- 1Department of Pharmacy, The Third Xiangya Hospital, Central South University, Changsha, China
- 2Xiangya School of Pharmaceutical Sciences, Central South University, Changsha, China
- 3Department of Hematology, The Third Xiangya Hospital, Central South University, Changsha, China
- 4Center of Clinical Pharmacology, The Third Xiangya Hospital, Central South University, Changsha, China
- 5Department of Pharmacy, Affiliated Hospital of Guilin Medical University, Guilin, China
Population pharmacokinetic (PopPK) models of posaconazole have been established to promote the precision dosing. However, the performance of these models extrapolated to other centers has not been evaluated. This study aimed to conduct an external evaluation of published posaconazole PopPK models to evaluate their predictive performance. Posaconazole PopPK models screened from the PubMed and MEDLINE databases were evaluated using an external dataset of 213 trough concentration samples collected from 97 patients. Their predictive performance was evaluated by prediction-based diagnosis (prediction error), simulation-based diagnosis (visual predictive check), and Bayesian forecasting. In addition, external cohorts with and without proton pump inhibitor were used to evaluate the models respectively. Ten models suitable for the external dataset were finally included into the study. In prediction-based diagnostics, none of the models met pre-determined criteria for predictive indexes. Only M4, M6, and M10 demonstrated favorable simulations in visual predictive check. The prediction performance of M5, M7, M8, and M9 evaluated using the cohort without proton pump inhibitor showed a significant improvement compared to that evaluated using the whole cohort. Consistent with our expectations, Bayesian forecasting significantly improved the predictive per-formance of the models with two or three prior observations. In general, the applicability of these published posaconazole PopPK models extrapolated to our center was unsatisfactory. Prospective studies combined with therapeutic drug monitoring are needed to establish a PopPK model for posaconazole in the Chinese population to promote individualized dosing.
1 Introduction
Posaconazole is commonly used for the prophylaxis or treatment of invasive fungal infections by inhibiting ergosterol biosynthesis in fungal cell membranes (US FDA, 2022; Wu et al., 2022), especially in patients such as acute myelogenous leukemia (AML), myelodysplastic syndrome (MDS), allogeneic hematopoietic stem cell transplantation, and other immunocompromised populations (Busca et al., 2021; Oh et al., 2021; Panagopoulou and Roilides, 2022). Posaconazole prophylaxis has the potential to reduce all-cause mortality and the incidence of adverse events in the aforementioned populations (Wang et al., 2020; Wong et al., 2020; Zeng et al., 2021).
Oral suspensions, delayed-release tablets, and injections are the three currently available formulations of posaconazole. Posaconazole oral suspension was approved by the U.S. Food and Drug Administration in 2006 for the prophylaxis of Aspergillus and Candida infections (US FDA, 2022). The effect of multiple factors on plasma exposure results in large inter-individual variability in posaconazole oral suspensions; however, posaconazole delayed-release tablets, approved in 2013, have improved the pharmacokinetics of posaconazole (Boglione-Kerrien et al., 2018; Gautier-Veyret et al., 2019; Sustained-Release, 2022). Nevertheless, it has been reported that high body weight, diarrhea, mucositis, hypoproteinemia, and concomitant use of proton pump inhibitors (PPIs), H2 receptor antagonists, and metoclopramide may lead to subtherapeutic concentrations of posaconazole oral suspensions and delayed-release tablets (Tang et al., 2017; Cojutti et al., 2018; Suh et al., 2018; Lai et al., 2020; Oh et al., 2020). Conversely, the intake of high-fat foods, nutritional supplements, and acidic beverages can facilitate the absorption of posaconazole oral suspension (Krishna et al., 2009). Intravenous formulation of posaconazole was approved in 2014, providing a new route of administration for patients with dysphagia and those in a coma (US FDA, 2022).
Posaconazole pharmacokinetics may be altered by inter- or intra-individual variability, formulations of posaconazole, and drug-drug interactions. Therapeutic drug monitoring (TDM) ensures optimal exposure to avoid breakthrough fungal infections or antifungal treatment failures resulting from low concentrations (Dolton et al., 2012; John et al., 2019). In addition, TDM is often combined with a population pharmacokinetic (PopPK) approach as a means of personalized medicine. PopPK, a mathematical modeling approach, is used to quantitatively assess the pharmacokinetic characteristics of absorption, distribution, metabolism, and excretion of an agent, as well as to explain inter-individual variability (Guidi et al., 2022). The validity and credibility of PopPK models can be evaluated with internal or external test datasets. Compared with internal evaluation, external evaluation is a rigorous and critical approach to examining the reproducibility and extrapolability of PopPK models for different patient populations and different scenarios (Siontis et al., 2015; Cheng et al., 2021; Ramspek et al., 2021).
In the last decade, PopPK models have been developed for posaconazole, incorporating multiple covariates to account for the variability in posaconazole exposure. However, external evaluation was often not performed for these models during their development. Thus, their predictive performance and applicability to other centers remain unexamined. Therefore, we conducted this study with the aim of evaluating published posaconazole PopPK models using an independent external dataset.
2 Materials and methods
2.1 Retrieval and screening of posaconazole PopPK models
Literature on posaconazole PopPK studies was searched in PubMed and MEDLINE databases until May 2022 using the following keywords: “posaconazole,” “population pharmacokinetic” and “nonlinear mixed effect model.” The reference lists of the selected publications were also inspected for a comprehensive search of published models.
Studies meeting the following criteria were included: 1) those conducted in humans; 2) those with posaconazole as the primary research drug; 3) those describing PopPK modeling of posaconazole. Literature with one of the following conditions was excluded: 1) review or external evaluation studies; 2) studies in which the available information was insufficient to reproduce the model; 3) studies including free and total plasma concentrations of posaconazole; 4) studies with non-compartment or non-parametric modeling methods.
