules (retrieved from DUD-E database) (Mysinger et al., 2012) and 10 known 3CLpro inhibitors (Han et al., 2022). The resulted mapping data was used to evaluate pharmacophore quality by solving the following equation (The GH score with greater than 0.6 indicated a good model):
2.3 Virtual screening
The virtual screening was performed to screen a chemical database containing 35,000 molecules (Zhou et al., 2019a). In the course of screening, we applied the Pharmacophore Search as a screening protocol to identify active compounds that have good matching with query features of the generated pharmacophore model (Zhou et al., 2019a). Hit molecules can be ranked by their RMSDx values which indicate how well the matching ligand annotation points of the chemical structures were mapped onto the query features of the model. A low RMSDx value indicates a good matching of the ligand annotation points with query features of the model.
2.4 Molecular docking experiments
In this study, we used the 3CLpro structure from SARS-CoV-2 with ML300 (PDB code: 7LME) from the pharmacophore study (Han et al., 2022). It has high resolution and relatively complete structure, so it is used as the docking model. By using default settings, the hit compounds obtained were docked into the 3CLpro active site containing some amino acid residues (such as Phe140, Leu141, Met165, Met49, Thr25, and Thr24) through the Triangle Matcher Docking protocol of MOE (Zhou et al., 2019a; Zhou et al., 2019b). Amber10:EHT forcefield was assigned to the system. The dG docking scoring function are selected to rank the compounds.
2.5 In silico ADME studies
ADMETlab web server (https://admetmesh.scbdd.com/) was used to predict the ADME properties of selected hits (Tian et al., 2022). The molecular weight (mol_MW), number of hydrogen bond acceptors (nHA), number of hydrogen bond donors (nHD), log of the octanol/water partition coefficient (logP), and log of the aqueous solubility (LogS) were evaluated.
2.6 Molecular dynamics simulations
According to a previously reported method (Tian et al., 2022), protein-hit complexes were investigated by molecular dynamics simulation using Groningen machine for chemical simulations software (GROMACS).
2.7 Microscale thermophoresis (MST) assay
The purified 3CLpro protein was purchased from Abcam (Cambridge, MA, United States). According to a previously reported method (Hang et al., 2019), the binding affinity of the compounds with SARS-CoV-2 3CLpro was detected by MST assay. Briefly, purified SARS-CoV-2 3CLpro was labelled with the Monolith NT Protein Labelling Kit RED (NanoTemper Technologies). Serially diluted compounds, with concentrations of 0.76 nM–25 μM, were mixed with 100 nM labelled SARS-CoV-2 3CLpro at room temperature and loaded into Monolith standard-treated capillaries. The fluorescent signal was detected by Monolith NT.115 (NanoTemper, Munich, Germany). The Kd value was calculated by fitting a standard binding curve. Experiment was performed in triplicate.
2.8 Inhibitory effects on SARS-CoV-2-3CLpro activity
According to a previously reported method (Han et al., 2022), 200 nM of SARS-CoV-2 3CLpro were incubated with different concentrations of compounds (0, 0.19, 0.76, 3.05, 12.21, 48.83, 195.31, 781.25, 3125, 12,500, and 50,000 nM) for 20 min. The reaction was initiated by adding fluorophore-quencher peptide substrate HiyteFluor-488ESATLQSGLRKAK-(QXL)-NH2, followed by 30 min incubation at 25°C. Fluorescence intensity was measured on a microplate reader (λex = 485 nm; λem = 528 nm). Experiment was performed in triplicate.
3 Results and discussion
3.1 Pharmacophore model generation and validation
Recently, researchers have reported the structural complex of SARS-CoV-2 3CLpro with ML300 (PDB code: 7LME) (Han et al., 2022). Therefore, we used this structure analyze the chemical features of 3CLpro-ML300 interaction (Figure 1A). Based on their interaction between 3CLpro and ML300 (Figure 1B), the complex structure was used to generate a 3CLpro-pharmacophore model (Figure 1C). This model consists of four features: F1 hydrophobic feature, F2 hydrogen-bond acceptor feature, F3 aromatic feature, and F4 aromatic feature. The F1 feature mapped with its aromatic ring of ML300 described hydrophobic interactions with residues Phe140 and Leu141 in the 3CLpro-active site (Figure 1D). The F2 feature mapped with the oxygen atom of ML300, formed an important hydrogen-bond interaction between ML300 and Glu166, while the aromatic rings of ML300 mapped on the aromatic features are involved in interactions with hydrophobic residues Met165 and Met49. The results indicates that the 3CLpro-model features could effectively map the interactions between ligands and 3CLpro-active residues.
