- 1Department of Pharmacy, The First Affiliated Hospital of Guangxi Medical University, Nanning, China
- 2Department of Organ Transplant, The First Affiliated Hospital of Guangxi Medical University, Nanning, China
Objective: To investigate the factors influencing the pharmacokinetics of mycophenolate mofetil (MMF) in pediatric patients after liver transplantation, and to establish a population pharmacokinetics model, which can provide a reference for clinical dosage adjustment.
Methods: A prospective study in a single center was performed on pediatric patients who were administrated with mycophenolate mofetil dispersible tablets (MMFdt) for at least 4 days after liver transplantation continuously. Blood samples were collected in ethylene diamine tetraacetic acid anticoagulant tubes before dosing and 0.5, 1, 2, 4, 8, and 12 h after the morning intake of MMFdt. The concentrations of mycophenolic acid (MPA) in plasma were assayed with a validated reverse-phase high-performance liquid chromatography method. UGT1A8 518C > G, UGT1A9 -275T > A, UGT1A9 -2152C > T, UGT2B7 211G > T, SLC O 1B1 521T > C polymorphism were determined by Sanger sequencing. Nonlinear mixed effects modeling was used to establish the population pharmacokinetics (PPK) model. The predictability and stability of the model were internally evaluated by the goodness of fit plots, visual prediction check, normalized prediction errors, and bootstraps.
Results: A two-compartment model with first-order absorption and first-order elimination was established with 115 MPA concentrations from 20 pediatric patients. The final model were: CL/F (L/h) = 14.8×(WT/7.5)0.75×(DOSE/11.16)0.452×е0.06, Ka (h−1) = 2.02×(WT/7.5)−0.25, Vc/F (L) = 6.01×(WT/7.5), Vp/F (L) = 269 (fixed), Q/F (L/h) = 15.4×(WT/7.5)0.75×е1.39. Where CL/F was the apparent clearance rate, Ka was the absorption rate constant, Vc/F was the apparent distribution volume of the central compartment, Vp/F was the apparent distribution volume of the peripheral compartment, Q/F was the atrioventricular clearance rate, WT was the body weight of the subject, and DOSE was the MMFdt administered dose. The model indicated there was large inter-individual variability in CL/F and Q/F after multiple dosing of MMFdt. Internal evaluation results showed that the final model had good stability and prediction performance.
Conclusion: A stable and predictive population pharmacokinetic model of MMFdt in pediatric patients after the early stage of liver transplantation was established. The pediatric patient’s weight and the dose of MMFdt can be a reference to adjust the MMFdt dose.
Introduction
With the rapid improvement of medical technology, liver transplantation has become an effective therapy for some end-stage liver diseases. Since 2001, Mycophenolate Mofetil (MMF) has been approved by the U.S. Food and Drug Administration for preventing acute rejection after liver transplantation in adults, and the combination of MMF, tacrolimus and glucocorticoid drugs has become the preferred immunosuppressive regimen in most medical centers at present (Perito et al., 2019; Hart et al., 2020). Clinical randomized double-blind trials have confirmed that MMF combined with Calcineurin inhibitors (CNIs) can significantly reduce the incidence of acute rejection of liver and kidney transplantation, while combined with low-dose MMF can reduce the side effects of CNIs and enhance the immunosuppressive effect, thus improving the long-term survival rate after transplantation (Miller et al., 2000; Squifflet et al., 2001; Takada et al., 2013).
As an active metabolite of MMF, Mycophenolic acid (MPA) is affected by plasma albumin (ALB) binding level, gene polymorphism connected to drug metabolism and transport, enterohepatic circulation, body weight, etc., making MPA has complex and variable pharmacokinetics (PK) characteristics in vivo (Jing, 2014; Bergan et al., 2021). A growing body of research indicates that MPA PK has significant inter-individual and intra-individual differences. (Shaw et al., 2003; Lobritto et al., 2007; Rong et al., 2021). Under the same dose, there was a tenfold difference in plasma concentrations between individuals, and the difference in area under the concentration-time curve (AUC) can attain fivefold (Staatz and Tett, 2007).
Currently, the recommended effective treatment window for MPA-AUC0-12 is 30–60 mg h/L in the liver transplant population. It has been reported that when the MPA-AUC0-12 in plasma is lower than 30 mg h/L, it is associated with acute rejection; while when the MPA-AUC0-12 is higher than 60 mg h/L, it can increase the incidence of adverse reactions such as diarrhea and myelosuppression (Bergan et al., 2021). Individualized MMF doses may help reduce potential toxic effects and improve clinical outcomes in pediatric liver transplant patients.
Up to now, many studies have explored the population pharmacokinetics (PPK) characteristics of MMF in kidney transplants, liver transplants, heart transplants, and other groups. However, studies based on Asian pediatric liver transplant patients have not been reported. Especially, the use of mycophenolate mofetil dispersible tablet (MMFdt) related PPK research has not been reported. Given the narrow therapeutic window of MPA, it is particularly important for the treatment of the disease and the growth and development of children to get safe and effective plasma concentrations. Therefore, it is urgent to carry out research on PPK in pediatric liver transplant patients.
The purpose of this study was to establish the MMFdt PPK model in pediatric liver transplant patients, which could explore various potential factors on MMFdt PPK, to provide a reference for the individualized medication of MMFdt.