2.2 External evaluation dataset
The retrospective external evaluation cohort consisted of patients who received posaconazole oral suspension at the Hematology Department of Third Xiangya Hospital of Central South University from 2019 to 2021 and had undergone TDM at least once. The dosage of posaconazole, ranging from 300 to 1,200 mg daily, was determined by a competent physician. Blood samples were collected intravenously before administration under the steady-state. Liquid chromatography-tandem mass spectrometry (LC-MS/MS) was used for the determination of posaconazole plasma concentrations with a linear range of 0.08–10 mg/L and an accuracy of 93.5%–106.04%. The following demographic and clinical information was collected from the electronic medical record system: age, sex, weight, gamma-glutamyl transferase (GGT), total protein, total bilirubin, albumin, and treatment information (disease diagnosis, purpose of medication, formulation, dosage, time of administration and sampling). In the absence of patient height data, body surface area (BSA) was estimated by Livingston et al.’s formula (Livingston and Lee, 2001): BSA (m2) = 0.1173 × weight (kg)0.6466. To explore the influence of the characteristics of the external cohort on the pharmacokinetics of posaconazole, we performed a statistical analysis of the correlation between sex, age, weight, diarrhea, PPI, and chemotherapy and plasma concentrations. Categorical variables (PPI, diarrhea, chemotherapy, sex) were presented as box plots, and continuous variables (age, body weight) were presented as scatter plots. This study was approved by the Ethics Committee of the Third Xiangya Hospital of Central South University. These data have not been used in the development of any models.
2.3 External evaluation of predictive performance of PopPK models
Parameter formulas and values were obtained from the published posaconazole PopPK models. Nonlinear mixed effect modeling (NONMEM version 7.5) software, assisted by Perl-Speaks-NONMEM (PsN, version 4.7.0; uupharmacometrics.github.io/PsN) and Pirana (version 2.9.2; www.pirana-software.com), was used for external evaluations, and R package (Version 4.1.2; http://www.r-project.org) was used for analysis and graphical processing of NONMEM output results.
2.3.1 Prediction-based diagnosis
In prediction-based diagnostics, goodness-of-fit plots characterizing the fitness of observations versus population predictions and observations versus individual predictions were constructed to assess the predictive performance of the models. Prediction error (PE) was calculated with Eq. 1:
where PRED is the concentration predicted using the final model and parameters in the literature, OBS is the concentration observed in the external evaluation data set.
PE is a classical prediction index of model accuracy and is presented as a boxplot in this study. Median prediction error (MPE) was used to examine the overall bias of models, with MPE >0 and MPE <0 indicating the tendency of the model to overestimate and underestimate the predicted value, respectively. The median absolute prediction error (MAPE) was used to characterize the precision of the models. Composite metrics F20 and F30, the percentage of PE between ±20% and ±30%, simultaneously characterize the accuracy and precision of model predictions. Models with MPE ≤ ±20%, MAPE ≤30%, F20 ≥ 35%, and F30 ≥ 50% are considered acceptable (Cai et al., 2020; Li et al., 2021).
2.3.2 Simulation-based diagnosis
The concordance between simulations and observations was demonstrated by a visual predictive check (VPC). Based on the observed data and the published model, 1,000 simulations were executed in NONMEM to generate the simulation dataset. The 95% confidence intervals were calculated for the 5th percentile, median, and 95th percentile of the simulated concentrations and compared to the percentile of the corresponding observed concentrations. The distribution characteristics of the observed and simulated data have been displayed as VPC plots using R software.
2.3.3 Bayesian forecasting
Maximum a posteriori Bayesian estimation (MAPB) was performed to assess the impact of prior observations on the predictability of the model using 0, 1, 2, and 3 previous observations to predict patients with ≥1 observation. In the NONMEM control file, $POSTHOC item ' MAXEVALS ′ was set to 0. R software was used to process the output results and generate the graphics. Individual prediction for the last observation of all subjects was predicted by the latest 1, 2, and 3 prior observations, respectively. The individual prediction error (IPE) and absolute individual prediction error (AIPE) were calculated according to the individual predicted concentrations (IPRED) and observed concentrations. The formulae are shown as Eqs 2, 3 below:
Median individual prediction error (MIPE) and median absolute individual prediction error (MAIPE) were used to evaluate the accuracy and precision of Bayesian forecasting, respectively. IF20 and IF30, which represented F20 and F30 of IPE, were also calculated (Zhao et al., 2016; Hanafin et al., 2021). MIPE% ≤ ± 20%, MAIPE% ≤ 30%, IF20 ≥ 35% and IF30 ≥ 50% were used to assess the predictive performance of the model when adding the prior concentrations.
3 Results
3.1 Review of published PopPK models of posaconazole
After retrieval and screening, 10 PopPK models of posaconazole (Abutarif et al., 2010; Störzinger et al., 2012; Vehreschild et al., 2012; Dolton et al., 2014; Petitcollin et al., 2017; Boonsathorn et al., 2019; Wasmann et al., 2020; Bentley et al., 2021; Elkayal et al., 2021; Peña-Lorenzo et al., 2022) published from 2010 to 2021 were finally included in our study. There were six single-center studies (Störzinger et al., 2012; Vehreschild et al., 2012; Petitcollin et al., 2017; Boonsathorn et al., 2019; Bentley et al., 2021; Peña-Lorenzo et al., 2022) and four multi-center studies (Abutarif et al., 2010; Dolton et al., 2014; Wasmann et al., 2020; Elkayal et al., 2021). In these studies, six were adult healthy volunteers or patients (Störzinger et al., 2012; Vehreschild et al., 2012; Dolton et al., 2014; Petitcollin et al., 2017; Wasmann et al., 2020; Peña-Lorenzo et al., 2022), three were pediatric patients (Boonsathorn et al., 2019; Bentley et al., 2021; Elkayal et al., 2021), and one included both adults and pediatric patients (Abutarif et al., 2010). The majority of the population included in the studies were patients with neutropenic hematologic diseases such as AML, MDS, and stem cell transplantation. Three studies were performed with a large sample size of over 100 subjects (Abutarif et al., 2010; Dolton et al., 2014; Boonsathorn et al., 2019). Except for one study (Wasmann et al., 2020) involving intravenous administration of posaconazole, patients in the other studies were administered posaconazole via the gastrointestinal tract in the form of oral suspensions or delayed-release tablets. Almost all plasma samples of these studies were determined with liquid chromatography or LC-MS/MS. Half of the studies (Abutarif et al., 2010; Vehreschild et al., 2012; Dolton et al., 2014; Boonsathorn et al., 2019; Elkayal et al., 2021) incorporated diarrhea and PPI into their final models, and three studies (Vehreschild et al., 2012; Boonsathorn et al., 2019; Wasmann et al., 2020) incorporated body weight. Few studies retained sex, race, total protein, bilirubin, GGT, mucositis, and concomitant medications such as chemotherapy, phenytoin, rifampin, fosamprenavir, nutritional supplements, and metoclopramide in the final model. The characteristics of the published models were summarized in Table 1.