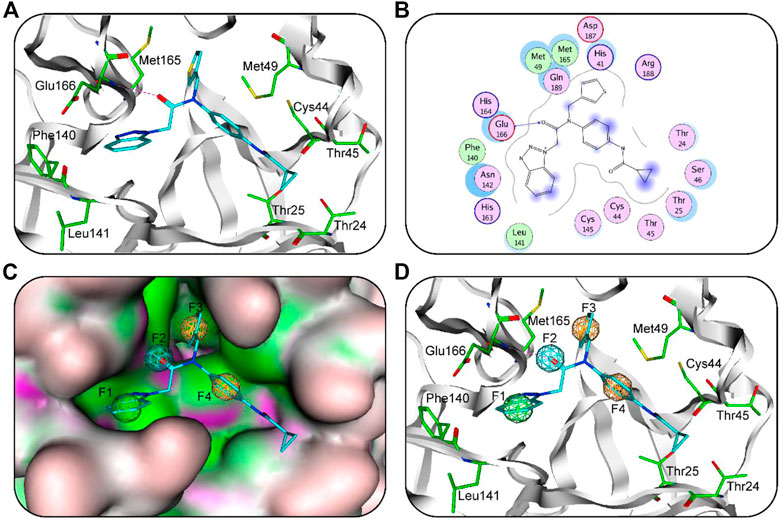
FIGURE 1. (A) 3D binding mode of ML300 and active-site residues of 3CLpro. Cyan stick represent ML300 and green color represents residues; tube form represents the protein backbone; Red dotted line represents the hydrogen-bonding interactions. (B) 2D binding mode of ML300 and active-site residues of 3CLpro. Blue dotted line indicates hydrogen-bond interaction; Pink represents polar amino acid residues; green represents greasy amino acid residues. (C) The generated 3CLpro-pharmacophore model. (D) The 3CLpro-model mapped onto ML300. Green, cyan, and orange represent F1 hydrophobic feature, F2 hydrogen-bond acceptor feature and two aromatic features (F3 and F4), respectively.
3.2 Pharmacophore model Validation
The quality of 3CLpro-model was validated using GH scoring method (Tian et al., 2022). The GH analysis were done by computing statistical parameters such as the enrichment factor (E) and goodness of hit score (GH). The 3CLpro-model was successful in retrieving 90% of active compounds from the decoys set (Table 1). Moreover, an enrichment factor of 89 and a GH score of 0.84 indicated good quality of the model. The result reveals that the model can distinguish active inhibitors of 3CLpro from the decoy set.
3.3 Virtual screening
The flowchart in Figure 2 is a detailed schematic diagram of our virtual screening process in this study. The validated SARS-CoV-2-3CLpro-model is applied as a 3D search query to virtually screen a 35,000-compound database. The obtained 922 compounds that could be mapped onto 3CLpro-model model were successfully retrieved, including 103 compounds with RMSDx values lower than 0.65 Å (the lower the value, the better the pharmacophore mapping). Subsequently, the retrieved 103 compounds were docked into the 3CLpro-active site to reduce the false positive molecules. The 3CLpro inhibitor ML300 with a docking score of -10.90 kcal/mol was used as a positive control. Finally, the top four hits (hits 1–4) with lower docking scores (lower than -10.90 kcal/mol) were selected (Table 2). The analysis of the model matching results of four hit compounds showed that the oxygen atoms of four hit compounds matched the F2 hydrogen-bond acceptor feature of 3CLpro-model while their two aromatic rings were mapped with the F3 and F4 aromatic features, respectively (Figure 3). Moreover, the hydrophobic groups of four hits matched the features of F1 hydrophobic feature. The superimposition results indicated that the four structurally similar hits match the 3CLpro-model very well. The structural and chemical similarities of four hits suggested good pharmacophore mapping and docking scores. Furthermore, additional characterization of hits one to four such as the molecular weight (mol_MW), number of hydrogen bond acceptors (nHA), number of hydrogen bond donors (nHD), log of the octanol/water partition coefficient (logP), and log of the aqueous solubility (LogS) was evaluated and their parameter values are in the optimal range (Supplementary Table S2), suggesting their druggable and pharmacokinetics properties. Finally, four candidate hits (hits 1–4) were used as candidate molecules for performing molecular dynamics simulations.