Methods
Study design and patients
Pediatric liver transplant patients with deceased or living donor between 2020 and 2021 at the First Affiliated Hospital of Guangxi Medical University were enrolled in this prospective study. The inclusion criteria for this study were: 1) age <18 years; 2) liver transplantation for the first time; 3) MMFdt, tacrolimus, and methylprednisolone as triple immunosuppressive regimen; 4) treatment with MMFdt for more than 4 days. The exclusion criteria were: 1) severe gastrointestinal disease or diarrhea; 2) received combined organ transplantation. Due to the unconfirmed safety and efficacy in the pediatric population and the absence of clear dosing recommendations for pediatric liver transplant patients, clinicians based on clinical experience, postoperatively initially administered the drug through oral or nasal feeding, with an initial dose of 10–15 mg/kg, q12h, and adjusted the dose according to the actual situation. All protocols were approved by the independent Clinical Research Ethics Committee of The First Affiliated Hospital of Guangxi Medical University, and all participants provided written informed consent before enrolment.
The mean half-life (T1/2) of MPA was about 17h, so we decided to start sampling after it reaching steady state (4th day). Blood samples were routinely collected on ethylenediamine tetraacetic acid (EDTA) by central venous catheterization before and 0.5, 1, 2, 4, 8, and 12 h after administration. The samples were centrifuged at 3,000 rpm for 3 min immediately after sampling, then the plasma was frozen at -80°C until the analysis.
MPA assay
Plasma concentrations of MPA were determined by automatic two-dimensional liquid chromatography (2D-HPLC, Demeter Instrument Co. Ltd., Hunan, China). Chromatographic conditions: the first-dimensional column: Aston SC2 (3.5 mm × 25 mm, 5 μm, ANAX, China), mobile phase: 10 mmol/L acetic acid solution-acetonitrile-isopropanol, flow rate: 0.4 ml/min; Intermediate column: Aston SBX4 (3.0 mm × 10 mm, 5 μm, ANAX, China), mobile phase: purified water; The second-dimensional column: Aston SCB (4.6 mm × 125 mm, 5 μm, ANAX, China), mobile phase: methanol, flow rate: 1.2 ml/min. Column temperature: 45°C. The detection wavelength was 304 nm.
Before the determination, 600 μL deproteinizing agent ACP-1B was added into a 1.5 ml EP tube, and then added 200 μL plasma sample exactly. After 1 min of vortex oscillation, high-speed centrifugation was performed for 8 min (14,500 r/min), 650 μL of supernatant was added to 65 μL of ACG-1 protectant into the injection flask, and the sample was shaken and mixed to be measured.
The sample size was 200 μL. The calibration range was 0.3–30.0 mg/L, The lower limit of quantitation (LLOQ) was 0.3 mg/L. The relative standard deviation (RSD) of intra- and inter-day precision is less than 5%. The recovery rate is more than 95%. The stability of the samples stored at room temperature for 24 h and -20°C for 30 days was investigated. The results showed that the stability of MPA in plasma was satisfied and the RSD was less than 5%.
Processing of data below the quantization limit
For the measured values below the quantization limit (BQL), the M3 method performs best in theory, which maximizes the likelihood for all the data treating BQL observation as censored. However, it can significantly increase the running time and then lead to operation process interruption and estimation failure. Therefore, the M5 method was preferred in this study to process BQL data, that is, to replace BQL with 1/2 of the LLOQ. In addition, double-panel visual predictive check plots were used to evaluate the above data processing methods (Bergstrand and Karlsson, 2009).
DNA extraction and SNP genotyping
Nucleic acids were extracted with nucleic acid extraction and purification reagent (20190719) from Baiao Technology Co. Ltd. (Shanghai, China) and amplified by ABI 9700-PCR (Applied Biosystems). The assessment of the polymorphisms UGT1A8 518C > G, UGT1A9 -275T > A, UGT1A9 -2152C > T, UGT2B7 211G > T and SLC O 1B1 521T > C was performed by Sanger sequencing. The primers for the loci mentioned above were synthesized by Tsingke Biotechnology Co., Ltd. (Nanning), which refers to the data provided in the literature (Yu et al., 2017; Wang et al., 2021).
Population pharmacokinetic analysis
PPK analysis was performed using nonlinear mixed effects modeling NONMEM (version 7.4.3 ICON Development Solution, Ellicott City, MD, USA) approach, facilitated by Perl-speaks-NONMEM (PSN) and Wings for NONMEM (Nick Holford, University of Auckland, New Zealand). The first-order conditional estimation with interaction method (FOCE-I) was selected to estimate the parameters and variability throughout the model-building procedure. ADVAN2 TRANS2 and ADVAN4 TRANS4 were compared in the base model determination.
Model selection criteria were: 1) the value of the objective function (OFV) was minimized; 2) the relative standard error percentage (RES%) of fixed effect parameter and random effect parameter were less than 30% and 50% respectively; 3) the condition number was less than 1,000; 4) the goodness-of-fit (GOF) was improved. Covariates were tested in a univariate fashion and included in the model if the OFV decreased by > 3.84 (p < 0.05, χ2 distribution, df = 1). After the inclusion of all significant covariates, the significance of the covariates was tested by removing each covariate, and the final model retained the covariates that increased the OFV by > 6.64 (p < 0.01, χ2 distribution, df = 1) or >9.21 (p < 0.01, χ2 distribution, df = 2).