3.2 External evaluation data set
After removing the 4.48% (10/223) of samples with concentration below limit of quantification according to the method of Irby et al. (2021), 213 trough concentrations at the steady-state of posaconazole from 97 (58 males and 39 females) patients were finally retained. The median (range) of posaconazole concentration was 0.77 (0.08–2.76) mg/L. Concomitant medications of PPI and chemotherapy agents were administrated in 74% and 38% of patients during the treatment with posaconazole, respectively. Only a few patients received a combination of nutritional supplements, phenytoin, and rifampicin. In addition, 12% of patients developed diarrhea during treatment. Detailed demographic information and clinical data were summarized in Table 2.
Statistical analysis showed significant differences in the plasma concentrations of posaconazole in subjects with and without PPI (p = 0.0013), as well as diarrhea (p = 0.0044). There was a weak positive correlation between body weight and posaconazole concentrations (R = 0.15, p = 0.029). The box plot and scatter plot results were shown in the Supplementary Figure S1. Therefore, we divided the evaluation dataset into two parts by subjects with or without PPI during posaconazole treatment. Due to changes in PPI use during treatment, some patients were double counted (n = 11) and some samples were removed (N = 16). Finally, 139 plasma samples from 71 patients were assigned to the external cohort with PPI (cohort 1), and 58 plasma concentrations from 37 patients were assigned to the external cohort without PPI (cohort 2). The whole cohort was used for the evaluation of all models included, and cohort 1 and cohort 2 were used for the evaluation of models that included PPI and models that did not include PPI, respectively. The models were not evaluated in groups by diarrhea because the number of patients with diarrhea in the external data was only 12.4%.
3.3 Results of external evaluation
3.3.1 Prediction-based diagnosis
The goodness-of-fit test results for the prediction error diagnostics using the entire cohort, cohort 1 and cohort 2, were presented in Supplementary Figures S2–S9. The box plots of the prediction errors were shown in Figure 1. In terms of accuracy and precision, M4, M6, and M10 were superior to other models. Regardless of which cohort was used for evaluation, no model had a median prediction error within an acceptable range of ±30%. In addition, MPE, MAPE, F20, and F30 for the 10 models were not within the acceptable range according to the predetermined criteria (Supplementary Table S1). We observed that M5, M7, M8 and M9 had a higher median prediction error and a wider distribution than the other models. Compared to being evaluated with the whole cohort, prediction errors were significantly lower for M5, M7, M8, and M9 when evaluated with cohort 2. No noticeable changes in diagnostic outcomes were observed for the other models.
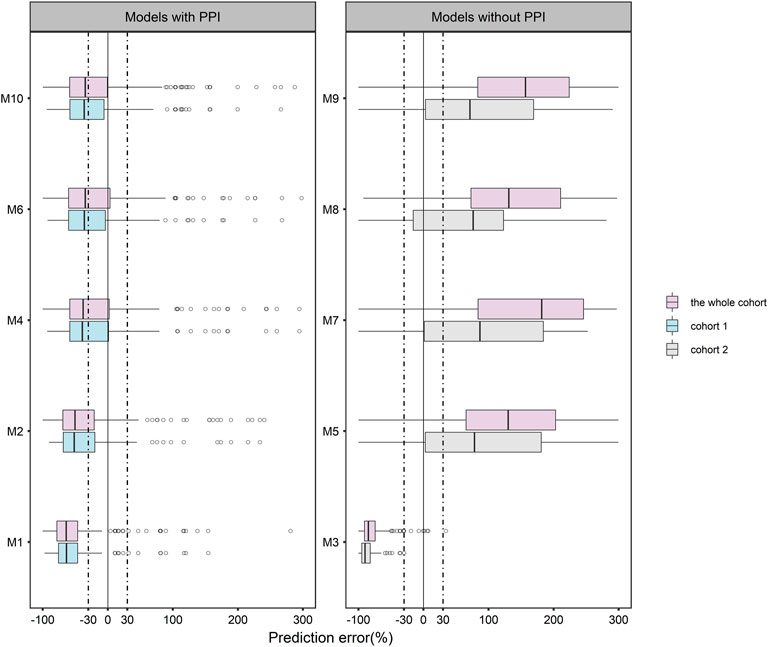
FIGURE 1. Boxplots of prediction error (PE) for 10 published population pharmacokinetic models of posaconazole.
3.3.2 Simulation-based diagnosis
The VPC results of each model were shown in Figure 2. The 5th, 50th, and 95th percentiles of most of the observed data of M4 (Dolton et al., 2014), M6 (Boonsathorn et al., 2019), and M10 (Elkayal et al., 2021) fall within the 95% prediction interval of the corresponding percentile of the simulated data. In addition, M2 (Vehreschild et al., 2012) and M9 (Bentley et al., 2021) suggest that the observed data had similar distribution characteristics to the simulated data near the 5th percentile. However, none of the other models fit well. The VPC results evaluated by PPI grouping were shown in the Supplementary Figure S10.