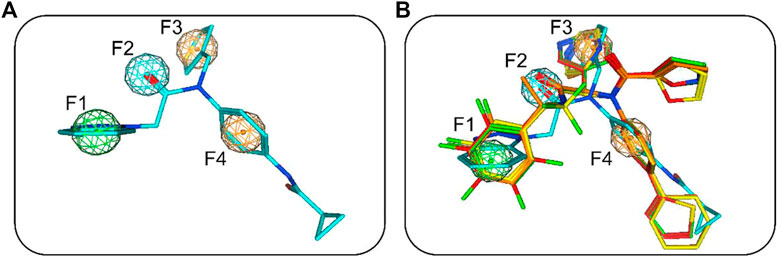
FIGURE 3. (A) SARS-CoV-2-3CLpro-model mapping with ML300. (B) Superimposition of ML300 and hits one to four with 3CLpro-model. Green, cyan, and orange represent F1 hydrophobic feature, F2 hydrogen-bond acceptor feature and two aromatic features (F3 and F4), respectively.
3.4 Molecular dynamics (MD) simulations
To evaluate the binding stability of each 3CLpro-hit complex from the docking study, we conducted 50 ns MD simulation to analyze the parameters such as root mean square deviation (RMSD) and root mean square fluctuation (RMSF). As shown in Figure 4, the movement of 3CLpro protein and each hit-3CLpro complex was monitored. We observed that 3CLpro protein and each 3CLpro-hit complex can exhibit stable internal motion throughout the simulation process. The RMSF was also calculated that provides knowledge on the flexibility of the protein residues. Figure 5 shows that compared with that of 3CLpro protein alone, the RMSF fluctuation values of the active residues (Glu166, Phe140, Leu141, Met165, Met49, Thr25, and Thr24) from all hit-3CLpro complexes were relatively small. In addition, we also monitor the movement of each ligand in the four complexes. As depicted in Supplementary Figure S1, the RMSD value for each individual hit (from each 3CLpro-hit complex during MD simulation) is below 0.33 nm, indicating its stability at the binding site. Overall, these data show that four hits can stably bind to the active site of 3CLpro.
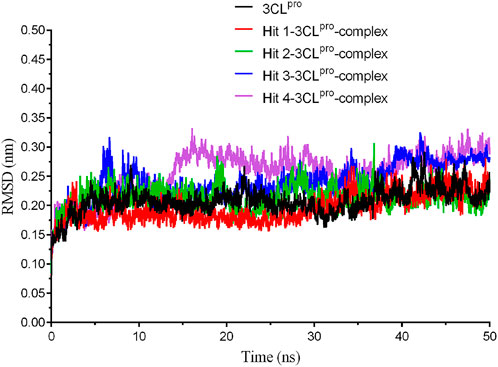
FIGURE 4. The root-mean-square deviation (RMSD) trajectories of 3CLpro protein and each hit-3CLpro complex during 50 ns simulations.
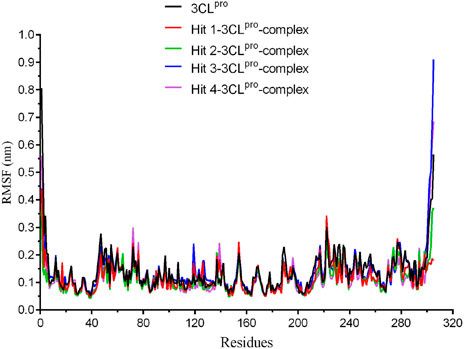
FIGURE 5. The root-mean-square fluctuation (RMSF) of amino acid residues from 3CLpro protein and each hit-3CLpro complex during 50 ns simulations.