Statistical analysis
Analysis results were expressed as the median and interquartile range (IQR). Hardy Weinberg equilibrium was applied to assess the deviation of allele and genotype frequencies. All statistical analyses were performed with IBM SPSS Statistics Version 22.0 (SPSS Inc., Chicago, IL, United States).
Model validation
Model internal validation was conducted by GOF plots, visual prediction check (VPC), normalized prediction distribution errors (NPDE), and bootstrap to evaluate the stability and prediction performance.
Results
Patient demographics
Altogether 20 pediatric liver transplant patients with 122 samples were included. The main demographic characteristics of the study population were listed in Table 1, as results shown in the patient’s biochemistry were within the normal range except for liver function.
Of the 122 samples, 7 concentration values below the detection limit were directly removed as missing data. The scatter plots of the remaining 115 concentration values and the time after the last dose were shown in Figure 1, from which it could be seen that the first peak of MPA blood concentrations were mainly concentrated in 0.5–2 h, and no obvious secondary peak of MPA blood concentrations caused by enterohepatic circulation (EHC) was observed. Among 115 concentration values, 21 concentration values were lower than LLOQ, accounting for 18.3%. Hence, the M5 method was used in this study to replace all 21 BQL data with 0.15 mg/L.
Figure 2 showed the frequency distribution of specific genotypes in the 20 pediatric liver transplant patients. Genotype and allele frequencies were not significantly different than expected, which represented the population was in Hardy-Weinberg equilibrium.
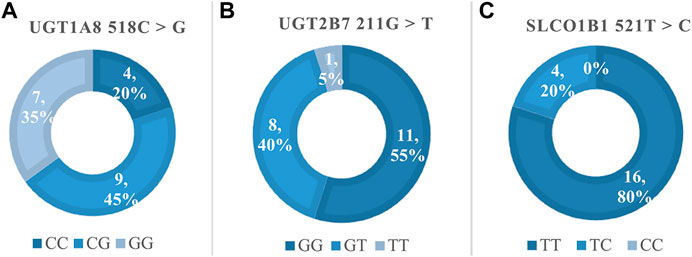
FIGURE 2. The genotype frequencies of MPA related polymorphisms. (A) Frequency of UGT1A8 518C > G, Hardy-Weinberg p > 0.05 (χ2 = 0.13); (B) Frequency of UGT2B7 211G > T, Hardy-Weinberg p > 0.05 (χ2 = 0.09); (C) Frequency of SLCO1B1 521T > C, Hardy-Weinberg p > 0.05 (χ2 = 0.25).
Population pharmacokinetic model
At last, a two-compartment pharmacokinetic model with first-order absorption was selected to describe the data. In the random effects model, both the inter-individual variation (IIV) and residual variation (RV) were represented as exponents for the best-fitting effect.
The OFV value of the model including the allometric scaling model decreased by 10.061. The dose of MMFdt was retained in the model as a covariate significantly affecting CL/F. The screening process of the covariate model were shown in Table 2, and the final model formula were as follows:
Final parameter estimation, interindividual variability with standard error estimation, and bootstrap statistics of the final PPK model were represented in Table 3.
Model evaluation
The GOF plots of the final model was shown in Figure 3. The trend line of individual prediction (IPRED), population prediction (PRED), and dependent variable (DV) of the final model was close to the reference line, which meant the deviation between the predicted value and the measured value was small, and the prediction performance of the model was improved.
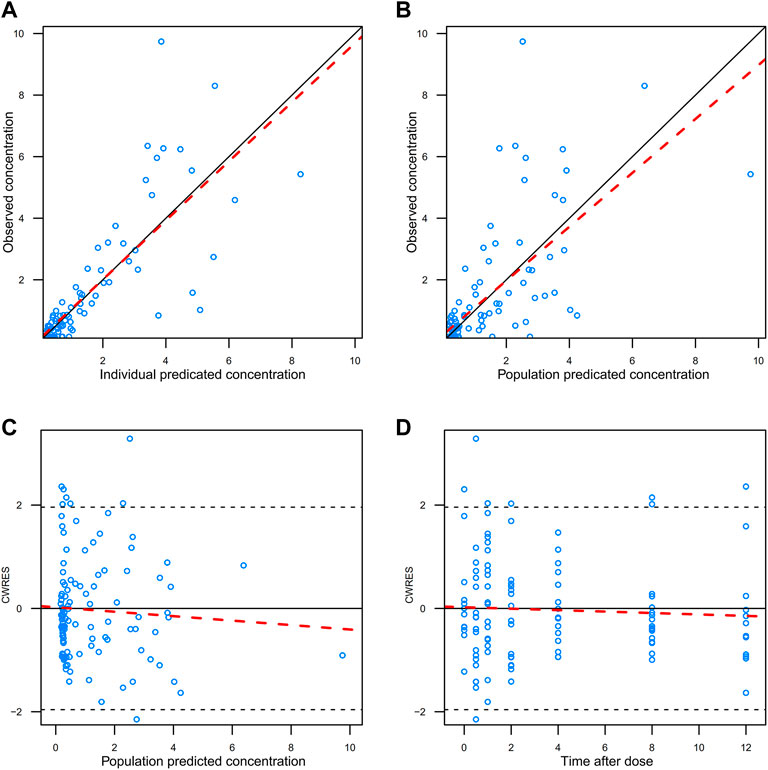
FIGURE 3. GOFs diagnosis diagram of the final model. (A) observed value vs. individual predicted value; (B) observed value vs. predicted population value; (C) conditional weight residuals vs. population predicted values; (D) conditional weighted residuals vs. time after dose. The red dotted line represents trendline, while the solid black line represents reference line.