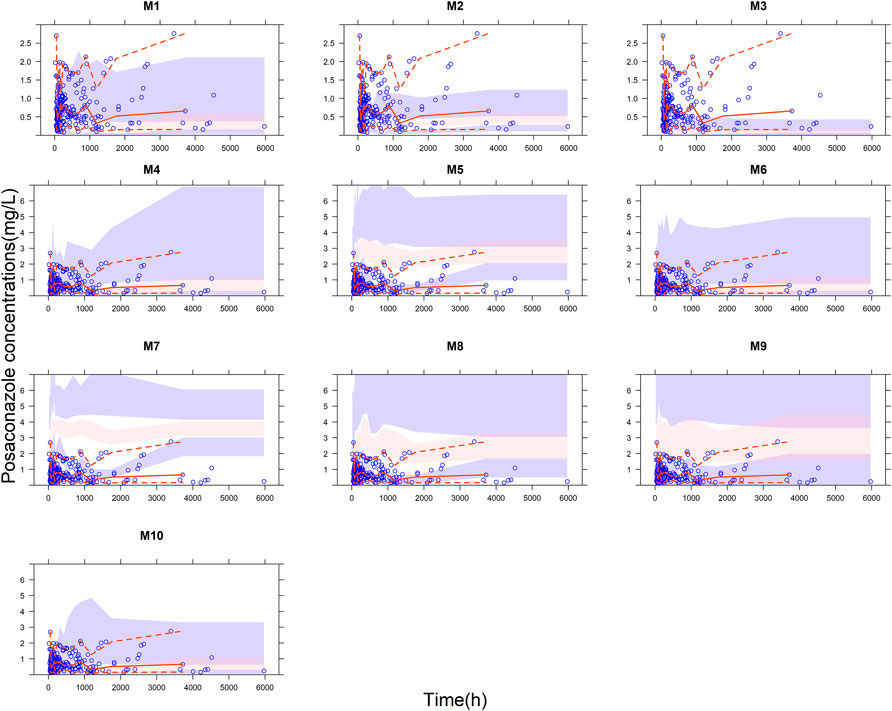
FIGURE 2. Visual predictive check (VPC) plots for the published model of posaconazole evaluated with the whole cohort. Blue points represent the observations, and red lines represent the 5th, 50th and 95th percentiles of the observed data. The color-shaded areas represent the 95% confidence intervals around the simulated 5th, 50th, and 95th percentiles.
3.3.3 Bayesian forecasting
The relationship between IPE and the number of prior samples was shown in Figure 3. The results indicated that prior exposure can significantly reduce the prediction error of the models. Overall, the individual predictive performance of the models was optimal with two or three prior collections. With the addition of three prior concentrations, M1 (Abutarif et al., 2010), M2 (Vehreschild et al., 2012), M3 (Störzinger et al., 2012), M5 (Petitcollin et al., 2017), M8 (Peña-Lorenzo et al., 2022), and M10 (Elkayal et al., 2021) showed good accuracy and precision, meeting MIPE < ±20%, MAIPE <30%, IF20 > 35%, and IF30 > 50%. Especially in M3 (Störzinger et al., 2012) and M5 (Petitcollin et al., 2017), IF30 exceeded 70%. M3 (Störzinger et al., 2012) and M10 (Elkayal et al., 2021) achieved high predictive performance even with only one prior sample.
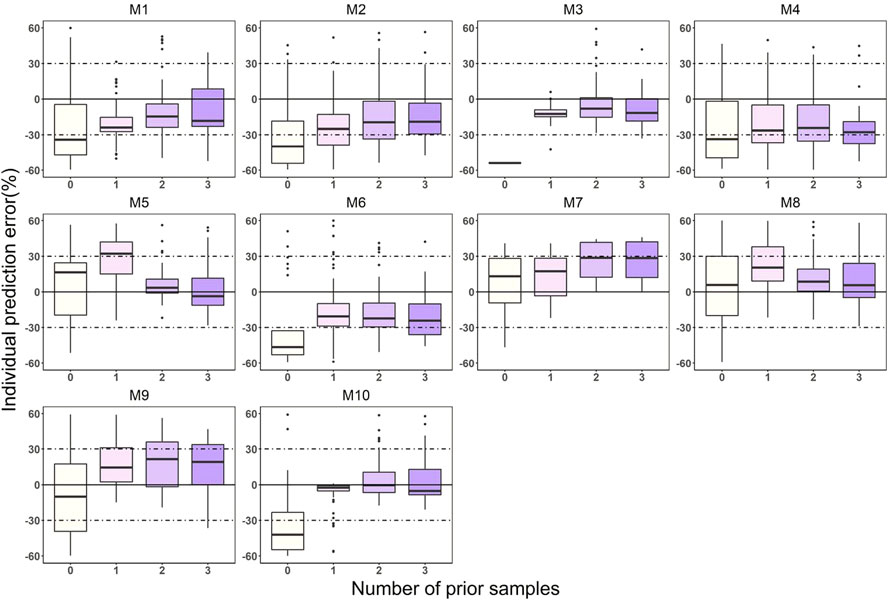
FIGURE 3. Box plots of individual prediction error (IPE) with Bayesian forecasting for 10 published PopPK models of posaconazole in different occasions. 0 represents predictions without prior information and 1-3 represents with prior one to three observations, respectively.
4 Discussion
PopPK models for posaconazole have been developed in some centers, although their applicability to other scenarios is unclear. This study provided the first external evaluation of ten published posaconazole PopPK models (Abutarif et al., 2010; Störzinger et al., 2012; Vehreschild et al., 2012; Dolton et al., 2014; Petitcollin et al., 2017; Boonsathorn et al., 2019; Wasmann et al., 2020; Bentley et al., 2021; Elkayal et al., 2021; Peña-Lorenzo et al., 2022) with an independent dataset to examine the clinical applicability of the models to our center and the heterogeneity among these models.
M4 (Dolton et al., 2014) presented a better predictive performance in the studies of adults than other models. Similar to M7 (Wasmann et al., 2020), M4 (Dolton et al., 2014) included both healthy volunteers and patients with various pathological states into the modeling population. Unlike other models, M4 (Dolton et al., 2014) retained most of the covariates examined in the final model under strict screening criteria, including phenytoin and rifampicin—inducers of the UDP - glucuronosyltransferases that mainly mediates the metabolism of posaconazole to posaconazole-glucuronide (Rae et al., 2001; Anderson, 2004; Ghosal et al., 2004).