3.5 Inhibitory effects on SARS-CoV-2-3CLpro activity
To characterize hits one to four targeting 3CLpro in vitro, we used the MST assay to measure the binding affinity of hits one to four to 3CLpro. The previously reported 3CLpro inhibitor ML300 was used as a positive control. MST assay showed that the Kd values of hits one to four to 3CLpro were ranged from 0.012 μM to 0.27 μM (Supplementary Figure S2). We also evaluated the inhibition effect of hits one to four on 3CLpro by enzyme inhibition assay. As shown in Table 2 and Supplementary Figure S3, all the four hits inhibited SARS-CoV-2-3CLpro activity, with IC50 values ranging from 0.017 to 0.83 μM. In particular, hit one is a promising 3CLpro inhibitor. Its inhibition activity (IC50 = 0.017 ± 0.003 µM) was about 236 times stronger than that of ML300 (IC50 = 4.01 ± 0.66 µM). Through the search analysis of PubChem and SciFinder, it was found that hits one to four with the inhibition activity of 3CLpro were reported for the first time, indicating that they are novel SARS-CoV-2-3CLpro inhibitors. These experimental data demonstrate that our virtual screening protocol is very reliable in identifying novel and effective 3CLpro inhibitors.
3.6 Analysis of the interaction mode
The binding modes of hit 1 with SARS-CoV-2-3CLpro were further analyzed. The oxygen atom of hit one formed a hydrogen-bond interaction with Glu166 and hydrophobic interactions with key residues including Phe140, Leu141, Met165, Met49, Thr25, and Thr24 (Figure 6A). As shown in Figure 6B, it can be observed that the 3CLpro-active site has multiple hydrophobic sites, and the active compound targeting SARS-CoV-2-3CLpro should occupy each site at the same time. Our molecular docking results indicated that hit one showed excellent geometric matching with SARS-CoV-2-3CLpro. In addition, we also measured the inter-atomic distance profile of the important interacting atom pairs (hydrogen bond: for details see Supplementary Table S3) of the 3CLpro and hits during the MD simulation. The result indicates that the oxygen atoms of all hits can form hydrogen-bond interactions with Glu166. In order to further evaluate the stability of the 3CLpro in complex with hits one to four, we also used DSSP algorithm to monitor the secondary structure changes of each 3CLpro-hit complex during MD simulation (Supplementary Figure S4). The result suggests that the changes of structural elements (such as α- Helix and β-Sheet content) were not observed, indicating the stability of each hit-complex system. As shown in Supplementary Figure S5, it can be clearly observed that the 3CLpro complexes superpose well with the 3CLpro alone with a Cα-RMSD < 2.5 Å, indicating that all systems retained the structural integrity and maintained the same fold with minor changes of the loop region in the 3CLpro of SARS-Cov-2.
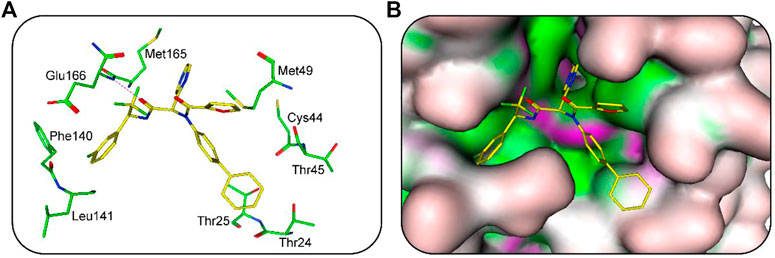
FIGURE 6. (A) Predicted binding mode of hit 1 (yellow, sticks) and the 3CLpro active-site residues (green, sticks). The hydrogen bonds are illustrated as red dotted lines. (B) Binding mode of 3CLpro-hit-1 complex.