The results of VPC verification of the final model were shown in Figure 4, which represented that the model adequately described the overall trend and variability in the observed data. As shown in the figure, the majority of MPA concentration values were within the 90% prediction interval, indicating that the model’s predictive performance was acceptable.
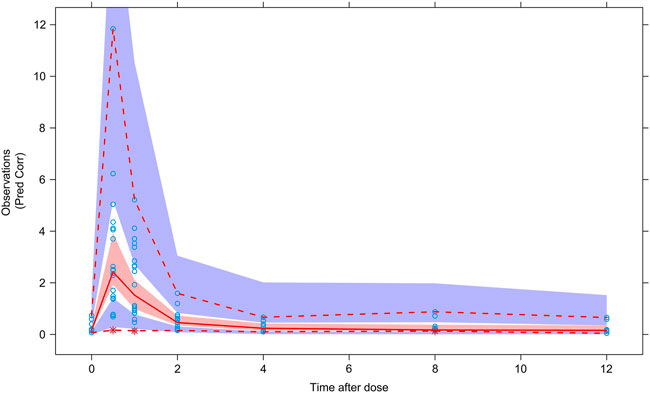
FIGURE 4. Visual predictive check of the final model. Circles represent observations. Red solid line stands for the median of simulated concentrations, and red imaginary lines stand for the 90% prediction interval (PI) (5%, 95%) of the predictive MPA concentrations. Shaded areas represent the 95% confidence interval (CI) for each line.
The NPDE diagnostic diagram indicates that the final model had an approximately normal distribution trend (Figure 5). The predicted concentrations of NPDE were randomly distributed near the reference line, and most of them were in the acceptable range (±2), and the Global test p-value was 0.422 (>0.05), which indicated that the prediction performance of the model was satisfied.
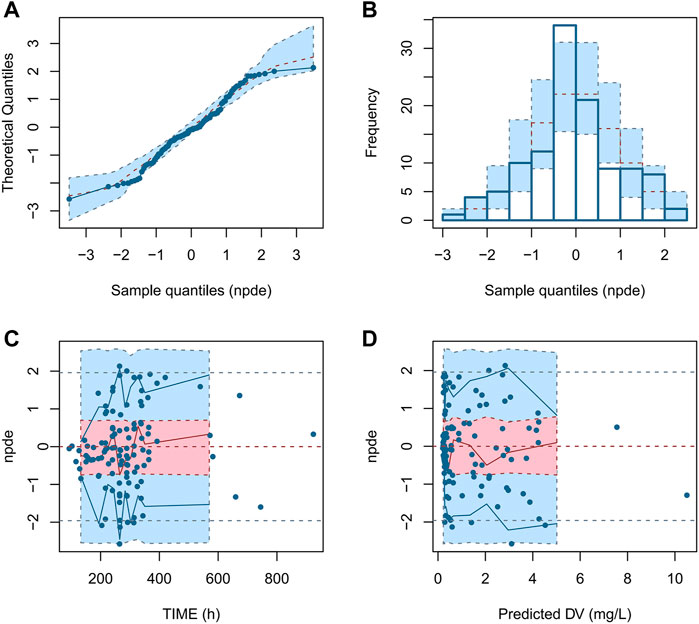
FIGURE 5. NPDE diagnostic diagram of the final model. (A) Q - Q plot of NPDE vs. normalized distribution; (B) Distribution diagram of predictive distribution error; (C) NPDE vs. TIME; (D) NPDE vs. PRED.
The robustness rate of the final model was 98.1%. The detailed results of bootstrap were set out in Table 3, which indicated the model was reliable with good accuracy and stability.
The results of BQL data processing with the M5 method was showed in Figure 6, we divided the blood drug concentration data into two parts: data higher than LLOQ and data lower than LLOQ. It can be seen that the measured BQL data were all located inside the shadow, which showed that the M5 method was suitable for processing BQL data.
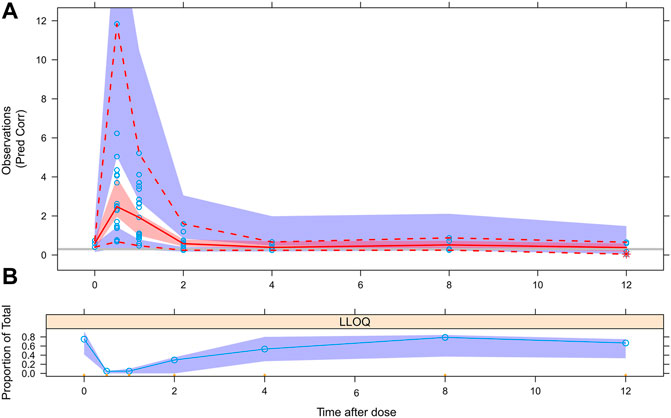
FIGURE 6. VPC evaluation of BQL data with M5 method. (A) Circles represent observations. Red solid line stands for the median of simulated concentrations, and red imaginary lines stand for the 90% PI (5%, 95%) of the predictive MPA concentrations. Shaded areas represent the 95% CI for each line. The horizontal gray line represents the LLOQ. (B) Circles represent BQL observations, shaded areas represent the 95% CI for predictive BQL concentrations.