For models developed with pediatric patients, both in prediction-based and simulation-based diagnostics, M6 (Boonsathorn et al., 2019) and M10 (Elkayal et al., 2021) were superior to M9 (Bentley et al., 2021), despite the PE being still slightly above 30%. Although only 16% (34/213) of the pediatric concentrations were included in the external evaluation dataset, we attempted to evaluate the models developed in the pediatric population to obtain a comparison between the adult and pediatric models. Studies have reported that rapid pharmacokinetic maturation tends to occur in the early stages from neonatal to infant (Rhodin et al., 2009; Germovsek et al., 2017; Salem et al., 2021). M6 (Boonsathorn et al., 2019) covers the population aged 0.5–18 years; however, the evaluation dataset lacks concentration data for children under 12 years of age, which may have an impact on the reliability of the evaluation results. M10 (Elkayal et al., 2021), a model developed with only 14 subjects, had large inter-individual variability (87.8%) in the estimated clearance, which may account for the poor predictive performance of the model. In addition, the dosing of M6 (Boonsathorn et al., 2019) and M10 (Elkayal et al., 2021) were based on BSA. However, the BSA for the evaluation data was estimated according to the formula provided in the literature. We have not been able to clarify the differences in the evaluation results caused by the different ways of obtaining BSA. M9 (Bentley et al., 2021) was established in patients with pulmonary fibrosis, while the evaluation population consisted mainly of patients with hematological diseases. Nevertheless, due to the limited number of published posaconazole PopPK models, we retained the models established in diverse populations. The difference in the pathological state between the modeling and the evaluation data may affect the evaluation of this model.
We observed that several models using tablets or intravenous preparations (M5, M7, M8, M9) (Petitcollin et al., 2017; Wasmann et al., 2020; Bentley et al., 2021; Peña-Lorenzo et al., 2022) showed a trend of overestimation and wide distribution of evaluation results compared to the models using oral suspensions. This may be related to the fact that the bioavailability of posaconazole varies considerably between formulations. The delayed-release tablets of posaconazole are less likely to recrystallize in the intestine due to the formulation improvements and are less affected by food, drugs that alter gastric pH and gastric motility, and therefore have a higher exposure compared to oral suspensions (Wiederhold, 2016; Van Daele et al., 2020). Since the population in the external dataset used oral suspensions, the accuracy and precision of the models for tablet and intravenous administration were not quite satisfactory.
In the external cohort, 74% (72/97) of patients were treated with concomitant PPI. Half of the models we evaluated included PPI as a covariate that could reduce posaconazole exposure. In the external cohort, plasma concentrations were significantly lower in patients with PPI than in those without PPI (p = 0.0013). The effect of concomitant PPI in the external cohort on the evaluation results of the models deserves to be considered. Therefore, we grouped the evaluation datasets according to the presence or absence of PPI. Compared to using the entire cohort, the results of the models evaluated with cohort 1 did not show significant differences, while the predictive performance of the models evaluated with cohort 2 showed a greater improvement. In particular, model improvements were more evident in the models using tablets and intravenous formulations (M5, M7, M8, M9), while little change in predictive performance could be observed in the models using oral suspensions. The predictions of the models that did not include PPI showed a higher trend when evaluated using the whole cohort. Furthermore, the number of patients with PPI was almost twice that of patients without PPI, which increased the impact of the evaluation cohort on the predictive performance of the models. This effect is mitigated when using cohort 1, so the prediction performance of the model is improved.
Except for the five models (Abutarif et al., 2010; Vehreschild et al., 2012; Dolton et al., 2014; Boonsathorn et al., 2019; Elkayal et al., 2021) that retained diarrhea and PPI, the other covariates differed considerably among models. As shown in the goodness-of-fit plots (Supplementary Figures S2–S5), the individual predicted values of some models were more consistent with the observed data than the population predicted values, which suggested that some potential covariates need to be further explored based on the structural model.
Based on the results of Bayesian forecasting, we can conclude that prior concentration can significantly improve the predictive performance of the model, which means that although these models are limited in the application of initial dose recommendation, they can play an important role in subsequent dose adjustment as long as two or three prior concentrations have been measured.
Nevertheless, the limitations of this study should be considered. First, the modeling population was mainly Caucasian, while the evaluation data were from Chinese patients. As reported by M1 (Abutarif et al., 2010), race may have an effect on posaconazole exposure, thus interfering with the veracity of the evaluation. Second, we must recognize that the variations between evaluation data and modeling data, including basic information on disease status, formulation, route of administration, concomitant medication and prandial state, to some extent, explained the results of unsatisfactory model evaluation. In addition, due to the limited data for the pediatric population in the evaluation dataset, the evaluation of models built with the pediatric patients may be less reliable.
5 Conclusion
External evaluation revealed that the currently published PopPK model has poor predictive performance for posaconazole concentrations and insufficient extrapolation to our center. Hence, it is necessary to establish a PopPK model of posaconazole for the Chinese population and to combine it with TDM for the recommendation and optimization of dosing regimens.
Data availability statement
The raw data supporting the conclusion of this article will be made available by the authors, without undue reservation.
Ethics statement
The studies involving human participants were reviewed and approved by the Ethics Committee of the Third Xiangya Hospital of Central South University. Written informed consent from the participants’ legal guardian/next of kin was not required to participate in this study in accordance with the national legislation and the institutional requirements.
Author contributions
QP and CW contributed to the conception and design of the study. NY and QD developed the methodology. QC and WL collected and organized the data. SH and ZS conducted the data anlalysis. SH and QD wrote the first draft of the manuscript. XC and YL performed the layout and editing of figures, tables, and text. QP received financial support for the project. All authors contributed to manuscript revision, read and approved the submitted version.
Funding
This research was funded by the Scientific Foundation of Hunan (grant no. 2022JJ30899), the Health Department Foundation of Hunan Province (grant no. 20201656), and the Changsha Municipal Natural Science Foundation (grant no. kq2014269).