4 Conclusion
With the fourth wave of COVID-19 sweeping the world, the development of COVID-19 therapeutics is still a major challenge. The 3CLpro plays a vital role in viral replication and has hence been considered as a potent drug target for SARS-CoV-2 infection. However, the development of highly effective 3CLpro inhibitors has made limited progress. Here, we proposed a combined screening strategy of structure-based virtual screening including model mapping, molecular docking and MD simulation. Through this virtual screening approach, four potential hit compounds were identified. The bioassay showed that all hits effectively inhibited 3CLpro with IC50 values less than 1 μM. Particularly, hit one showed highly potent 3CLpro inhibition activity at nanomolar concentration. These promising results illustrated that hit one could be a candidate prototype worth exploring further for the treatment of COVID-19.
Data availability statement
The original contributions presented in the study are included in the article/Supplementary Material, further inquiries can be directed to the corresponding authors.
Author contributions
HW, and XZ contributed to conception and design of the study. JW, YY, HL, and SW performed the laboratory experiments. HW, XZ, and SW wrote the first draft of the manuscript. JW, YY, XD, and CZ wrote sections of the manuscript. All authors contributed to manuscript revision, read, and approved the submitted version.
Funding
This research was funded by Chongqing science technology commission of China (2022WSJK046).
Conflict of interest
The authors declare that the research was conducted in the absence of any commercial or financial relationships that could be construed as a potential conflict of interest.
Publisher’s note
All claims expressed in this article are solely those of the authors and do not necessarily represent those of their affiliated organizations, or those of the publisher, the editors and the reviewers. Any product that may be evaluated in this article, or claim that may be made by its manufacturer, is not guaranteed or endorsed by the publisher.
Supplementary material
The Supplementary Material for this article can be found online at: https://www.frontiersin.org/articles/10.3389/fphar.2022.1036208/full#supplementary-material
References
Abdelnabi, R., Foo, C. S., Jochmans, D., Vangeel, L., De Jonghe, S., Augustijns, P., et al. (2022). The oral protease inhibitor (PF-07321332) protects Syrian hamsters against infection with SARS-CoV-2 variants of concern. Nat. Commun. 13, 719. doi:10.1038/s41467-022-28354-0
Adegbola, P. I., Semire, B., Fadahunsi, O. S., and Adegoke, A. E. (2021). Molecular docking and ADMET studies of Allium cepa, Azadirachta indica and Xylopia aethiopica isolates as potential anti-viral drugs for Covid-19. Virusdisease 32, 85–97. doi:10.1007/s13337-021-00682-7
Boras, B., Jones, R. M., Anson, B. J., Arenson, D., Aschenbrenner, L., Bakowski, M. A., et al. (2021). Preclinical characterization of an intravenous coronavirus 3CL protease inhibitor for the potential treatment of COVID19. Nat. Commun. 12, 6055. doi:10.1038/s41467-021-26239-2
Chakraborty, C., Bhattacharya, M., and Sharma, A. R. (2022). Emerging mutations in the SARS-CoV-2 variants and their role in antibody escape to small molecule-based therapeutic resistance. Curr. Opin. Pharmacol. 62, 64–73. doi:10.1016/j.coph.2021.11.006
Ferreira, J. C., Fadl, S., Ilter, M., Pekel, H., Rezgui, R., Sensoy, O., et al. (2021). Dimethyl sulfoxide reduces the stability but enhances catalytic activity of the main SARS-CoV-2 protease 3CLpro. Faseb J. 35, e21774. doi:10.1096/fj.202100994