Discussion
In this study, we prospectively gathered data and sought to determine the influence of PPK in pediatric liver transplant patients, establishing a two-compartment model of first-order absorption with body weight and dose as covariates by the NONMEM method. The internal evaluation indicated that the final model had satisfactory predictability, which could provide a reference for MMFdt clinical application.
To describe the in vivo process of MPA as accurately as possible, previous researchers mostly used the two-compartment model with a complex absorption or distribution process of MPA in different pediatric groups, such as renal transplantation, hematopoietic stem cell transplantation, and idiopathic nephrotic syndrome. The range of population typical values of CL/F, Ka, Vc/F, Vp/F, and Q/F were12.7–25.3 L/h, 0.39–5.21 h−1, 35–411 L, 4.75–64.7 L, and 3.74–113 L/h (Zhao et al., 2010; Sherwin et al., 2012; Dong et al., 2014) respectively. In our study, the typical values of CL/F, Ka, Vc/F, Vp/F, and Q/F in the final model are 14.8 L/h、2.02 h−1、6.01 L、269 L, and 15.4 L/h, respectively, which were within the range of population typical values of previous research models. Furthermore, prior studies had noted that body weight, age, and combined use of cyclosporine or tacrolimus were mostly included as covariables in CL/F and Vc/F, among which Q/F showed great variability in estimation (BOV% 26.6%–207.83%), and no covariables could be found to explain the variation (Rong et al., 2021), which was consistent with the results observed in our study. Vp was fixed to 269 L, as it gave the optimal parameter estimation, and the IIV and RV decreased significantly. According to the Vp value of transplant patients reported in previous literature in the range of 137 L–496 L (Rong et al., 2021), the result of our study was reasonable.
In 2012, Barau et al. studied MMF PK for the first time in pediatric liver transplant patients and established the MMF PK model by traditional pharmacodynamic method with the estimated values of CL/F, Vc/F, and Ka being 12.7 L/h、64.7 L and 3.9 h−1, respectively. This study indicated that Vc/F decreased with the increase of time after transplantation. The authors believed that the free fraction of MPA (f-MPA) increased due to the lower ALB and increased bilirubin level after liver transplantation, increasing Vc/F (Barau et al., 2012). Nevertheless, no significant effect of time after transplantation on MMF PK parameters was found in other pediatric populations. In comparison, the estimated value of Vc/F was significantly different from the results of our study, which may be caused by the weight difference between the two populations. It had been reported that Vc increased exponentially with increasing body weight in adult solid-organ transplant patients (Funaki, 1999), and the median body weight in our study was 7.5 kg while the median body weight reported by Barau et al. was 23.8 kg, which may explain the difference of Vc/F estimates between their and our study.
When shrinkage is higher than 20–30%, diagnostics based on Bayes estimates (EBEs) lack informativeness and may be misleading (Savic and Karlsson, 2009). In our study, the shrinkage values of interindividual variation in CL/F, Q/F, and RV of the final model were 19.8%, 9.8%, and 8.2%, respectively, which were all less than 20%. The diagnostics were relatively reliable in model building and evaluation.
We tried to introduce body weight into the PPK modeling process through the allometric scaling model, and the results showed that the allometric scaling model could significantly improve the fitting effect of the model. This theory-based allometric scaling uses body weight as a power model with an exponent of 0.75 for functional PK parameters (e.g., clearance), and it is considered a proper biologically scaling method to account for different body sizes (Holford et al., 2013).
According to previous studies, the bioavailability of MMF was not constant, and it significantly decreased with the increase in MMF dose, showing nonlinear PK characteristics (Dong et al., 2014; Catic-Dordevic et al., 2021). This may explain the phenomenon that CL/F of MPA increased with the increase of dose in this study. With the dose increased, the absorption of MPA in the intestine reached saturation, while the unabsorbed MPA was directly excreted. Another possible explanation for this was that when the amount of mycophenolic acid glucoside acid (MPAG) reached saturation in the enterohepatic circulation, the use of high-dose MMF would cause more MPAG to be directly excreted through the kidney, and the generation of MPA through the enterohepatic circulation would reduce, leading to MPA exposure reduction (de Winter et al., 2011). It had been reported that due to better absorption of MPA at lower pH, the combined use of proton pump inhibitors (PPIs) could also result in a decrease in the bioavailability of MPA (Bergan et al., 2021). Miura et al. found that MPA AUC was lower when MMF was combined with 30 mg lansoprazole compared to 10 mg rabeprazole or no PPIs (Miura et al., 2008). In addition to interacting with MPA absorption, PPIs can also lead to reductions in MPA, peak concentration, and AUC by inhibiting ABCB1 mediated transport (Pauli-Magnus et al., 2001; Wedemeyer and Blume, 2014). As regards the effect of dose on bioavailability, it could also be explained by the fact that clinicians may administer a higher dose in consideration of the possibility of higher clearance in some pediatric patients. Overall, data on the bioavailability of MPA are scarce in current studies, and no covariate has been found to explain the observed variation. It is worthy of note that modeling with further consideration of physiological factors may be needed to confirm this hypothesis in pediatric populations. Therefore, the possible impact of dose on the bioavailability of MPA should be considered in clinical therapeutic drug monitoring.