Conflict of interest
The authors declare that the research was conducted in the absence of any commercial or financial relationships that could be construed as a potential conflict of interest.
Publisher’s note
All claims expressed in this article are solely those of the authors and do not necessarily represent those of their affiliated organizations, or those of the publisher, the editors and the reviewers. Any product that may be evaluated in this article, or claim that may be made by its manufacturer, is not guaranteed or endorsed by the publisher.
Supplementary material
The Supplementary Material for this article can be found online at: https://www.frontiersin.org/articles/10.3389/fphar.2022.1005348/full#supplementary-material
Abbreviations
PopPK, population pharmacokinetic; AML, acute myelogenous leukemia; MDS, myelodysplastic syndrome; PPI, proton pump inhibitors; TDM, therapeutic drug monitoring; LC-MS/MS, liquid chromatography-tandem mass spectrometry; GGT, gamma-glutamyl transferase; BSA, body surface area; NONMEM, nonlinear mixed effect modeling; PE, prediction error; PRED, predicted concentration; OBS, observation; MPE, median prediction error; MAPE, median absolute prediction error; F20, the percentage of prediction error falling within 20%; F30, the percentage of prediction error falling within 30%; VPC, visual predictive check; IPE, individual prediction error; AIPE, absolute individual prediction error; IPRED, individual predicted concentration; MIPE, median individual prediction error; MAIPE, median absolute individual prediction error; IF20, the percentage of individual prediction error falling within 20%; IF30, the percentage of individual prediction error falling within 30%.
References
Abutarif, M. A., Krishna, G., and Statkevich, P. (2010). Population pharmacokinetics of posaconazole in neutropenic patients receiving chemotherapy for acute myelogenous leukemia or myelodysplastic syndrome. Curr. Med. Res. Opin. 26 (2), 397–405. doi:10.1185/03007990903485056
Anderson, G. D. (2004). Pharmacogenetics and enzyme induction/inhibition properties of antiepileptic drugs. Neurology 63 (10), S3–S8. doi:10.1212/wnl.63.10_suppl_4.s3
Bentley, S., Davies, J. C., Gastine, S., Donovan, J., and Standing, J. F. (2021). Clinical pharmacokinetics and dose recommendations for posaconazole gastroresistant tablets in children with cystic fibrosis. J. Antimicrob. Chemother. 76 (12), 3247–3254. doi:10.1093/jac/dkab312
Boglione-Kerrien, C., Picard, S., Tron, C., Nimubona, S., Gangneux, J. P., Lalanne, S., et al. (2018). Safety study and therapeutic drug monitoring of the oral tablet formulation of posaconazole in patients with haematological malignancies. J. Cancer Res. Clin. Oncol. 144 (1), 127–134. doi:10.1007/s00432-017-2523-2
Boonsathorn, S., Cheng, I., Kloprogge, F., Alonso, C., Lee, C., Booth, J., et al. (2019). Clinical pharmacokinetics and dose recommendations for posaconazole in infants and children. Clin. Pharmacokinet. 58 (1), 53–61. doi:10.1007/s40262-018-0658-1
Busca, A., Cinatti, N., Gill, J., Passera, R., Dellacasa, C. M., Manetta, S., et al. (2021). Management of invasive fungal infections in patients undergoing allogeneic hematopoietic stem cell transplantation: The turin experience. Front. Cell. Infect. Microbiol. 11, 805514. doi:10.3389/fcimb.2021.805514
Cai, X., Li, R., Sheng, C., Tao, Y., Zhang, Q., Li, J., et al. (2020). Systematic external evaluation of published population pharmacokinetic models for tacrolimus in adult liver transplant recipients. Eur. J. Pharm. Sci. 145, 105237. doi:10.1016/j.ejps.2020.105237
Cheng, Y., Wang, C. Y., Li, Z. R., Pan, Y., Liu, M. B., and Jiao, Z. (2021). Can population pharmacokinetics of antibiotics be extrapolated? Implications of external evaluations. Clin. Pharmacokinet. 60 (1), 53–68. doi:10.1007/s40262-020-00937-4
Cojutti, P. G., Candoni, A., Lazzarotto, D., Rabassi, N., Fanin, R., Pea, F., et al. (2018). Co-administration of proton pump inhibitors and/or of steroids may be a risk factor for low trough concentrations of posaconazole delayed-released tablets in adult patients with haematological malignancies. Br. J. Clin. Pharmacol. 84 (11), 2544–2550. doi:10.1111/bcp.13707
Dolton, M. J., Brüggemann, R. J., Burger, D. M., and Mclachlan, A. J. (2014). Understanding variability in posaconazole exposure using an integrated population pharmacokinetic analysis. Antimicrob. Agents Chemother. 58 (11), 6879–6885. doi:10.1128/AAC.03777-14
Dolton, M. J., Ray, J. E., Chen, S. C., Ng, K., Pont, L., and Mclachlan, A. J. (2012). Multicenter study of posaconazole therapeutic drug monitoring: Exposure-response relationship and factors affecting concentration. Antimicrob. Agents Chemother. 56 (11), 5503–5510. doi:10.1128/AAC.00802-12
Elkayal, O., Spriet, I., Uyttebroeck, A., Colita, A., Annaert, P., Smits, A., et al. (2021). A population pharmacokinetic modeling and simulation study of posaconazole oral suspension in immunocompromised pediatric patients: A short communication. Ther. Drug Monit. 43 (4), 512–518. doi:10.1097/FTD.0000000000000877
Gautier-Veyret, E., Bolcato, L., Roustit, M., Weiss, S., Tonini, J., Cornet, M., et al. (2019). Treatment by posaconazole tablets, compared to posaconazole suspension, does not reduce variability of posaconazole trough concentrations. Antimicrob. Agents Chemother. 63 (10), 004844-19. doi:10.1128/AAC.00484-19
Germovsek, E., Barker, C. I., Sharland, M., and Standing, J. F. (2017). Scaling clearance in paediatric pharmacokinetics: All models are wrong, which are useful? Br. J. Clin. Pharmacol. 83 (4), 777–790. doi:10.1111/bcp.13160
Ghosal, A., Hapangama, N., Yuan, Y., Achanfuo-Yeboah, J., Iannucci, R., Alton, K., et al. (2004). Identification of human UDP-glucuronosyltransferase enzyme(s) responsible for the glucuronidation of posaconazole (Noxafil). Drug Metab. Dispos. 32 (2), 267–271. doi:10.1124/dmd.32.2.267
Guidi, M., Csajka, C., and Buclin, T. (2022). Parametric approaches in population pharmacokinetics. J. Clin. Pharmacol. 62 (2), 125–141. doi:10.1002/jcph.1633
Hanafin, P. O., Nation, R. L., Scheetz, M. H., Zavascki, A. P., Sandri, A. M., Kwa, A. L., et al. (2021). Assessing the predictive performance of population pharmacokinetic models for intravenous polymyxin B in critically ill patients. CPT. Pharmacometrics Syst. Pharmacol. 10 (12), 1525–1537. doi:10.1002/psp4.12720
Intravenous (2022). Orange book: Approved drug products with therapeutic equivalence evaluations. Available at: https://www.accessdata.fda.gov/scripts/cder/ob/results_product.cfm?Appl_Type=N&Appl_No=205596#30433 (accessed 20222 27, 2022).