Gao, Q., Yang, L., and Zhu, Y. (2010). Pharmacophore based drug design approach as a practical process in drug discovery. Curr. Comput. Aided. Drug Des. 6, 37–49. doi:10.2174/157340910790980151
Guan, W. J., Ni, Z. Y., Hu, Y., Liang, W. H., Ou, C. Q., He, J. X., et al. (2020). Clinical characteristics of coronavirus disease 2019 in China. N. Engl. J. Med. 382, 1708–1720. doi:10.1056/NEJMoa2002032
Han, S. H., Goins, C. M., Arya, T., Shin, W. J., Maw, J., Hooper, A., et al. (2022). Structure-based optimization of ml300-derived, noncovalent inhibitors targeting the severe acute respiratory syndrome coronavirus 3CL protease (SARS-CoV-2-3CL(pro)). J. Med. Chem. 65, 2880–2904. doi:10.1021/acs.jmedchem.1c00598
Hang, S., Paik, D., Yao, L., Kim, E., Trinath, J., Lu, J., et al. (2019). Bile acid metabolites control TH17 and Treg cell differentiation. Nature 576, 143–148. doi:10.1038/s41586-019-1785-z
Hu, Q., Xiong, Y., Zhu, G. H., Zhang, Y. N., Zhang, Y. W., Huang, P., et al. (2022). The SARS-CoV-2 main protease (M(pro)): Structure, function, and emerging therapies for COVID-19. MedComm 3, e151. doi:10.1002/mco2.151
Jin, Z., Du, X., Xu, Y., Deng, Y., Liu, M., Zhao, Y., et al. (2020). Structure of M(pro) from SARS-CoV-2 and discovery of its inhibitors. Nature 582, 289–293. doi:10.1038/s41586-020-2223-y
Kitchen, D. B., Decornez, H., Furr, J. R., and Bajorath, J. (2004). Docking and scoring in virtual screening for drug discovery: Methods and applications. Nat. Rev. Drug Discov. 3, 935–949. doi:10.1038/nrd1549
Koutsakos, M., and Kedzierska, K. (2020). A race to determine what drives COVID-19 severity. Nature 583, 366–368. doi:10.1038/d41586-020-01915-3
Maga, G., Falchi, F., Garbelli, A., Belfiore, A., Witvrouw, M., Manetti, F., et al. (2008). Pharmacophore modeling and molecular docking led to the discovery of inhibitors of human immunodeficiency virus-1 replication targeting the human cellular aspartic acid-glutamic acid-alanine-aspartic acid box polypeptide 3. J. Med. Chem. 51, 6635–6638. doi:10.1021/jm8008844
Mysinger, M. M., Carchia, M., Irwin, J. J., and Shoichet, B. K. (2012). Directory of useful decoys, enhanced (DUD-E): Better ligands and decoys for better benchmarking. J. Med. Chem. 55, 6582–6594. doi:10.1021/jm300687e
Narayanan, A., Narwal, M., Majowicz, S. A., Varricchio, C., Toner, S. A., Ballatore, C., et al. (2022). Identification of SARS-CoV-2 inhibitors targeting Mpro and PLpro using in-cell-protease assay. Commun. Biol. 5, 169. doi:10.1038/s42003-022-03090-9
Nassau, D. E., Best, J. C., Kresch, E., Gonzalez, D. C., Khodamoradi, K., and Ramasamy, R. (2022). Impact of the SARS-CoV-2 virus on male reproductive health. BJU Int. 129, 143–150. doi:10.1111/bju.15573
Rockett, R., Basile, K., Maddocks, S., Fong, W., Agius, J. E., Johnson-Mackinnon, J., et al. (2022). Resistance mutations in SARS-CoV-2 delta variant after sotrovimab use. N. Engl. J. Med. 386, 1477–1479. doi:10.1056/NEJMc2120219
Stevens, L. J., Pruijssers, A. J., Lee, H. W., Gordon, C. J., Tchesnokov, E. P., Gribble, J., et al. (2022). Mutations in the SARS-CoV-2 RNA-dependent RNA polymerase confer resistance to remdesivir by distinct mechanisms. Sci. Transl. Med. 14, eabo0718. doi:10.1126/scitranslmed.abo0718
Tao, K., Tzou, P. L., Nouhin, J., Gupta, R. K., de Oliveira, T., Kosakovsky Pond, S. L., et al. (2021). The biological and clinical significance of emerging SARS-CoV-2 variants. Nat. Rev. Genet. 22, 757–773. doi:10.1038/s41576-021-00408-x
Tian, X., Zhao, Q., Chen, X., Peng, Z., Tan, X., Wang, Q., et al. (2022). Discovery of novel and highly potent inhibitors of SARS CoV-2 papain-like protease through structure-based pharmacophore modeling, virtual screening, molecular docking, molecular dynamics simulations, and biological evaluation. Front. Pharmacol. 13, 817715. doi:10.3389/fphar.2022.817715