Some studies had shown that the decrease of ALB level in the body and the reduction of its binding to MPA led to the increase of f-MPA concentration, which generated an increase in MPA clearance (Weber et al., 2008; Yoshimura et al., 2018). Reviewing the published pediatric population studies, only one study based on the cohort of children with idiopathic nephrotic syndrome included ALB in the final model (Zhao et al., 2010). However, no effect of ALB on MPA clearance was observed in our study, which might be interpreted as the normal level of ALB and renal function in the current study patients, failing to reach the threshold that can cause a significant reduction in MPA exposure (Kim et al., 2012).
Although polymorphisms of UGT1A8, UGT1A9, UGT2B7, and SLC O 1B1 genes were determined, which were considered to be important effect on the metabolism and transport process of MPA (Kuypers et al., 2005; Jiang and Hu, 2021), none had been verified in pediatric liver transplant patients. Unfortunately, no significant effect of these genotypes on MPA PK were observed in our study. At present, the effect of gene polymorphism on MPA PK is rarely observed in children, which may be because these liver drug enzyme activity of children is significantly lower than that of adults. According to published studies, the abundance of UGTs such as UGT1A9 and UGT2B7 reaches 50% of adult levels between 2.6 and 10.3 years of age, while the children in our study were all younger than 10 years old, and 75% of them (n = 15) were younger than 2 years old. In summary, no significant gene polymorphisms that affected MPA PK were observed in this study, perhaps due to the small sample size, short study duration (<30 d), or the young age of the study population with low metabolic and transporter activity (Miura et al., 2007; Badee et al., 2019).
There were several limitations for the current study. This study was a single-center study with a limited number of participants and only performed model internal evaluation, which should gather further data to expand the sample for a more rigorous external evaluation.
Yet, it is difficult to carry out population pharmacokinetic studies in pediatric liver transplant patients. Thus, the conclusions of this study still require to be further verification. We will continue to gather more patient data to improve the model and explore the PPK characteristics of MPA in pediatric liver transplant patients in China.
Conclusion
In summary, our study established a population pharmacokinetic model of MMFdt in pediatric patients after early liver transplantation. Based on this PPK analysis, we identified body weight and MMFdt dose as significant covariates for MPA clearance. The model’s internal evaluation methods showed the final model had good stability, reliability, and predictive performance, which might provide a reference for the individualized medication of MMFdt.
Data availability statement
The original contributions presented in the study are included in the article/Supplementary Material, further inquiries can be directed to the corresponding authors.
Ethics statement
The studies involving human participants were reviewed and approved by the Ethics Committee of the First Affiliated Hospital of Guangxi Medical University. Written informed consent to participate in this study was provided by the participants' legal guardian/next of kin.
Author contributions
YiW, TL, CD, and YC participated in the research design. YiW, DW, YC, JQ, YuW, RC, SZ, CL, LN, TW, and YX collected the information on pediatric patients. YiW, SZ, and DW analyzed and interpreted the data. YiW built the model and evaluated it. DW drafted the manuscript. YiW, DW, and TL reviewed and edited the manuscript. All authors have reviewed and approved the published version of the manuscript.
Acknowledgments
We would like to acknowledge and thank all the medical staff in the Department of Pharmacy and Organ Transplant Department of the First Affiliated Hospital of Guangxi Medical University for their kind assistance and support during the study. In addition, we are most grateful for all pediatric patients and their families participating in the research.
Conflict of interest
The authors declare that the research was conducted in the absence of any commercial or financial relationships that could be construed as a potential conflict of interest.
Publisher’s note
All claims expressed in this article are solely those of the authors and do not necessarily represent those of their affiliated organizations, or those of the publisher, the editors and the reviewers. Any product that may be evaluated in this article, or claim that may be made by its manufacturer, is not guaranteed or endorsed by the publisher.