Irby, D. J., Ibrahim, M. E., Dauki, A. M., Badawi, M. A., Illamola, S. M., Chen, M., et al. (2021). Approaches to handling missing or "problematic" pharmacology data: Pharmacokinetics. CPT. Pharmacometrics Syst. Pharmacol. 10 (4), 291–308. doi:10.1002/psp4.12611
John, J., Loo, A., Mazur, S., and Walsh, T. J. (2019). Therapeutic drug monitoring of systemic antifungal agents: A pragmatic approach for adult and pediatric patients. Expert Opin. Drug Metab. Toxicol. 15 (11), 881–895. doi:10.1080/17425255.2019.1671971
Krishna, G., Moton, A., Ma, L., Medlock, M. M., and Mcleod, J. (2009). Pharmacokinetics and absorption of posaconazole oral suspension under various gastric conditions in healthy volunteers. Antimicrob. Agents Chemother. 53 (3), 958–966. doi:10.1128/AAC.01034-08
Lai, T., Alffenaar, J. W., Kesson, A., Bandodkar, S., and Roberts, J. A. (2020). Evaluation of target attainment of oral posaconazole suspension in immunocompromised children. J. Antimicrob. Chemother. 75 (3), 726–729. doi:10.1093/jac/dkz481
Li, Y. Q., Chen, K. F., Ding, J. J., Tan, H. Y., Yang, N., Wu, C. F., et al. (2021). External evaluation of published population pharmacokinetic models of polymyxin B. Eur. J. Clin. Pharmacol. 77 (12), 1909–1917. doi:10.1007/s00228-021-03193-y
Livingston, E. H., and Lee, S. (2001). Body surface area prediction in normal-weight and obese patients. Am. J. Physiol. Endocrinol. Metab. 281 (3), E586–E591. doi:10.1152/ajpendo.2001.281.3.E586
Oh, J., Kang, C. I., Kim, S. H., Huh, K., Cho, S. Y., Jung, C. W., et al. (2020). Antifungal prophylaxis with posaconazole tablet and oral suspension in patients with haematologic malignancy: Therapeutic drug monitoring, efficacy and risk factors for the suboptimal level. Mycoses 63 (1), 89–94. doi:10.1111/myc.13020
Oh, S. M., Byun, J. M., Chang, E., Kang, C. K., Shin, D. Y., Kim, I., et al. (2021). Incidence of invasive fungal infection in acute lymphoblastic and acute myelogenous leukemia in the era of antimold prophylaxis. Sci. Rep. 11 (1), 22160. doi:10.1038/s41598-021-01716-2
Panagopoulou, P., and Roilides, E. (2022). Evaluating posaconazole, its pharmacology, efficacy and safety for the prophylaxis and treatment of fungal infections. Expert Opin. Pharmacother. 23 (2), 175–199. doi:10.1080/14656566.2021.1996562
Peña-Lorenzo, D., Rebollo, N., Sánchez-Hernández, J. G., Zarzuelo-Castañeda, A., Vázquez-López, L., Otera, M., et al. (2022). Population pharmacokinetics of a posaconazole tablet formulation in transplant adult allogeneic stem cell recipients. Eur. J. Pharm. Sci. 168, 106049. doi:10.1016/j.ejps.2021.106049
Petitcollin, A., Boglione-Kerrien, C., Tron, C., Nimubona, S., Lalanne, S., Bellisant, E., et al. (2017). Population pharmacokinetics of posaconazole tablets and Monte Carlo simulations to determine whether all patients should receive the same dose. Antimicrob. Agents Chemother. 61 (11), e01166–17. doi:10.1128/AAC.01166-17
Rae, J. M., Johnson, M. D., Lippman, M. E., and Flockhart, D. A. (2001). Rifampin is a selective, pleiotropic inducer of drug metabolism genes in human hepatocytes: Studies with cDNA and oligonucleotide expression arrays. J. Pharmacol. Exp. Ther. 299 (3), 849–857.