Tyndall, J. D. A. S (2022). S-217622, a 3CL protease inhibitor and clinical candidate for SARS-CoV-2. J. Med. Chem. 65, 6496–6498. doi:10.1021/acs.jmedchem.2c00624
Vuong, W., Khan, M. B., Fischer, C., Arutyunova, E., Lamer, T., Shields, J., et al. (2020). Feline coronavirus drug inhibits the main protease of SARS-CoV-2 and blocks virus replication. Nat. Commun. 11, 4282. doi:10.1038/s41467-020-18096-2
Wang, J., Kang, X., Kuntz, I. D., and Kollman, P. A. (2005). Hierarchical database screenings for HIV-1 reverse transcriptase using a pharmacophore model, rigid docking, solvation docking, and MM-PB/SA. J. Med. Chem. 48, 2432–2444. doi:10.1021/jm049606e
Wieder, M., Garon, A., Perricone, U., Boresch, S., Seidel, T., Almerico, A. M., et al. (2017). Common hits approach: Combining pharmacophore modeling and molecular dynamics simulations. J. Chem. Inf. Model. 57, 365–385. doi:10.1021/acs.jcim.6b00674
Xia, B., and Kang, X. (2011). Activation and maturation of SARS-CoV main protease. Protein Cell 2, 282–290. doi:10.1007/s13238-011-1034-1
Zehra, Z., Luthra, M., Siddiqui, S. M., Shamsi, A., Gaur, N. A., and Islam, A. (2020). Corona virus versus existence of human on the Earth: A computational and biophysical approach. Int. J. Biol. Macromol. 161, 271–281. doi:10.1016/j.ijbiomac.2020.06.007
Zheng, L., Ren, R., Sun, X., Zou, Y., Shi, Y., Di, B., et al. (2021). Discovery of a dual tubulin and poly(ADP-ribose) polymerase-1 inhibitor by structure-based pharmacophore modeling, virtual screening, molecular docking, and biological evaluation. J. Med. Chem. 64, 15702–15715. doi:10.1021/acs.jmedchem.1c00932
Zhou, Y., Chen, Y., Tan, Y., Hu, R., and Niu, M. M. (2021). An NRP1/MDM2-targeted D-peptide supramolecular nanomedicine for high-efficacy and low-toxic liver cancer therapy. Adv. Healthc. Mat. 10, e2002197. doi:10.1002/adhm.202002197
Zhou, Y., Di, B., and Niu, M. M. (2019). Structure-based pharmacophore design and virtual screening for novel tubulin inhibitors with potential anticancer activity. Molecules 24, E3181. doi:10.3390/molecules24173181
Zhou, Y., Tang, S., Chen, T., and Niu, M. M. (2019). Structure-based pharmacophore modeling, virtual screening, molecular docking and biological evaluation for identification of potential poly (ADP-Ribose) polymerase-1 (PARP-1) inhibitors. Molecules 24, E4258. doi:10.3390/molecules24234258
Keywords: SARS CoV-2, main protease, virtual screening, molecular dynamics simulation, inhibitor
Citation: Wang H, Wen J, Yang Y, Liu H, Wang S, Ding X, Zhou C and Zhang X (2022) Identification of highly effective inhibitors against SARS-CoV-2 main protease: From virtual screening to in vitro study. Front. Pharmacol. 13:1036208. doi: 10.3389/fphar.2022.1036208
Received: 04 September 2022; Accepted: 07 November 2022;
Published: 18 November 2022.
Edited by:
Mariusz Skwarczynski, The University of Queensland, AustraliaReviewed by:
Darren Fayne, Trinity College Dublin, IrelandPaola Storici, Elettra Sincrotrone Trieste, Italy
Copyright © 2022 Wang, Wen, Yang, Liu, Wang, Ding, Zhou and Zhang. This is an open-access article distributed under the terms of the Creative Commons Attribution License (CC BY). The use, distribution or reproduction in other forums is permitted, provided the original author(s) and the copyright owner(s) are credited and that the original publication in this journal is cited, in accordance with accepted academic practice. No use, distribution or reproduction is permitted which does not comply with these terms.
*Correspondence: Chunqiao Zhou, zcqiao163@163.com; Xuelin Zhang, zhangxuelin719@163.com
†These authors have contributed equally to this work