References
Badee, J., Fowler, S., de Wildt, S. N., Collier, A. C., Schmidt, S., and Parrott, N. (2019). The ontogeny of UDP-glucuronosyltransferase enzymes, recommendations for future profiling studies and application through physiologically based pharmacokinetic modelling. Clin. Pharmacokinet. 58 (2), 189–211. doi:10.1007/s40262-018-0681-2
Barau, C., Furlan, V., Debray, D., Taburet, A. M., and Barrail-Tran, A. (2012). Population pharmacokinetics of mycophenolic acid and dose optimization with limited sampling strategy in liver transplant children. Br. J. Clin. Pharmacol. 74 (3), 515–524. doi:10.1111/j.1365-2125.2012.04213.x
Bergan, S., Brunet, M., Hesselink, D. A., Johnson-Davis, K. L., Kunicki, P. K., Lemaitre, F., et al. (2021). Personalized therapy for mycophenolate: Consensus report by the international association of therapeutic drug monitoring and clinical toxicology. Ther. Drug Monit. 43 (2), 150–200. doi:10.1097/FTD.0000000000000871
Bergstrand, M., and Karlsson, M. O. (2009). Handling data below the limit of quantification in mixed effect models. AAPS J. 11 (2), 371–380. doi:10.1208/s12248-009-9112-5
Catic-Dordevic, A., Pavlovic, I., Spasic, A., Stefanovic, N., Pavlovic, D., Damnjanovic, I., et al. (2021). Assessment of pharmacokinetic mycophenolic acid clearance models using Monte Carlo numerical analysis. Xenobiotica. 51 (4), 387–393. doi:10.1080/00498254.2020.1871532
de Winter, B. C., Mathot, R. A., Sombogaard, F., Vulto, A. G., and van Gelder, T. (2011). Nonlinear relationship between mycophenolate mofetil dose and mycophenolic acid exposure: Implications for therapeutic drug monitoring. Clin. J. Am. Soc. Nephrol. 6 (3), 656–663. doi:10.2215/CJN.05440610
Dong, M., Fukuda, T., Cox, S., de Vries, M. T., Hooper, D. K., Goebel, J., et al. (2014). Population pharmacokinetic-pharmacodynamic modelling of mycophenolic acid in paediatric renal transplant recipients in the early post-transplant period. Br. J. Clin. Pharmacol. 78 (5), 1102–1112. doi:10.1111/bcp.12426
Funaki, T. (1999). Enterohepatic circulation model for population pharmacokinetic analysis. J. Pharm. Pharmacol. 51 (10), 1143–1148. doi:10.1211/0022357991776831
Hart, A., Smith, J. M., Skeans, M. A., Gustafson, S. K., Wilk, A. R., Castro, S., et al. (2020). OPTN/SRTR 2018 annual data report: Kidney. Am. J. Transpl. 20 (1), 20–130. doi:10.1111/ajt.15672
Holford, N., Heo, Y. A., and Anderson, B. (2013). A pharmacokinetic standard for babies and adults. J. Pharm. Sci. 102 (9), 2941–2952. doi:10.1002/jps.23574
Jiang, Z., and Hu, N. (2021). Effect of UGT polymorphisms on pharmacokinetics and adverse reactions of mycophenolic acid in kidney transplant patients. Pharmacogenomics 22 (15), 1019–1040. doi:10.2217/pgs-2021-0087
Jing, L. (2014). Ethnic difference in Mycophenolate mofetil pharmacokinetics between Chinese and Caucasian 344 healthy subjects. Master's thesis. (Shanghai, China: Fudan university).
Kim, H., Long-Boyle, J., Rydholm, N., Orchard, P. J., Tolar, J., Smith, A. R., et al. (2012). Population pharmacokinetics of unbound mycophenolic acid in pediatric and young adult patients undergoing allogeneic hematopoietic cell transplantation. J. Clin. Pharmacol. 52 (11), 1665–1675. doi:10.1177/0091270011422814
Kuypers, D. R., Naesens, M., Vermeire, S., and Vanrenterghem, Y. (2005). The impact of uridine diphosphate-glucuronosyltransferase 1A9 (UGT1A9) gene promoter region single-nucleotide polymorphisms T-275A and C-2152T on early mycophenolic acid dose-interval exposure in de novo renal allograft recipients. Clin. Pharmacol. Ther. 78 (4), 351–361. doi:10.1016/j.clpt.2005.06.007
Lobritto, S. J., Rosenthal, P., Bouw, R., Leung, M., Snell, P., and Mamelok, R. D. (2007). Pharmacokinetics of mycophenolate mofetil in stable pediatric liver transplant recipients receiving mycophenolate mofetil and cyclosporine. Liver Transpl. 13 (11), 1570–1575. doi:10.1002/lt.21274
Miller, J., Mendez, R., Pirsch, J. D., and Jensik, S. C. (2000). Safety and efficacy of tacrolimus in combination with mycophenolate mofetil (MMF) in cadaveric renal transplant recipients. FK506/MMF Dose-Ranging Kidney Transplant Study Group. Transplantation 69 (5), 875–880. doi:10.1097/00007890-200003150-00035
Miura, M., Satoh, S., Inoue, K., Kagaya, H., Saito, M., Inoue, T., et al. (2007). Influence of SLCO1B1, 1B3, 2B1 and ABCC2 genetic polymorphisms on mycophenolic acid pharmacokinetics in Japanese renal transplant recipients. Eur. J. Clin. Pharmacol. 63 (12), 1161–1169. doi:10.1007/s00228-007-0380-7
Miura, M., Satoh, S., Inoue, K., Kagaya, H., Saito, M., Suzuki, T., et al. (2008). Influence of lansoprazole and rabeprazole on mycophenolic acid pharmacokinetics one year after renal transplantation. Ther. Drug Monit. 30 (1), 46–51. doi:10.1097/FTD.0b013e31816337b7
Pauli-Magnus, C., Rekersbrink, S., Klotz, U., and Fromm, M. F. (2001). Interaction of omeprazole, lansoprazole and pantoprazole with P-glycoprotein. Naunyn. Schmiedeb. Arch. Pharmacol. 364 (6), 551–557. doi:10.1007/s00210-001-0489-7