Ramspek, C. L., Jager, K. J., Dekker, F. W., Zoccali, C., and van Diepen, M. (2021). External validation of prognostic models: What, why, how, when and where? Clin. Kidney J. 14 (1), 49–58. doi:10.1093/ckj/sfaa188
Rhodin, M. M., Anderson, B. J., Peters, A. M., Coulthard, M. G., Wilkins, B., Grubb, A., et al. (2009). Human renal function maturation: A quantitative description using weight and postmenstrual age. Pediatr. Nephrol. 24 (1), 67–76. doi:10.1007/s00467-008-0997-5
Salem, F., Johnson, T. N., Hodgkinson, A., Ogungbenro, K., and Rostami-Hodjegan, A. (2021). Does "birth" as an event impact maturation trajectory of renal clearance via glomerular filtration? Reexamining data in preterm and full-term neonates by avoiding the creatinine bias. J. Clin. Pharmacol. 61 (2), 159–171. doi:10.1002/jcph.1725
Siontis, G. C., Tzoulaki, I., Castaldi, P. J., and Ioannidis, J. P. (2015). External validation of new risk prediction models is infrequent and reveals worse prognostic discrimination. J. Clin. Epidemiol. 68 (1), 25–34. doi:10.1016/j.jclinepi.2014.09.007
Störzinger, D., Borghorst, S., Hofer, S., Busch, C. J., Lichtenstern, C., Weigand, M., et al. (2012). Plasma concentrations of posaconazole administered via nasogastric tube in patients in a surgical intensive care unit. Antimicrob. Agents Chemother. 56 (8), 4468–4470. doi:10.1128/AAC.06167-11
Suh, H. J., Yoon, S. H., Yu, K. S., Cho, J. Y., Park, S. I., Koh, Y., et al. (2018). The genetic polymorphism UGT1A4*3 is associated with low posaconazole plasma concentrations in hematological malignancy patients receiving the oral suspension. Antimicrob. Agents Chemother. 62 (7), e02230–e02217. doi:10.1128/AAC.02230-17
Sustained-Release (2022). Orange book: Approved drug products with therapeutic equivalence evaluations. Available at: https://www.accessdata.fda.gov/scripts/cder/ob/results_product.cfm?Appl_Type=N&Appl_No=205053#30185 (accessed 20222 27, 2022).
Tang, L. A., Marini, B. L., Benitez, L., Nagel, J. L., Miceli, M., Berglund, C., et al. (2017). Risk factors for subtherapeutic levels of posaconazole tablet. J. Antimicrob. Chemother. 72 (10), 2902–2905. doi:10.1093/jac/dkx228
US FDA (2022). Noxafil instruction. Available at: https://www.accessdata.fda.gov/drugsatfda_docs/label/2022/022003Orig1s027lbl.pdf (accessed 212 2022, 2022).
Van Daele, R., Spriet, I., and Maertens, J. (2020). Posaconazole in prophylaxis and treatment of invasive fungal infections: A pharmacokinetic, pharmacodynamic and clinical evaluation. Expert Opin. Drug Metab. Toxicol. 16 (7), 539–550. doi:10.1080/17425255.2020.1764939
Vehreschild, J. J., Müller, C., Farowski, F., Vehreschild, M. J., Cornely, O. A., Kohl, V., et al. (2012). Factors influencing the pharmacokinetics of prophylactic posaconazole oral suspension in patients with acute myeloid leukemia or myelodysplastic syndrome. Eur. J. Clin. Pharmacol. 68 (6), 987–995. doi:10.1007/s00228-012-1212-y
Wang, J., Zhou, M., Xu, J. Y., Zhou, R. F., Chen, B., and Wan, Y. (2020). Comparison of antifungal prophylaxis drugs in patients with hematological disease or undergoing hematopoietic stem cell transplantation: A systematic review and network meta-analysis. JAMA Netw. Open 3 (10), e2017652. doi:10.1001/jamanetworkopen.2020.17652
Wasmann, R. E., Smit, C., van Donselaar, M. H., van Dongen, E., Wiezer, R., Burger, D. M., et al. (2020). Implications for IV posaconazole dosing in the era of obesity. J. Antimicrob. Chemother. 75 (4), 1006–1013. doi:10.1093/jac/dkz546
Wiederhold, N. P. (2016). Pharmacokinetics and safety of posaconazole delayed-release tablets for invasive fungal infections. Clin. Pharmacol. 8, 1–8. doi:10.2147/CPAA.S60933
Wong, T. Y., Loo, Y. S., Veettil, S. K., Wong, P. S., Divya, G., Menon, R., et al. (2020). Efficacy and safety of posaconazole for the prevention of invasive fungal infections in immunocompromised patients: A systematic review with meta-analysis and trial sequential analysis. Sci. Rep. 10 (1), 14575. doi:10.1038/s41598-020-71571-0
Wu, D., Mi, Y., Weng, J., Zhuang, J., Ke, X., Wang, C., et al. (2022). Phase 1b/3 pharmacokinetics and safety study of intravenous posaconazole in adult asian participants at high risk for invasive fungal infections. Adv. Ther. 39 (4), 1697–1710. doi:10.1007/s12325-021-02012-1
Zeng, H., Wu, Z., Yu, B., Wang, B., Wu, C., Wu, J., et al. (2021). Network meta-analysis of triazole, polyene, and echinocandin antifungal agents in invasive fungal infection prophylaxis in patients with hematological malignancies. BMC Cancer 21 (1), 404. doi:10.1186/s12885-021-07973-8
Keywords: posaconazole, population pharmacokinetics, external evaluation, predictive performance, therapeutic drug monitoring
Citation: Huang S, Ding Q, Yang N, Sun Z, Cheng Q, Liu W, Li Y, Chen X, Wu C and Pei Q (2022) External evaluation of published population pharmacokinetic models of posaconazole. Front. Pharmacol. 13:1005348. doi: 10.3389/fphar.2022.1005348
Received: 28 July 2022; Accepted: 13 September 2022;
Published: 30 September 2022.
Edited by:
Ahmed F. El-Yazbi, Alexandria University, EgyptReviewed by:
Nikunjkumar Patel, Certara, United StatesGregory Wiedman, Seton Hall University, United States
Tjip S. Van Der Werf, University of Groningen, Netherlands
Copyright © 2022 Huang, Ding, Yang, Sun, Cheng, Liu, Li, Chen, Wu and Pei. This is an open-access article distributed under the terms of the Creative Commons Attribution License (CC BY). The use, distribution or reproduction in other forums is permitted, provided the original author(s) and the copyright owner(s) are credited and that the original publication in this journal is cited, in accordance with accepted academic practice. No use, distribution or reproduction is permitted which does not comply with these terms.
*Correspondence: Cuifang Wu, d3VfY3VpX2ZhbmdAMTI2LmNvbQ==; Qi Pei, cGVpcWkxMDI4QDEyNi5jb20=
†These authors have contributed equally to this work and share first authorship