Perito, E. R., Martinez, M., Turmelle, Y. P., Mason, K., Spain, K. M., Bucuvalas, J. C., et al. (2019). Posttransplant biopsy risk for stable long-term pediatric liver transplant recipients: 451 percutaneous biopsies from two multicenter immunosuppression withdrawal trials. Am. J. Transpl. 19 (5), 1545–1551. doi:10.1111/ajt.15255
Rong, Y., Jun, H., and Kiang, T. K. L. (2021). Population pharmacokinetics of mycophenolic acid in paediatric patients. Br. J. Clin. Pharmacol. 87 (4), 1730–1757. doi:10.1111/bcp.14590
Savic, R. M., and Karlsson, M. O. (2009). Importance of shrinkage in empirical bayes estimates for diagnostics: Problems and solutions. AAPS J. 11 (3), 558–569. doi:10.1208/s12248-009-9133-0
Shaw, L. M., Korecka, M., Venkataramanan, R., Goldberg, L., Bloom, R., and Brayman, K. L. (2003). Mycophenolic acid pharmacodynamics and pharmacokinetics provide a basis for rational monitoring strategies. Am. J. Transpl. 3 (5), 534–542. doi:10.1034/j.1600-6143.2003.00079.x
Sherwin, C. M., Sagcal-Gironella, A. C., Fukuda, T., Brunner, H. I., and Vinks, A. A. (2012). Development of population PK model with enterohepatic circulation for mycophenolic acid in patients with childhood-onset systemic lupus erythematosus. Br. J. Clin. Pharmacol. 73 (5), 727–740. doi:10.1111/j.1365-2125.2011.04140.x
Squifflet, J. P., Backman, L., Claesson, K., Dietl, K. H., Ekberg, H., Forsythe, J. L., et al. (2001). Dose optimization of mycophenolate mofetil when administered with a low dose of tacrolimus in cadaveric renal transplant recipients. Transplantation 72 (1), 63–69. doi:10.1097/00007890-200107150-00014
Staatz, C. E., and Tett, S. E. (2007). Clinical pharmacokinetics and pharmacodynamics of mycophenolate in solid organ transplant recipientsClin. Pharmacokinet. 46 (1), 13–58. doi:10.2165/00003088-200746010-00002
Takada, Y., Kaido, T., Asonuma, K., Sakurai, H., Kubo, S., Kiuchi, T., et al. (2013). Randomized, multicenter trial comparing tacrolimus plus mycophenolate mofetil to tacrolimus plus steroids in hepatitis C virus-positive recipients of living donor liver transplantation. Liver Transpl. 19 (8), 896–906. doi:10.1002/lt.23679
Wang, Z., Xu, H., Xiang, T., Liu, D., Xu, F., Zhao, L., et al. (2021). An accessible insight into genetic findings for transplantation recipients with suspected genetic kidney disease. NPJ Genom. Med. 6 (1), 57. doi:10.1038/s41525-021-00219-3
Weber, L. T., Hoecker, B., Armstrong, V. W., Oellerich, M., and Tönshoff, B. (2008). Long-term pharmacokinetics of mycophenolic acid in pediatric renal transplant recipients over 3 years posttransplant. Ther. Drug Monit. 30 (5), 570–575. doi:10.1097/FTD.0b013e31818752d9
Wedemeyer, R. S., and Blume, H. (2014). Pharmacokinetic drug interaction profiles of proton pump inhibitors: An update. Drug Saf. 37 (4), 201–211. doi:10.1007/s40264-014-0144-0
Yoshimura, K., Yano, I., Yamamoto, T., Kawanishi, M., Isomoto, Y., Yonezawa, A., et al. (2018). Population pharmacokinetics and pharmacodynamics of mycophenolic acid using the prospective data in patients undergoing hematopoietic stem cell transplantation. Bone Marrow Transpl. 53 (1), 44–51. doi:10.1038/bmt.2017.213
Yu, Z. C., Zhou, P. J., Wang, X. H., Francoise, B., Xu, D., Zhang, W. X., et al. (2017). Population pharmacokinetics and Bayesian estimation of mycophenolic acid concentrations in Chinese adult renal transplant recipients. Acta Pharmacol. Sin. 38 (11), 1566–1579. doi:10.1038/aps.2017.115
Keywords: mycophenolate mofetil, population pharmacokinetics, liver transplantation, pediatric pharmacology, nonlinear mixed-effect modeling
Citation: Wei Y, Wu D, Chen Y, Dong C, Qi J, Wu Y, Cai R, Zhou S, Li C, Niu L, Wu T, Xiao Y and Liu T (2022) Population pharmacokinetics of mycophenolate mofetil in pediatric patients early after liver transplantation. Front. Pharmacol. 13:1002628. doi: 10.3389/fphar.2022.1002628
Received: 25 July 2022; Accepted: 30 September 2022;
Published: 13 October 2022.
Edited by:
Catherine M. T. Sherwin, Wright State University, United StatesReviewed by:
Joanna Sobiak, Poznan University of Medical Sciences, PolandXie-An Yu, Shenzhen Institute For Drug Control, China
Qin Wan Huang, Chengdu University of Traditional Chinese Medicine, China
Chao-Zhan Lin, Guangzhou University of Chinese Medicine, China
Copyright © 2022 Wei, Wu, Chen, Dong, Qi, Wu, Cai, Zhou, Li, Niu, Wu, Xiao and Liu. This is an open-access article distributed under the terms of the Creative Commons Attribution License (CC BY). The use, distribution or reproduction in other forums is permitted, provided the original author(s) and the copyright owner(s) are credited and that the original publication in this journal is cited, in accordance with accepted academic practice. No use, distribution or reproduction is permitted which does not comply with these terms.
*Correspondence: Taotao Liu, liutaotao@gxmu.edu.cn; Yiyu Chen, raincoat7571@qq.com
†These authors have contributed equally to this work and share first authorship